DOI:
10.1039/C7RA05918C
(Review Article)
RSC Adv., 2017,
7, 47416-47424
Brain grants permission of access to Zika virus but denies entry to drugs: a molecular modeling perspective to infiltrate the boundary†
Received
26th May 2017
, Accepted 19th September 2017
First published on 9th October 2017
Abstract
The magnetism of the Zika virus to neuronal cells proves to be one of the major concerns in the development of effective inhibitors. Although the blood–brain barrier limits the entry of most drugs, tailored small molecule inhibitors and drug delivery systems are currently being designed to overcome this obstacle. We have identified the core challenge to be addressed – blood–brain barrier permeability – and provided insight into strategies that can be used to improve drug delivery to the brain. We have compiled drugs that have previously been proposed as potential Zika virus inhibitors and classified chemical features of those drugs, which influence blood–brain barrier permeability. Thereafter, we created a route map to design drugs with improved blood–brain barrier permeability. An alternative approach using drug delivery systems to transport membrane-impermeable Zika virus inhibitors to the brain is also proposed, along with descriptions of known drug carriers. This review provides information for further research toward inhibitors of Zika virus.
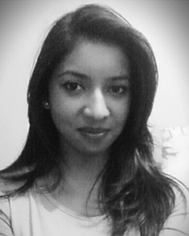 Nikita Devnarain | Nikita Devnarain obtained her BSc Biomedical Science degree in 2013 and graduated with her Honours and Masters in Medical Biochemistry and Chemical Pathology at UKZN by the end of 2016. She is now a Ph.D. student in Prof Mahmoud E. S. Soliman's Molecular Bio-computation and Drug Design Laboratory at the Department of Pharmaceutical Sciences. Her current research directions include in silico design and exploration of inhibitors against the Zika virus. |
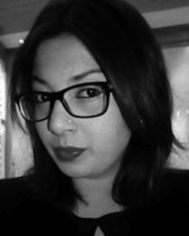 Pritika Ramharack | Pritika Ramharack currently holds a postdoctoral position at the Molecular Bio-computation and Drug Design Laboratory, Department of Pharmaceutical Sciences, University of Kwa-Zulu Natal, South Africa. Her current research concentrates on the Design of Inhibitors against numerous infectious diseases including HIV and Tuberculosis. |
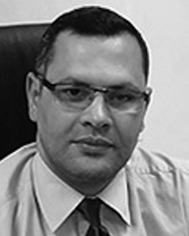 Mahmoud E. Soliman | Prof. Mahmoud Soliman (B. Pharm., M. Pharm., MPhil/PhD) is the Dean and Head of School of Health Sciences, UKZN, Westville Campus, and the Head and principal investigator of Molecular Bio-computation and Drug Design Laboratory. Soliman has joined the School in 2012 after completing his postgraduate degrees (MPhil/PhD – Oct. 2009) at the University of Bath, United Kingdom in field of Molecular Modeling, computational chemistry and drug design, under supervision of Prof. Ian Williams. Prof. Soliman is Editor of Journal of Cell Biochemistry and Biophysics (Springer), Editor-in-Chief of the journal of Organic and Bimolecular Simulations (JOBS), editor of Journal of Pharmaceutical Chemistry, Journal of Drug Design and Research and International Journal of Molecular Biology and Medicine. Soliman is also academic visitor at the University of Bath, UK, collaborating with Prof. Ian Williams' lab. Prof. Soliman is a C-rated NRF researcher. Soliman's research mainly focuses on studying of biomolecular systems and drug–protein interactions at molecular level. |
The tale of Zika virus
The Zika virus (ZIKV), a mosquito-borne virus, belongs to the Flaviviridae family and has similar characteristics to other flaviviruses such as Dengue virus, West Nile virus and Japanese Encephalitis virus.1 The rapid disseminating potential and repercussion in humans are attributed to the various modes of transmission, primarily through the bite of an infected Aedes aegypti mosquito.2 The ZIKV is also transmitted through sexual intercourse,3 blood transfusions4 and from a mother to child perinatally.5
The ZIKV was originally isolated in Uganda in the Zika forest in 1947.6 For nearly 7 decades thenceforth, sporadic infections caused by ZIKV were reported in several countries worldwide. These include more equatorial countries of Africa such as Tanzania, Egypt, Kenya, Nigeria, Central African Republic, and Gabon; some Asian countries including Pakistan, India, Malaysia, Thailand, the Philippines and Indonesia; and many islands in the Pacific Ocean.7–10 The most devastating and highly publicized outbreak that captured the world's attention occurred in Brazil in 2015, which triggered global panic as it rapidly spread across America.11 In 2016, the ZIKV broadened its geographic spectrum to North American Florida and Texas where the infection was locally transmitted.10
There has been prior ambiguity regarding the diagnosis of ZIKV, as its infection manifests similarly to common colds and other flavivirus infections.12 These symptoms include fever, headaches, conjunctivitis, joint pain, muscle pain and skin rash.13,14 The speculation of ZIKV infection depends on its manifestation and history of mosquito bites.12 The ZIKV is detectable in bodily fluids such as saliva,15 semen,16,17 urine18 and amniotic fluid,19 which can be verified in the laboratory.20 The various modes of transmission of ZIKV make the human body highly susceptible to infection. When ZIKV enters the body through an infected female mosquito bite, the infection manifests as a rash in the vicinity of the bite.9 This occurs due to the release of virions into dermal and epidermal layers of the skin, where ZIKV is introduced to the bloodstream and advances to the lymph nodes to replicate and cause viremia.21
The ZIKV is an enveloped icosahedral virus that is made up of a single-stranded, positive-sense genome. The enveloped virion comprises of an 11 kilobase genome consisting of 10
794 nucleotides encoding 3419 amino acids.22 The open reading frame (ORF) of the 5′ and 3′ untranslated region (UTR) encodes a polyprotein that is cleaved into three structural proteins, being the capsid (C), precursor membrane (prM), and envelope (E).23 Seven non-structural (NS) proteins are also found in this assembly, namely, NS1, NS2a, NS2b, NS3, NS4a, NS4b, and NS5 (largest viral protein).24 The genomic protein organization is 5′-C-prM-E-NS1-NS2a-NS2b-NS3-NS4a-NS4b-NS5-3′25 and contains an m7gpppAmpN2 at the 5′ end and lacks a poly-A tail at the 3′ end.26 A highly conserved 90–120-nucleotide strand is situated close to the 3′end that develops into a hairpin loop and is vital for replication.26,27 Of the non-structural proteins, NS1, NS3 and NS5 are highly conserved whereas the NS2a, NS2b, NS4a and NS4b are small and hydrophobic.24 Of critical importance is the proteolytic cleavage of prM to produce the pr and M protein by furin-like protease located in the trans-Golgi network during the egress of the particles as this promotes maturation of virions.28
The ramifications of ZIKV infections have heavily impacted thousands worldwide, particularly in newborns, since ZIKV-infected pregnant women have given birth to babies with congenital brain abnormalities, predominantly microcephaly and intracranial calcification.13 The ZIKV infection has also been shown to elicit Guillain–Barré Syndrome, which ultimately advances to paralysis and death.29
Studies have shown tropism of the ZIKV for cells of the nervous system, whereby entry into neuronal and endothelial cells occur via AXL receptors situated on the cell surface.30–32 The ZIKV has also affected retinal cells that line the blood-retinal barrier (i.e. retinal pigment epithelium and retinal endothelium) in mice, which also express AXL receptors. This presents as conjunctivitis.33
Blood–brain barrier permeability as a core challenge in ZIKV therapy
Treating the symptoms of ZIKV will not yield permanent results; hence nipping the cause at the bud may be the best route to a solution. The blood–brain barrier (BBB) and placental barrier are surrounded by lipophilic membranes and junctions, through which only certain compounds can permeate.34 It has been shown that the placenta is permissive to most drugs as it serves to allow for the exchange of nutrients for its biological purpose.35 The ZIKV can penetrate these membranes, which is evident by its downstream pathogenic effects in fetal nervous systems.13,26,29,30
The specific characteristics of a compound govern the method by which it is transported across the BBB, or whether or not it is transported at all. Compounds that have surface hydrogen bonds (hydrophilic compounds) are only permissive through tight junctions of the BBB, which ultimately serve to prevent the passage of molecules between cells of the endothelium. These hydrophilic compounds are impermeable through the lipophilic endothelium and require lipid-mediated transport in order to permeate transcellularly. Large molecules, such as insulin and transferrin, require receptors, whereas small molecules require carrier-mediated transport to move across the barrier. There is also active efflux transport for endogenous BBB transporters (Fig. 1 – BBB).34,36–38
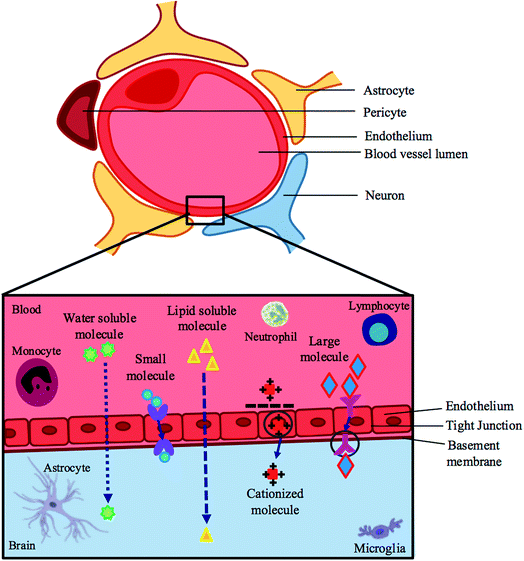 |
| Fig. 1 A schematic representation of the blood–brain barrier and pathways across this barrier. | |
Bioavailability features of screened drugs as prospective ZIKV inhibitors
There are FDA-approved drugs that have been proposed as anti-ZIKV drugs based on their diverse antiviral/antimicrobial/antibacterial activities in diseases other than ZIKV,39 however, the ability of those drugs to pass the BBB, their properties as hydrophilic/lipophilic compounds, and their ability to be absorbed by the gastrointestinal tract (GIT) have not yet been clearly elucidated. To this effect, we have taken a step further and utilized a chemical data base, PubChem, along with ADME prediction tools, Swiss ADME40 to predict specific characteristics of these candidate anti-ZIKV drugs and to verify whether or not the proposed compounds by Barrows et al. (2016) could be potential anti-ZIKV drugs with BBB-permeable profiles. SWISS ADME is a “website allows you to compute physicochemical descriptors as well as to predict ADME parameters, pharmacokinetic properties, druglike nature and medicinal chemistry friendliness of one or multiple small molecules to support drug discovery”.40 The proposed compounds are listed in Table 1.
Table 1 Predicted bioavailability features of prospective anti-ZIKV inhibitors
Drug name |
Lipid solubility (log P) |
BBB permeation |
GIT absorption |
Auranofin |
0.00 |
No |
High |
Azathioprine |
0.72 |
No |
Low |
Bortezomib |
0.00 |
No |
High |
Clofazimine |
4.72 |
No |
Low |
Cyclosporine A |
6.16 |
No |
Low |
Dactinomycin |
5.33 |
No |
Low |
Daptomycin |
0.79 |
No |
Low |
Deferasirox |
2.48 |
No |
High |
Digoxin |
4.69 |
No |
Low |
Fingolimod |
3.76 |
Yes |
High |
Gemcitabine HCl |
0.00 |
No |
High |
Ivermectin |
6.31 |
No |
Low |
Mebendazole |
1.27 |
No |
High |
Mefloquine HCl |
0.00 |
No |
Low |
Mercaptopurine hydrate |
0.47 |
No |
High |
Methoxsalen |
2.22 |
Yes |
High |
Micafungin |
−0.72 |
No |
Low |
Mycophenolate mofetil |
3.67 |
No |
High |
Mycophenolic acid |
2.38 |
No |
High |
NITD008 |
1.30 |
No |
Low |
Palonosetron HCl |
0.00 |
Yes |
High |
Pyrimethamine |
2.15 |
Yes |
High |
Sertraline |
3.40 |
Yes |
High |
Sofosbuvir |
3.05 |
No |
Low |
Sorafenib tosylate |
3.84 |
No |
Low |
Thioguanine |
0.14 |
No |
High |
The partition coefficient (log
P) measures how hydrophilic or hydrophobic a molecule is. The desired log
P value of a molecule likely to permeate lipophilic membranes should lie between 0–5.41 The ability of a drug to pass through the BBB is influenced by their unique but varying properties.36 In Table 1, ∼80% of the drugs described have the ability to pass through lipophilic membranes, however, less than 20% of those drugs can penetrate the BBB.40 Efficient GIT absorption of orally administered drugs is required for entry into the bloodstream and sufficient drug delivery,42 although, just half the drugs mentioned are highly absorbed via the GIT. The results of this table suggest that the ability of a compound to pass through the BBB depends on factors additional to lipophilicity. From this exercise we highlight 5 compounds that are predicted to pass the BBB and the hydrophobic spots of each compound are shadowed in yellow in Fig. 2.
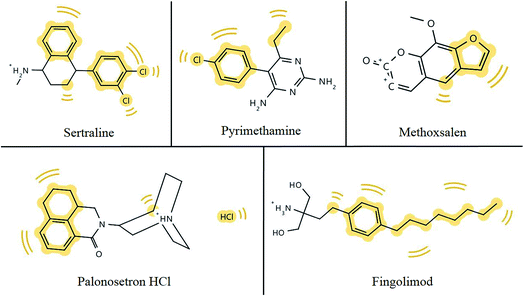 |
| Fig. 2 Hydrophobic footprints (highlighted in yellow) in chemical structures of potential anti-ZIKV compounds which have the ability to permeate the BBB. | |
Hydrophobic groups of compounds are required for hydrophobic interactions with target molecules. Hydrophobic interactions are comparably stronger than some weak intermolecular forces, such as hydrogen bonds or van der Waals interactions, and ensure protein–ligand complexes remain stable and biologically active.43 As depicted in Fig. 2, all five compounds from Table 1 that have the ability to pass the BBB bear hydrophobic groups and therefore, possess the potential to form hydrophobic interactions with target molecules. Although, fingomolid and sertraline have more hydrophobic spots than the other compounds in Fig. 2, and therefore, are more likely to form stronger hydrophobic interactions.
Systematic approach to tackle the challenge
To overcome the hurdle faced by most drug therapies, we are presenting two main strategies that could potentially assist with the design and bioavailability of compounds with an improved BBB permeability profile. Furthermore, we include an approach that relies on the pre-existing BBB-impermeable drugs conjugated to drug delivery systems. These strategies are: (1) improve the inhibitor and (2) carry the cargo.
Improve the inhibitor
In this approach, in silico tools may be used to model and optimize potential compounds (Fig. 3), followed by compound synthesis and biological testing. Phase 1 includes targeted selection of potential anti-ZIKV compounds. This incorporates screening for potential compounds with specific physicochemical properties and antiviral activities using chemical databases, and the use of absorption, distribution, metabolism, excretion (ADME) prediction tools such as Swiss ADME, in order to filter out compounds that encompass the ability to pass the BBB. The ability of a compound to pass through the BBB is governed by a function of lipophilicity, the molecular characteristics of charged and hydrophobic residues of the compound as well as molecular weight.37 The main lipophilic properties that must be considered include the Hansch constant (π), hydrophobic fragmental constant (f), log
P, capacity factor values from RP-HPLC (log
kw), calculated log
P values (CLOGP) and molecular lipophilic potential (MLP).44 With regard to the charge of the compound, only uncharged molecules can diffuse across the membrane to become reprotonated once it leaves the membrane and enters the brain fluid.37 As the size of a compound gets larger, its ability to permeate the BBB decreases.45
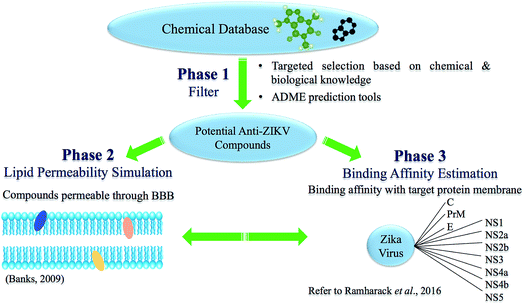 |
| Fig. 3 Phases involved in the suggested approach to improve the BBB-permeability profile of the inhibitor. | |
Phase 2 involves the prediction of lipid permeability of the potential anti-ZIKV compounds using molecular dynamic simulations and 3D-modelled lipid bilayer simulations. Due to the surrounding lipid membrane in the BBB, it is necessary to assess compound interactions with the target enzyme within lipid membrane.46
Phase 3 involves the estimation of binding affinities between potential compounds, which pass through the BBB, and viral enzymes. This may be achieved via binding free energy calculations or molecular docking of the compound of interest into the active site of the target enzyme.47
Following the design process, the compounds must be synthesized for further testing. Synthesis of the compound involves construction of the carbon framework and the addition/deletion/transformation of functional groups for functionality of compound. Validation of synthesis is carried out by ligand-binding assays which involves placing the target enzyme and compound of interest into a solvent (e.g. water) to allow the compound to interact with the active site residues of the enzyme.48 Binding studies provide reliable assessments of binding affinities, errors and binding mode.48 Further studies including biological testing will investigate toxicity and efficacy of the compound, including in vitro studies (cellular level), in vivo studies (organism level) and ultimately clinical trials. Microscale thermophoresis (MST) may also be used in order to analyze the interaction between the inhibitors and receptors, experimentally, which is established on the controlled movement of particles along a temperature gradient.49
Carry the cargo
An alternate to creating new BBB-permeable drugs will involve the utilization of drug carriers that have already been successful in delivering drugs to the brain. This approach eliminates the additional time and expense required to design and formulate new drugs and drug delivery systems. A drug carrier could be used to transport a BBB-impermeable drug to the brain and allow for the drug to carry out its function against ZIKV.
There are existing drugs that have been proven to inhibit ZIKV replication in isolated ZIKV infected cells, such as NITD008 (ref. 50) and sofosbuvir,51 however the drugs cannot pass the BBB to counteract the virus (Table 1). With half the battle won due to their ability to inhibit ZIKV, ultimate triumph over ZIKV would entail overcoming impermeability, which requires the potential ZIKV inhibitors to be transported across the BBB.
Previously utilized membrane-permeable drug delivery approaches have been successful in transporting membrane-impermeable drugs across the BBB for other infections and diseases, such as Parkinson's disease and Alzheimer's disease.52–55 Some known drug delivery systems used in various disease cases include polymers and polymeric nanoparticles such as micro/nanospheres, micro/nanocapsules, dendrimers, liposomes, hydrogels, gold nanoparticles, micelles; others include lipoproteins and aptamers (Table 2).54,56
Table 2 Pre-existing drug delivery systems and their principle roles in disease and virus therapies
Drug delivery system |
Description |
Disease/viral target |
References |
Dendrimer |
• Hyperbranched, monodispersed, water soluble (1–100 nm) macromolecule |
• Inhibitors of haemagglutinin of human erythrocytes by Influenza virus |
56 and 57 |
• Encapsulated drug in its interior or adsorbs drug on and conjugates to its surface groups |
• Amino groups of dendrimer react with nucleic acid phosphate groups to form transfection complexes |
• Releases free 5-fluorouracil upon hydrolysis |
• Intracellular delivery of poorly soluble drugs |
• Carriers in gene therapy |
Microsphere & nanocapsule |
• Microsphere – drug is dispersed within polymer throughout particle |
• Entraps luteinizing hormone-releasing hormone in prostate cancer |
57 and 58 |
• Nanocapsule-cavity contains drug surrounded by polymer membrane |
• Drug release through diffusion through polymer or degradation of polymer |
Liposomes |
• Amphiphilic vesicular structures made up of cholesterol & phospholipids |
• Facultative intracellular bacteria-mediated infections |
56, 59 and 60 |
• Core suited for hydrophilic drug delivery |
• Parasites (e.g. Leishmania) |
• Phospholipid membrane encapsulate hydrophobic drugs |
• Viruses |
• Systemic fungal diseases in cancer |
• Melanomas |
Micelles |
• Core comprised of hydrophobic polymers |
• Targets tumour sites in cancer by active/passive mechanisms |
56, 61 and 62 |
• Shell comprised of hydrophilic polymers |
• Suitable for drugs with poor solubility |
• Nanosize; in vivo endurance; remains stable in plasma |
• Delivery of drugs & small interfering RNA |
Hydrogel |
• Network of natural/synthetic hydrophilic polymers that are cross-linked |
• Local & systemic diseases |
56, 63 and 64 |
• Highly absorbent, biodegradable, high porosity, biocompatible |
• Oral delivery of insulin in diabetes; salmon calcitonin for postmenopausal osteoporosis; growth hormone for decelerated/stunted growth-associated diseases |
• Swell rapidly in aqueous solutio006E |
• Used in oral & topical drug delivery |
Gold/silver nanoparticle |
• Low toxicity; high specificity & selectivity |
• Selective damage of tumour cells |
65–68 |
• Easily controlled & modifiable |
• Certain infectious & dermal diseases |
• High ratio of surface area:amount |
• H1N1, H3N2, H5N1 Influenza A virus |
• Conjugation of proteins on colloidal gold nanoparticles occurs via electrostatic interactions between citrate (−) on surfaces of gold nanoparticles & groups (+) on proteins |
• Herpes simplex virus |
• Effortless cellular penetration |
• Human immunodeficiency virus |
• Hepatitus B virus |
• Metapneumovirus |
• Respiratory syncytial virus |
Lipid nanoparticle |
• 10–1000 nm |
• Humoral immunity against Ebola infection |
56 and 69–72 |
• Carriers with dispersed melted lipid in surfactant |
• Silencing of hepatitis C virus replication |
• Colloidal system with hydrophobic core that encloses drug & surface coated with hydrophilic polymers |
• Gene therapy |
Aptamer |
• Short, single-stranded (ss) DNA or RNA that have definitive 2° & 3° structures that strongly bind to specific target proteins |
• α-Thrombin in thrombosis |
73–77 |
• Low immunogenicity & toxicity |
• PTK7 and nucleoin in cancer |
• Variety of targets & modifiable chemical structure |
• IGHM in lymphoma |
• VEGF in age-related macular degeneration |
• A1 domain of vWF in thrombotic microangiopathies & carotid artery disease |
• Neutralizes r5 strains of HIV-1 |
• Blocks gp120-CCRF interaction |
The inhibitor–carrier approach will involve techniques similar to the previous suggested approach. The first step would be to create an inhibitor–carrier complex by binding the known ZIKV inhibitor to the carrier. The complex must then be simulated to analyze the trajectory of the complex as well as the potential energy of the entire complex through a lipid bilayer. Once there is computational evidence to show that the inhibitor can theoretically bind to the carrier and move through a lipid membrane, the inhibitor–carrier complex must be synthesized to test it biologically (e.g. in vivo testing), which will be used to confirm drug delivery through the BBB to the brain. Examples of computationally docking a compound–polymer complex are provided in the ESI.†
Technical guidance
A number of tools are available which can be utilized to screen for compounds on chemical databases based on a set of criteria. Structure-based virtual screening will allow searching through combinatorial chemistry libraries for compounds that may be potential inhibitors of a target protein and will rapidly dock them into the 3D target's active pocket.47,78 Screening for potential compounds can be carried out on ZINC Database79 or ZincPharmer.80 Several molecules may have the potential to bind to the active site of the protein; therefore, the free binding energy of every pose is calculated. Binding affinity estimations may be carried out using molecular docking approaches and free binding energy calculations. This will generate a scoring function to rank the ligands that best suit the target protein.47 Computational software that can be used to calculate binding affinities include UCSF Chimera81 and AutoDock Vina.82 Protein–ligand complexes of lowest free binding energy may be used as inhibitor candidates, which may subsequently be validated via molecular dynamic simulations, as binding affinity predictions may not be one hundred percent accurate.78,83–86
Molecular dynamic simulations calculate the potential energy of a system and analyze free binding energies and binding modes between compounds and enzymes.87 Force fields are used to calculate potential energies of particles and electrostatic forces that occur between atoms in a system.88 Some force fields that can be used for molecular dynamic simulations include NAMD,89 Gromacs,90 Amber91 and Charrm.92 Complexes can also be simulated through lipid bilayer, in cases where potential compounds need to enter certain target tissues, which are surrounded by lipophilic membranes. This can give a prediction as to whether or not the potential compound will be able to pass through the lipid membrane or not. Softwares that can be used to generate a 3D lipid bilayer model include CHARRM-GUI and Visual Molecular Dynamics (MEMBPLUGIN).46,93 A brief background and the applications of the methods that have been mentioned above are shown in Table 3.
Table 3 Various molecular modeling approaches in drug discovery
|
Description/application |
Reference |
Molecular mechanics (MM) calculations |
Molecular systems are modeled using MM, which employs force fields to calculate a system's potential energy. MM may be used to analyze molecular systems of various sizes and complexity, and is the preferred approach for the simulation of proteins |
25, 94 and 95 |
Quantum mechanics (QM/MM) calculations |
The hybrid QM/MM method is a molecular dynamic simulation approach that integrates the accuracy of QM and speed of MM, to allow for chemical processes within proteins and solutions to be studied. QM/MM provides enhanced mechanistic aspects and binding energies of the interaction between a ligand and receptor |
66, 94 and 96 |
Virtual screening |
Virtual screening involves screening through databases for compounds with similar features. There are two types of approaches involved in virtual screening: (1) ligand or pharmacophore-based virtual screening which requires knowledge of an active ligand, and (2) structure-based virtual screening which relies on a known 3D structure of the protein |
79, 83, 84, 86 and 97 |
Molecular docking |
Molecular docking is utilized for: (1) studying potential ligand conformations within a protein's binding site and (2) approximation of the force of ligand–protein interactions. During docking, Cartesian coordinates of a separate ligand and receptor are utilized to anticipate the conformation of the ligand that fits into the binding site of the protein the best, producing a ligand–protein complex. Molecular docking uses a scoring function, which ranks each docking “pose” according its binding energy |
47, 78, 87 and 97 |
Molecular dynamics |
Influential tool for analyzing energetics, mechanisms and dynamics of proteins and ligand–protein complexes. Molecular dynamics provide a perspective of the interaction between a ligand and protein at a molecular level, presenting an improved understanding of the experimental conclusions. These are effective in the identification of specific biological properties, e.g., binding mode, per-residue protonation state, and protein flexibility |
87–89, 92, 97 and 98 |
A full expansion of the methods explained in Table 3 may be found in Honarparvar et al., 2014.97
Conclusion
The various challenges associated with ZIKV treatment has led to the ongoing search for a cure – one of the major problems being drug delivery across the BBB. The approaches described in this review serve to provide information that can be used for further research into the design of drugs with improved BBB-permeability profile that may have a greater ability to inhibit ZIKV. Though experimental validation is necessary, this is not the scope of the current study. Instead, this study serves as a cornerstone that will open doors to further experimental and molecular validation regarding ZIKV therapy.
Conflicts of interest
Authors declare no potential conflicts of interest.
Acknowledgements
The authors acknowledge the College of Health Sciences, UKZN, and the National Research Foundation (NRF) of South Africa.
References
- I. Briguglio, S. Piras, P. Corona and A. Carta, Int. J. Med. Chem., 2011, 2011, 1–22 Search PubMed.
- R. K. Singh, K. Dhama, Y. S. Malik, M. A. Ramakrishnan, K. Karthik, R. Tiwari, S. Saurabh, S. Sachan and S. K. Joshi, Vet. Q., 2016, 36, 150–175 CrossRef PubMed.
- D. Musso, C. Roche, E. Robin, T. Nhan, A. Teissier and V. M. Cao-Lormeau, Emerging Infect. Dis., 2015, 21, 359–361 CrossRef CAS PubMed.
- D. Musso, T. Nhan, E. Robin, C. Roche, D. Bierlaire, K. Zisou, A. Shan Yan, V. M. Cao-Lormeau and J. Broult, Eurosurveillance, 2014, 19, 14–16 CrossRef.
- M. Besnard, S. Lastère, A. Teissier, V. M. Cao-Lormeau and D. Musso, Eurosurveillance, 2014, 19, 8–11 CrossRef.
- G. W. A. Dick, S. F. Kitchen and A. J. Haddow, Trans. R. Soc. Trop. Med. Hyg., 1952, 55, 509–520 CrossRef.
- M. R. Duffy, C. Tai-Ho, T. Hancock, A. M. Powers, J. L. Kool, R. S. Lanciotti, M. Pretrick, C. Dubray, L. Guillaumot, A. Griggs, M. Bel, A. J. Lambert, J. Laven, O. Kosoy, A. Panella, B. J. Biggerstaff, M. Fischer and E. B. Hayes, N. Engl. J. Med., 2009, 360, 2536–2543 CrossRef CAS PubMed.
- D. Musso, E. J. Nilles and V. M. Cao-Lormeau, Clin. Microbiol. Infect., 2014, 20, O595–O596 CrossRef CAS PubMed.
- V. Sikka, V. K. Chattu, R. K. Popli, T. J. Galwankar, C. Sagar, D. Kelkar, S. G. Sawicki, S. P. Stawicki and T. J. Papadimos, J. Global Infect. Dis., 2016, 8, 3–15 CrossRef PubMed.
- S.-I. Yun and Y.-M. Lee, J. Microbiol., 2017, 55, 204–219 CrossRef CAS PubMed.
- G. S. Campos, A. C. Bandeira and S. I. Sardi, Emerging Infect. Dis., 2015, 21, 1885–1886 CrossRef PubMed.
- D. Musso and D. J. Gubler, Nature, 2016, 11, 10–20 Search PubMed.
- F. Krauer, M. Riesen, L. Reveiz, O. T. Oladapo, R. Martínez-Vega, T. V Porgo, A. Haefliger, N. J. Broutet and N. Low, PLoS Med., 2017, 14, 1–27 Search PubMed.
- P. Ramharack and M. E. S. Soliman, RSC Adv., 2016, 6, 68719–68731 RSC.
- D. Musso, C. Roche, T. X. Nhan, E. Robin, A. Teissier and V. M. Cao-Lormeau, J. Clin. Virol., 2015, 68, 53–55 CrossRef PubMed.
- J. M. Mansuy, M. Dutertre, C. Mengelle, C. Fourcade, B. Marchou, P. Delobel, J. Izopet and G. Martin-Blondel, Lancet Infect. Dis., 2016, 16, 405 CrossRef PubMed.
- J. M. Turmel, P. Abgueguen, B. Hubert, Y. M. Vandamme, M. Maquart, H. Le Guillou-Guillemette and I. Leparc-Goffart, Lancet, 2016, 387, 2501 CrossRef.
- A. C. Gourinat, O. O'Connor, E. Calvez, C. Goarant and M. Dupont-Rouzeyrol, Emerging Infect. Dis., 2015, 21, 84–86 CrossRef CAS PubMed.
- G. Calvet, R. S. Aguiar, A. S. O. Melo, S. A. Sampaio, I. de Filippis, A. Fabri, E. S. M. Araujo, P. C. de Sequeira, M. C. L. de Mendonça, L. de Oliveira, D. A. Tschoeke, C. G. Schrago, F. L. Thompson, P. Brasil, F. B. dos Santos, R. M. R. Nogueira, A. Tanuri and A. M. B. de Filippis, Lancet Infect. Dis., 2016, 16, 653–660 CrossRef PubMed.
- O. Faye, O. Faye, A. Dupressoir, M. Weidmann, M. Ndiaye and A. Alpha Sall, J. Clin. Virol., 2008, 43, 96–101 CrossRef CAS PubMed.
- R. Hamel, O. Dejarnac, S. Wichit, P. Ekchariyawat, A. Neyret, N. Luplertlop, M. Perera-Lecoin, P. Surasombatpattana, L. Talignani, F. Thomas, V. M. Cao-Lormeau, V. Choumet, L. Briant, P. Despres, A. Amara, H. Yssel and D. Misse, J. Virol., 2015, 89, 8880–8896 CrossRef CAS PubMed.
- O. Faye, C. C. M. Freire, A. Iamarino, O. Faye, J. Velasco, C. De Oliveira, M. Diallo, P. M. A. Zanotto and A. A. Sall, PLoS Neglected Trop. Dis., 2014, 8, 1–10 Search PubMed.
- H. Tian, X. Ji, X. Yang, W. Xie, K. Yang, C. Chen, C. Wu, H. Chi, Z. Mu, Z. Wang and H. Yang, Protein Cell, 2016, 7, 450–454 CrossRef PubMed.
- H. Tian, X. Ji, X. Yang, Z. Zhang, Z. Lu, K. Yang, C. Chen, Q. Zhao, H. Chi, Z. Mu, W. Xie, Z. Wang, H. Lou, H. Yang and Z. Rao, Protein Cell, 2016, 7, 562–570 CrossRef CAS PubMed.
- B. Ganguly and E. Ganguly, bioRxiv, 2016, p. 54486 Search PubMed.
- M. K. White, H. S. Wollebo, J. David Beckham, K. L. Tyler and K. Khalili, Ann. Neurol., 2016, 80, 479–489 CrossRef CAS PubMed.
- N. J. da Fonseca Jr, et al., Biochem. Biophys. Res. Commun., 2017 DOI:10.1016/j.bbrc.2017.01.041.
- G. Li, M. Poulsen, C. Fenyvuesvolgyi, Y. Yashiroda, M. Yoshida and J. M. Simard, Proc. Natl. Acad. Sci. U. S. A., 2016, 114, 201619735 Search PubMed.
- P. Brasil, P. C. Sequeira, A. D. Freitas, H. E. Zogbi, G. A. Calvet, R. V de Souza, A. M. Siqueira, M. C. de Mendonca, R. M. Nogueira, A. M. de Filippis and T. Solomon, Lancet, 2016, 387, 1482 CrossRef.
- J. J. Miner and M. S. Diamond, Cell Stem Cell, 2016, 18, 559–560 CrossRef CAS PubMed.
- T. J. Nowakowski, A. A. Pollen, E. Di Lullo, C. Sandoval-Espinosa, M. Bershteyn and A. R. Kriegstein, Cell Stem Cell, 2016, 18, 591–596 CrossRef CAS PubMed.
- H. Retallack, E. Di Lullo, C. Arias, K. A. Knopp, M. T. Laurie, C. Sandoval-Espinosa, W. R. M. Leon, R. Krencik, E. M. Ullian, J. Spatazza, A. A. Pollen, C. Mandel-Brehm, T. J. Nowakowski, A. R. Kriegstein, J. L. Derisi, N. Sestan and P.-Y. Shi, Proc. Natl. Acad. Sci. U. S. A., 2016, 113, 14408–14413 CrossRef CAS PubMed.
- P. K. Singh, J.-M. Guest, M. Kanwar, J. Boss, N. Gao, M. S. Juzych, G. W. Abrams, F.-S. Yu and A. Kumar, JCI Insight, 2017, 2(4) DOI:10.1172/jci.insight.92340.
- N. R. Saunders, M. D. Habgood, K. Møllgård and K. M. Dziegielewska, F1000Research, 2016, 5, 1–15 CrossRef PubMed.
- M. R. Syme, J. W. Paxton and J. a. Keelan, Clin. Pharmacokinet., 2004, 43, 487–514 CrossRef CAS PubMed.
- W. A. Banks, BMC Neurol., 2009, 9, S3 CrossRef PubMed.
- A. Seelig, R. Gottschlich and R. M. Devant, Proc. Natl. Acad. Sci. U. S. A., 2016, 91, 68–72 CrossRef.
- J. M. Tarbell and M. Y. Pahakis, J. Intern. Med., 2006, 259, 339–350 CrossRef CAS PubMed.
- N. J. Barrows, R. K. Campos, S. T. Powell, K. R. Prasanth, G. Schott-Lerner, R. Soto-Acosta, G. Galarza-Muñoz, E. L. McGrath, R. Urrabaz-Garza, J. Gao, P. Wu, R. Menon, G. Saade, I. Fernandez-Salas, S. L. Rossi, N. Vasilakis, A. Routh, S. S. Bradrick and M. A. Garcia-Blanco, Cell Host Microbe, 2016, 20, 259–270 CAS.
- A. Daina, O. Michielin and V. Zoete, Sci. Rep., 2017, 7, 1–13 CrossRef PubMed.
- A. Daina, O. Michielin and V. Zoete, J. Chem. Inf. Model., 2014, 54, 3284–3301 CrossRef CAS PubMed.
- Y. N. Gavhane and A. V. Yadav, Saudi Pharm. J., 2012, 20, 331–344 CrossRef PubMed.
- P. Atkins and J. De Paula, Physical chemistry for the life sciences, 2011 Search PubMed.
- P. P. Kore, M. M. Mutha, R. V. Antre, R. J. Oswal and S. S. Kshirsagar, J. Med. Chem., 2012, 2, 139–148 CAS.
- J. A. Arnott and S. L. Planey, Expert Opin. Drug Discovery, 2012, 7, 863–875 CrossRef CAS PubMed.
- R. Guixà-Gonzá Lez, I. Rodriguez-Espigares, J. M. Ramírez-Anguita, P. Carrió-Gaspar, H. Martinez-Seara, T. Giorgino and J. Selent, Bioinformatics, 2014, 30, 1478–1480 CrossRef PubMed.
- X.-Y. Meng, H.-X. Zhang, M. Mezei and M. Cui, Curr. Comput.-Aided Drug Des., 2011, 7, 146–157 CrossRef CAS PubMed.
- E. C. Hulme and M. A. Trevethick, Br. J. Pharmacol., 2010, 161, 1219–1237 CrossRef CAS PubMed.
- T. H. Scheuermann, S. B. Padrick, K. H. Gardner and C. A. Brautigam, Anal. Biochem., 2016, 496, 79–93 CrossRef CAS PubMed.
- Y. Q. Deng, N. N. Zhang, C. F. Li, M. Tian, J. N. Hao, X. P. Xie, P. Y. Shi and C. F. Qin, Open Forum Infect. Dis., 2016, 3, 6–9 Search PubMed.
- S. E. Reznik and C. R. Ashby, Int. J. Infect. Dis., 2017, 55, 29–30 CrossRef CAS PubMed.
- C. Roney, P. Kulkarni, V. Arora, P. Antich, F. Bonte, A. Wu, N. N. Mallikarjuana, S. Manohar, H.-F. Liang, A. R. Kulkarni, H.-W. Sung, M. Sairam and T. M. Aminabhavi, J. Controlled Release, 2005, 108, 193–214 CrossRef CAS PubMed.
- C. Spuch and C. Navarro, J. Drug Delivery, 2011, 2011, 1–12 CrossRef PubMed.
- M. S. Gunay, A. Y. Ozer and S. Chalon, Curr. Neuropharmacol., 2016, 14, 376–391 CrossRef CAS PubMed.
- E. Blanco, H. Shen and M. Ferrari, Nat. Biotechnol., 2015, 33, 941–951 CrossRef CAS PubMed.
- A. Srivastava, T. Yadav, S. Sharma, A. Nayak, A. Kumari and N. Mishra, J. Biosci. Med., 2016, 4, 69–84 Search PubMed.
- W. B. Liechty, D. R. Kryscio, B. V. Slaughter and N. A. Peppas, Annu. Rev. Chem. Biomol. Eng., 2010, 1, 149–173 CrossRef CAS PubMed.
- Z. Abouelfadel and D. Crawford, Ther. Clin. Risk Manage., 2008, 4, 513–526 CrossRef CAS.
- A. Coune, Infection, 1988, 16, 141–147 CrossRef CAS PubMed.
- M. A. Tran, R. J. Watts and G. P. Robertson, Pigm. Cell Melanoma Res., 2009, 22, 388–399 CrossRef CAS PubMed.
- U. Kedar, P. Phutane, S. Shidhaye and V. Kadam, Nanomedicine, 2010, 6, 714–729 CrossRef CAS PubMed.
- X.-B. Xiong, H. Uludağ and A. Lavasanifar, Biomaterials, 2010, 31, 5886–5893 CrossRef CAS PubMed.
- D. Bhowmik, H. Gopinath, B. Pragati Kumar, S. Duraivel and K. P. Sampath Kumar, Pharma Innovation, 2012, 1, 12–31 CAS.
- L. A. Sharpe, A. M. Daily, S. D. Horava and N. A. Peppas, Expert Opin. Drug Delivery, 2014, 11, 901–915 CrossRef CAS PubMed.
- X. Yu, I. Trase, M. Ren, K. Duval, X. Guo and Z. Chen, J. Nanomater., 2016 DOI:10.1155/2016/1087250.Design.
- H. Daraee, A. Eatemadi, E. Abbasi, S. Fekri Aval, M. Kouhi and A. Akbarzadeh, Artif. Cells, Nanomed., Biotechnol., 2014, 1401, 1–13 Search PubMed.
- W. Tao, B. L. Hurst, A. K. Shakya, M. J. Uddin, R. S. J. Ingrole, M. Hernandez-Sanabria, R. P. Arya, L. Bimler, S. Paust, E. B. Tarbet and H. S. Gill, Antiviral Res., 2017, 141, 62–72 CrossRef CAS PubMed.
- M. Rai, S. D. Deshmukh, A. P. Ingle, I. R. Gupta, M. Galdiero and S. Galdiero, Crit. Rev. Microbiol., 2014, 42, 46–56 Search PubMed.
- S. Mukherjee, S. Ray and R. S. Thakur, Indian J. Pharm. Sci., 2009, 71, 349–358 CrossRef CAS PubMed.
- J. D. Bazzill, C. L. Cooper, Y. Fan, S. Bavari and J. J. Moon, J. Immunol., 2016, 196(1 Suppl.), 76 Search PubMed.
- J. Torrecilla, A. del Pozo-Rodríguez, M. ÿngeles Solinís, P. S. Apaolaza, B. Berzal-Herranz, C. Romero-López, A. Berzal-Herranz and A. Rodríguez-Gascón, Colloids Surf., B, 2016, 146, 808–817 CrossRef CAS PubMed.
- A. del Pozo-Rodríguez, M. Á. Solinís and A. Rodríguez-Gascón, Eur. J. Pharm. Biopharm., 2016, 109, 184–193 CrossRef PubMed.
- A. K. Dey, M. Khati, M. Tang, R. Wyatt, S. M. Lea and W. James, J. Virol., 2005, 79, 13806–13810 CrossRef CAS PubMed.
- F. Jiang, B. Liu, J. Lu, F. Li, D. Li, C. Liang, L. Dang, J. Liu, B. He, S. A. Badshah, C. Lu, X. He, B. Guo, X. B. Zhang, W. Tan, A. Lu and G. Zhang, Int. J. Mol. Sci., 2015, 16, 23784–23822 CrossRef CAS PubMed.
- S. D. Jayasena, Clin. Chem., 1999, 45, 1628–1650 CAS.
- P. Ray and R. R. White, Pharmaceuticals, 2010, 3, 1761–1778 CrossRef CAS PubMed.
- M. Blind and M. Blank, Mol. Ther.--Nucleic Acids, 2015, 4, e223 CrossRef PubMed.
- E. Lionta, G. Spyrou, D. K. Vassilatis and Z. Cournia, Curr. Top. Med. Chem., 2014, 14, 1923–1938 CrossRef CAS PubMed.
- J. J. Irwin and B. K. Shoichet, J. Chem. Inf. Model., 2005, 45, 177–182 CrossRef CAS PubMed.
- D. R. Koes and C. J. Camacho, Nucleic Acids Res., 2012, 40, 409–414 CrossRef PubMed.
- E. F. Pettersen, T. D. Goddard, C. C. Huang, G. S. Couch, D. M. Greenblatt, E. C. Meng and T. E. Ferrin, J. Comput. Chem., 2004, 25, 1605–1612 CrossRef CAS PubMed.
- O. Trott and A. J. Olson, J. Comput. Chem., 2010, 31, 445–461 Search PubMed.
- N. Mhlongo and M. E. Soliman, Lett. Drug Des. Discovery, 2016, 13, 1033–1046 CrossRef CAS.
- F. N. Cele, R. Muthusamy and M. E. Soliman, Drug Des., Dev. Ther., 2016, 10, 1365–1377 Search PubMed.
- A. Skelton, Y. Maharaj and M. E. Soliman, Cell. Mol. Bioeng., 2014, 7, 45–57 CrossRef CAS.
- E. O. Pyzer-Knapp, C. Suh, R. Gómez-Bombarelli, J. Aguilera-Iparraguirre and A. Aspuru-Guzik, Annu. Rev. Mater. Res., 2015, 45, 195–216 CrossRef CAS.
- H. Alonso, A. A. Bliznyuk and J. E. Gready, Med. Res. Rev., 2006, 26, 531–568 CrossRef CAS PubMed.
- M. A. González, Collection SFN, 2011, 12, 169–200 CrossRef.
- M. Nelson, W. Humphrey, A. Gursoy, A. Dalke, L. Kal, R. D. Skeel and K. Schulten, Int. J. High Perform. Comput. Appl., 1996, 251–268, 251–268 Search PubMed.
- B. Hess, C. Kutzner, D. Van Der Spoel and E. Lindahl, J. Chem. Theory Comput., 2008, 4, 435–447 CrossRef CAS PubMed.
- D. A. Case, T. E. Cheatham, T. Darden, H. Gohlke, R. Luo, K. M. Merz, A. Onufriev, C. Simmerling, B. Wang and R. J. Woods, J. Comput. Chem., 2005, 26, 1668–1688 CrossRef CAS PubMed.
- B. R. Brooks, R. E. Bruccoleri, B. D. Olafson, D. J. States, S. Swaminathan and M. Karplus, J. Comput. Chem., 1983, 4, 187–217 CrossRef CAS.
- Y. Qi, X. Cheng, J. Lee, J. V. Vermaas, T. V. Pogorelov, E. Tajkhorshid, S. Park, J. B. Klauda and W. Im, Biophys. J., 2015, 109, 2012–2022 CrossRef CAS PubMed.
- A. W. Duster, C.-H. Wang, C. M. Garza, D. E. Miller and H. Lin, WIREs Computational Molecular Science, 2017 DOI:10.1002/wcms.1310.
- K. Vanommeslaeghe, O. Guvench and A. D. Mackerell, Curr. Pharm. Des., 2014, 20, 3281–3292 CrossRef CAS PubMed.
- S. B. Vepuri, H. C. Devarajegowda and M. E. Soliman, J. Mol. Struct., 2016, 1105, 194–204 CrossRef CAS.
- B. Honarparvar, T. Govender, G. E. M. Maguire, M. E. S. Soliman and H. G. Kruger, Chem. Rev., 2013, 114(1), 493–537 CrossRef PubMed.
- A. Ganesan, M. L. Coote and K. Barakat, Drug Discovery Today, 2017, 22, 249–269 CrossRef CAS PubMed.
Footnote |
† Electronic supplementary information (ESI) available. See DOI: 10.1039/c7ra05918c |
|
This journal is © The Royal Society of Chemistry 2017 |