Self-efficacy and academic performance in first-semester organic chemistry: testing a model of reciprocal causation
Received
19th May 2016
, Accepted 5th July 2016
First published on 21st July 2016
Abstract
Self-efficacy is an affective learning outcome that has been associated with academic performance and retention in STEM. Self-efficacy has been defined as students' beliefs about their ability to complete a given task, and it can be affected by a student's positive or negative experience in a course. In this study, students' chemistry self-efficacy in an organic chemistry course and its reciprocal causation relationship with performance in the course was examined using structural equation modeling (SEM). Organic chemistry self-efficacy (OCSE) was measured five times during the semester and students' scores in four term exams and a final exam were used as measures of performance. Five different models were proposed and tested to explain the relationship between OCSE and performance. A reciprocal causation model with an exam snowball effect was found to best explain the relationship between OCSE and performance compared to other alternative models including a reciprocal causation model without the snowball effect and an autoregressive model. This model suggests a significant positive relationship between OCSE and performance throughout the semester, providing empirical support for the reciprocal relationship of these two measures. Implications for research and instruction are discussed in terms of the reciprocal relationship between self-efficacy and performance.
Introduction
Self-efficacy is an affective learning outcome associated with increased content learning (i.e., academic performance) in STEM courses and retention in STEM degree programs. Much of the self-efficacy research in chemical education has focused on single or pre/post administrations of self-efficacy assessment instruments (Uzuntiryaki and Aydin, 2009; Cook, 2013; Ferrell and Barbera, 2015; Uzuntiryaki-Kondakci and Senay, 2015); very few of them have looked at trends in self-efficacy throughout a semester (Dalgety and Coll, 2006; Villafañe et al., 2014). Affective learning develops across time and is influenced by episodic events of which graded assessments, including examinations, have a large impact. The cumulative nature of an undergraduate organic chemistry curriculum provides a unique opportunity to explore how self-efficacy develops over a semester-long course and is influenced by examination performance.
Retention in STEM related fields is a concern for researchers and educators in the United States. Reports have stressed the need for the U.S. to improve the STEM workforce and increase the number of professionals in those fields in the next decade (National Science Foundation, 2003, 2010; PCAST, 2012). As educators and researchers, we have seen the STEM pipeline narrow in recent years; fewer students are completing undergraduate STEM degrees (PCAST, 2012). According to the President's Council of Advisors on Science and Technology (PCAST), less than 40% of the students who enter college with the intention to complete a degree in STEM-related fields complete a degree in those fields (PCAST, 2012). This statistic is concerning; understanding which factors could potentially have an impact on a student's decision to stay or leave a STEM field is very important. Potential factors are cognitive and non-cognitive; these factors can influence performance and retention. Cognitive domain factors related to students' performance in chemistry include prior math achievement (Cooper and Pearson, 2012; Scott, 2012; Xu et al., 2013) and prior conceptual knowledge (Seery, 2009; Wagner et al., 2002; Xu et al., 2013). Non-cognitive domain factors related to performance and retention include attitude (House, 1995; Cukrowska et al., 1999; Bauer, 2008; Xu and Lewis, 2011; Xu et al., 2013), self-concept (Bauer, 2005; Lewis et al., 2009), and motivation (Black and Deci, 2000; Akbas and Adnan, 2007).
In the following sections, we will discuss self-efficacy and the need to develop task-specific self-efficacy assessment instruments, the cumulative nature of the first-semester organic chemistry course, and the reciprocal relationship between self-efficacy and task achievement. The proposed reciprocal relationship and alternative structural equation models were tested with data from the first-semester of an undergraduate organic chemistry course. Implications for the assessment of affective learning and teaching of undergraduate organic chemistry will be outlined.
Theoretical framework
Self-efficacy is a construct from Bandura's social cognitive theory (Bandura, 1986, 1997). This construct has been defined as a person's beliefs about their ability to successfully perform a given task. Self-efficacy has gained a lot of attention in science education over the last two decades (Pajares, 1996a; Britner and Pajares, 2001; Lawson et al., 2007; Zeldin et al., 2008; Scherer, 2013; Jansen et al., 2015; Larson et al., 2015; Miller et al., 2015) and more recently, in chemistry education research (Uzuntiryaki and Aydin, 2009; Cook, 2013; Villafañe et al., 2014; Cheung, 2015; Ferrell and Barbera, 2015; Holme et al., 2015; Uzuntiryaki-Kondakci and Senay, 2015; Boz et al., 2016). Self-efficacy is a dimension of success that has been linked to student learning and perseverance (Bandura, 1986; Britner and Pajares, 2001). Student learning of a given topic depends on several factors (e.g., previous knowledge, motivation, course structure and materials, etc.); one of these factors is confidence. According to the social cognitive theory, if a student does not feel confident that they can successfully complete a given task, or a set of tasks, they will try to avoid the task. Task avoidance is a way of delaying what a student has to do to learn the topic. This avoidance behavior has been related to lower self-efficacy. Students with low self-efficacy not only delay their attempts to complete tasks, but also may give up after unsuccessful trials. If a student has high self-efficacy, they are more likely to try and choose more challenging learning tasks, and persist and complete those tasks successfully (Britner and Pajares, 2001; Pajares and Schunk, 2001; Margolis and McCabe, 2003; Zeldin et al., 2008). Therefore, students with higher self-efficacy are expected to have more positive outcomes related to performance and retention than students with lower self-efficacy (Bandura, 1986; Britner and Pajares, 2001).
Students' perceptions of their ability to perform a given task depend on several factors such as performance and feedback; in general though, self-efficacy increases with positive experiences and decreases with negative experiences (Dalgety and Coll, 2006). For example, if a student successfully completes a given task, they will feel confident and will be more willing to try the next task; their perception of their ability will increase. This positive experience creates a judgment of their competence in completing a task which is one of the sources of self-efficacy (i.e. mastery experience) that influences self-efficacy beliefs (Britner and Pajares, 2006; Usher and Pajares, 2008). According to Bandura (1986, 1997) there are four sources of self-efficacy. First is mastery experience; this source refers to the experience a person has when performing a given task as presented in the above example. The second is vicarious experience, referring to a person's perception of performing a given task because someone similar to themselves can do it. For example, if a student scored lower in an exam relative to their peers, that student's self-efficacy will likely decrease, but if the score is higher relative to the peers, then the self-efficacy will likely increase. The third is social persuasion; this source takes place when a person is encouraged by someone else to perform a given task. In this case, the instructor could have a direct influence on students' self-efficacy by the feedback and encouragement provided to the student after completing a task. The last is emotional and psychological states, referring to the anxiety and stress that a person faces when performing a given task. High anxiety or stress when performing a given task is interpreted by students as a lack of skill or ability to complete the task and will likely have a negative impact on students' self-efficacy (Usher and Pajares, 2008).
Self-efficacy is a construct that is context and task dependent (Pajares, 1996b). For a measure of self-efficacy to be meaningful it must be domain-specific. For example, a general measure of self-efficacy (example item: how well can you complete your homework?) would be inappropriate for understanding student application of evolutionary processes leading to homo sapiens from a first-semester general biology course. Pajares (1996b) would argue that this item would not be associated with achievement in biology because it does not clearly ask about a specific task from the course. In our study, we are interested in assessing students' beliefs about their ability to complete organic chemistry tasks thus we measure students' organic chemistry self-efficacy and performance in an organic chemistry course.
Relationship of CSE with performance
Self-efficacy is associated with performance, persistence, and the willingness to try challenging tasks. Several studies have investigated self-efficacy and its relationship with performance in science (Multon et al., 1991; Andrew, 1998; Pajares, 2002; Lawson et al., 2007), in math (Williams and Williams, 2010; Peters, 2013; Parker et al., 2014; Kalaycioglu, 2015), and in engineering (Purzer, 2011). These works have found a positive relationship between self-efficacy and performance. The relationship of chemistry self-efficacy and chemistry performance has also been studied (Zusho et al., 2003; Kan and Akbas, 2006; Lalich et al., 2006; Uzuntiryaki-Kondakci and Senay, 2015; Boz et al., 2016); a similar positive relationship was found. In an introductory college chemistry course, Lalich et al. (2006), Kan and Akbas (2006), and Zusho et al. (2003) all found a medium size correlation between self-efficacy and final achievement; Uzuntiryaki-Kondakci and Senay (2015), using structural equation modeling, found chemistry self-efficacy to be a significant predictor of chemistry achievement for 11th grade Turkish students. The results obtained from these studies in science and in chemistry specifically suggest the importance of the construct of self-efficacy for students' performance in science related courses. Missing from this work are studies in non-introductory chemistry courses (such as organic chemistry) and measures of self-efficacy across time in those courses.
Context: a first-semester organic chemistry course
Organic chemistry is a course that is at the core of most health-related careers as well as for chemistry majors. The first semester of the yearlong organic chemistry course in the U.S. is focused on naming chemical structures, understanding the three-dimensionality of chemical structures, introducing functional group transformations and chemical reactions. Most textbooks are organized such that the structure and nomenclature are taught first in the course. The curriculum is designed so that knowledge from earlier chapters is necessary in subsequent chapters. For example, different representation systems (e.g., wedged-dashed formulae, Newman projections, chair confirmations of cyclohexane derivatives) are taught when introducing a chemical structure; later in the course these representations are used to teach the stereoselectivity and regioselectivity of elimination reactions. Based on the current organization of topics in this first semester course, the curriculum is inherently cumulative.
Organic chemistry is a prerequisite course to many upper level chemistry courses (in particular, biochemistry) and in some cases, cell and molecular biology courses. Thus, organic chemistry is considered to be a barrier to progression in degree programs built on prerequisite upper-level courses, and entrance to graduate and professional degree programs (e.g., medical school). Retention rates are typically low and drop/withdraw/fail rates are high for chemistry courses, thus focusing on students' self-efficacy as a way to understand students' decisions of staying or not staying in the course is important. Self-efficacy has been proposed as a factor that influences student retention (Bandura, 1986; Britner and Pajares, 2001; Pajares and Schunk, 2001; Margolis and McCabe, 2003; Zeldin et al., 2008), and it has been hypothesized that students with higher self-efficacy are more likely to persist on given tasks longer than students with lower self-efficacy. Therefore, understanding the role that self-efficacy plays in performance across organic chemistry courses will provide insights into how and when to promote affective learning in the curriculum.
Reciprocal causation model
Self-efficacy and academic performance are interconnected; self-efficacy influences academic performance and at the same time academic performance influences self-efficacy. A reciprocal causation model provides the means to test this interconnected relationship across time (Marsh et al., 2005; Pekrun et al., 2014). Our model presupposes that prior self-efficacy influences future self-efficacy, prior performance influences future performance, self-efficacy influences performance, and performance influences self-efficacy (see Fig. 1).
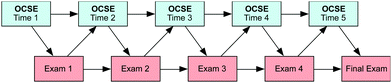 |
| Fig. 1 Reciprocal Causation Model for organic chemistry self-efficacy and performance (OCSE = Organic Chemistry Self-Efficacy). | |
The reciprocal causation model has been examined in different settings: job-related (Jonge et al., 2001; De Lange et al., 2004) and academic-related (Ma, 1997; Marsh et al., 2005; Marsh and O'Mara, 2008; Pekrun et al., 2014). In job-related settings, Jonge et al. (2001) found a reciprocal relationship between job satisfaction, job demands, and workplace social support; while De Lange et al. (2004) found a reciprocal causal relationship between work characteristics, such as job demands, job control and supervisor support, and the mental health of the employees. In the academic setting, the reciprocal relationship between academic self-concept and achievement has been studied with 7th grade students (Marsh et al., 2005), as well as with a seven-year longitudinal study that includes students starting in 10th grade (Marsh and O'Mara, 2008). In both cases, evidence for a positive reciprocal relationship between self-concept and achievement was found. In another study, Ma (1997) found that there was a positive reciprocal relationship between attitude toward mathematics from high school students and their mathematics achievement. Pekrun et al. (2014) examined the relationship between academic boredom and performance in an introductory college psychology course and they found that boredom had a consistent negative effect on the subsequent performance and performance also had a negative effect on subsequent boredom. While the reciprocal causation model has gained some attention in the educational research setting relating to the longitudinal interaction of affective learning measures such as boredom (Pekrun et al., 2014), self-concept (Marsh et al., 2005) and attitude (Ma, 1997), it has not been used in chemistry education research when investigating comparable affective learning.
Self-efficacy is a construct that is cyclic in nature, changing with positive or negative experiences (Dalgety and Coll, 2006). Therefore, we expect self-efficacy to fluctuate throughout the semester with positive and negative performances. Because of this expectation, it is reasonable to propose the reciprocal causation model to examine the relationship between self-efficacy and performance in an organic chemistry course. This study will be the first to look at this relationship using a reciprocal causation model.
Research hypotheses
The goal for the present study is to examine students' chemistry self-efficacy in an organic chemistry course and its reciprocal causation relationship with performance in the course. We will use structural equation modeling to test the hypothesis that a reciprocal causation model will fit self-efficacy and achievement data better than alternative models (i.e., self-efficacy effects model, performance effects model, autoregressive model, and reciprocal model without a snowball effect). Also, we hypothesize that there is a positive relationship between organic chemistry self-efficacy and performance.
Method
Study measures
Organic chemistry self-efficacy (OCSE).
Students' self-efficacy in organic chemistry was measured five times during the semester using items developed by authors SMV and JRR. The self-efficacy items were developed to examine students' confidence completing specific tasks related to the content covered in the course. Students were asked the question, “How well can you…”, and the students responded to a specific task (item) using a five-point scale, which included the following options: very poorly, poorly, average, well and very well. Five different measures of OCSE were developed which were cumulative in nature, as shown in Table 1. The first OCSE measure included three items on basic content covered in general chemistry or during the first few weeks of the course, such as “Draw resonance structures for a given molecule or ion?” and “Identify functional groups present from an Infrared (IR) spectrum?” These tasks are foundational; students should know and be confident that they can do them before being able to complete more challenging tasks. The second OCSE measure included seven items, three from the first measure and four new items corresponding to the “new” tasks covered in the course. The new items in the second measure included “Determine the most stable chair conformation of a substituted cyclohexane?” and “Convert between a bond line structure and a Newman projection?” The third OCSE measure included the first seven items and three items were added. The new items included “Assign (R) or (S) configuration to a chiral center?” and “Predict the product of a reaction from starting materials and reagents?” The fourth OCSE measure included 11 items, 10 items from the third measure and one new item, “Determine the relative stability of a series of ions?” The last OCSE measure included a total of 12 items and only one item was added, “Determine the relative stability of a series of radicals?”
Table 1 Organic chemistry self-efficacy (OCSE) items by administration time
Item (How well can you…) |
Time |
1 |
2 |
3 |
4 |
5 |
(1) Draw resonance structures for a given molecule or ion? |
* |
* |
* |
* |
* |
(2) Determine the relative boiling points for a series of compounds? |
* |
* |
* |
* |
* |
(3) Identify functional groups present from an Infrared (IR) spectrum? |
* |
* |
* |
* |
* |
(4) Determine the most stable chair conformation of a substituted cyclohexane? |
|
* |
* |
* |
* |
(5) Convert between a bond line structure and a Newman projection? |
|
* |
* |
* |
* |
(6) Construct an energy diagram for the sigma bond rotation of a molecule? |
|
* |
* |
* |
* |
(7) Convert between a drawn structure and structure built from a model kit? |
|
* |
* |
* |
* |
(8) Assign (R) or (S) configuration to a chiral center? |
|
|
* |
* |
* |
(9) Convert between a bond line structure and a Fischer projection? |
|
|
* |
* |
* |
(10) Predict the product of a reaction from starting materials and reagents? |
|
|
* |
* |
* |
(11) Determine the relative stability of a series of ions? |
|
|
|
* |
* |
(12) Determine the relative stability of a series of radicals? |
|
|
|
|
* |
Academic performance.
Performance in the organic chemistry course was measured using two different measures. The first measure used in this study is four term exams prepared by the instructor. These exams consisted of eleven to fourteen open-ended/free-response items; the instructor and a group of teaching assistants graded the exams following a predefined rubric. A particular grader was assigned a single exam item or page of items to grade, providing consistency in scoring across a given exam. Exams were spot checked by the course instructor and students had the opportunity to request regrades (most commonly for incorrect total score determination). Any grade changes due to regrades are reflected in the data used in this study. The total points for each exam were 100 points, 15% of the total course grade per exam (i.e., a total of 60% of the course grade). The second measure was the First Term Organic Chemistry Exam from the Examinations Institute of the American Chemical Society Division of Chemical Education, used as the final exam for the course. This exam consisted of 70 multiple-choice questions (ACS Examinations Institute, 2014). The Final Exam was worth 150 points, representing 20% of the course grade.
Participants and procedure
Participants were students enrolled in the first semester of a yearlong organic chemistry course during Fall 2015 at a large southeastern research-intensive university in the United States. Students in the course completed the OCSE questionnaire within 72 hours prior to taking each of the four term exams and the final exam. The first three OCSE questionnaires were administered during class time via paper and pencil. The fourth and fifth questionnaires were completed online via Qualtrics; one reminder email was sent to students to complete the questionnaire on the day of the examination. IRB approval was obtained to conduct this study.
A total of 240 students completed at least one administration of the OCSE. In the course: 63.3% were female, 55.6% were non-white, and 72.5% were in their second year of studies at the institution. Of the 240 students who began the study, 173 students completed all five iterations of the OCSE questionnaire; these students will herein be referred to as study participants. The response rate for each administration ranged from 94% to 98%. The study participants were 68.8% female, 59.5% non-white, and 72.3% in their second year of studies at the institution. Students received bonus points for completing each OCSE.
Model specification for the reciprocal causation model
Structural equation modeling (SEM) was used to specify and test five different models in this study (see Fig. 2 and 3). For testing the models, data from OCSE and exams were converted to z-scores; standardized scores are necessary to account for the strong conceptual overlap of the measures yet limited comparability of the measures across the study (i.e., each exam and self-efficacy measure had different, yet conceptually overlapping items). The first SEM model is the reciprocal causation model (Model 1), which is represented in Fig. 2. This model is our hypothesized model for this study, where OCSE at time 1 influences students' performance at Exam 1; at the same time students' performance in Exam 1 will influence their confidence at time 2 (OCSE at time 2). This reciprocal relationship is observed with the other four OCSE questionnaires (Time 2 to Time 5) and exams (Exam 2 to Final Exam). Also, this model has a direct relationship between Exam 1 and Exam 2, but also between Exam 1 and the rest of the Exams (Exams 2, 3, 4, and Final Exam). This relationship is represented in the diagram with curved arrows from Exam 1 and it will be referred to in this study as the snowball effect. This snowball effect is observed as a result of the cumulative nature of the content in the course; whereby the content from Exam 1 is the foundation for the rest of the material covered in the course. For example, students are assessed on their ability to convert between condensed, bond-line, and wedge-dashed formulae in the first exam; this skill is necessary for success in determining stereochemistry, evaluating structure property relationships, and predicting the product of reactions. For OCSE, the snowball effect was not added to the model given that the OCSE measures were developed in a way to measure the cumulative aspect of self-efficacy throughout the semester (i.e., each administration of the OCSE had all previous items and several new items that had been introduced since the last OCSE administration).
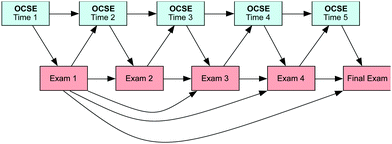 |
| Fig. 2 Reciprocal causation model (Model 1). | |
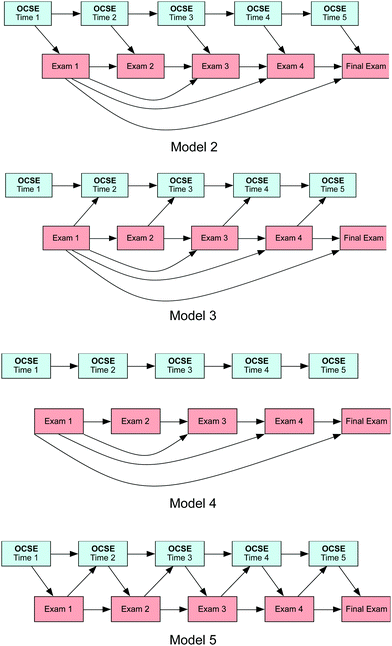 |
| Fig. 3 Alternative Pathway Models: Model 2: OCSE effects model; Model 3: performance effects model; Model 4: autoregressive model; Model 5: reciprocal model without the snowball effect. | |
In addition to our proposed model, four alternate models were specified and tested as presented in Fig. 3 (Models 2 through 5). These models have more constraints than the reciprocal causation model (Fig. 2) and are being tested to compare their fit to the proposed model. The first alternate model, Model 2, is a unidirectional model that estimates the effects of OCSE on subsequent performance (exams), but constrains the effect of performance on OCSE to zero. The next model, Model 3, is a unidirectional model, but in this case it is estimating the effects of performance (exams) on OCSE but constrains the effect of OCSE on the subsequent performance to zero. Model 4 is an autoregressive model that constrained all effects of OCSE on performance and vice versa, to zero; Model 4 assumes that there is no relationship between OCSE and performance at any time. The last model, Model 5, is the reciprocal causation model; however, it constrains the snowball effect of Exam 1 on Exams 3, 4 and the Final Exam.
Data analysis
Descriptive statistics.
Students with complete data for the organic chemistry self-efficacy and achievement were used in the analysis. Descriptive statistics for the set of variables (OCSE and Exams) were obtained using SAS 9.3 (N = 173 study participants). General trends in mean, standard deviation, univariate normality using skewness and kurtosis values, and correlation using Pearson correlation coefficients for each variable were examined. Missing data for OCSE and Exams were also examined for patterns and no evidence of bias on the results was found in this sample.
OCSE psychometrics and reciprocal causation models.
Reliability of the OCSE scores for each administration was assessed using Cronbach's alpha using SAS 9.3. Cronbach's alpha coefficient was used to examine the internal consistency of the scores and determine if the items in each of the questionnaires yielded consistent scores. The most common cutoff for Cronbach's alpha is 0.7 (Cortina, 1993; Murphy and Davidshofer, 2005).
Validity evidence for the internal structure of the scores was gathered using Confirmatory Factor Analysis (CFA) for each OCSE administration (Arjoon et al., 2013; American Educational Research Association, American Psychological Association, National Council on Measurement in Education, 2014). CFA was performed using MPlus 7.1 to estimate the model fit of the proposed model for each administration. The proposed model in each case involves a one-factor solution where all the items' scores for a given questionnaire are measuring students' beliefs about their confidence in completing different tasks related to organic chemistry at a given time. CFA was run using the Robust Maximum Likelihood (MLR) estimator. The goodness of fit for the proposed model was determined via a Comparative Fit Index (CFI) greater than 0.90 and a Standardized Root-Mean Squared Residual (SRMR) less than 0.08 (Hu and Bentler, 1999; Cheng and Chan, 2003).
For the evaluation of the reciprocal causation model and the alternate models, the same CFA fit indices and guidelines were used. The robust Maximum Likelihood (MLR) estimator was also used to estimate the parameters for these models. To compare the alternate models to the reciprocal causation model (Pekrun et al., 2014), a scaled difference chi-square test was used to compare the fitness of nested models, appropriate when the MLR estimation approach is used (Satorra and Bentler, 2001). Akaike information criterion (Akaike, 1974) and the sample-size adjusted Bayesian information criterion (Schwarz, 1978) are used for comparison of nest and non-nested models. The absolute AIC or BIC value of a single model does not have a certain range indicating fit. For multiple models from the same dataset, smaller AIC and BIC values are representative of better fitting models.
Results and discussion
Descriptive statistics
An organic chemistry self-efficacy (OCSE) questionnaire was administered five times during the semester within 72 hours prior to each exam and the final exam. Descriptive statistics for each administration of the OCSE questionnaire as well as for each exam and the final exam are presented in Table 2. The mean for the OCSE scores ranged from 3.18 to 3.68, which suggests that students have positive beliefs regarding their confidence in completing organic chemistry related tasks at each administration. Since the OCSE measures at each time are different, the mean scores are only presented as descriptive information and not for comparison. In terms of performance, the mean for each exam ranged from 81.59 in Exam 1 to 52.12 in Exam 4. For the Final Exam, the mean score was 81.87 out of 150 points, which represents 54.38%. The univariate normality was assessed via skewness and kurtosis values for each variable. As presented in Table 1, the absolute values for skewness and kurtosis for the majority of the variables are less than 1 and 2, respectively, which indicates an approximately normal distribution for those scores. The only variable with an absolute value for skewness and kurtosis higher than 1 is Exam 1. The deviation is not large from the recommended cutoff and therefore is not a concern for the normality of the scores in general.
Table 2 Descriptive statistics and Pearson correlation coefficients for study variables
|
OCSE 1 |
OCSE 2 |
OCSE 3 |
OCSE 4 |
OCSE 5 |
Exam 1 |
Exam 2 |
Exam 3 |
Exam 4 |
Final exam |
Note: *p < 0.05, **p < 0.01. |
Mean |
3.18 |
3.44 |
3.68 |
3.63 |
3.56 |
81.59 |
77.18 |
63.15 |
52.12 |
81.87 |
SD |
0.63 |
0.64 |
0.63 |
0.64 |
0.67 |
12.31 |
12.95 |
12.97 |
18.22 |
19.08 |
Min |
1.67 |
1.86 |
1.9 |
2.18 |
1.00 |
36 |
33 |
23 |
4 |
34.09 |
Max |
5.00 |
5.00 |
5.00 |
5.00 |
5.00 |
102 |
97 |
93 |
96 |
136.36 |
Skew |
0.21 |
0.02 |
−0.36 |
0.01 |
−0.3 |
−1.2 |
−0.95 |
−0.31 |
−0.39 |
0.16 |
Kurtosis |
−0.29 |
−0.41 |
−0.39 |
−0.66 |
0.47 |
2.11 |
0.77 |
0.21 |
−0.3 |
−0.43 |
|
OCSE 1 |
|
0.51** |
0.42** |
0.43** |
0.40** |
0.09 |
0.19* |
0.20** |
0.17* |
0.13 |
OCSE 2 |
|
|
0.67** |
0.67** |
0.59** |
0.24** |
0.34** |
0.40** |
0.30** |
0.30** |
OCSE 3 |
|
|
|
0.78** |
0.72** |
0.29** |
0.39** |
0.39** |
0.36** |
0.37** |
OCSE 4 |
|
|
|
|
0.83** |
0.21** |
0.30** |
0.37** |
0.32** |
0.28** |
OCSE 5 |
|
|
|
|
|
0.24** |
0.31** |
0.41** |
0.37** |
0.34** |
Exam 1 |
|
|
|
|
|
|
0.57** |
0.57** |
0.63** |
0.61** |
Exam 2 |
|
|
|
|
|
|
|
0.60** |
0.62** |
0.56** |
Exam 3 |
|
|
|
|
|
|
|
|
0.68** |
0.69** |
Exam 4 |
|
|
|
|
|
|
|
|
|
0.67** |
Pearson-product moment correlation coefficients were obtained for the set of variables and are presented in Table 2. As shown, all correlations are significant between OCSE and exams, except for OCSE 1 and Exam 1. The correlation between OCSE at time 1 and Exam 1 is 0.09. This small relationship is not surprising since the first OCSE questionnaire was given before any measure of actual performance. If we look at their scores, students' OCSE scores at time 1 had the lowest mean, while Exam 1 has the highest mean score of the semester. Also, we observed a small non-significant relationship between OCSE at time 1 and the Final Exam. This correlation is also not surprising given that the organic chemistry tasks in OCSE 1 although relevant for the final exam are not assessed in OCSE at time 1.
OCSE psychometrics
The internal consistency reliability was assessed using Cronbach's alpha coefficient. Cronbach's alpha was obtained for each of the OCSE administrations and their values ranged from 0.77 to 0.90 for OCSE 2 to OCSE 5. For the first administration (OCSE 1), the value was lower than subsequent administrations (0.46), which indicates that students were not as consistent in their responses in the first questionnaire. This suggests that students' beliefs about their confidence in completing the organic chemistry tasks were not consistent at the beginning of the semester. This can be expected since some of the tasks were first learned in general chemistry, while others are more organic specific and were introduced for the first time. Also, due to the cumulative nature of the OCSE measure to cover the course content during the semester, the number of items increased each time. Since the first questionnaire had only three items and Cronbach's alpha is influenced by the number of items (Cortina, 1993; Murphy and Davidshofer, 2005), the low value is not surprising. However, this OCSE measure with only three items still provides more useful information than using a single item or not having the measure (Villafañe et al., 2011). Therefore, collectively these Cronbach's alpha values suggest good internal consistency for the OCSE scores and provide valuable information about the scores.
Validity evidence for the internal structure of the OCSE scores was determined using Confirmatory Factor Analysis (CFA). Fit indices for each OCSE questionnaire indicate a reasonable fit for the 1-factor solution. The CFI index ranged from 0.87 to 0.99 and SRMR values ranged from 0.01 to 0.06. Therefore, it is reasonable to interpret the scores of the OCSE questionnaires as measuring one construct, i.e. organic chemistry self-efficacy beliefs, or more specifically, students' belief regarding how confident they feel about completing a set of tasks related to organic chemistry. We will therefore use a factor score for each OCSE administration when evaluating our proposed reciprocal causation model.
Reciprocal causation model and alternate models
A reciprocal causation model was tested with the set of variables in this study. The model, as shown in Fig. 2, has an interconnected relationship between organic chemistry self-efficacy (OCSE) and chemistry performance where OCSE influences subsequent chemistry performance while at the same time the chemistry performance influences subsequent OCSE. Alternate models, as presented in Fig. 3 (Models 2 through 5), were also tested following the procedure performed by Pekrun et al. (2014). The results for each of the tested models as well as the comparison between them are presented in Table 3.
Table 3 Model fit indices results for reciprocal causation model and alternative models
Model |
χ
2
|
df |
p-value |
CFI |
SRMR |
AIC |
ΔAIC |
BIC |
Δdf |
SBΔχ2 |
Note: *ΔAIC greater than 10 indicates a poorer model fit; **fit significantly worse than the relaxed model with p<0.001. Model 1: reciprocal causation model; Model 2: OCSE effects model; Model 3: performance effects model; Model 4: autoregressive model; Model 5: reciprocal model without the snowball effect. |
1 |
75 |
25 |
<0.001 |
0.95 |
0.056 |
3483 |
— |
3603 |
— |
|
2 |
101 |
29 |
<0.001 |
0.93 |
0.12 |
3504 |
21* |
3611 |
4 |
27** |
3 |
101 |
30 |
<0.001 |
0.93 |
0.11 |
3497 |
14* |
3601 |
5 |
24** |
4 |
126 |
34 |
<0.001 |
0.90 |
0.20 |
3518 |
35* |
3609 |
9 |
50** |
5 |
145 |
28 |
<0.001 |
0.88 |
0.10 |
3549 |
66* |
3659 |
3 |
71** |
In general, the results suggest that the alternate models (2 through 5), which removed the reciprocal causation relationship between OCSE and chemistry performance fit the data significantly worse than the reciprocal causation model (Model 1). Fit indices such as chi-square (χ2), Comparative Fit Index (CFI), and the Standardized Root Mean Square Residual (SRMR) are used to assess the fitness of the model to the data. The criteria of CFI greater than 0.95 and SRMR value less than 0.05 were used to indicate a good model fit, while CFI > 0.90 and SRMR < 0.08, indicate an acceptable fit. The chi-square (χ2) test for model 1 indicates a lack of model fit: χ2 (n = 173, df = 25, p < 0.001) = 75; however, the χ2 test is sensitive to sample size, thus other fit indices that are insensitive to sample size are used to assess the fit as well (Bentler and Bonett, 1980; Jöreskog and Sörbom, 1993). The other fit indices, CFI = 0.95 and SRMR = 0.056, indicate that the reciprocal causation model fits the data reasonably well. For Models 2 and 3, the two unidirectional models, the model fit is worse, especially when we consider SRMR values, which increase to 0.12 and 0.11, respectively. CFI values also get worse, but the values are still in the acceptable range. For Model 4, the autoregressive model, we observed that the CFI value decreases to 0.90 and the SRMR value increases to 0.20. Both values indicate that this model does not fit our data well. The last model, Model 5, has the lowest CFI value of all models (CFI = 0.88) and the SRMR is 0.10. The results for these fit indices suggest that not only is organic chemistry self-efficacy influenced by performance, but also, performance influences how confident a student perceives themself in completing organic chemistry tasks.
Table 3 also presents results for a statistical comparison of the different models tested (Pekrun et al., 2014). This is performed using a scaled difference chi-square test to compare the fitness of nested models when the MLR estimation approach is used (Satorra and Bentler, 2001). In this comparison, the change in χ2(Δχ2) is relative to the change in degrees of freedom (Δdf) for the models, from Model 1 (reciprocal causation) to the alternative models (Models 2 through 5), which have more constraints. With constraints added, the χ2 change indicates a worse model fit. If the change in the χ2 (SBΔχ2) is not significantly large with the change in df, the null hypothesis fails to be rejected and the model with the added constraint for that step is tenable. In our case, the SBχ2 change is significant for the alternative models, further supporting the reciprocal causation model. Moreover, the AIC and the sample size adjusted BIC were lower for the reciprocal model. The difference in AIC (ΔAIC) values are all greater than 10, indicating that the alternate models 2–5 fail to account for a substantial portion of explainable variance in this dataset (Akaike, 1974; Schwarz, 1978; Burnham and Anderson, 2003; Pekrun et al., 2014). This finding suggests that the reciprocal model is preferable to the constrained model. Overall, these results indicate that the reciprocal causation model could be accepted as a model to better understand the interconnected relationship between self-efficacy and performance in organic chemistry courses.
Reciprocal causation model: model effects
After comparing the different models, the reciprocal causation model fits the data better than the alternate models; therefore, the reciprocal causation model is reasonable to understand the relationship between students' organic chemistry self-efficacy and their performance in organic chemistry. The reciprocal causation model along with the standardized parameter coefficients are shown in Fig. 4. The standardized parameter coefficients for OCSE are positive, significant and increase over time. Their values ranged from 0.49 at time 1 to 0.79 at time 5. This positive relationship suggests that students' confidence at the beginning of the semester and before any examination is related to the next measure of self-efficacy. In general, students' confidence beliefs in completing a specific organic task at one time predict their confidence at the subsequent time point across the semester.
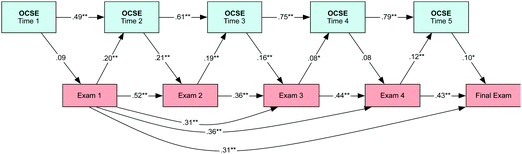 |
| Fig. 4 Results for Model 1 (reciprocal effects model). *p < 0.05. **p < 0.01. | |
The standardized path coefficients for the performance measures (Exam 1 to Final Exam) are also shown in Fig. 4. These values are positive and significant, and range from 0.36 to 0.52. There is also a significant positive relationship between Exam 1 and the subsequent exams (Exams 2–4 and Final Exam), which indicates that students' performance on their first exam predicts their performance on the other exams. This influence was already predicted from the strong Pearson correlations (see Table 2) between the exam measures. The strong relationship between Exam 1 and the other exams is indicative of the cumulative nature of the organic chemistry curriculum where the foundational tasks covered during the first examination are needed to perform well in the other exams.
The path coefficients between OCSE and Exams were also estimated. These standardized coefficients are smaller than what was observed for the repeated measures of OCSE (OCSE 1–5) and Exams (Exams 1–4, Final Exam); however, these relationships are significant. The strongest influences were observed between Exam 1 and OCSE 2 and between OCSE 2 and Exam 2, 0.20 and 0.21, respectively. In both cases, we can see that there is a moderate influence. These results indicate that students' confidence in completing the organic chemistry tasks at time 2 is influenced by their performance in the first exam (Exam 1), but at the same their OCSE 2 will influence their performance on the next exam (Exam 2). Also, we observed that the reciprocal relationships between Exam 2 and OCSE 3 and between OCSE 3 and Exam 3 are 0.19 and 0.16, respectively. The path coefficients are smaller and nonsignificant between Exam 3 and OCSE 4 and OCSE 4 and Exam 4; however, at the end of the semester, a small but significant influence of Exam 4 on OCSE 5 is observed and at the same time on OCSE 5 of the Final Exam.
The results obtained using this model provide empirical evidence for a reciprocal causation relationship between self-efficacy and performance in organic chemistry. The findings add to the research literature by documenting that self-efficacy positively predicts students' performance in organic chemistry over time. This is consistent with previous research that has shown that self-efficacy and performance in science and in chemistry are positively correlated (Lalich et al., 2006; Uzuntiryaki-Kondakci and Capa-Aydin, 2013; Uzuntiryaki-Kondakci and Senay, 2015). However, the results from this study go beyond correlational evidence by using a reciprocal causation model, testing the directional effects between self-efficacy and performance across a semester of an organic chemistry course. Specifically, the results obtained using the model indicate that in general organic chemistry self-efficacy has a positive influence on students' chemistry performance as measured by exams and the final exam. These positive effects are in line with propositions derived from theory that posits students' confidence will have a positive effect on learning and performance outcomes (Bandura, 1986; Britner and Pajares, 2001).
These results add to our understanding of factors that affect students' self-efficacy. The findings from this study indicate that performance, in turn, had positive effects on self-efficacy, implying that doing well in the exams can enhance students' self-efficacy, whereas doing poorly will lower the self-efficacy. This is consistent with the theory that the experience of mastery is an important source of a person's self-efficacy (Bandura, 1977, 1994) and consistent with research findings (Britner and Pajares, 2006; Hutchison et al., 2006; Tschannen-Moran and Hoy, 2007; van Dinther et al., 2011). Our findings extend the literature on how positive experiences increase students' self-efficacy and at the same time how increased confidence has a positive impact on performance.
Conclusion and implications
Self-efficacy is a construct that has been linked to performance and retention in STEM-related careers. Self-efficacy has been proposed as an important factor and predictor of science performance; but performance also has been proposed as a factor that influences the self-efficacy of an individual. In this study, the interconnected relationship between self-efficacy and performance in an organic chemistry course has been examined using a reciprocal causation model. This study is the first to introduce the reciprocal causation model in examining the interrelated relationship between students' chemistry self-efficacy in an organic chemistry course and performance throughout the course. This approach allows us to include the variable of interest measured at multiple times in a single model and have an overall picture of the quantitative relationship among the variables. Student empirical data in this study lend support to the hypothesis that self-efficacy has a consistent effect on examination performance and vice versa on the organic chemistry course.
Two research hypotheses were addressed in this study. The first examined different models to determine which model better explains the relationship between self-efficacy and performance in organic chemistry. Five different models, including the reciprocal causation model and four alternate models, were specified and tested. The comparison between the models suggest that a reciprocal causation model fits the data better, providing empirical support for the reciprocal relationship between self-efficacy and performance as proposed by the social cognitive theory (Bandura, 1986, 1997), whereby self-efficacy increases with positive experience and those experiences also impact subsequent self-efficacy (Dalgety and Coll, 2006).
The second hypothesis examined the effects of self-efficacy on performance and of performance on self-efficacy using the reciprocal causation model. The effects between self-efficacy and performance are small but positive, which indicate as hypothesized by theory that there is a direct influence on the effect of one on the other. As expected, the influence of a measure of OCSE on the subsequent OCSE measure is positive and significant; as well, the influence of exams on subsequent measures of performance is positive and significant. Finally, a snowball effect examined the impact of Exam 1 and its direct influence on Exams 2, 3 and 4 and the Final Exam. These effects are also positive and significant.
This study has its limitations. First, structural equation modeling was used to support the reciprocal causal relationship, but this approach does not provide powerful evidence for causation as an experimental design approach (Pekrun, 1992; Marsh et al., 2005; Pekrun et al., 2014). Second, the data were collected from a unique population of organic chemistry students in a single semester and at the same institution. Findings from this study might not be representative of other institutions and student populations. This study should be replicated with more students at multiple institutions for maximization of generalizability. Third, the sample size is small; which limits the complexity of the models being tested. A larger sample of students would have allowed for combining the OCSE measurement component with the reciprocal causation pathway analysis. Lastly, the assessment tool for organic chemistry self-efficacy and performance needs future improvement to establish evidence of reliability and validity of the scores to measure the intended constructs.
Despite the limitations in this study, there are several implications for chemistry education researchers and instructors. For researchers, this study has provided support for the reciprocal causation model as a way to gather evidence for the relationship between self-efficacy and performance. This model can be tested with other factors that have been hypothesized to effect performance such as motivation, interest, and effort beliefs. Also, previous studies have found that trends in self-efficacy can vary by sex and race/ethnicity groups (Villafañe et al., 2014); therefore with larger sample sizes, the models could be tested with different groups of students. Our findings have implications for instructional innovation. In order to improve student course performance, student self-efficacy in domain specific knowledge and skills should not be ignored. Instructional innovations have been used to improve student performance as well as self-efficacy (Gist et al., 1989). Quasi-experimental studies have been conducted for the effectiveness of instruction effort in STEM areas (Crippen and Earl, 2007; Nbina and Viko, 2010). In chemistry, the metacognitive self-assessment strategy and self-explanation have been used on students' chemistry self-efficacy and achievement at the levels of senior secondary school and college (Crippen and Earl, 2007; Nbina and Viko, 2010). Team discourse has been used to support self-efficacy and achievement of engineering students (Purzer, 2011); while training methods have been applied to high school students regarding the effect on computer self-efficacy (Chou, 2001). All of these instructional innovations are important to build or strengthen student confidence and help their performance at the same time.
Competing financial interest
The authors declare no competing financial interest.
Appendix
Table 4
Table 4 Variance and Covariance Matrix of Study Variables
|
OCSE 1 |
OCSE 2 |
OCSE 3 |
OCSE 4 |
OCSE 5 |
Exam 1 |
Exam 2 |
Exam 3 |
Exam 4 |
Final exam |
OCSE 1 |
0.39 |
0.20 |
0.16 |
0.17 |
0.17 |
0.72 |
1.52 |
1.60 |
1.94 |
1.50 |
OCSE 2 |
0.20 |
0.40 |
0.27 |
0.27 |
0.25 |
1.91 |
2.76 |
3.31 |
3.51 |
3.69 |
OCSE 3 |
0.16 |
0.27 |
0.39 |
0.31 |
0.30 |
2.22 |
3.18 |
3.14 |
4.10 |
4.45 |
OCSE 4 |
0.17 |
0.27 |
0.31 |
0.41 |
0.36 |
1.62 |
2.48 |
3.10 |
3.75 |
3.47 |
OCSE 5 |
0.17 |
0.25 |
0.30 |
0.36 |
0.45 |
1.95 |
2.69 |
3.61 |
4.55 |
4.34 |
Exam 1 |
0.72 |
1.91 |
2.22 |
1.62 |
1.95 |
151.49 |
91.51 |
90.42 |
141.61 |
143.71 |
Exam 2 |
1.52 |
2.76 |
3.18 |
2.48 |
2.69 |
91.51 |
167.80 |
101.31 |
146.59 |
137.59 |
Exam 3 |
1.60 |
3.31 |
3.14 |
3.10 |
3.61 |
90.42 |
101.31 |
168.20 |
160.45 |
169.96 |
Exam 4 |
1.94 |
3.51 |
4.10 |
3.75 |
4.55 |
141.61 |
146.59 |
160.45 |
331.96 |
232.92 |
Final Exam |
1.50 |
3.69 |
4.45 |
3.47 |
4.34 |
143.71 |
137.59 |
169.96 |
232.92 |
364.04 |
Acknowledgements
This material is based on support from the National Science Foundation (DUE-1432085), ACS Exams, and the University of South Florida. Any opinions, findings, and conclusions or recommendations expressed in this material are those of the authors and do not necessarily reflect the views of the National Science Foundation, ACS Exams, or the University of South Florida.
References
- ACS Examinations Institute, (2014), American Chemical Society Division of Chemical Education, First Term Organic Chemistry (Form 2014), Ames, IA: Iowa State University.
- Akaike H., (1974), A new look at the statistical model identification, IEEE Trans. Automat. Contr., 19(6), 716–723.
- Akbas A. and Adnan K., (2007), Affective factors that influence chemistry achievement (motivation and anxiety) and the power of these factors to predict chemistry achievement-II, J. Turkish Sci. Educ., 4(1), 10.
- American Educational Research Association, American Psychological Association, National Council on Measurement in Education, Joint Committee on Standards for Educational, and Psychological Testing (US), (2014), Standards for educational and psychological testing, Washington, DC: AERA Publication.
- Andrew S., (1998), Self-efficacy as a predictor of academic performance in science, J. Adv. Nurs., 27(3), 596–603.
- Arjoon J. A., Xu X. and Lewis J. E., (2013), Understanding the state of the art for measurement in chemistry education research: examining the psychometric evidence, J. Chem. Educ., 90(5), 536–545.
- Bandura A., (1977), Self-efficacy: toward a unifying theory of behavioral change, Psychol. Rev., 84(2), 191.
- Bandura A., (1986), Social foundations of thought and action: a social cognitive theory, Englewood Cliffs, NJ: Prentice Hall.
- Bandura A., (1994), Self-efficacy, Wiley Online Library.
- Bandura A., (1997), Self-efficacy: the exercise of control, New York: W. H. Freeman.
- Bauer C. F., (2005), Beyond “student attitudes”: chemistry self-concept inventory for assessment of the affective component of student learning, J. Chem. Educ., 82(12), 1864.
- Bauer C. F., (2008), Attitude towards chemistry: a semantic differential instrument for assessing curriculum impacts, J. Chem. Educ., 85(10), 1440–1445.
- Bentler P. M. and Bonett D. G., (1980), Significance tests and goodness of fit in the analysis of covariance structures, Psychol. Bull., 88(3), 588.
- Black A. E. and Deci E. L., (2000), The effects of instructors' autonomy support and students' autonomous motivation on learning organic chemistry: a self-determination theory perspective, Sci. Educ., 84(6), 740–756.
- Boz Y., Yerdelen-Damar S., Aydemir N. and Aydemir M., (2016), Investigating the relationships among students' self-efficacy beliefs, their perceptions of classroom learning environment, gender, and chemistry achievement through structural equation modeling, Res. Sci. Techonol. Educ., DOI: http://10.1080/02635143.2016.1174931.
- Britner S. L. and Pajares F., (2001), Self-efficacy beliefs, motivation, race, and gender in middle school science, J. Women Minor. Sci. Eng., 7(4), 15.
- Britner S. L. and Pajares F., (2006), Sources of science self-efficacy beliefs of middle school students, J. Res. Sci. Teach., 43(5), 485–499.
- Burnham K. P. and Anderson D. R., (2003), Model selection and multimodel inference: a practical information-theoretic approach, Springer Science and Business Media.
- Cheng S. and Chan A., (2003), The development of a brief measure of school attitude, Educ. Psychol. Meas., 63(6), 1060–1070. DOI: http://10.1177/0013164403251334.
- Cheung D., (2015), The combined effects of classroom teaching and learning strategy use on students' chemistry self-efficacy, Res. Sci. Educ., 45(1), 101–116.
- Chou H.-W., (2001), Effects of training method and computer anxiety on learning performance and self-efficacy, Comput. Hum. Behav., 17(1), 51–69.
- Cook A. F., (2013), Exploring freshmen college students' self-efficacy, attitudes, and intentions toward chemistry, (Honors College Capstone Experience/Thesis Projects), West Kentucky University.
- Cooper C. and Pearson P., (2012), A genetically optimized predictive system for success in general chemistry using a diagnostic algebra test, J. Sci. Educ. Technol., 21(1), 197–205.
- Cortina J. M., (1993), What is coefficient alpha? An examination of theory and applications, J. Appl. Psychol., 78(1), 98–104.
- Crippen K. J. and Earl B. L., (2007), The impact of web-based worked examples and self-explanation on performance, problem solving, and self-efficacy, Comput. Educ., 49(3), 809–821.
- Cukrowska E., Staskun M. G. and Schoeman H. S., (1999), Attitudes towards chemistry and their relationship to student achievement in introductory chemistry courses, South Afr. J. Chem., 52(1), 8–14.
- Dalgety J. and Coll R. K., (2006), Exploring first-year science students' chemistry self-efficacy, Int. J. Sci. Math. Educ., 4(1), 97–116. DOI: http://10.1007/s10763-005-1080-3.
- De Lange A. H., Taris T. W., Kompier M. A., Houtman I. L. and Bongers P. M., (2004), The relationships between work characteristics and mental health: examining normal, reversed and reciprocal relationships in a 4-wave study, Work and Stress, 18(2), 149–166.
- Ferrell B. and Barbera J., (2015), Analysis of students' self-efficacy, interest, and effort beliefs in general chemistry, Chem. Educ. Res. Pract., 16(2), 318–337.
- Gist M. E., Schwoerer C. and Rosen B., (1989), Effects of alternative training methods on self-efficacy and performance in computer software training, J. Appl. Psychol., 74(6), 884.
- Holme T., Emenike M., Hughes S., Linenberger K., Luxford K. and Raker J., (2015), Are content tests all the assessment we need?, in Orna M. V. (ed.) Sputnik to smartphones: a half-century of chemistry education, ACS Symposium Series, Washington, D.C.: American Chemical Society.
- House D. J., (1995), Noncognitive predictors of achievement in introductory college chemistry, Res. High. Educ., 36(4), 473–490.
- Hu L. and Bentler P. M., (1999), Cutoff criteria for fit indexes in covariance structure analysis: conventional criteria versus new alternatives, Struct. Equat. Model., 6, 1–55.
- Hutchison M. A., Follman D. K., Sumpter M. and Bodner G. M., (2006), Factors influencing the self-efficacy beliefs of first-year engineering students, J. Eng. Educ., 95(1), 39–47.
- Jansen M., Scherer R. and Schroeders U., (2015), Students' self-concept and self-efficacy in the sciences: differential relations to antecedents and educational outcomes, Contemp. Educ. Psychol., 41, 13–24.
- Jonge J., Dormann C., Janssen P. P., Dollard M. F., Landeweerd J. A. and Nijhuis F. J., (2001), Testing reciprocal relationships between job characteristics and psychological well-being: a cross-lagged structural equation model, J. Occup. Organ. Psychol., 74(1), 29–46.
- Jöreskog K. G. and Sörbom D., (1993), LISREL 8: structural equation modeling with the SIMPLIS command language, Scientific Software International.
- Kalaycioglu D. B., (2015), The influence of socioeconomic status, self-efficacy, and anxiety on mathematics achievement in England, Greece, Hong Kong, the Netherlands, Turkey, and the USA, Educ.
Sci. Theory Pract., 15(5), 1391–1401.
- Kan A. and Akbas A., (2006), Affective factors that influence chemistry achievement (attitude and self efficacy) and the power of these factors to predict chemistry achievement-I, J. Turkish Sci. Educ., 3(1), 30.
- Lalich I. J., Taylor M. J. and Pribyl J. R., (2006), Identification of the correlation between student self-efficacy and final course percentage in a general chemistry course, Paper presented at the conference in Minnesota State University, Mankato (April, 2006).
- Larson L., Pesch K., Surapeni S., Bonitz V., Wu T. and Werbel J., (2015), Predicting graduation: the role of mathematics/science self-efficacy, J. Career Assessment, 23(3), 399–409.
- Lawson A. E., Banks D. L. and Logvin M., (2007), Self-efficacy, reasoning ability and achievement in college biology, J. Res. Sci. Teach., 44(5), 706–724.
- Lewis S. E., Shaw J. L., Heitz J. O. and Webster G. H., (2009), Attitude counts: self-concept and success in general chemistry, J. Chem. Educ., 86(6), 744.
- Ma X., (1997), Reciprocal relationships between attitude toward mathematics and achievement in mathematics, J. Educ. Res., 90(4), 221–229.
- Margolis H. and McCabe P. P., (2003), Self-efficacy: a key to improving the motivation of struggling learners, Prev. Sch. Fail., 47(4), 162–169.
- Marsh H. and O'Mara A., (2008), Reciprocal effects between academic self-concept, self-esteem, achievement, and attainment over seven adolescent years: unidimensional and multidimensional perspectives of self-concept, Pers. Soc. Psychol. Bull., 34(4), 542–552.
- Marsh H., Trautwein U., Lüdtke O., Köller O. and Baumert J., (2005), Academic self-concept, interest, grades, and standardized test scores: reciprocal effects models of causal ordering, Child Dev., 76(2), 397–416.
- Miller K., Schell J., Ho A., Lukoff B. and Mazur E., (2015), Response switching and self-efficacy in Peer Instruction classrooms, Phys. Rev. ST Phys. Educ. Res., 11(1), 010104.
- Multon K. D., Brown S. D. and Lent R. W., (1991), Relation of self-efficacy beliefs to academic outcomes: a meta-analytic investigation, J. Couns. Psychol., 38(1), 30.
- Murphy K. R. and Davidshofer C. O., (2005), Psychological testing: principles and testing, 6th edn, Upper Sadler River, New Jersey.
- National Science Foundation, National Science Board, (2003), The science and engineering workforce realizing America's potential. Retrieved from http://www.nsf.gov/nsb/documents/2003/nsb0369/nsb0369.pdf.
- National Science Foundation, National Science Board, (2010), Preparing the next generation of STEM innovators: identifying and developing our nation's human capital. Retrieved from http://www.nsf.gov/nsb/publications/2010/nsb1033.pdf.
- Nbina J. B. and Viko B., (2010), Effect of instruction in metacognitive self-assessment strategy on chemistry students' self-efficacy and achievement, Academia Arena, 2(1), 1–10.
- Pajares F., (1996a), Self-efficacy beliefs and mathematical problem-solving of gifted students, Contemp. Educ. Psychol., 21(4), 325–344.
- Pajares F., (1996b), Self-efficacy beliefs in academic settings, Rev. Educ. Res., 66(4), 543–578.
- Pajares F., (2002), Gender and perceived self-efficacy in self-regulated learning, Theor. Pract., 41(2), 116–125.
- Pajares F. and Schunk D., (2001), The development of academic self-efficacy, in Wigfield A. and Eccles J. (ed.) Development of achievement motivation, San Diego: Academic Press.
- Parker P. D., Marsh H. W., Ciarrochi J., Marshall S. and Abduljabbar A. S., (2014), Juxtaposing math self-efficacy and self-concept as predictors of long-term achievement outcomes, Educ. Psychol., 34(1), 29–48. DOI: http://10.1080/01443410.2013.797339.
- PCAST, (2012), Report to the President: Engage to excel: Producing one million additional college graduates with degree in science, technology, engineering, and mathematics. Retrieved from http://https://www.whitehouse.gov/sites/default/files/microsites/ostp/pcast-engage-to-excel-final_2-25-12.pdf.
- Pekrun R., (1992), Expectancy-value theory of anxiety: overview and implications, in Forgays D. G., Sosnowski T. and Wrzesniewski K. (ed.) Anxiety: recent developments in cognitive, psychophysiological, and health research. Series in health psychology and behavioral medicine, Washington, DC, US: Hemisphere Publishing Corp.
- Pekrun R., Hall N. C., Goetz T. and Perry R. P., (2014), Boredom and academic achievement: testing a model of reciprocal causation, J. Educ. Psychol., 106(3), 696.
- Peters M. L., (2013), Examining the relationships among classroom climate, self-efficacy, and achievement in undergraduate mathematics: a multi-level analysis, Int. J. Sci. Math. Educ., 11(2), 459–480.
- Purzer S., (2011), The relationship between team discourse, self-efficacy, and individual achievement: a sequential mixed-methods study, J. Eng. Educ., 100(4), 655–679.
- Satorra A. and Bentler P. M., (2001), A scaled difference chi-square test statistic for moment structure analysis, Psychometrika, 66(4), 507–514.
- Schwarz G., (1978), Estimating the dimension of a model, Ann. Stat., 6(2), 461–464.
- Scott F. J., (2012), Is mathematics to blame? An investigation into high school students' difficulty in performing calculations in chemistry, Chem. Educ. Res. Pract., 13(3), 330–336.
- Scherer R., (2013), Further evidence on the structural relationship between academic self-concept and self-efficacy: on the effects of domain specificity, Learn. Individ. Differ., 28, 9–19.
- Seery M. K., (2009), The role of prior knowledge and student aptitude in undergraduate performance in chemistry: a correlation-prediction study, Chem. Educ. Res. Pract., 10(3), 227–232.
- Tschannen-Moran M. and Hoy A. W., (2007), The differential antecedents of self-efficacy beliefs of novice and experienced teachers, Teach. Teach. Educ., 23(6), 944–956.
- Usher E. L. and Pajares F., (2008), Sources of self-efficacy in school: critical review of the literature and future directions, Rev. Educ. Res., 78(4), 751–796.
- Uzuntiryaki E. and Aydin Y. C., (2009), Development and validation of chemistry self-efficacy scale for college students, Res. Sci. Educ., 39(4), 539–551.
- Uzuntiryaki-Kondakci E. and Capa-Aydin Y., (2013), Predicting critical thinking skills of university students through metacognitive self-regulation skills and chemistry self-efficacy, Educ. Sci. Theor. Pract., 13(1), 666–670.
- Uzuntiryaki-Kondakci E. and Senay A., (2015), Predicting chemistry achievement through task value, goal orientations, and self-efficacy: a structural model, Croatian J. Educ., 17(3), 725–753.
- van Dinther M., Dochy F. and Segers M., (2011), Factors affecting students' self-efficacy in higher education, Educ. Res.
Rev., 6(2), 95–108.
- Villafañe S. M., Bailey C. P., Loertscher J., Minderhout V. and Lewis J. E., (2011), Development and analysis of an instrument to assess student understanding of foundational concepts before biochemistry coursework, Biochem. Mol. Biol. Educ., 39(2), 102–109.
- Villafañe S. M., Garcia C. A. and Lewis J. E., (2014), Exploring diverse students' trends in chemistry self-efficacy throughout a semester of college-level preparatory chemistry, Chem. Educ. Res. Pract., 15(2), 114–127.
- Wagner E. P., Sasser H. and DiBiase W. J., (2002), Predicting students at risk in general chemistry using pre-semester assessments and demographic information, J. Chem. Educ., 79(6), 749–755.
- Williams T. and Williams K., (2010), Self-efficacy and performance in mathematics: reciprocal determinism in 33 nations, J. Educ. Psychol., 102(2), 453.
- Xu X. and Lewis J. E., (2011), Refinement of a chemistry attitude measure for college students, J. Chem. Educ., 88(5), 561–568.
- Xu X., Villafane S. M. and Lewis J. E., (2013), College students' attitudes toward chemistry, conceptual knowledge and achievement: structural equation model analysis, Chem. Educ. Res. Pract., 14, 188–200.
- Zeldin A. L., Britner S. L. and Pajares F., (2008), A comparative study of the self-efficacy beliefs of successful men and women in mathematics, science, and technology careers, J. Res. Sci. Teach., 45(9), 1036–1058.
- Zusho A., Pintrich P. R. and Coppola B., (2003), Skill and will: the role of motivation and cognition in the learning of college chemistry, Int. J. Sci. Educ., 25(9), 1081–1094.
|
This journal is © The Royal Society of Chemistry 2016 |
Click here to see how this site uses Cookies. View our privacy policy here.