DOI:
10.1039/C7RA07873K
(Paper)
RSC Adv., 2017,
7, 44990-44996
High-throughput lipidomics enables discovery of the mode of action of huaxian capsule impacting the metabolism of sepsis†
Received
17th July 2017
, Accepted 3rd September 2017
First published on 20th September 2017
Abstract
Severe sepsis (SS) is a major cause of mortality and morbidity in the intensive care unit and requires rapid diagnosis and treatment. Lipids are key regulators of cellular function; hence, in this study, we hypothesized that lipid levels could be altered in patients suffering from SS. Lipidomics is the comprehensive analysis of molecular lipid species. In this study, we used an untargeted lipidomics approach coupled with multivariate data analysis and bioinformatics analysis to profile the metabolic changes of sepsis induced by cecal ligation and puncture (CLP) in rats and investigated the treatment effects of huaxian capsule (HXC), a herbal medicine with putative effects in SS treatment. Ultra-performance liquid chromatography/quadrupole time-of-flight mass spectrometry (UPLC-Q-TOF-MS) and pattern recognition methods were employed to evaluate the metabolic phenotypic changes. Differentially expressed lipids have been further analyzed using the ingenuity pathway analysis (IPA) software, a tool that discloses functional pathways associated with lipids of interest. As a result, ten lipids involved in multiple metabolic pathways, such as in glycerophospholipid metabolism, ether lipid metabolism, purine metabolism, and tryptophan metabolism representing characteristic phenotypes, were identified by pathway analysis. HXC administration can reverse lipid phenotype changes induced by CLP; this indicates that the effectiveness of HXC as an SS treatment depends on modulation of the lipid metabolic changes. Our study shows that lipidomics is a valuable method that can provide additional insight into the underlying mechanisms of HXC in the SS treatment.
1. Introduction
Severe sepsis (SS), with its high morbidity and mortality rate, is a major health problem in the world.1–3 It is necessary to have additional biomarkers for early diagnosis and prevention of SS. Traditional Chinese medicine has been used for the treatment of many diseases for thousands of years because of the global therapeutic effects of its combination.4,5 Huaxian capsule (HXC) has been widely used for the clinical treatment of SS for many years.6 However, due to the limited scientific data on the mechanisms of its action, the therapeutic effect of HXC has not been completely recognized. Therefore, aiming to add the therapeutic mechanisms of HXC in a model of SS, further study was conducted to better understand the underlying mechanisms of HXC in the treatment of SS.
Lipidomics can provide an unbiased field for the investigation of lipids and serve as a valuable approach in understanding the mechanisms and clinical diagnosis of the disease and its response to therapeutic interventions.7–10 It is an analytical approach for holistic investigation of living systems based on the lipid metabolic profiles.11,12 Moreover, lipidomics analysis can lead to better understanding of new therapeutic agents.13 Mass spectrometry and chromatography techniques have greatly promoted the developments and applications of lipidomics.14–17 Ultra-performance liquid chromatography coupled with quadrupole time-of-flight mass spectrometry (UPLC-QTOF/MS) is most suitable for untargeted lipidomics.18,19 Plasma is widely used in lipidomics mainly due to its availability.20
Clinically, HXC is the commonly used Chinese herbal formula for the treatment of SS.6 Although the clinical efficacy of HXC in the treatment of the SS has been demonstrated, the underlying biochemical mechanisms of its effect remain uncertain. Lipids are key regulators of cellular function, and lipid levels can be altered in patients suffering from SS. Herein, we reported a lipidomics study using UPLC-Q-TOF/MS-based shotgun lipidomics on the evaluation of the effects of HXC on the lipidomes of a mouse model of SS that was induced by cecal ligation and puncture (CLP). Additionally, lipidomics studies allowed us to uncover the biochemical mechanisms responsible for the altered lipidomes of the SS model and follow its treatments. Differentially expressed lipids were used for bioinformatics analyses via the ingenuity pathway analysis (IPA).
2. Materials and methods
2.1 Chemicals and reagents
Acetonitrile, HPLC grade, was purchased from Fisher Corporation (Loughborough, UK); ultra-pure water was produced by a Milli-Q water system (Millipore, USA). Lipid standards including lysoPC(O-18:0), glycerophosphocholine, guanosine triphosphate, 3-hydroxybutyric acid, lysoPE(0:0/16:0), PC(16:0/18:0), 14,15-DiHETrE, lysoPC(16:0), 2-aminobenzoic acid, and phytosphingosine were acquired from Avanti Polar Lipids, Inc. (Alabaster, AL). Huaxian capsule (HXC) was a clinical preparation and obtained from First Affiliated Hospital of Heilongjiang University of Chinese Medicine.
2.2 Animal
Adult male Wistar rats (weighing 220 ± 20 g) were purchased from the SLAC Laboratory Animal Co. (Shanghai, China) and kept in SPF-grade Experimental Animal Houses of Heilongjiang University of Chinese Medicine (Harbin, China) with free access to food and water under standard temperature conditions at 24 ± 2 °C with 40 ± 5% humidity and a 12 h light/dark cycle. The experimental procedures were approved by the Animal Care and Ethics Committee at the Heilongjiang University of Chinese Medicine, and all experiments were performed in accordance with the declaration of Helsinki.
2.3 Sepsis model
The cecal ligation and puncture (CLP) method was used as an SS model, as described in the literature.21 Under ether anesthesia, a midline incision was made, and the cecum was divided carefully while avoiding all blood vessels. The distal two-third of the cecum was punctured twice with an 18-gauge needle and ligated tightly. Subsequently, the cecum was placed back in the peritoneal cavity, and the abdominal cavity was closed. In sham surgical controls, the cecum was exposed, but not punctured and ligated before being returned to the abdominal cavity. The animals were allowed to acclimatize and placed in the metabolism cages.
2.4 Sample collection
The rats were randomly divided into a model group (n = 8, treated with 0.2 mL solution immediately and 24 h after CLP), HXC treatment group (n = 8, orally administered at a dose of 2 g kg−1 of body weight once a day and 24 h after CLP), and sham (control) group (n = 8, treated with sham operation and the same volume of 0.9% saline solution). Drug was administered between 8:00 and 9:00 to minimize any effects of the circadian rhythm. After 3 consecutive days of administration, all the rats were sacrificed by exsanguination from the abdominal aorta under isoflurane anesthesia. The blood was obtained in tubes and centrifuged at 10
000 rpm for 10 min at 4 °C. Then, the plasma was obtained and stored at −80 °C for analysis.
2.5 Lipid extraction
Lipid extraction was carried out in high grade polypropylene deep well plates. Herein, fifty μL of diluted plasma (100 mL) was mixed with 200 mL of ammonium bicarbonate solution, and then, 200 mL of methyl tert-butyl ether/methanol (7
:
2, v/v) solution was added. The plate was then shaken at 4 °C for 10 min, and spun down (3000g, 10 min) to facilitate separation of the liquid phases and clean-up of the upper organic phase. The organic phase was evaporated under N2 and the residue was dissolved in methanol/chloroform (9
:
1, vol/vol). After being mixed for 30 s and centrifuged for 15 min at 3000g and 4 °C, the samples were transferred to glass vials for UPLC/MS analyses.
2.6 Non-targeted lipidomics
Non-targeted LC-MS-based lipidomics analysis was performed by a hyphenated liquid chromatography-mass spectrometry (LC-MS) system equipped with ACQUITY™ ultra-performance liquid chromatography (UPLC) plus a mass spectrometer (Waters; Milford, MA, USA) was employed for global lipidomics profiling. QC samples were analyzed using all 8 samples within a run. The acquity UPLC BEH-C18 column (2.1 mm × 100 mm × 1.8 μm) was used for all analyses. The column was maintained at 40 °C, and a gradient of 0.1% formic acid in water as the mobile phase A and acetonitrile as mobile phase B was used. The gradient comprised 0 min elution (5% B), 2 min elution (40% B), 6 min elution (80% B), 7 min elution (100% B), and 9 min elution (100% B). The injected sample volume was 2 μL for each run. The flow rate of the mobile phase was 0.4 mL min−1. The samples were maintained at 4 °C during the analysis. The mass spectrometry parameters were set as follows: capillary voltage, 3.0 kV; source temperature, 120 °C; desolvation temperature, 550 °C; cone gas flow, 50 L h−1; and desolvation gas flow, 450 L h−1. Centroid data were obtained from 50 to 1000 m/z at a scan time of 0.03 s, and an interscan delay of 0.02 s. Data were processed further using MassLynx 4.1 (Waters, Manchester, U.K.).
2.7 Data analysis and post-processing
Lipid identities were determined based on accurate mass, chromatographic retention, and tandem mass spectrometry (MS/MS) fragmentation patterns, facilitated by software QI (Waters; Milford, MA, USA). Data post-processing and normalization were performed using an in-house developed data management system. Nomenclature and abbreviations of the lipid species were provided following the classification system proposed by LIPIDMAPS (http://www.lipidmaps.org/). The EZinfo 2.0 software (Waters Corp, Milford, USA) was employed for multi-variable analysis, including principal component analysis (PCA) and partial least squares-discriminate analysis (PLS-DA) and orthogonal partial least-squares discriminate analysis (OPLS-DA). Heat map visualization test was conducted by the open-source software Metaboanalyst (http://www.metaboanalyst.ca).
2.8 Pathway analysis
The metabolic pathways of lipid could be constructed via metaboanalyst using a hypergeometric distribution-based approach, based on database sources including KEGG (http://www.kegg.jp/), HMDB (http://www.hmdb.ca/), and SMPD (http://www.smpdb.ca/), to analyze and visualize the metabolic pathways and facilitate further biological interpretation. SPSS 17.0 using the t test for Windows was used for the statistical analysis.
2.9 Enrichment analysis
To investigate the significance of the altered lipid observed by LC-MS, we analyzed the relevant biological function networks and candidate lipids using the ingenuity pathway analysis (IPA) software (Ingenuity Systems, http://www.ingenuity.com). We uploaded the lipid lists (with KEGG IDs) and the changes of the related metabolites onto an IPA server.
3. Results and discussion
Using the optimal chromatography conditions as described, the representative LC/MS spectra of the control and HXC group or model group were obtained in positive and negative modes (Fig. 1). PCA analysis was conducted to exhibit the metabolic distinction between the control and SS groups from lipidomics. A pronounced separation between controls and model samples was revealed by the PCA score plot (Fig. 2A and B), indicating distinct alterations of lipid metabolism in SS. Significant differences between endogenous metabolites in the sham operated and model groups were determined from the VIP-plot of the OPLS-DA. The VIP-plots from the OPLS analysis (Fig. 2C and D) helped identify the variables that accounted for this significant separation. They were selected using the VIP (variable importance in the projection) values (>8) combined with Student's t test (p < 0.05) with a false discovery rate (FDR) < 0.05. Based on the VIP plot, ten endogenous lipids including lysoPC(O-18:0), glycerophosphocholine, 14,15-diHETrE, guanosine triphosphate, 3-hydroxybutyric acid, lysoPE(0:0/16:0), PC(16:0/18:0), lysoPC(16:0), 2-aminobenzoic acid, and phytosphingosine, were selected as potential biomarkers (Table 1). Following the identification process, these lipids were identified over a narrow ±5 ppm, with 7 identified in the positive mode and 3 identified in the negative mode. Interestingly, 7 of the metabolites detected were found to be up-regulated, whereas 3 were found to be down-regulated.
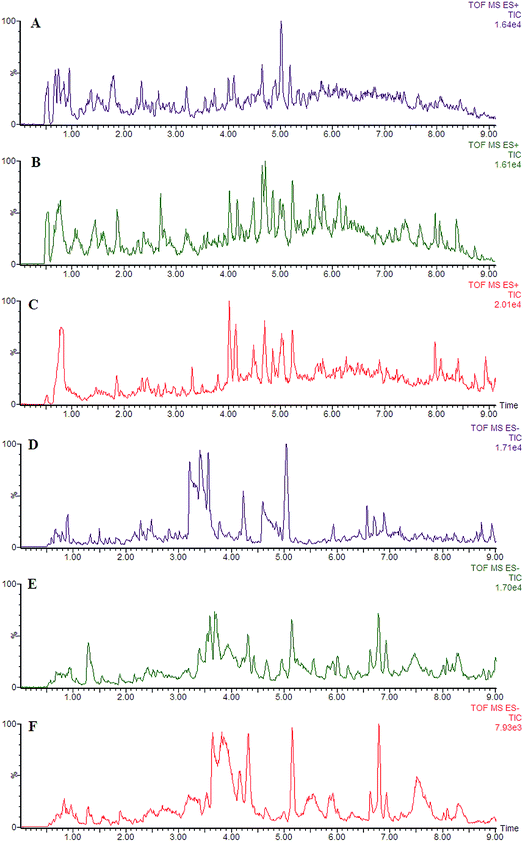 |
| Fig. 1 Representative liquid chromatography/mass spectrometry spectra of the control, model, and HXC groups in the ESI+ and ESI− mode. Note: control group (A), model group (B), and HXC group (C) in the ESI+ mode; control group (D), model group (E), and HXC group (F) in the ESI− mode. | |
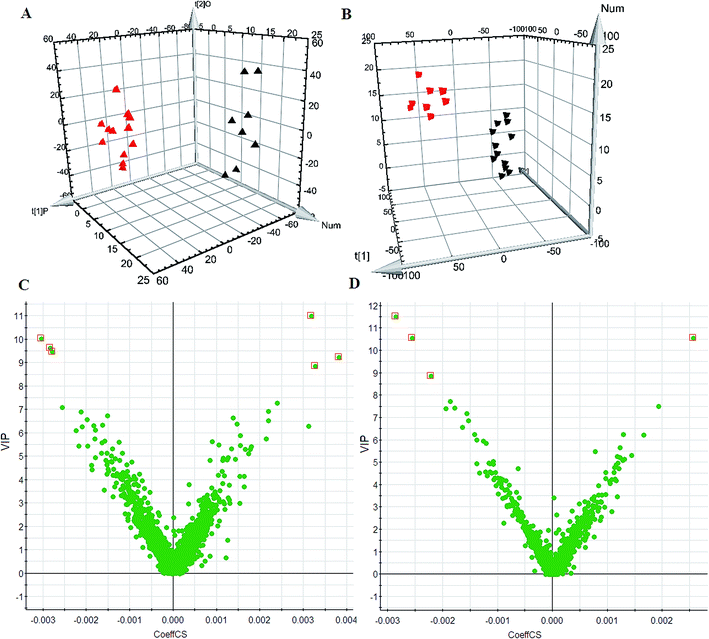 |
| Fig. 2 Untargeted lipidomics analysis using ultra-performance liquid chromatography/quadrupole time-of-flight mass spectrometry. PCA score plots of untargeted lipidomics dataset from control (red) and model group (black) in ESI+ (A) and ESI− (B) mode; VIP-plots constructed from the supervised OPLS analysis in the ESI+ (C) and ESI− (D) mode. | |
Table 1 Identification of lipids by ultra-performance liquid chromatography/quadrupole time-of-flight mass spectrometry
No. |
VIP |
Retention time (min) |
m/z |
Compound ID |
Adducts |
Formula |
Mass error (ppm) |
Compound |
Anova (p) |
Max fold change |
1 |
9.48 |
4.66 |
532.3712 |
HMDB11149 |
M + Na |
C26H56NO6P |
−1.98 |
LysoPC(O-18:0) |
0.0000 |
13.57 |
2 |
9.66 |
1.89 |
280.0929 |
HMDB00086 |
M + Na |
C8H20NO6P |
2.46 |
Glycerophosphocholine |
0.0000 |
142.09 |
3 |
8.87 |
4.15 |
361.2358 |
HMDB02265 |
M + Na |
C20H34O4 |
2.57 |
14,15-DiHETrE |
0.0000 |
5.60 |
4 |
10.04 |
2.37 |
545.9849 |
HMDB01273 |
M + Na |
C10H16N5O14P3 |
1.59 |
Guanosine triphosphate |
0.0000 |
167.04 |
5 |
9.25 |
1.98 |
127.0371 |
HMDB00357 |
M + Na |
C4H8O3 |
0.84 |
3-Hydroxybutyric acid |
0.0000 |
14.33 |
6 |
11.00 |
3.55 |
476.2797 |
HMDB11473 |
M + Na |
C21H44NO7P |
1.90 |
LysoPE(0:0/16:0) |
0.0000 |
2.24 |
7 |
11.52 |
1.69 |
784.5851 |
LMGP01010573 |
M + Na |
C42H84NO8P |
3.16 |
PC(16:0/18:0) |
0.0000 |
5.34 |
8 |
10.56 |
4.25 |
495.3424 |
HMDB10382 |
M − H |
C24H49NO7P |
2.29 |
LysoPC(16:0) |
0.0009 |
9.83 |
9 |
10.57 |
1.87 |
137.0562 |
HMDB01123 |
M − H |
C7H6NO2 |
2.37 |
2-Aminobenzoic acid |
0.0000 |
170.93 |
10 |
8.88 |
4.21 |
317.3008 |
HMDB04610 |
M − H |
C18H38NO3 |
0.54 |
Phytosphingosine |
0.0000 |
156.60 |
To determine the possible pathways contributing to SS, MetPA analysis was performed to identify metabolic pathways and their networks using an online database. This analysis resulted in the construction of 10 metabolic pathways in the plasma (Fig. 3A and Table S1†) that was important for host-responses to SS. Among the identified metabolic pathways, ether lipid metabolism (impact-value: 0.2143), glycerophospholipid metabolism (impact-value: 0.2065), purine metabolism (impact-value: 0.0114), and tryptophan metabolism (impact-value: 0.0019) in plasma were determined to be most important. This change indicated that SS had significantly altered lipid metabolism as compared to its normal state. A previous study determined that22 using untargeted lipidomics approach, glycerophospholipid metabolism was shown to be disturbed in the SS disease patients, and relevant lipids were detected. In the network function analysis of the differentially-expressed metabolites performed by IPA, the altered lipids tended to gather into an integrated network (Fig. 3B). These discoveries supported our results indicating that lipid metabolism played an important role in SS disease.
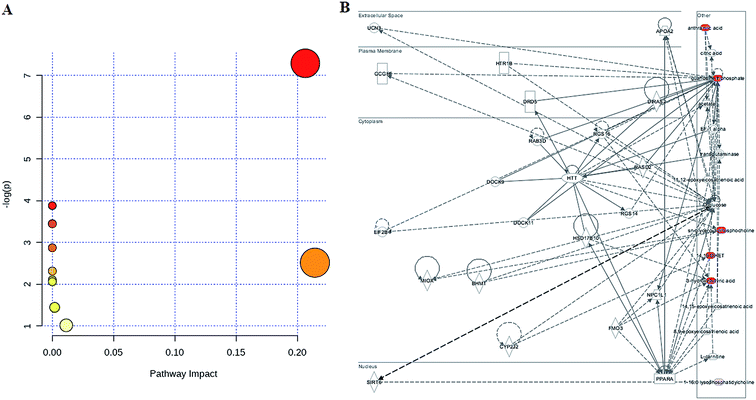 |
| Fig. 3 Construction of the altered pathways using MetPA analysis (A) and the merged networks of the identified lipids imported into the ingenuity pathway analysis tool based on the publication database (B). | |
PCA was used to investigate the metabolic effects of intervention with HXC. In the PCA score plot, metabolic profile of the control and that of SS groups were clearly separated from each other (Fig. 4); this suggested remarkable alteration of plasma metabolites in the SS model in the positive mode and 3 were identified in the negative mode. In addition, HXC groups affected the metabolite variations, and PLS-DA score plot showed a clear separation between the HXC group and the SS group. However, as shown in Fig. 4A and B, the HXC group is closer to the control group than the SS groups; this indicates that HXC group has a better therapeutic effect. In addition, we analyzed the intensity changes of ten lipids between HXC, control groups, and model groups. Fig. 4C presents the differential lipids revealing the relatively up-regulated (red) or down-regulated (green) intensities of the lipid compound in the control, model, and HXC groups. The results showed that HXC possessed certain curative effect by normalizing or partially reversing the SS-induced alterations.
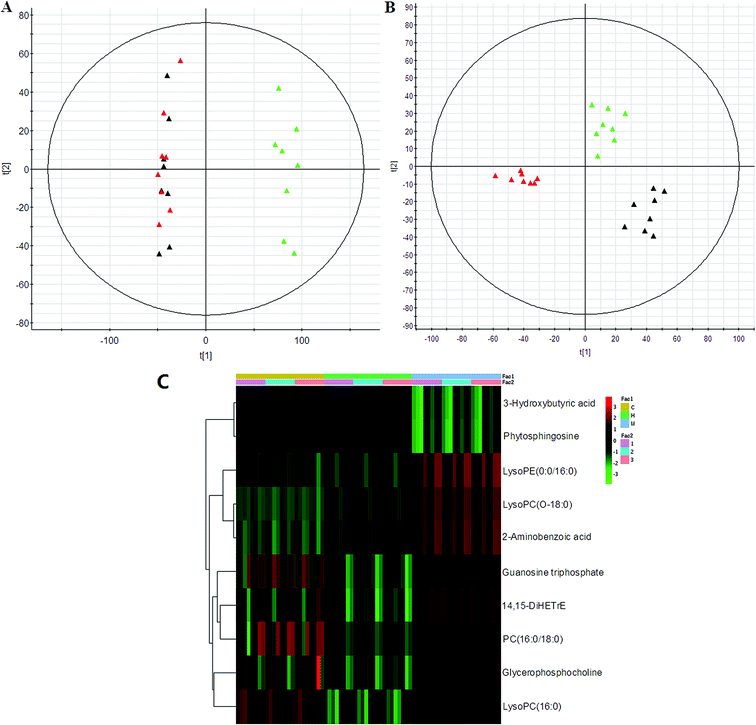 |
| Fig. 4 Lipidomics revealed aberrant lipid metabolism of HXC affecting SS rats in the (A) ESI+ and (B) ESI− mode. Note: control group (red), model group (green), and HXC group (black). Heat map visualization of the differential lipid composition (C). The columns show the expression levels and each row represents a lipid. The red color indicates upregulated lipid, whereas the green color represents downregulated lipid. C, control group; M, model group; H, and HXC group. | |
4. Conclusions
To the best of our knowledge, for the first time, plasma lipidomics technology was employed to explore lipid changes in the pathophysiology of SS rats and for the response treatment of SS with HXC. Herein, ten potential lipids were identified, and it was suggested that multiple metabolic pathways such as ether lipid metabolism, glycerophospholipid metabolism, purine metabolism, and tryptophan metabolism were disturbed in SS rats. Metabolic profiling analyses verified the therapeutic effect of HXC. The results demonstrated that concise signatures of lipids may serve as biomarkers of SS. This suggests that high-throughput lipidomics coupled with multivariate data analysis and pathway analysis provides a feasible way to efficiently discover regulated lipid signatures.
Conflicts of interest
The authors declare no competing financial interests.
Acknowledgements
We thank BGI for excellent technical assistance. This work was supported by the grants received from the Key Program of Natural Science Foundation of State (Grant No. 81470196, 81302905), Natural Science Foundation of Heilongjiang Province of China (H2015038), and Youth Innovative Talent Program of Heilongjiang Province of China (UNPYSCT-2015118).
References
- X. Shi, F. Yang, Y. N. Zheng, H. Zhang, X. X. Wang, G. J. Shao and X. L. Lai, Metabolomic approach for the identification of therapeutic targets of erythropoietin against sepsis in rat models, Eur. Rev. Med. Pharmacol. Sci., 2016, 20(3), 537–546 CAS.
- Q. Liang, H. Liu, T. Zhang, Y. Jiang, H. Xing and A. Zhang, High-throughput metabolic profiling for discovering metabolic biomarkers of sepsis-induced acute lung injury, RSC Adv., 2016, 6, 11008–11013 RSC.
- M. Garcia-Simon, J. M. Morales, V. Modesto-Alapont, V. Gonzalez-Marrachelli, R. Vento-Rehues, A. Jorda-Miñana, J. Blanquer-Olivas and D. Monleon, Prognosis Biomarkers of Severe Sepsis and Septic Shock by 1H NMR Urine Metabolomics in the Intensive Care Unit, PLoS One, 2015, 10(11), e0140993 Search PubMed.
- Q. Liang, H. Liu, T. Zhang, Y. Jiang, H. Xing and A. Zhang, Potential urine biomarkers from a high throughput metabolomics study of severe sepsis in a large Asian cohort, RSC Adv., 2015, 5, 102204–102209 RSC.
- S. Li, J. Xu, Y. Jiang, C. Zhou, X. Yu, Y. Zhong, J. Chen and X. Yan, Lipidomic analysis can distinguish between two morphologically similar strains of nannochloropsis oceanica, J. Phycol., 2015, 51(2), 264–276 CrossRef CAS PubMed.
- Q. Liang, H. Liu and H. Xing, et al., Urinary UPLC-MS metabolomics dissecting the underlying mechanisms of Huaxian capsule protects against sepsis, RSC Adv., 2016, 6(46), 40436–40441 RSC.
- Y. Y. Zhao, H. Miao, X. L. Cheng and F. Wei, Lipidomics: Novel insight into the biochemical mechanism of lipid metabolism and dysregulation-associated disease, Chem.-Biol. Interact., 2015, 240, 220–238 CrossRef CAS PubMed.
- Y. Y. Zhao, X. L. Cheng and R. C. Lin, Lipidomics applications for discovering biomarkers of diseases in clinical chemistry, Int. Rev. Cell Mol. Biol., 2014, 313, 1–26 Search PubMed.
- C. Hu, M. Wang and X. Han, Shotgun lipidomics in substantiating lipid peroxidation in redox biology: methods and applications, Redox Biol., 2017, 12, 946–955 CrossRef CAS PubMed.
- Y. Y. Zhao, X. L. Cheng, R. C. Lin and F. Wei, Lipidomics applications for disease biomarker discovery in mammal models, Biomarkers Med., 2015, 9(2), 153–168 CrossRef CAS PubMed.
- W. Hewelt-Belka, J. Nakonieczna, M. Belka, T. Bączek, J. Namieśnik and A. Kot-Wasik, Untargeted Lipidomics Reveals Differences in the Lipid Pattern among Clinical Isolates of Staphylococcus aureus Resistant and Sensitive to Antibiotics, J. Proteome Res., 2016, 15(3), 914–922 CrossRef CAS PubMed.
- H. Begum, B. Li, G. Shui, A. Cazenave-Gassiot, R. Soong, R. T. Ong, P. Little, Y. Y. Teo and M. R. Wenk, Discovering and validating between-subject variations in plasma lipids in healthy subjects, Sci. Rep., 2016, 6, 19139, DOI:10.1038/srep19139.
- J. E. Kyle, X. Zhang, K. K. Weitz, M. E. Monroe, Y. M. Ibrahim, R. J. Moore, J. Cha, X. Sun, E. S. Lovelace, J. Wagoner, S. J. Polyak, T. O. Metz, S. K. Dey, R. D. Smith, K. E. Burnum-Johnson and E. S. Baker, Uncovering biologically significant lipid isomers with liquid chromatography, ion mobility spectrometry and mass spectrometry, Analyst, 2016, 141(5), 1649–1659 RSC.
- T. Cajka and O. Fiehn, Toward Merging Untargeted and Targeted Methods in Mass Spectrometry-Based Metabolomics and Lipidomics, Anal. Chem., 2016, 88(1), 524–545 CrossRef CAS PubMed.
- Y. Y. Zhao, S. P. Wu, S. Liu, Y. Zhang and R. C. Lin, Ultra-performance liquid chromatography-mass spectrometry as a sensitive and powerful technology in lipidomic applications, Chem.-Biol. Interact., 2014, 220, 181–192 CrossRef CAS PubMed.
- Q. Liang, et al., Lipidomics Analysis Based on Liquid Chromatography Mass Spectrometry for Hepatocellular Carcinoma and Intrahepatic Cholangiocarcinoma, RSC Adv., 2015, 5, 63711–63718 RSC.
- M. Wang, C. Wang, R. H. Han and X. Han, Novel advances in shotgun lipidomics for biology and medicine, Prog. Lipid Res., 2016, 61, 83–108 CrossRef CAS PubMed.
- Q. Liang, T. Zhang, Y. Jiang and A. Zhang, Untargeted lipidomics study of coronary artery disease by FUPLC-Q-TOF-MS, Anal. Methods, 2016, 8, 1229–1334 RSC.
- J. Song, X. Liu, J. Zhu, M. Tootoonchi, J. M. Keith, S. P. Meduna, C. A. Dvorak, W. Eccles, P. J. Krawczuk, J. M. Blevitt, J. Wu, N. L. Rao, A. D. Lebsack and M. E. Milla, Polypharmacology of Small-Molecule Modulators of the 5-Lipoxygenase Activating Protein (FLAP) Observed via a High-throughput Lipidomics Platform, J. Biomol. Screening, 2016, 21(2), 127–135 CrossRef CAS PubMed.
- Q. Liang, C. Wang, B. Li and A. H. Zhang, Lipidomics Analysis Based on Liquid Chromatography Mass Spectrometry for Hepatocellular Carcinoma and Intrahepatic Cholangiocarcinoma, RSC Adv., 2015, 5, 63711–63718 RSC.
- K. A. Wichterman, A. E. Baue and I. H. Chaudry, Sepsis and septic shock–a review of laboratory models and a proposal, J. Surg. Res., 1980, 29(2), 189–201 CrossRef CAS PubMed.
- M. Ferrario, A. Cambiaghi, L. Brunelli, S. Giordano, P. Caironi, L. Guatteri, F. Raimondi, L. Gattinoni, R. Latini, S. Masson, G. Ristagno and R. Pastorelli, Mortality prediction in patients with severe septic shock: a pilot study using a target metabolomics approach, Sci. Rep., 2016, 6, 20391 CrossRef CAS PubMed.
Footnote |
† Electronic supplementary information (ESI) available. See DOI: 10.1039/c7ra07873k |
|
This journal is © The Royal Society of Chemistry 2017 |
Click here to see how this site uses Cookies. View our privacy policy here.