DOI:
10.1039/C7RA00370F
(Paper)
RSC Adv., 2017,
7, 15917-15925
Novel anti-tubulin agents from plant and marine origins: insight from a molecular modeling and dynamics study
Received
10th January 2017
, Accepted 6th March 2017
First published on 10th March 2017
Abstract
The screening of a variety of botanical species and marine organisms provided satisfactory novel tubulin binding agents (TBAs). The current study aims to quantify the binding capabilities of several TBAs including vinca alkaloids, colchicine and other taxol-domain binding agents with microtubule. The stability of the bound complexes and detailed interactions within the active site are the endeavor of the study. Different natural extracts reported as TBAs, have been screened against the refined structure of αβ-tubulin hetero-dimers using ligand docking. The molecular dynamics simulation of the best-docked poses for 50 ns demonstrates that molecules 7 (discodermolide) and 10 (laulimalide) exhibit better tendencies of binding with the microtubule. Average RMSD analysis and dynamical pathway observations indicate that these molecules transit quickly to a dynamically stable configuration and seem to achieve a comfort zone by remaining stable throughout the dynamics. The results obtained, may form the foundation for the future synthesis and evaluation of new compounds with potential tubulin binding properties.
1. Introduction
Microtubules are highly dynamic, cylindrical, cytoskeletal protein filaments that play a relevant role in the regulation of diverse cellular functions, for instance, transport and intracellular migration, cellular architecture maintenance, cell signaling, and mitosis. Microtubules are built by the polymerization of α- and β-tubulin subunits that come together to form the αβ-heterodimer, which bind collectively in a filamentous tube-shaped structure.1 This arrangement gives rise to the formation of long protein fibers known as protofilaments. Approximately, thirteen protofilaments assemble in parallel to acquire a C-shaped protein sheet, which afterward curls around into a pipe-like structure described as microtubule. In cells, microtubules exist in a continuously dynamic state of growing and shortening through the reversible association and disassociation of αβ-tubulin heterodimers. This dynamic behavior is controlled by the exchange of one GTP molecule for GDP in the β-subunit of a microtubule end while other GDP unit is strongly bound to α-tubulin and is non-exchangeable. A microtubule with a GTP molecule at the β-end is stable and continues to produce, whereas a microtubule with the GDP molecule at the β-end is unstable and quickly depolymerize. This process and the control of microtubule lengths are vital to the appropriate implementation of the mitotic spindle in cell division.1,2 Due to the decisive role in mitotic events, microtubules give out significant drug targets for anticancer compounds. Ligands targeting tubulin are broadly known as Tubulin Binding Agents (TBAs) and comprise a wide class of chemically diverse compounds that disrupt microtubule dynamics and inhibit mitosis, ultimately leading to cell death. TBAs can be classified into four main general types with respect to their binding site on the αβ-tubulin dimer: (a) the first group includes compounds which bind to the vinca alkaloids site which is located at the interface amid two longitudinally aligned αβ-tubulin heterodimers; (b) the second group comprises compounds that bind to the colchicines site at the interface between the α- and β-subunits of the same tubulin heterodimer; (c) the third group is formed by the compounds that bind to the paclitaxel (Taxol) site on the luminal area of the β-tubulin subunit, and (d) the fourth group comprises of compounds that bind to the laulimalide site on the exterior of β-tubulin. These four binding sites are targeted by a number of structurally unrelated compounds, which limits the identification of consistent pharmacophores for TBAs binding at the specific interaction sites. Moreover, the very recent discovery of new tubulin binding domains has evidenced the versatility and still unexplored features of this protein as a receptor for anticancer compounds and has broadened the possibilities for the discovery of novel TBAs.3 From a mechanistic point of view, TBAs can be classified as either inhibitor of tubulin polymerization (microtubule destabilizing agents) or promoters of microtubule assembly and stabilization (microtubule stabilizing agents). Polymerization inhibitors typically bind to the vinca or colchicines sites, and decrease microtubule polymer mass by blocking the addition of tubulin dimers (e.g. colchicine) or by promoting a conformational change or microtubule ends that impedes further tubulin self-association (e.g. vinblastine). In contrast, polymerization promoters bind to the paclitaxel (e.g., paclitaxel and epothilones) or laulimalide (e.g. laulimalide and peloruside A) sites and increase microtubule polymer mass by promoting tubulin self-association and impeding microtubule depolymerization. Although the molecular basis for the action of TBAs is not yet fully understood, the conformational changes induced by TBA binding in the protein, are recognized as key factors for the biological activity of stabilizing and de-stabilizing agents.4,5 Over the past years, TBAs such as paclitaxel, docetaxel, vinblastine, and vincristine have been widely employed in the treatment of various kinds of cancer.6 However, severe problems such as acquired and intrinsic resistance, negative side effects, neurotoxicity, low solubility, and poor bioavailability have limited their clinical success and encourage the search for novel, more potent active species with improved therapeutic properties.7,8 Currently, a number of TBAs are in different stages of preclinical and clinical development and have entered clinical use, and more active compounds are still being discovered.9,10 Most of these species are synthetic or semisynthetic analogs of parent TBAs or are natural compounds with structures similar to known tubulin-targeting ligands.11 Nevertheless, a major challenge regarding this issue involves the discovery of new chemical scaffolds capable of efficiently and adequately interacting with tubulin to overcome the current limitations of TBAs. To address this need, computer-aided drug design methods have emerged as valuable tools for improving the efficacy and reducing the costs associated with the synthesis and discovery of new TBAs as well as for enhancing the current understanding of the molecular basis underlying the biological activity of these compounds.12 To date, a number of ligand-based (e.g. QSAR, COMFA, and pharmacophore modeling)13 and structure-based (e.g. molecular docking and molecular dynamics),14,15 computational studies have been aimed at the elucidating binding modes of TBAs and the molecular features accountable for their effective interaction with tubulin. Nevertheless, the structural diversity of TBAs and the insufficient knowledge about the three-dimensional details of the TBA-tubulin association have traditionally hindered computational approaches from leading to significant advances in the development of novel active ligands. However, in the past few years, a number of high-resolution three-dimensional structures of tubulin complexes with a series of TBAs have been released,16,17 providing a valuable resource for the discovery of novel ligands targeting tubulin. Surprisingly, despite that the number high-quality crystalline structures for TBA tubulin complexes have augmented fast, there are very few reports dealing with the rational design of novel TBAs using structure-based computational methods18,19 which constitute a unique research opportunity and is the main goal of the present study.
2. Methodology
The X-ray crystallographic 3D structure of the refined αβ-tubulin dimer was obtained from RCSB protein data bank (PDB ID:1JFF). The chemical structures of the ligands have been taken from the reported entries.20 PRIME and Protein Preparation wizards of the SCHRODINGER suite have been utilized for the correction and preparation of protein structure as have been reported in our earlier communications.21–23 The β-subunit of the microtubule contains the taxol binding site, which was retained while the other subunit and substrates were deleted. Ligands (molecules 1–12 and taxol) were optimized for their minimum energy configurations. Various conformers of the ligands were generated and subsequently, their various ionization states, stereochemistries, tautomers and ring conformations were produced. After the electron affinity grid generation, the docking of ligand molecules was performed within the classical taxol binding site using standard precision (SP), extra precision (XP) and induced fit docking (IFD) modes of the GLIDE.24–27 To get better geometries of the docking configurations, post-docking minimizations have also been carried out. Docking scores and binding energy calculations were calculated on the basis of final docking results. Better docked complexes of four better ligands, as screened through Glide docking, have been taken for the MD simulation studies of 50 ns each. Methodology for molecular simulation has been adopted as in our earlier paper.23,28
3. Results and discussion
Molecular modeling studies and X-ray crystallographic structures reveal the presence of amino acid residues surrounding and interacting with the taxol binding site of the β-tubulin, where molecular docking of the twelve natural extracts: cevipabulin (1), eribulin (2), ombrabulin (3), 2-methoxyestradiol (4), indibulin (5), ixabepilone (6), discodermolide (7), cyclostreptin (8), eleutherobin (9), laulimalide (10), noscapine (11), estramustine (12) along with taxol (TXL) (Fig. 1) has been carried out. Cevipabulin (1), a semisynthetic molecule, shows taxane site binding capability and works against a variety of tumors including those resistant to paclitaxel (Taxol).29 Developed from sea sponge ‘Halichondria Okadai’, eribulin (2), is used in the treatment of advanced breast cancer.30 Ombrabulin (3), a combretastatin A-4 derivative, isolated from Combretum caffrum (a South African bushwillow tree) shows promising antitubulin activities but now it has been discontinued at phase III clinical trial level.31 2-Methoxyostradiol (4) is a natural metabolite of estradiol which prevents the formation of new blood vessels that are required for the growing of tumors.32 This is being developed as microtubule inhibitor effective against prostate cancer. In order to overcome neurotoxicity as exhibited by several anticancer drugs, indibulin (5) is being developed as microtubule inhibitor.33 Ixabepilone (6), isolated from myxobacterium Sorangium cellulosum, is efficient in the healing of breast cancer.34 Discodermolide (7) is a poly hydroxylated lactone, isolated from marine sponge ‘Discodermia dissoluta’ has been found to stabilize microtubule.35 The first microtubule-stabilizing agent cyclostreptin (8) was discovered the whose mechanism of action involve the formation of a covalent bond with tubulin.36 Eleutherobin (9) is a novel natural product isolated from a marine soft coral is enormously powerful for inducing tubulin polymerization similar to that of Taxol.37 Isolated from a marine sponge, laulimalide (10) is structurally distinct from taxanes. However, it binds to tubulin and enhances microtubule assembly and stabilization.38 Noscapine (11), an isoquinoline alkaloid found in opium latex has been introduced as an anti-mitotic agent which might be effective in the case of resistance to other anti-cancer drugs such as paclitaxel.39 Estramustine (12) is a cytotoxic nitrogen-mustard derivative of estradiol-17b-phosphate which is accepted in the treatment of advanced prostate carcinoma. In view of the potential importance of these anticancer drugs, the molecular modeling studies and the binding capabilities within the binding site of the microtubule have been carried out.
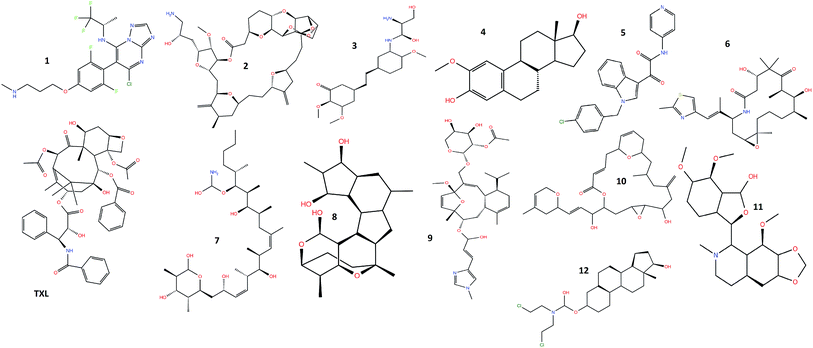 |
| Fig. 1 Chemical structure of molecules (1–12 and taxol) undertaken for the study. | |
3.1 Glide docking
The results of the standard precision (SP), extra precision (XP) and induced fit glide (IFD) docking of taxol and other twelve compounds are summarized in Table 1. It has been found that, through all the methods, taxol demonstrates the minimum docking energy values. However, glide scores of taxol docking are better through SP and XP methods while second best in the case of IFD docking. In the case of SP docking, docked glide energy for taxol is −112.580 kcal mol−1 and glide score is −8.769 kcal mol−1 respectively, while that of XP docking the values come out to be −111.340 and −10.392 kcal mol−1. The glide energy values of the best fit compounds indicate that the minimum docked energy and score for the second best compound (10) attain the values −80.409 kcal mol−1 and −7.866 kcal mol−1 respectively, through SP docking and −70.938 and −8.540 kcal mol−1 through XP docking. The third best result is exhibited by molecule 7 through SP and XP docking methods having scores −7.627 and −7.616 kcal mol−1, respectively. In the case of IFD docking, the relative strength of molecules 7 and 10 are reversed, as molecule 7 shows the best while molecule 10 demonstrate second best docking results with glide scores −14.108 and −13.803 kcal mol−1 respectively. Molecule 11 exhibits the fourth best docking score of −7.101 and −11.225 kcal mol−1 respectively, through XP and IFD methods. The glide energy through IFD is the best for taxol (−162.683 kcal mol−1) followed by compound 10 (−111.897 kcal mol−1) and compound 7 (−105.029 kcal mol−1).
Table 1 Glide scores and glide energies of the best docked complexes of compounds with 1JFF as obtained through Glide docking
Compound |
SP docking |
XP docking |
Induced fit docking |
Glide score (kcal mol−1) |
Glide energy (kcal mol−1) |
Glide score (kcal mol−1) |
Glide energy (kcal mol−1) |
Glide score (kcal mol−1) |
Glide energy (kcal mol−1) |
Taxol |
−8.769 |
−112.580 |
−10.392 |
−111.340 |
−13.314 |
−162.683 |
1 |
−6.097 |
−60.839 |
−6.123 |
−53.055 |
−9.253 |
−74.343 |
2 |
−6.574 |
−65.806 |
−5.360 |
−47.033 |
−10.064 |
−86.366 |
3 |
−6.775 |
−63.965 |
−5.928 |
−55.642 |
−10.580 |
−90.299 |
4 |
−5.386 |
−42.075 |
−4.361 |
−38.535 |
−7.815 |
−49.813 |
5 |
−5.828 |
−53.227 |
−6.251 |
−63.991 |
−8.961 |
−89.041 |
6 |
−6.728 |
−58.890 |
−5.710 |
−63.777 |
−10.108 |
−96.075 |
7 |
−7.627 |
−75.675 |
−7.616 |
−53.863 |
−14.108 |
−105.029 |
8 |
−6.064 |
−58.671 |
−6.230 |
−57.512 |
−11.075 |
−61.128 |
9 |
−5.998 |
−54.486 |
−7.408 |
−60.530 |
−9.136 |
−90.636 |
10 |
−7.866 |
−80.409 |
−8.540 |
−70.938 |
−13.803 |
−111.897 |
11 |
−5.672 |
−58.437 |
−7.101 |
−50.691 |
−11.225 |
−104.645 |
12 |
−4.105 |
−44.936 |
−4.716 |
−45.695 |
−5.977 |
−57.060 |
The docking of the molecules, within the taxol binding site of 1JFF, exhibits several interactions. On the basis of SP-docking, the best-docked complex of taxol exhibit hydrogen bonding interactions, with residues Asp26 and Gly370. XP docking demonstrates additional hydrogen bonding interactions involving Thr276 and Asp26 (Fig. 2). Residue His229 divulge aromatic π–π interactions with taxol. Hydrogen bonding interactions in the best docking pose of compound 7 (discodermolide) are demonstrated with Pro274, Thr276, Gly370, and Arg284 through Glide-SP while with Asp226, Gly225, Lys19 and Thr275 through Glide-XP docking. The best XP docking complex of molecule 10 demonstrates hydrogen bonding interactions with Asp26, Gly370, and Asp226. Residue Arg278 also interact with molecule 10 which is observed through SP docking. The SP docking of compound 11, demonstrate hydrogen-bonding interactions with residues His229 and Thr276 while through XP docking, two hydrogen bondings with Thr276. Residues Leu286, Leu371, Ala233, Pro360, Val23, Phe83, Leu219, Cys213, Phe272, Leu230, Leu275, Leu217, Pro274, and Leu227 etc. form a hydrophobic enclosure to the binding site. Polar interactions with the ligands have been observed through residues Gln282, Ser277, Thr276, His229, Ser236. Salt bridge interactions with ligands are also possible through Arg369, Arg320, Arg278, Asp26, Asp226, Glu22 and Glu27 residues which are present at the active site of the protein.
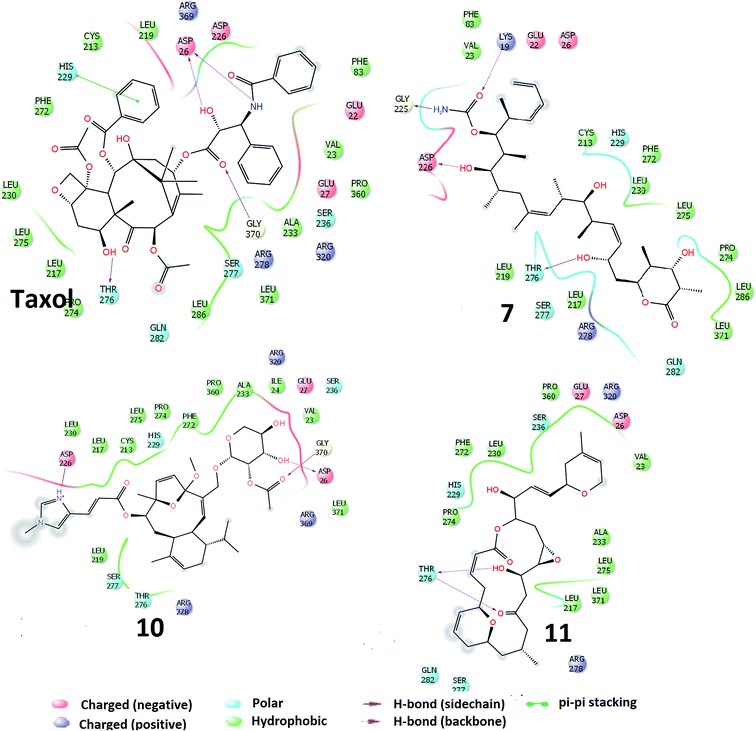 |
| Fig. 2 Interactions of the molecules (TXL, molecules 7, 10 and 11) within the conserved active sites of the microtubule as obtained through Glide-XP docking. | |
The best docking poses of the best four molecules (TXL, 7, 10 and 11) as obtained through induced fit docking are shown in Fig. 3. The interactions of molecules within the taxol binding site are similar to that of SP and XP docking modules but with some additional interactions. The earlier combined experimental and computational study indicate that discodermolide (molecule 7) exhibit binding with the luminal site as well as pore site of the microtubule.40,41 It has been reported through AUTODOCK4.2 docking with 1JFF that discodermolide show hydrogen bonding interactions with Lys218, Phe214, Val93, Phe94, Thr220 and His229.40 However, in present work, it has been observed that residues Arg278, Pro274, Thr276, Glu27, Gly370, and Asp26 interacted with the molecule 7 via hydrogen bonding interactions whereas Leu275, Leu217, Pro27, Val23, Pro360, Ala233, Phe272, Leu230 and Leu371 form hydrophobic enclosure. It has been reported that laulimalide (molecule 10) delineate greater activity of binding than peloruside A and exhibit various hydrophobic and electrostatic interactions within binding site of the β-tubulin.42 Through IFD, hydrogen bonding interactions exhibited by molecule 10 within the binding site involve residues Lys372, Gln281, Arg284, Gln282, Gly370, and Asp26 while hydrophobic interactions are demonstrated by residues Tyr283, Ala285, Pro274, Leu371, Pro360, Val23, Ala233, Phe272, Leu275, Leu230, Leu217, Lue286. Noscapine (molecule 11) and its derivatives show tubulin binding activity and considerably affect the tubulin polymerization.43 In the best docking pose of molecule 11, six hydrogen bonding interactions are exhibited involving residues Pro274, Arg278, Gln282, Thr276, Ser277, and His229. Residues Leu275, Ala233, Pro274, Phe272, Pro360, Leu371, Ile212, Met302, Leu230, and Leu217 are responsible for the hydrophobic contacts. Similar types of hydrogen bonding and hydrophobic interactions have been found in the IFD docking complexes of Taxol with 1JFF. In addition, aromatic π–π interactions are exhibited involving residues Arg320 and His229.
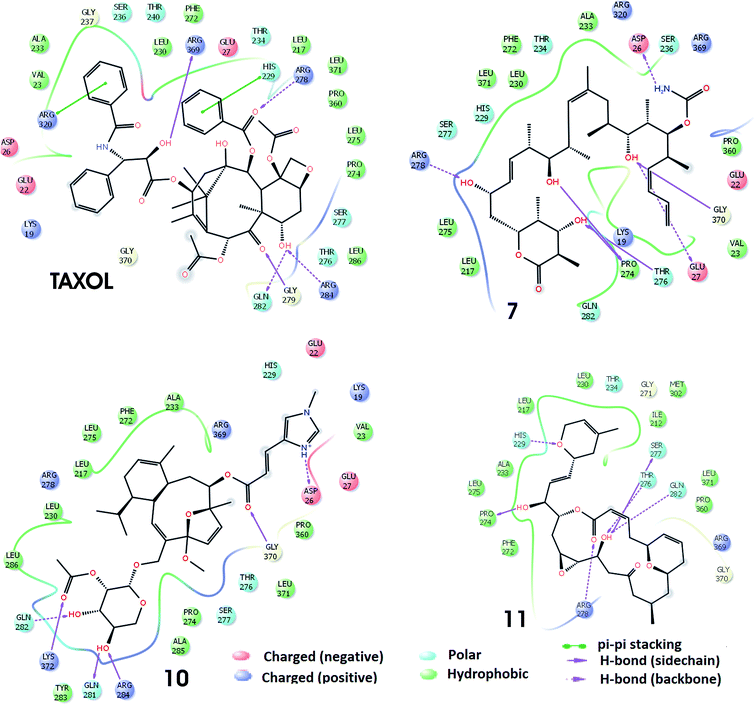 |
| Fig. 3 Various noncovalent interactions, as obtained through induced fit docking, in the best docking complexes of the four molecules (TXL, 7, 10 and 11) within the taxol binding site of the microtubule. | |
3.2 Molecular dynamics simulation
On the basis of docking results, the better-docked complexes have been extracted to study their conformational stability and time-dependent behavior. The molecular dynamics simulation of the better-docked poses of molecules: Taxol, molecule 7, molecule 10, and molecule 11, as obtained through XP docking, have been run for 50 ns using DESMOND. The equilibration of the dynamics was examined with the help of MD trajectory. Snapshots were recorded at a periodic interval of 0.5 ns and placed over on the initial structure. Overall, the physical manifestation of the MD simulated structures strongly bears a resemblance to that of the initial structure, subject to the thermal fluctuations. Fully convergences of the simulated complexed structures were confirmed by calculating the RMS deviations between the average structures and the initial structure for all the frames in trajectory, in the case of the four complexes (Fig. 4). Overall, considering slight changes in comparison to the initial structure, in addition to the fraying of the outermost atoms, over the course of the simulation, the small heavy atom RMSDs indicate that the structures were significantly converged.
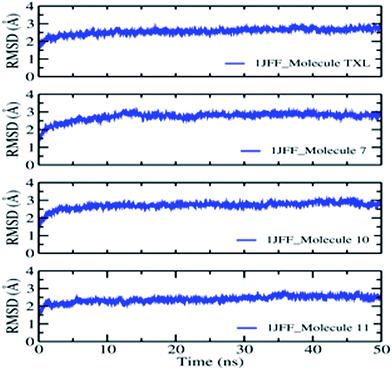 |
| Fig. 4 Average RMSD of heavy atoms of the complexes during MD simulation. | |
RMSD of the heavy atoms during MD simulation of 1JFF complexed with TXL show small fluctuations in the beginning which increase smoothly from 1.00 Å to 1.98 Å up to 12 ns dynamics. After a small variation at 12.0 ns, the RMS deviation becomes almost constant and it rises from 1.98 Å up to 2.12 Å at the end of the 50.0 ns dynamics. For the duration of dynamics from 41 ns to 45 ns, the RMSD acquires smaller values of about 1.89 Å. The results indicate reasonably small fluctuations centered on the average RMSD about a distance of ±0.04 Å. The RMSDs of the atoms of the ligands have been estimated after the alignment of the protein backbone of the reference structure. Heavy atom RMSD of Taxol shows fluctuations in the beginning and attains an almost constant value of 1.5 Å after the simulation of 14.0 ns dynamics and remains constant up to 28.5 ns. After the dynamics of 14.0 ns, RMSD of the ligand becomes about 1.0 Å which is maintained up to 48.0 ns dynamics. However, values observed are appreciably lesser than those of the RMSD of the protein. Thus, it is likely that the fluctuations of the ligand atoms continue to be fall from its initial binding site. As usual, N- and C-terminals fluctuate more than the other parts of the protein structure which are observed by the average RMS fluctuations of the protein residues. α-Helices and β-strands are usually stiffer than the formless part of the protein, and accordingly, alter less than the loop county. Interactions of the binding site residues with the ligands have been examined throughout the simulation. These interactions can be grouped by their types and go over as shown in the plot (Fig. 5). There are four types of protein–ligand interactions: hydrogen bonds, polar, water bridges, and hydrophobic interactions. The stacked bar charts of the interactions with various residues of the binding site are normalized over the course of the simulation. Fig. 5 demonstrate that residue ASP26 makes the highest number of interactions with taxol which remains conserved during the simulation period. Other residues which make interactions during the course of 50.0 ns dynamics with more than 50% simulation period are; His 229, Pro274, Thr276, and Pro360. Because of their strong specificity, drug metabolism, and adsorption, hydrogen bonds are important in protein–ligand binding and drug designing. During the course of the simulation, residues Asp26, His229, Pro274, Thr276, Gln282, Arg369 and Gly370 provide hydrogen bonding interactions with the Taxol (ligand). Residue Asp26 provide the most prominent and strong hydrogen bonding interaction. Hydrophobic amino acid residues are responsible for hydrophobic contacts when exposed to the aromatic or aliphatic group on the ligand e.g. π–cation, π–π and other nonspecific interactions having hydrophobic side chain within 3.6 Å of ligand. Val23 and Pro360 residues form the hydrophobic interfaces for about 50% of the simulation time. Other residues which outline hydrophobic contacts during MD simulation are Leu217, Leu219, His 229, Leu230, Phe272, Leu275, Arg278, and Leu371. When a water molecule mediates hydrogen bond, it is called as water bridge interaction. Residues Asp26, His229, Pro274, Thr276, Ser277, Arg278, Gln282, Arg369, Gly370 and Ser374 form water bridges between protein and ligands.
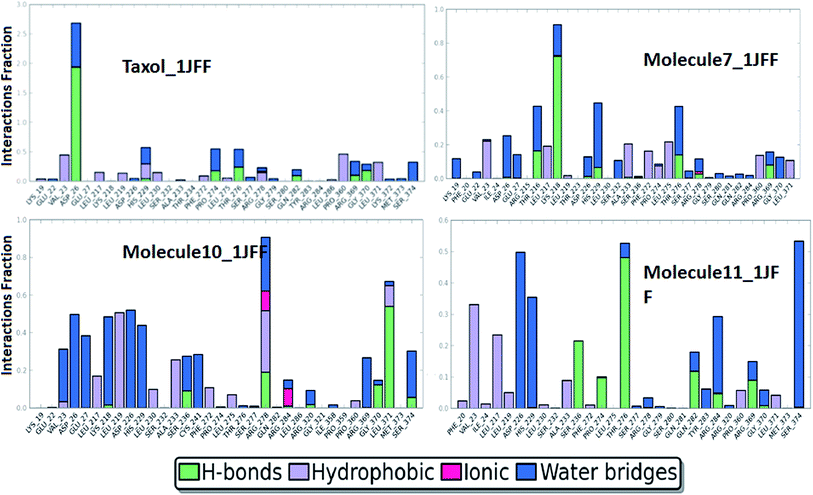 |
| Fig. 5 Normalized stacked bar chart representation of interactions and contacts over the course of the MD trajectory. Values > 1.0 are possible as some residues make multiple contacts of the same subtype with ligands. | |
The behaviors and interactions of proteins and ligands in the 1JFF_molecule 7 complex, during the course of dynamics, can be observed from Fig. 4 and 5. It is observed that RMS deviation increases in the beginning from 1.0 Å to 2.1 Å at 14.0 ns. After some fluctuations at about 14 to 15 ns, RMSD attains an average value of 2.2 Å with small oscillations (±0.05 Å) around it. RMSDs of Cα, side chain, backbone atoms, and ligands fit on protein and ligand itself attribute a constant variation within the stability limit during 50 ns dynamics simulations. An interactions fraction diagram exhibit that the residue Lys218 (interactions fraction 0.95) is attributed to the largest number of interactions with the ligand during the course of the simulation. About 80% of the interactions with Lys218 are due to hydrogen bonding. Residues Thr216, His 229, Thr276 have interactions fraction greater than 0.4. Other residues which have interactions more than 30% of the simulation period are Val23, Asp26, Leu217, Ala233, Phe272, and Leu275. Major sources of noncovalent interactions are hydrophobic and water bridges.
The RMSD observation of heavy atoms of the 1JFF_molecule 10 complex demonstrates very small oscillations around the averaged structure and shows a very smooth and slight increment during the course of the dynamics. After equilibration, the heavy atom RMSD acquires the value of 2.4 Å which remain constant up to 38.3 ns dynamics. Some fluctuation is observed during the course of dynamics of 39.2 to 48.1 ns, afterword it attains the same constant value 2.4 Å. The RMSDs of Cα-atoms, backbone atoms, and side chain atoms remain almost constant throughout the whole dynamics of 50 ns with average values of 2.0 Å, 2.1 Å, and 2.4 Å respectively. On the whole, the time-averaged fluctuations of protein backbone are analogous to protein side chain. Protein interactions with the ligand have been monitored and normalized by a timeline depiction over the course of the trajectory and have shown in Fig. 4. The interactions like H-bonds, ionic, hydrophobic, and water bridge interactions have been illustrated in Fig. 5. The crucial interactions have been demonstrated by Arg278 and Leu371 with interactions fraction 0.9 and 0.7 respectively. A hydrogen bond is a prominent interaction with residue Leu371 whereas Arg278 interacts mainly through hydrophobic interactions and water bridges. Other residues which maintain interactions during the course of dynamics (with interactions fraction larger than 0.3) are Val23, Asp26, Glu27, Lys218, Leu219, Asp226, His229, Ala233, Ser236, Cys241, Arg369, and Ser374. Ionic interactions are exhibited by Arg278 and Arg284 residues of the binding site.
To observe the average change in the dislocation of an assortment of atoms RMS deviations of heavy atoms, Cα atoms, backbone and side chain atoms have also been studied during the molecular dynamics simulation of the 1JFF_molecule 11 complex for 50 ns. The RMSDs of Cα, as well as all heavy atoms and backbone atoms, follow the same path throughout the dynamics simulation. Starting from 1.6 Å at 2.0 ns, it has obtained the value of 1.8 Å at 36 ns dynamics. However, an average of 1.8 Å RMSD, with small oscillations around it, has been found throughout the rest of the dynamics. Similar is the situation with side chain RMSD. It starts with 2.8 Å at 2.0 ns which smoothly increases up to 2.9 Å at 36 ns dynamics which remains constant right through the rest of dynamics. Residues Asp226, Thr276, and Ser374 show interactions with the ligand with interactions fraction greater than 0.5. Hydrogen bonding interaction is shown by Residue Thr276 whereas residues Asp226 and Ser374 interact through water bridges. Other residues which maintain significant interactions with ligand throughout the dynamics are Val23, Leu217, His229, Ser236, Pro274, Gln282, Arg284, and Arg369.
3.3 ADME/T prediction
In order to ensure success at the clinical trial level, Absorption, Distribution, Metabolism and Excretion (ADME) properties of the compounds under study have been calculated using QikProp3.5 module of the SCHRODINGER (Table 2). It has been observed that all the molecules have ADME parameters within the range of 95% drugs, and show violations of Lipinski's ‘rule of 5’ varying from 0 to 2 except taxol which has three number of violations. Molecules violating more than two or three violations of the ‘rule of five’ indicate difficulty with the bioavailability of the compounds. Molecule 6 has a large value of octanol–water partition coefficient while all other molecules possess log
P values within the range of 95% drugs. Toxicity of the molecules has been predicted using Tox-Tree software which indicates that taxol is highly toxic whereas other molecules are comparatively less toxic.23
Table 2 Some peculiar molecular predictions of the ligands through Qikprop [MW: molecular weight; n(OHNH): number of hydrogen-bond donors (OH and NH groups); n(ON): number of hydrogen bond acceptors (O and N atoms); n(Rotb): number of rotatable bonds; PSA: van der Waals polar surface area; volume: molecular volume; log
P: octanol–water partition coefficient; n(Metab): number of primary metabolites; Abs.: % human oral absorption in GI (±20%); n(Viol5): number violations of rule of 5
Molecule |
MW |
n(OHNH) |
n(ON) |
n(Rotb) |
PSA |
Volume |
log P |
n(Metab) |
Abs |
n(viol5) |
TXL |
866.17 |
9.5 |
22.7 |
32.0 |
181.9 |
2341.408 |
1.793 |
3 |
42 |
3 |
1 |
839.11 |
5.0 |
202 |
13.0 |
98.0 |
2357.791 |
2.695 |
2 |
43 |
2 |
2 |
478.93 |
5.0 |
10.2 |
7.0 |
43.9 |
1250.000 |
−0.533 |
2 |
47 |
0 |
3 |
735.95 |
4.0 |
17.8 |
19.0 |
132.9 |
1995.350 |
21.692 |
10 |
64 |
2 |
4 |
418.57 |
5.0 |
12.7 |
14.0 |
104.2 |
1240.507 |
−0.271 |
5 |
54 |
0 |
5 |
308.46 |
2.0 |
5.1 |
3.0 |
49.9 |
956.052 |
2.540 |
2 |
100 |
0 |
6 |
414.03 |
4.0 |
8.4 |
8.0 |
57.3 |
1119.586 |
0.781 |
4 |
57 |
0 |
7 |
605.89 |
8.0 |
11.2 |
27.0 |
141.9 |
1911.990 |
3.480 |
4 |
47 |
2 |
8 |
516.77 |
5.0 |
11.3 |
22.0 |
101.8 |
1511.776 |
1.954 |
5 |
41 |
2 |
9 |
378.50 |
3.0 |
7.6 |
3.0 |
79.0 |
1121.580 |
2.153 |
3 |
90 |
0 |
10 |
672.89 |
5.0 |
18.6 |
16.0 |
132.4 |
1950.882 |
1.385 |
3 |
35 |
2 |
11 |
528.72 |
3.0 |
12.9 |
23.0 |
110.9 |
1516.377 |
2.411 |
5 |
76 |
1 |
12 |
427.53 |
1.0 |
13.9 |
4.0 |
69.4 |
1138.376 |
0.021 |
1 |
83 |
0 |
4. Conclusion
Here, we have used computational methods for evaluating the effect of compounds binding to tubulin and examined the stability and strength by monitoring the conformational variations. A series of compounds which are capable of acquiring comfort zone with microtubule were selected and extensive docking was carried out. The screening of a variety of botanical species and organisms from marine origins has provided adequate novel anti tubulin agents. With the help of molecular dynamics simulation, we attempted here to give some anti-tubulin drugs which can be a substitute for microtubule binding agents. A set of twelve different molecules along with taxol were selected and interacted with microtubule within the taxol binding site. Molecular docking simulation has been performed through SP, XP and induced fit docking modules of GLIDE. Better docked complexes have been subjected to molecular dynamics simulation and the results have been analyzed. On the basis of docking energy and interactions, apart from taxol molecule, molecules 7 (discodermolide), 10 (laulimalide) and 11 (noscapine) were found to have a better tendency of binding with the microtubule. In order to further validate binding capabilities, molecular dynamics simulations of the best-docked complexes were carried out for 50 ns using DESMOND. Through simulation, it has been observed that almost all the complexes show interactions with His229 and Thr276 residues throughout the dynamics. Average RMSD analysis and dynamical pathway observations indicate that molecules 7 and 10 transit quickly to a comfort zone and remain stable throughout the dynamics. Taxol shows the best interactions and binding capability within the active site than others. Apart from Taxol, molecules 7 and 10 show better stability than other molecules, undertaken for study. With the help of these observed outcomes, we are of the idea that these compounds, being less toxic than taxol, perhaps may be considered as a better substitute for taxol. Moreover, this study would help in the identification of compounds, being less toxic than taxol, but with comparable binding capabilities and also strengthen the idea of rational drug designs and syntheses of ligands for the development of compounds as better substitute of taxol.
Acknowledgements
U. Yadava thanks, University Grants Commission, New Delhi, India for the award of Raman Fellowship for post-doctoral studies in the USA.
References
- J. J. Field, J. F. Diaz and J. H. Miller, Chem. Biol., 2013, 20, 301 CrossRef CAS PubMed.
- A. S. Negi, Y. Gautam, S. Alam, D. Chanda, S. Luqman, J. Sarkar, F. Khan and R. Kanwar, Bioorg. Med. Chem., 2015, 23, 373 CrossRef CAS PubMed.
- T. Mahaddalkar, C. Suri, P. K. Naik and M. Lopus, Eur. J. Pharmacol., 2015, 760, 154 CrossRef CAS PubMed.
- A. E. Porta, K. Bargesten, D. Zurwerra, J. J. Field, J. F. Diaz, K. H. Altmann and N. O. Steinmetz, Science, 2013, 339, 587 CrossRef PubMed.
- H. Yajima, T. Ogura, R. Nitta, Y. Okada, C. Sato and N. Horokawa, J. Cell Biol., 2012, 198, 315 CrossRef CAS PubMed.
- A. K. Singhal, A. Garg and D. Aggarwal, Int. J. Pharm., 2002, 235, 179 CrossRef.
- A. T. Oosterom and D. Schriivers, Anti-Cancer Drugs, 1995, 6, 356 CrossRef PubMed.
- M. Kavallaris, Nat. Rev. Cancer, 2010, 10, 194 CrossRef CAS PubMed.
- C. F. Brogdon, F. Y. Lee and R. M. Canetta, Anti-Cancer Drugs, 2014, 25, 599 CrossRef CAS PubMed.
- T. Tuyen, T. Ngoc, C. G. Reimers, B. Schoelermann, B. Stanitzki, T. Henkel, H. Waldman and S. Ziegler, Bioorg. Med. Chem., 2014, 22, 5110 CrossRef PubMed.
- M. Gordaliza, Clin. Transl. Oncol., 2007, 9, 767 CrossRef CAS PubMed.
- W. L. Jorgensen, Science, 2004, 303, 1813 CrossRef CAS PubMed.
- M. Botta, S. Forli, M. Magnani and F. Manetti, Molecular Modeling Approaches to Study the Binding Mode on Tubulin of Microtubule Destabilizing and Stabilizing Agents, in Tubulin-Binding Agents: Synthetic Structural and Mechanistic Insights, ed. T. Carlomagno, 2009, p. 279 Search PubMed.
- S. Ducki, G. Mackenzie, N. J. Lawrence and J. P. Snyder, J. Med. Chem., 2005, 48, 457 CrossRef CAS PubMed.
- V. Jimenez, J. B. Alderete and K. R. Navarrete, J. Biomol. Struct. Dyn., 2015, 33, 789 CAS.
- A. F. Porta, K. Bargesten, P. T. Northcote, M. Marsh, K. H. Altmann, J. H. Miller, J. F. Diaz and M. O. Steinmetz, Angew. Chem., Int. Ed., 2014, 53, 1621 CrossRef PubMed.
- T. Tuyen, T. Ngoc, C. G. Reimers, B. Schoelermann, B. Stanitzki, T. Henkel, H. Waldman and S. Ziegler, Bioorg. Med. Chem., 2014, 22, 5110 CrossRef PubMed.
- A. T. Ayoub, M. Klobukowski and J. Tuszynski, J. Mol. Graphics Modell., 2013, 44, 188 CrossRef CAS PubMed.
- R. Cao, M. Liu, M. Yin, Q. Liu, Y. Yang and H. Huang, J. Chem. Inf. Model., 2012, 52, 2730 CrossRef CAS PubMed.
- C. Dumontet and M. A. Jordan, Nat. Rev. Drug Discovery, 2010, 9, 790–803 CrossRef CAS PubMed.
- W. Sherman, T. Day, M. P. Jacobson, R. A. Friesner and R. Farid, J. Med. Chem., 2006, 49, 534 CrossRef CAS PubMed.
- U. Yadava, M. Singh and M. Roychoudhury, J. Biol. Phys., 2013, 39, 419–438 CrossRef CAS PubMed.
- U. Yadava, H. O. Gupta and M. Roychoudhury, J. Biol. Phys., 2015, 41, 117–133 CrossRef CAS PubMed.
- Glide, version 5.8, Schrödinger, LLC, New York, 2014 Search PubMed.
- T. A. Halgren, R. B. Murphy, R. A. Friesner, H. S. Beard, L. L. Frye, W. T. Pollard and J. L. Banks, J. Med. Chem., 2004, 47, 1750 CrossRef CAS PubMed.
- R. A. Friesner, R. B. Murphy, M. P. Repasky, L. L. Frye, J. R. Greenwood, T. A. Halgren, P. C. Sanschagrin and D. T. Mainz, J. Med. Chem., 2006, 49, 6177–6196 CrossRef CAS PubMed.
- U. Yadava, H. O. Gupta and M. Roychoudhury, Med. Chem. Res., 2012, 21(9), 2162 CrossRef CAS.
- R. K. Yadav and U. Yadava, Mol. Simul., 2016, 42, 25 CrossRef CAS.
- S. Ayral-Kaloustian, N. Zhang and C. Beyer, Methods Find. Exp. Clin. Pharmacol., 2009, 31(7), 443–447 CrossRef CAS PubMed.
- P. G. Morris, Anticancer Drugs, 2010, 21(10), 885 CrossRef CAS PubMed.
- K. Hori, S. Saito and K. Kubota, Br. J. Cancer, 2002, 86(10), 1604 CrossRef CAS PubMed.
- N. J. Lakhani, M. A. Sarkar, J. Venitz and W. D. Figg, Pharmacotherapy, 2003, 23(2), 165 CrossRef CAS PubMed.
- . A. Wienecke and G. Bacher, Cancer Res., 2009, 69(1), 171 CrossRef PubMed.
- J. A. Sparano, E. Vrdoljak, O. Rixe, B. Xu, A. Manikhas, C. Medina, S. Crocamo, V. Costa, J. Ro, G. Rubio, M. Rondinon, G. P. Manga, R. Peck, V. Poulart and P. Conte, J. Clin. Oncol., 2010, 28, 3256 CrossRef CAS PubMed.
- A. Arefolov and J. S. Panek, J. Am. Chem. Soc., 2005, 127(15), 5596 CrossRef CAS PubMed.
- E. Calvo, I. Barasoain, R. Matesanz, B. Pera, E. Camafeita, O. Pineda, E. Hamel, C. D. Vanderwal, J. M. Andreu, J. A. López and J. F. Díaz, Biochemistry, 2012, 51(1), 329 CrossRef CAS PubMed.
- B. H. Long, J. M. Carboni, A. J. Wasserman, L. A. Cornell, A. M. Casazza, P. R. Jensen, T. Lindel, W. Fenical and C. R. Fairchild, Cancer Res., 1998, 58(6), 1111 CAS.
- J. Liu, M. J. Towle, H. Cheng, P. Saxton, C. Reardon, J. Wu, E. A. Murphy, G. Kuznetsov, C. W. Johannes, M. R. Tremblay, H. Zhao, M. Pesant, F. G. Fang, M. W. Vermeulen, B. M. Jr Gallagher and B. A. Littlefield, Anticancer Res., 2007, 27(3B), 1509 CAS.
- M. Mahmoudian and P. Rahimi-Moghaddam, Recent Pat. Anti-Cancer Drug Discovery, 2009, 4, 92 CrossRef CAS PubMed.
- A. Canales, J. Rodríguez-Salarichs, C. Trigili, L. Nieto, C. Coderch, J. M. Andreu, I. Paterson, J. Jimenez-Barbero and J. F. Díaz, ACS Chem. Biol., 2009, 6, 789 CrossRef PubMed.
- A. Canales, R. Matesanz, N. M. Gardner, J. M. Andreu, I. Paterson, J. F. Diaz and J. Jimenez-Barbero, Chem.–Eur. J., 2008, 14, 7557 CrossRef CAS PubMed.
- C. D. Churchill, M. Klobukowski and J. A. Tuszynski, Chem. Biol. Drug Des., 2015, 86(2), 190 CAS.
- R. C. Mishra, S. R. Gundala, P. Karna, M. Lopus, K. K. Gupta, M. Nagaraju, D. Hamelberg, V. Tandone, D. Pandag, M. D. Reidh and R. Aneja, Bioorg. Med. Chem. Lett., 2015, 25(10), 2133 CrossRef CAS PubMed.
|
This journal is © The Royal Society of Chemistry 2017 |
Click here to see how this site uses Cookies. View our privacy policy here.