DOI:
10.1039/C6RA24152B
(Paper)
RSC Adv., 2017,
7, 1915-1922
A metabolomics approach for predicting the response to intravenous iron therapy in peritoneal dialysis patients with anemia
Received
28th September 2016
, Accepted 2nd December 2016
First published on 7th December 2016
Abstract
Anemia is an almost universal complication of chronic kidney disease (CKD), and nearly all patients with end-stage renal disease (ESRD) and approximately 70% of those with earlier stages of CKD receive treatment for anemia. Due to its significance in the treatment of anemia, there is increased reliance on iron in the renal anemia population. In clinical practice, not every patient benefits from intravenous (IV) iron therapy. In order to identify patients who will respond to IV iron therapy and who will not respond to it, our goals were to identify the potential serum biomarkers that could predict the response to IV iron therapy in renal anemia patients. The metabolic profiles of serum from 41 renal anemia patients with complete, partial or non-response to IV iron therapy were studied using a combination of liquid chromatography coupled with mass spectrometry (LC-MS) and multivariate analysis methods to identify the potential biomarkers that could predict the response to IV iron therapy in renal anemia patients. Oleamide and ascorbate 2-sulfate (AS) were identified and verified as the potential biomarkers. A prediction model constructed with oleamide and AS correctly identified approximately 83.3% of patients who were non-responsive to IV iron therapy and 87.5% of patients who had a complete response to IV iron therapy. The model has excellent discriminant performance, with an AUC of 0.901. These results show promise for larger studies that could advance more personalized treatment protocols for renal anemia patients.
Introduction
Iron is an essential ingredient for human health, which plays an important role in many vital biological process such as the synthesis of heme which forms the basis of hemoglobin (Hb), the oxygen-carrying protein of the blood, the formation of myoglobin, energy metabolism, neurotransmitter production, the formation of collagen and immune system function.1,2 Nonetheless, iron deficiency (ID) is one of the principal causes of anemia in the general population, affecting approximately 25% of the world's population.3 In 2010, global anemia prevalence was 32.9%, affecting over 2.2 billion people.4 Iron deficiency anemia (IDA) is a frequently reported complication of pregnancy and conditions such as CKD, cancer, chronic heart failure, inflammatory bowel disease (IBD), and heavy uterine bleeding, and the management of anemia that can occur during the postpartum period and after gastric bypass surgery,5–13 which can further worsen the prognosis and patient's quality of life, increase morbidity and mortality. Thus, treatment of IDA has important meaning for improving prognosis and quality of life for these patients.
Iron supplementation combined with erythropoiesis-stimulating agents (ESAs) is the standard procedure for management of IDA.14 Iron supplementation can be achieved by oral or IV administration, each with its own set of advantages and disadvantages.15–17 Oral iron generally is safe but can cause gastrointestinal side effects and poor compliance. Although IV iron preparations are fast, easy, well tolerated and effective, their indiscriminate use can have serious adverse consequences such as infection, oxidative stress, cardiovascular disease, iron overload and even life-threatening anaphylactic reactions that may go undetected in short-term clinical trials.18 Therefore, screening predictors of response to the iron supplementation is vital for the treatment of IDA. By far, as a set of suboptimal iron indices, transferrin saturation (TSAT) and serum ferritin (SF) are frequently used to predict ID but limited by sensitivity.19 In a small, single-arm interventional trial, the sensitivity of iron indices to predict responsiveness to intravenous iron in anemic patients with peritoneal dialysis-dependent chronic kidney disease (PD-CKD) was reported as low.20 In summary, looking for new biomarkers as predictors for response to iron supplements combined with existing biomarkers is critical for the timely adjustment of iron supplements strategies and the improvement of clinical outcomes in IDA treatment.
Based on the premise that the interactions of iron with biological systems consistently affects metabolisms, thus leading to altered levels of endogenous metabolites, metabolomics (sometimes referred as “metabolic profiling” or “metabonomics”) involving the quantitative detection of hundreds of small molecules in biological samples that are sensitive to pathophysiological stimuli or genetic modification.21–26 Pharmacometabonomics, as part of metabolomics, has been reported to be wildly used to map and predict the interventional outcome of drugs (such as toxicity and xenobiotic metabolism in biological systems) based on mathematical models derived from pre-dose, biofluid metabolite profiles,27 which is a quite suitable tool to be used to predict the response to IV iron therapy in IDA patients. In the past few years, LC-MS, an information-rich analytical technique, has become one of the most popular and useful tools.28 Importantly, serum tests based on metabolic profiles are relatively cheap, rapid and automated.29 Although metabolomics has been widely used in molecule discovery for early diagnosis, disease detection, targeted therapy and drug response,30–32 so far almost no investigation have been performed in the biomarker discovery for predicting the response to iron supplements in IDA patients.
In this prospective study, serum metabolite profiling was performed to identify the potential biomarkers that could predict the response to IV iron therapy for PD patients with renal anemia. The metabolic profiles of serum from PD patients with a complete, partial and non-response to IV iron therapy were studied using a combination of LC/MS and multivariate analysis methods. The predictive performance was evaluated in terms of sensitivity, specificity and accuracy based on the prediction model constructed by the potential biomarkers. These results showed promise for larger studies that would provide more personalized treatment protocols for renal anemia patients.
Materials and methods
Study group
Between September 2012 and December 2013, two-hundred PD patients were selected if they were older than 18 years and had been receiving maintenance PD regimen for three months, their condition had been stable for at least 1 month, and they were iron deficiency, with SF level < 500 ng mL−1, TSAT < 30%, and they had Hb < 110 g L−1 (men) or 100 g L−1 (women). Exclusion criteria were the following: history of sensitive to iron preparations; history of severe liver disease or hypersplenism; history of hemorrhage or active ulcer; receipt of blood transfusion within the last one month; active infection; malignant tumors; history of chronic inflammation disease; severe malnutrition.
Treatments
All patients were first administered a test dose of 25 mg IV iron dextran preparation (Cosmofer) mixed in 100 mL normal saline which was infused over 60 min with monitoring for any possible adverse reactions. If no reaction occurred, iron dextran was administered as a TDI of 475 mg diluted in 500 mL normal saline over a period of 4–6 h with careful monitoring. Other routine drugs, including folic acid (10 mg, 3 times per day) and vitamin B12 (500 mg, daily), were continued. All patients received erythropoietin (EPO) (Shenyang Sansheng Pharmaceutical, Shenyang, China) at a dose of 100–150 IU per kg per week (depending on previous treatment). If Hb reached 120 g L−1 (men) or 110 g L−1 (women), the EPO dose was decreased by 25%.
Side effects
Any reactions such as gastrointestinal symptoms, flushing, palpitation, or hypersensitivity during the intravenous administration were recorded.
Blood biochemistry
Blood samples were drawn before the first IV infusion (0 months) and after three months for a hemogram and a ferrokinetic profile which was comprised of Hb, Hct, reticulocytes, SF, serum iron and total iron binding capacity. TSAT was calculated using the following equation: serum iron/total iron binding capacity × 100. Based on laboratory test results after three months, Hb rising no less than 10 g L−1 or Hct rising no less than 4% can be defined as good response (Complete Response, CR); Hb increasing less than 10 g L−1 or Hct increasing less than 4% can be defined as poor response (Partial Response, PR); no increase in Hb or Hct can be defined as no response (Stable Disease, SD).
Sample collection and preparation
Written consent was collected from all of the patients who participated in this study. The protocol of the study and the procedures designed for sample collection were reviewed and approved by the ethical committee of The Second Military Medical University, Shanghai, China. All procedures involving the human subjects were carried out in accordance with the recommendations of the Helsinki Declaration. Venous blood samples were obtained from patients recruited at the Department of Nephrology, Changhai Hospital of the Second Military Medical University. Blood sample collection from the patient was done at the same day. Parameters including gender, age, duration of PD, haemoglobin, serum albumin, BUN, creatinine, corrected calcium, phosphate, total cholesterol, triglyceride, iPTH levels from each patient were recorded at the sampling. Venous blood was collected into a 5 mL Vacutainer tube containing chelating agent ethylene diamine tetraacetic acid (EDTA). The tube was centrifuged at 3000 rpm for 15 minutes. The supernatant (serum sample) was aliquoted and stored at −80 °C until analysis. No sample went more than two freeze–thaw cycles prior to a LC-MS analysis.
The serum sample (100 μL) were thawed at 4 °C followed by the addition of 400 μL methanol/acetonitrile (1
:
1). The mixture was then vortexed vigorously for 30 s followed by centrifugation at 14
000 × g for 15 min at 4 °C. The supernatant (50 μL) was transferred to an autosampler vial and an aliquot of 4 μL was injected for LC-MS analysis.
Global metabolite profiling
In this study, an Agilent 1290 Infinity LC system configured with an Agilent 6530 Accurate-Mass Quadrupole Time-of-Flight (Q-TOF) mass spectrometer (Agilent, USA) was used to perform the LC-MS analysis. An ACQUITY UPLC HSS T3 column (2.1 mm × 100 mm, 1.8 μm, Waters, Milford, MA, USA) was applied to separate serum samples at 45 °C with a flow rate of 0.4 mL min−1. The mobile phase: A, 0.1% formic acid; B, ACN modified with 0.1% formic acid. The gradient program was used as follows: 100% A at 0–2 min, 100–85% A at 2–10 min, 85–70% A at 10–14 min, 70–5% A at 14–17 min, 5% A at 17–19 min, 5–100% A at 19–20 min and followed by 5 min column re-equilibration.
An Agilent 6530 Accurate Mass Quadrupole Time-of-Flight (Q-TOF) mass spectrometer (Agilent, Santa Clara, CA, USA) was adapted to detect ion peaks. The cone gas was nitrogen with a flow rate of 11 L h−1. The following detection parameters was used: fragment voltage, 120 V; capillary voltage, 3.5 kV; gas temperature, 350 °C; source temperature, 120 °C. The full MS scan mode was monitored at the mass range of 50–1000 m/z. In the analyzing process, 10 mM purine (m/z 121.0508) and 2 mM hexakis phosphazinen (m/z 922.0097) were applied as the internal standards to guarantee mass accuracy and reproducibility. The centroid data were collected from the instrument. Subsequently, a MS/MS experiment was performed and the experiment parameters was set as follows: MS spectrum acquisition rate, 2 spectra per s; MS/MS spectrum acquisition rate, 0.5 spectra per s; medium isolation window, 4 m/z; collision energy, 20 V.
Data handing
Data processing used the method previously published by our group with minor modifications.33 The raw data in instrument specific format (.d) were converted to common data format (.mzData) files using a conversion software program (file converter program available in Agilent MassHunter Qualitative software), in which the isotope interferences were eliminated. The program XCMS (version, 1.40.0) (http://masspec.scripps.edu/xcms/xcms.php) was used for nonlinear alignment of the data in the time domain and automatic integration and extraction of the peak intensities. XCMS parameters were default settings (major default parameters: profmethod = bin; method = matched filter; step = 0.1) except for the following: full width at half maximum (FWHM) = 8, bandwidth (bw) = 10 and snthresh = 5, due to narrower peaks obtained by the use of the column packed with 1.8 μm particles. The variables presenting in at least 80% of either group were extracted, and the variables with a retention time less than 0.5 min (near to the dead time) were excluded due to a high degree of ion suppression that they suffered. For each chromatogram, the intensity of each ion was normalized to the total ion intensity, in order to partially compensate for the concentration bias of metabolites between samples and to obtain the relative intensity of metabolites. The resulting three-dimensional matrix, including retention time and m/z pairs (variable indices), sample names (observations), and normalized ion intensities (variables), was exported to multivariate data analysis.
The normalized data was introduced to SIMCA-P V11.0 (Umetrics, Sweden) for principal component analysis (PCA) and partial least squares discriminant analysis (PLS-DA) after mean-centering and pareto scaling, a technique that increased the importance of low abundance ions without significant amplification of noise. The quality of the models was evaluated with the permutation test. T-test was performed in succession to reveal the statistical differences for the variables between the three groups (CR & PR & SD).
Results
Study groups and their characteristics
Between September 2012 and December 2013, 41 eligible previously untreated patients who met the inclusion and exclusion criteria were enrolled in this prospective study, of whom 16 patients were diagnosed as CR, 13 patients were PR, while the remaining 12 patients were non-responsive to IV iron therapy based on the biochemical examination. The demographic and clinical characteristics of the prospective cohort are shown in Table 1. The baseline characteristics were comparable in each group. In addition, a single baseline ferritin (Fe) was used as classification criteria, patients whose Fe < 100 ng mL−1 were divided into two categories, patients with Fe < 50 ng mL−1 were regarded as class 1, and the remainders were regarded as class 2. Only about 60% patients who defined as CR were belongs to class 1. Similarly, the baseline TSAT was used as classification criteria, patients whose TSAT < 20% were divided into two categories, patients with TSAT < 10% were regarded as class 1, the rest were regarded as class 2. Only about 60% patients who defined as CR were belongs to class 1 (data not shown). Therefore, lower baseline SF or TSAT does not necessarily indicate better iron therapy response.
Table 1 Baseline biochemical parameters in the three groupsa
Parameters |
SD |
PR |
CR |
P Value |
Hb, hemoglobin; Hct, hematocrit; Kt/V, dialyzer clearance of urea, dialysis time, volume of distribution of urea, approximately equal to patient's total body water; TSAT, transferrin saturation; Fer, ferritin; Alb, albumin; iPTH, intact parathyroid hormone; CR: complete response; PR: partial response; SD: stable disease; N.S, no significance. |
Age, years |
57.34 ± 13.41 |
60.05 ± 16.91 |
58.98 ± 12.24 |
N.S |
Dialysis vintage, months |
21.35 ± 15.29 |
26.88 ± 13.48 |
23.79 ± 12.83 |
N.S |
Hb, g L−1 |
89.68 ± 10.39 |
80.77 ± 11.24 |
83.65 ± 15.87 |
N.S |
Hct, % |
22.62 ± 5.45 |
21.09 ± 4.71 |
13.96 ± 7.96 |
N.S |
Kt/V |
1.84 ± 0.38 |
1.72 ± 0.32 |
1.71 ± 0.21 |
N.S |
TSAT, % |
19.22 ± 5.08 |
15.36 ± 6.52 |
13.87 ± 6.94 |
0.057 |
Fer, μg L−1 |
224.58(124.34–302.46) |
167.45(78.24–202.45) |
135.91(89.25–254.17) |
0.036 |
Alb, g L−1 |
37.25 ± 4.25 |
39.36 ± 3.22 |
38.58 ± 5.54 |
N.S |
iPTH, pg mL−1 |
278.17(123.14–356.43) |
224.45(109.52–295.75) |
248.67(97.29–309.05) |
N.S |
Serum metabolic profiles
In order to visualize the classification performance of the metabolic profiling, the PLS-DA score plot is depicted (Fig. 1a and d). Fig. 1a and d reveal a clear separation trend between CR and SD. The PR group lies in the between CR and SD, while no clear separation was found between CR and PR in Fig. 1d and no clear separation was found between SD and PR in Fig. 1a. To validate the model, permutation tests with 200 iterations were further performed. These permutation tests compared the goodness of fit of the original model with the goodness of fit of randomly permuted models. As shown in Fig. 1c and f, the validation plot indicates that the original model is valid. The criteria for validity are as follows: all the permuted R2 (cum) and Q2 (cum) values to the left are lower than the original point to the right, and the blue regression line of the Q2 (cum) points has a negative intercept.
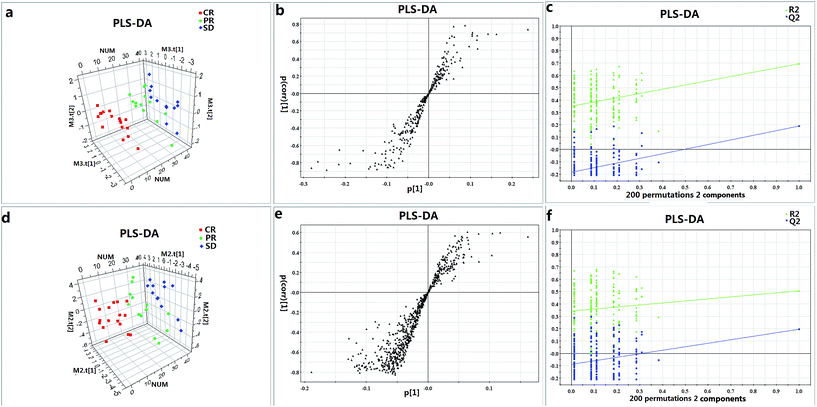 |
| Fig. 1 Multivariate data analysis. (a) PLS-DA score map for the CR, PR and SD patients in positive mode; (b) S-plot of the PLS-DA model in positive mode; (c) validation plot obtained from 200 permutation tests in positive mode; (d) PLS-DA score map for the CR, PR and SD patients in negative mode; (e) S-plot of the PLS-DA model in negative mode; (f) validation plot obtained from 200 permutation tests in negative mode. | |
The discovery and identification of metabolic biomarkers
Metabolites were carefully screened before being approved as potential biomarkers. First, significant original variables were extracted from the S-plot, which is a covariance-correlation-based procedure, and thus the risk of false positives in metabolite selection was reduced. The S-plot (Fig. 1b and e), derived from the first component of the combined model, explains most of the variables in data set, in which the ions furthest away from the origin contribute significantly to the clustering of the three groups and may be regarded as potential biomarkers (as showed in Fig. 1b and e). Next, the variable importance for projection (VIP) reflecting the importance of variables has been applied to filter the important metabolites in the model (VIP ≥ 1). Furthermore, unpaired Student's t-tests were performed as the final testing procedure, and the critical p-value was set to 0.05 for significantly differential variables. Following the criterion above, 11 metabolite ions (as showed in Table 2) were selected as potential biomarkers related to response to iron therapy. In addition, the bar plots for the 11 potential biomarkers are given in Fig. 2, it is seen that the concentrations of LysoPC (18
:
1(11Z)), linoleic acid and arachidonic acid had the consistently decreased trend from CR to PR to SD, the concentrations of oleamide, 1-linoleoyl glycerol, citric acid, AS, mesaconic acid, LysoPC (20
:
1(11Z)) and alpha-hydroxyhippuric acid had the consistently increased trend from CR to PR to SD. Thus, these metabolites can be further used to test their prediction performance for response to iron therapy for PD patients.
Table 2 Summary of the potential biomarkersa
|
No. |
Metabolites |
m/z |
RT (min) |
Adduct |
Formula |
VIP |
p Value |
ESI+, electrospray ionization in positive mode; ESI−, electrospray ionization in negative mode; VIP, variable importance in the project. |
ESI+ |
1 |
LysoPC (18 : 0) |
524.37 |
15.02 |
[M + H]+ |
C26H54NO7P |
3.4 |
0.04 |
2 |
LysoPC (18 : 1(11Z)) |
522.36 |
13.90 |
[M + H]+ |
C26H52NO7P |
3.01 |
0.02 |
3 |
Oleamide |
282.28 |
16.71 |
[M + H]+ |
C18H35NO |
2.07 |
0.04 |
4 |
Linoleic acid |
281.25 |
16.90 |
[M + H]+ |
C18H32O2 |
1.04 |
0.04 |
5 |
1-Linoleoyl glycerol |
355.28 |
16.10 |
[M + H]+ |
C21H38O4 |
1.01 |
0.03 |
ESI− |
1 |
LysoPC (18 : 0) |
568.36 |
14.87 |
[M + FA-H]− |
C26H54NO7P |
3.17 |
0.03 |
6 |
Citric acid |
191.02 |
0.83 |
[M − H]− |
C6H8O7 |
2.79 |
0.03 |
7 |
Ascorbate 2-sulfate |
254.98 |
1.01 |
[M − H]− |
C6H8O9S |
2.42 |
0.01 |
8 |
Mesaconic acid |
129.02 |
1.01 |
[M − H]− |
C5H6O4 |
1.73 |
0.01 |
9 |
Arachidonic acid |
303.23 |
16.74 |
[M − H]− |
C20H32O2 |
1.72 |
0.05 |
10 |
LysoPC (20 : 1(11Z)) |
594.38 |
15.36 |
[M + FA-H]− |
C28H56NO7P |
1.27 |
0.04 |
11 |
α-Hydroxyhippuric acid |
194.05 |
3.43 |
[M − H]− |
C9H9NO4 |
1.24 |
0.04 |
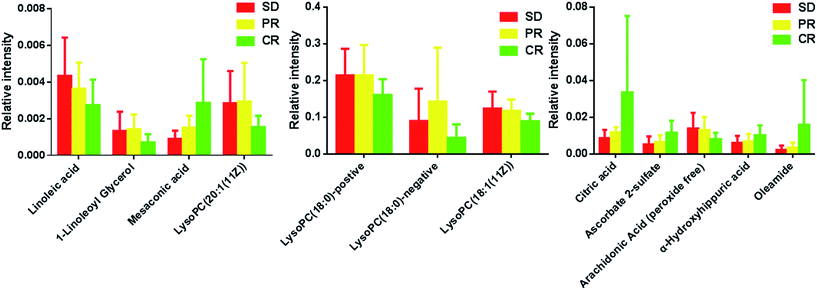 |
| Fig. 2 Bar plots showing fluctuations in relative signal intensities of potential biomarkers for SD, PR and CR patients. | |
Biomarkers for the prediction of a response to IV iron therapy
To further validate the potential diagnostic effectiveness of these metabolite signature, the receiver operating characteristic curve (ROC-curve) was plotted individually using relative intensities of these metabolites (data not shown). In addition, the purpose of the study was to screen the potential biomarkers that can distinguish CR from SD, CR from PR, CR from (SD & PR) and PR from SD, thus based on the criteria of area under the ROC-curve (AUROC) greater than 0.5, sensitivity greater than 0.5 and specificity greater than 0.5, oleamide and AS were finally identified as the potential biomarkers that used to predict the response to IV iron therapy.
Further analysis focused on evaluation the performance of the metabolites in combination. Fig. 3 shows the prediction results using the model constructed by the two candidate markers for different patients groups. Logistic regression was used to combine the two variables into a multivariable. The results indicated that a panel of two metabolites generated an AUC of 0.901 with a sensitivity of 87.5% and a specificity of 83.3% for distinguishing CR and SD, an AUC of 0.850 with a sensitivity of 81.2% and a specificity of 76% for distinguishing CR and (PR & SD), an AUC of 0.813 with a sensitivity of 87.5% and a specificity of 69.2% for distinguishing CR and PR and an AUC of 0.667 with a sensitivity of 92.3% and a specificity of 33.3% for distinguishing PR and SD, respectively (Fig. 3a). According to the highest prediction sensitivity (87.5%) and specificity (83.3%) of the ROC curves, an optimal cutoff value of 0.4603 was obtained. Based on this cutoff value, it was found that 24 out of 28 samples (85.7%) could be accurately predicted for distinguishing CR and SD, similarly, 32 out of 41 samples (78%) could be accurately predicted for distinguishing CR and (PR & SD), 23 out of 29 samples (79.3%) could be accurately predicted for distinguishing CR and PR, 17 out of 25 samples (68%) could be accurately predicted for distinguishing PR and SD (Fig. 3b). This finding indicated that this simplified serum metabolite signature was a “good” classifier of CR, PR and SD patients.
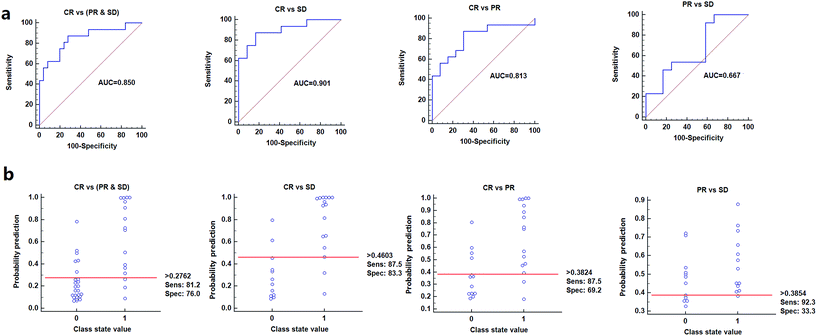 |
| Fig. 3 (a) ROC curves based on the binary logistic regression model using the combination of two serum metabolites (oleamide and ascorbate 2-sulfate); (b) their prediction plots based on the optimal cutoff value obtained from the ROC curves. | |
Discussion
In this study, we present the metabolomics approach for predicting the IV iron therapy response for renal anemia patients before iron infusion. By applying ultra-performance liquid chromatography coupled to time-of-flight mass spectrometry, a powerful technique that has a high sensitivity and specificity, oleamide and AS are shown to be highly correlated with CR. Although there is heterogeneity in the clinical and histopathological characteristics of patients in each response group, the serum samples still can group them into distinct clusters.
The prediction model constructed with the selected model has a high sensitivity and specificity. Since the IV iron therapy response prediction for IDA remains challenging around the world, this promising metabolomics approach might open a new view for patients to select the promising treatment or even a truly ‘personalized treatment’ in clinical practice. Importantly, as seen from the multivariate regression analysis between the IV iron therapy responses and the predictors consisting of biomarkers, the selected biomarkers are strongly associated with the IV iron therapy response.
The prediction performance of the two biomarkers detected by LC-MS analysis was excellent and showed an AUC of 0.901 in the discrimination of CR from SD. In addition, the metabolites detected by LC-MS provide a better insight into the cellular metabolism and provide a more robust model that could effectively predict the iron therapy response when validated in a larger cohort of patients. Two metabolites from LC-MS distinguish the three renal anemia patients groups, CR, PR and SD with a good performance. The excellent classification performance through the serum metabolites suggests that the metabolomics approach might be particularly noteworthy when the prediction of the response to iron supplements in IDA patients remains challenging around the world.
The metabolic profiling difference between the three groups (CR, PR, SD) may indicate the further response to IV ion therapy in IDA patients. AS, a naturally occurring substance, is reported to be produced from ascorbic acid (AA). Moreover, AS, being more stable than AA, may be a storage from in vivo and serve as an AA source through enzymatic hydrolysis.34,35 However, previous study has reported that AA can affect the absorption and distribution of iron both at the absorption and metabolic level, have a positive effect on the absorption of iron from the gastrointestinal tract and have an important role in the release of ferritin-bound iron from the liver as well as in transfer of plasma iron to the liver and its incorporation into ferritin.36 The detailed action mechanism is that lysosomal autophagy catabolize excess ferritin into end-state hemosiderin complexes, however, AA can inhibit this process and block the degradation of cytoplasmic ferritin, and finally keep the iron stored in the body as ferritin-bound iron.37,38 In our study, a higher level of AAS can be found in the CR group, which means CR group have higher level of AA and leading to higher level of ferritin-bound iron be stored and used, which is consistent with the reported literatures. Oleamide, as one of the two identified biomarkers, is the amide of oleic acid, and is synthesized from oleic acid and ammonia by enzymatic amidation, and previous study showed that oleamide has high anti-inflammatory activity.39 However, the observation of higher levels of oleamide in good responders as compared with poor responders is not completely understood. Although further functional work is needed, we posit one conceive that may be responsible for this observation. The anti-inflammatory activity of oleamide may alleviate the intravenous iron stimuli in patients who underwent IV iron therapy and result in a better response.
Conclusions
We present a prediction model for the outcome of renal anemia IV iron therapy based on metabolic profiling studies. A combination of two metabolites (oleamide and AS) distinguish groups of patients with no, partial or complete response. While this pilot study was focused on a small patient cohort, it clearly indicates that several blood-based metabolite markers are sensitive to response, and that the approach is promising for predicting the response to IV iron therapy. In addition, the response evaluation in this study is not limited to IV iron therapy in PD patients, but can be extended to pregnancy and conditions such as cancer, chronic heart failure and IBD that accompanied by complication of IDA; however, further large scale cohort studies on these IDAs should be performed to substantiate the results. Validation of these results using a larger and independent sample cohort, and identification of additional metabolite markers, will provide better insights into the pathology at the molecular level that lead to different response outcomes for the three groups of patients. This approach, which clearly differentiates patients that respond to iron supplements from those that do not, may provide a useful tool for a non-invasive prognosis of the treatment outcome.
Abbreviations
CKD | Chronic kidney disease |
ESRD | End stage renal disease |
IV | Intravenous |
LC/MS | Liquid chromatography coupled with mass spectrometry |
AS | Ascorbate 2-sulfate |
ID | Iron deficiency |
IDA | Iron deficiency anemia |
IBD | Inflammatory bowel disease |
ESAs | Erythropoiesis-stimulating agents |
Hb | Hemoglobin |
TSAT | Transferrin saturation |
SF | Serum ferritin |
PD-CKD | Dialysis-dependent chronic kidney disease |
EPO | Erythropoietin |
Hct | Hematocrit |
CR | Complete response |
PR | Partial response |
SD | Stable disease |
PCA | Principal component analysis |
PLS-DA | Partial least squares discriminant analysis |
Acknowledgements
The study was funded by the National Natural Science Foundation of China (No. 81273472 & No. 81573396), China Postdoctoral Science Foundation Grant (2014M562591), Research Program from Health and Family Planning Commission of Shanghai (20134Y035) and Renal Research Grant of Baxter China (CHN-RENAL-IIS-2010-068).
Notes and references
- A. Robles-Mezcua, N. Gonzalez-Cruces, A. Ruiz-Salas, L. Morcillo-Hidalgo, J. Robledo-Carmona, J. J. Gomez-Doblas, E. de Teresa and J. M. Garcia-Pinilla, Int. J. Cardiol., 2016, 202, 118–120 CrossRef PubMed.
- R. D. Cançado, P. O. N. de Figueiredo, M. C. A. Olivato and C. S. Chiattone, Revista Brasileira de Hematologia e Hemoterapia, 2011, 33, 461–469 CrossRef PubMed.
- R. D. Cancado, P. O. de Figueiredo, M. C. Olivato and C. S. Chiattone, Revista Brasileira de Hematologia e Hemoterapia, 2011, 33, 439–443 CrossRef PubMed.
- M. Auerbach, J. Adamson, A. Bircher, C. Breymann, S. Fishbane, A. Gafter-Gvili, C. Gasche, J. Gilreath, G. Grazzini, D. Henry, G. Liumbruno, F. Locatelli, I. Macdougall, M. Munoz, D. Rampton, G. Rodgers and A. Shander, Haematologica, 2015, 100, e214–215 CrossRef PubMed.
- T. Lee, T. Clavel, K. Smirnov, A. Schmidt, I. Lagkouvardos, M. Lucio, A. Walker, B. Michalke, P. Schmitt-Kopplin, R. Fedorak and D. Haller, Gut, 2016, 10 CAS.
- C. Breymann, F. Gliga, C. Bejenariu and N. Strizhova, Int. J. Gynecol. Obstet., 2008, 101, 67–73 CrossRef CAS PubMed.
- C. Gasche, A. Berstad, R. Befrits, C. Beglinger, A. Dignass, K. Erichsen, F. Gomollon, H. Hjortswang, I. Koutroubakis, S. Kulnigg, B. Oldenburg, D. Rampton, O. Schroeder, J. Stein, S. Travis and G. Van Assche, Inflammatory Bowel Dis., 2007, 13, 1545–1553 CrossRef PubMed.
- A. A. Khalafallah and A. E. Dennis, J. Pregnancy, 2012, 2012, 630519 Search PubMed.
- A. L. Love and H. H. Billett, Am. J. Hematol., 2008, 83, 403–409 CrossRef PubMed.
- R. Shah and A. K. Agarwal, Clin. Interventions Aging, 2013, 8, 111–122 CAS.
- D. B. Van Wyck, A. Mangione, J. Morrison, P. E. Hadley, J. A. Jehle and L. T. Goodnough, Transfusion, 2009, 49, 2719–2728 CrossRef CAS PubMed.
- D. B. Van Wyck, M. Roppolo, C. O. Martinez, R. M. Mazey, S. McMurray and G. United States, Iron Sucrose Clinical Trials, Kidney Int., 2005, 68, 2846–2856 CrossRef CAS PubMed.
- G. R. Bailie, Am. J. Health-Syst. Pharm., 2012, 69, 310–320 CrossRef CAS PubMed.
- S. Sinha, D. Y. Chiu, G. Peebles, S. Kolakkat, E. Lamerton, S. Fenwick and P. A. Kalra, Journal of Renal Care, 2009, 35, 67–73 CrossRef PubMed.
- K. Jimenez, S. Kulnigg-Dabsch and C. Gasche, J. Gastroenterol. Hepatol., 2015, 11, 10 Search PubMed.
- P. A. Kalra and S. Bhandari, Curr. Opin. Nephrol. Hypertens., 2016, 25, 529–535 CrossRef CAS PubMed.
- S. Macher, C. Drexler, I. Lindenau, N. Sareban, P. Schlenke and K. Amrein, Trials, 2016, 17, 527 CrossRef PubMed.
- N. D. Vaziri, K. Kalantar-Zadeh and J. B. Wish, Am. J. Kidney Dis., 2015, 9 Search PubMed.
- A. Besarab, S. Frinak and J. Yee, J. Am. Soc. Nephrol., 1999, 10, 2029–2043 CAS.
- R. A. Zager, Clin. J. Am. Soc. Nephrol., 2006, 1, 353–355 CrossRef CAS PubMed.
- L. M. Yerges-Armstrong, S. Ellero-Simatos, A. Georgiades, H. Zhu, J. P. Lewis, R. B. Horenstein, A. L. Beitelshees, A. Dane, T. Reijmers, T. Hankemeier, O. Fiehn, A. R. Shuldiner and R. Kaddurah-Daouk, Clin. Pharmacol. Ther., 2013, 94, 525–532 CrossRef CAS PubMed.
- R. Kaddurah-Daouk, R. A. Baillie, H. Zhu, Z. B. Zeng, M. M. Wiest, U. T. Nguyen, S. M. Watkins and R. M. Krauss, Metabolomics, 2010, 6, 191–201 CrossRef CAS PubMed.
- J. H. Winnike, Z. Li, F. A. Wright, J. M. Macdonald, T. M. O'Connell and P. B. Watkins, Clin. Pharmacol. Ther., 2010, 88, 45–51 CrossRef CAS PubMed.
- Q. Liang, H. Liu, X. Li and A.-H. Zhang, RSC Adv., 2016, 6, 75499–75504 RSC.
- Y. Liu, Q. Song, J. Zheng, J. Li, Y. Zhao, C. Li, Y. Song and P. Tu, RSC Adv., 2016, 6, 81826–81837 RSC.
- Y. Xiao, Q. Zhai, G. Wang, X. Liu, J. Zhao, F. Tian, H. Zhang and W. Chen, RSC Adv., 2016, 6, 78445–78456 RSC.
- J. K. Nicholson, J. R. Everett and J. C. Lindon, Expert Opin. Drug Metab. Toxicol., 2012, 8, 5 CrossRef PubMed.
- Y. Hou, M. Yin, F. Sun, T. Zhang, X. Zhou, H. Li, J. Zheng, X. Chen, C. Li, X. Ning, G. Lou and K. Li, Mol. BioSyst., 2014, 10, 2126–2133 RSC.
- S. Wei, L. Liu, J. Zhang, J. Bowers, G. A. Gowda, H. Seeger, T. Fehm, H. J. Neubauer, U. Vogel, S. E. Clare and D. Raftery, Mol. Oncol., 2013, 7, 297–307 CrossRef CAS PubMed.
- M. D. Cao, G. F. Giskeodegard, T. F. Bathen, B. Sitter, A. Bofin, P. E. Lonning, S. Lundgren and I. S. Gribbestad, BMC Cancer, 2012, 12, 39 CrossRef CAS PubMed.
- I. F. Duarte, A. F. Ladeirinha, I. Lamego, A. M. Gil, L. Carvalho, I. M. Carreira and J. B. Melo, Mol. Pharm., 2013, 10, 4242–4251 CrossRef CAS PubMed.
- G. Huang, X. Liu, L. Jiao, C. Xu, Z. Zhang, L. Wang, Y. Li, C. Yang, W. Zhang and Y. Sun, Eur. J. Pharmacol., 2014, 729, 132–137 CrossRef CAS PubMed.
- L. Guo, G. Tan, P. Liu, H. Li, L. Tang, L. Huang and Q. Ren, Sci. Rep., 2015, 5, 15126 CrossRef CAS PubMed.
- L. V. Benitez and J. E. Halver, Proc. Natl. Acad. Sci. U. S. A., 1982, 79, 5 CrossRef.
- H. Hatanaka, F. Egami, T. Kato and T. Nagatsu, J. Biochem., 1975, 78, 4 Search PubMed.
- A. Mazur, S. Green and A. Carleton, J. Biochem., 1960, 235, 10 Search PubMed.
- K. R. Bridges, J. Biol. Chem., 1987, 262, 6 Search PubMed.
- A. Maage, R. Waagbø, P. E. Olsson, K. Julshamn and K. Sandnes, Fish Physiol. Biochem., 1990, 8, 8 Search PubMed.
- Y. Ano, M. Ozawa, T. Kutsukake, S. Sugiyama, K. Uchida, A. Yoshida and H. Nakayama, PLoS One, 2015, 10, e0118512 Search PubMed.
Footnote |
† Contributed equally to this work. |
|
This journal is © The Royal Society of Chemistry 2017 |
Click here to see how this site uses Cookies. View our privacy policy here.