DOI:
10.1039/C7RA05959K
(Paper)
RSC Adv., 2017,
7, 39185-39196
The effect of V155M mutation on the complex of hSTING and 2′3′-cGAMP: an in silico study case†
Received
28th May 2017
, Accepted 4th August 2017
First published on 10th August 2017
Abstract
Human stimulator of interferon genes protein (hSTING) is an essential signaling adaptor and a cytosolic DNA sensor (CDS) that functions as a homodimer in innate immunity. Natural mutation V155M in hSTING can cause hSTING-associated vasculopathy with onset in infancy (SAVI) which is an autoinflammatory disease. However, the mechanisms between the variant and SAVI are poorly understood. To explore the mechanisms, we performed all-atom molecular dynamics (MD) simulations on the complexes of wild type (WT)/mutated (V155M) hSTING and endogenous agonist 2′3′-cyclic guanosine monophosphate–adenosine monophosphate (2′3′-cGAMP) to investigate whether the interaction between hSTING and 2′3′-cGAMP could be affected by natural mutation V155M, which plays a crucial role in SAVI disease. Based on the MD simulations, the dynamic cross-correlation analysis and principal component analysis indicated that the single point mutation on residue 155 from valine to methionine changed some residues' motions of hSTING. Furthermore, MM/PBSA calculation, hydrogen bond analysis and energy decomposition analysis showed that this single point mutation increased the binding affinity of 2′3′-cGAMP with hSTING. Finally, residual network analysis indicated the way that the V155M mutation around 17 Å from the binding site controlled the strength of binding interaction and the conformation change of the substructure. These results will help in the understanding of the mechanism of SAVI disease induced by hSTING mutation at the molecular level, and provide new ideas to design innovative drugs targeted on hSTING.
Introduction
Human stimulator of interferon genes protein (hSTING) is a predominantly endoplasmic reticulum-localized protein acting as a key factor in mediating the type I interferon production in response to various forms of pathogen-derived DNA, as well as self-DNA by acting as an adaptor protein to recruit and activate TANK binding kinase (TBK1) and inhibitory kappa B kinases (IKK).1,2 In the human body, hSTING is mainly activated by binding to a second message when microbial or host DNA is delivered to the cytoplasm.3,4 All above biological functions of hSTING protein depend on the style of its homodimer which constructs the c-di-GMP-binding domain (CBD) with residues 153–340. Different ligands binding in CBD induce different degrees of conformational rearrangements and activation of hSTING. The endogenous ligand 2′3′-cGAMP was identified as the most affinity ligand for hSTING by Jiaxi Wu and Xu Zhang's work.5,6 Normally, this cGAMP–hSTING pathway doesn't cause hSTING-associated vasculopathy with onset in infancy (SAVI) due to the dynamic balance of hSTING conformation change between active and inactive conformational ensembles.7 Once the conformation balance is disturbed, it may result in serious disease. For example, the balance may be biased to the active ensembles in the natural variant V155M hSTING, consequently causing the terrible SAVI disease.8 Biological experimental studies have proved that the single point mutation on residue 155 from valine (VAL) to methionine (MET) is enough to induce SAVI as whole-exome sequencing studies have identified de novo mutation p.V155M in STING in patients.8 Therefore, protein hSTING is an attractive and direct target for treating SAVI and other hSTING relative autoinflammatory diseases.9–12 To explore its mechanism, great efforts have been made to investigate the X-ray crystal structures of the C-terminal domain of hSTING (residues 154–337, termed hSTINGCTD). These studies suggest that the ligand binding site of hSTING locates in the dimer interface of hSTING protein and consists of long twist β3–β4 loops (termed lid with residues 226–241), α1A part (residues 159, 162–163, and 166–167) and α2 tails (residues 261–266) as displayed ocean blue, red and magenta region in Fig. 1(A), respectively. Amino acids at positions around residue 155 are absolutely conserved across the hSTING orthologues,13 which locates on the α-helix 1A (Fig. 1(A)). The α-helix was identified to be part of the folded soluble CBD (c-diGMP/c-GAMP binding domain), mediating the homodimerization of hSTING.14 However, the static crystal structural studies may not result in accurate conclusions, especially for these functions in dynamic conformations.
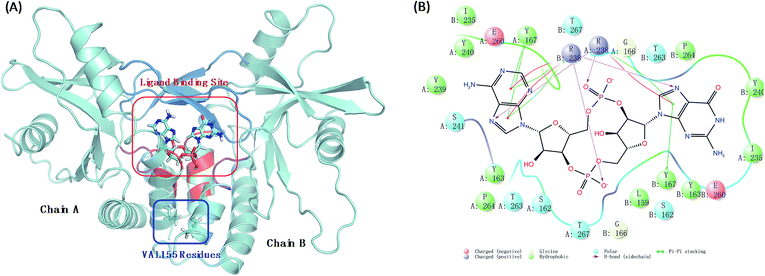 |
| Fig. 1 The interaction models of wild type hSTING and ligand 2′3′-cGAMP. (A) Homodimer form of hSTING and 2′3′-cGAMP complex (PDB: 4LOH). Ligand binding site mainly includes lid region (ocean blue), α1A part (red) and α2 tail part (purple); (B) the detailed interaction diagram of the residues constructing the ligand binding site and 2′3′-cGAMP was plotted using maestro 10.1 software. | |
Above all, the mechanism how the single point mutation V155M affects the activity of hSTING and breaks the conformation balance is still unknown. As the supplement and extension of the experimental work, molecular dynamics simulation is a very useful way to investigate the structural dynamics of biomolecular complexes and how they are linked to physiological functions and diseases. Thus, the goal of this work is to find out whether and how the ligand–receptor binding interaction and the conformation of hSTING are affected by the remote mutation (V155 → M155) from ligand binding site. As the choice of starting structure would make a significant influence on the result of MD simulation, we compared all crystal structures in PDB database and prudently choose the best suitable structure for our MD simulation study which was described in detail in Methods and experiment section. The mutant hSTING/2′3′-cGAMP complex (mutation type, MT), which was termed V155M as the mutation of 155 residue VAL to MET on both chains in this article, was first constructed based on the wild type (WT) hSTING/2′3′-cGAMP structure (PDB: 4LOH),7 then ran WT/MT molecular dynamics (MD) simulations, respectively. After that, the WT/MT MD simulations were analysed and compared to provide a new insight to how natural variance in the remote position from ligand binding site affects the conformation of hSTING and the interaction between hSTING and its agonist. The fluctuation of alpha C was characterized based on the RMSF and PCA analysis, which showed the natural variant V155M could increase the flexibility of B:β5–α4 loop. This transformation in residual flexibility might eventually change the conformational behavior of hSTING as observed from principal component analysis. Moreover, by the dynamic cross-correlation analysis, we found that the V155M mutation could trigger the effect of enhancing almost all the residual correlated motions of hSTING and reserve the relationship of residual pairs' motion. Although this site of natural mutation is far away (17 Å) from the ligand binding site, it can affect the binding site and induce the conformation change of substructure as residue interaction network detected. In addition, it was found that V155M mutation could enhance the interactions of 2′3′-cGAMP with SER162:B, ARG238:B and THR263:B of hSTING based on the energy decomposition and hydrogen bond analysis. These findings may provide a new insight to the possible molecular mechanism of SAVI disease induced by the V155M mutation.
Results and discussion
Equilibrium of the dynamics simulation
The root mean square deviation (RMSD) of backbone atoms (Cα, C, N and O) referring to the initial structures from the preparation procedure was calculated and displayed in Fig. 2(A), where both MT and WT systems generated converged ensembles from their starting structures behind 30 ns. The RMSD values of these two systems were around about 1.75 Å in the entire time, indicating that both systems reached equilibrium state during the last 50 ns (from 30 ns to 80 ns). Moreover, we also monitored the RMSD of the residues constructing the ligand binding site (Fig. 1(B)) and ligand 2′3′-cGAMP as shown in Fig. S1 and S2,† respectively. Both RMSD values are small than 1.5 Å.
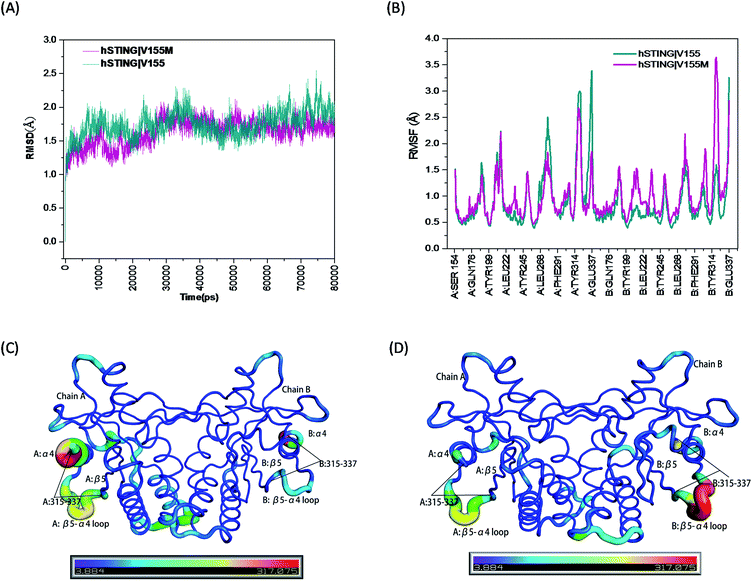 |
| Fig. 2 The RMSD, RMSF and B-factor of hSTING in WT/MT systems. (A) The RMSD of backbone atoms (O, C, Cα, N) in the MD simulations; (B) the RMSF of Cα atoms during the last 50 ns of the MD simulations; (C) the B-factors (Å2) of hSTING in wild type system during the last 50 ns of the MD simulation; (D) the B-factors (Å2) of hSTING in V155M system (MT system) during the last 50 ns of the MD simulation. | |
High flexibility loops of hSTING can always fit their conformations to bind other factors (correspond peptides, proteins and nucleic acids). For example, increasing flexibility of B:β5–α4 loop in MT system can make TBK1/IRF3 recruitment and activation easier.15 So we also calculated the root mean square fluctuation (RMSF) and B-factor of Cα atoms during the last 50 ns of the MD simulations which both can reflect the flexibilities of residues to study the dynamics stability of these two systems. As shown in Fig. 2(B), the V155M mutation obviously influences the stability of residues 315–337 where the position of highest peak for RMSF is different between in chain A and chain B. Comparing with WT system, V155M mutation largely enhances the flexibility of B:β5–α4 loop while makes A:α4 tail more stable in MT system (Fig. 2(C) and (D)). Moreover, there is a small enhanced flexibility of the segment (residues 302–307 in chain B) which near the large peak (residues 315–337 in chain B) as displayed in Fig. 2(B). These changes may be highly correlated with the V155M mutation, which will be further explored in the residual network analysis.
Dynamic cross-correlation (DCC) analysis
In order to analyze the change of residual motions induced by the single point mutation V155M, we calculated cross-correlation matrices of the alpha carbon fluctuations and plotted dynamic cross-correlation maps in the last 50 ns of both systems. In these two symmetric maps (Fig. 3(A) and (B)), highly positive regions (blue and dark purple) mean strong correlated motions that involved residues are in general moving in the same direction. While negative regions (red and dark red) stand for strong anticorrelation where related residues are generally moving in reverse directions. There are highly correlated motions nearby the diagonal as displayed in Fig. 3(A) and (B), which stands for the correlations of a residue with itself and neighbours. The degree of correlation between chain A and itself is stronger than its correlation with chain B in both complexes, as more blue color-block in left lower and right upper quadrant than left upper and right lower quadrant. Moreover, by contrast WT/MT maps, an overall enhanced correlated motion is observed in the mutated hSTING–agonist complex system as less red patches in the MT map. This suggests that only single point mutation V155M can trigger the effect which enhances almost all the residual correlated motions of hSTING. In detail, a large range region displayed anti-correlated motions (red color) in WT map while showed correlated motion (yellow and green color) in MT map is detected in the left upper and right lower quadrant. Other regions also display the same phenomenon, such as large red cross and yellow-green cross in the left lower and right top quadrant in WT and MT map, respectively.
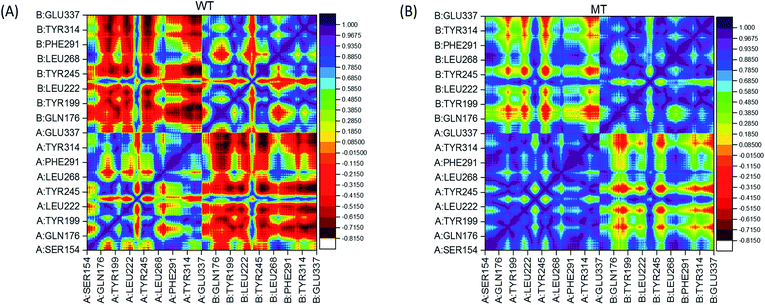 |
| Fig. 3 Dynamical cross-correlation maps. (A) WT (hSTING|V155); (B) MT (hSTING|V155M). The association strength of anti-correlated and correlated motions are color-coded. | |
Based on above results, it indicates that only one different factor (V155M) can reserve the relationship of residual pairs' motion in these regions, which may be related to V155M causing to SAVI.
Principal component analysis (PCA)
Biological functions of proteins usually coupling with their collective atomic motions, some diseases may be generated as the intrinsic motion changing of those proteins. Hence, it is very important to find out the different atomic motions of V155M system from normal hSTING in order to get insight into the mechanism of SAVI on the molecular level. To detected the significant different motions which may be related to SAVI disease in 2′3′-cGAMP bounded hSTING systems, we performed principal component analysis (PCA) for both WT and MT systems. The eigenvalue spectra show the eigenvalues rank versus the proportion of variances as shown in Fig. 4(A) and (B). The top 20 principal components (PC) can capture 71.4% and 72.2% of the variance of conformation changes observed in the equalized state from both systems. Scores for the first PCs for WT and MT system are 20.28% and 17.22%, while for the second PCs are 11.09% and 16.02%, respectively. The conformational changes displayed by the first two dimensional PCs plots are obviously different between the two systems (Fig. 4(C) and (D)). In WT system, there are two big groups conformations along the PC1 while the similar conformational behavior is not detected in the MT system. To explore how V155M mutation affects the motions represented by above PC1 and PC2, we calculated the residual displacements along these two PCs during the equilibrium state of MD. Fig. 4(E) and (F) show that subunit fluctuations of residues 315–337 are significant differences in both two chains. In V155M system (magenta line in Fig. 4(E) and (F)), there are a small peak and a large peak near residue B:315 along PC1 and PC2, respectively. Similarly, a large peak along PC1 and a small peak along PC2 near the α4 tail of chain A (A:315–337) in wild type system. These results are very similar to the former RMSF analysis. It indicates that the top two PCs dominate the conformational fluctuations of hSTING. The results from both analytical methods suggest that V155M translates the biggest fluctuation from one chain to another. As the dimer structure of hSTING which agonist 2′3′-cGAMP bounded is originally not completely symmetric,16 this different fluctuation caused by V155M mutation may directly contribute to induce the SAVI disease.
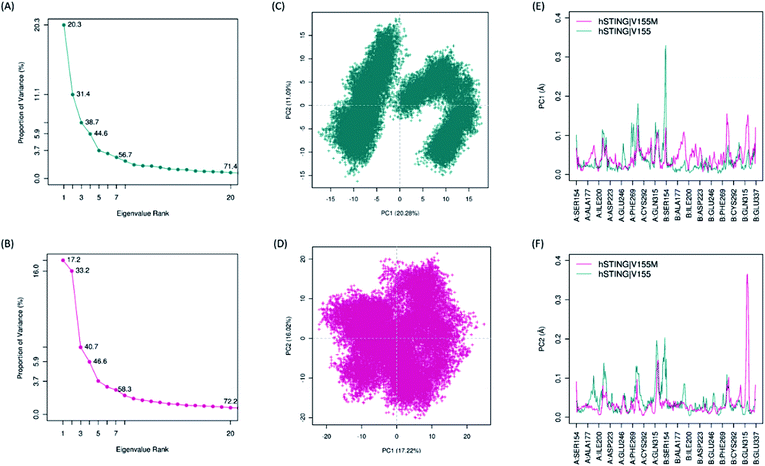 |
| Fig. 4 The results of principal component analysis. Magenta: hSTING|V155M (MT). Dark cyan: hSTING|V155 (WT). (A) and (B), Eigenvalue rank based on the percentage of the total mean square displacement (or variance) of atom positional fluctuations captured in each corresponding dimension from the final 50 ns of MD runs; (C) and (D), the projection of motion in the essential subspace along PC1 and PC2 for the WT hSTING and V155M hSTING, respectively; (E) and (F), the displacements of residues along the first PC and second PC, respectively. | |
Binding free energy (BFE) analysis
To explore how the natural point mutation affected the interaction between hSTING and agonist 2′3′-cGAMP, we calculated the individual energy components and binding free energies of these two systems by the Molecular Mechanics Poisson Boltzmann Surface Area (MMPBSA) method. As shown in Table 1, ΔEvdw, ΔEele and ΔGnonpol mainly contribute to the binding of ligand 2′3′-cGAMP, while the polar solvent energy (ΔEpol) impedes the binding. The binding free energies for 2′3′-cGAMP bounded hSTING are −12.31 and −15.68 kcal mol−1 in WT and MT system, respectively. Furthermore, the dissociation constant (KD) used to describe the affinity of 2′3′-cGAMP with hSTING was also calculated by the binding free energy. For wild type hSTING/2′3′-cGAMP system, the calculated KD 1.06 nM is very close to the experimental KD(exp) 3.79 nM.6 Although no KD(exp) for V155M hSTING/2′3′-cGAMP system, we predict that it has an extremely strong sub-picomolar affinity as its calculated KD 3.7 pM. The binding free energy and dissociation constant analyses indicates that V155M mutation enhances the binding affinity between hSTING and 2′3′-cGAMP. These data further suggest that comparing with normal hSTING, V155M hSTING can be activated more easily by agonist 2′3′-cGAMP, then causing SAVI disease.
Table 1 Free energy of binding and each energy component between hSTING and 2′3′-cGAMP calculated with MM-PBSA (unit: kcal mol−1)a
Energy term |
WT |
V155M |
ΔEele van der Waals interaction energies between hSTING and ligand. ΔEvdw electrostatic interaction energies between hSTING and ligand. ΔEpol polar contributions to the solvation free energy. ΔEnonpol nonpolar contributions to the solvation free energy. −TΔS the enthalpic contribution to binding in temperature 300 K. ΔGbind binding energy from hSTING–ligand complex. KD this term calculated from ΔGbind = RT ln KD with T = 300 K and R = 1.9858775 × 10−3 kcal K−1 mol−1. KD(exp) the experimental dissociation constant was determined from ITC experiment.6 But there is no KD(exp) for V155M mutation. |
ΔEele |
−118.16 ± 8.21 |
−125.70 ± 9.41 |
ΔEvdw |
−66.31 ± 2.58 |
−70.68 ± 3.75 |
ΔEpol |
158.20 ± 4.39 |
163.86 ± 6.80 |
ΔEnonpol |
−8.07 ± 0.20 |
−8.21 ± 0.16 |
−TΔS |
22.04 ± 4.85 |
25.04 ± 2.44 |
ΔGbind |
−12.31 ± 1.12 |
−15.68 ± 1.72 |
KD |
1.06 nM |
3.7 pM |
KD(exp) |
3.79 nM |
NA |
Hydrogen bonds analysis
In consideration of the main contribution of the electrostatic interaction to the binding of ligand 2′3′-cGAMP in both systems, we investigated the hydrogen bonding interactions between 2′3′-cGAMP and corresponding residues of hSTING in each system. All hydrogen bonds were defined as that angle cutoff for hydrogen bonds were over 135° and distance cutoff for hydrogen bonds were no more than 3.5 Å. Then, the probability of each hydrogen bond was calculated and ranked by the method as Liu reported to quantitatively describe the stability of the hydrogen bonds.18 Agonist 2′3′-cGAMP forms hydrogen bonds mainly with ARG238:AB, THR263:AB, and GLU260:AB (Fig. 5(A) and (B)) based on the trajectories of equilibrium state. The hydrogen bonds with fraction over 10% are further analyzed in Table 2 (more detailed hSTING-ligand information is shown in ESI Table S1†). As shown in Table 2, the hydrogen bonds in the V155M system are quite different from WT system. First, there are five hydrogen bonds with more than 50% of fraction between hSTING and 2′3′-cGAMP in V155M complex, while three in WT. Second, even though the stabilities of hydrogen bonds formed by 2′3′-cGAMP and ARG238 in both complexes are similar, the concrete atoms involved in these hydrogen bonds are different. In V155M complex, the fractions of the hydrogen bonds formed by 2′3′-cGAMP-O4/ARG238-NH2 and 2′3′-cGAMP-O13/ARG238-NH1 are 96.14% and 74.82% mainly in chain B (the order name of ligand atoms plotted in Fig. 5(C)). While in WT complex, the fractions of the hydrogen bonds formed by 2′3′-cGAMP-O10/ARG238-NH2 and 2′3′-cGAMP-O10/ARG238-NH1 are 91.22% and 73.60% mainly in chain A. Third, compared with the situation of ARG238, the atoms that participate in the hydrogen bonds of THR263 are the same in both complexes; however, the total fraction for THR263 in V155M is 212.16% (86.01%, 73.85% and 52.30%), which is larger than WT 144.57% (65.78%, 28.11%, 14.72%, 14.04% and 11.03%). These data directly illustrate V155M increasing the percentage of stable H-bonds. As ligand–receptor has more stable H-bonds, the complex is more stable.
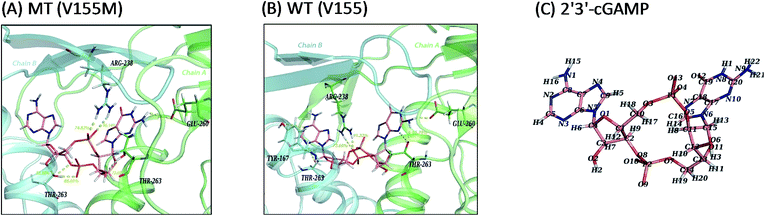 |
| Fig. 5 Ligand interaction diagrams and the structure of 2′3′-cGAMP. Chain A, chain B and ligand 2′3′-cGAMP are colored green, cyan and pink, respectively. Hydrogen bonds and polar interactions are indicated by yellow dash line. For clarity, only residues within 4 Å that have hydrogen bonds or polar interactions with 2′3′-cGAMP are shown and the fraction of hydrogen bonds above 50% are labeled in yellow number. (A) MT system (V155M); (B) WT system (V155); (C) the atom name of ligand 2′3′-cGAMP in MD simulations. | |
Table 2 Hydrogen bonds observed between 2′3′-cGAMP and key residues of hSTINGa
Acceptor |
Donor H |
Donor |
AvgD (Å) |
AvgA (°) |
Fra. (%) |
Angle cutoff for hydrogen bonds >135°, distance cutoff for hydrogen bonds <3.5 Å. |
V155M |
LIG369-O4 |
ARG238:B-HH22 |
ARG238:B-NH2 |
2.76 |
160.72 |
96.14 |
LIG369-O9 |
THR263:B-HG1 |
THR263:B-OG1 |
2.68 |
163.21 |
86.01 |
LIG369-O13 |
ARG238:B-HH12 |
ARG238:B-NH1 |
2.83 |
159.57 |
74.82 |
LIG369-O6 |
THR263:A-HG1 |
THR263:A-OG1 |
2.80 |
157.80 |
73.85 |
THR263:B-OG1 |
LIG369-H2 |
LIG369-O2 |
2.79 |
155.08 |
52.30 |
GLU260:A-OE2 |
LIG369-H22 |
LIG369-N9 |
2.77 |
146.08 |
32.99 |
LIG369-O4 |
ARG238:B-HH12 |
ARG238:B-NH1 |
2.87 |
144.80 |
14.06 |
SER241:B-O |
LIG369-H15 |
LIG369-N1 |
2.87 |
147.20 |
12.24 |
![[thin space (1/6-em)]](https://www.rsc.org/images/entities/char_2009.gif) |
WT |
LIG369-O10 |
ARG238:A-HH22 |
ARG238:A-NH2 |
2.77 |
155.32 |
91.22 |
LIG369-O10 |
ARG238:A-HH12 |
ARG238:A-NH1 |
2.81 |
149.47 |
73.60 |
THR263:B-OG1 |
LIG369-H2 |
LIG369-O2 |
2.68 |
162.62 |
65.78 |
GLU260:A-OE2 |
LIG369-H22 |
LIG369-N9 |
2.77 |
154.34 |
37.33 |
LIG369-O9 |
THR263:B-HG1 |
THR263:B-OG1 |
2.75 |
155.61 |
28.11 |
GLU260:A-OE1 |
LIG369-H21 |
LIG369-N9 |
2.77 |
156.90 |
26.70 |
LIG369-O13 |
ARG238:B-HH12 |
ARG238:B-NH1 |
2.79 |
157.38 |
25.36 |
LIG369-O4 |
ARG238:B-HH22 |
ARG238:B-NH2 |
2.82 |
158.48 |
20.93 |
LIG369-O13 |
ARG238:B-HH22 |
ARG238:B-NH2 |
2.83 |
151.25 |
18.34 |
THR263:A-OG1 |
LIG369-H22 |
LIG369-N9 |
2.88 |
156.76 |
14.72 |
THR263:A-OG1 |
LIG369-H21 |
LIG369-N9 |
2.88 |
154.44 |
14.04 |
GLU260:A-OE1 |
LIG369-H22 |
LIG369-N9 |
2.78 |
151.40 |
11.42 |
LIG369-O6 |
THR263:A-HG1 |
THR263:A-OG1 |
2.84 |
154.20 |
11.03 |
Binding free energy decomposition analysis
As the change of single hydrogen bonding may be not enough to reflect the variation of interaction between 2′3′-cGAMP and residues in hSTING. The total, electrostatic, van der Waals interaction and solvation energies between 2′3′-cGAMP and per-residue in hSTING were calculated for further analysis. Although V155 and M155 residues have little contribution to binding energy as shown in Fig. 6(A) and S3–S5,† V155M mutation has a significant effect on the interactions between 2′3′-cGAMP and residues in the ligand binding site of receptor. In Fig. 6(A), the main fluctuations of energy contribution are colored in lavender (α1A part), light blue (lid region) and light yellow (α2 tail) that mainly compose the ligand binding site. These significant variations of binding site are displayed in Fig. 6(B) in detail.
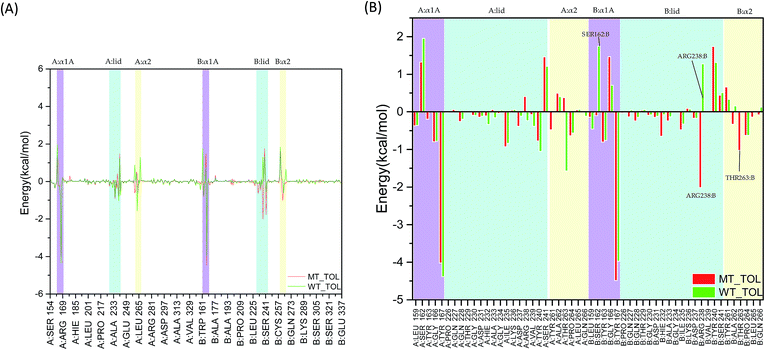 |
| Fig. 6 The total interaction energies decomposition of hSTING with the agonist. Lavender: α1A part (A:α1A and B:α1A); light blue: lid region (A:lid and B:lid); light yellow: α2 tail (A:α2 and B:α2). (A) Energy decomposition of residues 154–337; (B) energy decomposition of α1A part (A:α1A and B:α1A), lid region (A:lid and B:lid) and α2 tail (A:α2 and B:α2). | |
In the α1A part (residues 159, 162–163, and 166–167), the most different residue between WT and V155M is SER162:B, which reverses to a weak favorable one in V155M system (Fig. 6(B)). In the lid region (sequence 226–241), V155M enhances the interaction between agonist and ARG238:B, which is consistent with the previous hydrogen analysis. Although ARG238:A can form stable hydrogen bonds with the agonist in WT, it is not significant for the binding energy contribution considering on the effect of solvation energy (Fig. S5†). In the α2 tails (residues 261–266), V155M also increases the favorable energy between THR263:B and 2′3′-cGAMP. Based on the energy decomposition analysis, the differences of residues in the binding site between MT and WT systems are detected. As the binding energy changed caused by V155M mutation, the affinity of agonist 2′3′-cGAMP with hSTING increased in the MT system, which might lead the conformation balance of hSTING to favor active style, resulting in the SAVI disease occurrence.
Residue interaction network
Active site mutations of a receptor directly affect the binding ability of its ligands.17–19 Recently, distant mutations in non-active site changing the bio-function of a protein was also identified. This kind of regulating mechanisms of distant mutation are defined allosteric regulation which have been widely researched.20–22 For example, Houk's team reported that the 15 Å distant mutation from active site of the natural enzyme called LovD can alter conformational dynamics of the catalytic residues to greatly increase its enzyme activity.23 Interestingly, V155M mutation is distant from the ligand binding pocket of hSTING and has been verified triggering the SAVI disease by clinically experiments.8 To better understand how V155M mutation remote from the ligand binding site can enhance the binding affinity of 2′3′-cGAMP with hSTING and lead hSTING to favoring active style, we compared the conformational ensembles of WT hSTING and MT hSTING in MD simulations (Fig. 7) and constructed correlation networks to investigate residual interactions of hSTING (Fig. 8 and ESI Fig. S6†).
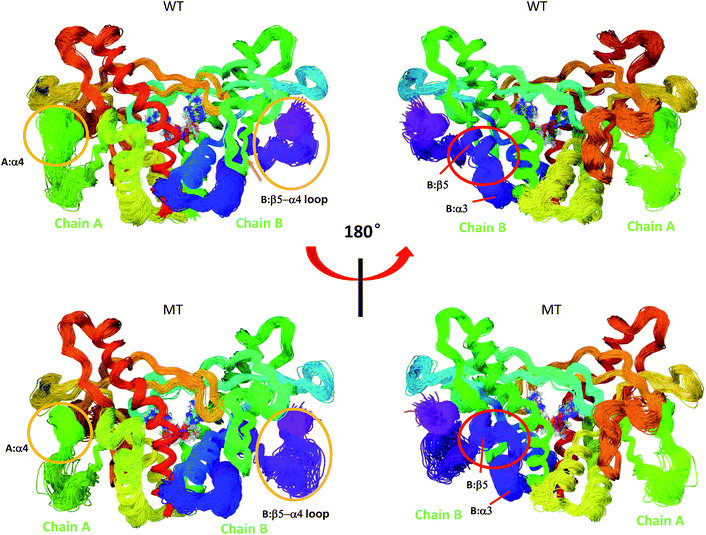 |
| Fig. 7 Superimposed conformational ensembles of the hSTING backbone illustrate the conformational space sampled in the last 50 ns MD simulations, including differences among WT hSTING and MT hSTING. Each conformation was extracted every 0.5 ns from the last 50 ns in MD simulations. Upper: Wild-type hSTING (WT); lower: mutated hSTING (MT). | |
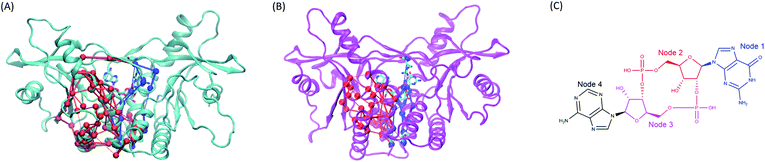 |
| Fig. 8 The comparison of the subset networks. (A) The red and blue subnetworks in wild type system. (B) The red and blue subset networks in V155M system. (C) The nodes assignment of 2′3′-cGAMP. | |
Overlays of sampled conformations provided a perspective of conformational fluctuations sampled in the final 50 ns simulations when both systems reached equilibrium state. As shown in Fig. 7, the largest differences in flexibility are seen in the A:α4 and B:β5–a4 loop as marked by orange circle which is consistent with the B-factors of hSTING in WT and MT systems. In addition, we detect the different conformations of the loop connecting α3 and β5 (residues 302–307) on chain B as marked by red circle in Fig. 7. Residues 302–307:B are more like helix structure in original hSTING than V155M variant, which can explain the RMSF result that the flexibility of residues 302–307:B in V155M system slightly increasing as shown in Fig. 2(B).
Moreover, the network analysis for the distant interactions between the residues 155:AB (both A and B chain) and residues 302–307:B implied why V155M mutation caused structural change of residues 302–307 in chain B. The red subnetwork starting at the residues 155:AB (both A and B chain) and ending at residues 302–307:B in wild-type hSTING system is more complicated than V155M system as shown in Fig. 8(A) and ESI Table S2.† The total number of paths between the residues 155:AB (both A and B chain) and residues 302–307:B are 127 in WT system and 34 in V155M system. As there are more links among nodes, complicated networks usually have stronger dynamic stability characteristics comparing with simple networks.21,23,24 It means residue V155 can communicate with the residues 302–307:B in multiple ways and those residues keep their relationship more stable. However, the red subset network between the residues 155:AB (both A and B chain) and residues 302–307:B is simple and the number of connections is decreasing in the V155M system, so residues 302–307:B become more variable which is reflected in increasing flexibility and losing the property of helix in structure.
On the other side, the network analysis for the distant interactions between the residues 155:AB (both A and B chain) and residue 238:B was also performed to explore the potential effects of V155M mutation on the affinity of ligand 2′3′-cGAMP with hSTING. The complexity and the number of connections of the blue subnetwork starting at residues 155:AB and ending with 238:B were similar in the MT and WT systems, but the ligand 2′3′-cGAMP displayed different communicating behaviors to residue ARG238:B in this subset network (Fig. 8 and ESI Table S3†). The molecule structure of 2′3′-cGAMP was defined as 4 nodes in the network analysis as shown in Fig. 8(C) and ESI Table S3.† Its node 1 and node 2 were involved in controlling the information that started from residues 155:AB flowing into ARG238:B in V155M system, while just node 2 took part in passing information to ARG238:B in wild type hSTING (Fig. 8 and ESI Video parts†). As more connections between residue 238:B and ligand 2′3′-cGAMP in MT system, their interaction was enhanced, which specifically embodied in more favorable binding energy and higher fraction of hydrogen bond between 2′3′-cGAMP and residue 238:B in the dynamic process as the results of binding energy decomposition and hydrogen bond analysis. Together, this V155M mutation which is around 17 Å (ESI Fig. S7†) away from the ligand can exert the effect on the binding site and modulate the conformation of substructure in hSTING. These changes caused by V155M mutation may be helpful to understand the mechanism of V155M causes SAVI disease.
Conclusions
The V155M mutation in hSTING can cause hSTING-associated vasculopathy with onset in infancy (SAVI), which is one of the autoinflammatory diseases.8 However, the mechanisms between the variant and SAVI are poorly explored. Molecular dynamics simulation is a powerful approach to research the structural and functional aspects of protein.25–27 Therefore, we used unbiased simulations to investigate the impact of V155M mutation on the conformational change of hSTING and the interaction between human hSTING and 2′3′-cGAMP. In this study, it was found that the V155M mutation might have a functional impact on the physiological agonist (2′3′-cGAMP) binding affinity. RMSF and PCA first revealed that the nature variant V155M increased the flexibility of B:β5–α4 loop which might make TBK1/IRF3 recruitment and activation easier.15 The transformation in residual flexibility eventually might change the conformational behavior of hSTING as observed from principal component analysis. Overall, the dynamic cross-correlation analysis suggested that the single point mutation (V155M mutation) could trigger the effect of enhancing almost all the residual correlated motions of hSTING and reserving the relationship of residual pairs' motion. Subsequently, we found that the V155M mutation strengthened the interactions of 2′3′-cGAMP with SER162:B, ARG238:B and THR263:B of hSTING based on the energy decomposition and hydrogen bond analysis. By residual network analysis, we further detected how distant mutation V155M affected the binding site and controlled the conformation change of substructure in hSTING. Above all, these findings in this study set the stage for elucidating the differences between hSTING and its nature variant V155M binding with 2′3′-cGAMP at atomic level, which provides a better understanding of the pathogenesis of hSTING-related SAVI induced by V155M mutation and a better strategy for developing new drugs targeting to SAVI disease. Certainly, for a more comprehensive understanding of the mechanism of V155M causing SAVI disease, the complete structure of hSTING including the transmembrane domain should be obtained by using X-ray diffraction or cryo-electron microscopy (cryoEM) and MD simulations maybe further used to explore the effect of this mutation on the transmembrane part of hSTING in our future work.
Methods and experiments
Initial structure choice
Selecting suitable structure from the PDB is very important for MD simulation study, because choosing unsuitable initial structure may need expensive computation to equilibrium state from unreasonable sate to reasonable state or lead nonsensical results. Nowadays, there are 19 crystal structures including monomer, dimer, mutated, wild type, ligand-bound and apo-state conformations of hSTING. Endogenous ligand 2′3′-cGAMP was the identified as the most affinity agonist for hSTING.5,6 Generally, the higher affinity of an agonist usually leads to the higher activation level of hSTING.5–7 As our study is to find out whether and how the 2′3′-cGAMP/hSTING binding interaction and the conformation of hSTING are affected by the single point mutation on residue 155 which is remote from the ligand binding site. So we searched the crystal structures with ligand 2′3′-cGAMP instead of other cyclic dinucleotides (CNDs). Only 4KSY and 4LOH meet this requirement.6,7 4KSY is the H232R mutant which can cause obvious conformational rearrangements of hSTING.3,6,7 Although 4LOH is the mutant S154, its conformation may be the most similar to the wild type hSTING/2′3′-cGAMP.7 Choosing unsuitable initial structure may need expensive computation to reach equilibrium state from unreasonable sate to reasonable state or lead nonsensical results. So we think that 4LOH used is currently the most suitable structure for our study.
Preparation for MD
The structure of hSTING homodimer with 2′3′-cGAMP (Fig. 1(A)) was acquired from the Brookhaven Protein Data Bank (PDB code: 4LOH) with sequence range 154–337 at 2.25 Å resolution.7 As the ligand has two poses, we chose the first conformation for our research. The detailed interaction diagram of the residues constructing the ligand binding site and 2′3′-cGAMP was plotted as Fig. 1(B) using maestro 10.1 software.28 Residue SER154 was mutated back to asparagine to get a wild type hSTING, as this crystal structure was a N154S variant. Then, V155M mutant was generated by using PYMOL software.29 All the above mutation processes were carried out on both chains. All hydrogen atoms were added by tleap integrated in AmberTools15. The Amber FF14SB force field was used for the receptor hSTING.30 The electrostatic potential of agonist 2′3′-cGAMP was calculated at B3LYP/6-31G* level using Gaussian 09 D software.31 To get the charge of 2′3′-cGAMP, we used the restrained electrostatic potential (RESP) method for charge fitting.32 And the force field of 2′3′-cGAMP were using with the general amber force field GAFF.33 Both MT and WT systems were neutralized by adding 12 Na+ ions and then solvated in a truncated octahedron box with TIP3P water molecules. The box volume was 487
430.61 Å3.
MD process
Molecular dynamics simulations of the V155M and wild type hSTING were using Amber14 suit.30 Energy minimization used steepest descent and conjugated gradient methods orderly.34,35 Then, two systems were gradually heated to 300 K for 200 ps using the Langevin thermostat method with the collision frequency 2 ps−1, and followed by 50 ps of density equilibration. Each system was equilibrated with unconstrained MD simulations for total 80 ns in an isothermal–isobaric (NPT) ensemble. To get more reliable results, we run parallel computations as Feig and Mirjalili suggested in this report.36 Each system was run 3 times with different initial velocities (ESI Fig. S8†). The total simulation time for these two systems is 480 ns. During the above steps, Particle Mesh Ewald (PME) method was used to treat long-range electrostatic interactions with a non-bonded cut-off 8 Å, while the SHAKE algorithm was chosen to constrain all covalent bonds between hydrogen and heavy atoms.37,38 Periodic boundary condition was also employed in the MD process. The time step was 2.0 fs. The trajectory coordinates and information were kept every 2 ps for results analysis in MD production state.
Dynamic cross-correlation analysis
In order to get meaningful results, translational and rotational motions were removed before the cross-correlation matrix calculation. To understand the correlations of the atomic motions in different domains, the dynamic cross-correlation analysis was carried out using the following equation: |
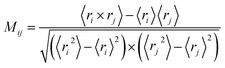 | (1) |
in which ri and rj indicated the value of the internal coordinates of Cα atom standing for number i and j residue positions. The angle bracket stand for an average over the sampled period. The value of Mij fluctuated between −1 and 1. Negative Mij values represented an anticorrelated motion between the i and j residue, while positive Mij values indicated a correlated motion.39
Principal components analysis (PCA) on MD simulations
To detect the principal models related to the conformational motions, the collective motions of WT and V155M hSTING were investigated by PCA.40,41 Rotational and translational motions were also removed prior to the covariance matrix calculation. The covariance matrix C for each element was calculated as the next equation: |
Cij = 〈(ri − 〈rj〉)(rj − 〈ri〉)〉
| (2) |
where ri and rj were the internal coordinates of atoms i and j. All analyses were performed with the CPPTRAJ module of AmberTools15. The covariance matrixes of Cα atoms' coordinates were diagonalized to yield the eigenvalues and eigenvectors. Each eigenvector represented a single correlated displacement of a collective atoms in a multidimensional space.
Binding free energy calculation
The method of Molecular Mechanics/Poisson Boltzmann Surface Area (MM/PBSA) was chosen to calculate binding free energies for hSTING and 2′3′-cGAMP by combining continuum solvation models and molecular mechanics calculations.42 The binding free energies ΔGbind was calculated as: |
ΔGbind = ΔH − TΔS = ΔEMM + ΔGsol − TΔS
| (3) |
|
ΔEMM = ΔEinternal + ΔEvdw + ΔEele
| (4) |
|
ΔGsol = ΔGnonpol + ΔGpol
| (5) |
In eqn (3), ΔEMM, ΔGsol and −TΔS were equivalent to the changes of the gas phase MM energy, the solvation free energy and the conformational entropy upon binding, respectively. In eqn (4), ΔEMM was the term including ΔEinternal (dihedral, angle and bond energies), van der Waals interaction ΔEvdw and electrostatic ΔEele energies. In eqn (5), ΔGsol was calculated as sum of the nonpolar (ΔGnonpol) and polar (ΔGpol) contribution.43 The nonpolar part of ΔGsol was estimated from the solvent accessible surface area (SASA) with γ = 0.0072 kcal mol−1 Å−2 for the surface tension. The polar part of ΔGsol was calculated by solving the linearized Poisson–Boltzmann equation.30 Both of ΔEMM and ΔGsol calculations were performed using all frames striped from the final 50 ns simulation. The conformational entropy change (−TΔS) upon ligand binding was computed with the NMODE program in AmberTools15 package.30 As the entropy calculations for large systems being exceedingly computationally expensive, only 250 snapshots were chosen with an interval of 200 ps from the 30–80 ns to calculate entropy change.
Free energy decomposition
The total, electrostatic, van der Waals interaction and solvation energies between ligand 2′3′-cGAMP and receptor hSTING were computed based on the Amber force field equation.44 Each energy component was estimated by using the same snapshots in above calculation processes of ΔEMM and ΔGsol.
Hydrogen bond analysis
All hydrogen bonds were defined as that angle cutoff for hydrogen bonds were over 135° and distance cutoff for hydrogen bonds were no more than 3.5 Å. As reported in this literature,18 the percentage of a hydrogen bond in MD runs was computed as: |
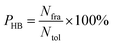 | (6) |
here, PHB the fraction of every specific hydrogen bond was calculated within range 0–100%. Nfra meant the number of frames that one specified hydrogen bond emerged. Ntol was the total figure of collected frames in 30–80 ns.
Residual interaction network
Network theory has provided useful strategies for thinking about and analyzing biological system in recent years.21,23,24,27 In the residual network of hSTING/2′3′-cGAMP system, each amino acid residue was assigned to one node and was centered Cα atom. Ligand 2′3′-cGAMP was assigned 4 nodes (Fig. 8(C) and Table S3†). Edges were draw between nodes whose heavy atoms within a cutoff distance 4.5 Å for at least 75 percent of the simulation time. All the network models were built using the NetworkView plugin of VMD.24,45
Author contributions
Bowen Tang and Baicun Li carried out experiments, analyzed experimental results and drafted the manuscript. Boqun Li and Yingkun Qiu proposed the protein (hSTING). Zan Li, Jingbo Qin and Xiaoxiao Zhou prepared the manuscript and revised the literature sources. Zhen Wu and Meijuan Fang supervised whole research project, revised manuscript and did manuscript final version approval. All authors read and approved the manuscript.
Conflicts of interest
The authors declare no conflict of interest.
Acknowledgements
This research was conducted by using the computational resources of the School of Pharmaceutical Sciences and the College of Chemistry and Chemical Engineering, Xiamen University. The work was supported by the National Natural Science Foundation of China (No. 81302652 and No. 81273400). This research was also financially supported by Fujian Science and Technology project (No. 2014N5012).
References
- Y. Tanaka and Z. J. Chen, Sci. Signaling, 2012, 5, ra20 CrossRef PubMed.
- G. N. Barber, Curr. Opin. Immunol., 2011, 23, 10–20 CrossRef CAS PubMed.
- P. Gao, M. Ascano, T. Zillinger, W. Wang, P. Dai, A. A. Serganov, B. L. Gaffney, S. Shuman, R. A. Jones, L. Deng, G. Hartmann, W. Barchet, T. Tuschl and D. J. Patel, Cell, 2013, 154, 748–762 CrossRef CAS PubMed.
- X.-D. Li, J. Wu, D. Gao, H. Wang, L. Sun and Z. J. Chen, Science, 2013, 341, 1390–1394 CrossRef CAS PubMed.
- J. Wu, L. Sun, X. Chen, F. Du, H. Shi, C. Chen and Z. J. Chen, Science, 2013, 339, 826–830 CrossRef CAS PubMed.
- X. Zhang, H. Shi, J. Wu, X. Zhang, L. Sun, C. Chen and Z. J. Chen, Mol. Cell, 2013, 51, 226–235 CrossRef CAS PubMed.
- P. J. Kranzusch, S. C. Wilson, A. S. Lee, J. M. Berger, J. A. Doudna and R. E. Vance, Mol. Cell, 2015, 59, 891–903 CrossRef CAS PubMed.
- Y. Liu, A. A. Jesus, B. Marrero, D. Yang, S. E. Ramsey, G. A. Montealegre Sanchez, K. Tenbrock, H. Wittkowski, O. Y. Jones, H. S. Kuehn, C. R. Lee, M. A. DiMattia, E. W. Cowen, B. Gonzalez, I. Palmer, J. J. DiGiovanna, A. Biancotto, H. Kim, W. L. Tsai, A. M. Trier, Y. Huang, D. L. Stone, S. Hill, H. Jeffery Kim, C. St. Hilaire, S. Gurprasad, N. Plass, D. Chapelle, I. Horkayne-Szakaly, D. Foell, A. Barysenka, F. Candotti, S. M. Holland, J. D. Hughes, H. Mehmet, A. C. Issekutz, M. Raffeld, J. McElwee, J. R. Fontana, C. P. Minniti, S. Moir, D. L. Kastner, M. Gadina, A. C. Steven, P. T. Wingfield, S. R. Brooks, S. D. Rosenzweig, T. A. Fleisher, Z. Deng, M. Boehm, A. S. Paller and R. Goldbach-Mansky, N. Engl. J. Med., 2014, 371, 507–518 CrossRef CAS PubMed.
- J. Ahn and G. N. Barber, Curr. Opin. Immunol., 2014, 31, 121–126 CrossRef CAS PubMed.
- J. Ahn, D. Gutman, S. Saijo and G. N. Barber, Proc. Natl. Acad. Sci. U. S. A., 2012, 109, 19386–19391 CrossRef CAS PubMed.
- N. Dobbs, N. Burnaevskiy, D. Chen, V. K. Gonugunta, N. M. Alto and N. Yan, Cell Host Microbe, 2015, 18, 157–168 CAS.
- J. Petrasek, A. Iracheta-Vellve, T. Csak, A. Satishchandran, K. Kodys, E. A. Kurt-Jones, K. A. Fitzgerald and G. Szabo, Proc. Natl. Acad. Sci. U. S. A., 2013, 110, 16544–16549 CrossRef CAS PubMed.
- S. Ouyang, X. Song, Y. Wang, H. Ru, N. Shaw, Y. Jiang, F. Niu, Y. Zhu, W. Qiu and K. Parvatiyar, Immunity, 2012, 36, 1073–1086 CrossRef CAS PubMed.
- J. Wu and Z. J. Chen, Annu. Rev. Immunol., 2014, 32, 461–488 CrossRef CAS PubMed.
- Q. Yin, Y. Tian, V. Kabaleeswaran, X. Jiang, D. Tu, M. J. Eck, Z. J. Chen and H. Wu, Mol. Cell, 2012, 46, 735–745 CrossRef CAS PubMed.
- G. Shang, D. Zhu, N. Li, J. Zhang, C. Zhu, D. Lu, C. Liu, Q. Yu, Y. Zhao, S. Xu and L. Gu, Nat. Struct. Mol. Biol., 2012, 19, 725–727 CAS.
- P. Kim, J. Zhao, P. Lu and Z. Zhao, Nucleic Acids Res., 2017, 45, D256–D263 CrossRef PubMed.
- M. Liu, L. Wang, X. Sun and X. Zhao, Sci. Rep., 2014, 4, 5095, DOI:10.1038/srep05905.
- A. B. Bleecker, M. A. Estelle, C. Somerville and H. Kende, Science, 1988, 241, 1086–1090 CAS.
- A. Allain, I. C. de Beauchêne, F. Langenfeld, Y. Guarracino, E. Laine and L. Tchertanov, Faraday Discuss., 2014, 169, 303–321 RSC.
- U. Doshi, M. J. Holliday, E. Z. Eisenmesser and D. Hamelberg, Proc. Natl. Acad. Sci. U. S. A., 2016, 113, 4735–4740 CrossRef CAS PubMed.
- R. Appadurai and S. Senapati, Biochemistry, 2016, 55, 1529–1540 CrossRef CAS PubMed.
- G. Jiménez-Osés, S. Osuna, X. Gao, M. R. Sawaya, L. Gilson, S. J. Collier, G. W. Huisman, T. O. Yeates, Y. Tang and K. Houk, Nat. Chem. Biol., 2014, 10, 431–436 CrossRef PubMed.
- A. Sethi, J. Eargle, A. A. Black and Z. Luthey-Schulten, Proc. Natl. Acad. Sci. U. S. A., 2009, 106, 6620–6625 CrossRef CAS PubMed.
- M. Karplus and J. A. McCammon, Nat. Struct. Mol. Biol., 2002, 9, 646–652 CAS.
- B. Tang, B. Li, Y. Qian, M. Ao, K. Guo, M. Fang and Z. Wu, RSC Adv., 2017, 7, 17193–17201 RSC.
- V. C. Nibali and M. Havenith, J. Am. Chem. Soc., 2014, 136, 12800–12807 CrossRef PubMed.
- Schrödinger Release 2015-1: Maestro, Schrödinger, LLC, New York, NY, 2015 Search PubMed.
- W. L. DeLano, The PyMOL Molecular Graphics System, 2002 Search PubMed.
- D. A. Case, J. T. Berryman, R. M. Betz, D. S. Cerutti, T. E. Cheatham III, T. A. Darden, R. E. Duke, T. J. Giese, H. Gohlke and A. W. Goetz, AMBER 2015, University of California, San Francisco, 2015 Search PubMed.
- M. Frisch, G. Trucks, H. B. Schlegel, G. Scuseria, M. Robb, J. Cheeseman, G. Scalmani, V. Barone, B. Mennucci and G. Petersson, Gaussian 09, Revision D. 01, Gaussian, Inc., Wallingford, CT, 2009, p. 200 Search PubMed.
- C. I. Bayly, P. Cieplak, W. Cornell and P. A. Kollman, J. Phys. Chem., 1993, 97, 10269–10280 CrossRef CAS.
- J. Wang, R. M. Wolf, J. W. Caldwell, P. A. Kollman and D. A. Case, J. Comput. Chem., 2004, 25, 1157–1174 CrossRef CAS PubMed.
- R. Fletcher and M. J. Powell, Comput. J., 1963, 6, 163–168 CrossRef.
- R. Fletcher and C. M. Reeves, Comput. J., 1964, 7, 149–154 CrossRef.
- M. Feig and V. Mirjalili, Proteins: Struct., Funct., Bioinf., 2016, 84, 282–292 CrossRef PubMed.
- T. Darden, D. York and L. Pedersen, J. Chem. Phys., 1993, 98, 10089–10092 CrossRef CAS.
- J.-P. Ryckaert, G. Ciccotti and H. J. Berendsen, J. Comput. Phys., 1977, 23, 327–341 CrossRef CAS.
- P. H. Hünenberger, A. E. Mark and W. F. vanGunsteren, J. Mol. Biol., 1995, 252, 492–503 CrossRef PubMed.
- M. A. Balsera, W. Wriggers, Y. Oono and K. Schulten, J. Phys. Chem., 1996, 100, 2567–2572 CrossRef CAS.
- S. Haider, G. N. Parkinson and S. Neidle, Biophys. J., 2008, 95, 296–311 CrossRef CAS PubMed.
- B. R. Miller, T. D. McGee Jr, J. M. Swails, N. Homeyer, H. Gohlke and A. E. Roitberg, J. Chem. Theory Comput., 2012, 8, 3314–3321 CrossRef CAS PubMed.
- D. Eisenberg and A. D. McLachlan, Nature, 1985, 319, 199–203 CrossRef PubMed.
- V. Hornak, R. Abel, A. Okur, B. Strockbine, A. Roitberg and C. Simmerling, Proteins: Struct., Funct., Bioinf., 2006, 65, 712–725 CrossRef CAS PubMed.
- J. Eargle and Z. Luthey-Schulten, Bioinformatics, 2012, 28, 3000–3001 CrossRef CAS PubMed.
Footnotes |
† Electronic supplementary information (ESI) available. See DOI: 10.1039/c7ra05959k |
‡ The first two authors are co-first authors. |
|
This journal is © The Royal Society of Chemistry 2017 |
Click here to see how this site uses Cookies. View our privacy policy here.