Connecting achievement motivation to performance in general chemistry
Received
30th June 2016
, Accepted 8th August 2016
First published on 10th August 2016
Abstract
Student success in chemistry is inherently tied to motivational and other affective processes. We investigated three distinct constructs tied to motivation: self-efficacy, interest, and effort beliefs. These variables were measured twice over the course of a semester in three sections of a first-semester general chemistry course (n = 170). We explored the connections that exist among these three constructs as well as their connections to course performance. Multiple regression and path analysis revealed that self-efficacy measured during week 12 was the strongest predictor of final course grade followed by situational interest. We also report that personal interest is a significant predictor of future self-efficacy. Our results add to the growing literature on psychological constructs within chemistry education by identifying variables related to motivation that have a significant connection to course performance among chemistry students. We briefly address how these variables could be targeted in the classroom.
Introduction
Achievement motivation is a multi-faceted and complex nexus of interconnected processes. In the context of education, the importance of motivation and other affective processes cannot be overstated. Over the last 50 years in the field of psychology, much work has been dedicated to the understanding of these processes in terms of what drives students' choices and persistence in education (Weiner, 1990). Many theories have been postulated over the years and with them, specific psychological constructs have been defined and operationalized in a myriad of studies. In chemistry education, research has centered on attitudes (Barbera et al., 2008; Bauer, 2008; Xu and Lewis, 2011; Chan and Bauer, 2014, 2015; Galloway et al., 2016; Villafane and Lewis, 2016) and several motivational beliefs and processes including self-efficacy (Smist, 1993; Dalgety, et al., 2003; Zusho et al., 2003; Villafane et al., 2014; Mataka and Kowalske, 2015), interest (Dalgety and Coll, 2006; Nieswandt, 2007; Uzuntiryaki and Aydin, 2009), self-regulation (Black and Deci, 2000), and self-concept (Bauer, 2005; Lewis et al., 2009; Nielsen and Yezierski, 2015).
To better understand academic motivation as a whole, constructs should be measured and studied together with the intent of establishing connections between them (Bathgate et al., 2014). In addition, knowledge of how they evolve over time is valuable for instructors interested in improving the motivational climate of their classrooms. In a prior study, we evaluated the psychometric properties of four scales that measured self-efficacy, personal interest, situational interest, and effort beliefs (Ferrell and Barbera, 2015). In the present study, we extend upon our previous work by investigating the connections between these constructs and how they predict course performance in a first-semester general chemistry course.
Background
Connections among motivational constructs
Self-efficacy, interest, and effort beliefs represent three distinct psychological constructs that can have an effect on motivation. In our previous publication, we provide a detailed description and literature review on each construct (Ferrell and Barbera, 2015). To date, no studies in secondary or post-secondary education have been found that link all three constructs together, either empirically, or theoretically. This is not surprising as effort beliefs research, in particular, is relatively sparse in the literature. However, several empirical studies have included two of the three constructs as measured variables.
Lent et al. (1994) applied social cognitive theory to career development by formulating a model that included self-efficacy, academic interest, choice, and performance. Central to their model was the notion that self-efficacy is a major mediator of choice and development, and guides one's decision-making. The authors reviewed 13 relevant studies and found that of all the correlations with self-efficacy, interest was the strongest (r = 0.53), and performance was moderately correlated (r = 0.38). More recent studies have also corroborated these findings (Smith and Fouad, 1999; Lent et al., 2001; Lent et al., 2008; Larson et al., 2014; Lee et al., 2014). The temporal ordering of self-efficacy and interest has proven more difficult to deconstruct. Lent et al. (2001), along with others (Silvia, 2003; Lent et al., 2008), have found evidence that self-efficacy is a causal precursor to career-related interest. Thus, students' confidence in a particular domain may lead to the development of interest. Others have suggested that self-efficacy and interest are reciprocally related (Nauta et al., 2002), meaning that self-efficacy leads to interest just as much as interest leads to self-efficacy. Still others have produced viable models of interest and self-efficacy that are temporally equivalent with both being caused by variables not included in the model (Lee et al., 2014). In any case, these studies highlight the salience of considering interest and self-efficacy in achievement motivation models, as well as career and college major choice models.
The empirical research on effort beliefs remains sparse, and as a result, there is little evidence that addresses connections with other motivational constructs. Nevertheless, a few studies do exist that have measured self-efficacy or interest in combination with effort beliefs (Abdullah, 2008; Jones et al., 2012). Abdullah (2008) found a strong, positive correlation (r = 0.51) between positive effort beliefs and self-efficacy with a sample of students. This is consistent with Bandura's (1997) prevailing theoretical model, which suggests people with high self-efficacy tend to display more effort and persist longer with tasks than those with low self-efficacy. Although a display of effort is not equivalent to believing that effort will lead to positive outcomes, we argue that positive effort beliefs toward a task likely precede the exertion of effort. The relationship between interest and effort beliefs was investigated in one study on ninth grade math students (Jones et al., 2012). Although the path models tested in the study included no significant causal relationship between interest and effort beliefs, data was presented demonstrating a moderate, positive correlation between effort beliefs and interest as well as a significant covariance among the residual terms.
Academic achievement and self-efficacy
Self-efficacy is the most widely studied of these constructs in terms of the link with academic achievement. Self-efficacy has been shown to consistently display a positive relationship with academic performance (Multon et al., 1991; Lightsey, 1999; Robbins et al., 2004). Regardless of ability level, researchers have found that students who report high self-efficacy tend to outperform their peers who report low self-efficacy (Bouffard-Bouchard et al., 1991; Collins, 1982 as cited in Bandura, 1997). Students at the college level are no exception. The positive correlation between self-efficacy and academic performance has been observed repeatedly in college courses (Siegel et al., 1985; Lent et al., 1986; Pajares and Miller, 1994, 1995; Pajares and Kranzler, 1995; Zusho et al., 2003). Multon et al. (1991) conducted a meta-analysis on studies that measured college students' self-efficacy. They reported an average correlation of 0.38 between self-efficacy and academic performance, and that self-efficacy accounted for 14% and 12% of the observed variance in academic performance and persistence, respectively. A subsequent meta-analysis conducted by Robbins et al. (2004) surveyed 109 studies where various psychosocial and study skills variables were compared with academic performance. The two strongest psychosocial predictors of college GPA were self-efficacy and achievement motivation (ρs = 0.496 and 0.303, respectively). Furthermore, due to the non-compulsory nature of college, many studies investigating self-efficacy among college-age students have examined the predictive power of self-efficacy on persistence (Lent et al., 1984; Hull-Blanks et al., 2005; Vuong et al., 2010). Wright et al. (2012) sampled 401 first-year undergraduates and found that course self-efficacy measured at the end of the semester was a significant predictor of persistence to enroll in the second semester. This effect was found after controlling for relevant variables such as gender, high school GPA, and ethnicity.
Academic achievement and interest
Although there has been less research linking interest to academic achievement, a few notable studies should be mentioned. It is important to highlight that interest can be conceptualized as having both trait (personal interest) and state (situational interest) aspects (Hidi and Renninger, 2006; Linnenbrink-Garcia et al., 2013). The following studies tested the connection between situational interest and course performance. Harackiewicz et al. (2000) investigated the short and long-term effects of college students' goal orientations, interest, and performance in an introductory psychology class. The authors utilized path modeling to test the causal ordering of the tested variables. Their results showed that in the short-term (one semester), students who reported higher situational interest received higher grades in the course. Although this effect was significant, the authors point out that students had already received feedback from two exams prior to the measurement of their interest. Hence, the notion that level of interest accounted for the performance level could be muddled by the timing of the measurements. In a related study, Hulleman and colleagues (2008) found that the utility value component of interest (i.e., importance for future), but not the intrinsic component (i.e., enjoyment of class), significantly predicted academic performance in college psychology. In this study, interest was measured prior to any exams, eliminating the problem of timing for causal ordering.
Academic achievement and effort beliefs
Very little research has been published with effort beliefs as a measured variable. Among those studies, no direct link between effort beliefs and academic performance has been established. Instead, Blackwell et al. (2007), in a study on sixth and seventh graders, identified positive effort beliefs as an important mediator between incremental theory of intelligence (Dweck, 2012) and positive learning strategies, which predicted grades. In a subsequent study, Jones et al. (2012) sought to replicate the model proposed by Blackwell et al. (2007) with ninth grade math students. They also found that positive effort beliefs mediated the relationship between an incremental theory of intelligence and positive learning strategies, which were predictive of current grades. Although limited in scope, the findings of these studies expand upon the importance of considering effort beliefs as a mediator between implicit theories and adaptive learning patterns that lead to higher achievement (Tempelaar et al., 2015).
Factors linked to achievement in chemistry
Prior studies in chemistry have demonstrated that cognitive variables such as spatial skills (Carter et al., 1987), math ability (Spencer, 1996; Lewis and Lewis, 2007), and prior conceptual knowledge (Xu et al., 2013) are linked to achievement. For example, Lewis and Lewis (2007) were interested in predicting at-risk students in general chemistry. They found that a significant amount of the variance in students' American Chemical Society (ACS) final exam scores was accounted for by their SAT math scores. This suggests that students' math ability plays an important role in their success in general chemistry. In a related study, House (1995) identified that students' beliefs about their math ability was the strongest predictor of achievement in a college introductory chemistry course, even when the American College Testing (ACT) composite exam scores were considered. While cognitive abilities are an integral part of academic performance in chemistry, one cannot ignore the underlying non-cognitive beliefs and processes. Several non-cognitive factors such as self-efficacy (Zusho et al., 2003), attitude (Xu et al., 2013), self-concept (Lewis et al., 2009), and utility value (Gonzalez and Paoloni, 2015b) have been correlated with achievement in chemistry courses. For example, Zusho et al. (2003), in a study with college chemistry students, found that self-efficacy accounted for the most variance in course performance, even after controlling for prior achievement. These studies underscore the fact that non-cognitive beliefs and processes are vital to student success in chemistry.
Present study
Our aim in this study was to explore the possible relationships that exist between self-efficacy, effort beliefs, personal interest, and situational interest as well as their connection to final grades in an introductory chemistry course. Toward this end, we used multiple regression and path analysis to test for plausible models that best represent these relationships. Previously, we adapted and modified four scales intended to measure these motivation-related variables in a chemistry setting (Ferrell and Barbera, 2015). We demonstrated that all four scales produced sufficient evidence for validity and reliability with both initial and cross-validation samples of first-semester general chemistry students, a necessary precursor to any further studies (Barbera and VandenPlas, 2011; Arjoon et al., 2013; Brandriet et al., 2013). Our data collection and subsequent analyses for the present study were guided by the following two research questions:
(1) What are the connections among self-efficacy, interest, and effort beliefs with general chemistry students?
(2) To what extent do self-efficacy, interest, and/or effort beliefs predict course performance in general chemistry?
We hypothesized that self-efficacy would be the best predictor of course performance in line with what others have reported (Pajares and Miller, 1995; Bong, 2001). In addition, we hypothesized that personal interest would predict situational interest, a relationship that has been theoretically established and tested in several studies (Hidi and Renninger, 2006; Nieswandt, 2007; Harackiewicz et al., 2008), and these would be related to course performance. Based on the lack of research on effort beliefs in college settings, we did not have specific hypotheses regarding these factors. We believe the results from this study will provide valuable insight for instructors interested in measuring the motivational climate of their classrooms as well as those who want to expand the evidence of impacts related to curriculum changes.
Methods
Participants
Participants for this study were recruited from three sections of a first-semester general chemistry course at a mid-sized Rocky Mountain region university during the fall of 2014. This is a required course for several science and health-related majors, and is the first in a two-semester sequence of general chemistry.
Course overview
Two different instructors taught the three different class sections of first-semester general chemistry during this term. Instructor A taught two sections and instructor B taught one section. These instructors were not only selected based on their willingness to participate in the study, but also on their backgrounds and similarities in course structure and teaching style. Each instructor had over five years experience teaching within the general chemistry sequence and had been recognized for their excellence in teaching the course. The instructors worked together, meeting regularly over the semester, to coordinate on a number of aspects for the courses. In addition to using the same textbook and homework system, the instructors agreed on the timing of assessments and weight percentages of assignments. Each course had an equal number of online homework assignments, weekly quizzes, and hour exams. While the content coverage of each was similar, based on the pacing of each instructor, they did not use matched homework or assessment items for security reasons. Both instructors administered an American Chemical Society examination for their final exam. Lecture was the main teaching style for all courses. While both instructors supplemented lectures with brief activities, problem solving tasks, and virtual or physical demonstrations, neither would categorize their style as active learning.
Measures
Interest scales.
To assess interest at two time points during the semester, personal and situational interest scales were needed. Both scales were originally developed by Harackiewicz et al. (2008) and very similar items were used in a subsequent study by Linnenbrink-Garcia et al. (2010). We adapted the items from both scales for use in college-level chemistry and found the psychometric properties of each to be acceptable (Ferrell and Barbera, 2015). The personal interest scale is comprised of seven items, three of which are feeling-related items, and the remaining four are value-related. The situational interest scale is comprised of eight items, with an equal split of feeling-related and value-related items. The personal interest scale is designed to measure the interest that students already have towards chemistry prior to taking the course. The situational interest scale, on the other hand, is comprised of items that assess interest aimed at students' feelings and value toward the material covered at the course level (Linnenbrink-Garcia et al., 2013). Both scales are measured using a five-point Likert scale ranging from “strongly disagree” to “strongly agree”.
Self-efficacy.
To measure self-efficacy, select items from the College Chemistry Self-Efficacy Scale were used (CCSS; Uzuntiryaki and Aydin, 2009), all of which were previously tested for adequate psychometric properties. We originally tested a scale that consisted of eight items, two of which were removed due to poor model fit (Ferrell and Barbera, 2015). The scale used in the present study consists of six items that ask students to rate how well they could complete tasks that would generally be encountered in an introductory chemistry course. The items are measured on a five-point Likert-type scale ranging from “very poorly” to “very well”.
Effort beliefs.
To measure students' effort beliefs about chemistry, we adapted a scale for chemistry originally developed by Sorich and Dweck (1997), subsequently published in Blackwell's (2002) dissertation, and used in studies by Blackwell et al. (2007) and Jones et al. (2012). The original scale consisted of nine items, however, our previous study produced evidence suggesting that the scale functioned better if three of the items were removed (Ferrell and Barbera, 2015). Hence, in the present study, we used the six-item scale measured with a five-point Likert scale ranging from “strongly disagree” to “strongly agree”.
Academic performance.
Although there are many ways to measure academic performance in a college classroom, course grade is the most common. We chose to use course grade percentage because it represents the final outcome of the course and is the highest stake for students. Final course grade percentages were based on content measures only including homework, quizzes, mid-term exams, and the ACS final exam. No points were awarded for participation or attendance, and the co-requisite lab was a separate grade entirely. Students with incomplete grades were not included in the analyses.
Data collection
Data for this study were collected at two time points during the lecture period of each section. Time 1 (T1) data were collected during the first week of the semester and time 2 (T2) data were collected during the 12th week of the semester. On the days when data were collected, the researcher made an announcement informing students that their participation was voluntary and that their identities would be kept completely confidential. The students were then provided the survey packet along with a consent form that was approved by the Institutional Review Board. Students were given approximately 10 minutes to complete all of the scales (19 items at time 1 and 20 items at time 2) and seven demographic items (only given at time 1). Due to the nature of this study, only students who completed all items at both time points were included in subsequent analyses.
Data analysis
Descriptives.
Descriptive statistics including mean, standard deviation, skew and kurtosis values were determined for all scales at both time points. We considered acceptable skew and kurtosis values to be −1 to +1 (Huck, 2012). Statistical Package for the Social Sciences (SPSS) 20.0 software was used for these analyses.
Multiple regression.
Multiple regression analysis is a method used to predict or explain the variation in a dependent variable by examining its relations to several independent variables (Pedhazur, 1997). By utilizing multiple regression analyses, the researcher is able to identify and separate the individual effects of distinct independent variables on a dependent variable. Multiple regression analysis was employed in this study to examine to what extent self-efficacy, interest, and effort beliefs predict final grades. All necessary assumptions were examined including univariate normality, linearity, homoscedasticity, and normal distribution of residuals. In addition, multicollinearity was checked among all of the independent variables.
Path analysis.
Path analysis is similar to multiple regression, but allows the researcher to estimate the effects of independent variables on each other as well as on the dependent variable and the causal directions associated with these effects (Pedhazur, 1997). A related technique, structural equation modeling is often used to specify a full model with latent and observed variables along with a path diagram representing the interconnections between the variables (Xu et al., 2013; Gonzalez and Paoloni, 2015b). However, path analysis has no latent variables and generates a path diagram with path coefficients based solely on observed variables with only one indicator (Raykov and Marcoulides, 2000). The observed variables that only have outgoing paths are referred to as exogenous variables, with the variance in these being explained by factors not included in the model. In addition, they are assumed to be measured without error. Although measurement error cannot ever be fully eliminated, the measures used here have been well supported with evidence of validity and reliability (Ferrell and Barbera, 2015). The paths which have at least one incoming path are referred to as endogenous variables. The variance of endogenous variables is assumed to be explained by only the other variables included in the model, plus a disturbance term, which is analogous to an error term (Streiner, 2005). One of the advantages of using path analysis versus multiple regression is the ability to test how well competing models fit a given data set. To do this, each model is examined for overall fit. There are a myriad of fit indices that researchers use to support the fit of a model, but we will use only the most common for path analysis. Global model fit was tested using the χ2 test for goodness-of-fit, in which the desired result is to retain the null hypothesis (Streiner, 2006). While this is a good indicator of how well a model fits the data, it can be influenced by large sample sizes, which often leads the researcher to reject the null hypothesis (Schermelleh-Engel et al., 2003). Thus, other fit indices, which are less influenced by the size of the sample, are often used to further evaluate model fit. We chose to use three of these, Root Mean Squared Error of Approximation (RMSEA) (Steiger, 1990), Goodness-of-Fit Index (GFI), and the Comparative Fit Index (CFI) (Bentler, 1990). We describe the CFI and RMSEA in detail in a separate publication (Ferrell and Barbera, 2015). Briefly, the acceptable values for RMSEA and CFI are <0.08 and >0.95, respectively (Browne and Cudeck, 1992; Hu and Bentler, 1999). The GFI is an absolute fit index that compares the amount of variance and covariance explained by the hypothesized model to no model at all (Byrne, 2013). GFI values >0.85 are considered indicative of acceptable fit, but >0.90 indicates good fit (Schermelleh-Engel et al., 2003). To generate our path diagrams, a set of a priori path models were tested using LISREL 8.80 (Jöreskog and Sörbom, 2006) with the Maximum Likelihood (ML) estimator.
Results
Demographics
During the first week of classes, 299 participants (91% of enrolled students) turned in survey packets. Of these, 170 had complete data sets at the end of the semester, resulting in approximately 43% attrition from the study. The reasons for this attrition were missing data due to absences, withdrawal from the course, or lack of consent. Participants completed a demographic survey only at T1. Of the 170 participants included in the study, 73.5% were female, and 92.9% had taken high school chemistry. Most of the students (79.3%) were at the freshman or sophomore level and 81.7% reported this class as being their first chemistry class in college. With regard to race/ethnicity, 73.5% were Caucasian, 17.1% were Hispanic, 4.1% were African American, 1.2% were Asian American, and 4.1% were self-identified as Other. The breakdown of majors was as follows: 14% chemistry, 23% other science (biology, physics, mathematics), and 63% were non-science (nursing, sports and exercise science, other).
Data screening and descriptive statistics
All data sets were screened for careless responses (e.g., selection of the same option for every item) and none were found. Descriptive statistics and internal consistency estimates (Cronbach's alpha) for the students' scores are reported in Table 1. A final combined sample size of 170 was analyzed from all three sections of the course. The skew and kurtosis values were within the range of −1 to +1, with the exception of the value-related personal interest scale. As a result, the inferences drawn from the scores from this scale should be interpreted with caution as multiple regression and maximum likelihood estimation are not robust to univariate nonnormality (Curran et al., 1996; Osborne and Waters, 2002). The internal consistency analysis for each scale reveals that Cronbach's alpha (α) values are acceptable (>0.70), with the exception of the effort beliefs scores at T1 (α = 0.68). This borderline internal consistency value was taken into consideration when interpreting the results below. The descriptive statistics reported here are comparable to those reported in our previous study using these scales, suggesting consistency in the measurement (Ferrell and Barbera, 2015).
Table 1 Descriptive statistics for each measured scale (n = 170)
Scale (time) |
Mean |
SD |
Skewness |
Kurtosis |
Cronbach's α |
Note: PI – personal interest, EB – effort beliefs, SE – self-efficacy, SI – situational interest. |
PI-feel (T1) |
3.25 |
0.90 |
−0.30 |
−0.20 |
0.92 |
PI-value (T1) |
3.87 |
1.00 |
−1.61 |
2.68 |
0.89 |
EB (T1) |
4.06 |
0.52 |
−0.26 |
−0.54 |
0.68 |
SE (T1) |
2.90 |
0.71 |
−0.28 |
0.19 |
0.88 |
SI-feel (T2) |
3.25 |
0.97 |
−0.39 |
−0.30 |
0.94 |
SI-value (T2) |
3.51 |
0.84 |
−0.36 |
−0.03 |
0.86 |
EB (T2) |
3.94 |
0.68 |
−0.75 |
0.43 |
0.83 |
SE (T2) |
3.72 |
0.60 |
−0.50 |
0.36 |
0.85 |
Final% |
75.81 |
12.68 |
0.25 |
−0.54 |
— |
Multiple regression
Multiple regression analysis was conducted to determine the effects of personal interest, situational interest, effort beliefs, and self-efficacy on final course grade. Correlations between all mean scale scores and final course grade are reported in Table 2. Tests for the assumptions of homoscedasticity, linearity, and normal distribution of residuals were conducted and met. The level of multicollinearity was checked and found to be acceptable based on low variance inflation factors (VIFs) (Pedhazur, 1997).
Table 2 Pearson correlations of all scales and final course grade
Scale |
PI-feel |
PI-value |
EB T1 |
SE T1 |
SI-feel |
SI-value |
EB T2 |
SE T2 |
Fpct |
Note: PI – personal interest, EB – effort beliefs, SE – self-efficacy, SI – situational interest, Fpct – final course percent. * Significant at p < 0.05. |
PI-feel |
— |
|
|
|
|
|
|
|
|
PI-value |
0.668* |
— |
|
|
|
|
|
|
|
EB T1 |
0.409* |
0.589* |
— |
|
|
|
|
|
|
SE T1 |
0.319* |
0.184* |
0.059 |
— |
|
|
|
|
|
SI-feel |
0.503* |
0.197* |
0.214* |
0.243* |
— |
|
|
|
|
SI-value |
0.361* |
0.188* |
0.185* |
0.195* |
0.662* |
— |
|
|
|
EB T2 |
0.177* |
0.111 |
0.423* |
0.075 |
0.568* |
0.486* |
— |
|
|
SE T2 |
0.322* |
0.218* |
0.298* |
0.400* |
0.541* |
0.474* |
0.495* |
— |
|
Fpct |
0.151* |
0.120 |
0.102 |
0.228* |
0.392* |
0.231* |
0.312* |
0.536* |
— |
Final course grade was regressed on self-efficacy, effort beliefs, and both feeling and value-related components for interest (personal for T1 and situational for T2) at both time points. We used a hierarchical regression method to test the incremental effects of T2 variables over the T1 variables in the model. The first model was significant (F(4,165) = 2.741, p = 0.030, R2 = 0.062, R2 adjusted = 0.040) with only one significant main effect, self-efficacy T1 (β = 0.205, p = 0.011). The second model was significant as well (F(8,161) = 10.074, p < 0.001, R2 = 0.334, R2 adjusted = 0.300, R2 change = 0.271, p < 0.001). Only situational interest feel (β = 0.277, p = 0.011) and self-efficacy T2 (β = 0.477, p < 0.001) were significant main effects in the second model. None of the T1 variables accounted for a significant amount of variance in final course grade when the T2 variables were introduced into the model. This data suggests that variables measured later in the semester account for significantly more variance in final course grade than T1 variables, even after the variance from T1 variables were accounted for.
Path analyses
Path analysis was used to test a set of path models, which describe a network of relationships simultaneously. Path analysis extends beyond multiple regression by allowing for relationships among predictor variables to be tested as well as allowing for defined constraints on the model. As with the multiple regression, all models were tested with the entire data set (n = 170).
To generate the most parsimonious model, the first set of relationships tested in model 1, effort beliefs and value-related interest components were excluded (see Fig. 1). These variables were left out of the path analysis for two reasons. First, they were not shown to be significant predictors of course grade in the multiple regression analysis. Secondly, we had no theoretical basis for assigning a causal path between effort beliefs or the value-related interest components and any of the other constructs tested. Thus, only self-efficacy, feeling-related personal and situational interest, and course grade were included in model 1.
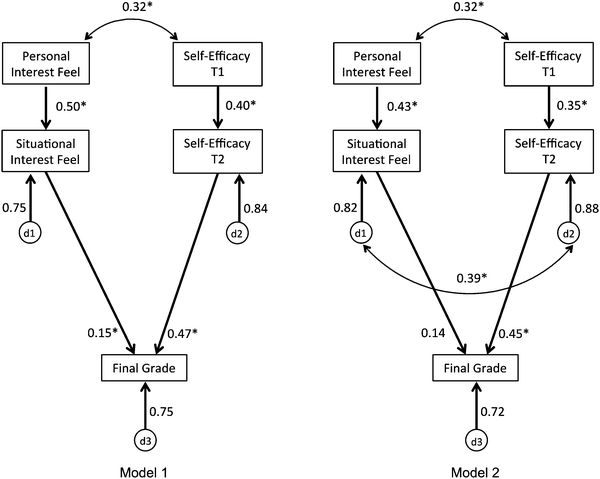 |
| Fig. 1 Path diagram of models 1 and 2. Standardized path coefficients are reported. * Significant at p < 0.05. | |
The results showed that model 1 was not a good fit to the data. Only the GFI (0.91) was within an acceptable range (see Table 3). The R2 value for model 1 indicates that 25% of the variance in course grade can be explained by the way students responded to the scales as outlined by the model.
Table 3 Fit statistics for each model tested, bold indicates value within acceptable range for the type of statistic
Model |
χ
2 (df) |
p-Value |
RMSEA |
CFI |
GFI |
R
2
|
R
2 reported as variance accounted for in final grade.
|
1 |
44.25 (5) |
<0.001 |
0.22 |
0.81 |
0.91
|
0.25 |
2 |
13.58 (4) |
<0.01 |
0.12 |
0.96
|
0.97
|
0.28 |
3 |
10.46 (3) |
0.02 |
0.12 |
0.97
|
0.98
|
0.29 |
4 |
3.59 (3) |
0.31
|
0.03
|
0.99
|
0.99
|
0.30 |
To explore the possibility of model misspecification, we examined the modification indices (MIs). Modification indices are equivalent to a one degree of freedom χ2 statistic that can only be applied to parameters which are fixed. The MIs provide an estimate of how much the overall χ2 statistic will drop when the parameter in question is freely estimated (Byrne, 2013). The MIs for model 1 indicated that correlating the disturbance terms of self-efficacy T2 and situational interest feel would improve the overall fit of the model. Disturbance terms are analogous to error terms in multiple regression and structural equation modeling. They are estimates of the variance not accounted for by the incoming path or paths to a given term. By allowing the correlations of the disturbance terms to be freely estimated, we utilized a method known as correlated uniqueness (CU) (Kenny, 1979). Although somewhat contentious in the literature, CU allows the researcher to account for common method variance that can be particularly problematic when using the same survey and scale type to measure multiple variables (Marsh and Bailey, 1991; Podsakoff et al., 2003). When we re-specified the model to include this change (model 2, Fig. 1), the fit improved dramatically over model 1 (see Table 3). Nevertheless, the χ2 goodness-of-fit test was still significant and the RMSEA value was not within an acceptable range.
In addition to the links between pre/post scores of the same construct, we were interested in testing the predictive relationships between self-efficacy and interest. Toward this end, we tested competing models – the first of which had personal interest feel predicting self-efficacy T2 (model 4, Fig. 2) and the second had self-efficacy T1 predicting situational interest feel (model 3, Fig. 2). Models 3 and 4 are identical to model 2 (Fig. 1) with the exception of their respective added paths between interest and self-efficacy. Both of these relationships are supported by the literature, in addition to a reciprocal relationship between self-efficacy and interest, as explained above. However, in the context of chemistry, we are unaware of any evidence that supports either hypothesis, therefore, both paths need to be tested.
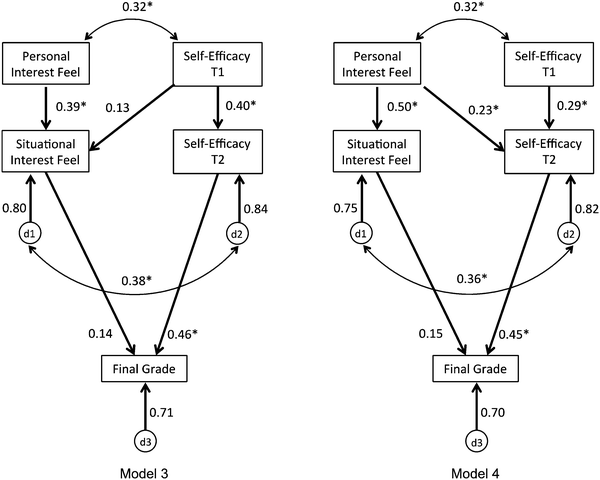 |
| Fig. 2 Path diagram of models 3 and 4. Standardized path coefficients are reported. * Significant at p < 0.05. | |
A path from self-efficacy T1 to situational interest feel was added in model 3 (Fig. 2). There was a modest improvement in model fit over model 2 (see Table 3), but the path coefficient (β = 0.13) between self-efficacy T1 and situational interest feel was not significant at p < 0.05. This suggests that, on average, self-efficacy scores at the start of the semester do not predict future levels of feeling-related situational interest in chemistry. All other significant paths from model 2 were similar in direction and magnitude in model 3.
When a path was added from personal interest feel to self-efficacy T2 in model 4 (Fig. 2), there was a dramatic improvement in fit over model 3 (see Table 3), but unlike in model 3, the path coefficient (β = 0.23) between personal interest feel and self-efficacy T2 was significant (p < 0.05). This finding provides evidence that, on average, interest in chemistry at the start of a course is an important factor in predicting students' self-efficacy at the end of the course. The indirect effects of self-efficacy T1 and personal interest feel on course grade were also tested in model 4, but were not found to be significant (p < 0.05).
Discussion
In this study, we explored the connections between self-efficacy, personal interest, situational interest, and effort beliefs as well as their impact on course grade in a first-semester general chemistry course. Participants were evaluated on these measures at two time points (week 1 and week 12). This work builds on our prior study (Ferrell and Barbera, 2015) by investigating the temporal links and interconnections, via path analysis, that exist between these motivational variables. In addition, we analyzed the extent to which students' grades were predicted by the path models tested. In our best-fitting model (model 4, Fig. 2), we found that T1 variables were good predictors of their T2 counterparts and that the feeling-related component of personal interest was a good predictor of self-efficacy T2. It is important to note here that self-efficacy T2 is a strong predictor of course grade (β = 0.45) and the correlation between personal interest feel and course grade is significant (r = 0.15). Hence, there could be a mediating effect of self-efficacy T2 on the relationship between personal interest feel and course grade. Additionally, 30% of the total variance in course grade was accounted for by the variables included in model 4. By squaring the path coefficient (β = 0.45), we see that the strongest predictor of course grade was self-efficacy at T2 that accounts for 20.3% of the total variance explained in the model.
Multiple regression analysis
To begin, we regressed final course grade on all measured variables at both time points. Based on prior research, we expected self-efficacy and interest to be potential predictors of course grade (Smist, 1993; Harackiewicz et al., 2000; Zusho et al., 2003; Gore, 2006). Our results are concurrent with this, suggesting that self-efficacy, measured at the end of the semester, is the strongest predictor of course grade. Feeling-related situational interest also accounts for a significant portion of the variance in course grade in some of our models. These results were obtained even when personal interest feel and T1 self-efficacy were accounted for in a hierarchical regression model. Effort beliefs at either time point was found to not be a significant predictor of course grade in our regression model. This is in line with what has been previously reported (Blackwell et al., 2007; Jones et al., 2012). However, effort beliefs T2 did exhibit strong correlations with all other T2 variables (see Table 2), demonstrating a clear relationship with distinct, motivation-related constructs. To further understand effort beliefs as a psychological construct, we are conducting a qualitative investigation among general chemistry students that will be reported at a later date.
It is important to note here that the timing of measurement should be a central consideration when assessing students' motivation. At the start of the semester, self-efficacy T1 was a very weak predictor of final course grade and personal interest was not a significant predictor. By the end of the semester, self-efficacy T2 and situational interest feel accounted for a much larger portion of the variance than the T1 variables. The predictive effect of self-efficacy measured in the middle of the semester, versus the beginning, on performance has been observed consistently by others (Bong, 2001; Gore, 2006; Lee et al., 2014). We postulate this could be due to two reasons. First, students are not well calibrated in their confidence when they walk in the door on the first day of chemistry. Hence, they may under or over-estimate their capability to complete certain tasks they will encounter in the course. Others have reported evidence of high school students' overconfidence in math, but suggested that college students would likely have better calibration (Pajares and Kranzler, 1995). Although most of the students in this study had chemistry in high school, some did not, which would further complicate the issue of calibration because of the lack of knowledge, skill, and prior attainments (Gist and Mitchell, 1992; Pajares, 1996). By the end of the semester, students had more recent experience with the tasks included in the self-efficacy scale and were better able to self-appraise their abilities (Wright et al., 2012). Bandura (1986) also notes that self-appraisals of ability should improve with time. Secondly, students, by the end of the semester, had considerably more performance feedback to draw upon and with which to match their appraisals. Thus, it is likely that their confidence would better line up with their actual performance thereby improving the correlation between self-efficacy and final course grade. To begin accounting for this, we suggest that future classroom studies consider collecting a set of data after students have had some graded performance feedback. This can then be compared to data collected at the end of a course to get a better gauge of the impacts of the classroom environment. Additionally, we encourage future qualitative studies of the reported reasons for students' change on these variables and which aspects of the environment (if any) are reflected on as the nature of the change.
Path analysis
We were not only interested in total variance explained, but also the predictive power of the independent variables in our model. Our first model (Fig. 1) included self-efficacy and situational interest feel as endogenous T2 variables predicting final course grade. The T2 variables were preceded by their respective T1 variables (personal interest feel and self-efficacy). Value-related interest and effort beliefs were removed from the initial model (model 1) because neither variable was a significant predictor of course grade in the multiple regression analysis. Model 1 demonstrated poor fit to the data, but when we allowed the disturbance terms of situational interest feel and self-efficacy T2 to be freely estimated, the model fit improved (model 2). Correlated disturbances (or residuals) represent shared variance between two variables included in a model and some cause outside the model (Landis et al., 2009). Often modification indices will be generated that estimate improved model fit should certain residuals be allowed to correlate. It should be noted, however, that modification indices are not a substitute for substantive theoretical backing. Rather, they can be viewed as suggestions that should be interpreted in light of prior research and accepted theory. Despite this adjustment, the model was still a poor fit to the data. It was only when we explored the connection between self-efficacy and interest that the model fit improved significantly.
Feeling-related aspects of personal interest were determined to be a significant predictor of self-efficacy at T2. We noted above the mixed results obtained from others (Nauta et al., 2002; Lent et al., 2008) on the causal link between self-efficacy and interest. We tested both causal directions – the first, self-efficacy predicting situational interest feel, the second, personal interest feel predicting T2 self-efficacy. Our results revealed that personal interest was a better predictor of future self-efficacy than self-efficacy predicting future interest. Put simply, students who come into chemistry with a fascination or positive feelings toward chemistry will tend to leave the course with more confidence than those who do not. However, as Nauta et al. (2002) found, the directionality could change with subsequent measurements of interest and self-efficacy. Thus, we do not suggest our results are in conflict with what has been reported previously, but rather a snapshot of what is certainly a larger motivational landscape.
Implications for research and instruction
There are several implications of this study with respect to chemical education research and instruction. While this study did not include designed attempts to positively impact the measured variables, our study highlights the salience of considering affective and motivational processes in the classroom as influencing factors of course performance. An instructor cannot control what beliefs students' hold when they enter the classroom, but our results suggest that instructors could target interest and self-efficacy in their teaching strategies, which could impact course performance. Bandura (1986) argues that of the sources of self-efficacy, authentic mastery experiences are the most important. Obviously, all instructors hope their students achieve these experiences, but perhaps designing a curriculum or series of interventions around this idea would promote students' confidence, leading them to more adaptive motivational processes and ultimately, to success (Bandura, 1997; Zusho et al., 2003).
Self-efficacy is not the only target variable our model suggests addressing for enhanced course performance. While individual interest is not usually associated with performance in education, some of our results suggest that students with higher levels of feeling-related situational interest by the end of the semester performed better than those with lower levels of interest. Several interventions and teaching styles have been developed with the aim of impacting students' interest (Häussler and Hoffmann, 2002; Hulleman and Harackiewicz, 2009; Hulleman et al., 2010). Hulleman et al. (2010) incorporated a brief intervention in a college psychology class whereby students in the treatment group were asked to write a short essay about the relevance of a topic being covered to a significant person in their lives. This short assignment resulted in a significant effect, both on increased interest in the course and on their final grades. Furthermore, the effect was most pronounced for students with low expectancies for success in the course, who are likely most at-risk for failure. Social-psychological interventions such as these should not be written off as hand waving or magical. There are substantial effects that have been observed for very simple, but carefully planned interventions. Yeager and Walton (2011) have this to say about social-psychological interventions,
“Brief exercises that do not teach academic content but instead target students’ thoughts, feelings, and beliefs in and about school – have had striking effects on educational achievement over months and years.”
Limitations of study
Our study has several limitations that should be made known. First, our sample was derived from one institution during one semester and did not include all sections of the course during that semester. Also, of the original sample, little more than half of the participants provided all of the necessary criteria for inclusion in the final analyses. Finally, those who were included were primarily female and Caucasian. Thus, the generalizability of our results to other populations of general chemistry students is limited. We encourage other researchers to test our model and other competing models with more diverse student populations.
Second, we recognize that our models are imperfect and that all models are mis-specified to some degree (MacCallum, 2003). Although an assumption of path analysis and multiple regression is that the independent variables are measured without error, this can never be fully achieved. As noted earlier, our previous study (Ferrell and Barbera, 2015) supports the quality of the measures in terms of validity and reliability. We thoroughly investigated the psychometric properties of each scale used in the present study, a critical first step to any substantive investigation using self-report data. By having measures that have been properly designed and target the variables appropriately is critical to reducing the effects of systematic measurement error. Despite our efforts, one reliability estimate is borderline (EB (T1), α = 0.68), therefore the statistical power and parameter estimates in our model may be incorrectly estimated to a certain degree (Cole and Preacher, 2014). Furthermore, we acknowledge that without a full structural model, we cannot account for the measurement error of individual items. Path analysis was used instead due to our relatively small sample size (n = 173). While many others have used path analysis in a similar manner as we have (Bong, 2001; Lent et al., 2008; Jones et al., 2012), full structural models are superior for testing and generating theory. The models reported here are merely an estimation of parameters and do not necessarily accurately describe the motivational phenomena at play. Therefore, we encourage others to cross-validate our models using a different sample to ensure that the pattern of relationships observed here is not a capitalization on chance (Hermida, 2015).
We encourage others to examine additional longitudinal sets of relationships among grades, self-efficacy, interest, and effort beliefs as well as other affective processes such as student engagement. It is possible that effort beliefs or self-efficacy at the end of the semester could be an outcome of mid-term exam scores based on their reciprocal relationship (Williams and Williams, 2010). Jones et al. (2012) found that mid-term math grades had a positive relationship with incremental theory of intelligence measured later in the year. In addition, there could be possible mediator relationships that we were unable to explore in this study due to the timing of measurements. Gonzalez and Paoloni (2015a) found that situational interest was a mediator between indicators of student engagement (effort, persistence, and attention) and performance in physics. Student engagement has been linked to numerous motivation-related processes such as persistence, interest, and effort (Skinner et al., 2009; Ainley, 2012). By staggering the assessments of students' self-efficacy, interest, and effort beliefs across time, it becomes possible to look at temporally causal and mediator relationships among them. This work is vital for understanding a bigger picture of how student motivation develops and evolves over time in introductory chemistry courses.
Despite these limitations, we believe the work presented here is important for the chemical education community by adding to the burgeoning base of research on affective and motivational processes of chemistry students. We have presented the performance impacts of several motivational variables and highlighted the connections that exist between them. More research is needed to further our understanding of the complexities that exist with respect to academic motivation in the hopes of improving our curricula to enhance learning among future students.
References
- Abdullah M., (2008), Children's implicit theories of intelligence: its relationships with self-efficacy, goal orientations, and self-regulated learning, Int. J. Learn., 15(2), 47–56.
- Ainley M., (2012), Students' interest and engagement in classroom activities, in Christenson S., Reschly A., and Wylie C. (ed.), Handbook of research on student engagement, New York: Springer, pp. 283–302.
- Arjoon J. A., Xu X. and Lewis J. E., (2013), Understanding the State of the Art for Measurement in Chemistry Education Research: Examining the Psychometric Evidence, J. Chem. Educ., 90(5), 536–545.
- Bandura A., (1986), Social foundations of thought and action: a social cognitive theory, Englewood Cliffs, NJ: Prentice Hall.
- Bandura A., (1997), Self-efficacy: the exercise of control, New York: Freeman.
- Barbera J. and VandenPlas J. R., (2011), All Assessment Materials Are Not Created Equal: The Myths about Instrument Development, Validity, and Reliability, in Investigating Classroom Myths through Research on Teaching and Learning, American Chemical Society, vol. 1074, pp. 177–193.
- Barbera J., Adams W. K., Wieman C. E. and Perkins K. K., (2008), Modifying and validating the Colorado Learning Attitudes about Science Survey for use in chemistry, J. Chem. Educ., 85(10), 1435–1439.
- Bathgate M. E., Schunn C. D. and Correnti R., (2014), Children's Motivation Toward Science Across Contexts, Manner of Interaction, and Topic, Sci. Educ., 98(2), 189–215. DOI: http://10.1002/sce.21095.
- Bauer C. F., (2005), Beyond “student attitudes”: chemistry self-concept inventory for assessment of the affective component of student learning, J. Chem. Educ., 82(12), 1864–1870.
- Bauer C. F., (2008), Attitude toward Chemistry: A Semantic Differential Instrument for Assessing Curriculum Impacts, J. Chem. Educ., 85(10), 1440.
- Bentler P. M., (1990), Comparative fit indexes in structural models, Psychol. Bull., 107(2), 238–246.
- Black A. E. and Deci E. L., (2000), The effects of instructors' autonomy support and students' autonomous motivation on learning organic chemistry: a self-determination theory perspective, Sci. Educ., 84(6), 740–756.
- Blackwell L., (2002), Psychological mediators of student achievement during the transition to junior high school: the role of implicit theories, (Unpublished Doctoral Dissertation), Columbia University, New York.
- Blackwell L., Trzesniewski K. H. and Dweck C. S., (2007), Implicit theories of intelligence predict achievement across an adolescent transition: a longitudinal study and an intervention, Child Dev., 78(1), 246–263.
- Bong M., (2001), Role of self-efficacy and task-value in predicting college students' course performance and future enrollment intentions, Contemp. Educ. Psychol., 26(4), 553–570.
- Bouffard-Bouchard T., Parent S. and Larivee S., (1991), Influence of self-efficacy on self-regulation and performance among junior and senior high-school age students, Int. J. Behav. Dev., 14(2), 153–164.
- Brandriet A. R., Ward R. M. and Bretz S. L., (2013), Modeling meaningful learning in chemistry using structural equation modeling, Chem. Educ. Res. Pract., 14(4), 421–430.
- Browne M. W. and Cudeck R., (1992), Alternative ways of assessing model fit, Socio. Meth. Res., 21(2), 230–258.
- Byrne B. M., (2013), Structural equation modeling with LISREL, PRELIS, and SIMPLIS: basic concepts, applications, and programming, Psychology Press.
- Carter C., LaRussa M. A. and Bodner G. M., (1987), A study of two measures of spatial ability as predictors of success in different levels of general chemistry, J. Res. Sci. Teach., 24(7), 645–657.
- Chan J. Y. K. and Bauer C. F., (2014), Identifying At-Risk Students in General Chemistry via Cluster Analysis of Affective Characteristics, J. Chem. Educ., 91(9), 1417–1425.
- Chan J. Y. K. and Bauer C. F., (2015), Effect of peer-led team learning (PLTL) on student achievement, attitude, and self-concept in college general chemistry in randomized and quasi experimental designs, J. Res. Sci. Teach., 52(3), 319–346.
- Cole D. A. and Preacher K. J., (2014), Manifest variable path analysis: potentially serious and misleading consequences due to uncorrected measurement error, Psychol. Methods, 19(2), 300–315.
- Curran P. J., West S. G. and Finch J. F., (1996), The robustness of test statistics to nonnormality and specification error in confirmatory factor analysis, Psychol. Methods, 1(1), 16–29.
- Dalgety J. and Coll R. K., (2006), The influence of first-year chemistry students' learning experiences on their educational choices, Assessment and Evaluation in Higher Education, 31(3), 303–328.
- Dalgety J., Coll R. K. and Jones A., (2003), Development of Chemistry Attitudes and Experiences Questionnaire (CAEQ), J. Res. Sci. Teach., 40(7), 649–668.
- Dweck C. S., (2012), Implicit Theories, in Lange P. V., Kruglanski A. and Higgins T. (ed.), Handbook of Theories of Social Psychology, London: Sage, vol. 2, pp. 43–61.
- Ferrell B. and Barbera J., (2015), Analysis of students' self-efficacy, interest, and effort beliefs in general chemistry, Chem. Educ. Res. Pract., 16(2), 318–337.
- Galloway K. R., Malakpa Z. and Bretz S. L., (2016), Investigating Affective Experiences in the Undergraduate Chemistry Laboratory: Students' Perceptions of Control and Responsibility, J. Chem. Educ., 93(2), 227–238. DOI: http://10.1021/acs.jchemed.5b00737.
- Gist M. E. and Mitchell T. R., (1992), Self-Efficacy: A Theoretical Analysis of Its Determinants and Malleability, Acad. Manage. Rev., 17(2), 183–211.
- Gonzalez A. and Paoloni P. V., (2015a), Engagement and performance in physics: the role of class instructional strategies, and student's personal and situational interest, Revista de Psicodidáctica, 20(1), 25–45.
- Gonzalez A. and Paoloni V. P., (2015b), Perceived autonomy-support, expectancy, value, metacognitive strategies and performance in chemistry: a structural equation model in undergraduates, Chem. Educ. Res. Pract., 16(3), 640–653.
- Gore P. A., (2006), Academic self-efficacy as a predictor of college outcomes: two incremental validity studies, J. Career Assessment, 14(1), 92–115.
- Harackiewicz J. M., Barron K. E., Tauer J. M., Carter S. M. and Elliot A. J., (2000), Short-term and long-term consequences of achievement goals: predicting interest and performance over time, J. Educ. Psychol., 92(2), 316–330.
- Harackiewicz J. M., Durik A. M., Barron K. E., Linnenbrink-Garcia L. and Tauer J. M., (2008), The role of achievement goals in the development of interest: reciprocal relations between achievement goals, interest, and performance, J. Educ. Psychol., 100(1), 105–122.
- Häussler P. and Hoffmann L., (2002), An intervention study to enhance girls' interest, self-concept, and achievement in physics classes, J. Res. Sci. Teach., 39(9), 870–888. DOI: http://10.1002/tea.10048.
- Hermida R., (2015), The problem of allowing correlated errors in structural equation modeling: concerns and considerations, Comput. Meth. Soc. Sci., 3(1), 1–17.
- Hidi S. and Renninger K. A., (2006), The four-phase model of interest development, Educ. Psychol., 41(2), 111–127.
- House D. J., (1995), Noncognitive predictors of achievement in introductory college chemistry, Res. High. Educ., 36(4), 473–490.
- Hu L. T. and Bentler P. M., (1999), Cutoff criteria for fit indexes in covariance structure analysis: conventional criteria versus new alternatives, Struct. Equ. Modeling, 6(1), 1–55.
- Huck S., (2012), Reading Statistics and Research, 6th edn, Boston, MA: Pearson.
- Hull-Blanks E., Kurpius S. E. R., Befort C., Sollenberger S., Nicpon M. F. and Huser L., (2005), Career goals and retention-related factors among college freshmen, J. Career Dev., 32(1), 16–30.
- Hulleman C. S., Durik A. M., Schweigert S. B. and Harackiewicz J. M., (2008), Task values, achievement goals, and interest: an integrative analysis. J. Educ. Psychol., 100(2), 398–416.
- Hulleman C. S. and Harackiewicz J. M., (2009), Promoting interest and performance in high school science classes, Science, 326(5958), 1410–1412.
- Hulleman C. S., Godes O., Hendricks B. L. and Harackiewicz J. M., (2010), Enhancing interest and performance with a utility value intervention, J. Educ. Psychol., 102(4), 880–895. DOI: http://10.1037/a0019506.
- Jones B. D., Wilkins J. L. M., Long M. H. and Wang F. H., (2012), Testing a motivational model of achievement: how students' mathematical beliefs and interests are related to their achievement, Eur. J. Psychol. Educ., 27(1), 1–20.
- Jöreskog K. G. and Sörbom D., (2006), LISREL 8.80 for Windows, Computer Software, Lincolnwood, IL: Scientific Software International, Inc.
- Kenny D. A., (1979), Correlation and Causality, New York: Wiley.
- Landis R., Edwards B. D. and Cortina J., (2009), Correlated residuals among items in the estimation of measurement models, in Vandenberg R. J. and Lance C. E. (ed.), Statistical and methodological myths and urban legends: doctrine, verity and fable in the organizational and social sciences, New York: Routledge.
- Larson L. M., Stephen A., Bonitz V. S. and Wu T. F., (2014), Predicting Science Achievement in India Role of Gender, Self-Efficacy, Interests, and Effort, J. Career Assessment, 22(1), 89–101.
- Lee W., Lee M. J. and Bong M., (2014), Testing interest and self-efficacy as predictors of academic self-regulation and achievement, Contemp. Educ. Psychol., 39(2), 86–99.
- Lent R. W., Brown S. D. and Larkin K. C., (1984), Relation of self-efficacy expectations to academic achievement and persistence, J. Couns. Psychol., 31(3), 356.
- Lent R. W., Brown S. D. and Larkin K. C., (1986), Self-efficacy in the prediction of academic performance and perceived career options, J. Couns. Psychol., 33(3), 265–269.
- Lent R. W., Brown S. D. and Hackett G., (1994), Toward a unifying social cognitive theory of career and academic interest, choice, and performance, J. Vocat. Behav., 45(1), 79–122.
- Lent R. W., Brown S. D., Brenner B., Chopra S. B., Davis T., Talleyrand R. and Suthakaran V., (2001), The role of contextual supports and barriers in the choice of math/science educational options: a test of social cognitive hypotheses, J. Couns. Psychol., 48(4), 474–483.
- Lent R. W., Sheu H. B., Singley D., Schmidt J. A., Schmidt L. C. and Gloster C. S., (2008), Longitudinal relations of self-efficacy to outcome expectations, interests, and major choice goals in engineering students, J. Vocat. Behav., 73(2), 328–335.
- Lewis S. E. and Lewis J. E., (2007), Predicting at-risk students in general chemistry: comparing formal thought to a general achievement measure, Chem. Educ. Res. Pract., 8(1), 32–51.
- Lewis S. E., Shaw J. L., Heitz J. O. and Webster G. H., (2009), Attitude Counts: Self-Concept and Success in General Chemistry, J. Chem. Educ., 86(6), 744. DOI: http://10.1021/ed086p744.
- Lightsey R., (1999), Albert Bandura and the Exercise of Self-Efficacy, J. Cognit. Psychother., 13(2), 158–166.
- Linnenbrink-Garcia L., Durik A. M., Conley A. M., Barron K. E., Tauer J. M., Karabenick S. A. and Harackiewicz J. M., (2010), Measuring Situational Interest in Academic Domains, Educ. Psychol. Meas., 70(4), 647–671.
- Linnenbrink-Garcia L., Patall E. A. and Messersmith E. E., (2013), Antecedents and consequences of situational interest, Brit. J. Educ. Psychol., 83(4), 591–614. DOI: http://10.1111/j.2044–8279.2012.02080.x.
- MacCallum R. C., (2003), 2001 Presidential address: working with imperfect models, Multivar. Behav. Res., 38(1), 113–139.
- Marsh H. W. and Bailey M., (1991), Confirmatory factor analyses of multitrait-multimethod data: a comparison of alternative models, Appl. Psych. Meas., 15(1), 47–70.
- Mataka L. M. and Kowalske M. G., (2015), The influence of PBL on students' self-efficacy beliefs in chemistry, Chem. Educ. Res. Pract., 16(4), 929–938.
- Multon K. D., Brown S. D. and Lent R. W., (1991), Relation of self-efficacy beliefs to academic outcomes: a meta-analytic investigation, J. Couns. Psychol., 38(1), 30.
- Nauta M. M., Kahn J. H., Angell J. W. and Cantarelli E. A., (2002), Identifying the antecedent in the relation between career interests and self-efficacy: is it one, the other, or both? J. Couns. Psychol., 49(3), 290–301.
- Nielsen S. E. and Yezierski E., (2015), Exploring the Structure and Function of the Chemistry Self-Concept Inventory with High School Chemistry Students, J. Chem. Educ., 92(11), 1782–1789.
- Nieswandt M., (2007), Student affect and conceptual understanding in learning chemistry, J. Res. Sci. Teach., 44(7), 908–937.
- Osborne J. and Waters E., (2002), Four assumptions of multiple regression that researchers should always test, Practical Assess. Res. Eval., 8(2), 1–9.
- Pajares F., (1996), Self-efficacy beliefs in academic settings, Rev. Educ. Res., 66(4), 543–578.
- Pajares F. and Kranzler J., (1995), Self-efficacy beliefs and general mental ability in mathematical problem-solving, Contemp. Educ. Psychol., 20(4), 426–443.
- Pajares F. and Miller D. M., (1994), Role of self-efficacy and self-concept beliefs in mathematical problem solving: a path analysis, J. Educ. Psychol., 86(2), 193–203.
- Pajares F. and Miller D. M., (1995), Mathematics self-efficacy and mathematics performances: the need for specificity of assessment, J. Couns. Psychol., 42(2), 190–198.
- Pedhazur E. J., (1997), Multiple regression in behavioral research: explanation and prediction, 3rd edn, Fort Worth: Harcourt Brace.
- Podsakoff P. M., MacKenzie
S. B., Lee J. Y. and Podsakoff N. P., (2003), Common method biases in behavioral research: a critical review of the literature and recommended remedies, J. Appl. Psychol., 88(5), 879–903.
- Raykov T. and Marcoulides G. A., (2000), A First Course in Structural Equation Modeling. Mahwah N.J.: Lawrence Erlbaum Associates, Inc.
- Robbins S. B., Lauver K., Le H., Davis D., Langley R. and Carlstrom A., (2004), Do psychosocial and study skill factors predict college outcomes? A meta-analysis, Psychol. Bull., 130(2), 261–288.
- Schermelleh-Engel K., Moosbrugger H. and Müller H., (2003), Evaluating the fit of structural equation models: tests of significance and descriptive goodness-of-fit measures, Meth. Psychol. Res., 8(2), 23–74.
- Siegel R. G., Galassi J. P. and Ware W. B., (1985), A comparison of two models for predicting mathematics performance: social learning versus math aptitude–anxiety, J. Couns. Psychol., 32(4), 531–538.
- Silvia P. J., (2003), Self-efficacy and interest: experimental studies of optimal incompetence, J. Vocat. Behav., 62(2), 237–249.
- Skinner E., Kinderman T. and Furrer C., (2009), A motivational perspective on engagement and disaffection. Conceptualization and assessment of children's behavioral and emotional participation in the academic activities in the classroom, Educ. Psychol. Meas., 69(3), 493–525.
- Smist J. M., (1993), General Chemistry and Self-Efficacy, Paper presented at the Paper presented at the American Chemical Society national meeting, Chicago, IL.
- Smith P. L. and Fouad N. A., (1999), Subject-matter specificity of self-efficacy, outcome expectancies, interests, and goals: Implications for the social–cognitive model, J. Couns. Psychol., 46(4), 461–471.
- Sorich L. and Dweck C. S., (1997), Reliability data for new scales measuring students' beliefs about effort and responses to failure, Unpublished raw data. Columbia University.
- Spencer H. E., (1996), Mathematical SAT test scores and college chemistry grades, J. Chem. Educ., 73(12), 1150–1153.
- Steiger J. H., (1990), Structural model evaluation and modification: an interval estimation approach, Multivar. Behav. Res., 25(2), 173–180.
- Streiner D. L., (2005), Finding our way: an introduction to path analysis, Can. J. Psychiat., 50(2), 115–122.
- Streiner D. L., (2006), Building a Better Model: an Introduction to Structural Equation Modelling, Can. J. Psychiat., 51(5), 317–324.
- Tempelaar D. T., Rienties B., Giesbers B. and Gijselaers W. H., (2015), The Pivotal Role of Effort Beliefs in Mediating Implicit Theories of Intelligence and Achievement Goals and Academic Motivations, Soc. Psychol. Educ., 18(1), 101–120.
- Uzuntiryaki E. and Aydin Y. C., (2009), Development and Validation of Chemistry Self-Efficacy Scale for College Students, Res. Sci. Educ., 39(4), 539–551.
- Villafane S. M. and Lewis J. E., (2016), Exploring a measure of science attitude for different groups of students enrolled in introductory college chemistry, Chem. Educ. Res. Pract., DOI: 10.1039/C5RP00185D.
- Villafane S. M., Garcia A. C. and Lewis J. E., (2014), Exploring diverse students' trends in chemistry self-efficacy throughout a semester of college-level preparatory chemistry, Chem. Educ. Res. Pract., 15(2), 114–127.
- Vuong M., Brown-Welty S. and Tracz S., (2010), The effects of self-efficacy on academic success of first-generation college sophomore students, J. Coll. Student Dev., 51(1), 50–64.
- Weiner B., (1990), History of motivational research in education, J. Educ. Psychol., 82(4), 616–622.
- Williams T. and Williams K., (2010), Self-efficacy and performance in mathematics: reciprocal determinism in 33 nations, J. Educ. Psychol., 102(2), 453.
- Wright S. L., Jenkins-Guarnieri M. A. and Murdock J. L., (2012), Career development among first-year college students: college self-efficacy, student persistence, and academic success, J. Career Dev., 292–310.
- Xu X. and Lewis J. E., (2011), Refinement of a Chemistry Attitude Measure for College Students, J. Chem. Educ., 88(5), 561–568.
- Xu X., Villafane S. M. and Lewis J. E., (2013), College students' attitudes toward chemistry, conceptual knowledge and achievement: structural equation model analysis, Chem. Educ. Res. Pract., 14(2), 188–200.
- Yeager D. S. and Walton G. M., (2011), Social-psychological interventions in education: they're not magic, Rev. Educ. Res., 81(2), 267–301.
- Zusho A., Pintrich P. R. and Coppola B., (2003), Skill and will: the role of motivation and cognition in the learning of college chemistry, Int. J. Sci. Educ., 25(9), 1081–1094.
|
This journal is © The Royal Society of Chemistry 2016 |
Click here to see how this site uses Cookies. View our privacy policy here.