DOI:
10.1039/C6RA27357B
(Paper)
RSC Adv., 2017,
7, 52923-52929
Metabolomics biomarker analysis of threatened abortion in polycystic ovary syndrome: a clinical discovery study†
Received
26th November 2016
, Accepted 12th September 2017
First published on 15th November 2017
Abstract
Metabolomics is the comprehensive assessment of endogenous metabolites of a biological system in a holistic context. Threatened abortion in polycystic ovary syndrome (PCOS) is a heterogeneous disorder with a miscarriage rate of up to 30–50% in women. However, metabolic changes regulated by threatened abortion in women with polycystic ovary syndrome are not clearly understood. Current pregnancy screening tools to identify women with PCOS that are at risk of threatened abortion lack both specificity and sensitivity. In this study, using an advanced metabolomics platform based on ultra-performance liquid chromatography quadrupole time-of-flight mass spectrometry (UPLC-QTOF-MS), we found that pregnancy significantly altered the profile of metabolites in the plasma of women with PCOS. Mothers with PCOS and healthy controls could be clustered into two distinct groups based on multivariate statistical analysis. The current metabolomics approach based on UPLC-QTOF-MS indicated 7 ions (6 in the positive mode and 1 in the negative mode) as differentiating metabolites which, to some extent, contribute to the progress of threatened abortion. All these different metabolites led to abnormalities of the fatty acids, lipid metabolism and beta-oxidation of fatty acids in mothers with PCOS. This study was designed to explore the holistic metabolomic profile of threatened abortion as a result of PCOS. To our knowledge, this work is the first report using a metabolomic approach to propose metabolic changes in plasma in the threatened abortion of women with PCOS. Thus, our method is an efficient procedure for the pathogenesis of threatened abortion with mothers with PCOS.
1. Introduction
Polycystic ovary syndrome (PCOS) is the most common cause of anovulatory infertility,1 which affects 5–10% of women of reproductive age,2 and may be associated with adverse pregnancy outcomes. Some studies have shown that the reproductive effects of PCOS extend to a higher risk of many adverse outcomes in pregnancy, with early pregnancy loss and gestational diabetes (GDM) having been most explored.3 However, it is difficult to assess how much of this abortion risk results from PCOS. A healthy woman and her developing fetus may face various risks caused by preternatural variations, which may lead to changes in physiology during pregnancy. However, these changes, especially those relating to the metabolism of pregnant women with PCOS, are still unexplored. In light of these reasons, we have sought to explore the metabolic differences between women with PCOS and healthy women during pregnancy to explain the cause of abortion.
Metabolomics is a powerful approach to identify organisms in the pathophysiological state and to quantify all metabolites in biological systems.4 It has been successfully used to study the changes of disease in both biofluids and tissues and has demonstrated significant potential markers in many fields such as disease diagnosis and drug discovery.5 It enables the collection of quantitative data on metabolites and facilitates global interpretation of the metabolism associated with conditions of disease,6 from diagnostic biomarkers that can lead to appropriate preventive actions. Recently, there has been a focus on the study of diseases in reproduction, such as polycystic ovary syndrome, gestational diabetes and poor pregnancy outcomes.7–9
A good deal of metabolic changes happen to the maternal body in a healthy pregnancy. In early pregnancy, these changes may be regarded as an anabolic condition to meet an increased requirement for nutrients. The effects of PCOS in pregnancy are especially obvious, and are proposed by the American College of Obstetrics and Gynecology in their amendment to the Committee Opinion on Obesity during Pregnancy published in January 2013.10 Our objective was mainly to examine the different metabolites between mothers with PCOS and healthy mothers, which may relate to abortion.
2. Materials and methods
2.1 Study participants
26 pregnant women with a history of PCOS and 26 healthy pregnant women were recruited from the Gynaecological Clinic of First Affiliated Hospital, Heilongjiang University of Chinese Medicine between April 2014 and November 2015. This study was approved by the Institute of Ethics Committee of the First Affiliated Hospital, Heilongjiang University of Chinese Medicine (HZYLL201200401). All participants gave written informed consent at the time of collection for the study. All the subjects enrolled were aged between 20 and 35 years and at 6–8 weeks gestation. All healthy pregnant women had no prior history of abortion in the control group. Pregnant women with PCOS met the European Society for Human Reproduction and Embryology/American Society for Reproductive Medicine criteria for PCOS diagnosis from the Rotterdam meeting in 2003, which require at least two of the following features:11 oligo- or anovulation, clinical and/or biochemical hyperandrogenism, polycystic ovaries by ultrasound examination after excluding other related disorders (congenial adrenal hyperplasia, Cushing’s syndrome, androgen secreting tumors and thyroid disease), and simultaneously conforming with the diagnostic criteria of threatened abortion, which was based on obstetrics and gynecology.12 The detailed diagnostic criteria of threatened abortions are as follows: ① history: history of menopause or reaction to pregnancy; ② symptoms: mainly related to backache, stomach distention and pain, or accompanied by a small amount of vaginal bleeding; ③ gynecological examination: disinfection examination, visible unopened cervix, uterine size and menopause in line with what was expected; ④ laboratory examination: the urine pregnancy test is positive, the blood progesterone and β-HCG levels are checked during pregnancy with tips; B ultrasound clew intrauterine pregnancy, or gestational sac is complete, or the original cardiovascular pulsation can be seen.
2.2 Sample and clinical biochemical data
Blood samples were collected and detected for all the subjects in the study at 6–8 weeks of pregnancy. Fasting venous blood samples were collected in the morning before breakfast. These blood samples were centrifuged (3000g, 4 °C, 10 min) within 30–60 min of collection, and supernatants were collected and stored at −80 °C until analysis. After sample collection, all subjects underwent the following anthropometric measurements: height, weight, waist circumference and hip circumference. Clinical biochemical index assessment included serum levels of estradiol (E2), progesterone (P), human chorionic gonadotropin (HCG), testosterone (T), dehydroepiandrosterone sulfate (DHEAS), androstenedione (AND), sex hormone binding globulin (SHBG), fasting insulin (FINS), fasting blood glucose (FBG), total cholesterol (TC), triglyceride (TG), high density lipoprotein (HDL), and low density lipoprotein (LDL).
2.3 Metabolomics analysis
All serum samples were thawed at 4 °C for several hours. A 50 μl sample was deproteinized with 200 μl methanol including 12 internal standards (carnitine C2:0-d3, carnitine C10:0-d3, carnitine C16:0-d3, LPC 19:0, FFA 18:0-d3, FFA 16:0-d3, tryptophan-d5, cholic acid-d4, phenylalanine-d5, chenodeoxycholic acid-d4, leucine-d3 and valine-d8), vortexed (30 s), then centrifuged (14000g, 4 °C, 10 min), and the supernatant was extracted and dried. The samples were re-dissolved in 50 μl of acetonitrile/water (2
:
8) and analyzed by an ultra-high performance liquid chromatography (UPLC) system coupled with Q Exactive HF (Thermo Fisher Scientific, Rockford, IL, USA) mass spectrometry.
For the ESI positive ion mode, the metabolites’ separation was performed on a 2.1 × 100 mm Waters BEH 1.7 μm C8 column (Waters, Ireland), and the mobile phase contained water with 0.1% formic acid (A) and acetonitrile with 0.1% formic acid (B). The gradient program started with 10% B and was kept for 1 min, it linearly increased to 40% B in 5 min, then changed to 100% B in 12 min and was held for 5 min, and it finally recovered to 10% B. For the ESI negative ion mode, the metabolite separation was performed on a 2.1 × 100 mm ACQUITY UPLC HSS 1.8 μm T3 column (Waters, Ireland), and the mobile phase contained a 6.5 mM ammonium bicarbonate water solution (C) and a 6.5 mM ammonium bicarbonate in 95% methanol and water (D). The gradient program was 0% D for 1 min, it then increased to 40% D in 2 min, and then linearly changed to 100% D within 13 min and was held for 6 min, finally going back to 0% D. Both in positive and negative ion modes, the flow rate was 0.35 ml min−1, the column temperature was kept at 50 °C and the injection volume was 5 μl.
Mass spectrometry detections were operated in the positive ion mode (full scan mode from m/z 80–1200) with a spray voltage of 3.50 kV, and in the negative ion mode (full scan mode from m/z 70–1100) with a spray voltage of 3.00 kV. The capillary temperature was 300 °C and the auxiliary gas heater temperature was 350 °C. The sheath gas flow rate and the auxiliary gas flow rate were 45 and 10 (in arbitrary units). Full scan mode was adopted with the resolution at 12 × 104.
2.4 Pattern recognition and pathway analysis
All of the data were normalized to the summed total ion intensity per chromatogram, and the resultant data matrices were imported into the SIMCA-P software for pattern recognition analysis. Potential markers of interest were extracted from loading plots constructed following the analysis with OPLS-DA, and markers were chosen based on their contribution to the variation and correlation within the data set. With regard to the identification of biomarkers, the ion spectrum was matched with the structure message of metabolites acquired from available biochemical databases, such as HMDB (http://www.hmdb.ca/), METLIN (http://metlin.scripps.edu/) and MassBank (http://www.massbank.jp/). The reconstruction, interaction, and pathway analysis of potential biomarkers was performed with the MetPA software based on the above database sources to identify metabolic pathways.
2.5 Statistical methods
The analyses of all the clinical data and metabolomics results were performed with SPSS 19.0 version software. Measurement data was expressed with the percentage and mean ± standard deviation (
± s), respectively. T-tests were used to test the differences between the groups, and P < 0.05 was considered as statistically significant.
3. Results
3.1 Clinical characteristics
Clinical characteristics for pregnant subjects with PCOS and those for the controls are shown in Table S1.† The control patients and pregnant patients with PCOS were well-matched in terms of age and gestation week, and there were no significant differences in relation to the BMI and waist–hip ratio. The rates of spontaneous abortion occurrences in the PCOS group were higher compared to those of the control group (26.92% vs.7.69%). The risk of miscarriage increased for women with PCOS. In addition, we also observed a higher rate of pregnancy complications in women with PCOS when compared to the control group, such as pre-term deliveries (28.57% vs. 4.1%) and gestational diabetes mellitus (33.33% vs. 4.1%). However, we found that there were no significant differences in gestational hypertension between the two groups.
3.2 Comparison of the biochemical index
From the biochemical data (Table S1†), blood hormone and blood lipids of pregnant patients with PCOS showed evident differences compared with those of the control group. Compared with the control subjects, pregnant patients with PCOS showed an obviously lower level of E2, P, β-HCG, SHBG, and HDL and a higher level of T, AND, TG, TC and LDL. Disorders of blood glucose metabolism in pregnant patients with PCOS compared with the control group were not indicated.
3.3 Metabolic profiling analysis
From the metabolic profiling analysis using the UPLC-MS conditions described in Section 2.4, the representative Based Peak Intensity (BPI) chromatograms for the plasma samples from pregnant patients with PCOS and the control groups in positive and negative modes are shown in Fig. 1, and the supervised OPLS model was applied to enhance biomarker discovery efforts. In order to obtain better discrimination between the control group and pregnant patients with PCOS, the OPLS-DA score plots approach was applied to the metabolic profiles, which can separate samples into two blocks (Fig. 2). To exhibit the responsibility of each ion for these variations more intuitively, S-plots and VIP-value plots were combined. The ions showed a significant difference in abundance in the two groups in positive and negative modes (Fig. 3). The black point graph is the S-plot, and most of the ions were clustered around the origin point, and only a few of them were scattered in the margin region, and just these few ions contributed to the clustering observed in the score plot and were also the differentiating metabolites. The red point graph is the VIP-value plot, which represents the value of each ion. The farther away from the origin, the higher the VIP value of the ions was. The black points and red points were in a one-to-one correspondence in the combination.
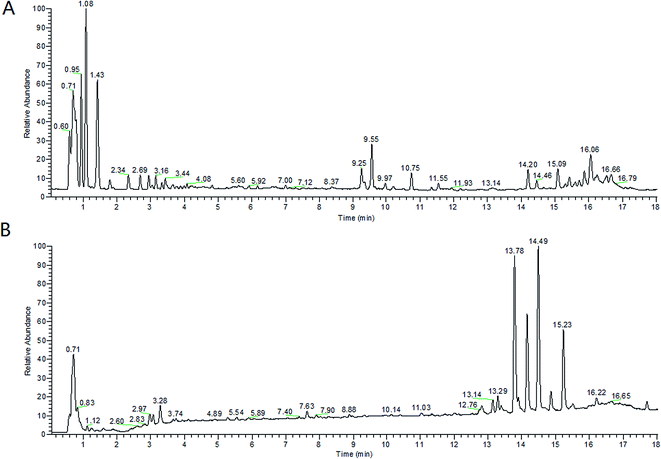 |
| Fig. 1 BPI chromatograms of two subjects’ serum samples analyzed by UPLC-HDMS. (A) positive mode and (B) negative mode. | |
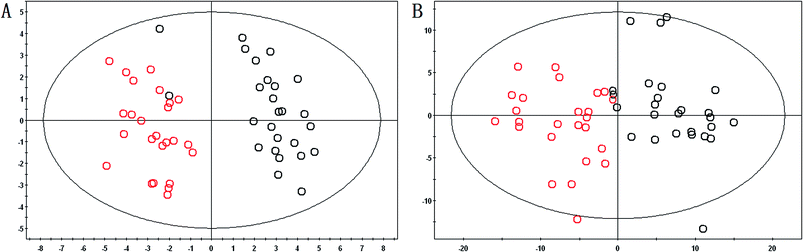 |
| Fig. 2 Scores plots of the OSC PLS-DA model separating control women from women with PCOS (A and B). (Black: control women; red: women with PCOS). (A): ESI positive ion mode; (B) ESI negative ion mode. | |
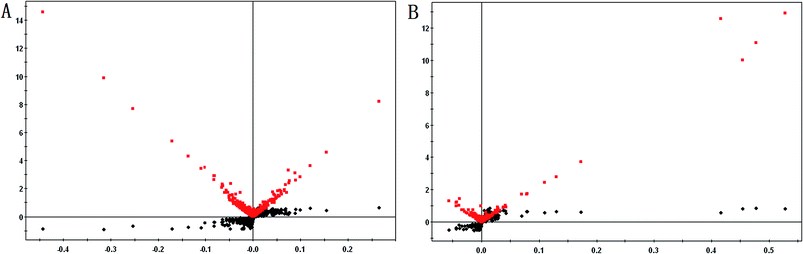 |
| Fig. 3 Combination of S-plot and VIP values comparing control women and women with PCOS (A and B). (A): positive negative ESI mode; (B): negative ESI mode. | |
3.4 Differentiating metabolite identification
All selected metabolites were filtered by a t-test and VIP. The metabolites with a significant difference (p < 0.05) and VIP > 3 were kept. Combining the results of the OPLS analysis with the S-plots and the VIP-plot, 6 ions were selected in the positive mode and 1 ion was selected in the negative mode as potential markers between the control group and pregnant patients with PCOS. The significantly upregulated LPC 16:0 sn-1, LPC 16:0 sn-2, LPC 18:0 sn-1, and LPC 18:1 sn-1 and the downregulated FFA 18:1, carnitine C2:0 and carnitine C10:0 were observed in patients with PCOS (Table S2†) as can also be seen in Fig. 4. The UPLC-MS analysis platform provided the retention time, precise molecular mass and VIP of the biomarkers. The potential biomarkers responsible for the differences between the control and pregnant patients with PCOS were identified. The precise molecular mass was determined within measurement errors (<5 ppm) by Q-TOF, and at the same time the potential elemental composition, degree of unsaturation and fractional isotope abundance of the compounds were obtained, which were utilized to search databases including KEGG, PubChem compound, METLIN, and the Human Metabolome Database.13 The metabolites were then validated with available standards. This difference of metabolites may denote their potential as targeted biomarkers for differentiating healthy pregnant women and threatened abortion in pregnant patients with PCOS. We used a heatmap to observe the metabolite changes intuitively among the two groups (Fig. 5).
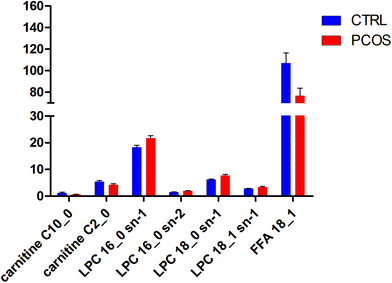 |
| Fig. 4 Comparison of the intensities of differential metabolites (blue: control women; red: women with PCOS). | |
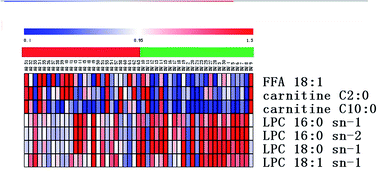 |
| Fig. 5 Heatmap of the differential metabolites corresponding to Table S2.† (Red: control women; green: women with PCOS). | |
4. Discussion
From the information of the studied subjects, there were no significant differences in age and gestation week among the two groups. Controls and women with PCOS were well-matched in terms of BMI and waist–hip ratio. As the waist–hip ratio primarily reflects the distribution of visceral adipose tissue,14 it can be implied that the adipose tissue is distributed differently in the patients with PCOS and the control group. To our knowledge, PCOS is a complex disease related to metabolic dysfunction, with high abortion rates in pregnancy. In this study, we have demonstrated that maternal hormone concentrations of E2, P and β-HCG in mothers with PCOS are significantly lower compared to those in healthy mothers, and the concentrations of T and AND are higher compared to those in healthy mothers. The most commonly used markers in the assessment of pregnancy viability have been maternal serum β-HCG and progesterone.15 Our data support the idea, to some extent, that elevated concentrations of T and androgens in mothers with PCOS affect the embryo. These data also support that a maternal hyperandrogenic and low-estrogen environment in mothers with PCOS contributes to abortion. The pathogenesis of PCOS may be associated with insulin-like antagonistic substances secreted from the placenta, and these substances were elevated with increases of gestational age. From the information of the studied subjects, fasting blood glucose (FBG) of mothers with PCOS showed significant differences when compared with that of control subjects. Disorders of glucose metabolism were also evident in patients with PCOS.
In order to investigate the metabolite differences between mothers with PCOS and healthy mothers, a comprehensive metabolomics approach based on UPLC-QTOF-MS and multivariate statistical analysis was applied to understand the metabolite changes between pregnant women with PCOS and healthy pregnant controls. Hence, chemometrics such as OPLS were applied to metabolic data to find the differentiating metabolites that contributed to the clustering observed in the score plots. Finally, 7 differentiating metabolites were preliminary identified in the negative and positive modes, which had relationships with the metabolic pathways of lipid metabolism and carnitine metabolism. The differential metabolites revealed metabolism disorders of mothers with PCOS mainly in lipid metabolism. The result was similar to that found by Zhao et al.,7 but we did not find the differences in androgen metabolism. We revealed the disorder of blood hormones. Unfortunately, we referred to a large number of articles, but did not find similar results to ours, including for non-pregnant women with PCOS.
In the positive mode, LPC was the most responsible ion for discrimination, which included LPC 16:0 sn-1, LPC 16:0 sn-2, LPC 18:1 sn-1 and LPC 18:0 sn-1. These changes in LPC could be attributed to excess sugars being transferred into lipid metabolism, especially in glycerophospholipid metabolism.16 In our study, FPG in the PCOS group was, in fact, higher than that of the control group. To some extent, the results are consistent with a prior study by Dudzik D. et al.17, but we did not find similar studies in the first trimester just in the second and third trimesters. LPC is well-known to induce an inflammatory response, apoptosis and tumor cell invasiveness.18,19 Recent studies have shown that LPCs may play an important role in glucose metabolism,20 which may also be considered as marker molecules of insulin resistance.21
Carnitine is required by the developing fetus for both fetal maturation and fatty acid oxidation in the placental-fetal unit.22 In this study, the levels of carnitine 2:0 and carnitine 10:0 were significantly higher in the control group than in the mothers with PCOS, which coincides with other research on GDM23 and on disorders of glucose metabolism, which also appeared in patients with PCOS. There is evidence that the human placenta and fetus are capable of complete carnitine biosynthesis,24 but under conditions of poor maternal carnitine supply. Carnitine is an essential compound required for fatty acid metabolism, which occurs in the mitochondria of cells. During gestation, women undergo many physiological changes, including metabolic changes, in order to adapt to the high fatty acid conditions and substrates required for fetal development. Changes in plasma concentration of carnitine may play an important role in determining appropriate fetal growth.
In the negative mode, FFA 18:1 was the responsible ion for discrimination. To our knowledge, this is the first study to examine the potential of first-trimester metabolites in identifying mothers with PCOS at risk of abortion. Given the growing incidence of abortion in women with PCOS, early detection of gestation provides an opportunity for primary intervention strategies which would decrease the risk of abortion. Of the many monounsaturated fatty acids (MUFAs), the most studied is oleic acid (FFA 18:1), which helps lower blood sugar levels,28 and reduces the level of LDL25 to adjust blood lipids.26 In other observational studies,27 it was revealed that Baru almonds (Dipteryx alata Vog.) have considerable amounts of monounsaturated fatty acids (MUFAs), which could exert positive effects in serum lipids and markers of oxidation. FFA 18:1 as a MUFA, which is also the main ingredient of a classical prescription named Shou Taiwan of traditional Chinese medicine, also played a role in the prevention and treatment of thrombotic disease by improving vascular reactivity,28 assisting in the protection of the fetus. In the present study, the content of FFA 18:1 as a MUFA decreased in the mothers with PCOS, which may be indicative of early pregnancy failure. However, we do not know whether MUFAs play a pivotal role in supporting the gestational sac development.
5. Conclusion
In this study, dynamic metabolic profiles were investigated with UPLC-MS combined with multivariate statistical analysis. All results demonstrated that mothers with PCOS have different metabolic profiles compared to healthy subjects, and the 7 ions (6 in the positive mode and 1 in the negative mode) identified may be useful in differentiating metabolites for the clinical forecast and diagnosis of threatened abortion resulting from PCOS. At the same time, these results suggest that the UPLC-MS metabolomic approach is a useful tool to investigate the metabolic abnormality of mothers with PCOS. Therefore, the differences in metabolites could not only serve as biomarkers to assist the diagnosis of threatened abortion resulting from PCOS, but they could also be used to remind clinicians to predict the occurrence of threatened abortion in advance. In conclusion, this is the first demonstration of a metabolomic approach to delineate metabolic changes in threatened abortions resulting from PCOS. These potential biomarker candidates also confirmed that the pathogenesis of threatened abortion resulting from PCOS is closely related to multiple etiologies and pathogenesis.
Conflicts of interest
None declared.
References
- S. Palomba, A. Falbo, T. Russo, A. Tolino, F. Orio and F. Zullo, Pregnancy in women with polycystic ovary syndrome: The effect of different phenotypes and features on obstetric and neonatal outcomes, Fertil. Steril., 2010, 94, L1805–L1811 CrossRef PubMed.
- R. Azziz, K. S. Woods, R. Reyna, T. J. Key, E. S. Knochenhauer and B. O. Yildiz, The prevalence and features of the polycystic ovary syndrome in an unselected population, J. Clin. Endocrinol. Metab., 2004, 89, 2745–2749 CrossRef CAS PubMed.
- G. S. Ghazeeri, A. H. Nassar, Z. Younes and J. T. Awwad, Pregnancy outcomes and the effect of metformin treatment in women with polycystic ovary syndrome: An overview, Acta Obstet. Gynecol. Scand., 2012, 91, 658–678 CrossRef PubMed.
- A. Zhang, H. Sun and X. Wang, Mass spectrometry-driven drug discovery for development of herbal medicine, Mass Spectrom. Rev., 2016 DOI:10.1002/mas.21529.
- Y. Zhang, P. Liu and Y. Li, et al., Exploration of metabolite signatures using high-throughput mass spectrometry coupled with multivariate data analysis, RSC Adv., 2017, 7(11), 6780–6787 RSC.
- J. K. Nicholson and J. C. Lindon, Systems biology: metabonomics, Nature, 2008, 455, 1054–1056 CrossRef CAS PubMed.
- X. Zhao, F. Xu, B. Qi, S. Hao, Y. Li, Y. Li, L. Zou, C. Lu, G. Xu and L. Hou, Serum Metabolomics Study of Polycystic Ovary Syndrome Based on Liquid Chromatography–Mass Spectrometry, J. Proteome Res., 2014, 13, 1101–1111 CrossRef CAS PubMed.
- X. Wang, J. Li and A. H. Zhang, Urine metabolic phenotypes analysis of extrahepatic cholangiocarcinoma disease using ultra-high performance liquid chromatography-mass spectrometry, RSC Adv., 2016, 6(67), 63049–63057 RSC.
- Y. Li, S. Qiu and A. Zhang, High-throughput metabolomics to identify metabolites serve as diagnostic biomarkers of prostate cancer, Anal. Methods, 2016, 8, 3284–3290 RSC.
- ACOG Committee on Obstetric Practice, Obesity in pregnancy. ACOG committee opinion. No. 549, Obstet. Gynecol., 2013, 121, 213–217 CrossRef.
- E. Rotterdam, PASRM-Sponsored. Revised 2003 consensus on diagnostic criteria and long-term health risks related to polycystic ovary syndrome (PCOS), Hum. Reprod., 2004, 19, 41–47 CrossRef.
- X. Xie and W. Gou, Obstetrics and Gynecology[M], People’s Medical Publishing House, 2013, pp. 47–49 Search PubMed.
- J. Chen, X. Zhao, J. Fritsche, P. Yin, P. Schmitt-Kopplin, W. Wang, X. Lu, H. U. Haring, E. D. Schleicher, R. Lehmann and G. Xu, Practical approach for the identification and isomer elucidation of biomarkers detected in a metabonomic study for the discovery of individuals at risk for diabetes by integrating the chromatographic and mass spectrometric information, Anal. Chem., 2008, 80(4), 1280–1289 CrossRef CAS PubMed.
- S. Owens, M. Litaker, J. Allison, S. Riggs, M. Ferguson and B. Gutin, Prediction of visceral adipose tissue from simple anthropometric measurements in youths with obesity, Obes. Res., 1999, 7(1), 16–22 CrossRef CAS PubMed.
- M. Al-Azemi, D. Kyrou, E. M. Kolibianakis, P. Humaidan, I. Van Vaerenbergh, P. Devroey and H. M. Fatemi, Elevated progesterone during ovarian stimulation for IVF, Reprod. BioMed. Online, 2012, 24, 381–388 CrossRef CAS PubMed.
- L. Tianhu, L. Jiaxun and X. Fengcheng, et al., Comprehensive analysis of serum metabolites in gestational diabetes mellitus by UPLC/Q-TOF-MS., Anal. Bioanal. Chem., 2016, 408(4), 1125–1135 CrossRef PubMed.
- D. Dudzik, M. Zorawski and M. Skotnicki, et al., Metabolomics fingerprint of gestational diabetes mellitus, J. Proteome Res., 2014, 103, 57–71 CrossRef CAS PubMed.
- M. Iwase, K. Sonoki, N. Sasaki, S. Ohdo, S. Higuchi, H. Hattori and M. Iida, Lysophosphatidylcholine contents in plasma LDL in patients with type 2 diabetes mellitus: Relation with lipoprotein associated phospholipase A(2) and effects of simvastatin treatment, Atherosclerosis, 2008, 196(2), 931–936 CrossRef CAS PubMed.
- G. Perez-Chacon, A. M. Astudillo, V. Ruiperez, M. A. Balboa and J. Balsinde, Signaling role for lysophosphatidylcholine acyltransferase 3 in receptor-regulated arachidonic acid reacylation reactions in human monocytes, J. Immunol., 2010, 184(2), 1071–1078 CrossRef CAS PubMed.
- K. Yea, J. Kim, J. H. Yoon, T. Kwon, J. H. Kim, B. D. Lee, H.-J. Lee, S. J. Lee, J.-I. Kim, T. G. Lee, M.-C. Baek, H. S. Park, K. S. Park, M. Ohba, P.-G. Suh and S. H. Ryu, Lysophosphatidylcholine activates adipocyte glucose uptake and lowers blood glucose levels in murine models of diabetes, J. Biol. Chem., 2009, 284(49), 33833–33840 CrossRef CAS PubMed.
- X. Zhao, J. Fritsche, J. Wang, J. Chen, K. Rittig, P. Schmitt- Kopplin, A. Fritsche, H.-U. Haering, E. D. Schleicher, G. Xu and R. Lehmann, Metabonomic fingerprints of fasting plasma and spot urine reveal human pre-diabetic metabolic traits, Metabolomics, 2010, 6(3), 362–374 CrossRef CAS PubMed.
- J. Arenas, J. C. Rubio, M. A. Martin and Y. Campos, Biological roles of L-carnitine in perinatal metabolism, Early Hum. Dev., 1998, 53, S43–S50 CrossRef CAS PubMed.
- R. Bentley-Lewis, J. Huynh and G. Xiong, Metabolomic profiling in the prediction of gestational diabetes mellitus, Diabetologia, 2015, 58, 1329–1332 CrossRef CAS PubMed.
- N. A. Oey, N. Van Vlies, F. A. Wijburg, R. J. A. Wanders, T. Attie-Bitach and F. M. Vaz, L-Carnitine is synthesized in the human fetal-placental unit: Potential roles in placental and fetal metabolism, Placenta, 2006, 27, 841–846 CrossRef CAS PubMed , PMID: 16300828.
- S. S. Baroni, M. Amelio and Z. Sangiorgi, et al., Solid monounsaturated diet lowers LDL unsaturation trait and oxidizability in hypercholesterolemic (type II b) patients, Free Radical Res., 1999, 30, 275–285 CrossRef CAS PubMed.
- C. Thomsen, O. Rasmussen and C. Christiansen, et al. Comparison of the effects of a monounsaturated fat diet and a high carbohydrate diet on cardiovascular risk factors in first degree relatives to type-2 diabetic subjects, Eur. J. Clin. Nutr., 1999, 53, 818–823 CrossRef CAS PubMed.
- A. P. Bento, C. Cominetti, A. Simões Filho and M. M. Naves, Baru almond improves lipid profile in mildly hypercholesterolemic subjects: a randomized, controlled, crossover study, Nutr., Metab. Cardiovasc. Dis., 2014, 24(12), 1330–1336 CrossRef CAS PubMed.
- R. A. Vogel, M. C. Corretti and G. D. Plotnick, The postprandial effect of components of the Mediterranean diet on endothelial function, J. Am. Coll. Cardiol., 2000, 36, 1455–1460 CrossRef CAS PubMed.
Footnotes |
† Electronic supplementary information (ESI) available. See DOI: 10.1039/c6ra27357b |
‡ These authors contributed equally to this work and are co-first authors. |
|
This journal is © The Royal Society of Chemistry 2017 |