DOI:
10.1039/C6NR05609A
(Paper)
Nanoscale, 2017,
9, 349-354
A protein corona-enabled blood test for early cancer detection†
Received
15th July 2016
, Accepted 22nd November 2016
First published on 23rd November 2016
Abstract
Pancreatic cancer is a very aggressive malignancy that is often diagnosed in the advanced stages, with the implication that long-term survivors are extremely rare. Thus, developing new methods for the early detection of pancreatic cancer is an urgent task for current research. To date, nanotechnology offers unprecedented opportunities for cancer therapeutics and diagnosis. The aim of this study is the development of a new pancreatic cancer diagnostic technology based on the exploitation of the nano-bio-interactions between nanoparticles and blood samples. In this study, blood samples from 20 pancreatic cancer patients and 5 patients without malignancy were allowed to interact with designed lipid nanoparticles, leading to the formation of a hard “protein corona” at the nanoparticle surface. After isolation, the protein patterns were characterized by sodium dodecyl sulphate polyacrylamide gel electrophoresis (SDS PAGE). We found that the protein corona of pancreatic cancer patients was much more enriched than that of healthy individuals. Statistical analysis of SDS-PAGE results allowed us to discriminate between healthy and pancreatic cancer patients with a total discriminate correctness rate of 88%.
Introduction
Despite recent advances in molecular diagnostics, non-invasive screening tests for early stage tumor detection are almost nonexistent for most cancer types. Furthermore, no validated screening tools are available for several neoplasms. Among these, pancreatic cancer is a very lethal tumor with high mortality within a year after diagnosis and a median survival of less than six months.1 Five-year survival rates of 15–20% are reported when radical surgical resection is performed. Unfortunately, at the moment of diagnosis, surgery is feasible for only 20% of patients. The surgical approach is precluded for 80% of patients, due to the potential for local invasion of vascular structures, or for distant metastases. Avoiding delayed diagnosis is therefore crucial. However, the asymptomatic nature, absence of non-invasive imaging diagnostic tools and lack of any sensitive and specific biomarkers make the early diagnosis of pancreatic cancer challenging. Moreover, the complex biology of the tumor and its impenetrable architecture present a hard basis for the development of an effective treatment in the advanced stages to improve the outcomes.2,3 Pancreatic intraepithelial neoplasia (PanIN) lesions, several intraductal papillary mucinous neoplasms (IPMN) and mucinous cystic neoplasms (MCN) have been identified as pancreatic cancer precursors,4 but their diagnosis is often challenging for both radiologists and endoscopists. Moreover, chronic pancreatitis can lead to pancreatic neoplasms and cyto-histological analysis can be unsatisfying.5 To date, a non-biopsy-based-test detecting serum carbohydrate antigen 19-9 (CA19-9) is routinely used. However, this approach fails to be specific and is poorly sensitive. Therefore, it becomes a priority to develop more specific and sensitive diagnostic tests. In this field, Melo et al. recently detected and isolated pancreatic cancer-cell-derived exosomes circulating in blood, and through mass spectrometric analysis, identified a cell surface proteoglycan, glipican-1 (GPC1+), specifically enriched on cancer-cell-derived exosomes.6 GPC1+ exosomes were detected in the serum of pancreatic cancer patients, allowing healthy subjects and patients with benign pancreatic disease to be distinguished from those with early- and late-stage pancreatic cancer. More recently, Xie et al. identified two long non-coding RNAs (lncRNAs) in pancreatic cancer patients’ saliva. They found that salivary levels of HOTAIR and PVT1 lncRNAs were significantly higher in pancreatic cancer patients, compared to healthy subjects.7 Using quantitative PCR (qPCR), they demonstrated that both salivary lncRNAs discriminated pancreatic cancer patients from healthy controls and patients having benign pancreatic lesions, with sensibilities ranging from 60 to 97%. However, despite being promising, the above-mentioned methods depend on numerous and laborious steps (i.e., exosome isolation, identification of cell surface proteoglycan using mass spectroscopy or immunogold-TEM and qPCR). These methods are also extremely expensive and time consuming and are thus not appropriate for early cancer detection. As a consequence, the development of fast, cheap, user-friendly and non-invasive tools for the early diagnosis of pancreatic cancer is needed. Recent advances in nanotechnology have brought many innovative approaches and solutions to biomedical research. Due to the peculiar properties that arise when a material is reduced to the nanoscale (1–100 nm), nanoparticles (NPs) are being utilized in almost limitless applications.8 When NPs are dispersed in a biological fluid, they encounter an immediate and dramatic challenge to their “synthetic identity”,9 due to chemical–physical interactions between the NP surface and medium components. The result is the development of a new interface on the NPs surface, the so-called “bio-nano interface”, composed of biomolecules such as proteins, sugars and lipids. This biomolecular shell is dynamic by nature, and given the protein enrichment, it is usually referred to as the protein corona (PC).10,11 PC is made of two components, the ‘hard’ corona (HC) and the ‘soft’ corona (SC). HC is made of proteins strongly adsorbed to the bare NP surface, and is the interface “seen” and processed by living systems; thus, it influences various biological responses, such as pharmacological and toxicological effects,12–14 and cell targeting.15 Nowadays, it is well recognized that the formation and composition of HC depends on several factors:16–24 (i) surface properties of NPs (i.e. size, shape, curvature, surface chemistry and surface charge); (ii) characteristics of biological media, including protein concentration, protein source, and temperature; (iii) incubation time. The protein pattern in cancer patients’ blood differs from that of healthy donors; thus, the molecular composition of the HC formed around NPs could change between cancer and non-cancer patients blood. These alterations are often small and difficult to be detected by conventional blood analyses. On the other side, the HC can act as a “nano-concentrator”25 of those serum proteins with affinity for the NP surface. Characterization of HC could therefore allow detection of minor changes in protein concentration at the very early stages of disease development or even after chemotherapy or surgery, i.e., when alterations in the circulating levels of proteins are undetectable by blood tests. In this work, we explored the feasibility of developing a novel technology for pancreatic cancer detection, based on the recently reported differences in the HC formed on lipid NPs after exposure to pancreatic cancer and non-cancer blood serum samples26 (Fig. 1). To this end, we employed a liposomal formulation of a lipid composition made of HSPC (hydrogenated soy phosphatidylcholine), cholesterol (Chol) and DSPG (1,2-distearoyl-sn-glycero-3-[phospho-rac-(1-glycerol)]). This lipid formulation is the basis for the liposomal amphotericin B. Blood samples from 20 pancreatic cancer patients and 5 patients without malignancy (Table S1†) were allowed to interact with designed lipid nanoparticles leading to the formation of a HC at the nanoparticle surface. Notably, we found that the HCs varied between pancreatic cancer and healthy human blood. On the other hand, common blood tests did not allow discrimination between blood samples. Our results show that emerging HC-based technologies could open new horizons towards pancreatic cancer early diagnosis.
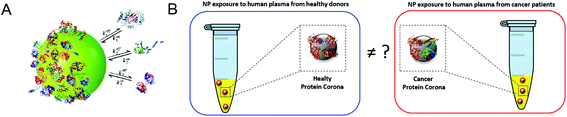 |
| Fig. 1 (A) When nanoparticles come into contact with physiological environments (e.g. blood, interstitial fluids etc.), they are immediately surrounded by proteins, forming a long-standing coating referred to as the protein corona. (B) Following exposure to blood samples, the protein corona works as a “nano-accumulator” and allows the detection of alterations in circulating proteins, such as those occurring in cancer. A key question towards bringing the protein corona from preclinical studies to the clinic is whether the protein coronas formed upon interaction with the blood of pancreatic cancer patients are the same as those from healthy volunteers. | |
Results and discussion
The lipid NPs were small in size (particle diameter ≈ 130 ± 5 nm) and negatively charged (surface charge ≈ −30 ± 3 mV). Following 1 hour of exposure to the human plasma (HP) of healthy volunteers, the HCs were detached from the NP surface and analyzed by 1D SDS/PAGE.27 We observed that (i) five major protein bands located at ≈110 kDa, 90 kDa, 75 kDa, 50 kDa and 25 kDa were common to various healthy cases (Fig. S1, panel A in the ESI†); (ii) the intensity of the same protein band from different healthy cases may vary. Collectively, these findings indicate that the HCs formed in the HP of healthy subjects are similar to each other. The observed changes in protein band intensities likely arise from plasma alterations occurring among healthy people, due to several factors such as genetic background, life style and geographical origin.17 It is known that circulating levels of proteins can be altered by clinical manifestations. However, no protein biomarker has so far been clinically validated to diagnose pancreatic cancer. Carcinoembryonic antigen (CEA) has been proposed as a potential biomarker for pancreatic cancer,28 but the protein is not produced by all pancreatic tumours and has lower sensitivity than CA19-9.29 On the other hand, the HC of NPs could work as a “nano-concentrator”15,25 of those serum proteins with affinity for the bare NP surface. Some of them could be simultaneously altered by cancer, not only in concentration, but also in their structure and function.30 Slight protein changes (i.e., not detectable by routine blood tests) could have a deep impact on both nanoparticle–protein and protein–protein interactions within HC. As a result of such changes, exposure of NPs to HP from pancreatic cancer patients could lead to modified HCs. The present exploration was therefore aimed at establishing whether the exposure of NPs to HP samples from healthy persons versus cancer patients may result in significant modifications of the HC. 1D SDS/PAGE was therefore used to analyse the protein patterns of the HC of pancreatic cancer patients. By visual inspection, the same five protein bands identified for healthy individuals (≈110 kDa, 90 kDa, 75 kDa, 50 kDa and 37 kDa) dominated the protein patterns of pancreatic cancer patients (Fig. S1,† panel B); the protein patterns of both healthy and pancreatic cancer patients were extremely rich. Thus, a densitometry analysis of electrophoretic gels was performed for semi-quantitative comparison. First, we calculated the intensity profile for each lane of the electrophoretic gels. Then, we averaged the intensity profiles of healthy individuals (Fig. 2, panel A) and those of pancreatic cancer patients (Fig. 2, panel B). As panels C and D of Fig. 2 clearly show, the average lane intensity profiles of healthy individuals and pancreatic cancer patients were appreciably different from each other. To properly evaluate changes in the HC, the intensity profiles of healthy individuals (Fig. 2, panel A) and those of pancreatic cancer patients (Fig. 2, panel B) were reduced to the following four regions of molecular weight (MW): (i) MW < 25 kDa; (ii) 25 < MW < 50; (iii) 50 < MW < 120; (iv) MW > 120 kDa (Fig. S2 in the ESI†). Subsequently, the integral area was calculated for each region. Lastly, PCA was performed using the four integral areas as variables. As Fig. 3A clearly shows, pancreatic cancer patients and healthy persons were strongly separated in the plane of the principal components (PC1, PC2). This result clearly demonstrates that the PCA performed using the intensities of the four selected protein bands is a powerful tool for discriminating between the two groups. To provide a quantitative estimation of group separation, LDA on PCs was accomplished. In Fig. 3B, we report the PC centroids of the two groups and the linear discriminant function that splits the PCs Cartesian planes in two prediction regions. Notably, statistical analysis of the intensity of the four major molecular weight regions found in the protein coronas’ patterns correctly identified 17 of 20 cancer patients and 5 of 5 healthy subjects. The test with 100% specificity and 85% sensitivity in distinguishing the healthy controls from cancer cases reached an overall correctness of 88% (Table S2†). To compare the prediction ability of the particle-enabled technology with that feasible by means of routine blood testing, PCA and LDA were replicated using the 24 hematological values as input parameters (Table S3†). As panels C and D of Fig. 3 clearly show, sample centroids were almost super-imposed in the PCs Cartesian planes. When the 24 hematological values were used as variables for the PCA, the test resulted in much lower specificity and sensitivity (20% and 60% respectively). Likewise, total correctness decreased to 52% (Table S2†). Our findings imply that statistical analysis applied to the results of blood tests does not allow us to accurately distinguish cancer from non-cancer patients. We underline that the conclusions of our study are far from general, due to the small number of samples; however, recent studies have employed similar numbers of patients to investigate the potential effectiveness of PC biomarkers.31 Moving from descriptive to predictive modelling will require a larger series of experiments; however, our findings represent the proof of concept that the protein corona technology is a promising tool for the early diagnosis of pathological conditions where alterations of circulating proteins occur.
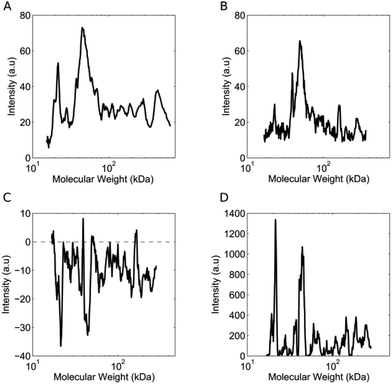 |
| Fig. 2 (A) Average lane intensity profile of healthy individuals as a function of molecular weight. The profile is the average of 10 independent intensity profiles (2 for each of the 5 healthy subjects). (B) Average lane intensity profile of pancreatic cancer patients. The profile is the average of 40 independent profiles (2 profiles for each of the 20 pancreatic cancer patients). (C) Difference between the intensity profiles shown in panels A and B. (D) Square of the intensity profile reported in panel C. | |
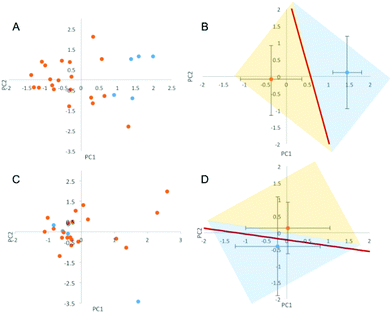 |
| Fig. 3 (A) Principal components (PC1, PC2) calculated from the four integral areas of the total lane intensity profiles calculated in the following four regions of molecular weight: (i) MW < 25 kDa; (ii) 25 < MW < 50; (iii) 50 < MW < 120; (iv) MW > 120 kDa. Blue and orange circles refer to the pancreatic cancer and healthy patients, respectively. (B) Centroids of the distributions of panel A. The straight line separates the healthy volunteers group from the pancreatic cancer patients group, according to the results of the linear discriminant analysis (LDA). (C) Principal components (PC1, PC2) calculated using the set of 24 hematological values reported in Table S3† as input parameters. (D) Centroids of the two distributions reported in panel C are almost superimposed. | |
Implications could be extremely relevant in pancreatic cancer, wherein early diagnosis is the only change in access to treatment with curative intent. In the future, nanotechnological solutions based on the exploitation of the protein corona will open new horizons for clinical diagnosis.
Materials and methods
Demographic characteristics and clinical laboratory tests
Blood samples from 20 consecutive, histologically proven pancreatic adenocarcinoma (median age 72 years) and from a group of 5 patients (median age 66 years) affected by surgical benign diseases that met the criteria reported in Table S1,† were collected. In the present manuscript, the last group of patients is considered “healthy”. “Healthy” means that they were not affected by malignancies and represent the control group. Data regarding medical history and clinical-instrumental work-up have been assessed for each patient; particularly, total protein, serum albumin and serum protein electrophoresis have been considered in both groups. Total protein and albumin were dosed on a Dimension Vista (Siemens) system. Obtained values are expressed in g dL−1 for both albumin and total protein. Electrophoresis was performed on a Capillarys Sebia electrophoresis system for half an hour (zonal electrophoresis in liquid phase); it was a semi-quantitative analysis and values of various protein fractions, α1 (e.g., anti-trypsin), α2 globulin (e.g., α2-macroglobulin, aptoglobine, ceruloplasmine), β1 globulin (e.g., transferrin), β2 globulin (e.g. β2-microglobulin), γ globulin (e.g., immunoglobulin), are expressed as percentage intervals. For patients in the pancreatic cancer group, CEA and ca. 19.9 were also evaluated. Blood collection and plasma preparation and storage were performed according to methods already described.32 According to their clinical stages, pancreatic cancer patients underwent surgery, neoadjuvant chemo-radiotherapy or chemotherapy. For surgically resected patients, pathological staging data were collected. Clinical and pathological stages are reported according to the TNM staging system.33 The Ethical Committee of University “Campus Bio-Medico di Roma” approved the present study. Tables S4–S6† show the demographic and clinical characteristics of our series.
Liposome preparation
Hydrogenated soy phosphatidylcholine (HSPC) and 1,2-distearoyl-sn-glycero-3-[phospho-rac-(1-glycerol)] (sodium salt) (DSPG) were purchased from Avanti Polar Lipids (Alabaster, AL) Cholesterol (Chol) was purchased from Sigma-Aldrich (Milan, Italy). Ambisome-like liposomes were synthesized according to standard protocols at the molar ratio of HSPC
:
DSPG
:
Chol (2.5
:
1
:
1.2).34 Lipid films were hydrated with phosphate saline buffer (PBS) 10 mmol L−1 (pH = 7.4) to a final lipid concentration 1 mg mL−1. The obtained liposome suspension was extruded 20 times through a 0.05 μm polycarbonate carbonate filter with the Avanti Mini-Extruder (Avanti Polar Lipids, Alabaster, AL). Measurements of size and surface charge were performed at room temperature on a Zetasizer Nano ZS90 (Malvern, UK) equipped with a 5 mW HeNe laser (wavelength λ = 632.8 nm) and a digital logarithmic correlator. More detailed information can be found elsewhere.35
Liposome–protein complexes
Liposome–protein complexes were prepared by incubating liposomes with HP (1
:
1 v/v) at 37 °C for 1 hour. Experimental conditions (i.e., plasma concentration, temperature and incubation time) were chosen according to previous investigations.35–38 After incubation, liposome–protein complexes were isolated by centrifugation for 15 min at 14
000 rpm. The pellets were then washed three times with PBS to remove unbound proteins, obtaining the “hard corona”.
1D-SDS-PAGE experiments
The HC-coated NPs were re-suspended in 20 μL of Laemmli loading buffer 1× (10 μL βME/1 mL Laemmli loading buffer) and boiled for 5 min at 100 °C. Identical volumes (10 μL) of each sample were loaded on a gradient polyacrylamide gel (4–20% Criterion TGX precast gels, Bio-Rad), and run at 100 V for about 150 minutes. Gels were washed in double-distilled water (ddH2O) and fixed over night for 12 hours in a de-staining solution (MeOH 50% in ddH2O), with gentle agitation at room temperature, with at least one solution change. In order to determine differences in corona composition, we stained the proteins with highly sensitive silver-ammonia solution for 30 minutes. The gels were rinsed 3 times in ddH2O, 40 seconds for each rinse, then incubated with acidic developer solution for 3 to 5 minutes. Once all the protein bands started to clearly appear, developer solution was removed and stop solution (acetic acid 10%, methanol 45% in ddH2O) was added. Pictures of gels were captured by KODAK Digital DC120. Densitometry analysis of gels was performed using ImageJ software (NIH Image; http://rsbweb. nih.gov/ij/).
Statistical analysis
Principal component analysis (PCA) is a classical, well-established method, frequently used in the physical, as well as social and biological sciences.39 Its aim is to project a multidimensional phenomenon onto a reduced set of orthogonal axes (principal component scores or eigenfunctions) with minimal loss of information.40 The new coordinates are linear combinations of the original ones: they are orthogonal by construction, each representing an independent aspect of the data set. Moreover, the principal components are endowed with the property of explaining the system variability in a hierarchical way; this implies that we can save the meaningful (signal-like) part of the information retained by the first principal components and discard the noise, which is supposed to concentrate on the minor components. The set of original variables obtained under each explored condition is thus linearly transformed by the PCA into a new set of variables (called PC1, PC2, etc.), endowed with the property of being orthogonal to each other, and ordered by the decreasing amount of explained variance (information) in the data. We took extensive advantage of that property to include within some of principal components a major fraction of the information present in the hematological values describing the patients’ conditions. By means of this analytical technique, we compared two different, even if correlated, datasets. One dataset was composed by 24 different hematological parameters. The second dataset was composed of 4 variables, i.e., the integral areas of the average lane intensity profiles calculated for (i) MW < 25 kDa; (ii) 25 < MW < 50; (iii) 50 < MW < 120; (iv) MW > 120 kDa. Thus, we clustered and classified the patients by means of Linear Discriminant Analysis (LDA), performed on the principal components.41 LDA is the method of election to determine whether meaningful differences exist between the groups and to identify the discriminating power of each variable.41 The technique is constructed of a set of linear functions of predictors, known as discriminant functions, such that L = b1x1 + b2x2 + … + bnxn + c, where the bi are discriminant coefficients the xi are the input variables or predictors, and c is a constant. L is a class index that usually takes the values of 1 and −1, respectively, for the two classes to be discriminate, so that a positive value of L points to class A and a negative value to class B. We performed LDA on the two principal components of the two considered datasets.
Conclusions
In this work, we have investigated the feasibility of developing a new pancreatic cancer diagnostic technology based on the exploitation of the nano-bio-interactions between nanoparticles and blood samples. This work was not intended as a claim of biomarker effectiveness for the detection of pancreatic cancer. Extensive clinical investigations are necessary to validate the clinical applications of the new protein corona-based test, and rigorously evaluate the factors that may influence its specificity and sensitivity. This would further validate the potential of protein corona for early detection of pancreatic cancer, thus leading to novel opportunities in terms of treatment and outcomes.
Acknowledgements
The Italian Minister of Health (“Progetto Giovani Ricercatori 2011–2012”, Grant No. GR-2011-02350094) is gratefully acknowledged.
Notes and references
- R. L. Siegel, K. D. Miller and A. Jemal, CA-Cancer J. Clin., 2015, 65, 5–29 CrossRef PubMed.
- P. Ghaneh, E. Costello and J. P. Neoptolemos, Gut, 2007, 56, 1134–1152 CrossRef CAS PubMed.
- J. Werner, S. E. Combs, C. Springfeld, W. Hartwig, T. Hackert and M. W. Büchler, Nat. Rev. Clin. Oncol., 2013, 10, 323–333 CrossRef CAS PubMed.
- L. W. Traverso, E. A. Peralta, J. A. Ryan and R. A. Kozarek, Am. J. Surg., 1998, 175, 426–432 CrossRef CAS PubMed.
- M. I. Canto, F. Harinck, R. H. Hruban, G. J. Offerhaus, J.-W. Poley, I. Kamel, Y. Nio, R. S. Schulick, C. Bassi and I. Kluijt, Gut, 2013, 62, 339–347 CrossRef PubMed.
- S. A. Melo, L. B. Luecke, C. Kahlert, A. F. Fernandez, S. T. Gammon, J. Kaye, V. S. LeBleu, E. A. Mittendorf, J. Weitz and N. Rahbari, Nature, 2015, 523, 177–182 CrossRef CAS PubMed.
- Z. Xie, X. Chen, J. Li, Y. Guo, H. Li, X. Pan, J. Jiang, H. Liu and B. Wu, Oncotarget, 2016, 7, 25408–25419 Search PubMed.
- J. T. Cole and N. B. Holland, Drug Delivery Transl. Res., 2015, 5, 295–309 CrossRef CAS PubMed.
- C. D. Walkey and W. C. W. Chan, Chem. Soc. Rev., 2012, 41, 2780–2799 RSC.
- M. P. Monopoli, C. Åberg, A. Salvati and K. A. Dawson, Nat. Nanotechnol., 2012, 7, 779–786 CrossRef CAS PubMed.
- G. Caracciolo, Nanomedicine, 2015, 11, 543–557 CAS.
- A. Bigdeli, S. Palchetti, D. Pozzi, M. R. Hormozi-Nezhad, F. Baldelli Bombelli, G. Caracciolo and M. Mahmoudi, ACS Nano, 2016, 10, 3723–3737 CrossRef CAS PubMed.
- E. Valsami-Jones and I. Lynch, Science, 2015, 350, 388–389 CrossRef CAS PubMed.
- G. Ciasca, M. Papi, M. Chiarpotto, M. Rodio, G. Campi, C. Rossi, P. De Sole and A. Bianconi, Appl. Phys. Lett., 2012, 100, 073703 CrossRef.
- G. Caracciolo, F. Cardarelli, D. Pozzi, F. Salomone, G. Maccari, G. Bardi, A. L. Capriotti, C. Cavaliere, M. Papi and A. Laganà, ACS Appl. Mater. Interfaces, 2013, 5, 13171–13179 CAS.
- M. Mahmoudi, S. N. Saeedi-Eslami, M. A. Shokrgozar, K. Azadmanesh, M. Hassanlou, H. R. Kalhor, C. Burtea, B. Rothen-Rutishauser, S. Laurent and S. Sheibani, Nanoscale, 2012, 4, 5461–5468 RSC.
- M. J. Hajipour, S. Laurent, A. Aghaie, F. Rezaee and M. Mahmoudi, Biomater. Sci., 2014, 2, 1210–1221 RSC.
- D. Pozzi, V. Colapicchioni, G. Caracciolo, S. Piovesana, A. L. Capriotti, S. Palchetti, S. De Grossi, A. Riccioli, H. Amenitsch and A. Lagana, Nanoscale, 2014, 6, 2782–2792 RSC.
- G. Caracciolo, D. Pozzi, A. L. Capriotti, C. Cavaliere, S. Piovesana, H. Amenitsch and A. Laganà, RSC Adv., 2015, 5, 5967–5975 RSC.
- M. J. Hajipour, J. Raheb, O. Akhavan, S. Arjmand, O. Mashinchian, M. Rahman, M. Abdolahad, V. Serpooshan, S. Laurent and M. Mahmoudi, Nanoscale, 2015, 7, 8978–8994 RSC.
- V. Mirshafiee, R. Kim, M. Mahmoudi and M. L. Kraft, Int. J. Biochem. Cell Biol., 2015, 000 Search PubMed.
- A. Bigdeli, S. Palchetti, D. Pozzi, M. R. Hormozi-Nezhad, F. Baldelli Bombelli, G. Caracciolo and M. Mahmoudi, ACS Nano, 2016, 10, 3723–3737 CrossRef CAS PubMed.
- C. Corbo, R. Molinaro, A. Parodi, N. E. Toledano Furman, F. Salvatore and E. Tasciotti, Nanomedicine, 2016, 11, 81–100 CrossRef CAS PubMed.
- S. Palchetti, V. Colapicchioni, L. Digiacomo, G. Caracciolo, D. Pozzi, A. L. Capriotti, G. La Barbera and A. Laganà, Biochim. Biophys. Acta, Biomembr., 2016, 1858, 189–196 CrossRef CAS PubMed.
- T. Zheng, N. Pierre-Pierre, X. Yan, Q. Huo, A. J. Almodovar, F. Valerio, I. Rivera-Ramirez, E. Griffith, D. D. Decker and S. Chen, ACS Appl. Mater. Interfaces, 2015, 7, 6819–6827 CAS.
- V. Colapicchioni, M. Tilio, L. Digiacomo, V. Gambini, S. Palchetti, C. Marchini, D. Pozzi, S. Occhipinti, A. Amici and G. Caracciolo, Int. J. Biochem. Cell Biol., 2016, 75, 180–187 CrossRef CAS PubMed.
- P. Del Pino, B. Pelaz, Q. Zhang, P. Maffre, G. U. Nienhaus and W. J. Parak, Mater. Horiz., 2014, 1, 301–313 RSC.
- H. Imaoka, N. Mizuno, K. Hara, S. Hijioka, M. Tajika, T. Tanaka, M. Ishihara, Y. Hirayama, N. Hieda and T. Yoshida, Pancreatology, 2016, 16, 859–864 CrossRef CAS PubMed.
- J. R. Bergquist, C. A. Puig, C. R. Shubert, R. T. Groeschl, E. B. Habermann, M. L. Kendrick, D. M. Nagorney, R. L. Smoot, M. B. Farnell and M. J. Truty, J. Am. Coll. Surg., 2016, 23, 52–65 CrossRef PubMed.
- Z. J. Deng, M. Liang, M. Monteiro, I. Toth and R. F. Minchin, Nat. Nanotechnol., 2011, 6, 39–44 CrossRef CAS PubMed.
- S. Nie, A. Lo, J. Wu, J. Zhu, Z. Tan, D. M. Simeone, M. A. Anderson, K. A. Shedden, M. T. Ruffin and D. M. Lubman, J. Proteome Res., 2014, 13, 1873–1884 CrossRef CAS PubMed.
- G. Caracciolo, D. Caputo, D. Pozzi, V. Colapicchioni and R. Coppola, Colloids Surf., B, 2014, 123, 673–678 CrossRef CAS PubMed.
- J. R. Egner, J. Am. Med. Assoc., 2010, 304, 1726–1727 CrossRef CAS.
- J. Adler-Moore and R. T. Proffitt, J. Antimicrob. Chemother., 2002, 49, 21–30 CrossRef CAS PubMed.
- A. L. Barrán-Berdón, D. Pozzi, G. Caracciolo, A. L. Capriotti, G. Caruso, C. Cavaliere, A. Riccioli, S. Palchetti and A. Laganaì, Langmuir, 2013, 29, 6485–6494 CrossRef PubMed.
- G. Caracciolo, D. Pozzi, A. L. Capriotti, C. Cavaliere, P. Foglia, H. Amenitsch and A. Laganà, Langmuir, 2011, 27, 15048–15053 CrossRef CAS PubMed.
- M. Mahmoudi, A. M. Abdelmonem, S. Behzadi, J. H. Clement, S. Dutz, M. R. Ejtehadi, R. Hartmann, K. Kantner, U. Linne and P. Maffre, ACS Nano, 2013, 7, 6555–6562 CrossRef CAS PubMed.
- S. Tenzer, D. Docter, J. Kuharev, A. Musyanovych, V. Fetz, R. Hecht, F. Schlenk, D. Fischer, K. Kiouptsi and C. Reinhardt, Nat. Nanotechnol., 2013, 8, 772–781 CrossRef CAS PubMed.
- H. Abdi and L. J. Williams, Wiley Interdiscip. Rev.: Comput. Stat., 2010, 2, 433–459 CrossRef.
- M. Colafranceschi, M. Papi, A. Giuliani, G. Amiconi and A. Colosimo, Pathophysiol. Haemostasis Thromb., 2006, 35, 417–427 CrossRef CAS PubMed.
- T. Jombart, S. Devillard and F. Balloux, BMC Genet., 2010, 11, 1–15 CrossRef PubMed.
Footnotes |
† Electronic supplementary information (ESI) available: Fig. S1: Representative one-dimensional SDS-PAGE gel of plasma proteins obtained from lipid NPs following in vitro incubation with plasma of healthy subjects and pancreatic cancer patients. Fig. S2: Procedure followed to determine the variables for the protein component analysis. Table S1: Inclusion criteria in healthy and pancreatic cancer groups. Table S2: Nanoparticle versus blood test results. Table S3: Measured concentrations of clinically relevant proteins in histologically proven pancreatic cancer patients and healthy patients. Tables S4–S6: Demographic and clinical characteristics of pancreatic cancer patients and healthy patients. See DOI: 10.1039/c6nr05609a |
‡ Equal contribution. |
|
This journal is © The Royal Society of Chemistry 2017 |
Click here to see how this site uses Cookies. View our privacy policy here.