DOI:
10.1039/C5TX00378D
(Paper)
Toxicol. Res., 2016,
5, 107-115
Cumulative metabolic effects of low-dose benzo(a)pyrene exposure on human cells†
Received
15th October 2015
, Accepted 13th November 2015
First published on 18th November 2015
Abstract
Benzo(a)pyrene (B[a]P) is a common environmental and foodborne pollutant which has been identified as a Group I carcinogen. Although the carcinogenicity of B[a]P has been illustrated, its comprehensive influence on metabolism and further relevance in adverse health outcomes are not well understood. To investigate the global metabolic effects of long-term B[a]P exposure at environmental dosage, we utilized the human SMMC-7721 cell-based B[a]P exposure models to perform a metabolomics study and network analysis. A total of 316 biochemicals were identified and 104 metabolites were found to be significantly altered. Bioinformatics analysis showed that the amino acid, carbohydrate, and lipid metabolism pathways and the nucleotide metabolism pathway were influenced by prolonged B[a]P exposure. Notably, the metabolic effects of B[a]P varied with different dosages. In addition, B[a]P exposure caused a decline in the glycolysis process but enhanced the glycolytic capability of SMMC-7721 cells in vitro. These findings establish the overall B[a]P-induced metabolic network, characterize the metabolic effects of chronic and environmental B[a]P exposure on human-relevant cells, and enhance the understanding of the adverse outcome pathway frame of B[a]P.
Introduction
Benzo(a)pyrene (B[a]P), a prototypical member of the polycyclic aromatic hydrocarbons (PAHs),1,2 is formed by the process of incomplete combustion of organic materials.3,4 B[a]P has been listed as a Group 1 carcinogen by the International Agency for Research on Cancer (IARC).5 As a typical environmental and foodborne pollutant, B[a]P exposure is almost inevitable for humans through the ingestion of charcoal-grilled foods and contaminated water, the inhalation of engine exhaust fumes and cigarette smoke.6,7 Therefore, it is important to reveal the cumulative toxicity and the molecular effects of B[a]P in the environment thoroughly.
Once taken up into cells, B[a]P is metabolized to form various reactive metabolites, which elicit toxicity through binding covalently to cellular elements such as DNA and generating reactive oxygen species to damage cellular macromolecules.8,9 However, although the toxic effects of B[a]P including teratogenicity, carcinogenicity, neurotoxicity, immunotoxicity, etc., have been studied,10–13 most of the understanding of the bio-safety assessment of B[a]P has been obtained from the high-dose toxicity evaluation with laboratory animals, which is not conclusive for illustrating the effects of prolonged B[a]P exposure at lower environmental concentrations. It is recommended by the U.S. National Research Council to transform the toxicity testing from high-dose animal studies to pathway-based approaches using human-relevant cells.14,15 We have established a long-term and low-dose B[a]P exposure model based on human SMMC-7721 cells,16 which provides a useful tool for exploring the cumulative toxicity of low-dose B[a]P on human cells.
To achieve a comprehensive understanding of the toxicological behavior of B[a]P, it is necessary to analyze the global molecular responses in the cells. Among them, metabolic perturbation, which usually manifests as metabolite profiling changes, is a common event in pollutant-induced toxicity. In the adverse outcome pathway (AOP) frame, metabolism perturbation represents a typical key event (KE) which originates from the molecular initiating events (MIE) such as gene/protein expression changes and subsequently induces cell phenotypic effects. Metabolomics is capable of providing the global metabolic status and the whole-cell response,17 which makes it a reasonable and effective technique for determining the metabolic profiles induced by B[a]P exposure. However, although some studies about the metabolic effects of B[a]P in animals, including fish, earthworms and rats, have been reported,18–20 the potential influence of prolonged B[a]P exposure, especially at lower environmental doses, on metabolic disruption of human cells is rarely reported.
In this study, we investigated the chronic toxicological effects of B[a]P on the global metabolic profiling in human cells with long-term and low-dose B[a]P exposure. The metabolites significantly altered were identified using the gas chromatography/mass spectrometry (GC/MS) and liquid chromatography/mass spectrometry (LC/MS/MS) based approaches. The potentially altered metabolic process and pathways were explored through bioinformatics analyses. This study characterizes the metabolic effects of chronic and environmental B[a]P exposure on human SMMC-7721 cells and contributes to the more comprehensive understanding of the toxicity of B[a]P.
Materials and methods
Cell cultures
SMMC-7721 cells originally from the Cell Bank of the Shanghai Institutes for Biological Sciences, Chinese Academy of Sciences (SIBS, CAS) were cultured in RPMI 1640 medium supplemented with 10% fetal bovine serum, 100 μg mL−1 penicillin, and 100 μg mL−1 streptomycin and maintained in an incubator under a humidified atmosphere of 5% CO2 at 37 °C. The cells were co-cultured by B[a]P (0.01 nM, 1 nM, 100 nM) or 0.1% DMSO for a month and subjected to the subsequent analyses as described previously.16
Metabolomics analysis
Cells were washed with 10 mL of PBS, trypsinized with 0.25% Trypsin-EDTA, and washed with ice-cold PBS. About 9 × 106 cells from each triplicate sample were pelleted, flash frozen in liquid nitrogen, and stored at −80 °C. Cell pellets were shipped to Metabolon, Inc. (SJTU-Metabolon Joint Metabolomics Laboratory), on dry ice for metabolomics analysis. At the time of analysis, samples were extracted and split into equal parts for analysis by GC/MS and LC/MS/MS platforms.21,22 Several technical replicate samples created from a homogeneous pool containing a small amount of all study samples were also included. Global biochemical profiles, from DMSO-treated SMMC-7721 cells and cells treated for one month with varying doses of B[a]P (0.01 nM, 1 nM, 100 nM) were compared.
The LC/MS portion of the platform was based on a Waters ACQUITY UPLC (Waters, Milford, MA) and a Thermo-Finnigan LTQ mass spectrometer (Thermo Electron Corporation, San Jose, CA), which consisted of an electrospray ionization source and a linear ion-trap mass analyzer. The sample extract was split into two aliquots, dried, and then reconstituted in acidic or basic LC-compatible solvents, each of which contained 11 or more injection standards at fixed concentrations. One aliquot was analyzed using acidic positive ion-optimized conditions and the other using basic negative ion-optimized conditions in two independent injections using separate UPLC columns (Waters UPLC BEH C18-2.1 × 100 mm, 1.7 μm). Extracts reconstituted under acidic conditions were gradient-eluted using water and 95% methanol, both containing 0.1% formic acid, while the basic extracts were gradient-eluted with water and 95% methanol containing 6.5 mM ammonium bicarbonate. The MS instrument scanned 99–1000 m/z and alternated between MS and MS2 scans using dynamic exclusion with approximately 6 scans per second.
The samples destined for GC/MS analysis were re-dried under vacuum desiccation for >24 h prior to being derivatized under dried nitrogen using bistrimethyl-silyl-trifluoroacetamide (BSTFA). The GC column was a 5% phenyl dimethyl silicone column with helium as the carrier gas and the temperature ramp was from 40 to 300 °C in a 16 min period. Samples were analyzed on a Thermo-Finnigan Trace DSQ fast-scanning single-quadrupole mass spectrometer operated at unit mass resolving power with electron impact ionization and a 50–750 atomic mass unit scan range. The information output from the raw data files was automatically extracted.
Metabolites were identified by automated comparison of the ion features in the experimental samples to a reference library of chemical standard entries that included retention time, molecular weight (m/z), preferred adducts, and in-source fragments as well as their associated MS/MS2 spectra. This library allowed the rapid identification of metabolites in the experimental samples with high-confidence.
Network construction and analysis
Cytoscape software (Version 3.1.1; http://www.cytoscape.org) and the MetScape plugin (Version 3.0.2) were used to construct the Compound-Reaction-Enzyme-Gene (CREG) metabolic network. The enriched pathways of metabolic networks were from an internal relational database stored at NCIBI which integrates data from Kyoto Encyclopedia of Genes and Genomes (KEGG) and Edinburgh Human Metabolic Network (EHMN). With the plugin BiNGO (Version 3.0.2) in Cytoscape, the Gene Ontology (GO) terms that statistically overrepresented are obtained from the genes in the CREG metabolic network.
Metabolic flux analysis
The glycolytic flux of SMMC-7721 cells was determined by directly measuring the extracellular acidification rate (ECAR) using an XF24 extracellular flux analyzer (Seahorse Bioscience, MA, USA). DMSO- or B[a]P-treated cells were seeded in a XF24 microplate at a density of 35
000 cells per well and incubated overnight. On the day of metabolic flux analysis, the culture medium was replaced with a XF Glycolysis Stress Test Assay Medium and incubated at 37 °C in a non-CO2 incubator for 1 h. The cells were immediately analyzed by a Seahorse XF24 Extracellular Flux Analyzer following injection of compounds: glucose (10 mM), oligomycin (1 μM), and 2-DG (50 mM). ECAR was calculated by the Seahorse XF-24 software. Data are presented as Mean ± SD.
Statistical analysis
For metabolomics results, Welch's two-sample t-test was used to identify biochemicals that differed significantly between B[a]P treatment and control groups. Differences between groups were considered significant (p ≤ 0.05) or approaching significance (0.05 < p < 0.10). For metabolic flux results, Student's t-test was used to examine the statistical significance of differences. p < 0.05 was considered statistically significant.
Results
Metabolomics analysis
We have established a low-dose and long-term B[a]P-exposed model based on human SMMC-7721 cell lines, which is capable of metabolically activating B[a]P.16 To explore the environmentally-relevant toxicity, we used a range of concentrations, which are comparable to the serum B[a]P levels of populations exposed environmentally (≤3.88 ± 2.22 nM),23 and a continuous exposure for 1 month. To investigate the metabolic effects of B[a]P on SMMC-7721 cells, DMSO and B[a]P-treated SMMC-7721 cells were collected for metabolomics analysis by GC/MS and LC/MS/MS. Overall, a total of 316 biochemicals were identified (Table S1†). Of these, 22 (p ≤ 0.05) or 28 (0.05 < p < 0.10) compounds in 0.01 nM groups, 27 (p ≤ 0.05) or 22 (0.05 < p < 0.10) compounds in 1 nM groups, and 21 (p ≤ 0.05) or 22 (0.05 < p < 0.10) compounds in 100 nM groups were significantly altered by B[a]P exposure compared to control groups. In 0.01 and 100 nM groups, most of the significantly altered biochemicals were increased, while in 1 nM groups, B[a]P induced the reduced biochemicals almost as much as the increased biochemicals (Table 1).
Table 1 B[a]P-induced metabolic changes in SMMC-7721 cells
Welch's two-sample t-test |
No. of biochemicals (p ≤ 0.05) total (↑|↓) |
No. of biochemicals (0.05 < p < 0.10) total (↑|↓) |
0.01 nM vs. Ctrl |
22 (18|4) |
28 (20|8) |
1 nM vs. Ctrl |
27 (12|15) |
22 (12|10) |
100 nM vs. Ctrl |
21 (21|0) |
22 (20|2) |
Network analysis
In order to mine for more useful information and eliminate the noise signal, the bioinformatics approaches were performed to predict the metabolic pathways.24 After the exclusion of repeated entries, 104 altered compounds (p < 0.1) were identified, and 70 of them could be mapped to KEGG IDs (Table S2†), which were used to create a Compound-Reaction-Enzyme-Gene (CREG) metabolic network through the Metscape software.25 The CREG graph gives an overview of all components of the B[a]P-induced metabolic network. By integrating the metabolites, reactions, enzymes and genes, the CREG network could provide comprehensive information about B[a]P-induced metabolic responses. Among them, the potential B[a]P-altered genes in the network are shown in Table S3.† In our CREG network, the largest two subnetworks occupy 86% of the full network and contain most differential metabolites (Fig. S1†). The experimental differential metabolites and related metabolic pathways in the two major subnetworks are marked in Fig. 1. To determine the overrepresented Gene Ontology (GO) categories in the B[a]P-induced metabolic network, we used the BiNGO plugin26 to map the predominant functional themes from the genes in the CREG network and visualize them as a Cytoscape graph. The major functional superpathways of GO terms were the amino acid, carbohydrate, and lipid metabolism pathways and the nucleotide metabolism pathway (Fig. 2), suggesting that these metabolic processes were influenced significantly by B[a]P.
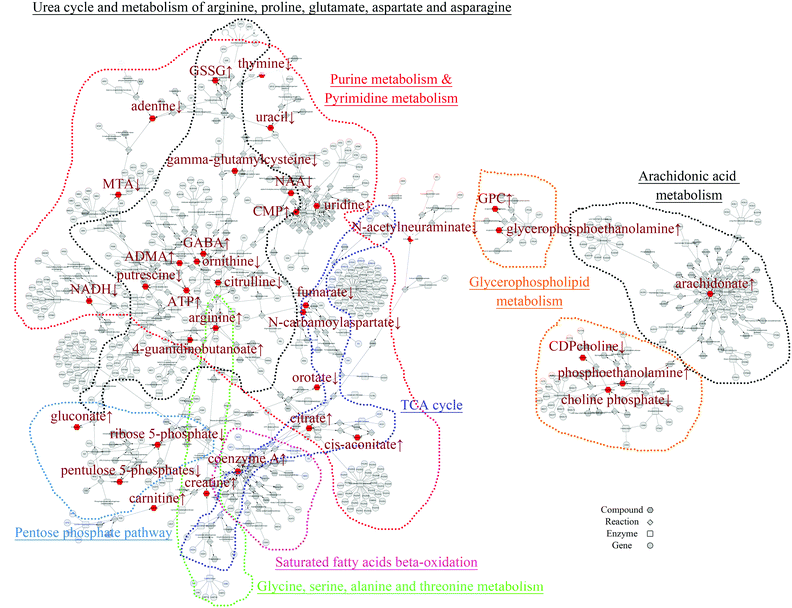 |
| Fig. 1 Two major subnetworks within the full B[a]P-induced CREG metabolic network were visualized in Cytoscape. The input metabolites altered significantly are shown in red and the different metabolic pathways were marked by color traces. | |
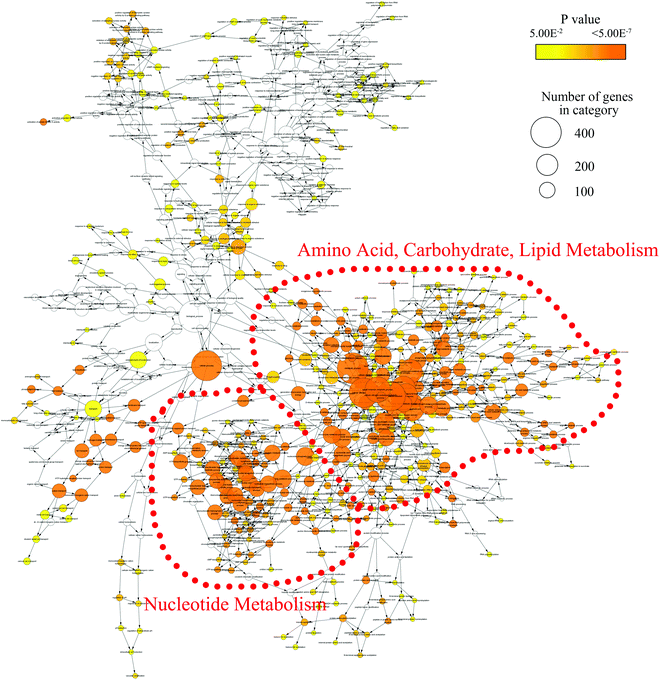 |
| Fig. 2 The network of GO biological processes enriched by the genes from the B[a]P-induced CREG network. The node size shows the number of genes annotated in each GO term and the node color represents the significance of the enrichment. Two functional categories of GO terms are highly connected in the network (red trace). | |
Nucleotide metabolism
The catabolism of purine especially adenosine was enhanced after B[a]P treatment, as the end product of purine breakdown, urate, was accumulated, and the levels of intermediates, such as adenine, hypoxanthine and xanthine, were diminished (Fig. 3A). However, B[a]P treatment elevated the levels of inosine 5′-monophosphate (IMP) at a dosage of 100 nM (Fig. 3A), which is the first nucleotide formed in purine de novo synthesis and indicated that 100 nM of B[a]P enhanced the activity of purine anabolism. Consistently, the levels of purine biosynthesis intermediates, including adenylosuccinate, adenosine 5′-monophosphate (AMP), adenosine 5′-diphosphate (ADP) and guanosine 5′-monophosphate (5′-GMP) were higher in 100 nM B[a]P-treated groups (Fig. 3A). The enhanced purine anabolism also resulted in the increase of adenosine and inosine (Fig. 3A).
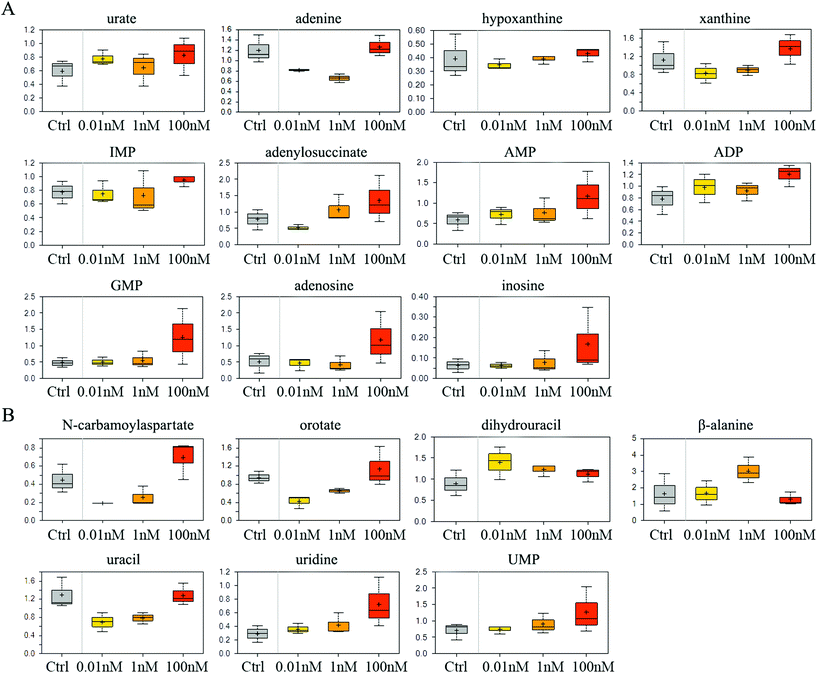 |
| Fig. 3 Effect of B[a]P on purine and pyrimidine metabolism. Levels of metabolites in purine metabolism (A) and pyrimidine metabolism (B) were measured. | |
In the 0.01 and 1 nM groups, the contents of uracil anabolites (carbamoylaspartate, orotate) were reduced (Fig. 3B), and the catabolites of uracil, (dihydrouracil, β-alanine) were increased (Fig. 3B), suggesting that B[a]P treatment inhibited uracil anabolism and promoted uracil catabolism at 0.01 and 1 nM. Also, the level of uracil was reduced in 0.01 and 1 nM B[a]P-treated groups (Fig. 3B), which was partially due to the decreased anabolism and enhanced catabolism, but also because of the increased transformation of uracil into uridine (Fig. 3B). However, with the increase of concentration, the anabolism of uracil enhanced and the catabolism weakened gradually. B[a]P treatment at 100 nM results in elevated levels of carbamoylaspartate and orotate (Fig. 3B). Simultaneously, dihydrouracil and β-alanine were decreased as compared to those in 0.01 and 1 nM treatment groups (Fig. 3B). Overall, B[a]P promoted the synthesis of uridine and uridine monophosphate (UMP) (Fig. 3B).
Carbohydrate and energy metabolism
SMMC-7721 cells exposed to 0.01 and 1 nM of B[a]P exhibited reduced glucose and elevated sorbitol and fructose levels compared to vehicle controls (Fig. 4A), which may suggest a change in glucose uptake and/or utilization. Notably, 0.01 nM of B[a]P resulted in a modest accumulation of the glycolytic intermediates 3-phosphoglycerate and phosphoenolpyruvate (PEP) as well as the end products pyruvate and lactate (Fig. 4B). However, PEP and pyruvate levels were diminished in 1 and 100 nM B[a]P-treated groups, potentially suggesting a concentration-dependent decrease in glycolytic metabolism (Fig. 4B). Besides, B[a]P treatment at the 1 nM dosage resulted in lower levels of multiple pentose phosphate pathway metabolites including 6-phosphogluconate, pentulose 5-phosphates, and ribose 5-phosphate (Fig. 4C).
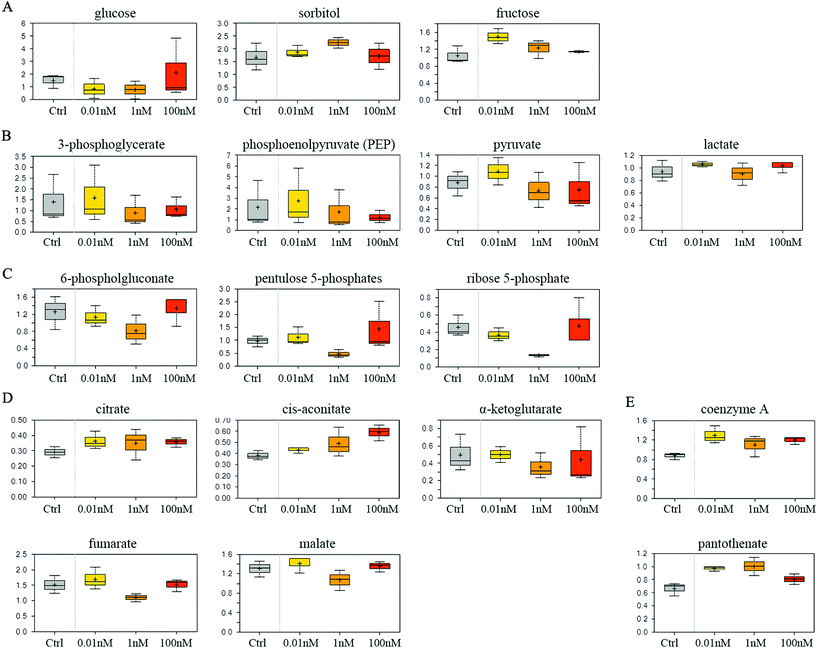 |
| Fig. 4 Effect of B[a]P on carbohydrate and energy metabolism. Levels of monosaccharide and the derivative (A), glycolysis metabolites (B), multiple pentose phosphate pathway metabolites (C), TCA cycle intermediates (D) and mitochondrial metabolism products (E) were measured. | |
Aside from glucose metabolism, B[a]P treatment promoted a selective accumulation of the TCA cycle intermediates, citrate and cis-aconitate. In contrast, α-ketoglutarate (α-KG), fumarate, and malate were modestly reduced at 1 nM, but not at 0.01 or 100 nM dosages (Fig. 4D). Higher levels of citrate may promote lipogenesis. Similarly, elevated coenzyme A and pantothenate levels (Fig. 4E) may also impact mitochondrial metabolism as coenzyme A participates in oxidative metabolism. Altogether, these findings may suggest that B[a]P has significant effects on carbohydrate and energy metabolism.
Arginine metabolism
Compared to vehicle controls, B[a]P-exposed cells exhibited an accumulation of dimethylarginine (Fig. 5A), which is produced from the degradation of methyl arginine containing proteins. Accordingly, multiple peptides such as phenylalanylglutamate and leucylglycine were elevated (Fig. 5A). These results may reflect a change in proteolytic processing and/or protein synthesis. 0.01 and 1 nM B[a]P also induced high levels of creatinine and creatine (Fig. 5A), which are synthesized from glycine, arginine, and methionine, and thus may reflect a change of the arginine utilization to creatine. Consistently, another arginine-related metabolite, ornithine, was diminished in response to B[a]P treatment (Fig. 5A). These changes may consequently restrict the availability of polyamine synthesis (putrescine and spermidine), as suggested by decreased levels of the synthetic by-product, 5-methylthioadenosine (MTA) (Fig. 5A).
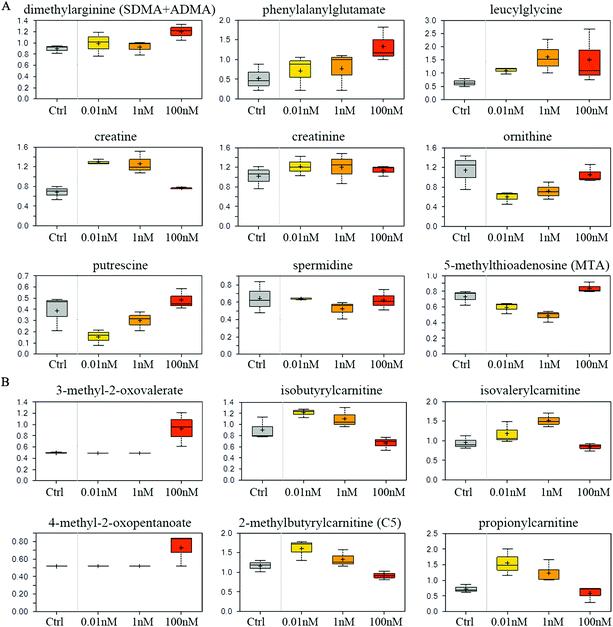 |
| Fig. 5 Effect of B[a]P on amino acid metabolism. Levels of metabolites in arginine metabolism (A) and branched chain amino acid metabolism (B) were measured. | |
Branched chain amino acid metabolism
Although levels of the branched chain amino acids (BCAA) valine, isoleucine, and leucine were similar between different groups (data not shown), 100 nM of B[a]P selectively induced the α-keto acid analogues (3-methyl-2-oxovalerate and 4-methyl-2-oxopentanoate) (Fig. 5B), suggesting a potential disruption in BCAA catabolism. Consistent with this, 100 nM of B[a]P treatment resulted in lower levels of the downstream catabolites (isovalerylcarnitine, isobutyrylcarnitine, and 2-methylbutyrylcarnitine) and the end product (propionylcarnitine) compared to 0.01 and 1 nM B[a]P-treated cells (Fig. 5B). Thus, B[a]P may induce the disruption of BCAA catabolism at 100 nM dosage.
Glycolysis rate
To confirm that a decrease in glucose uptake/utilization in the B[a]P-exposed cells corresponds with a decreased rate of glycolysis, the extracellular acidification rate (ECAR), a surrogate of glycolysis, was measured using a Seahorse XF24 extracellular flux analyzer. The basal ECAR significantly decreased after B[a]P treatment (Fig. 6A), indicating that the glycolysis rate decreased in SMMC-7721 cells after B[a]P exposure. However, after injection of oligomycin, an ATP synthase inhibitor, which shifts the energy production to glycolysis and reveals the cellular maximum glycolytic capacity, the increases of ECAR in B[a]P-exposed SMMC-7721 cells were more sharp as compared to those in the control group (Fig. 6B). These results suggest that long-term B[a]P exposure could confer SMMC-7721 cells with more robust glycolytic capability, which may benefit the cells under harsh conditions in vivo.
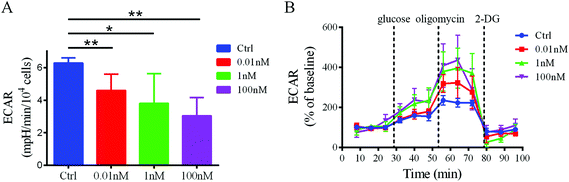 |
| Fig. 6 Effect of B[a]P on ECAR profiles in SMMC-7721 cells. (A) Basal ECAR in cells exposed to B[a]P with different concentrations were shown. (B) Metabolic flux of SMMC-7721 cells was determined with a Seahorse flux analyzer. Addition of the glycolysis activity modulator (glucose, oligomycin, and 2-DG) was indicated. | |
Discussion
In this study, we investigated the potential metabolic effects of long-term B[a]P exposure at environmental doses on human-relevant cells SMMC-7721. Metabolomics study and bioinformatics analysis showed that prolonged B[a]P exposure could cause metabolic perturbation in amino acid, carbohydrate, and lipid metabolism pathways and the nucleotide metabolism pathway. Metabolic flux analysis also showed the influence of B[a]P exposure on glycolysis and the glycolytic capability. Overall, we characterized B[a]P-induced cumulative metabolic effects on SMMC-7721 cells.
Systems biology provides a powerful approach to discover the molecular perturbations of environmental pollutants on biological systems. The metabolism perturbation usually represents the sensitive and common events in pollutant-induced toxicity. Moreover, the metabolic toxicity always acts as a KE to link MIE (gene/protein expression) and the specific adverse outcome (phenotype), thus it is important to depict the metabolic effects of environmental pollutants on the AOP network. Although the carcinogenic and mutagenic toxicities of B[a]P have been studied, the metabolic effects of B[a]P, especially the prolonged exposure at environmental doses, are still not well understood. To characterize the metabolic toxicity of B[a]P, we used a range of doses comparable to the serum B[a]P levels of populations exposed environmentally23 and continuously treated them for 1 month to assess the cumulative toxicological effects.
It is known that B[a]P could form DNA adducts through its metabolite, 7,8-dihydroxy-9,10-epoxy-7,8,9,10-tetrahydrobenzo[a]pyrene (BPDE).27 However, we found that prolonged B[a]P exposure could alter the nucleotide metabolism even at low dosages. This metabolic effect of B[a]P varied with different dosages. Within a concentration range of 0.01 and 100 nM, B[a]P tends to induce stronger activity of nucleotide catabolism at a lower dose, and nucleotide anabolism is likely to be enhanced by a higher dose of B[a]P exposure. How these alterations affect the cumulative effects of B[a]P on SMMC-7721 cells need further investigation. Overall, the ultimate levels of the nucleotide (AMP, GMP, UMP, and CMP) were increased gradually with the concentrations of B[a]P, which may be the cellular adaptive responses to overcome the BPDE-DNA adduct-induced DNA replication stress and damage. This process may lead to errors in DNA replication and introduce gene mutations, thus increasing the risk of cancer.
In addition to DNA damage, the process of B[a]P metabolism also generates reactive oxygen species (ROS) and causes oxidative damage in cells.9 Consistently, in our study, B[a]P treatment elevated the levels of oxidized glutathione (GSSG), which indicates that B[a]P induced a disruption of redox homeostasis and oxidative stress in cells. Furthermore, B[a]P-treated cells also exhibited higher levels of the lipid peroxidation products (13-HODE and 9-HODE) (Table S1†), thus confirming the lipid oxidative damage induced by B[a]P. Continued oxidative stress can damage a variety of organelles and macromolecules, leading to chronic inflammation, and represents an important cancer risk factor.
The reprogramming energy metabolism is another hallmark of cancer.28 In our study, both the metabolomics analysis and metabolic flux detection indicated that prolonged B[a]P exposure decreased the glycolysis rate in SMMC-7721 cells. Interestingly, B[a]P conferred SMMC-7721 cells with more robust glycolytic capability. These results may suggest that long-term B[a]P exposure makes cancer cells more adaptable to a wide variety of environments. Under culture conditions in vitro, which is a sufficient oxygen environment, B[a]P-treated cells show low levels of glycolytic activity, and use more efficient aerobic respiration. However, under some harsh conditions in vivo especially in an oxygen-deficient environment, B[a]P-treated cancer cells, which have accessed a higher glycolytic capacity, could switch to elevated glycolysis and acquire adequate energy. This is consistent with our previous report that B[a]P promoted cancer aggressiveness and progression in SMMC-7721 cells.16
Conclusions
In summary, we have established an overall B[a]P-induced metabolic network, revealed the global influences of long-term and low-dose B[a]P exposure on the metabolism in SMMC-7721 cells, and identified the major altered pathways including amino acid, carbohydrate, and lipid metabolism pathways and the nucleotide metabolism pathway. These metabolic alterations may further contribute to cancer deterioration and offer potential to explore more sensitive biomarkers for the early detection of B[a]P toxicity, thus contributing to a more comprehensive understanding of the toxicity of B[a]P.
Conflict of interests
The authors declare that they have no conflict of interest.
Acknowledgements
This work was supported by grants from the National Natural Science Foundation of China (81573161, 81427805, 91529305, 81302809 and 31401611), the Ministry of Science and Technology of China (2012CB720804), the Key Research Program (KSZD-EW-Z-021 and KSZD-EW-Z-019) and the Instrument Developing Project of the Chinese Academy of Sciences, the Science and Technology Commission of Shanghai Municipality (14391901800), and the Key Laboratory of Food Safety Research of INS, SIBS, CAS.
References
- D. H. Phillips, Nature, 1983, 303, 468–472 CrossRef CAS PubMed.
- S. K. Srivastava, H. Xia, A. Pal, X. Hu, J. Guo and S. V. Singh, Cancer Lett., 2000, 153, 35–39 CrossRef CAS PubMed.
- H. V. Gelboin, Physiol. Rev., 1980, 60, 1107–1166 CAS.
- D. Benford, M. Dinovi and R. W. Setzer, Food Chem. Toxicol., 2010, 48(Suppl. 1), S42–S48 CrossRef CAS PubMed.
- Iarc Working Group on the Evaluation of Carcinogenic Risks to Humans, IARC Monogr. Eval. Carcinog. Risks Hum., 2010, 92, 1–853 Search PubMed.
- K. Srogi, Environ. Chem. Lett., 2007, 5, 169–195 CrossRef CAS.
- D. H. Phillips, Mutat. Res., 1999, 443, 139–147 CAS.
- E. Rivedal and T. Sanner, Cancer Res., 1981, 41, 2950–2953 CAS.
- H. Rubin, Carcinogenesis, 2001, 22, 1903–1930 CrossRef CAS PubMed.
- A. P. Wolterbeek, E. J. Schoevers, A. A. Rutten and V. J. Feron, Cancer Lett., 1995, 89, 107–116 CAS.
- D. R. Davila, D. L. Romero and S. W. Burchiel, Toxicol. Appl. Pharmacol., 1996, 139, 333–341 CrossRef CAS PubMed.
- P. Mendola, S. G. Selevan, S. Gutter and D. Rice, Ment. Retard. Dev. Disabil. Res. Rev., 2002, 8, 188–197 CrossRef PubMed.
- L. Min, S. He, Q. Chen, F. Peng, H. Peng and M. Xie, Toxicol. Mech. Methods, 2011, 21, 374–382 CrossRef CAS PubMed.
- Y. Adeleye, M. Andersen, R. Clewell, M. Davies, M. Dent, S. Edwards, P. Fowler, S. Malcomber, B. Nicol, A. Scott, S. Scott, B. Sun, C. Westmoreland, A. White, Q. Zhang and P. L. Carmichael, Toxicology, 2015, 332, 102–111 CrossRef CAS PubMed.
- S. Gibb, Reprod. Toxicol., 2008, 25, 136–138 CrossRef CAS PubMed.
- Q. Ba, J. Li, C. Huang, H. Qiu, J. Li, R. Chu, W. Zhang, D. Xie, Y. Wu and H. Wang, Environ. Health Perspect., 2015, 123, 246–254 Search PubMed.
- M. Viant and U. Sommer, Metaboliomics, 2013, 9, 144–158 CrossRef CAS.
- T. D. Williams, H. Wu, E. M. Santos, J. Ball, I. Katsiadaki, M. M. Brown, P. Baker, F. Ortega, F. Falciani, J. A. Craft, C. R. Tyler, J. K. Chipman and M. R. Viant, Environ. Sci. Technol., 2009, 43, 6341–6348 CrossRef CAS PubMed.
- B. P. Lankadurai, D. M. Wolfe, A. J. Simpson and M. J. Simpson, Environ. Pollut., 2011, 159, 2845–2851 CrossRef CAS PubMed.
- X. Wang, J. Zhang, Q. Huang, A. Alamdar, M. Tian, L. Liu and H. Shen, Mol. BioSyst., 2015, 11, 753–759 RSC.
- T. Ohta, N. Masutomi, N. Tsutsui, T. Sakairi, M. Mitchell, M. V. Milburn, J. A. Ryals, K. D. Beebe and L. Guo, Toxicol. Pathol., 2009, 37, 521–535 CrossRef CAS PubMed.
- A. M. Evans, C. D. DeHaven, T. Barrett, M. Mitchell and E. Milgram, Anal. Chem., 2009, 81, 6656–6667 CrossRef CAS PubMed.
- M. S. Neal, J. Zhu and W. G. Foster, Reprod. Toxicol., 2008, 25, 100–106 CrossRef CAS PubMed.
- E. C. Butcher, E. L. Berg and E. J. Kunkel, Nat. Biotechnol., 2004, 22, 1253–1259 CrossRef CAS PubMed.
- A. Karnovsky, T. Weymouth, T. Hull, V. G. Tarcea, G. Scardoni, C. Laudanna, M. A. Sartor, K. A. Stringer, H. V. Jagadish, C. Burant, B. Athey and G. S. Omenn, Bioinformatics, 2012, 28, 373–380 CrossRef CAS PubMed.
- S. Maere, K. Heymans and M. Kuiper, Bioinformatics, 2005, 21, 3448–3449 CrossRef CAS PubMed.
- G. Talaska, M. Jaeger, R. Reilman, T. Collins and D. Warshawsky, Proc. Natl. Acad. Sci. U. S. A., 1996, 93, 7789–7793 CrossRef CAS.
- D. Hanahan and R. A. Weinberg, Cell, 2011, 144, 646–674 CrossRef CAS PubMed.
Footnotes |
† Electronic supplementary information (ESI) available. See DOI: 10.1039/c5tx00378d |
‡ These authors contributed equally to this work. |
|
This journal is © The Royal Society of Chemistry 2016 |
Click here to see how this site uses Cookies. View our privacy policy here.