DOI:
10.1039/C6LC01302C
(Paper)
Lab Chip, 2017,
17, 830-841
A nano flow cytometer for single lipid vesicle analysis†
Received
20th October 2016
, Accepted 18th January 2017
First published on 19th January 2017
Abstract
We present a nanofluidic device for fluorescence-based detection and characterization of small lipid vesicles on a single particle basis. The device works like a nano flow cytometer where individual vesicles are visualized by fluorescence microscopy while passing through parallel nanochannels in a pressure-driven flow. An experiment requires less than 20 μl sample volume to quantify both the vesicle content and the fluorescence signals emitted by individual vesicles. We show that the device can be used to accurately count the number of fluorescent synthetic lipid vesicles down to a vesicle concentration of 170 fM. We also show that the size-distribution of the vesicles can be resolved from their fluorescence intensity distribution after calibration. We demonstrate the applicability of the assay in two different examples. In the first, we use the nanofluidic device to determine the particle concentration in a sample containing cell-derived extracellular vesicles labelled with a lipophilic dye. In the second, we demonstrate that dual-color detection can be used to probe peptide binding to synthetic lipid vesicles; we identify a positive membrane-curvature sensing behavior of an arginine enriched version of the Antennapedia homeodomain peptide penetratin. Altogether, these results illustrate the potential of this nanofluidic-based methodology for characterization and quantification of small biological vesicles and their interactors without ensemble averaging. The device is therefore likely to find use as a quantitative analytical tool in a variety of fields ranging from diagnostics to fundamental biology research. Moreover, our results have potential to facilitate further development of automated lab-on-a-chip devices for vesicle analysis.
1. Introduction
Living organisms contain a multitude of phospholipid-based vesicles with distinct biochemical, structural and physical properties that allow them to fulfill well-defined biological functions. Cell-secreted extracellular vesicles represent a category of such vesicles and have attracted considerable attention recently for their emerging significance in intercellular communication. In particular exosomes, extracellular vesicles of endocytic origin,1,2 have been identified as key players for example in the regulation of various biological processes.3–8 It has also been suggested that extracellular vesicles, including exosomes, are important in the pathobiology of cancer9,10 and neurodegenerative diseases;10,11 these vesicles have therefore been pointed out as promising diagnostic markers.12 Furthermore, artificial lipid vesicles, also called liposomes, are useful membrane mimics for fundamental studies of biomolecular interactions at the membrane interface13–17 or as drug delivery vehicles.18–20
In light of the high intrinsic prevalence and increasing significance of phospholipid-based vesicles in biological systems and of the pharmaceutical potential of liposome formulations, there is an urgent need for development of new and accurate methods capable of analyzing lipid-vesicle-containing samples with respect to size, structure, composition and content. Characterization of small biological vesicles has, however, proven highly challenging, mainly due to their small size (typically a few tens to a few hundreds of nanometers),21 but also due to the enormous degree of heterogeneity that is characteristic for native vesicle samples.1,22–26 Body fluids, for example, contain a broad variety of secreted vesicles which differ in size, biomolecular finger prints and lipid contents, reflecting the multitude of possible cellular origins and biogenesis mechanisms.1,25 In view of this heterogeneity, it has not only become increasingly essential to determine the biomolecular identity of nanovesicles one by one, it has also become important to accurately quantify vesicle numbers and to discriminate between vesicle fractions belonging to different subpopulations. In the context of exosome research, such analytic capability would, for example, make it possible to identify and quantify vesicles carrying a specific disease marker or to determine the tissue of origin of the different vesicles found in samples collected from humans or model organisms, allowing for better understanding of biological functions and disease.
Electron microscopy (EM) has historically been a preferred method to visualize single lipid vesicles, as it allows imaging with nanometer resolution.27–29 Moreover, the possibility to combine EM with immuno-gold staining has made it possible to detect specific molecules on the vesicle surface and thereby to identify vesicle subpopulations.30,31 While this technique can provide detailed information on vesicle morphology and identity, its ability to accurately determine vesicle size and subpopulation fractions is limited by low throughput, artifacts due to sample preparation and limited possibilities of multiplexing. The advent of techniques that are capable of detecting fluorescence and scattering signals from individual biological vesicles promises to increase throughput in the characterization of vesicle size and numbers, but also to facilitate their multiplexed biochemical profiling on a single vesicle level using fluorescent reporter probes and immunostaining. In this context, nanoparticle tracking analysis (NTA), which allows for the visualization of the light scattered or fluorescence emitted by individual particles upon laser illumination,32 has gained in popularity over the last years. With this method, vesicle numbers can be quantified and the size distribution in samples can be estimated upon tracking of their Brownian motion.24,33 Furthermore, fluorescence-based detection makes it possible to characterize fluorescently labelled vesicle subpopulations.33 However, an accurate quantification of the intensity of the emitted signal is challenging due to the three-dimensional motion of the particle across the focal plane.34 Furthermore, multiparametric detection of selected individual vesicles is yet to be achieved with commercial instruments although possible, in principle.
Multiparametric detection of the fluorescence and scattering signals generated from individual vesicles can be achieved upon immobilization on a surface and using fluorescence microscopy or label-free microscopy setups.35–38 Surface-based fluorescence detection has been used, for example, to probe protein binding to membranes,14 to investigate heterogeneities in vesicle lipid composition39 and to probe membrane degradation on a single vesicle level.17,40 While surface-based detection allows for a sensitive analysis of vesicle populations,41 surface-immobilization has the drawback of often requiring optimized surface chemistries and several sample preparation steps which is time consuming and can damage the sample. Furthermore, sample recovery for further analysis, in particular in conjunction with sorting of vesicle subpopulations, is cumbersome. An alternative and faster method that overcomes the latter limitation is flow cytometry which allows for solution-based high-throughput multiparametric detection of particles. The method is mostly used to analyse cells or microparticles but has also been adapted for lipid vesicles.42 It is, however, only recently that a high enough sensitivity could be achieved to detect viruses or extracellular vesicles with diameters below 100 nm.43–46 Flow cytometry in combination with fluorescence-activated sorting makes it possible to recover subpopulations of analyzed particles.46 Conventional flow cytometers however operate with quite large sample volumes47 which has prompted the development of chip-based flow cytometers47–50 where the scaling down of the dimensions of the channels to a few hundred nanometers makes it possible to analyze sub-micrometer vesicles,51 viruses52 or even individual biomolecules.53
In this study, we implement and validate a nanofluidic device for single vesicle analysis of biological samples that functions as a miniturized nano flow cytometer. The device consists of hundreds of parallel nanochannels in which lipid vesicles can be introduced under a steady flow by means of pressure. The device, which has previously mostly been used in a stationary mode to study DNA and DNA–protein complexes,54,55 can be imaged under a standard epi-fluorescence microscope, offering cheap and easily implemented means of measurement and detection. Our nanofluidic-based assay allows solution-based quantification of the fluorescence signal of individual vesicles with sizes in the 100 nm range and for quantification of their concentration down to femtomolars. Two potential applications are presented; in the first we quantify the concentration of extracellular vesicles isolated from cell culture media and in the second we show that a short drug delivery peptide with cell-penetrating properties binds to a membrane with a hitherto unreported curvature-dependent affinity. Taken together, our device is fast, easy to operate, compatible with standard epi-configuration microscopy and requires very low amounts of starting material; this makes it a promising tool in the study of extracellular vesicle subpopulations and other types of heterogeneous artificial or biological nanoparticles.
2. Experimental
2.1. Nanofluidic device fabrication
The design of the nanofluidic device is shown in Fig. 1. In brief, it consists of a series of 250 parallel nanochannel with a period of 2 μm. Each individual channel has a square profile with 300 nm sides. The nanochannels are connected by two microchannels facilitating device loading. The nanofluidic devices were fabricated using standard micro- and nanofabrication techniques as described in detail elsewhere.55 In brief, the fabrication comprised five processing steps based on optical lithography (OL, system MA 6/Suss MicroTec), electron beam lithography (EBL, system JBX-9300FS/JEOL Ltd), reactive ion etching (RIE, system Plasmalab 100 ICP180/Oxford Plasma Technology), deep reactive ion etching (DRIE, system STS ICP/STS), fusion bonding (system AWF 12/65/Lenton) and dicing (system DAD3350/Disco). Alignment marks for both OL and EBL were etched into the surface of a p-type 4′′-Si wafer with 2 μm thermal oxide using OL and fluorine based RIE. Subsequently, arrays of nanochannels were defined in a resist layer with EBL and transferred into the thermal oxide with fluorine based RIE. After that, microfluidic structures connecting to the nanochannels were fabricated with OL and fluorine based RIE, before using OL and DRIE to etch holes through the substrates, serving as inlets and reservoirs. The substrate was then fusion bonded to a 200 μm thick 4′′-pyrex wafer in Ar atmosphere for 5 h at 550 °C and diced into individual chips.
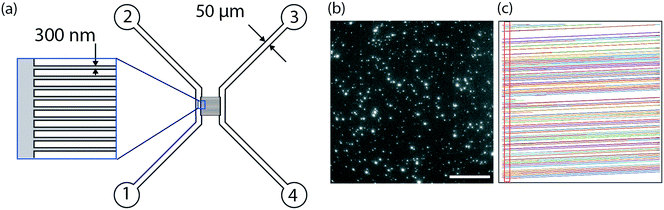 |
| Fig. 1 Nanofluidic device. (a) Schematic representation of the nanofluidic device consisting of parallel nanochannels with a square profile of 300 nm connected by two microchannels. The microchannels are used for sample injection; the vesicles are observed by recording time lapse movies while the sample is passing through the nanochannels. (b) Snap shot (single image frame) of individual vesicles containing 0.6 mol% ATTO 550 imaged in the nanofluidic device using an epi-fluorescence microscope. Scale bar: 50 μm. (c) Detected movement of individual vesicles in the nanofluidic channels. For vesicle quantification, the vesicles were counted when passing the gate displayed as a red rectangle. | |
2.2. Lipid vesicle preparation
The lipids 1-palmitoyl-2-oleoyl-sn-glycero-3-phosphocholine (POPC), 1,2-dioleoyl-sn-glycero-3-phosphocholine (DOPC), 1,2-dioleoyl-sn-glycero-3-phospho-(1′-rac-glycerol) (DOPG), 1,2-distearoyl-sn-glycero-3-phosphoethanolamine-N-[amino(polyethylene glycol)-2000] (ammonium salt) (DSPE-PEG2000) and the fluorescently labelled lipid 1-palmitoyl-2-{6-[(7-nitro-2-1,3-benzoxadiazol-4-yl)amino]hexanoyl}-sn-glycero-3-phosphocholine (NBD-PC) were purchased from Avanti Polar Lipids Inc. (Alabaster, USA). Fluorescently labelled ATTO 550-DOPE was purchased from Atto-Tec GmbH (Siegen, Germany). Fluorescent vesicles for channel passivation were made of POPC containing 1 wt% NBD-PC. Vesicles for the peptide binding assay were prepared from a mixture of DOPC/DOPG/DSPE-PEG2000/ATTO 550-DOPE at a molar ratio of 60
:
37
:
2.5
:
0.5 where DSPE-PEG2000 lipids were included to avoid peptide-induced lipid vesicle aggregation.56 All other vesicles were prepared from POPC doped with small amounts of ATTO 550 (between 0.02 mol% and 2.2 mol%). All vesicles were prepared by the lipid film hydration and extrusion method.57 In brief, lipids dissolved in chloroform were mixed in a round bottom flask followed by solvent evaporation under a gentle nitrogen stream whilst constantly rotating the flask. The dried film was then left in high vacuum for at least 2 h to remove any residual solvent. The resulting dry lipid film was hydrated using the indicated buffer solution and extruded 11× in an Avanti Mini Extruder (Avanti Polar Lipids Inc., Alabaster, USA) at a pressure of 10 psi. Vesicles for channel passivation58 were extruded through a 30 nm pore membrane. All other vesicles were prepared using a 100 nm pore membrane and yielded vesicle populations with a mean diameter of around 150 nm. In all cases, except for the vesicles used in the peptide binding assay, the dry lipid film was hydrated in phosphate buffered saline (PBS; 0.01 M phosphate, 0.0027 M potassium chloride and 0.137 M sodium chloride, PH 7.4, Sigma Aldrich, Stockholm, Sweden). The vesicles used in the peptide binding assay were produced in 10 mM HEPES, 5 mM NaOH, 1 mM EDTA, 0.1 M NaCl, pH 7.4.
The theoretical average number of lipids (N) per vesicle was calculated from simple geometrical considerations assuming that the vesicles were monodisperse and unilamellar. The mode diameter, i.e. the value that appears most often in the distribution, measured by nanoparticle tracking analysis (NTA), was used as an average vesicle size (d). An average lipid surface area (A) of 0.65 nm2 (ref. 59) was assumed for all lipids regardless of whether they were in the inner or outer leaflet. The number of lipids was therefore estimated as:
2.3. Nanofluidic device measurements
Prior to each measurement, the surface of the nanochannels in the fluidic device was passivated against non-specific vesicle and peptide binding by introducing a POPC supported lipid bilayer formed by surface-induced vesicle rupture.58 This passivation was achieved by loading 20 μl of a vesicle suspension (0.5 mg ml−1 lipids) into inlet 1. The vesicle solution was then flushed to the inlets of the nanochannels by applying pressure to inlet 1 and subsequently into the nanochannels by applying a pressure of 0.5 bar to inlets 1 and 2 (see Fig. 1a) for at least 30 min. In all experiments, except for those performed with carboxyfluorescein-labelled peptides, successful formation of a supported lipid bilayer in the channel was visually verified by fluorescence microscopy using vesicle containing 1 wt% NBD-PC. In the experiments with carboxyfluorescein-labelled peptides, non-fluorescent POPC vesicles were used for channel passivation. Uniform light intensities along typically >95% of the nanochannels for a successful experiment, indicated even spreading of the passivating bilayer and that the majority of channels were open (ESI† S1).
After passivation, the bilayer was rinsed with buffer followed by deposition of a 20 μl sample droplet in inlet 1 of the nanofluidic device. Pressure was applied to drive the sample into the microchannel; followed by subsequent and uniform application of pressure on inlet 1 and 2 which drives the sample into the nanochannels. If not stated otherwise, the applied pressure across the nanochannels was 25 mbar and the total recording time was 2 minutes.
2.4. Fluorescence microscopy
All nanofluidic device measurements were carried out with the device mounted in a custom made sample holder that in turn was mounted on a Nikon Eclipse Ti-E inverted microscope using a 60× magnification (NA = 1.49) oil immersion objective (Nikon Corporation, Tokyo, Japan). The microscope was equipped with a mercury lamp (Intensilight C-HGFIE; Nikon Corporation) for excitation, a TRITC and FITC filter cube (Nikon Corporation) and an Andor iXon+ EMCCD camera (Andor Technology Ltd, Belfast, Northern Ireland) for detection. A beam splitter (Optosplit II, Andor technology Ltd) equipped with a dual bandpass filter (bandpass centers at 468/533 nm), a dual bandpass dichroic mirror (bandpass centers at 493/574 nm), a dichroic mirror (cutoff at 562 nm) and two emission filters (green: 514/30 nm; red: 617/73 nm) was used for simultaneous two-channel fluorescence detection.
2.5. Vesicle tracking
The collected image series (time-lapse movies) were processed and analyzed with MatLab (MathWorks, Inc., Natick, USA) using in-house scripts. Vesicles were detected using an intensity threshold chosen individually for each time-lapse movie. The fluorescence cutoff threshold was chosen after visual inspection in order to detect a maximum number of vesicles while excluding the background noise. Single particle tracking was implemented using local nearest-neighbor linking60 and events whose displacements were below a set maximal distance were connected across frames. The maximal distance was determined for each time-lapse movie individually; the parameters were refined by visual inspection of the tracking result. Additionally, the maximum allowed movement angle was set to a fixed value of 15° to avoid artifactual crossing of the track into neighboring channels. An extrapolating tracking function was further used to facilitate the tracking of vesicles which due to their low signal-to-noise ratio were found to disappear and reappear in consecutive frames. Specifically, a vesicle was only taken into account if it could be tracked for at least 4 consecutive frames. If a vesicle disappeared, a theoretical event was placed at the expected extrapolated position. A maximum of 4 extrapolated positions was tolerated before the vesicle was considered lost. These additions to the particle tracking algorithm, unique for vesicles flowing in a one-dimensional channel, significantly improved the quality of the tracking algorithm as further illustrated in the ESI† (S2). All parameters (i.e. maximum allowed travel distance, minimum allowed distance between two vesicles and set background level) were optimized by visual inspection of the tracking result. For each frame, each detected vesicle was located with sub-pixel accuracy by applying a Gaussian fit to its intensity distribution.
For two-color fluorescence detection, the images corresponding to the individual fluorescence channel on the microscope were first evaluated individually as described above. The images for both channels were then cropped to the same dimension and accurately overlaid using the convolution theorem of the Fourier transform61 yielding the relative displacement of the movies in the individual fluorescence channels and allowing for a connection between them.
2.6. Vesicle quantification
The vesicle concentration in a sample was determined by counting the number of vesicles passing through the nanochannels of the device during the time period of the experiment. The total sample volume (analysed within the time period) was determined from the sample flow rate, the nanochannel area, the number of channels in the image and the experiment time. For vesicle counting, a detection rectangle was defined with a width set to the maximum travel distance (section 2.3). To minimize artifacts due to possible bleaching, the rectangle was placed where the vesicles enter the nanochannels (see Fig. 1c). The sample flow rate was estimated as the average mean displacement of all vesicles in the measurement. It was therefore assumed that the recorded vesicle speed reflects the fluid velocity and therefore that the friction acting on the vesicles is negligible both for large and small vesicles. This assumption is supported by the observation that the vesicle speed is independent of the vesicle intensity, which relates to its size (see ESI† S3). The number of open channels in the field of view was obtained from recorded images that showed all detected tracks. Some channels (typically <5%) were clogged, most likely due to defects during fabrication or unsuccessful passivation, and were excluded from the analysis. To ensure good statistics, we made sure that at least 300 events were recorded in the field of view (containing ∼100 nanochannels), leading to a 95% probability that a vesicle has passed through an open channel. In this case, the underestimation of the number of open channels is therefore below 5%. To gain a rough estimate of the number of vesicles found in the microchannels (control experiment shown in ESI† S4), the number of vesicles detectable on at least 5 individual images were counted. The total volume was taken as the area of an image multiplied by the thickness of the microchannel (1 μm). The thickness was smaller than the imaging depth.
2.7. Vesicle fluorescence intensity analysis
In order to determine the fluorescence intensity of individual vesicles flowing through the nanochannels of the device, the pressure was set to the minimum value that allows for a regular unidirectional flow. This resulted in flow speeds of approximately 20 μm s−1. This was done to avoid artifacts due to signal smearing at high flow rates and thereby ensure maximal sensitivity.
The total intensity of an event (defined as one vesicle in one image) was calculated by summing up all pixel values inside a square around the detected position of the event. The size of this square was approximately (value rounded to integer values) three times the standard deviation of a Gaussian fit to its intensity on a given image. For the vesicles labelled with ATTO 550, the intensity was found by averaging the intensities of all events on the track since for those vesicles bleaching did not significantly affect the measurement. For the dual channel measurements where we detected emission from carboxyfluorescein, which is a comparatively weak emitter and sensitive to photo-bleaching, the intensity was determined by fitting a straight line to all recorded events and evaluating it at the first detected location of the vesicle, to minimize the effect of bleaching.
The recorded intensity distributions of vesicle samples were plotted as vesicle fraction versus square root of the measured intensity and fitted with a log-normal function. For less intense vesicle samples, fluorescence data fitting became difficult due to the absence of data points in the low intensity range. To improve the accuracy of the fit, a truncated fit with a cutoff at the vesicle with the lowest intensity was used. This truncated fit neglects the non-detectable fraction in the experimental data below the cutoff and thereby allows for an improved determination of the mode value as visualized in ESI† S5. The squared mode value of the fit, herein referred to as mode intensity, was used as a single measure of the overall ensemble-averaged sample intensity.
2.8. Extracellular vesicle experiments
Extracellular vesicles floating at a buoyant density between 1.24 g mL−1 and 1.31 g mL−1 were extracted from the conditioned media of a human mast cell line (HMC-1.2) following the protocol previously published by Lässer et al.62 The total protein content of the vesicle stock was determined to be 0.52 mg ml−1 using the Pierce bicinchoninic acid (BCA) protein assay kit (ThermoFisher Scientific, Gothenburg, Sweden) according to the manufacturer's instructions. Prior to each measurement, the vesicles were fluorescently labelled with the fluorescent cell linker PKH26 kit (Sigma-Aldrich, Stockholm, Sweden), in which the lipophilic PKH26 dye labels lipid membranes by intercalation into the bilayer.63 In brief, the dye stock (100 μM, ethanol) was diluted 50× in the diluent C solution accompanying the kit. The vesicles were diluted 12.5× in the same solution yielding a final extracellular vesicle concentration of 42 μg ml−1. The two solutions were subsequently mixed at equal volumes and incubated for 5 min at 6 °C. Dye and diluent C were then separated from the labelled extracellular vesicles using MicroSpin S-200 HR size exclusion columns (GE Healthcare Life Sciences, Uppsala, Sweden) centrifuged at 700 × g for 2 minutes. The running buffer contained 10 mM TRIS-HCl, 1 mM EDTA, (pH 7.4). Without taking eventual material loss in the filters into account, the final exosome suspension after dilution contained 21 μg ml−1 extracellular vesicles (total protein content). The extracellular vesicles were thereafter loaded into the nanofluidic device and analyzed for 10 minutes.
2.9. Peptide binding experiments
The DOPC/DOPG/DSPE-PEG2000/ATTO 550-DOPE vesicle stock solution was diluted to 50 μg ml−1 (total lipid content) and then mixed with a carboxyfluorescein-labeled arginine-enriched variant of the Antennapedia homeodomain peptide penetratin denoted PenArg64 in which all lysines have been substituted for arginines.65,66 The peptide was prepared by solid phase synthesis (80% purity) by Innovagen AB (Lund, Sweden). The samples were incubated under constant shaking for at least one hour at room temperature and thereafter loaded into the nanofluidic device for analysis. 10 mM HEPES, 5 mM NaOH, 1 mM EDTA, 100 mM (pH 7.4) was used as peptide binding buffer.
2.10. Nanoparticle tracking analysis
Vesicle size distributions were obtained using a NanoSight LM10 (Malvern Instruments Ltd, Malvern, UK) equipped with a 640 nm laser. For each sample, 5 measurements were performed and averaged. NTA data using both light scattering and fluorescence were obtained using a NanoSight LM10 equipped with a 532 nm laser and a 565 nm emission filter. The movies were analyzed with the software NTA 2:2 (Malvern Instruments Ltd, Malvern, UK).
2.11. Fluorimeter measurements
The average (bulk) fluorescence intensities of suspensions containing vesicles labelled with ATTO 550 lipids were obtained using a QM-4/2005 spectrofluorimeter (Photon Technology International Inc., North Edison, USA). The sample was excited at a wavelength of 550 nm and emission spectra were recorded between 570 nm and 720 nm. The emission value at the peak was used as a representative value of the fluorescence intensity.
3. Results and discussion
3.1. Nanofluidic device
In this work, nanofluidic devices fabricated in a silicon wafer were used as nano flow cytometers to detect individual flowing fluorescently labeled lipid vesicles, ∼100 nm in diameter, as they passed through a detection volume. The fluorescence signal was detected using standard epi-fluorescence microscopy (Fig. 1, ESI† Movie M1). As illustrated in Fig. 1a, the device consisted of a series of parallel nanochannels (250 channels with a period of 2 μm) connected by two microchannels. The microchannels were used for fast and efficient loading of the device while the nanochannels were used for vesicle observation. The nanochannels in the device used in this study had a square profile with 300 nm sides, but the dimensions can be tuned to suit the application of interest. Channels with restricted diffusion in the direction perpendicular to the imaging plane, i.e. “one-dimensional” nanochannels (allowing free movement only in one dimension) or “two-dimensional” nanoslits (allowing free movement in two dimensions) offer the advantage over deeper channels that they keep the vesicles in focus throughout the experiment. This makes it possible to accurately quantify the fluorescence intensity even of weakly fluorescent objects without surface immobilization and with a standard epi-fluorescence microscope. An advantage of one-dimensional nanochannels over two-dimensional nanoslits is that they ensure that the vesicles are well-separated, facilitating the unique detection of individual vesicles by the tracking software. Indeed, track crossing, which causes major problems during single particle tracking, can be excluded, making it possible to perform experiments at high vesicle concentrations. A further advantage of one-dimensional nanochannels is that the trajectory is fully predictable even at lower flow rates, which increases the speed of the data analysis significantly. Trajectory prediction increases sensitivity because it facilitates the detection of vesicles with intensities close to the background intensity, or of labels whose intensity may fluctuate (e.g. quantum dots), even if they are lost for a few frames. Trajectory prediction also makes it possible to discriminate the signal from the background noise which is random and therefore non-unidirectional and to colocalize two or more colors in a straight forward fashion.
The use of a miniaturized device, such as the one presented here, offers the possibility of analyzing vesicle-containing samples with very low sample consumption. For convenience, 20 μl of sample was loaded in a typical experiment, although this could be scaled down to a few microliters in the current device, or even less if the design of the inlet is adjusted. During the experiment, the amount of sample actually analyzed was typically in the nanoliter range. Without pushing the limits of our assays (see section 3.2), samples containing ∼109 vesicles per ml (low picomolar regime), i.e. concentrations of relevance in the context of cell-secreted vesicles,26 could be studied without pre-concentrating the sample. This is a major advantage and illustrates how our method is well-suited for analysis of rare and precious biological samples.
3.2. Vesicle quantification
A first key aspect in the context of characterizing vesicle samples is the possibility to accurately quantify vesicle numbers.26 This is, for example, essential for the development of extracellular-vesicle-based diagnostics where extracellular vesicle secretion profiles of healthy and diseased individuals are compared. Absolute quantification is also important for accurate determinations of the potency of vesicles with respect to their ability to induce a biological response in a recipient cell; this is for example relevant in the context of liposome-based drug delivery or for understanding exosome-mediated intercellular communication.
To demonstrate the potential of our nanofluidic-based tool to quantify the number of vesicles present in a sample, proof-of-principle experiments were performed using a dilution series of a sample containing fluorescently labeled lipid vesicles doped with 0.6 mol% ATT550-DOPE. At this dye concentration, the average-sized vesicles in our samples contained approximately 1000 fluorophores and the smallest approximatively 400 fluorophores, making them clearly visible. As seen in Fig. 2a, a linear dependence between the detected number of vesicles and the lipid concentration was observed for a lipid concentration range spanning over 3 orders of magnitude (from 130 nM to 254 μM of starting lipid material). This result indicates that the measurement performance was independent of the actual sample concentration, at least in the tested range. The corresponding concentration of vesicles detected in our experiment ranged from 170 fM to 500 pM (Fig. 2a). The limiting factor for low concentrations is the time required to collect enough data points. While a concentration determination at 500 pM could be carried out within 2 minutes, yielding as many as 5000 events, the concentration determination of the 170 fM sample required a runtime of 2 hours to ensure good statistics (i.e. a couple of 100 detected events). A major limitation at high concentrations is that the channels become too crowded to accurately resolve individual vesicles. This can be overcome by diluting the sample before loading into the device, extending the dynamic range dramatically. The absolute vesicle concentration of the samples measured in the nanofluidic device were found to be in excellent agreement with concentrations estimated from fluorescence-based NTA experiments carried out with Malvern's NanoSight, a commercial instrument commonly used for vesicle quantification (Fig. 2b).24,32 As shown in Fig. 2b the measured concentrations were also in good agreement with theoretical calculated values (yielding the absolute maximum of vesicles without considering lipid losses during preparation), estimated from the total amount of lipids weighed in at the start of the vesicle preparation, the volume and geometrical considerations based on a monodisperse vesicle sample (see section 2.2 for details). This indicates that our nanofluidic device functions at least as well as a commercial instrument with respect to counting the number of fluorescent vesicles present in solution and that the number of detected vesicles is realistic. A control experiment comparing the number of vesicles present in the micro- and nanochannels showed that the difference in the number of vesicles detected in the both channel types was <10% (see ESI† S4), confirming that there is no discrimination in our device at the step where the vesicles enter into the nanochannels.
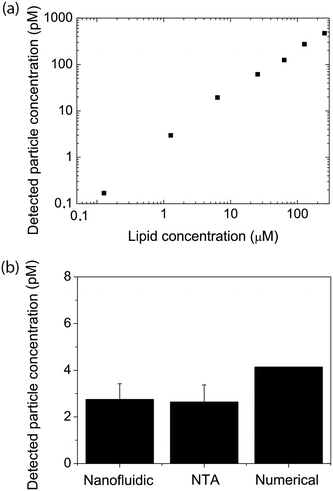 |
| Fig. 2 Vesicle quantification. (a) Measured vesicle concentration (vesicles containing 0.6 mol% of ATTO 550-DOPE) as a function of the amount of lipids used to prepare the films, for a dilution series of the same sample. (b) Measured vesicle concentration for a sample containing 1 μg ml−1 lipid. The vesicle concentration was either estimated from geometrical considerations (numerical) or measured using the nanofluidic-based assay or fluorescence-based nanoparticle tracking analysis (NTA). Measured values are the average and standard deviations of at least 3 measurements. | |
3.3. Intensity measurements and size determination
An essential aspect of the characterization of heterogeneous samples is the possibility to detect and quantify the fluorescence emitted by individual vesicles. This makes it possible, for example, to investigate membrane-mediated biomolecular recognition processes on a single vesicle, to characterize biomolecular heterogeneity of individual vesicles via immunostaining, and to identify vesicle subpopulations.
The second part of this work was therefore devoted to accurately quantifying the fluorescence intensity emitted by single lipid vesicles. A representative intensity distribution obtained with vesicles containing 0.6 mol% ATTO 550-DOPE (i.e. ∼1000 fluorophores per vesicle if the size is assumed to be 150 nm (Fig. 3a)) can be seen in Fig. 3b. Under the assumption that the fluorophores are equally distributed among vesicles of different size, the square root of the intensity is expected to be proportional to the diameter of the vesicles and the data can therefore be fitted with a log-normal distribution67 (solid line in Fig. 3b). Importantly, no cutoffs in the data were observed in either the high or low intensity value ranges. This indicates that the whole vesicle population entered the channels and that not even the largest vesicles present in the samples were restricted. Furthermore, it indicates that all vesicles present in the sample, even the smallest and thus least intense ones, were confidently detected. On the other hand, for vesicles prepared with a lower fraction of fluorophores, a cutoff in the low intensity range was observed (Fig. 3c). To ensure maximal accuracy in the determination of the mode value also for these distributions, a truncated fit with a cutoff at the vesicle with the lowest intensity was applied (see materials and methods section 2.7 and ESI† S5).
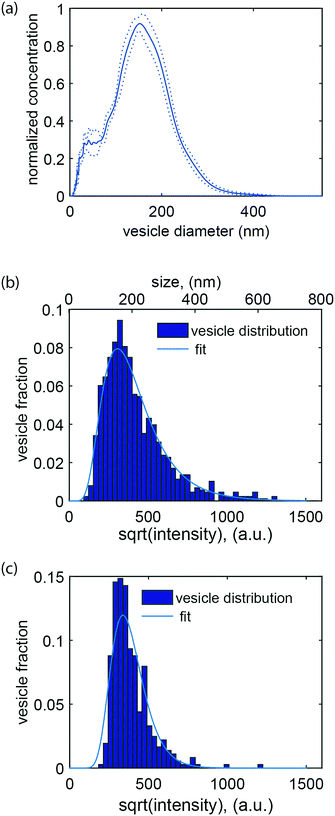 |
| Fig. 3 Size and intensity distribution of vesicles labeled with a fluorescent lipid. (a) Size distribution of the vesicles as measured with nanoparticle tracking analysis, NTA (mean (solid line) ± standard deviation (dotted lines)). The mode of the distribution is at 152 nm. (b) Square root of the intensity (lower y axis) versus vesicle fraction for vesicles containing 0.6 mol% ATTO 550 (exposure time: 5 ms). The distribution can be fitted with a log-normal function. Calibration of the mode value against the NTA measurement shown in (a) yields the diameter value in nanometer (upper y axis). (c) Square root of the intensity versus vesicle fraction for vesicles containing 0.02 mol% ATTO 550 (exposure time 50 ms). | |
It is possible to obtain the size of the vesicles analysed in our device under the assumption that the fluorescent lipids are equally distributed among vesicles of different sizes and hence that the fluorescence intensity is directly proportional to the vesicle's surface area. To achieve this, we calibrated our data by nanoparticle tracking analysis (NTA), but other calibration methods that provide absolute size determination, such as for example dynamic light scattering (DLS), should also be suitable. Upon calibration, it was possible to compare the mode value of the log-normal fit to our data and the maximum of the size distribution measured by NTA, using the same sample. This allowed us to directly determine the vesicle size from the corresponding fluorescence intensity (Fig. 3b). Altogether, size determination makes it possible to relate biomolecular recognition with the vesicle size, for example, in the context of studying curvature-sensing proteins.68
The ability to accurately determine intensity distributions and the measurement reproducibility was further investigated using vesicles that contained between 0.02 mol% and 2.4 mol% ATTO 550, i.e. on average between 40 and 4000 fluorophores per vesicle. As further detailed in materials and methods (section 2.7), the mode of the intensity distribution was used as a representative of the overall sample intensity measured with the nanofluidic device.
This value was compared to the average fluorescence intensity of the vesicle sample measured with a fluorimeter. As shown in Fig. 4a, the signal obtained from our nanofluidic-based assay scales linearly with the intensity determined by fluorimetry for fluorophore concentrations between 0.2 mol% and 2.4 mol%. At lower fluorophore concentrations, the average signal is overestimated, leading to a deviation from the linear behavior (see Fig. 4a and ESI† S6). Indeed, inaccuracies in the log-normal fit, due to an increased presence of non-detectable vesicles, lead to a shift in the peak of the log-normal distribution towards higher mode intensity values (see Fig. 4a).
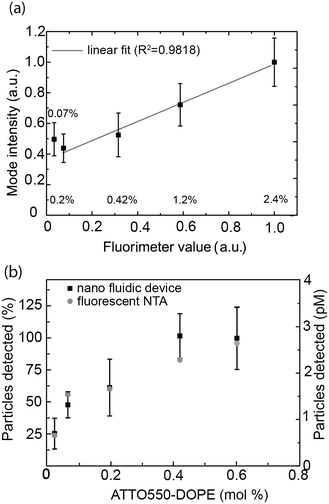 |
| Fig. 4 Influence of the fluorophore content on the vesicle intensity. (a) Vesicle mode intensity values as determined with the nanofluidic-based assay using 5 ms exposure times versus average fluorescence intensity as measured by fluorimetry. The average and standard deviation for at least 3 independent measurements is reported. The corresponding mol% of ATTO 550-lipids in the vesicles is indicated in the graph. Gray solid line: linear fit (0.07% point is excluded from the fit). (b) Average number of vesicles detected for measurements using the nanofluidic-based assay (black squares) and by fluorescence-based NTA (grey dots). The left y-axis is the data normalized using the number of vesicles detected at 0.6 mol% ATTO 550-DOPE (nanofluidic-based assay) as a reference (100%). The right y-axis is the absolute concentration measured. Nanofluidic-based assay data: average and standard deviation of 3 independent measurements. | |
To gain further insights into the percentage of vesicles detectable for different concentrations of ATTO 550-DOPE, we quantified the number of vesicles counted for vesicles containing different amounts of fluorophores. As shown in Fig. 4b, the number of detected vesicles plateaued at ATTO 550-DOPE concentrations above 0.4 mol%, reaching 100%, indicating that most vesicles are detected at the highest fluorophore concentrations. The percentage of vesicles detectable as function of the vesicle's ATTO 550-DOPE content estimated from the nanofluidic-based assay was compared to the one obtained with the commercial fluorescence-based NTA. Both techniques had comparable sensitivities (Fig. 4b) and the current limit of detection with our experimental setup and choice of dyes lies at ∼100 fluorophores per vesicle. This was estimated from the percentage of vesicles detectable and the known size distribution, making it possible to estimate the smallest lipid vesicle detectable and its fluorophore content. However, it should be stressed that the number of fluorophores detectable mainly depends on the quality of the dye and on the experimental setup (microscope, camera). Indeed using bright dyes and appropriate instrumentation, sensitivities down to the single fluorophore level have been reached in nanofluidic channels.69
3.4. Detection and quantification of extracellular vesicles
To demonstrate the applicability to study biological samples, we used the nanofluidic-based assay for extracellular vesicle concentration quantification. Extracellular vesicles were obtained from the supernatant of a human mast cell line and labelled using the self-inserting membrane dye PKH26 (see materials and methods section 2.8). The vesicles were successfully visualized (Fig. 5a), and flowed through the nanochannels without hinderance; >95% of the channels were found to be open (Fig. 5b). This indicates that the lipid passivation of the nanochannels is suitable also for biological samples. The labelled extracellular vesicles exhibited rather weak intensities (compared to background) but due to the steady flow and unidirectional motion of the vesicles in the nanofluidic device it was possible to accurately predict their movement and thus detection was possible. The extracellular vesicle concentration of our stock sample of 0.52 mg ml−1 (total protein content) was estimated to be 80 pM. This is in the same order of magnitude as that estimated in a fluorescence-based NTA measurement of an equally prepared extracellular vesicle sample while the protein-to-particle number ratio is on the same order of magnitude as the one reported in other studies.70 Furthermore, assuming a buoyant density of 1.28 g ml−1 and an average vesicle diameter of 150 nm, it can be estimated that the proteins contribute to about 20% of the vesicle's buoyant mass.
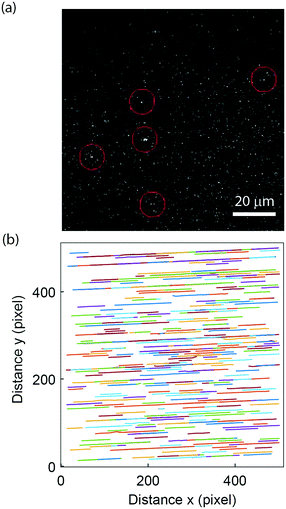 |
| Fig. 5 Extracellular vesicle visualization. (a) Visualization of individual extracellular vesicles labelled with PKH26 in the nanofluidic channel. The red circles mark the position of the vesicles. (b) Plot of the detected tracks showing no sticking events and 99 open channels (i.e. <5% of the channels are empty), thereby indicating than most channels in the device are open during the experiment. | |
3.5. Two-colour recording of peptide binding to vesicles
Microscopy-based imaging of vesicles in the nanochannel device allows for simultaneous recording of at least two emission colors, which makes it possible to carry out co-localization experiments. Using the nanofluidic-based assay, we demonstrate that co-localization of two emission colors and ratiometric intensity measurements can be used to analyze the binding of a peptide to the lipid vesicle membrane. As model we used an arginine-enriched variant of the Antennapedia homeodomain peptide penetratin (PenArg).66 This cationic cell-penetrating peptide exhibits superior uptake characteristics compared to wild type penetratin.71 It adopts an amphipathic α-helical configuration upon interaction with negatively charged lipid vesicle membranes and binds in the interface region, parallel to the membrane surface.72 We used a PenArg variant labelled with carboxyfluorescein (CF) at the N-terminus to investigate its binding to negatively charged vesicles containing 37 mol% DOPG lipids and 0.5 mol% ATTO 550-DOPE. The peptide concentrations ranged between 5 μM and 20 μM. The possibility of predicting the position of the vesicle from the relatively bright red lipid signal and then measuring the fluorescence intensity at the determined position for the much weaker green peptide signal significantly improved the detection of the peptide signal.
Since the data collected gives information on the size-distribution of the vesicles via analysis of the red lipid signal, we could directly analyze our data for curvature-related effects. This was done by considering the dependence of the ratio of the green and the red signal, which reflects the peptide density at the membrane surface, on vesicle size (absolute intensity of the red signal). As shown in Fig. 6a, the PenArg peptide displays an increased affinity for smaller vesicles, i.e. for larger positive membrane curvature. This was observed at all peptide concentrations as further illustrated in Fig. 6b. Furthermore, in all cases, the peptide surface-density increased with increased peptide concentration in bulk for concentrations up to 20 μM, indicating that at those concentrations the peptide does not saturate the membrane surface. At peptide concentrations higher than 20 μM, the incubated vesicles started to aggregate, as also reported by others,73 and resulted in clogging of the channels.
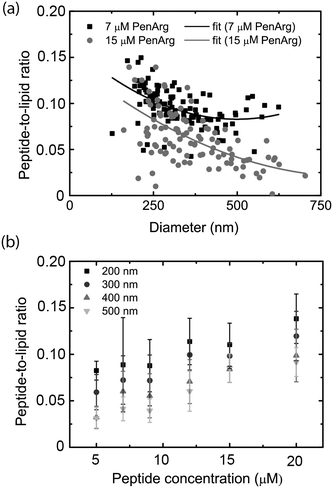 |
| Fig. 6 Penetratin (PenArg) binding to individual lipid vesicles. The binding of carboxyfluorescein-labelled PenArg (green) to DOPC/DOPG/DSPE-PEG2000 vesicles containing 0.6 mol% ATTO 550-DOPE is investigated using the nano fluidic device. (a) Representative ratio between green and red signal (peptide-to-lipid ratio), proportional to the peptide surface density on the membrane versus the vesicle diameter. The data is displayed for two PenArg bulk concentrations: 7 μM (gray) and 15 μM (black). The solid lines show a polynomial fit to guide the eye. (b) Average values of the ratio between green and red signals (peptide-to-lipid ratio) for vesicles populations of 4 different sizes. The data points are the average of all data points that fall between 150–250 (denoted 200 nm), 250–350 (denoted 300 nm) 350–450 (denoted 400 nm) and 450–550 (denoted 500 nm), respectively. The error bars are the standard deviations. | |
Curvature dependence, in particular increased affinity for small vesicles, has been demonstrated for several peptides and also other molecules binding to lipid membranes,14,68,74,75 but has not been discussed before in the context of penetratin and its variants. Positive-curvature sensing has mainly been associated with the increased amount of defects, e.g. hydrophobic pockets, exposed upon membrane bending into small vesicles.76 These defects can increase binding of certain types of proteins by facilitating the insertion of hydrophobic residues.77 It is likely that a similar mechanism is responsible for positive-curvature sensing in the case of PenArg, in particular since this peptide is known to bind lipid vesicles as an amphipathic helix.72 Taken together, these results illustrate the potential of the nanofluidic-based assay in the context of probing biomolecular interactions occurring at membrane surfaces. A major advantage over other techniques, including surface-based ones, is that sampling is carried out within a couple of minutes since no surface anchoring procedure is required.
4. Conclusion
We have presented a number of experiments based on a nanofluidic device setup functioning as a nano flow cytometer. This new methodology allows for quantification and characterization of fluorescent vesicles in solution and for simultaneous multi-channel quantification of fluorescence intensities. The device exhibits low sample consumption, is easy to operate and is compatible with standard epi-fluorescence microscopy. The device allows for single vesicle analysis and particle concentration determination with an accuracy and sensitivity equaling that of standard NTA, however with the advantage of allowing multi-color detection. We demonstrate that the device can be used to count single vesicles in biological samples, such as exosomes, and to detect binding reactions occurring at the surface of individual vesicles. This paves the way to a number of exciting applications in biological and medical sciences. In the context of the study of extracellular vesicles, it will, for example, offer the possibility to investigate vesicle subpopulations characterized by the presence of specific biomolecules on the surfaces, providing understanding of their role in intercellular communication in health and disease. The assay is also likely to find applications in the study of liposomes, in the context of the development of drug delivery vehicles as well as for fundamental studies of membrane proteins and bilayer-mediated binding reactions. Furthermore, the possibility of quantifying the number of particles found in solution makes our tool well-suited for diagnostic and environmental purposes, for example for virus detection or in disease diagnostics where specific vesicle types can serve as valuable markers. It is important to stress that the nanochannels are well suited for integration in fully automated lab-on-a-chip devices where further analytical components, including modules for extracellular vesicle purification,78 collection79 lysis80 or further analysis80 can be integrated.
Acknowledgements
The Swedish Research Council (Vetenskapsrådet, 621-2012-5024 to MB, 2011-4324 to FW, and 2011-04844 to EKE), Kristina Stenborg Stiftelse, the Marie Curie Actions, the Area of Advance Nanoscience and Nanotechnology at Chalmers University of Technology, and the Wenner-Gren foundations are acknowledged for financial support. The authors would like to thank Prof. Jan Lötvall (Gothenburg University) for providing the extracellular vesicles, Déborah Rupert for help with extracellular vesicle staining and Agnieszka Siupa for her support with NTA measurements.
References
- E. van der Pol, A. N. Boing, P. Harrison, A. Sturk and R. Nieuwland, Pharmacol. Rev., 2012, 64, 676–705 CrossRef CAS PubMed.
- B. Gyorgy, T. G. Szabo, M. Pasztoi, Z. Pal, P. Misjak, B. Aradi, V. Laszlo, E. Pallinger, E. Pap, A. Kittel, G. Nagy, A. Falus and E. I. Buzas, Cell. Mol. Life Sci., 2011, 68, 2667–2688 CrossRef CAS PubMed.
- A. Bobrie, M. Colombo, G. Raposo and C. Thery, Traffic, 2011, 12, 1659–1668 CrossRef CAS PubMed.
- C. Thery, M. Ostrowski and E. Segura, Nat. Rev. Immunol., 2009, 9, 581–593 CrossRef CAS PubMed.
- M. Alexander, R. Hu, M. C. Runtsch, D. A. Kagele, T. L. Mosbruger, T. Tolmachova, M. C. Seabra, J. L. Round, D. M. Ward and R. M. O'Connell, Nat. Commun., 2015, 6, 7321 CrossRef CAS PubMed.
- E. I. Buzas, B. Gyorgy, G. Nagy, A. Falus and S. Gay, Nat. Rev. Rheumatol., 2014, 10, 356–364 CrossRef CAS PubMed.
- T. N. Lamichhane, S. Sokic, J. S. Schardt, R. S. Raiker, J. W. Lin and S. M. Jay, Tissue Eng., Part B, 2015, 21, 45–54 CrossRef CAS PubMed.
- C. Admyre, E. Telemo, N. Almqvist, J. Lotvall, R. Lahesmaa, A. Scheynius and S. Gabrielsson, Allergy, 2008, 63, 404–408 CrossRef CAS PubMed.
- K. Al-Nedawi, B. Meehan and J. Rak, Cell Cycle, 2009, 8, 2014–2018 CrossRef CAS PubMed.
- K. M. Candelario and D. A. Steindler, Trends Mol. Med., 2014, 20, 368–374 CrossRef CAS PubMed.
- A. Schneider and M. Simons, Cell Tissue Res., 2013, 352, 33–47 CrossRef CAS PubMed.
- R. J. Simpson, J. W. E. Lim, R. L. Moritz and S. Mathivanan, Expert Rev. Proteomics, 2009, 6, 267–283 CrossRef CAS PubMed.
- Y. H. M. Chan and S. G. Boxer, Curr. Opin. Chem. Biol., 2007, 11, 581–587 CrossRef CAS PubMed.
- M. B. Jensen, V. K. Bhatia, C. C. Jao, J. E. Rasmussen, S. L. Pedersen, K. J. Jensen, R. Langen and D. Stamou, J. Biol. Chem., 2011, 286, 42603–42614 CrossRef CAS PubMed.
- M. Bally, K. Bailey, K. Sugihara, D. Grieshaber, J. Voros and B. Stadler, Small, 2010, 6, 2481–2497 CrossRef CAS PubMed.
- K. Bailey, M. Bally, W. Leifert, J. Voros and T. McMurchie, Proteomics, 2009, 9, 2052–2063 CrossRef CAS PubMed.
- S. R. Tabaei, M. Rabe, V. P. Zhdanov, N. J. Cho and F. Hook, Nano Lett., 2012, 12, 5719–5725 CrossRef CAS PubMed.
- T. M. Allen and P. R. Cullis, Adv. Drug Delivery Rev., 2013, 65, 36–48 CrossRef CAS PubMed.
- G. Gregoria, E. J. Wills, C. P. Swain and A. S. Tavill, Lancet, 1974, 1, 1313–1316 CrossRef.
- V. P. Torchilin, Nat. Rev. Drug Discovery, 2005, 4, 145–160 CrossRef CAS PubMed.
- R. K. Anand and D. T. Chiu, Curr. Opin. Chem. Biol., 2012, 16, 391–399 CrossRef CAS PubMed.
- J. Larsen, N. S. Hatzakis, E. Elizondo, I. Cabrera, J. Veciana, N. Ventosa and D. Stamou, Biophys. J., 2012, 102, 426a CrossRef.
- S. Mathiasen, S. M. Christensen, J. J. Fung, S. G. F. Rasmussen, J. F. Fay, S. K. Jorgensen, S. Veshaguri, D. L. Farrens, M. Kiskowski, B. Kobilka and D. Stamou, Nat. Methods, 2014, 11, 931–934 CrossRef CAS PubMed.
- C. X. Chen, S. B. Zhu, T. X. Huang, S. Wang and X. M. Yan, Anal. Methods, 2013, 5, 2150–2157 RSC.
- J. Kowal, G. Arras, M. Colombo, M. Jouve, J. P. Morath, B. Primdal-Bengtson, F. Dingli, D. Loew, M. Tkach and C. Thery, Proc. Natl. Acad. Sci. U. S. A., 2016, 113, E968–E977 CrossRef CAS PubMed.
- D. L. Rupert, V. Claudio, C. Lasser and M. Bally, Biochim. Biophys. Acta, 2017, 1861, 3164–3179 CrossRef CAS PubMed.
- P. Wolf, Br. J. Haematol., 1967, 13, 269–288 CrossRef CAS PubMed.
- B. T. Pan, K. Teng, C. Wu, M. Adam and R. M. Johnstone, J. Cell Biol., 1985, 101, 942–948 CrossRef CAS PubMed.
- P. M. Frederik and D. H. Hubert, Methods Enzymol., 2005, 391, 431–448 Search PubMed.
- T. Pisitkun, R. F. Shen and M. A. Knepper, Proc. Natl. Acad. Sci. U. S. A., 2004, 101, 13368–13373 CrossRef CAS PubMed.
- N. Arraud, R. Linares, S. Tan, C. Gounou, J. M. Pasquet, S. Mornet and A. R. Brisson, J. Thromb. Haemostasis, 2014, 12, 614–627 CrossRef CAS PubMed.
- A. Malloy and B. Carr, Part. Part. Syst. Charact., 2006, 23, 197–204 CrossRef.
- R. A. Dragovic, C. Gardiner, A. S. Brooks, D. S. Tannetta, D. J. P. Ferguson, P. Hole, B. Carr, C. W. G. Redman, A. L. Harris, P. J. Dobson, P. Harrison and I. L. Sargent, J. Nanomed. Nanotechnol., 2011, 7, 780–788 CrossRef CAS PubMed.
- E. van der Pol, F. A. W. Coumans, A. Sturk, R. Nieuwland and T. G. van Leeuwen, Nano Lett., 2014, 14, 6195–6201 CrossRef CAS PubMed.
- B. Agnarsson, A. Lundgren, A. Gunnarsson, M. Rabe, A. Kunze, M. Mapar, L. Simonsson, M. Bally, V. P. Zhdanov and F. Hook, ACS Nano, 2015, 9, 11849–11862 CrossRef CAS PubMed.
- P. Kukura, H. Ewers, C. Muller, A. Renn, A. Helenius and V. Sandoghdar, Nat. Methods, 2009, 6, 923–927 CrossRef CAS PubMed.
- G. G. Daaboul, A. Yurt, X. Zhang, G. M. Hwang, B. B. Goldberg and M. S. Unlu, Nano Lett., 2010, 10, 4727–4731 CrossRef CAS PubMed.
- H. Ewers, V. Jacobsen, E. Klotzsch, A. E. Smith, A. Helenius and V. Sandoghdar, Nano Lett., 2007, 7, 2263–2266 CrossRef CAS PubMed.
- J. Larsen, N. S. Hatzakis and D. Stamou, J. Am. Chem. Soc., 2011, 133, 10685–10687 CrossRef CAS PubMed.
- M. Rabe, S. R. Tabaei, H. Zetterberg, V. P. Zhdanov and F. Hook, Angew. Chem., Int. Ed., 2015, 54, 1022–1026 CrossRef CAS PubMed.
- S. M. Christensen and D. G. Stamou, Sensors, 2010, 10, 11352–11368 CrossRef CAS PubMed.
- K. Vorauer-Uhl, A. Wagner, N. Borth and H. Katinger, Cytometry, 2000, 39, 166–171 CrossRef CAS PubMed.
- E. J. van der Vlist, E. N. M. Nolte-'t Hoen, W. Stoorvogel, G. J. A. Arkesteijn and M. H. M. Wauben, Nat. Protoc., 2012, 7, 1311–1326 CrossRef PubMed.
- E. N. M. Nolte-'t Hoen, E. J. van der Vlist, M. de Boer-Brouwer, G. J. A. Arkesteijn, W. Stoorvogel and M. H. M. Wauben, J. Leukocyte Biol., 2013, 93, 395–402 CrossRef PubMed.
- E. N. M. Nolte-'t Hoen, E. J. van der Vlist, M. Aalberts, H. C. H. Mertens, B. J. Bosch, W. Bartelink, E. Mastrobattista, E. V. B. van Gaal, W. Stoorvogel, G. J. A. Arkesteijn and M. H. M. Wauben, J. Nanomed. Nanotechnol., 2012, 8, 712–720 CrossRef PubMed.
- R. Gaudin and N. S. Barteneva, Nat. Commun., 2015, 6, 6022 CrossRef CAS PubMed.
- T. D. Chung and H. C. Kim, Electrophoresis, 2007, 28, 4511–4520 CrossRef CAS PubMed.
- Y. S. Zhang, B. R. Watts, T. Y. Guo, Z. Y. Zhang, C. Q. Xu and Q. Y. Fang, Micromachines, 2016, 7(4), 70 CrossRef.
- A. Y. Fu, C. Spence, A. Scherer, F. H. Arnold and S. R. Quake, Nat. Biotechnol., 1999, 17, 1109–1111 CrossRef CAS PubMed.
- P. S. Dittrich and P. Schwille, Anal. Chem., 2003, 75, 5767–5774 CrossRef CAS PubMed.
- P. G. Schiro, J. C. Gadd, G. S. Yen and D. T. Chiu, J. Phys. Chem. B, 2012, 116, 10490–10495 CrossRef CAS PubMed.
- S. Faez, Y. Lahini, S. Weidlich, R. F. Garmann, K. Wondraczek, M. Zeisberger, M. A. Schmidt, M. Orrit and V. N. Manoharan, ACS Nano, 2015, 9, 12349–12357 CrossRef CAS PubMed.
- B. R. Cipriany, P. J. Murphy, J. A. Hagarman, A. Cerf, D. Latulippe, S. L. Levy, J. J. Benitez, C. P. Tan, J. Topolancik, P. D. Soloway and H. G. Craighead, Proc. Natl. Acad. Sci. U. S. A., 2012, 109, 8477–8482 CrossRef CAS PubMed.
- S. L. Levy and H. G. Craighead, Chem. Soc. Rev., 2010, 39, 1133–1152 RSC.
- F. Persson and J. O. Tegenfeldt, Chem. Soc. Rev., 2010, 39, 985–999 RSC.
- P. E. G. Thoren, D. Persson, E. K. Esbjörner, M. Goksor, P. Lincoln and B. Norden, Biochemistry, 2004, 43, 3471–3489 CrossRef CAS PubMed.
- R. C. Macdonald, R. I. Macdonald, B. P. M. Menco, K. Takeshita, N. K. Subbarao and L. R. Hu, Biochim. Biophys. Acta, 1991, 1061, 297–303 CrossRef CAS.
- F. Persson, J. Fritzsche, K. U. Mir, M. Modesti, F. Westerlund and J. O. Tegenfeldt, Nano Lett., 2012, 12, 2260–2265 CrossRef CAS PubMed.
- G. Lantzsch, H. Binder and H. Heerklotz, J. Fluoresc., 1994, 4, 339–343 CrossRef CAS PubMed.
-
E. Meijering, O. Dzyubachyk and I. Smal, Imaging and
Spectroscopic Analysis of Living Cells: Optical and Spectroscopic Techniques, 2012, vol. 504, pp. 183–200 Search PubMed.
-
R. O. Duda and P. E. Hart, Pattern Classification and Scene Analysis, John Wiley & Sons, Inc, 1973 Search PubMed.
- C. Lässer, V. Seyed Alikhani, K. Ekström, M. Eldh, P. Torregrosa Paredes, A. Bossios, M. Sjöstrand, S. Gabrielsson, J. Lötvall and H. Valadi, J. Transl. Med., 2011, 9, 9 CrossRef PubMed.
- P. K. Horan, M. J. Melnicoff, B. D. Jensen and S. E. Slezak, Methods Cell Biol., 1990, 33, 469–490 CrossRef CAS PubMed.
- A. Joliot, C. Pernelle, H. Deagostinibazin and A. Prochiantz, Proc. Natl. Acad. Sci. U. S. A., 1991, 88, 1864–1868 CrossRef CAS.
- D. Persson, P. E. G. Thoren, P. Lincoln and B. Norden, Biochemistry, 2004, 43, 11045–11055 CrossRef CAS PubMed.
- P. E. Thoren, D. Persson, P. Isakson, M. Goksor, A. Onfelt and B. Norden, Biochem. Biophys. Res. Commun., 2003, 307, 100–107 CrossRef CAS PubMed.
- A. H. Kunding, M. W. Mortensen, S. M. Christensen and D. Stamou, Biophys. J., 2008, 95, 1176–1188 CrossRef CAS PubMed.
- A. L. Christensen, C. Lohr, S. M. Christensen and D. Stamou, Lab Chip, 2013, 13, 3613–3625 RSC.
- S. Tyagi, V. VanDelinder, N. Banterle, G. Fuertes, S. Milles, M. Agez and E. A. Lemke, Nat. Methods, 2014, 11, 297–300 CrossRef CAS PubMed.
- D. L. M. Rupert, C. Lasser, M. Eldh, S. Block, V. P. Zhdanov, J. O. Lotvall, M. Bally and F. Hook, Anal. Chem., 2014, 86, 5929–5936 CrossRef CAS PubMed.
- H. L. Åmand, K. Fant, B. Norden and E. K. Esbjörner, Biochem. Biophys. Res. Commun., 2008, 371, 621–625 CrossRef PubMed.
- C. E. B. Caesar, E. K. Esbjörner, P. Lincoln and B. Norden, Biochemistry, 2006, 45, 7682–7692 CrossRef CAS PubMed.
- D. Persson, P. E. G. Thoren and B. Norden, FEBS Lett., 2001, 505, 307–312 CrossRef CAS PubMed.
- B. J. Peter, H. M. Kent, I. G. Mills, Y. Vallis, P. J. G. Butler, P. R. Evans and H. T. McMahon, Science, 2004, 303, 495–499 CrossRef CAS PubMed.
- H. T. McMahon and J. L. Gallop, Nature, 2005, 438, 590–596 CrossRef CAS PubMed.
- S. Vanni, L. Vamparys, R. Gautier, G. Drin, C. Etchebest, P. F. Fuchs and B. Antonny, Biophys. J., 2013, 104, 575–584 CrossRef CAS PubMed.
- N. S. Hatzakis, V. K. Bhatia, J. Larsen, K. L. Madsen, P. Y. Bolinger, A. H. Kunding, J. Castillo, U. Gether, P. Hedegard and D. Stamou, Nat. Chem. Biol., 2009, 5, 835–841 CrossRef CAS PubMed.
- K. Lee, H. L. Shao, R. Weissleder and H. Lee, ACS Nano, 2015, 9, 2321–2327 CrossRef CAS PubMed.
- M. He, J. Crow, M. Roth, Y. Zeng and A. K. Godwin, Lab Chip, 2014, 14, 3773–3780 RSC.
- D. Taller, K. Richards, Z. Slouka, S. Senapati, R. Hill, D. B. Go and H. C. Chang, Lab Chip, 2015, 15, 1656–1666 RSC.
Footnote |
† Electronic supplementary information (ESI) available. See DOI: 10.1039/c6lc01302c |
|
This journal is © The Royal Society of Chemistry 2017 |