DOI:
10.1039/C7RA04337F
(Paper)
RSC Adv., 2017,
7, 30060-30069
Plasma metabolic profiling and novel metabolite biomarkers for diagnosing prostate cancer†
Received
18th April 2017
, Accepted 30th May 2017
First published on 9th June 2017
Abstract
Prostate cancer (PCa) is the second leading cause of cancer death among men and associated with profound metabolic changes. It is important to discover new biomarkers for the diagnosis of PCa and metabolomics technology has made progress toward identifying metabolic alterations in diseases that may provide clinically useful biomarkers. The purpose of this work was to determine the distinctive metabolic signatures from PCa. Plasma samples from PCa patients and age-matched healthy controls were investigated using high resolution ultrahigh performance liquid chromatography-mass spectrometry (UPLC/MS) and the datasets were analyzed using pattern recognition methods. Metabolic differences among PCa and control subjects were identified based on multivariate statistical analyses. As a result, 19 distinguishable metabolites were detected and 11 metabolic pathways were established. The altered metabolic pathways were associated with synthesis and degradation of ketone bodies, and phenylalanine metabolism, etc. To demonstrate the utility of plasma biomarkers for the diagnosis of PCa, three metabolites comprising sarcosine, acetylglycine and coreximine that contributed to the combined model were selected as candidate biomarkers. Distinctive signature with these metabolites could significantly increase the diagnostic performance of PCa. In this study, metabolomics technology has proved to be a powerful tool for the discovery of new biomarkers for disease and suggest that panels of metabolites may be valuable to translate our findings to clinically useful diagnostic tests.
Introduction
Prostate cancer (PCa) is the second most diagnosed cancer in men, and principally affecting men over 50 years old worldwide.1 Its incidence and associated, mortality rates of PCa are increasing rapidly with the growing aging population.2 However, the diagnosis and possibility of predicting the outcome for PCa patients remain troublesome. Currently, detection of PCa mainly involves testing of serum prostate-specific antigen (PSA) level.3–5 However, recent studies suggested that PSA is not a specific biomarker of PCa and the diagnostic paradigm suffers from low specificity of PSA. There are further concerns regarding possible over-diagnosis of PCa by PSA in patients with limited potential for disease progression.6 Although several promising candidates have been proposed,7–9 the ideal biomarker or group of biomarkers for PCa remains elusive. Therefore, it is necessary to search a rapid and high efficiency method for increasing the accuracy of diagnosis.
Biomarkers can improve PCa diagnosis and treatment. There is a close relationship between metabolism and cancer. Metabolomics is a promising avenue for biomarker discovery.10 Recent reports suggest that molecular biomarkers have been identified and provided by metabolomic studies.11 Metabolomics is an approach that allows the analysis of the entire metabolites in a biological system. In metabolomics, a large number of small molecules (metabolites) can be detected in a single sample. It has been expanding in scope, from its primary use in the clinic for a new diagnostic role in diseases.12 Metabolomics could provide insights of the physiological status from a biological system. The alterations in the normal metabolome may be indicative of diseases.13–15 These alterations in the normal metabolome have the potential to discover new diagnostic biomarkers for the diseases. The diagnostic capacity of plasma metabolite analyses has also been confirmed by several studies.16
In this study, we mainly aim to identify the potential metabolic biomarkers and further to explore whether plasma metabolomics method could be used for diagnosing PCa. Metabolites were measured after analysis of plasma samples with ultrahigh performance liquid chromatography coupled to mass spectrometry (UPLC-MS) instrumentation. Multivariate parametric statistical test and receiver operating characteristics analysis was performed to evaluate diagnostic performance of the metabolites. Besides, we will significantly contribute to a deeper understanding of the biochemical metabolic networks and pathways in PCa.
Experimental
Chemicals and reagents
Methanol (HPLC grade) and acetonitrile (HPLC grade) were purchased from Merck (Darmstodt, Germany). Ultrapure water was provided by a Milli-Q water purification system (Millipore, Billerica, USA). Formic acid and leucine enkephalin was purchased from Sigma-Aldrich (St. Louis, MO, USA).
Study participants
All patients and control individuals consisted of healthy men were recruited from the First Affiliated Hospital, Heilongjiang University of Chinese Medicine, and all patients signed informed consents before the study began. Criteria for selection of the PCa group was the following: PCa diagnosis based on digital rectal examination, transrectal ultrasonography and examination of biopsy tissue sample, no other coexisting cancers, no PCa treatment. The control group has no cancer and no chronic diseases. A total of 32 patients together with 32 normal control cases were enrolled. Characteristics of the PCa group and control group were reflected in ESI Table 1.† Before collection of the samples, patients had given consent and had undergone therapeutic interventions. The study was endorsed by the Review Board of Heilongjiang University of Chinese Medicine (HUCM-2016-0652) and complied with the provisions of the Good Clinical Practice Guidelines and the Declaration of Helsinki.
Sample collection and preparation for metabolomics analysis
Plasma samples were collected from PCa patients and control group. Fasting venous blood samples were collected using vacuum blood collection tube that contained anticoagulant dipotassium EDTA. Plasma was separated by centrifugation at 5000 rpm for 10 min and the supernatant was stored at −80 °C until further analysis. To ensure the stability and repeatability of UPLC/MS, the blank samples and quality control samples were used in this study. All the plasma samples were thawed at 4 °C and a volume 300 μL of cold methanol were added to 100 μL of plasma for deproteinization, and then centrifuged at 5000 rpm for 10 min. Next, the supernatants were recovered, evaporated using a vacuum rotary dryer and re-suspended in 100 μL acetonitrile/water (1
:
3, v/v), vortex-mixed for 5 min, then centrifuged at 5000 rpm for 10 min, and the supernatant was held for UPLC/MS analysis.
LC-MS analysis
Chromatographic separation was performed on Ultra Performance Liquid Chromatography (UPLC, Waters Corporation, Manchester, UK) system. The instrument was equipped with a degasser, binary pump, thermostatted autosampler, and column oven (Waters, Milford, USA). Samples were performed on UPLC™ BEH C18 column (50 mm × 2.1 mm i.d., 1.7 μm, Waters, Milford, USA). The column was maintained at 45 °C. The flow rate throughout the separation was maintained at 0.5 mL min−1. The mobile phase was consisted of acetonitrile/water (50
:
50 v/v) containing 0.1% formic acid (solvent A) and acetonitrile/water (90
:
10 v/v) containing 0.1% formic acid (solvent B). The gradient conditions were 0–2 min 1–15% B; 2–6 min 15–50% B; 6–10 min 50–95% B; 10–12 min 95% B followed by re-equilibration to the initial starting condition. The samples were kept at 4 °C, and a constant volume of 4 μL was injected for analysis.
MS full scan was performed positive ion mode and negative ion mode using a time of flight mass spectrometer (Waters, Milford, USA), which was coupled to the UPLC system. The mass scanning range was 50–1500 m/z in the full scan mode, with a capillary voltage of 3000 V, a collision gas flow rate of 10 L min−1, and a nebulizer gas flow rate of 35 L min−1, the capillary temperature of 300 °C, the source temperature of 120 °C, and desolvation gas temperature of 400 °C. Nitrogen was used as the collision gas at a collision cell pressure of 2.0 × 10−5 Torr. The flow rates of cone and desolvation gas were set at 60 L h−1 and 400 L h−1, respectively.
Multivariate statistical analyses
LC-MS data were analyzed to identify potential discriminant biomarkers. The peak finding, filtering, and alignment were performed by MarkerLynx 4.1 application manager (Waters, Manchester, UK). The MS matrix was then introduced to EZinfo 2.0 software (Waters, Manchester, UK) for orthogonal signal correction-partial least squares discriminant analysis (OPLS-DA) which produces a score plot showing the separation of the groups based on the content of the loading discriminatory metabolites. R2Y (cum) and Q2 (cum) parameters were used for the evaluation of the models, indicating the fitness and prediction ability, respectively. VIP-plot from OPLS-DA was utilized to rank the metabolites, according to their importance to the classification. The differential metabolites were identified based on the MS/MS fragment comparison with the standard compounds, or via search of the candidate compounds in the database including the HMDB, METLIN and ChemSpider.
Metabolic pathway and network analysis
To identify the most relevant metabolic pathways involved in PCa, metabolic pathway analysis was employed to perform the pathway topology analysis using the software MetaboAnalyst 3.0 (http://www.metaboanalyst.ca). The metabolites with VIP scores >1.0 in the partial least squares were examined and selected for their metabolic pathway analysis.
Discrimination performance of potential biomarkers
Metabolomics was performed to explore whether metabolomic signatures had the potential discrimination ability between PCa and control group. To obtain a final diagnostic score, receiver operating characteristic (ROC) curves were generated using the rocplot function program. It allows characterization of diagnostic accuracy, and evaluates the predictive accuracy. ROC curves were used to estimate the accuracy of the combined signatures model.
Statistical analysis
The areas under curve (AUC) of ROC curves were used to determine the diagnostic effectiveness of important metabolites using the online software MetaboAnalyst 3.0 (http://www.metaboanalyst.ca). The SVM algorithm was used to calculate the importance of all variables and rank them. The t test analysis of covariance was performed using SPSS software (version 19.0; SPSS, Inc., Chicago, IL), with p < 0.05 deemed to be significant.
Results
Baseline clinical characteristics
Characteristics of the study population are presented in ESI Table 1.† The mean ages, sex, body mass index, 2 hour postprandial glucose, total cholesterol, triglycerides, systolic blood pressure, and diastolic blood pressure were not significantly different between patients with PCa cases and age-matched healthy controls. The PSA was 9.8 ± 5.6 for PCa patients, indicating a fairly advanced stage of this disease. The serum PSA level significantly differed between the controls and PCa patients (P < 0.05).
Overall metabolomics analysis of plasma samples
The first aim of this work was to analyze the global metabolic differences between PCa and health cases. To do this, we applied global metabolomics focusing on the profiles of low molecular weight metabolites. In this study, the plasma samples were analyzed by UPLC-MS tool. The separation conditions of plasma samples were optimized. Representative base peak intensity chromatograms of plasma samples from the controls and the PCa cases in positive ion mode were presented in Fig. 1. The serum spectra contained high-intensity signals from the small molecules and the utilization of multivariate statistical approach could enlarge metabolite identification. The variables were exported into EZinfo 2.0 software (Waters, Manchester, UK) for multivariate data analysis to detect metabolite. Statistical evaluation by principal component analysis (PCA) showed a clearly different distribution in the positive ion model (Fig. 2A) and negative ion mode (Fig. 2B). The wider spread of the samples in the PCA was caused by altered levels of metabolites. OPLS-DA was then applied to evaluate the more marked variations and statistical meaning of those ions.
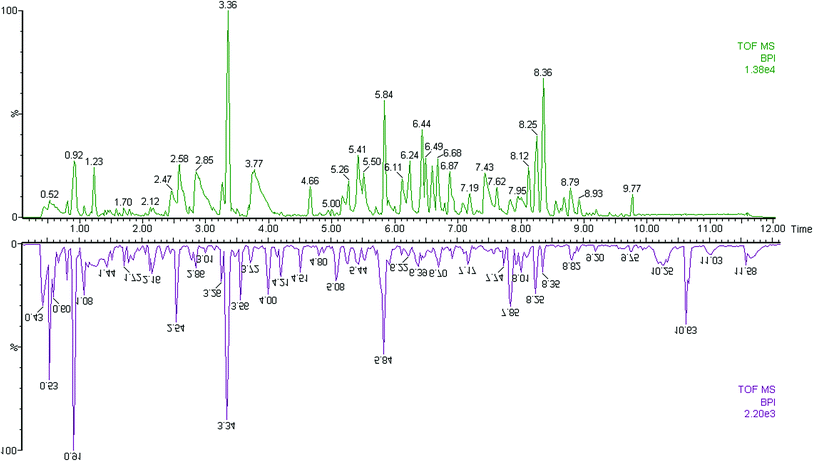 |
| Fig. 1 Typical base peak chromatograms of plasma samples from control subjects (up) and PCa patients (down) obtained by UPLC-MS tool. | |
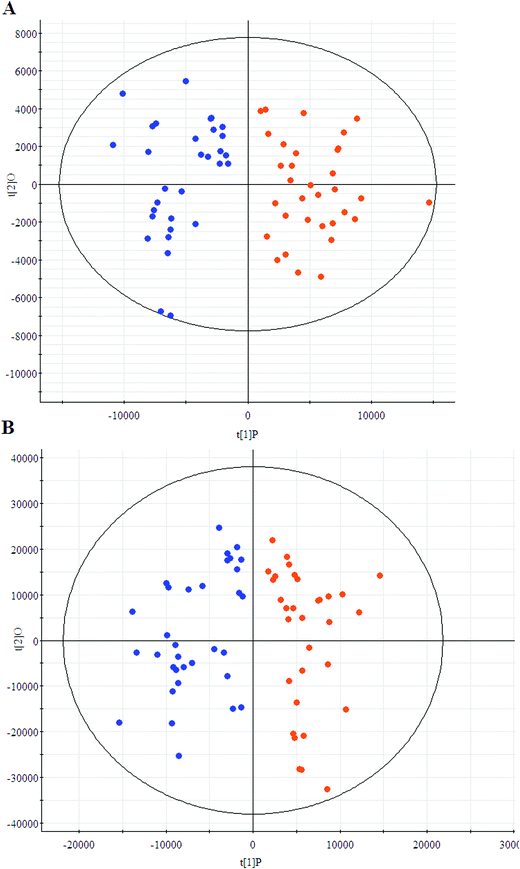 |
| Fig. 2 Principal component analysis score plots of the patients (blue) and controls (red) group in positive ion model (A) and negative ion model (B). | |
Plasma metabolites related to PCa
We employed an OPLS-DA statistical way termed VIP-plot to select metabolites that highly contributed to the behaviors. VIP-plot of PCa patients vs. control individuals was shown in Fig. 3, which is a scatter plot that combines the covariance and correlation. Higher values of VIP indicate metabolites that are more important to the classification. Variables with VIP value greater than 6 were considered as great value. A t test was performed in variables with significant differences between PCa cases and control individuals (P < 0.01) were retained. According to the VIP scores, a total of 19 discriminate variables (listed in ESI Table 2†) as interesting candidates were found in PCa. Elemental composition was estimated using the Masslynx 4.1 software. And then, it was finally confirmed by comparison with a standard sample. Then, the metabolites were analyzed using a heat map and it indicated that the two groups could be separated based on these metabolites (Fig. 4).
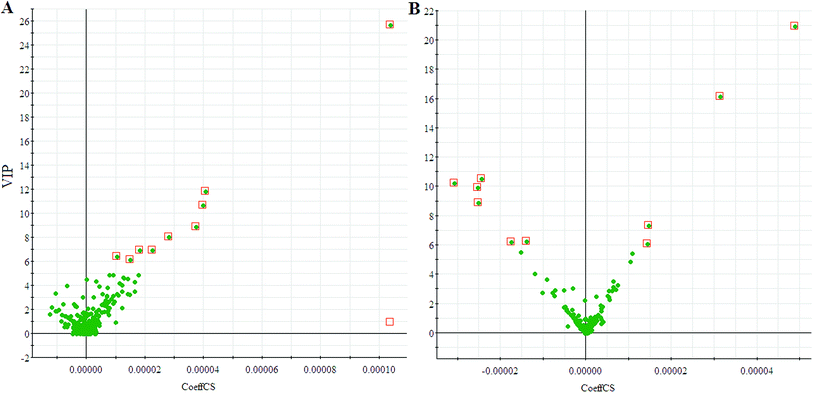 |
| Fig. 3 VIP-plot for screening of significantly changed metabolites in positive electrospray mode (A) and negative electrospray modes (B). | |
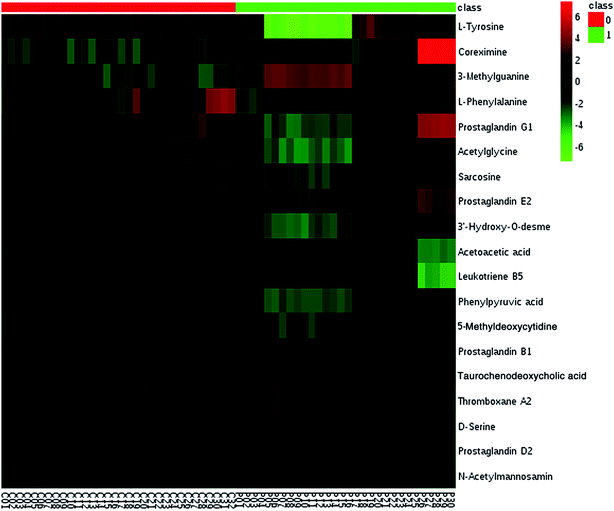 |
| Fig. 4 Heatmap visualization analysis of metabolites statistically significant with a potential identity. | |
Metabolite pathways related to PCa
All of the attributed metabolites were analyzed utilizing Metaboanalyst 3.0. The pathway analysis results showed the metabolic network reprogramming of PCa with detailed impact (Fig. 5). The most influenced metabolic pathway was set as a pathway influence >0.01. The biological pathway analysis revealed 11 metabolic pathways, including synthesis and degradation of ketone bodies, phenylalanine metabolism, arachidonic acid metabolism, glycine, serine and threonine metabolism, tyrosine metabolism, butanoate metabolism, propanoate metabolism, amino sugar and nucleotide sugar metabolism, primary bile acid biosynthesis, phenylalanine, tyrosine and tryptophan biosynthesis, arginine and proline metabolism (ESI Table 3†). To visualize biological connectivity of the significantly changed metabolites, the network-generating algorithm of ingenuity pathway analysis was used to maximize the interconnectedness of molecules based on the database. It revealed that the PCa patients possessed a highly unique metabolic phenotype characterized by these metabolic pathways (Fig. 6).
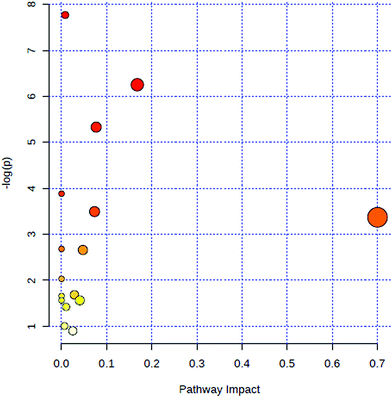 |
| Fig. 5 Summary of metabolic pathways of significantly changed metabolites with MetPA. Circles represent metabolism (ESI Table S3†). All matched pathways are plotted according to p-value from pathway enrichment analysis and pathway impact score from pathway topology analysis. Colour gradient and circle size indicate the significance of the pathway ranked by p-value (yellow: higher p-values and red: lower p-values) and pathway impact score (the larger the circle the higher the impact score), respectively. | |
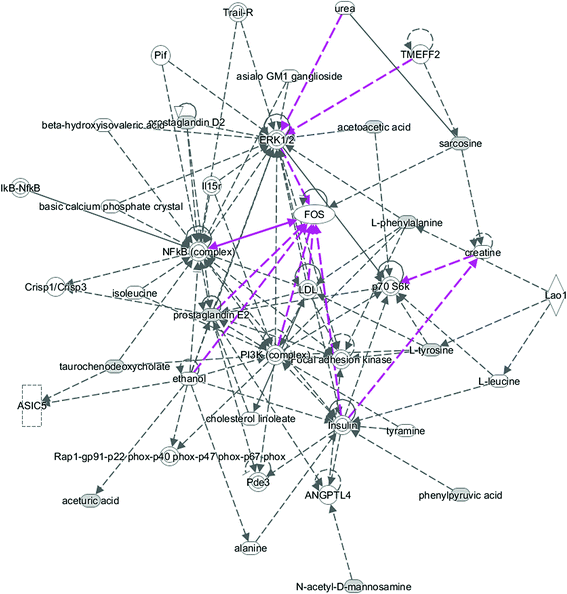 |
| Fig. 6 Receiver operator characteristic analysis of metabolites significantly associated with PCa. | |
Potential diagnostics of metabolites
We have performed ROC analysis to further characterize the predictive value of these individual metabolites independently. We found 4 metabolites (sarcosine, acetylglycine, coreximine and 3-methylguanine (p = 0.0000)) with an area under the curve (AUC) >0.7. Among the metabolites, we found with highest significant the sarcosine (Fig. 7 and ESI Table 4†). The results of univariate ROC curve analyses indicated that in the plasma samples, 7 metabolites with high AUC above 0.70 were: sarcosine, acetylglycine, coreximine, 3-methylguanine, 5-methyldeoxycytidine, D-serine, and leukotriene B5 (ESI Table 4†).
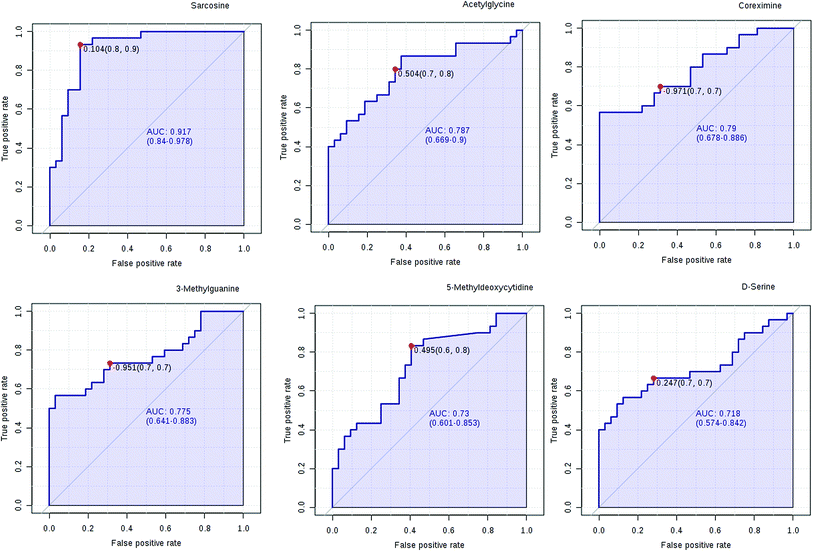 |
| Fig. 7 Ingenuity pathway analysis of the significantly changed metabolites. | |
PCa diagnostics related metabolites
Currently, detection of PCa using test of PSA level showed a low discriminator (AUC = 92.6%) (Fig. 8). It is necessary to establish a novel method for increase the accuracy of diagnosis. To improve the prediction of PCa, a combination of more than one discriminatory metabolite was developed via logistic regression analysis (Fig. 9A). Fig. 9A shows that the combination of metabolites was a better discriminator (AUC > 94%) than each metabolite individually (AUC < 94%), which reinforced the improved capacity of biomarker patterns to make a distinction between different groups. Thus, the distinctive signature with the 3 metabolites had achieved the highest AUC value and significantly increased the diagnostic performance of PCa. The designed model performance was assessed by the AUC. Notably, for the feature ranking method, top 3 metabolites including sarcosine, acetylglycine and coreximine contributed to the combined model (Fig. 9B). Thus, the distinctive signature of the 3 metabolites significantly increased the diagnostic performance of PCa.
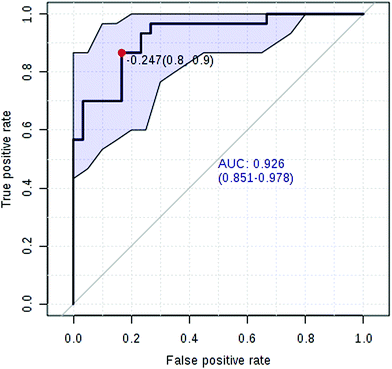 |
| Fig. 8 ROC curve analysis for the serum prostate-specific antigen level. | |
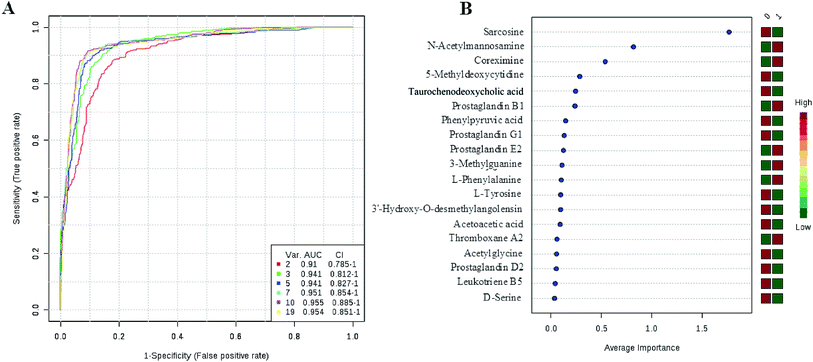 |
| Fig. 9 ROC curve for the predictive model. A combination metabolites model calculated from the logistic regression analysis (A); features ranked by their contributions to classification accuracy (B). | |
Discussion
PCa is a significant cause of morbidity and mortality among men worldwide. Current diagnosis of PCa is based on prostate biopsies, but it can lead to false-negative and false-positive results.17 The better biomarkers are urgently needed to improve the specificity of PCa diagnosis and avoid unnecessary biopsies. For the discovery of new biomarkers, the application of omics is emerging, especially metabolomics screening techniques. Cancer has been associated with marked metabolic change. Metabolites can be altered in cancer, because the metabolites produced are indicators of what is happening in the metabolism of disease conditions.18 Metabolomics has significantly contributed to a deeper understanding of the metabolic pathways in cancer. Some studies have demonstrated that dysregulated metabolism is associated with the survival of cancers in patients.19 Identification of the dysfunctional metabolic pathways of cancer via metabolomics can be used to discover biomarkers and targets.20 Some previous studies have attempted to use metabolomics to discover biomarkers that might apply the detection of cancer.21 Plasma is a readily available biofluid that may contain metabolites of interest for diagnosis of diseases. Indeed, a growing number of studies have used plasma-based metabolomics as a method of discovering biomarkers for diagnosing PCa.22
In the present study, a non-targeted UPLC/MS plasma metabolism method was used to explore the metabolic characteristics related to the PCa patients and to screen meaningful predictors. We compared plasma metabolic profiles of 32 patients with PCa to identify its metabolic signatures. PCA showed a clearly different distribution in the positive ion mode and negative ion mode. The wider spread of the samples in the PCA was caused by different levels of metabolites. We employed VIP-plot of OPLS-DA statistical approach to select metabolites that highly contributed to the clear separation behaviors. According to the VIP scores, a total of 19 discriminate variables as interesting candidates were found in PCa. We identified 9 metabolites in ESI+ mode and 10 metabolites in ESI− mode related to the PCa (Fig. 3 and ESI Table 2†). In the present study, we provided evidence that there exists a high relationship between these metabolites and PCa. The most influenced metabolic pathway includes synthesis and degradation of ketone bodies, phenylalanine metabolism, arachidonic acid metabolism, glycine, serine and threonine metabolism, tyrosine metabolism, butanoate metabolism, propanoate metabolism, amino sugar and nucleotide sugar metabolism, primary bile acid biosynthesis, phenylalanine, tyrosine and tryptophan biosynthesis, arginine and proline metabolism.
In recent years, numerous findings have been published that using the marker candidates could potentially improve the diagnosis and help to identify patients.23–26 Establishing a diagnostic model to predict the PCa was difficult due to the distinct metabolic profile of PCa consisting of the 11 altered metabolic pathways and 19 corresponding metabolites. Now, detection of PCa using test of PSA level showed a low discriminator (AUC = 92.6%). We then performed ROC analysis to characterize the predictive value of those metabolites for discriminating PCa. Evaluation of biomarkers by ROC analysis showed that 7 metabolites with high AUC above 0.70. A combination of more than one discriminatory metabolite was developed via logistic regression analysis to construct an effective diagnostic model for PCa. Notably, for the feature ranking method, we found that top 3 metabolites including sarcosine, acetylglycine and coreximine contributed to the combined model and had achieved the highest AUC value (AUC = 94.1%). Therefore, the distinctive signature of the 3 metabolites significantly increased the diagnostic performance of PCa. It revealed the potential pathogenesis of PCa, and also provided a feasible diagnostic tool for PCa populations through detection of plasma metabolites.
The development of biomarkers to diagnose PCa is meaningful for both patient care and research. In this study, we had investigated whether the alterations in plasma metabolites are available for detection of PCa, and performed based on the UPLC/MS technique supported by the advanced chemometric analysis. The aim of this study is to find metabolite biomarkers that would allow the discovery and diagnosis of PCa. We found that the combination of metabolites was a better discriminator than each metabolite individually. In this study, plasma metabonomics analysis for identifying potential biomarkers to diagnose PCa was successfully demonstrated, which has the advantages of reliable, simple, and low-cost. In conclusion, our research highlights the potential advantages of the plasma metabolomics in real clinical diagnostics.
Conclusions
This is the first study to discover the ideal biomarker, or set of biomarkers, significantly increase the diagnostic performance of PCa. In this study, we applied plasma metabolomics approach based UPLC-MS combined with pattern recognition approach in both positive and negative modes to investigate the discriminated variables. 17 potential biomarkers in the PCa subjects were significantly different from the control subjects. Additionally, plasma metabolomics study presented the dysregulated metabolism related to the PCa and several altered pathways aberrantly expressed were found at metabolic level, including synthesis and degradation of ketone bodies, phenylalanine metabolism, etc. We found that top 3 metabolites including sarcosine, acetylglycine, and coreximine were selected as candidate biomarkers and contributed to the combined model as well as achieved the highest diagnosis value of PCa. These findings provide the basis for a novel diagnostic metabolomics paradigm that could improve the clinical diagnostics of PCa patients. Furthermore, future studies are needed to validate the findings in larger number of patients and investigate their potential clinical utilities in PCa.
Conflict of interest
The authors declare no competing financial interests.
Acknowledgements
This work was supported by grants from the Key Program of Natural Science Foundation of State (Grant No. 81302905), Natural Science Foundation of Heilongjiang Province of China (H2015038), Doctoral Science Foundation of Heilongjiang University of Chinese Medicine (2014bs08), Youth Innovative Talent Program of Heilongjiang Province of China (UNPYSCT-2015118).
References
- O. Twu, D. Dessí, A. Vu, F. Mercer, G. C. Stevens, N. De Miguel and P. J. Johnson, Proc. Natl. Acad. Sci. U. S. A., 2014, 111, 8179–8184 CrossRef CAS PubMed.
- L. P. Bokhorst, C. H. Bangma, G. J. van Leenders, J. J. Lous, S. M. Moss, F. H. Schröder and M. J. Roobol, Eur. Urol., 2014, 65, 329–336 CrossRef PubMed.
- J. C. Byrne, M. R. Downes, N. O'Donoghue, C. O'Keane, A. O'Neill, Y. Fan and R. W. G. Watson, J. Proteome Res., 2008, 8, 942–957 CrossRef PubMed.
- A. Heidenreich, P. A. Abrahamsson, W. Artibani, J. Catto, F. Montorsi, H. Van Poppel and N. Mottet, Eur. Urol., 2013, 64, 347–354 CrossRef PubMed.
- S. H. Strand, T. F. Orntoft and K. D. Sorensen, Int. J. Mol. Sci., 2014, 15, 16544–16576 CrossRef CAS PubMed.
- M. S. Leapman and P. R. Carroll, Urol. Oncol., 2017, 35, 42–50 CrossRef PubMed.
- V. Medina-Villaamil, S. Martinez-Breijo, P. Portela-Pereira, M. Quindos-Varela, I. Santamarina-Cainzos, L. M. Anton-Aparicio and F. Gómez-Veiga, Actas Urológicas Españolas, 2014, 38, 633–639 CrossRef CAS PubMed.
- V. M. Barnes, A. D. Kennedy, F. Panagakos, W. Devizio, H. M. Trivedi, T. Jönsson and F. A. Scannapieco, PloS one, 2014, 9, e105181 Search PubMed.
- J. Zheng, R. A. Dixon and L. Li, Anal. Chem., 2012, 84, 10802–10811 CrossRef CAS PubMed.
- Q. Liang, H. Liu, H. Xing, Y. Jiang and A. H. Zhang, RSC Adv., 2016, 6, 43293–43298 RSC.
- Q. Liang, H. Liu, T. Zhang, Y. Jiang, H. Xing and A. H. Zhang, RSC Adv., 2015, 5, 102204–102209 RSC.
- T. J. Wang, M. G. Larson, R. S. Vasan, S. Cheng, E. P. Rhee, E. McCabe and C. J. O'donnell, Nat. Med., 2011, 17, 448–453 CrossRef CAS PubMed.
- Q. Liang, H. Liu, Y. Jiang, H. Xing, T. Zhang and Z. Ai-hua, RSC Adv., 2016, 6, 11008–11013 RSC.
- Q. Liang, H. Liu, X. Li and A. H. Zhang, RSC Adv., 2016, 6, 75499–75504 RSC.
- A. H. Zhang, H. Sun, Y. Han, G. L. Yan, Y. Yuan, G. C. Song and X. J. Wang, Anal. Chem., 2013, 85, 7606–7612 CrossRef CAS PubMed.
- X. Zang, C. M. Jones, T. Q. Long, M. E. Monge, M. Zhou, L. D. Walker and F. M. Fernandez, J. Proteome Res., 2014, 13, 3444–3454 CrossRef CAS PubMed.
- W. Struck-Lewicka, M. Kordalewska, R. Bujak, A. Y. Mpanga, M. Markuszewski, J. Jacyna and M. J. Markuszewski, J. Pharm. Biomed. Anal., 2015, 111, 351–361 CrossRef CAS PubMed.
- D. Kumar, A. Gupta, A. Mandhani and S. N. Sankhwar, J. Proteome Res., 2015, 14, 1455–1464 CrossRef CAS PubMed.
- A. M. Mondul, S. C. Moore, S. J. Weinstein, S. Männistö, J. N. Sampson and D. Albanes, Metabolomics, 2014, 10, 5 CrossRef PubMed.
- A. Zhang, G. Yan, Y. Han and X. Wang, Appl. Biochem. Biotechnol., 2014, 174, 6–12 CrossRef CAS PubMed.
- Y. Zhang, P. Liu, Y. Li and A. H. Zhang, RSC Adv., 2017, 7, 6780–6787 RSC.
- Q. Liang, H. Liu, L. X. Xie, X. Li and A. H. Zhang, RSC Adv., 2017, 7, 2587–2593 RSC.
- A. Zhang, H. Sun and X. Wang, RSC Adv., 2017, 7, 14966–14973 RSC.
- Y. Li, S. Qiu and A. H. Zhang, Anal. Methods, 2016, 8, 3284–3290 RSC.
- X. Wang, J. Li and A. H. Zhang, RSC Adv., 2016, 6, 63049–63057 RSC.
- Y. Zhou, R. Song, C. Ma, L. Zhou, X. Liu, P. Yin and G. Xu, Oncotarget, 2017, 8, 20719 Search PubMed.
Footnotes |
† Electronic supplementary information (ESI) available. See DOI: 10.1039/c7ra04337f |
‡ Co-authors. |
|
This journal is © The Royal Society of Chemistry 2017 |
Click here to see how this site uses Cookies. View our privacy policy here.