DOI:
10.1039/C6RA05406D
(Review Article)
RSC Adv., 2016,
6, 47844-47857
Risk assessment of environmental mixture effects
Received
1st March 2016
, Accepted 6th May 2016
First published on 9th May 2016
Abstract
In the environment, organisms are exposed to a diverse array of chemicals in complex mixtures. The majority of approaches that aim to assess the risk of environmental chemical mixtures, including those used by regulatory bodies, use toxicity data generated from the individual component chemicals to predict the overall mixture toxicity. It is assumed that the behaviour of chemicals in a mixture can be predicted using the concepts of concentration or dose addition for chemicals with similar mechanisms of action or response addition for dissimilarly acting chemicals. Based on empirical evidence, most traditional risk assessment methods, such as toxic equivalency factors and the hazard index, make the assumption that the components of a mixture adhere to the concentration addition model. Thus, mixture toxicity can be predicted by the summation of the individual component toxicities. However in some mixtures, interactions can occur between chemicals or at target sites that alter the toxicity so that it is more or less than expected from the constituents. Many regulatory and experimental methods for predicting mixture toxicity rely on the use of a concentration addition model so that if interactions occur in mixtures, the risk posed may have been significantly underestimated. This is particularly concerning when considering environmental mixtures which are often highly complex and composed of indeterminate chemicals. Failure to accurately predict the effects chemicals will have if released into the environment, where they can form mixtures, can lead to unexpected detrimental effects on wildlife and ecosystems. The number of confounding factors that may alter the ecotoxicity of a mixture and the accuracy of predictive methods makes risk assessment of environmental mixtures a complex and intimidating task. With this in mind, this review aims show why accurate risk assessment of mixtures is vital by demonstrating the effect they can have on organisms in the environment. Furthermore, it also aims to look at the current challenges facing the assessment of mixture effects and examines future areas of focus that seek to develop methodologies more suitable for environmental mixtures.
1. Introduction
Most studies only consider the toxicity of chemicals in isolation whereas in the environment, organisms are exposed to a large number of different chemicals at the same time. The assumption of mixture toxicity based on the individual component data can lead to a significant under- or over-estimation of the potential risk that a mixture may present. This is particularly pertinent where regulatory bodies must make assessments on the usage of chemicals that may be used in the environment or have the potential for release into it. It has long since been acknowledged that the presence of a chemical in a mixture and its known toxicity in isolation is no guarantee of the effects when combined with other agents. Although considerable focus has shifted towards looking at mixtures as a whole, there are still gaps in our knowledge and our ability to assess them as such. It is not necessarily possible to know which combinations of chemicals will arise in the environment or to test for the innumerable agents and mixtures which may occur. Although environmental mixtures are often highly complex and vast in composition, a recent review of mixture studies performed by Kortenkamp et al. found that of the experiments considered, less than 25% looked at mixtures with seven or more agents.1 With this in mind, the aim of this review is to look at how components in a mixture may interact and what effect this can have on organisms in the environment when they are exposed, simultaneously, to different pollutants. It will also consider current approaches used to evaluate the effect of such mixtures in order to understand how this impacts the assessment of risk that pollutants can pose to the environment.
2. Reasons for ecotoxicity risk assessment of mixtures
Around the world, environmental pollutants are found in various matrices and can affect organisms at any trophic levels in ecosystems of all different kinds. Research has shown that anthropogenic pollution can cause morbidity and mortality in organisms by affecting processes such as reproduction and development.2,3 Although some exist transiently and will degrade, other chemicals are very stable and will remain in the environment for a long period of time. Such chemicals are of particular concern for top level predators that accumulate pollutants and are considered particularly important to ecotoxic risk assessment due to their longevity and resultant possibility for exposure to combination mixtures.4 It is clear that environmental pollution can have a significant effect on wildlife even before the consideration of mixture effects which adds a further level of complexity.
Environmental mixtures typically exist at fairly low levels, the exact composition is often not known and there may be other external, non-chemical stressors that play a role in the combined effects too. Although not all mixtures will have ecotoxic effects, those that do can be significantly damaging to wildlife. There are a huge number of potential chemical combinations and it is not practical nor always possible to test them all for ecotoxic effects, so there is an obvious need for robust approaches to assess toxicity.5 A common school of practice, often used for regulatory framework, is that if individual components of a mixture fall below certain toxicity or concentration thresholds then the overall mixture will be ‘safe’. It is easy to see from the available literature, that this does not always match the real world situation and if components of a mixture interact the overall risk to environmental organisms can be much greater or smaller than expected. There are many classes of pollutants that can form mixtures in the environment and to consider just three of these groups, heavy metals, pharmaceutical drugs and pesticides, serves to illustrate the importance of accurate assessment of environmental mixtures.
2.1. Heavy metals
Heavy metals are a major source of environmental pollution caused by anthropogenic activities and are well known as a considerable risk to the health of wildlife. Both single toxicant studies,6–8 and those looking at mixtures have shown that due to wide industrial use, heavy metal pollution spans many ecosystems and many aquatic, marine and terrestrial organisms are constantly exposed to complex metal mixtures and the associated toxicities.9,10 There are numerous studies showing that metals in mixtures that are found in the environment or mixtures that simulate them are toxic to environmental organisms. The studies show that the mixtures affect organisms at all tropic levels of the ecosystem. Due to the nature of heavy metal pollution, aquatic ecosystems are particularly at risk. Binary mixture studies of nickel, cadmium and lead as well as arsenic and cadmium increase mortality rates and cause immobilisation in Daphnia magna, a sentinel aquatic organism.11,12 Combinations of cadmium, copper and zinc alter the filtration rate of Dreissena polymorpha, a freshwater zebra mussel.13,14 In frogs, co-exposure to cadmium and chromium can cause increased accumulation of heavy metals in the kidneys.15 In fat head minnows, a mixture of six different heavy metals that were each at individual water criterion levels was found to impair growth.10 It is clear even from a small sample of the available data, that mixtures of heavy metals represent a risk of considerable concern.
Such studies have also highlighted that it is not always straightforward to predict the toxicity of heavy metal mixtures from tests that use single chemicals and the toxicity of a mixture can be greater or less than expected. Some mixtures of heavy metals are relatively simple to assess, for example D. magna exposure to copper, cadmium, lead and zinc mixtures have been accurately predicted a number of times,16,17 but toxicity predictions of other heavy metal mixtures have been incorrect due to effects on additional pathways that are not involved in single treatments.11,12 Due to their chemistry, many heavy metal species will interact with each other as well as with other chemicals and biological structures rather than simply exerting their own toxic effects. A comprehensive analysis looking at all possible combinations of some of the most common heavy metal contaminants (copper, lead, zinc and cadmium) in a sea urchin assay found that in the majority of mixture combinations, the metals interacted.18 In many cases, heavy metal interactions increase the toxic effects such mortality rate in lavae,19 renal damage,20 embryonic toxicity and spermiotoxicity18 but some also yield a decrease in expected toxicity.21,22 There are also many other factors that influence the overall toxicity of a heavy metal mixture such as whether exposure is acute or chronic.10 Derivations from the expected toxicity cause concern that current prediction and assessment methods are not adequate and may lead to toxic mixtures that will harm biota in the natural environment.
Heavy metal mixtures are not limited to aquatic environments, their effects are also seen in terrestrial ecosystems. Heavy metals have exhibited interactions that alter the expected mixture toxicity in a number of terrestrial species such as isopods,23 earthworms24 and nematodes.25 There is little experimental data from higher vertebrates on which to base ecotoxic risk assessment due to the difficulty of working with species that have more complex biological systems, longer life spans and the push to reduce animal testing. Given our knowledge that single agent heavy metal exposure causes detrimental health effects in birds26–28 and mammals,29 we can assume that at least some heavy metal mixtures will also have deleterious effects, potentially greater than would be expected from individual exposure data. To add a further level of concern, the co-exposure of metals with other types of pollutants can lead unique combination effects. Studies that have focused on the effect of heavy metals in combination with other chemicals have found that they can interact with other agents, particularly pesticides, which can also lead to altered mixture toxicity.30–32
2.2. Pharmaceutical drugs
Pharmaceutical chemicals are designed with their effect on humans and animals in mind and specific care is taken to evaluate the risks of mixing with other chemicals or drugs. The risk of unpredicted, unregulated mixture effects is low during their intended use but the potential for release into the environment is significant and thus there is a great risk of such agents becoming components of toxic mixtures in the environment.33 Due to frequent usage and the nature of pharmaceutical excretion into bodily fluids, which enter waste water treatment plants, mixtures of pharmaceuticals in water bodies are often highly complex with multiple interactions occurring between chemicals.34–36 The vast range of pharmaceutical compounds that make their way into the environment makes predicting such interaction difficult: non-steroidal anti-inflammatory drugs (NSAIDs), hormones used as contraceptives, medications, antimicrobials and more are all frequently detected.37
Experimental evidence demonstrates that often mixtures of such drugs have unexpected toxicity compared to individual chemicals.38 In a study using D. magna, the toxicity of a mixture of NSAIDs, including ibuprofen and acetylsalicylic acid, was significant even at levels where the individual drugs showed little toxicity.39 Similar results have also been found with other types of drugs. For example, one study found that clofibrinic acid, a cholesterol lowering drug, alone caused 1% of D. magna to become immobilised and carbamazepine, an anticonvulsant, alone immobilised 16% but in a mixture, they caused the immobilisation of 95% of the organisms.40 This represents a significantly greater toxicity than expected, which would have been underestimated using traditional models. Another study showed that clofibrinic acid and fluoxetine, an antidepressant, cause higher rates of death and deformity than predicted by the single agents.41 Antimicrobial mixtures have also been a source of concern as interactions between agents is a fairly common occurrence. In fact, some antibiotics are purposely used in combination in order to enhance their efficacy thus increasing the likelihood that antimicrobial mixtures will be more toxic than expected.42 The intended usage of such agents is to inhibit or kill bacteria but when released into the environment, antimicrobial mixtures are potentially toxic to many non-target bacterial species as well other microorganisms that are critical to ecosystems.43–45
Although much of the work looking at pharmaceutical mixtures focuses on lower trophic organisms, in the environment, this often confers risk throughout the ecosystem.46,47 Evidence shows that mixtures of these agents also endanger higher species such as fish and birds.48–50
2.3. Pesticides
The term ‘pesticide’ covers a wide range of compounds and chemicals and refers to one of the most commonly used category of pollutants, representing a multibillion dollar industry. Pesticides are designed to be toxic to at least one species which has meant they have been fairly well-studied in order to define risk assessment legislature related to their use. Different types of pesticides are often purposefully used in mixtures in order to target ‘pests’ or to increase efficiency so co-exposures may occur to non-target species right from the time of release. Due to this, a substantial amount of work has gone into investigating the effects of pesticide mixtures on wildlife. It has been shown that mixtures of commonly used pesticides can increase mortality in bees51 and even pesticide solvents can be toxic when combined with insecticide agents.52 They can have adverse effects of many fish species including damaging their olfactory tissues which are essential for processes such as migration and predator detection.53–55 It has also been suggested that aquatic pesticide mixtures can lower the immune responses of bivalves thus putting them at risk of bacterial infections56 and are highly toxic to estuarine amphipods.57 Pesticide mixtures have been an area of particular worry in amphibian research due to the exposure risk inherent in having both amphibious and terrestrial life cycle stages. Insecticide mixtures have been found to dramatically increase the mortality rate of frog species58 and slow larval growth.59,60
As with many types of chemical mixtures, there is an increasing body of data showing that some pesticides will be more toxic when in mixtures than expected based on the component chemical toxicities and so traditional assessment methods may miscalculate the risk to the environment.61 For example a study looking at salmon, a species considered under ecological threat, found that sub-lethal combinations of organophosphate and carbamate pesticides exhibit considerable synergy of acetylcholinesterase inhibition, which is essential for salmon survival.62 Another found that commonly used fungicides that are often used simultaneously or in close proximity, can cause up to a twelve-fold increase in immobilization of D. magna than that predicted by single chemicals.57 The usage of many pesticides that are damaging to the environment is now restricted or banned and so regulatory mixture assessments only apply to current-use chemicals. However, many pesticides are persistent in the environment and recent research has shown that legacy pesticides can still be found at levels that are capable of causing ecotoxic effects.63 It is an issue of significant concern that there may be environmental organisms that are exposed to mixtures containing ecotoxic pesticides, which are not captured in current evaluations of mixture safety.
3. Mode of effects of chemical mixtures
Early work in the field, from the 1930's onwards, led to the development of the three main models of mixture effects (see Fig. 1). The first two were termed concentration addition and independent action64,65 and these apply where there is no interaction between the components in a mixture. The third category is applied to mixtures where there is interaction between the component chemicals leading to potentiation or antagonism of toxicity.66 In the environment, mixtures are not always made up of simply similarly or dissimilarly acting chemicals and during risk assessment, all three models may need to be considered particularly for more complex mixtures.
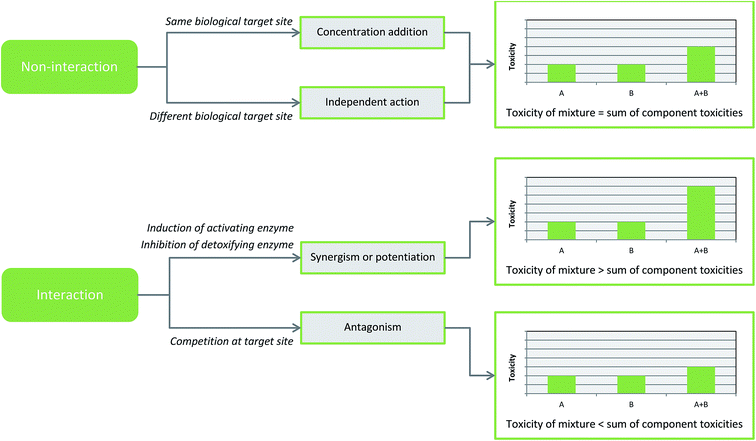 |
| Fig. 1 Schematic demonstrating the theoretical models of chemical mixture effects. | |
3.1. Concentration addition and independent action
Both concentration addition and independent action use the model of non-interaction so mixture toxicity is predicted based on the assumption that components within a mixture will not interact or interfere with each other. In theory, a concentration addition model also known as an additive model applies to chemicals in a mixture where the summation of the individual component toxicities is equal to the toxicity of the mixture as a whole.67 The model assumes that the different agents in the mixture share the same mechanism of toxicity and the same target site but are diluted by each other and so do not influence each other's toxicity. Therefore, all components of the mixture contribute to the total toxicity depending on their concentration and potency, so that even if all components are at levels below the toxicity threshold, the overall mixture would have toxicity due to the additive effect.68 Many approaches towards mixture risk assessment, often used by regulatory bodies, work on the assumption that a mixture of chemicals will have a concentration additive toxic effect.69 A good example of a class of chemicals that generally adhere to the assumptions of concentration addition are xenoestrogens which can have significantly detrimental effects on the health of humans70 and wildlife.71,72 Studies have shown that even if the individual estrogenic compounds are at levels where no effect is observed (when applied alone), the overall mixture has toxic effects due to concentration addition.73,74
Independent action, sometimes called response addition, refers to chemicals that have different modes of toxicity and so affect different biological target sites. As the name suggests, the components of the mixture are acting independently and so they do not impact the toxicity of each other.75 Thus, in a mixture where the components exhibit independent action, it is expected that the overall mixture would have no toxicity providing that all the individual agents are at subtoxic levels. In terms of risk assessment, a mixture is often assumed to exhibit independent action if there is evidence to show the toxicity is not additive.76 Unlike concentration addition, which has been well-studied in a range of different organisms and with multiple chemicals, there is considerably less literature on the potential of independent action and its accuracy.77 The studies that have been done, mainly focus on microorganisms, bacteria78,79 and algae,80 have found that independent action is a reliable way to predict the effect of dissimilarly acting chemicals in a mixture and is more accurate for such chemicals than concentration addition. More recently, studies looking at higher organisms have also found similar results.81
3.2. Interactions
In some cases, relatively few yet environmentally significant, the toxicity of a mixture differs from that expected using the assumptions of concentration addition or independent action. In these cases, mixture components influence each other to result in the overall toxicity being stronger or weaker than predicted. This is due to interactions. When the mixture toxicity exceeds that of the individual chemicals together, it is known as synergy or potentiation. Multiple studies have shown that heavy metals, in particular, often have potentiated toxicity due to interaction with each other in mixtures.82–84 The term potentiation is sometimes used interchangeably with the word synergism but they each refer to distinct occurrences. Mixture toxicity is described as synergistic if only one compound is present at a toxic level and the other components in a mixture are present at subtoxic levels.67,85 Therefore, the chemical present at a subtoxic level would have no effect if applied in isolation and only has toxicity in the context of the mixture. This component would be known as the synergist.86 Potentiation or synergism occurs when a chemical in a mixture alters the way another is metabolised. In a complex mixture it is possible that there may be more than one interaction where metabolism is changed making the overall outcome even harder to predict. There are two main ways in which a chemical can affect the metabolism of the other. The first is if it causes a second chemical to be activated quicker; this usually happens as a result of the first chemical inducing the expression of enzymes that are involved in the activation of a second chemical. The second way that metabolism can be altered is when a chemical prevents another from being degraded by inhibiting an enzyme, or its expression, that is involved in detoxification.67,87
Carbon disulphide is a well-studied hepatotoxic pollutant that exhibits synergistic toxicity in certain mixtures. It can cause considerably greater levels of toxicity than predicted by concentration or response addition due to its influence on mixed function oxidases (MFOs) detoxifying enzymes, specifically cytochrome P450 (CYP) enzymes.88 CYP2E1 enzymes metabolise and activate carbon sulphide89 and in the presence of chemicals that also induce the expression of CYP enzymes, potentiation of carbon disulphide toxicity occurs as it is activated significantly quicker than if applied alone. This effect happens in both simultaneous and sequential exposures.90 Another example of altered metabolism leading to potentiation is that of malathion, an extensively used pesticide that is often contaminated during manufacture with an impurity called isomalathion. In combination, a mixture of the two has greater than expected cytotoxicity and genotoxicity91 due to inhibition of carboxylesterases, another family of detoxification enzymes, by isomalathion. As detoxification of malathion is significantly slower, mixture toxicity is enhanced.92
The second type of interaction is known as antagonism. This occurs when toxicity of a mixture is less than expected using a concentration or response addition model, i.e., less than that of its individual components. This can happen due to direct interaction between chemicals, competition at receptors or altered metabolism.93 A well-studied, environmental example of antagonism is the interaction between mercury and selenium. The presence of selenium is thought to reduce the assimilation of methylmercury in the body thus reducing its toxicity.94 The selenium is able to sequester the mercury and so decreases its bioavailability and ability to cause toxic effects. Sequestration prevents the action of selenium-dependant enzymes which contain sulphur molecules essential for a significant part of the toxicity of mercury.95,96 In ecotoxic risk assessment, antagonistic interactions are not as concerning as synergistic or potentiated interactions as the toxicity of the mixture is underestimated rather than being more dangerous than expected.
4. Current approaches to mixture risk assessment
Environmental risk assessment is something of a complicated task; consideration of multiple chemicals, species, mechanisms of action, exposure ranges etc. all have their part to play and may be needed for the risk to be accurately predicted. In a laboratory situation, it is impractical if not impossible to experimentally recreate and test each combination of chemicals, which might be found in the environment. For this reason, robust predictive models are critical to our understanding of how pollutants interact so that they can be appropriately evaluated. There are two ways to approach the risk assessment of a mixture: (a) looking at the whole mixture; or, (b) using data on the component chemicals. Fig. 2 demonstrates the data used in both whole mixture and component based approaches and provides example risk assessment methods appropriate for each.
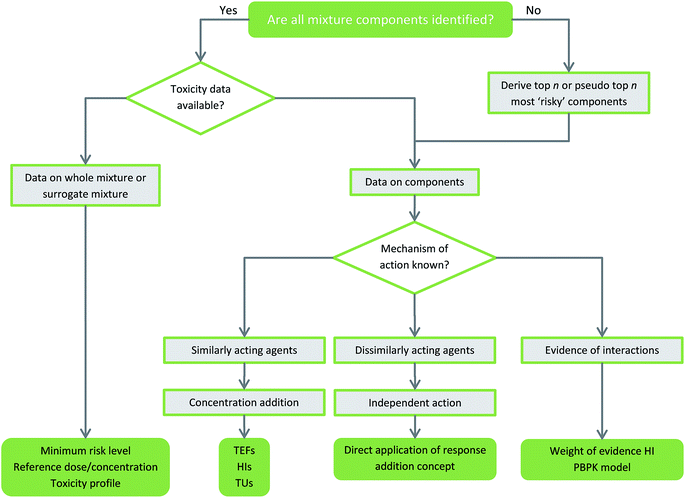 |
| Fig. 2 Flow chart of general guidance on the depth of toxicity data available for chemical mixtures and appropriate risk assessment methods. | |
4.1. Whole mixture based approaches
Whole mixture approaches, sometimes called top-down approaches, use toxicity data in the form of the biological response to an entire mixture or from fractions of it in the case of hydrocarbons mixtures.97 It is also possible to perform a risk assessment on a surrogate mixture if it is considered similar enough to the mixture under investigation. On initial consideration, whole mixture approaches may seem the most logical as they appear to best represent the simultaneous exposure that organisms in the environment encounter. A key advantage of this type of ecotoxic risk assessment is that by using the whole mixture, any interactions between the component chemicals that may have been missed in a component-based approach are accounted for.76 In an environmental setting, it is sometimes more appropriate to look at whole mixture data if the mix in question is poorly characterised, such as in sludge, sediment or effluent water;98 if there are any unknown or unidentified constituents, they are also captured in the assessment.
However, there are some important limitations; the information resulting from a whole mixture assessment is highly specific to that particular mixture and cannot be extrapolated to other mixtures or situations. It is only applicable to mixtures that are very stable in the environment as it does not account for any change in composition that is typical of complex mixtures. This type of approach does not generate any information on the mechanism of action of components within the mixture.99 It is only possible to determine if there is an additive response (from some assessments) but nothing further can be concluded about potential interactions. Even if a specific toxic endpoint such as genotoxicity is identified and measured, it is not possible to identify the toxicant responsible for this outcome. For ecotoxicity risk assessment, whole mixture approaches are often not achievable as it is not possible to extract or recreate an entire environmental mixture due to the sheer scale of potential component-based approaches.100
4.2. Component based approaches
When it is possible to identify all of the components in a mixture, risk assessment is often carried out using toxicity data on the constituent parts. Quantitative toxicity data is paired with risk and/or hazard classification to provide a full risk assessment. Usually, the type of mixture effect is determined using knowledge of the mode of action and this, along with the type of exposure and toxicity, directs the selection of the most appropriate risk assessment method.101 Due to availability of data and practicality restrictions, the mode of action might not be known so for environmental toxicity assessments, many methods assume a concentration addition model. Although component-based approaches solve many of the limitations of whole mixture assessments, the assumption of non-interaction in lieu of mode of action data may result in potentially ecotoxic mixture interactions being missed.102 However, evidence suggests that for the majority of cases, concentration addition is adequate to predict mixture toxicity. Commonly used component-based approaches that assume additivity include Relative Potency Factor (RPF) methods such as Toxic Equivalency Factors (TEFs), the Toxic Unit (TU) summation approach and the hazard index (HI).103 Herein, we will discuss a few of the most used methods, but Table 1 provides a more comprehensive list of approaches.
Table 1 Component-based methods of chemical mixture risk assessment approaches categorised by the assumed mechanism of action
Component-based approaches |
Concentration addition |
Independent action |
Interactions |
Direct application |
Direct application |
Weight of evidence HI |
Toxic Equivalency Factors (TEFs) |
|
Physiologically-based pharmacokinetic (PBPK) model |
Hazard Index (HI) |
|
Interaction profiles |
Relative Potency Factors (RPFs) |
|
|
Combined margin of exposures (MOETs) |
|
|
Point of departure index (PODI) |
|
|
The summation of TUs is the most direct application of the concentration addition model and it is extensively used in ecotoxicology risk assessment.104,105 The TU of a chemical is derived as a fraction where the concentration of the individual mixture component is divided by a toxic endpoint definer such as the EC50 (the dose at which a 50% effect is induced when that chemical is in isolation). For long-term exposures, the no observed effect level (NOEL) can also be used. The overall toxic unit of the mixture is calculated by the summation of the individual TUs.76,106
Risk assessment methods that use RPFs are generally used for groups of chemicals where the toxicity and dose–response of one particular individual chemical in the class is well-characterised and studied.107,108 This chemical is known as the index compound. Using the assumption that others in the same class have the same mechanism of action, the toxicity of the chemical under question is expressed relative to that of the index compound. RPFs can be considered scaling factors that allow toxicity to be defined relative to how much of the index compound that would be needed in order to generate the same toxicity as the investigated chemical.109 For evaluating mixture effects, RPFs assume a concentration addition model so the toxicity of a mixture can be calculated by adding up the equivalent index compound doses. The most commonly used type of RPF method is the TEF but a similar RPF known as the potency equivalency factor (PEC) has also been developed for use with more diverse groups of chemicals including polyaromatic hydrocarbons.107
TEFs are a specific type of RPF that are extensively used in environmental regulatory circumstances to assess the toxicity of dioxins, furans and other dioxin-like compounds, such as polychlorinated biphenyls (PCBs), that bind to the aryl hydrocarbon receptor.110,111 These chemicals have diverse ecotoxic properties and are highly persistent in the environment leading to accumulation up food chains. TEFs express the equivalent toxicity of these compounds, between 0.00001 and 1, relative to the most potent dioxin: 2,3,7,8-tetrachlorodibenzo-p-diozin (TCDD).112 For cumulative assessment, the sum of equivalent TCDD doses for each dioxin-like compound is used, which is generated by multiplying the dose by the TEF for that particular compound. Although highly useful, the TEF method makes some central assumptions that restrict the mixtures it can be applied to. The first is that the components of the mixture must exert toxicity via the aryl hydrocarbon receptor as the requirement for a similar mode of action validates the use of an additive approach.113 As TEFs can be used for human and wildlife mixture assessments, it also assumes that the chemicals behave the same or similarly in different species.114 Finally, it also assumes that equivalent toxicity between the chemicals and TCDD remains the same at all doses. Although these rules represent potential limitations, TEFs are regarded as easy to use and easier to standardise across compound classes as they are based on potency rather than exposure which can have many factors. There have been multiple studies that have shown that the TEF approach works well to accurately predict the toxicity of mixtures of dioxin-like substances.115,116
The HI is more often used in human health risk assessment but it is increasingly being used to investigate environmental mixtures, particularly those composed of pharmaceutical agents.117–120 A HI is generated by the addition of hazard quotients for each component chemical in a mixture. It is often used when the mechanism of action is not known as unlike the TEF approach, the HI does not use a RPF scaling factor built from exposure data but instead uses a minimum risk reference level, usually derived from benchmark doses or the no observed adverse effect level (NOAEL).121 Hazard quotients are derived by comparing the exposure of each chemical to a reference level that is specific for that compound.122 The HI is used for assessing mixtures of similarly acting compounds so an overall HI is generated by summing the hazard quotients. Calculating a HI gives a qualitative estimate of mixture risk and is stated in terms of whether it exceeds unity or not. A HI of a mixture that is more than one is considered to exceed acceptable levels of toxicity and is not ‘safe’ in the environment.123 The HI is considered to be more flexible than using TEFs as the exact mechanism of action does not need to be known. Also, different types of data can be used interchangeably as exposure data and acceptable limits as long as they are expressed in the same limits. The comparison to a reference level strengthens the HI approach as it is a well-characterised acceptable risk level and providing they are already derived, calculating the HI is relatively fast. However, this can also be a downside to the method as the way that the reference value is calculated may differ, due to the use of uncertainty factors, for each mixture component resulting in inconsistencies in the hazard quotients. Ideally, standardised data should be used for each chemical to solve this issue and make the HI a more robust tool.
For mixtures where the components have a similar mechanism of action and the compounds have a linear dose–response relationship, assessment of risk using either concentration addition or independent action should give the same estimations. Independent action is rarely used as an assumption for ecotoxicity assessment methods as previous evidence has suggested concentration addition is more applicable to mixtures. Recently however, it has been suggested that a combined, tiered approach may be more appropriate for environmental risk assessment. The initial stages are used to determine whether there is a need for further, higher tier testing. It has been proposed that the first tier involves the use of concentration addition assumptions and the higher tier uses independent action if needed.106,124
5. Challenges of environmental risk assessment
5.1. Interactions
As described above, the majority of regulatory risk assessments for environmental mixtures are based on the assumption of similarly acting chemicals, also called concentration addition. Although for many mixtures (Table 2) this may provide a conservative measure of risk, it does not account for interactions. For chemical mixtures where the constituent chemicals influence each other's toxicity, the risk that a mixture poses to organisms in the environment can be under or overestimated using this assumption. For environmental regulation, synergistic interactions are of more concern than antagonistic ones as the mixture is more toxic to wildlife than predicted and may cause harm. Mixture effects caused by heavy metals, in particular, may be difficult to assess using traditional methods as interactions are relatively common.125 As well as chemical interactions, there are also a number of abiotic factors relating to habitat that can combine with the effects of chemical mixtures and lead to synergy or antagonism of the mixture toxicity.126
Table 2 Examples of recent applications of common risk assessment methods and the mixtures they were used to evaluate
Methodology |
Mixture under investigation |
Reference |
Whole mixture assessment |
Industrial wastewater containing cosmetics waste such as surfactants, preservatives and phenol derivatives |
152 |
Mixture of welding fumes containing toxic metals and gases |
153 |
Boreal sediments containing metal emissions |
154 |
Toxic Equivalency Factor (TEF) |
Binary mixtures of metals containing copper, lead and cadmium |
155 |
Binary mixtures of benzo[a]pyrene and dibenzo[a,l]pyrene as well as complex mixtures of PAHs |
156 |
Hazard Index (HI) |
Air samples collected from classrooms |
157 |
A mixture of nine phthalates in food items |
158 |
Pesticide mixtures on fruit and vegetable |
159 |
Adjusted or weight of evidence HI |
Predicted environmental mixture of 15 antibiotics |
160 |
Physiologically-based pharmacokinetic (PBPK) modelling |
Mixtures of trihalomethanes from reclaimed water |
161 |
A mixture of 109 chemicals in gasoline |
162 |
Mixture of pesticide residues |
163 |
Interactions in mixtures occur only in specific situations and are particular for that specific mixture, dose, organism, etc., so it is generally difficult to capture them in risk assessments. In order to address this, various approaches have been developed that aim to account for interactions in mixtures. The adjusted HI or weight of evidence (WOE) HI modification incorporates pairwise assessment of possible interactions between all chemicals in a mixture. It is used when the reference levels taken for the derivation of the HI are based on a different toxic endpoint than the other chemicals in the mixture.127,128 There are also other factors that are incorporated into the WOE score such as quality of the data and type of interaction. The outcome is a numerical score that indicates whether toxicity is likely to be under- or over-estimated using the traditional HI method.129 This adjusted HI is limited to mixtures where all the components are known as this information is needed to enable binary interaction assessment. It is also fairly time intensive and needs a lot of data to use. This approach has been developed for human risk assessment and although the concepts can be applied to environmentally relevant mixtures, appropriate data needs to be accessible for successful application.
Another approach that aims to predict mixture toxicity and risk by including interactions is the physiologically-based pharmacokinetic (PBPK) model. PBPK modelling aims to predict pharmacokinetic interactions in mixtures at the tissue level by assessing interactions of binary pairs of chemicals in the mix. It works on the assumption that interactions at a binary level can be used to predict interactions in the whole, more complex mixtures.130 The model views an organism as a set of connected tissue compartments and factors such as metabolism, uptake and interactions are included to provide a more realistic biological basis for mixture assessment.131 It has been shown that PBPK modelling is also able to predict changes of chemical concentration in tissues due to mixture interactions.132,133 This type of model is amenable to various types of data and in theory can be adapted to incorporate many types of toxicological endpoints, interactions, etc.134
Unfortunately, interaction-based risk prediction methods require trained specialists to carry out the assessments and a wealth of data on which to model mixture effects. Even for human risk assessment, a great deal more development is needed and even more so to make them applicable in an environmental context. Therefore, these methods are not currently able to be used as a standard protocol for risk assessments meaning that mixture interactions may still go unidentified (Table 3).
Table 3 A summary of the advantages and disadvantages of the most commonly used mixture risk assessment methods
Methodology |
Advantages |
Disadvantages |
Whole mixture assessment |
Interactions between components are captured |
Cannot extrapolate assessment to any other mixtures |
Can be used to study poorly characterised mixtures such as sludge or sediment |
Can only be used for stable mixtures |
|
No mechanistic information can be determined |
|
Cannot identify component(s) responsible for effects |
Toxic equivalency factor (TEF) |
Relatively simple to use |
Chemicals in question must exert toxicity via the AhR so only applicable for certain agents |
Easier to standardise than other methods |
|
Assumes that the equivalent toxicity between the chemical and the reference is the same at all concentrations |
Hazard Index (HI) |
Flexible as exact mechanism of action does not have to be determined |
Use of uncertainty factors can result in inconsistencies in the hazard quotient |
Different types of data can be used as risk reference levels |
|
Uses well characterised ‘acceptable risk levels’ for reference |
|
Adjusted or weight of evidence HI |
Accounts for interactions in mixture |
Mixture must be fully characterised |
Can be used when chemicals have different toxicological endpoints |
Time and data intensive |
Physiologically-based pharmacokinetic (PBPK) modelling |
Accounts for interactions in mixture |
Needs a trained specialist to perform |
Can incorporate various data types, toxicological endpoints, interactions etc. |
Requires a lot of data for model |
5.2. Multiple species assessment
The main difference between mixture risk assessment for humans and for the environment is that the latter requires consideration of multiple species. The aim of environmental risk assessment is to protect the ecosystem as a whole, not just individuals. This can present significant problems when trying to predict the risk of chemical mixtures. Assessment is hampered by a lack of knowledge of chemical mechanism of action as well as the potency in all species in a community as toxicity of mixture components will differ depending on physiological and pharmacokinetic differences between organisms.106 Toxicity of substances may also vary dramatically between different life stages, e.g., egg, larvae or adult of the same organism. Such variations in sensitivity can be caused by metabolism differences if detoxification or activation enzymes are involved in the mechanism of action. There may also be alterations in target sites that are needed for the mixture to exert toxicity.135 Overall, this presents a very complex situation with many factors to be accounted for in the characterisation of mixture effects.
Many environmental regulation approaches involve the use of predicted no effect concentrations (PNECs).136 PNECs are generated from laboratory-based standardised tests performed on the most sensitive organism in the ecosystem, which are then adjusted to account for factors such as inter-laboratory variation. Such organisms are from different trophic orders and an assumption is made that protection at lower levels of the ecosystem will confer safety to higher trophic species. In Europe, standard REACH (Registration, Evaluation, Authorisation and restriction of Chemicals) regulation requires that three species datasets are provided, one from each of the main trophic levels: primary producer, primary consumer, secondary consumer. For example, for aquatic ecosystems it is required that data for an algal species, a crustacean and a fish species are provided.137 The extrapolation of this data is often very restricted as it does not account for inherent dissimilarities between species at different trophic levels. As well, there may even be large variances between organisms within the same trophic categorisation. Furthermore, the assumption of protection to higher trophic species does not necessarily hold for chemicals that bioaccumulate up the food chain. The essential need to consider multiple species in a community has meant that typical regulatory mixture assessments are often unsuitable and may underestimate the risk of ecotoxic mixture effects.
There have been proposals for methods that aim to look at risk assessment at a community level rather than an individual level. The recent development of a tissue residue approach suggests a tiered method. The first tier assesses the toxicity of a mixture in individual species and then the second and third tiers use tissue residue data to derive a level which would provide protection to a specified percentage of organisms in a community.138 Further to this, species specific distributions (SSDs) take species sensitivities and predict the fraction of species in the total community which will experience toxic effects from mixtures using a known statistical distribution.139,140 Another way to address the issue might be the use of adverse outcome pathways. These models aim to use mechanistic data relating to single chemicals and mixtures to integrate population level responses into risk assessments.141,142
So far, these methods are still being developed and have come under some criticism due to inconsistencies in the derivation of sensitivity data. However, with further work they could have substantial implications for regulatory risk assessment of environmental mixtures providing more exposure data is generated for use in SSDs, etc.
5.3. Simple vs. complex mixtures
The approaches that are used to assess the risk of simple mixtures, those that have fewer than ten components, may not be appropriate to evaluate the potential toxicity of complex mixtures. Complex mixtures can have in excess of hundreds of chemical constituents, not all of which may have been identified. Mixtures with numerous components are also more likely to change over time and more likely to have potential interactions between chemicals. Many risk assessment approaches, particularly those focused on human health protection, are based on the assumption of binary pair toxicity predicting the mixture effects of an overall mixture. In an environmental context where there are so many potential combinations, often with unidentified components, assessment approaches need to consider how to handle highly complex mixtures that are composed of potentially innumerable chemicals.
It is possible that grouping compounds within complex mixtures may make them more manageable for risk assessment. Grouping can be done on the basis of toxicological or structural similarity to form assessment or risk groups. Two suggested methods that can be used to handle the prediction of complex mixture risk are the top n and pseudo top n approaches.99,143 The top n approach identifies a given number (‘n’) of the most risky chemicals in the mixture, for example the top ten components that pose the most toxic risk might be characterised. The pseudo top n identifies the top classes of chemical that present the most risk. Then by grouping chemicals based on similarities such as mechanism of action, a chemical is identified to represent each class. Once the actual top or pseudo top n chemicals have been identified, the risk of the mixture can be assessed using the same methods as are used for simple mixtures. It is assumed that the overall mixture risk is captured by focusing on the most risky chemicals.144 A method comparable to this has previously been used in a human health risk assessment framework and it is possible this kind of approach could be used for environmental mixtures as well.145
Depending on the amount and quality of data, it may also be possible to use PKBK approaches to extrapolate data from simple, binary mixtures to predict the toxicity of those with more than two constituents. Using pairwise interaction data as building blocks, PBPK models can add further ‘connections’ to incorporate more components providing there is qualitative data available on the mechanism of action. Theoretically such a model could be applied to a mixture with as many components as desired.131 This type of method requires a certain amount of data and as complete exposure data for such a large number of possible combinations will not always be available (or possible to obtain), computational approaches have been developed with the aim to bridge this gap.146 Although there is still significant work needed, they represent a promising new approach to more accurate risk assessment of complex environmental mixtures. This is particularly important as although the field of simple mixture risk assessment is now advancing rapidly, the development of methods for dealing with complex mixtures is often lagging behind due to a lack of good quality, quantitative data.
6. Future focus
As this review has shown, there are a number of challenges specific to the assessment of environmental mixtures that further complicate an already difficult task and when considering whether we can accurately predict the risk of environmental mixtures. It becomes apparent that this may only be possible to do in data rich situations where factors such as components and mechanisms of action have been characterised. There is an obvious necessity in all types of mixture risk assessment for data gaps, such as chemical mechanisms of action and species sensitivities, to be identified and perhaps a systematic approach implemented towards rectifying them. Further development of predictive models is another key step towards improved risk assessment of mixtures. Models that account for biological factors such as metabolism and body distributions, e.g., the PBPK model have been suggested as offering the most refined method for predictive purposes and are increasingly being used.147 Although it has been pointed out that such models need validation using commonly encountered chemical mixtures, once such data is generated, they might be used for standard regulatory assessments.103,147
For environmental mixture assessment, focus towards better modelling of ecosystems and involvement of multiple species in environmental risk prediction has already begun with methods such as the SSD. There are a number of assumptions made by currently-used methods that may work for human risk assessment but are not applicable to environmental mixtures. For example, data on toxicity endpoints is often only needed for one lifestage (often the adult stage) whereas some mixtures may be more toxic to developing organisms.148 Also, due to the large number of organism in ecosystems, toxicity data is often only used from a select few species. However, these species may not be representative of the ecosystem as a whole; for example, aquatic assessment methods for mixtures use data from D. magna, which have a number of unique characteristics such as asexual reproduction.149 Although pitfalls like these need to be amended, for ecotoxic chemicals, assessment at the population level is much more relevant than looking at toxicity at the individual level. The use of data from mesocosms or model ecosystems may offer a better solution when looking at mixture effects of a community, so higher tier assessment models will require more attention and work in the future.150 Finally, consideration must be given to external, abiotic factors in the ecosystem such as exposure route. Part of the risk characterisation of chemicals relies on determination of a dose–response relationship in a specific media but in the environment, organisms can be exposed to chemicals via food, air, water, etc. The route by which organisms are exposed to chemicals in a mixture will affect the overall toxicity and incorporation of partitioning and route information will improve the accuracy of risk assessments. The development of multimedia fate models has been suggested as a dynamic option for looking at chemical fate and mixture exposure route and will likely see further progress in the future.151
There are many areas where mixture risk assessment methods need concerted effort and work in order to make them more useable for ecotoxic mixtures. By focusing on those issues which significantly impair the accuracy of risk assessments, it may be possible for new and improved models to overcome such issues and ultimately be used in a regulatory context to ensure mixture toxicity in the environment are not above acceptable levels.
7. Conclusions
In the environment, organisms are simultaneously exposed to a great variety of chemicals with diverse properties. The way in which chemicals in a mixture influence the overall toxicity depends on many factors including their concentration, target site and mechanism of action. The toxicity of a mixture can be predicted using toxicity data on either the individual components or the mixture as a whole. Current approaches that aim to characterise the risk of chemical mixtures use component-based methods based on concentration addition such as TEFs and HIs. Chemicals in mixtures such as pharmaceuticals, heavy metals and pesticides can cause detrimental health effects to organisms and for those mixtures where there are interactions between the components, these traditional risk assessment methods may lead to an underestimation of toxicity which could endanger wildlife.
Currently, our ability to accurately predict the ecotoxic effect of chemicals in mixtures is restricted by major challenges, such as multi-species considerations and a lack of consideration of interactions, which hinder the development of better predictive models. The vast number of factors that need to be considered and then incorporated into risk assessments makes it appear an almost insurmountable task. However, the establishment of newer assessment methods such as PBKB and SSD models aim to overcome issues that make traditional risk assessments unsuitable for environmental mixtures. There is considerable focus on how such methods can be improved for risk assessment in general but future work will also need to address developing models that are more suited to the specific and daunting task of environmental mixture risk assessment such as population level assessment models and consideration of highly complex, poorly defined mixtures.
Acknowledgements
Kelly Heys is a NERC-CEH algorithm student.
References
- A. Kortenkamp, R. Evans, M. Faust, F. Kalberlah, M. Scholze and U. Schuhmacher-Wolz, Supporting Publications 2012, EN-232, p. 233, http://www.efsa.europa.eu/publications.
- C. E. Lundholm, Comparative Biochemistry and Physiology Part C: Pharmacology, Toxicology and Endocrinology, 1997, 118, 113–128 CrossRef CAS.
- S. Ahmed, K. B. Ahsan, M. Kippler, A. Mily, Y. Wagatsuma, A. W. Hoque, P. T. Ngom, S. El Arifeen, R. Raqib and M. Vahter, Toxicol. Sci., 2012, 129, 305–314 CrossRef CAS PubMed.
- R. Lohmann, K. Breivik, J. Dachs and D. Muir, Environ. Pollut., 2007, 150, 150–165 CrossRef CAS PubMed.
- N. Cedergreen, PLoS One, 2014, 9, 96580 Search PubMed.
- B. Daus, H. Weiss and R. Altenburger, Chemosphere, 2010, 78, 307–312 CrossRef CAS PubMed.
- K. Lock and C. Janssen, Biol. Fertil. Soils, 2001, 34, 219–221 CrossRef CAS.
- F. Gagné, J. Auclair, P. Turcotte, M. Fournier, C. Gagnon, S. Sauve and C. Blaise, Aquat. Toxicol., 2008, 86, 333–340 CrossRef PubMed.
- K. E. Biesinger, G. Christensen and J. Fiandt, Ecotoxicol. Environ. Saf., 1986, 11, 9–14 CrossRef CAS PubMed.
- R. L. Spehar and J. T. Fiandt, Environ. Toxicol. Chem., 1986, 5, 917–931 CrossRef CAS.
- T.-H. Le, E.-S. Lim, N.-H. Hong, S.-K. Lee, Y. S. Shim, J. R. Hwang, Y.-H. Kim and J. Min, Chemosphere, 2013, 93, 2341–2348 CrossRef CAS PubMed.
- T. Vandenbrouck, A. Soetaert, K. van der Ven, R. Blust and W. De Coen, Aquat. Toxicol., 2009, 92, 18–29 CrossRef CAS PubMed.
- M. H. Kraak, D. Lavy, H. Schoon, M. Toussaint, W. H. Peeters and N. M. van Straalen, Environ. Toxicol. Chem., 1994, 13, 109–114 CrossRef CAS.
- M. Kraak, S. Stuijfzand and W. Admiraal, Bull. Environ. Contam. Toxicol., 1999, 63, 805–812 CrossRef CAS PubMed.
- N. Loumbourdis, I. Kostaropoulos, B. Theodoropoulou and D. Kalmanti, Environ. Pollut., 2007, 145, 787–792 CrossRef CAS PubMed.
- J. H. Yim, K. W. Kim and S. D. Kim, J. Hazard. Mater., 2006, 138, 16–21 CrossRef CAS PubMed.
- E. Enserink, J. Maas-Diepeveen and C. Van Leeuwen, Water Res., 1991, 25, 679–687 CrossRef CAS.
- X. Xu, Y. Li, Y. Wang and Y. Wang, Toxicol. in Vitro, 2011, 25, 294–300 CrossRef CAS PubMed.
- B. Zhu, Z.-F. Wu, J. Li and G.-X. Wang, Ecotoxicol. Environ. Saf., 2011, 74, 2193–2202 CrossRef CAS PubMed.
- P. R. Palaniappan and S. Karthikeyan, J. Environ. Sci., 2009, 21, 229–236 CrossRef CAS.
- C. Vellinger, M. Parant, P. Rousselle and P. Usseglio-Polatera, Ecotoxicology, 2012, 21, 1817–1827 CrossRef CAS PubMed.
- C. Vellinger, E. Gismondi, V. Felten, P. Rousselle, K. Mehennaoui, M. Parant and P. Usseglio-Polatera, Aquat. Toxicol., 2013, 140, 106–116 CrossRef PubMed.
- J. Odendaal and A. Reinecke, Arch. Environ. Contam. Toxicol., 2004, 46, 377–384 CrossRef CAS PubMed.
- H. Qiu, M. G. Vijver and W. J. Peijnenburg, Environ. Toxicol. Chem., 2011, 30, 2084–2093 CrossRef CAS PubMed.
- H. L. Martin, C. Svendsen, L. J. Lister, J. L. Gomez-Eyles and D. J. Spurgeon, Environ. Toxicol. Chem., 2009, 28, 97–104 CrossRef CAS PubMed.
- D. Pain, I. Carter, A. Sainsbury, R. Shore, P. Eden, M. Taggart, S. Konstantinos, L. Walker, A. Meharg and A. Raab, Sci. Total Environ., 2007, 376, 116–127 CrossRef CAS PubMed.
- G. Heinz and D. Hoffman, Arch. Environ. Contam. Toxicol., 2003, 44, 0257–0264 CrossRef CAS PubMed.
- L. Gangoso, P. Álvarez-Lloret, A. A. Rodríguez-Navarro, R. Mateo, F. Hiraldo and J. A. Donázar, Environ. Pollut., 2009, 157, 569–574 CrossRef CAS PubMed.
- A. Sánchez-Chardi, C. Peñarroja-Matutano, C. A. O. Ribeiro and J. Nadal, Chemosphere, 2007, 70, 101–109 CrossRef PubMed.
- D. Ivanković, J. Pavičić, V. Beatović, R. S. Klobučar and G. I. V. Klobučar, Environ. Toxicol., 2010, 25, 198–211 Search PubMed.
- F. Dondero, M. Banni, A. Negri, L. Boatti, A. Dagnino and A. Viarengo, BMC Genomics, 2011, 12, 195 CrossRef CAS PubMed.
- V. Maria and M. Bebianno, Comp. Biochem. Physiol., Part C: Toxicol. Pharmacol., 2011, 154, 56–63 CrossRef CAS PubMed.
- T. Heberer, Toxicol. Lett., 2002, 131, 5–17 CrossRef CAS PubMed.
- S. Öllers, H. P. Singer, P. Fässler and S. R. Müller, J. Chromatogr. A, 2001, 911, 225–234 CrossRef.
- P. H. Roberts and K. V. Thomas, Sci. Total Environ., 2006, 356, 143–153 CrossRef CAS PubMed.
- L. H. Santos, A. Araújo, A. Fachini, A. Pena, C. Delerue-Matos and M. Montenegro, J. Hazard. Mater., 2010, 175, 45–95 CrossRef CAS PubMed.
- K. Kümmerer, J. Environ. Manage., 2009, 90, 2354–2366 CrossRef PubMed.
- F. Pomati, C. Orlandi, M. Clerici, F. Luciani and E. Zuccato, Toxicol. Sci., 2008, 102, 129–137 CrossRef CAS PubMed.
- M. Cleuvers, Ecotoxicol. Environ. Saf., 2004, 59, 309–315 CrossRef CAS PubMed.
- M. Cleuvers, Toxicol. Lett., 2003, 142, 185–194 CrossRef CAS PubMed.
- C. M. Flaherty and S. I. Dodson, Chemosphere, 2005, 61, 200–207 CrossRef CAS PubMed.
- P. Yeh, A. I. Tschumi and R. Kishony, Nat. Genet., 2006, 38, 489–494 CrossRef CAS PubMed.
- T. Backhaus, M. Scholze and L. Grimme, Aquat. Toxicol., 2000, 49, 49–61 CrossRef CAS PubMed.
- A. M. Christensen, F. Ingerslev and A. Baun, Environ. Toxicol. Chem., 2006, 25, 2208–2215 CrossRef CAS PubMed.
- M. González-Pleiter, S. Gonzalo, I. Rodea-Palomares, F. Leganés, R. Rosal, K. Boltes, E. Marco and F. Fernández-Piñas, Water Res., 2013, 47, 2050–2064 CrossRef PubMed.
- S. M. Richards, C. J. Wilson, D. J. Johnson, D. M. Castle, M. Lam, S. A. Mabury, P. K. Sibley and K. R. Solomon, Environ. Toxicol. Chem., 2004, 23, 1035–1042 CrossRef CAS PubMed.
- R. A. Brain, D. J. Johnson, S. M. Richards, M. L. Hanson, H. Sanderson, M. W. Lam, C. Young, S. A. Mabury, P. K. Sibley and K. R. Solomon, Aquat. Toxicol., 2004, 70, 23–40 CrossRef CAS PubMed.
- M. Galus, J. Jeyaranjaan, E. Smith, H. Li, C. Metcalfe and J. Y. Wilson, Aquat. Toxicol., 2013, 132, 212–222 CrossRef PubMed.
- R. F. Shore, M. A. Taggart, J. Smits, R. Mateo, N. L. Richards and S. Fryday, Philos. Trans. R. Soc., B, 2014, 369, 20130570 CrossRef PubMed.
- S. Markman, C. T. Müller, D. Pascoe, A. Dawson and K. L. Buchanan, J. Appl. Ecol., 2011, 48, 391–397 CrossRef.
- T. Blacquiere, G. Smagghe, C. A. Van Gestel and V. Mommaerts, Ecotoxicology, 2012, 21, 973–992 CrossRef CAS PubMed.
- W. Zhu, D. R. Schmehl, C. A. Mullin and J. L. Frazier, PLoS One, 2014, 9, 77547 Search PubMed.
- K. B. Tierney, J. L. Sampson, P. S. Ross, M. A. Sekela and C. J. Kennedy, Environ. Sci. Technol., 2008, 42, 4996–5001 CrossRef CAS PubMed.
- J. W. Deneer, Pest Manage. Sci., 2000, 56, 516–520 CrossRef CAS.
- J. B. Belden, R. J. Gilliom and M. J. Lydy, Integr. Environ. Assess. Manage., 2007, 3, 364–372 CrossRef CAS.
- B. Gagnaire, M. Gay, A. Huvet, J.-Y. Daniel, D. Saulnier and T. Renault, Aquat. Toxicol., 2007, 84, 92–102 CrossRef CAS PubMed.
- B. Anderson, B. Phillips, J. Hunt, K. Siegler, J. Voorhees, K. Smalling, K. Kuivila, M. Hamilton, J. A. Ranasinghe and R. Tjeerdema, Environ. Monit. Assess., 2014, 186, 1801–1814 CrossRef CAS PubMed.
- R. A. Relyea, Oecologia, 2009, 159, 363–376 CrossRef PubMed.
- T. B. Hayes, P. Case, S. Chui, D. Chung, C. Haeffele, K. Haston, M. Lee, V. P. Mai, Y. Marjuoa and J. Parker, Environ. Health Perspect., 2006, 114, 40 CrossRef PubMed.
- R. A. Relyea, Environ. Toxicol. Chem., 2004, 23, 1737–1742 CrossRef CAS PubMed.
- A. Coors and T. Frische, Environ. Sci. Eur., 2011, 23, 1–18 CrossRef.
- C. A. Laetz, D. H. Baldwin, T. K. Collier, V. Hebert, J. D. Stark and N. L. Scholz, Environ. Health Perspect., 2009, 117, 348–353 CrossRef CAS PubMed.
- J. J. Rasmussen, P. Wiberg-Larsen, A. Baattrup-Pedersen, N. Cedergreen, U. S. McKnight, J. Kreuger, D. Jacobsen, E. A. Kristensen and N. Friberg, Water Res., 2015, 84, 25–32 CrossRef CAS PubMed.
- C. I. Bliss, Ann. Appl. Biol., 1939, 26, 585–615 CrossRef CAS.
- R. Plackett and P. Hewlett, Ann. Appl. Biol., 1948, 35, 347–358 CrossRef CAS PubMed.
- R. Plackett and P. Hewlett, J. Roy. Stat. Soc. B, 1952, 14, 141–163 Search PubMed.
- C. H. Walker, R. M. Sibly, S. P. Hopkin and D. B. Pakall, Principals of Ecotoxicology, CRC Press, Florida, USA, 2012 Search PubMed.
- B. I. Escher and J. L. Hermens, Environ. Sci. Technol., 2002, 36, 4201–4217 CrossRef CAS PubMed.
- T. Backhaus, M. Faust and A. Kortenkamp, Integr. Environ. Assess. Manage., 2013, 9, 547–548 CrossRef PubMed.
- F. Massart, J. C. Harrell, G. Federico and G. Saggese, J. Perinatol., 2005, 25, 282–288 CrossRef CAS PubMed.
- P. Silva, M. J. Rocha, C. Cruzeiro, F. Malhão, B. Reis, R. Urbatzka, R. A. Monteiro and E. Rocha, Aquat. Toxicol., 2012, 124, 1–10 CrossRef PubMed.
- J. V. Brian, C. A. Harris, M. Scholze, T. Backhaus, P. Booy, M. Lamoree, G. Pojana, N. Jonkers, T. Runnalls and A. Bonfà, Environ. Health Perspect., 2005, 113, 721 CrossRef CAS PubMed.
- F. S. Vom Saal, B. G. Timms, M. M. Montano, P. Palanza, K. A. Thayer, S. C. Nagel, M. D. Dhar, V. Ganjam, S. Parmigiani and W. V. Welshons, Proc. Natl. Acad. Sci. U. S. A., 1997, 94, 2056–2061 CrossRef CAS.
- N. Rajapakse, E. Silva and A. Kortenkamp, Environ. Health Perspect., 2002, 110, 917 CrossRef CAS PubMed.
- N. Cedergreen, A. M. Christensen, A. Kamper, P. Kudsk, S. K. Mathiassen, J. C. Streibig and H. Sørensen, Environ. Toxicol. Chem., 2008, 27, 1621–1632 CrossRef CAS PubMed.
- A. Kortenkamp, T. Backhaus and M. Faust, European Commission Report, 2009 Search PubMed.
- T. Backhaus, Å. Arrhenius and H. Blanck, Environ. Sci. Technol., 2004, 38, 6363–6370 CrossRef CAS PubMed.
- T. Backhaus, R. Altenburger, W. Boedeker, M. Faust, M. Scholze and L. H. Grimme, Environ. Toxicol. Chem., 2000, 19, 2348–2356 CrossRef CAS.
- F. Liu, Ecotoxicol. Environ. Saf., 2011, 74, 1600–1606 CrossRef PubMed.
- M. Faust, R. Altenburger, T. Backhaus, H. Blanck, W. Boedeker, P. Gramatica, V. Hamer, M. Scholze, M. Vighi and L. Grimme, Aquat. Toxicol., 2003, 63, 43–63 CrossRef CAS PubMed.
- S. Ermler, M. Scholze and A. Kortenkamp, Arch. Toxicol., 2013, 88, 1–16 Search PubMed.
- V. P. Utgikar, N. Chaudhary, A. Koeniger, H. H. Tabak, J. R. Haines and R. Govind, Water Res., 2004, 38, 3651–3658 CrossRef CAS PubMed.
- S. Chaperon and S. Sauve, Soil Biol. Biochem., 2007, 39, 2329–2338 CrossRef CAS.
- K. Wah Chu and K. L. Chow, Aquat. Toxicol., 2002, 61, 53–64 CrossRef CAS PubMed.
- D. Paul, in Methods for the Discovery and Characterization of G Protein-Coupled Receptors, Springer, New York, 2011, pp. 305–319 Search PubMed.
- R. Altenburger, T. Backhaus, W. Boedeker, M. Faust and M. Scholze, Environ. Toxicol. Chem., 2013, 32, 1685–1687 CrossRef CAS PubMed.
- R. L. Metcalf, Annu. Rev. Entomol., 1967, 12, 229–256 CrossRef CAS PubMed.
- P. Dalvi, T. Wilder-Kofie, B. Mares, R. Dalvi and L. Billups, Pestic. Biochem. Physiol., 2002, 74, 85–90 CrossRef CAS.
- R. R. Dalvi, A. L. Hunter and R. A. Neal, Chem.-Biol. Interact., 1975, 10, 349–361 CrossRef CAS.
- P. S. Dalvi, R. R. Dalvi and L. H. Billups, Toxicol. Int., 2013, 20, 132 CrossRef CAS PubMed.
- R. Josse, A. Sharanek, C. C. Savary and A. Guillouzo, Chem.-Biol. Interact., 2014, 209, 68–76 CrossRef CAS PubMed.
- A. F. Hernández, T. Parrón, A. M. Tsatsakis, M. Requena, R. Alarcón and O. López-Guarnido, Toxicology, 2013, 307, 136–145 CrossRef PubMed.
- R. C. James, S. M. Roberts and P. L. Williams, in Principles of Toxicology: Environmental and Industrial Applications, John Wiley and Sons Inc., New York, 2000, pp. 3–4 Search PubMed.
- J. L. Luque-Garcia, P. Cabezas-Sanchez, D. S. Anunciação and C. Camara, Anal. Chim. Acta, 2013, 801, 1–13 CrossRef CAS PubMed.
- E. G. Sørmo, T. M. Ciesielski, I. B. Øverjordet, S. Lierhagen, G. S. Eggen, T. Berg and B. M. Jenssen, Environ. Sci. Technol., 2011, 45, 6561–6566 CrossRef PubMed.
- F. Dang and W.-X. Wang, Environ. Sci. Technol., 2011, 45, 3116–3122 CrossRef CAS PubMed.
- P. F. Landrum, P. M. Chapman, J. Neff and D. S. Page, Integr. Environ. Assess. Manage., 2012, 8, 217–230 CrossRef CAS PubMed.
- S. Antunes, R. Pereira and F. Gonçalves, Arch. Environ. Contam. Toxicol., 2007, 53, 207–213 CrossRef CAS PubMed.
- J. P. Groten, V. J. Feron and J. Sühnel, Trends Pharmacol. Sci., 2001, 22, 316–322 CrossRef CAS PubMed.
- A. Boobis, R. Budinsky, S. Collie, K. Crofton, M. Embry, S. Felter, R. Hertzberg, D. Kopp, G. Mihlan and M. Mumtaz, Crit. Rev. Toxicol., 2011, 41, 369–383 CrossRef CAS PubMed.
- A. Kortenkamp and M. Faust, Int. J. Androl., 2010, 33, 463–474 CrossRef CAS PubMed.
- K. Sexton and D. Hattis, Environ. Health Perspect., 2007, 115, 825–832 CrossRef CAS PubMed.
- L. K. Teuschler, Toxicol. Appl. Pharmacol., 2007, 223, 139–147 CrossRef CAS PubMed.
- A. Ginebreda, M. Kuzmanovic, H. Guasch, M. L. de Alda, J. C. López-Doval, I. Muñoz, M. Ricart, A. M. Romaní, S. Sabater and D. Barceló, Sci. Total Environ., 2014, 468, 715–723 CrossRef PubMed.
- J. C. López-Doval, N. De Castro-Català, I. Andrés-Doménech, J. Blasco, A. Ginebreda and I. Muñoz, Sci. Total Environ., 2012, 440, 194–203 CrossRef PubMed.
- T. Backhaus and M. Faust, Environ. Sci. Technol., 2012, 46, 2564–2573 CrossRef CAS PubMed.
- M. G. Barron, R. Heintz and S. D. Rice, Mar. Environ. Res., 2004, 58, 95–100 CrossRef CAS PubMed.
- R. A. Budinsky, D. Paustenbach, D. Fontaine, B. Landenberger and T. B. Starr, Toxicol. Sci., 2006, 91, 275–285 CrossRef CAS PubMed.
- T. Simon, J. K. Britt and R. C. James, Regul. Toxicol. Pharmacol., 2007, 48, 148–170 CrossRef CAS PubMed.
- M. Van den Berg, L. S. Birnbaum, M. Denison, M. De Vito, W. Farland, M. Feeley, H. Fiedler, H. Hakansson, A. Hanberg and L. Haws, Toxicol. Sci., 2006, 93, 223–241 CrossRef CAS PubMed.
- S. P. Bhavsar, E. J. Reiner, A. Hayton, R. Fletcher and K. MacPherson, Environ. Int., 2008, 34, 915–921 CrossRef CAS PubMed.
- M. Van den Berg, L. Birnbaum, A. Bosveld, B. Brunström, P. Cook, M. Feeley, J. P. Giesy, A. Hanberg, R. Hasegawa and S. W. Kennedy, Environ. Health Perspect., 1998, 106, 775 CrossRef CAS PubMed.
- S. H. Safe, Environ. Health Perspect., 1998, 106, 1051 CrossRef CAS PubMed.
- L. C. Haws, S. H. Su, M. Harris, M. J. DeVito, N. J. Walker, W. H. Farland, B. Finley and L. S. Birnbaum, Toxicol. Sci., 2006, 89, 4–30 CrossRef CAS PubMed.
- J. Hamm, C.-Y. Chen and L. Birnbaum, Toxicol. Sci., 2003, 74, 182–191 CrossRef CAS PubMed.
- N. J. Walker, P. W. Crockett, A. Nyska, A. E. Brix, M. P. Jokinen, D. M. Sells, J. R. Hailey, M. Easterling, J. K. Haseman and M. Yin, Environ. Health Perspect., 2005, 113, 43–48 CrossRef CAS PubMed.
- A. Ginebreda, I. Muñoz, M. L. de Alda, R. Brix, J. López-Doval and D. Barceló, Environ. Int., 2010, 36, 153–162 CrossRef CAS PubMed.
- Z. Yan, X. Yang, G. Lu, J. Liu, Z. Xie and D. Wu, Sci. Total Environ., 2014, 470, 171–179 CrossRef PubMed.
- M. J. García-Galán, M. S. Díaz-Cruz and D. Barceló, Environ. Int., 2011, 37, 462–473 CrossRef PubMed.
- J. Cristale, A. Katsoyiannis, A. J. Sweetman, K. C. Jones and S. Lacorte, Environ. Pollut., 2013, 179, 194–200 CrossRef CAS PubMed.
- USEPA (U.S. Environmental Protection Agency), Guidelines for Health Risk Assessment of Chemical Mixtures, Fed. Regist., 1986, vol. 51, pp. 34014–34025 Search PubMed.
- D. A. Sarigiannis and U. Hansen, Environ. Health, 2012, 11, S18 CrossRef PubMed.
- H. Sanderson, D. J. Johnson, T. Reitsma, R. A. Brain, C. J. Wilson and K. R. Solomon, Regul. Toxicol. Pharmacol., 2004, 39, 158–183 CrossRef CAS PubMed.
- J. Beyer, K. Petersen, Y. Song, A. Ruus, M. Grung, T. Bakke and K. E. Tollefsen, Mar. Environ. Res., 2014, 96, 81–91 CrossRef CAS PubMed.
- M. G. Vijver, E. G. Elliott, W. J. Peijnenburg and G. R. De Snoo, Environ. Toxicol. Chem., 2011, 30, 1482–1487 CrossRef CAS PubMed.
- C. A. Laetz, D. H. Baldwin, V. R. Hebert, J. D. Stark and N. L. Scholz, Aquat. Toxicol., 2014, 146, 38–44 CrossRef CAS PubMed.
- M. Mumtaz and P. Durkin, Toxicol. Ind. Health, 1991, 8, 377–406 Search PubMed.
- M. Mumtaz, I. Sipes, H. Clewell and R. Yang, Fundam. Appl. Toxicol., 1993, 21, 258–269 CrossRef CAS PubMed.
- V. J. Feron, P. W. van Vliet and W. R. Notten, Environ. Toxicol. Pharmacol., 2004, 18, 215–222 CrossRef CAS PubMed.
- R. C. Hertzberg and M. M. MacDonell, Science of the Total Environment, 2002, 288, 31–42 CrossRef CAS PubMed.
- K. Krishnan, S. Haddad, M. Béliveau and R. Tardif, Environ. Health Perspect., 2002, 110, 989 CrossRef CAS PubMed.
- S. Haddad, R. Tardif, G. Charest-Tardif and K. Krishnan, Toxicol. Appl. Pharmacol., 1999, 161, 249–257 CrossRef CAS PubMed.
- S. Haddad, G. Charest-Tardif, R. Tardif and K. Krishnan, Toxicol. Appl. Pharmacol., 2000, 167, 199–209 CrossRef CAS PubMed.
- S. Haddad, M. Béliveau, R. Tardif and K. Krishnan, Toxicol. Sci., 2001, 63, 125–131 CrossRef CAS PubMed.
- B. I. Escher, R. Ashauer, S. Dyer, J. L. Hermens, J. H. Lee, H. A. Leslie, P. Mayer, J. P. Meador and M. S. Warne, Integr. Environ. Assess. Manage., 2011, 7, 28–49 CrossRef CAS PubMed.
- X. Jin, J. Zha, Y. Xu, Z. Wang and S. S. Kumaran, Chemosphere, 2011, 84, 1506–1511 CrossRef CAS PubMed.
- E. C. Agency, Guidance on Information Requirements and Chemical Safety Assessment, 2008 Search PubMed.
- S. Dyer, S. J. Warne, J. S. Meyer, H. A. Leslie and B. I. Escher, Integr. Environ. Assess. Manage., 2011, 7, 99–115 CrossRef CAS PubMed.
- L. Posthuma and D. De Zwart, Environ. Toxicol. Chem., 2006, 25, 1094–1105 CrossRef CAS PubMed.
- D. de Zwart and L. Posthuma, Environ. Toxicol. Chem., 2005, 24, 2665–2676 CrossRef CAS PubMed.
- V. J. Kramer, M. A. Etterson, M. Hecker, C. A. Murphy, G. Roesijadi, D. J. Spade, J. A. Spromberg, M. Wang and G. T. Ankley, Environ. Toxicol. Chem., 2011, 30, 64–76 CrossRef CAS PubMed.
- M. P. Dent, P. L. Carmichael, K. C. Jones and F. L. Martin, Environ. Int., 2015, 83, 94–106 CrossRef CAS PubMed.
- V. J. Feron, J. P. Groten and P. J. van Bladeren, Arch. Toxicol., 1998, 20, 363–373 CAS.
- V. Feron and J. Groten, Food Chem. Toxicol., 2002, 40, 825–839 CrossRef CAS PubMed.
- B. L. Johnson and C. T. DeRosa, Toxicology, 1995, 105, 145–156 CrossRef CAS PubMed.
- J. Kim, S. Kim and G. E. Schaumann, Rev. Environ. Sci. Bio/Technol., 2013, 12, 235–256 CrossRef CAS.
- R. S. DeWoskin and C. M. Thompson, Regul. Toxicol. Pharmacol., 2008, 51, 66–86 CrossRef CAS PubMed.
- M. Breitholtz, C. Rudén, S. Ove Hansson and B.-E. Bengtsson, Ecotoxicol. Environ. Saf., 2006, 63, 324–335 CrossRef CAS PubMed.
- A. Buikema, D. Lee and J. Cairns, J. Test. Eval., 1976, 4, 119–126 CrossRef CAS.
- H. Koshikawa, K. Xu, Z. Liu, K. Kohata, M. Kawachi, H. Maki, M. Zhu and M. Watanabe, Estuarine, Coastal Shelf Sci., 2007, 71, 68–80 CrossRef.
- T. Gouin and T. Harner, Environ. Int., 2003, 29, 717–724 CrossRef CAS PubMed.
- J. B. Carbajo, J. A. Perdigón-Melón, A. L. Petre, R. Rosal, P. Letón and E. García-Calvo, Water Res., 2015, 72, 174–185 CrossRef CAS PubMed.
- K. Sriram, G. X. Lin, A. M. Jefferson, S. Stone, A. Afshari, M. J. Keane, W. McKinney, M. Jackson, B. T. Chen and D. Schwegler-Berry, Toxicology, 2015, 328, 168–178 CrossRef CAS PubMed.
- K. Väänänen, T. Kauppila, J. Mäkinen, M. Leppänen, M. Lyytikäinen and J. Akkanen, Integr. Environ. Assess. Manage., 2016 DOI:10.1002/ieam.1751.
- Y. Gao, J. Feng, F. Han and L. Zhu, Environ. Pollut., 2016, 213, 16–29 CrossRef CAS PubMed.
- I. W. Jarvis, C. Bergvall, M. Bottai, R. Westerholm, U. Stenius and K. Dreij, Toxicol. Appl. Pharmacol., 2013, 266, 408–418 CrossRef CAS PubMed.
- N. Mishra, G. A. Ayoko, T. Salthammer and L. Morawska, Environ. Sci. Pollut. Res., 2015, 22, 15080–15088 CrossRef PubMed.
- J. Chang, B. Yan, M. Chang, S. Tseng, Y. Kao, J. Chen and C. Lee, Environ. Pollut., 2014, 189, 77–84 CrossRef CAS PubMed.
- B. H. Jensen, A. Petersen, E. Nielsen, T. Christensen, M. E. Poulsen and J. H. Andersen, Food Chem. Toxicol., 2015, 83, 300–307 CrossRef CAS PubMed.
- C. Marx, V. Mühlbauer, P. Krebs and V. Kuehn, Sci. Total Environ., 2015, 524, 269–279 CrossRef PubMed.
- Z. Niu, X. Zang and Y. Zhang, J. Hazard. Mater., 2015, 285, 190–198 CrossRef CAS PubMed.
- M. N. Jasper, S. A. Martin, W. M. Oshiro, J. Ford, P. J. Bushnell and H. El-Masri, Environ. Sci. Technol., 2016, 50, 3231–3238 CrossRef CAS PubMed.
- G. de Sousa, A. Nawaz, J.-P. Cravedi and R. Rahmani, Toxicol. Sci., 2014, 141, 234–243 CrossRef CAS PubMed.
|
This journal is © The Royal Society of Chemistry 2016 |
Click here to see how this site uses Cookies. View our privacy policy here.