DOI:
10.1039/C6RA24952C
(Paper)
RSC Adv., 2017,
7, 13552-13560
Chiral recognition and determination of enantiomeric excess of chiral compounds by UV-visible-shortwave near infrared diffuse reflectance spectroscopy with chemometrics
Received
9th October 2016
, Accepted 18th January 2017
First published on 28th February 2017
Abstract
A simple approach is proposed for the chiral recognition and determination of enantiomeric excess of enantiomers, based on a UV-visible-shortwave near infrared diffuse reflectance spectroscopy (UV-vis-SWNIR DRS) technique combined with chemometrics. The results of chiral recognition show that principal component analysis (PCA) combined with UV-vis-SWNIR DRS is able to discriminate chiral compounds based on different chirality. Determination of enantiomeric excess value was performed by linear regression model partial least squares (PLSR) and non-linear regression model support vector machine regression (SVR) combined with UV-vis-SWNIR diffuse reflectance spectroscopy. After wavelength selection, spectral pre-treatments and parameter optimization, both models showed good prediction ability: the determination coefficients (R2) of prediction set by the PLSR model and the SVR model are 0.9921 and 0.9951, respectively, and mean standard errors (MSEs) are 0.0029 and 0.0020, respectively. The SVR model has a better prediction effect. The detection limit (LD) of this method was 0.059. The results prove that this approach can be used to discriminate chiral compounds and determine enantiomeric excess of enantiomers.
1 Introduction
The increased development of single-enantiomer pharmaceuticals has enhanced the need for rapid and convenient methods for chiral recognition and determination of enantiomeric excess of chiral compounds.1
There have been various methods for chiral analysis, such as circular dichroism (CD),2–4 polarimetry,5 NMR,1,6 high performance liquid chromatography (HPLC),7–9 gas chromatography (GC),10 capillary electrophoresis (CE),11–14 mass spectroscopy,15 fluorescence spectrometry,16–18 ultraviolet absorption spectroscopy,19–22 UV-visible absorption spectroscopy,23–25 near-infrared spectroscopy,26 resonance Rayleigh scattering spectroscopy,27 optically active Raman spectroscopy,28,29 vibrational circular dichroism spectroscopy,30,31 ITC,32,33 colorimetric probes34–36 and so on. Most of them are effective and powerful either in chiral recognition or in determination of enantiomeric excess.
The above methods have some drawbacks such as needing addition of chiral auxiliary for derivatization,7–10,15–26 requiring tedious sample pretreatment,34–36 using expensive and sophisticated instruments,2–4,6–9,28,29,32,33 being time-consuming,6,30,31 less sensitive,2–4 destructive,15 only for chiral recognition.32,33 Additionally, they are all wet analysis and can't be used for all types of chiral compounds. These make them not the first choice for rapid and convenient chiral analysis.
So far, there are only few papers devoted to chiral analysis in solid state. They are diffuse reflectance infrared Fourier transform spectroscopy (DRIFT),37,38 terahertz time domain spectroscopy (THz-TDS)39,40 and UV-vis-SWNIR DRS proposed recently by us.41,42 DRIFT needs to add KBr,37,38 THz-TDS needs to add magnesium oxide39 and is interfered with seriously by water in samples or the atmosphere.
UV-vis-shortwave near infrared diffuse reflectance spectroscopy (UV-vis-SWNIR DRS) has advantages containing a wealth of information regarding electron-energy level transition as well as vibrational motion of molecules, being simple, fast and non-destructive, while DRIFT has only information regarding vibrational motion of molecules. DRIFT is measured with potassium bromide. Potassium bromide is easy to absorb moisture, and the strong absorption of water interferes seriously with the DRIFT spectra of samples. Additionally, DRIFT instrument is more expensive than UV-vis-shortwave near infrared diffuse reflectance spectrometer used in our method. And DRIFT method is destructive, because the sample cannot be recovered. Comparing to DRIFT and THz-TDS, our proposed UV-vis-SWNIR DRS needs no addition of any reagents, neither chiral nor achiral reagents. It is unique and has a potential to be developed as a rapid, convenient and non-destructive quality control tool for isomeric purity testing of optically active substances.
The principle of UV-vis-SWNIR DRS for chiral analysis is based on the difference of diffuse reflectance spectra between enantiomers in powder. This is likely due to their different steric configuration, consequently due to different crystal structures and habits. It is not recent discovery that different polymorph can display different ultraviolet-visible spectra.43 The principle that different crystal structures may have different UV-vis-SWNIR DRS has been applied on the qualitative and quantitative analysis of polymorph of pharmaceuticals.44
The earlier study in our laboratory based on UV-vis-SWNIR DRS combined with chemometrics was investigated for discriminating enantiomers and their racemate based on chirality and manufacturing origins difference, using D-, L- and DL-alanine as model compounds.41 The objective of the study is to investigate the feasibility of applying UV-vis-SWNIR DRS combined with chemometrics on determination of enantiomeric excess of chiral enantiomers using tartaric acid as test substance and the universality of this method in chiral recognition, using tartaric acid, aromatic α-amino acids phenylalanine and tryptophan as test substances.
2 Experimental
2.1 Reagents
D-, L- and DL-tartaric acid (purity ≥ 99.5%) from Kelong chemical reagent factory in Chengdu, D-phenylalanine (purity of 98%) and L-phenylalanine (purity of 99%), D-tryptophan (purity of 99%) and L-tryptophan (purity of 98%) purchased from Shanghai Aladdin Bio-Chem Technology were grinded manually with a mortar and pestle, and sifted, in a sealed plastic bag containing desiccant silica gel to protect the samples against moisture absorption.
The D- and L-tartaric acid powers through 200-mesh sieve were used to measure X-ray diffraction patterns.
Tartaric acid powers through 100-mesh sieve and alanine and tryptophan powders through 160-mesh sieve were used to measure UV-vis-SWNIR DRS.
Racemic samples of the three chiral compounds were prepared by evenly mixed D-enantiomer and L-enantiomer power samples through 100- or 160-mesh sieve together in the same ratio.
The tartaric acid powers through 100-mesh sieve were accurately weighed and evenly mixed together according to predetermined ratios to prepare 72 samples with different enantiomeric excess value: −1, −0.95, −0.92, −0.9, −0.88, −0.86, −0.83, −0.8, −0.77, −0.74, −0.71, −0.68, −0.65, −0.62, −0.59, −0.56, −0.53, −0.5, −0.47, −0.44, −0.41, −0.38, −0.35, −0.32, −0.29, −0.26, −0.23, −0.2, −0.17, −0.14, −0.11, −0.08, −0.05, −0.02, 0, 0.01, 0.04, 0.07, 0.1, 0.13, 0.16, 0.19, 0.22, 0.25, 0.28, 0.3, 0.32, 0.34, 0.37, 0.4, 0.43, 0.46, 0.49, 0.52, 0.55, 0.58, 0.6, 0.62, 0.64, 0.67, 0.7, 0.73, 0.76, 0.79, 0.82, 0.85, 0.88, 0.9, 0.92, 0.94, 0.97, 1, in which the mass fraction of L-enantiomer was varied from 0 to 100%.
2.2 Apparatus
S3000 Fiber Optic Spectrometer (Race-Technology Co., Ltd, Hangzhou, China) equipped with a 3648-element linear silicon CCD array detector (Toshiba TCD 1305), a Y-type optical fiber probe with 100 cm in length and 0.4 mm in diameter, a light source (Oceans Optics Inc., USA) and a home-made sample cell made from dark gray PVC, was used to measure UV-vis-SWNIR diffuse reflectance spectra in wavelength range of 200–1100 nm.
X'Pert PRO powder diffractometer (PANalytical Company, Holland) with a Pixcel 1D detector and Cu Kα1 radiation were used to measure X-ray diffraction patterns of D- and L-tartaric acids in the range of 5–50° 2θ.
WZZ-3 automatic polarimeter (Shen Guang Instrument Co., Ltd, Shanghai, China) was used to measure specific rotation.
2.3 Experiment procedure
2.3.1 Specific rotation of tartaric acid, phenylalanine and tryptophan. The specific rotation of three chiral compounds were measured to check their optical purity by WZZ-3 Automatic Polarimeter, following the Chinese Pharmaceutical Industry Standard, in which 0.2 g ml−1 tartaric acid enantiomers aqueous solutions, 0.02 g ml−1 phenylalanine enantiomers aqueous solutions and 0.01 g ml−1 tryptophan enantiomers aqueous solutions are used.
2.3.2 X-ray diffraction patterns of D-, L-tartaric acid. The X-ray diffraction data of D- and L-tartaric acid through 200-mesh sieve were obtained by using an X'Pert PRO diffractometer with an Pixcel 1D detector and Cu Kα1 radiation (λ = 1.54056 Å, generator setting: 40 kV, 40 mA) at room temperature. Diffraction patterns were recorded in the range of 5–50° 2θ, using a step size of 0.013° 2θ and a count time of 29 s per step.
2.3.3 Acquisition of UV-vis-SWNIR DRS for chiral recognition. It has been recognized that the particle size of samples and the distance between optical probe and sample surface have influence on UV-vis-SWNIR DRS measurement and the performance of chiral analysis model.41 Preliminary tests showed that tartaric acid of 100 mesh, tryptophan and phenylalanine acid of 160 mesh can give a good modelling effect; 0.17 g of tartaric acid of 100 mesh, 0.18 g of tryptophan of 160 mesh and 0.1 g of phenylalanine acid of 160 mesh have an appropriate and almost same thickness after they had been filled in the sample-cell and flattened. Therefore, 0.17 g of tartaric acid of 100 mesh, 0.18 g of tryptophan and 0.1 g of phenylalanine acid of 160 mesh were used in the following experiment.0.17 g of D- or L- or DL or racemic tartaric acid through 100-mesh, or 0.1 g of D- or L- or racemic phenylalanine acid of 160 mesh, or 0.18 g of D- or L- or racemic of 160 mesh were weighed and filled in the sample cell, pressed by free fall impacts of a round rod to make the sample surface smooth. Then the optical probe was placed vertically on the upper surface of sample to acquire UV-vis-SWNIR DRS under the following conditions: the wavelength range from 200 to 1106 nm, a resolution of 3.3 nm, integral time of 250 ms for tartaric acid, 356 ms for phenylalanine and 373 ms for tryptophan, with a Spectralon as background reference. After a former measurement, the sample surface was stirred, again pressed and smoothed, to measure its next spectrum. Stirring-pressing-smoothing steps were repeated 45 times. Therefore, 45 spectra for each kind of powder sample were obtained, total 135 spectra for each chiral compound.
2.3.4 Acquisition of UV-vis-SWNIR DRS for determination of enantiomeric excess. UV-vis-SWNIR DRS of 72 samples with different enantiomeric excess value were measured under the conditions as described above. Each sample of 0.17 g was filled in the sample cell, pressed by free fall impacts of a round rod until the surface was smooth, then the optical probe was placed vertically on the upper surface of sample to acquire UV-vis-SWNIR spectra. Each sample was measured three times by resetting the optical probe and their average was treated as the original spectrum of the sample. Total 72 spectra were obtained.
2.3.5 Chiral recognition by principal component analysis (PCA). PCA is used for chiral recognition. PCA is a projection and dimension reduction method for transforming the original measurement variables into new, uncorrelated variables called principal components, to summarize the features of the data. And in traditional approach is to use the first few principal components (PCs) in data analysis since they retain much of the variability of the original dataset.45–47 The scores of PCs were then used as input to the multivariate analyses.46
2.3.6 Determination of enantiomeric excess.
2.3.6.1 Wavelength selection. Interval partial least square method (iPLS) and correlation coefficient method were utilized for wavelength selection. iPLS is a new graphically oriented local modeling procedure compared to full-spectrum partial least-squares.48 Root-mean square error of cross validation (RMSECV) is a parameter governing the variable selection. The wavelength interval or intervals with a RMSECV lower than the minimum RMSECV of a full-spectrum model was or were selected.In correlation coefficient method for wavelength selection, the correlation coefficient (R) between e.e value and absorbance at every wavelength is calculated first, to plot R vs. wavelength. Then the wave bands with R greater than certain thresholds set for R are tried in modelling and those giving good modelling results can be selected as optimum wavebands.
2.3.6.2 Spectral preprocessing. To enhance the spectral features and reduce or remove the influence of noise, the spectral data were subjected to various mathematical treatments,49 including mean center and scale, normalization (Nor), noise, baseline, spectroscopic, smoothing (SG), multiplicative scatter correction (MSC), standard normal transformation (SNV), the first derivative (1stD), the first derivative combined with SNV (1stD + SNV), SNV combined with the first derivative (SNV + 1stD), and so on.
2.3.6.3 Establishment of models. Partial least squares regression (PLSR) is a widely used linear regression method due to its simplicity to use, speed, relative good performance and easy accessibility, while support vector machine regression (SVR) is a non-linear regression method capable of dealing efficiently with high dimensional input vectors.50 The statistical parameters including large square correlation coefficient (R2), small root mean square error of calibration (RMSE), small mean square error of cross-validation (MSE), small standard error (SE) were utilized to evaluate the accuracy, predictive ability, and the robustness of a model.
3 Results and discussions
3.1 Characterization of three chiral compounds
Three chiral compounds were identified by automatic polarimeter and X-ray powder diffractometer.
3.1.1 Specific rotation. The determined specific rotation of D-tartaric acid and L-tartaric acid are −11.61° and 12.99°, D-phenylalanine and L-phenylalanine are 33.51° and −34.10°, D-tryptophan and L-tryptophan are 31.50° and −32.00°, respectively. By comparison with those listed in Chinese Pharmaceutical Industry Standards, the optical purity of the used three chiral compounds are reliable.
3.1.2 X-ray diffraction patterns. By searching and matching, the obtained X-ray diffraction patterns of D- and L-tartaric acid powders through 200-mesh sieve, as given in Fig. 1, are consistent with their corresponding standard X-ray diffraction patterns in the Powder Diffraction File (PDF) card in International Centre for Diffraction Data (ICDD). Processing the diffraction data by Jade 6 soft gave the detailed crystal information, which is consistent with the standard crystal information. These imply that the used D-, L-tartaric acids are reliable.
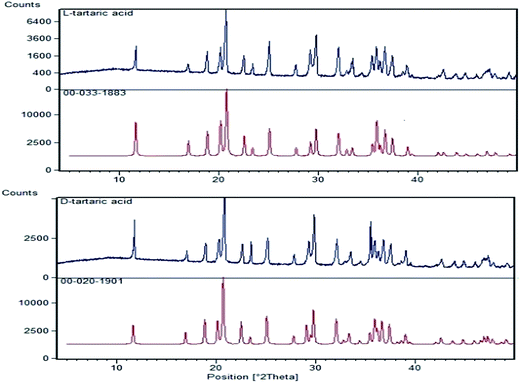 |
| Fig. 1 X-ray diffraction patterns of D-tartaric acid and L-tartaric acid. | |
3.2 Chiral recognition
3.2.1 UV-vis-SWNIR DRS of three compounds. Fig. 2A–C shows UV-vis-SWNIR DRS of three chiral compounds, the region of spectra confined by blocks show clear difference and there also have other small difference in the rest spectra region. These differences may provide the foundation of chiral analysis.
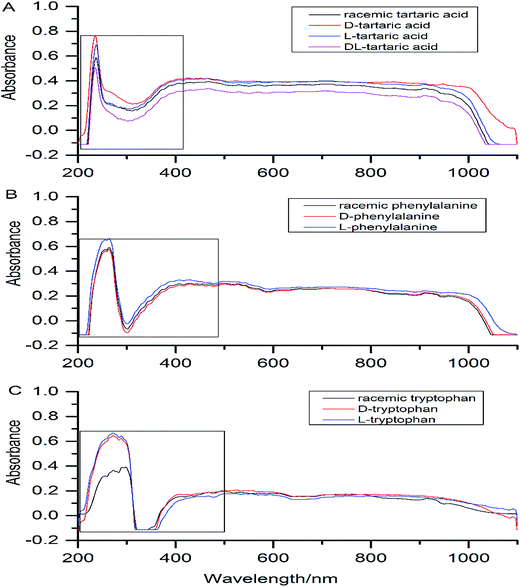 |
| Fig. 2 UV-vis-SWNIR DRS of three chiral compounds. | |
3.3 Determination of enantiomeric excess value
Determination of enantiomeric excess value was carried out by processing UV-vis-SWNIR DRS of the samples with different enantiomeric excess value using linear regression model partial least squares (PLSR) and non-liner regression model support vector machine regression (SVR). The 72 samples were divided into a calibration set with 53 samples and a prediction set with 19 samples by randomly for modeling.
3.3.1 Wavelength selection. Fig. 7 and 8 are the results of wavelength selection by iPLS method and correlation coefficient method. From Fig. 7 we can see that RMSECV of the eighth interval, i.e. the wavelength band of 1002–1106 nm is lower than the minimum RMSECV of a full-spectrum model, so the eighth interval was selected as the optimal wavelength band. Fig. 8 shows the variation of correlation coefficient (R) with wavelength, and the wavelength bands which have a correlation coefficient (R) larger than 0.6, 0.7 and 0.8, respectively, were tried and compared in SVR modelling.
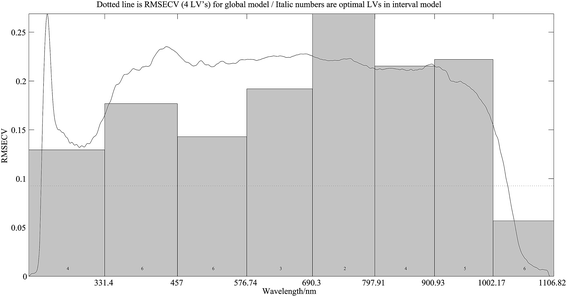 |
| Fig. 7 Interval partial least square method. (![[dash dash, graph caption]](https://www.rsc.org/images/entities/char_e091.gif) ) RMSECV of a full-spectrum model. | |
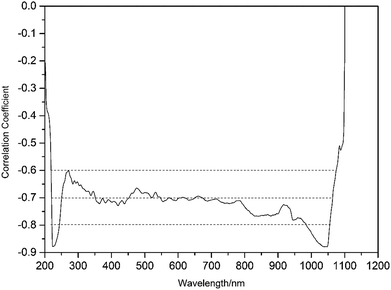 |
| Fig. 8 Correlation coefficient method. | |
3.3.2 Partial least squares regression (PLSR) modelling. A series of PLSR models were built by different data-processing on different wavelength bands. Their predictive results were compared in order to optimize model. As a result, PLSR modeling using the wavelength band of 1002–1106 nm gave better predictive results, as shown in Table 1.
Table 1 Effect of data preprocessing on PLSR modelling
Data preprocessing |
Calibration |
Validation |
Prediction |
MSEC |
R2 |
MSEV |
R2 |
MSEP |
R2 |
Raw spectra |
0.0027 |
0.9917 |
0.0038 |
0.9889 |
0.00358 |
0.9905 |
1stD |
0.0057 |
0.9826 |
0.0001 |
0.9778 |
0.0064 |
0.9830 |
SG |
0.0027 |
0.9917 |
0.0038 |
0.9892 |
0.0035 |
0.9905 |
SNV |
0.0048 |
0.9852 |
0.0058 |
0.9840 |
0.0053 |
0.9858 |
MSC |
0.0048 |
0.9853 |
0.0058 |
0.9821 |
0.0044 |
0.9883 |
1stD + SNV |
0.0080 |
0.9755 |
0.0099 |
0.9709 |
0.0097 |
0.9743 |
Baseline |
0.0027 |
0.9917 |
0.0038 |
0.9881 |
0.0035 |
0.9905 |
Spectroscopic |
0.0027 |
0.9918 |
0.0043 |
0.9866 |
0.0029 |
0.9921 |
Table 1 shows the result of PLSR modelling by different data preprocessing on the wavelength band of 1002–1106 nm, it can be seen that spectroscopic preprocess resulted in the optimal predicted result with a determination coefficient (R2) of 0.9921 and a mean standard error (MSE) of 0.0029 for the prediction set.
3.3.3 Support vector machine regression (SVR) modeling. The radial basis function (RBF) was used as the kernel function for SVR modelling. SVR model was established after data preprocessing on the wavelength bands with correlation coefficient R larger than 0.7 selected by correlation coefficient method. The results are shown in Table 2 under the conditions using default parameters (the kernel parameter g = 0.01, the regularization parameter c = 100, the insensitive coefficient p = 0.01), comprehensive considering the determination coefficient (R2) and mean square error (MSE) of calibration set and prediction set, the combination of 1stD with SNV gave the optimal modeling prediction with R2 of 0.9997 and 0.9894, and MSE of 0.00009 and 0.0054 for the calibration set and prediction set, respectively.
Table 2 Result of modeling SVR with data preprocessing methods
Data preprocessing |
Used parameters of the kernel function |
Calibration |
Prediction |
MSE |
R2 |
MSE |
R2 |
Raw spectra |
Default Parameters |
0.0017 |
0.9950 |
0.0041 |
0.9895 |
MSC |
0.0069 |
0.9800 |
0.0344 |
0.9124 |
Smoothing-SG |
0.0017 |
0.9949 |
0.0042 |
0.9894 |
Normalize |
0.3293 |
0.8334 |
0.3783 |
0.8055 |
Spectroscopic |
0.0006 |
0.9981 |
0.0018 |
0.9952 |
Baseline |
0.0030 |
0.9915 |
0.0030 |
0.9922 |
Noise |
0.0001 |
0.9997 |
0.0093 |
0.9762 |
Center and scale |
0.0017 |
0.9950 |
0.0041 |
0.9895 |
1stD |
0.2998 |
0.9514 |
0.3452 |
0.9608 |
SNV |
0.0001 |
0.9996 |
0.0019 |
0.9544 |
SNV + 1stD |
0.0153 |
0.9610 |
0.0184 |
0.9564 |
1stD + SNV |
0.00009 |
0.9997 |
0.0054 |
0.9894 |
1stD + SNV |
Optimal parameters |
0.000001 |
0.999996 |
0.0020 |
0.9951 |
By comparison of the results of 1stD + SNV and SNV + 1d 1stD, it was known that the effect of a combination of two spectral pretreatments differs with the order of combination.
Parameters (g, c, p) of SVR modeling are key to obtain good prediction results. They were optimized in the ranges g [0–0.1], c [0.001–500] and p [0.001–0.1], and the effects of optimization on modeling were evaluated by 10-fold cross-validation. The last row of Table 2 shows the prediction by SVR model after parameter optimization, it can be seen that R2 of calibration and prediction is 0.999996 and 0.9951, and MSEP is 0.000001 and 0.0020, respectively, when the parameters g, c and p were set at 0.002, 100 and 0.001, respectively. The predicted result was obviously improved.
3.4 Comparison of prediction results of PLSR and SVR model
Plots of predicted values of PLSR and SVR models vs. reference values and relative error of a predicted value against its reference value are given in Fig. 9a and b, respectively. It demonstrates that the R2 and MSEP of SVR model are better than those of PLSR model. The relative errors of the samples of no. 8 and no. 9 are obviously larger than the others. It is due to their enantiomeric excess close to zero (−0.05, 0.04), so a small deviation will result in a large relative error. The detection limit (LD) of this method was determined from multiple measurements (n = 10) of the spectral response of a single sample with enantiomeric excess value of 0.92. Based upon a standard deviation of 0.0192 and slope of 0.9748 of prediction curve of modeling SVR and an LD of 0.059 for enantiomeric excess value was calculated utilizing eqn (1). |
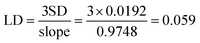 | (1) |
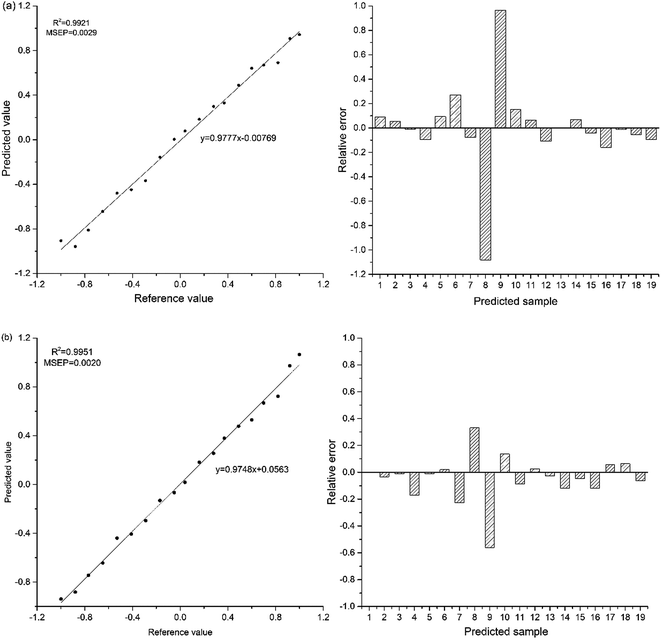 |
| Fig. 9 Plots of predicted values vs. reference values and relative error of a predicted value against its reference value (a) PLSR model; (b) SVR model. | |
4 Conclusions
In this study, chiral recognition of three chiral compounds and determination of the enantiomeric excess value of tartaric acid samples based on UV-vis-SWNIR DRS combined with chemometric is proposed.
PCA was used for chiral recognition. The cumulative variance contribution of the first three components of three chiral compounds were all over 95%. Projections of PCA show that different samples of each chiral compounds are classified and discriminated clearly by UV-vis-SWIR DRS combined with PCA.
Modeling PLSR and modeling SVR are applied to calibrate spectra. The determination coefficient (R2) of prediction set by PLSR model and SVR model are 0.9921, 0.9951, and mean standard error (MSE) of are 0.0029 and 0.0020, respectively. These prove that UV-vis-SWNIR DRS combined with regression models can be used as a simply sample-preprocessing, rapid, convenient and inexpensive method to determine the enantiomeric excess of chiral enantiomers.
Acknowledgements
This work was supported by the Science and Technology special fund of Sichuan Provincial Administration of Traditional Chinese Medicine, China (No. 2016Q058) and the Scientific Research Foundation of the Education Department of Sichuan Province, China (No. 16ZB0122).
References
- J. Thomas and J. D. Wilcox, Chirality, 2003, 15, 256 CrossRef PubMed.
- T. Hattori, Y. Minato, S. L. Yao, M. G. Finn and S. Miyano, Tetrahedron Lett., 2001, 42, 8015 CrossRef CAS.
- M. T. Reetz, K. M. Kuhling, H. Hinrichs and A. Deege, Chirality, 2000, 12, 479–482 CrossRef CAS PubMed.
- L. Kott, W. B. Holzheuer, M. M. Wong and G. K. Webster, J. Pharm. Biomed. Anal., 2007, 43, 57–65 CrossRef CAS PubMed.
- H. G. Brittain, J. Pharm. Biomed. Anal., 1998, 17, 933 CrossRef CAS PubMed.
- W. H. Pirkle and D. L. Sikkenga, Chem. Informationsdienst, 1977, 42, 1370 CAS.
- A. Péter, G. Török and D. W. Armstrog, J. Chromatogr. A, 1998, 793, 283 CrossRef.
- K. Monde, N. Harada, M. Takasugi, P. Kutschy, M. Suchy and M. Dzurilla, J. Nat. Prod., 2000, 63, 1312 CrossRef CAS.
- J. Chen and W. Shum, Tetrahedron Lett., 1993, 34, 7663 CrossRef CAS.
- V. Schurig, D. Schmalzing and M. Schleimer, Angew. Chem., Int. Ed., 1991, 30, 987–989 CrossRef.
- G. Gübitz and M. G. Schmid, J. Chromatogr. A, 1997, 792, 179 CrossRef.
- A. W. I. Wan, D. Hermawan and M. M. Sanagi, J. Chromatogr. A, 2007, 1170, 107 CrossRef PubMed.
- H. Y. Cheng, B. K. He, Q. L. Zhang and Y. Tu, Anal. Sci., 2010, 26, 1087 CrossRef CAS PubMed.
- D. Wistuba and V. Schurig, Enantiomer separation of chiral pharmaceuticals by capillary electrochromatography, J. Chromatogr. A, 2000, 875, 255 CrossRef CAS PubMed.
- L. M. Wu, E. C. Meurer and R. G. Cooks, Chiral morphing and enantiomeric quantification in mixtures by mass spectrometry, Anal. Chem., 2004, 76, 663 CrossRef CAS PubMed.
- C. D. Tran and D. Oliveira, Anal. Biochem., 2006, 356, 51 CrossRef CAS PubMed.
- S. O. Fakayode, M. A. Busch, D. J. Bellert and K. W. Busch, Analyst, 2005, 130, 233 RSC.
- Y. F. Xu and M. Mccarroll, J. Photochem. Photobiol., A, 2006, 178, 50 CrossRef CAS.
- C. D. Tran and S. F. Yu, J. Phys. Chem., 2005, 109, 12627 CrossRef CAS PubMed.
- S. O. Fakayode, M. A. Busch and K. W. Busch, Talanta, 2006, 8, 1574 CrossRef PubMed.
- S. O. Fakayode, I. M. Swamidoss, M. A. Busch and K. W. Busch, Talanta, 2005, 65, 838 CrossRef CAS PubMed.
- K. W. Busch, I. M. Swamidoss, S. O. Fakayode and M. A. Busch, Anal. Chim. Acta, 2004, 525, 53 CrossRef CAS.
- Q. Q. Li, J. Duan, L. J. Wu, Y. Huang, G. Tang and S. G. Min, Chin. Chem. Lett., 2012, 23, 1055 CrossRef CAS.
- Q. Q. Li, Y. Huang, J. Duan, L. J. Wu, G. Tang, Y. W. Zhu and S. G. Min, Spectrochim. Acta, Part A, 2013, 101, 349 CrossRef CAS PubMed.
- J. R. Ingle, K. W. Busch and M. A. Busch, Talanta, 2008, 75, 72–84 CrossRef PubMed.
- M. J. Politi, C. D. Tran and G. H. Ga, J. Phys. Chem., 1995, 99, 14137–14141 CrossRef CAS.
- J. D. Yang, Fenxi Kexue Xuebao, 2006, 22, 454 CAS.
- K. M. Spencer, R. B. Edmonds, R. D. Rauh and M. M. Carrabba, Anal. Chem., 1994, 66, 1269 CrossRef CAS PubMed.
- L. Hecht, A. L. Phillips and L. D. Barron, J. Raman Spectrosc., 1995, 26, 727 CrossRef CAS.
- K. M. Spencer, S. J. Cianciosi, J. E. Baldwin, T. B. Freedman and L. A. Nafie, Appl. Spectrosc., 1990, 44, 235 CrossRef CAS.
- L. A. Nafie, F. J. Long, T. B. Freedman, H. Buijs, A. Rilling, J. R. Roy and R. K. Dukor, The eleventh international conference on Fourier transform spectroscopy, 1998, vol. 430, p. 432 Search PubMed.
- Z. Guo, X. G. Hu, G. Y. Fang, S. Shao, A. Guo and H. Y. Liang, Thermochim. Acta, 2012, 534, 51 CrossRef CAS.
- Z. Guo, X. G. Hu, G. Y. Fang, S. Shao, A. Guo and H. J. Zhang, J. Chem. Eng. Data, 2011, 56, 2489 CrossRef.
- G. X. Song, F. L. Zhou, C. L. Xu and B. X. Li, Analyst, 2016, 141, 1257 RSC.
- G. X. Song, F. L. Zhou and B. X. Li, Sens. Actuators, B, 2015, 215, 504 CrossRef CAS.
- C. W. Liu, J. W. Lian, Q. Liu, C. L. Xu and B. X. Li, Anal. Methods, 2016, 8, 5794 RSC.
- S. Agatonovic-Kustrin, R. Beresford and M. Razzak, Anal. Chim. Acta, 2000, 417, 31 CrossRef CAS.
- S. Agatonovic-Kustrin and R. Alany, Anal. Chim. Acta, 2001, 449, 157 CrossRef CAS.
- M. Yamaguchi, F. Miyamaru, K. Yamamoto, M. Tani and M. Hangyo, Phys. Lett., 2005, 86, 822 Search PubMed.
- R. Nishikiori, M. Yamaguchi, K. Takano, T. Enatsu, M. Tani, N. Kawashita, T. Takagi, S. Morimoto, M. Hangyo and M. Kawase, Chem. Pharm. Bull., 2008, 56, 305 CrossRef CAS PubMed.
- X. L. Li, K. L. Xu, H. Li, S. Yao, Y. F. Li and B. Liang, RSC Adv., 2016, 6, 8395 RSC.
- B. Liang, X. L. Li, X. L Jing, T. Li and S. Yao, J. Sichuan Univ., Eng. Sci. Ed., 2016, 48, 191 Search PubMed.
- S. Datta and D. J. Grant, Nat. Rev. Drug Discovery, 2004, 3, 42 CrossRef CAS PubMed.
- Y. Y. Feng, X. L. Li, K. L. Xu, H. Y. Zou, H. Li and B. Liang, J. Pharm. Biomed. Anal., 2015, 104, 112 CrossRef CAS PubMed.
- P. Esseiva, F. Anglada, L. Dujourdy, F. Taroni, P. Margot, E. D. Pasquier, M. Dawson, C. Roux and P. Doble, Talanta, 2005, 67, 360 CrossRef CAS PubMed.
- S. Rezzi, D. E. Axelson, K. Héberger, F. Reniero, C. Mariani and C. Guillou, Anal. Chim. Acta, 2005, 552, 13 CrossRef CAS.
- I. T. Jolliffe, Principal Component Analysis, Springer, Berlin, New York, 2nd edn, 1986 Search PubMed.
- L. Norgaard, A. Saudland, J. Wagner, J. P. Nielsen, L. Munck and S. B. Engels, Appl. Spectrosc., 2000, 54, 413 CrossRef CAS.
- W. Luo, J. Wu, X. Wang, X. Lin and H. Li, Anal. Methods, 2013, 5, 1337 RSC.
- U. Thissen, M. Pepers, B. Üstün, W. J. Melssen and L. M. C. Buydens, Chemom. Intell. Lab. Syst., 2004, 73, 169 CrossRef CAS.
|
This journal is © The Royal Society of Chemistry 2017 |
Click here to see how this site uses Cookies. View our privacy policy here.