DOI:
10.1039/C5MB00603A
(Paper)
Mol. BioSyst., 2016,
12, 262-273
High resolution metabolomics technology reveals widespread pathway changes of alcoholic liver disease†
Received
7th September 2015
, Accepted 4th November 2015
First published on 23rd November 2015
Abstract
Alcoholic liver disease (ALD) is a significant cause of death and morbidity. However little is known regarding the widespread pathway changes of ALD disorder. This study utilized metabolomic profiling to examine the pathogenic mechanisms of ALD based on a rat model. A total of 21 metabolites with significant changes were identified, involving several key metabolic pathways such as pentose and glucuronate interconversions, starch and sucrose metabolism, cysteine and methionine metabolism. Furthermore, the differential proteins corresponding to alterations in metabolism across the metabolic network were identified using iTRAQ-based quantitative proteomics analysis. The proteins appear to be involved in protein binding, metabolism, immune response, and signal conduction. Interestingly, integrated omics profiling firstly reveals that p53 and Fc epsilon RI signaling pathways were closely related to ALD. Our study indicates that most of these proteins were found to play a pivotal role in the regulation of multiple metabolism pathways. Collectively, the current study provides insights into the molecular mechanisms of ALD from widespread pathway changes.
Introduction
Alcohol has been a part of human culture since the beginning of recorded history. Problems associated with excess alcohol consumption include social issues, increased accidents, chronic health problems and mortality.1 Alcohol related morbidity in developed countries is second only to tobacco use and is responsible for 2.5 million deaths globally each year, and costs 1% of the GDP of middle to high income countries.2 Alcohol misuse is a major public health problem worldwide and accounts for elevated social and economic costs. Alcoholic liver disease (ALD) is a common complication of alcohol misuse. The detection of ALD at an early stage could provide opportunities for more optimal management. The biomarkers including the erythrocyte mean cell volume, c-glutamyltransferase and carbohydrate-deficient transferring provide an objective measure of alcohol consumption, and they can assist in the detection of at-risk drinking.3 Clearly, there is a need for more effective and definitive treatment options in order to improve prognosis and outcome of patients with severe ALD. Fortunately, integrated omics technology has been used to explore the particular metabolites, potentially diagnostic and prognostic biomarkers for a deep understanding of the essence of diseases.4–6
At the end of the 20th century, genomics wrote out the ‘script of life’; proteomics decoded the script; and metabolomics came into bloom.7 These ‘omics’ quickly became the thrust of life sciences, pushing the discipline to a new high. At present, numerous studies have discovered potential markers of disease using proteomics.8 Isobaric tags for relative and absolute quantitation (iTRAQ), as a quantitative method, is a common tool in proteomics and has been extensively used for biomarker discovery in various disease contexts.9 Metabolomics is the endpoint of genotype functions and the biochemical phenotype in the body, and is linked closely to the alteration of functions in the body, and incorporates a ‘top-down’ strategy to reflect the terminal symptoms of a whole system.10 Recent advances have suggested that metabolite profiles will improve the understanding of the disease mechanisms.11–17 Global metabolomic profiling with alcoholic fibrosis has proceeded very slowly, and changes in the serum proteomes of ALD are rarely reported. There is an urgent need for the discovery of novel molecular signatures to understand the underlying biological basis for ALD. However, to date there have been few studies aimed at gaining deeper insights into ALD through an integrated metabolomics approach. Therefore, unlike other studies, this study was to devise a systematically integrated omics approach to focus and identify widespread pathway changes of ALD in rats.
Materials and methods
Reagents
HPLC grade acetonitrile was obtained from Merck (Darmstadt, Germany); methanol (HPLC grade) was purchased from Fisher Scientific Corporation (Loughborough, UK); water was produced using a Milli-Q Ultra-pure water system (Millipore, Billerica, USA); formic acid was obtained from Honeywell Company (Morristown, New Jersey, USA); leucine enkephalin was purchased from Sigma-Aldrich (St. Louis, MO, USA). The iTRAQ reagent multi-plex kit, containing the iTRAQ reagents, was bought commercially (Applied Biosystems, Foster City, CA, USA). The assay kits for alanine aminotransferase (ALT), aspartate amino transferase (AST), alkaline phosphatase (ALP), triglycerides (TG), total bilirubin (T-BIL), total protein (TP), albumin (ALB), total cholesterol (CHOL), hyaluronic acid (HA), laminin (LN), procollagen Iii (PIIINP), and collagen IV (CIV) were purchased from the Nanjing Jiancheng Biotech Company (Nanjing, China).
Animals
Male Wistar rats were maintained in a specific pathogen-free environment. The animals were allowed to acclimatize in metabolic cages for 1 week prior to treatment. The animals were randomly assigned to 4 groups of 8 rats each as follows: control, 7W, 11W and 12W groups. The rats in the model group were orally administrated at a dose of 0.8 ml/100 g mixture (6 g kg−1 alcohol liquor) and high-fat diet (high fat emulsion (10 ml kg−1)) for 12 consecutive weeks. The alcohol-fed model rats were fed ad libitum. Rats were sacrificed by an intraperitoneal injection of 1% pentobarbital sodium (0.15 ml/100 g body weight) at four time points: 4, 7, 11 and 12 weeks after initiation of injection. Livers were collected and washed three times with saline water. Each liver was cut into two pieces. The small piece was immediately fixed in buffered formalin for pathological staining. We collected plasma samples in heparinized tubes, kept them on ice for 1 h and centrifuged them at 5000 rpm for 20 min at 4 °C, flash frozen in liquid nitrogen and stored at −80 °C until the liver function tests and proteomics analyses were performed. Urine was collected daily (at 8:00 am) from the metabolic cages at ambient temperature throughout the entire procedure and centrifuged at 10
000 rpm at 4 °C for 5 min to remove any solid debris; the supernatants were then stored frozen at −80 °C for subsequent metabolomic analysis. The study was approved by the Ethical Committee of Heilongjiang University of Chinese Medicine and was conducted according to the principles expressed in the Declaration of Helsinki.
Liver histology and biochemical assay
We quantified the levels of ALT, AST, ALP, TG, T-BIL, TP, ALB, CHOL, HA, LN, PCIIINP, CIV activities using assay kits according to the manufacturer's instructions. Liver samples from each rat were fixed in 10% neutral buffered formaldehyde solution, embedded in paraffin, stained with hematoxylin-eosin (HE) and Masson trichrome collagen stain and then examined under an optical microscope.
Metabolomics analysis
Preparation of urine Samples.
Thawed urine samples were collected via centrifugation at 13
000 rpm for 10 minutes at 4 °C, and then filtered through a 0.22 μm syringe filter, 3 μL of the supernatant were injected into the UPLC/MS.
Chromatographic conditions.
Urine metabolite profiling was performed using a Waters ACQUITY UPLC system (Waters Corporation, Milford, MA). The chromatographic separation was conducted on an Acquity HSS T3 column (100 mm × 2.1 mm, 1.8 μm). The column oven temperature was set to be 45 °C, injection volume at 3 μL and the flow-rate at 0.4 ml min−1 without a split. The mobile phase consisted of phase A (acetonitrile containing 0.1% formic acid) and phase B (water with 0.1% formic acid). The gradient was as follows: 0–2.5 min, 99–89% B; 2.5–4.5 min, 89–79% B; 4.5–7.0 min, 79–60% B; 7.0–7.5 min, 60–50% B; 7.5–10 min, 50–1% B; 10–12 min, 1% B; 12–12.5 min, 1–99% B; 12.5–15 min, 99% B.
Accurate mass time-of-flight mass spectrometry.
A Waters Micromass Q-TOF micro Synapt High Definition Mass Spectrometer (Synapt HDMS, Waters, Manchester, UK) equipped with electrospray ionization in positive and negative modes was used. MS/MS spectra were acquired as two separate positive ion (ESI+) and negative ion (ESI−) polarity runs for each sample. The following parameters were employed: ESI+: a source temperature of 110 °C; capillary voltage was set at 3 kV; cone voltage at 25 V; an extraction cone voltage of 3.0 V; the desolvation temperature was set at 300 °C; a cone gas flow of 50 L h−1; a desolvation gas flow of 500 L h−1; ESI−: source temperature at 110 °C; capillary voltage was set at 3 kV; a cone voltage of 30 V; an extraction cone voltage of 2.5 V; the desolvation temperature was set at 300 °C; a cone gas flow of 50 L h−1; a desolvation gas flow of 400 L h−1. Nitrogen was used as the drying gas, the desolvation gas flow rate was set at 500 L h−1, and cone gas flow was maintained at 50 L h−1. Collision energy was set at 35 eV in the MS/MS mode for the identification of potential metabolites. All the data were acquired using an independent reference lock mass (Leucine enkephalin) via the LockSpray™ interface to ensure accuracy and reproducibility during the MS analysis. Centroid data were collected at a rate of 1 MS spectrum per second from 100 to 1000 m/z with a scan time of 0.2 s, an inter-scan delay of 0.1 s, and a lock spray frequency of 10 s.
Multivariate statistical analysis.
The ion intensities for each peak were normalized within each sample, and then were introduced to EZinfo 2.0 software for principal components analysis (PCA), partial least-squared discriminant analysis (PLS-DA), orthogonal partial least-squared discriminant analysis (OPLS-DA). EZinfo 2.0 analysis software was used to extract molecular features in each of the sample. The VIP-plot was constructed from the OPLS which was carried out to select distinct variables as potential biomarkers which were chosen based on their contribution to the variation and correlation within the data set. The ions furthest away from the origin in the VIP-plot may be therefore regarded as the differentiating metabolites. Student's t-test was performed to identify features with differential abundances across groups.
Metabolite identification.
Exact MS data from redundant m/z peaks were first used to help confirm the metabolite molecular mass. The candidate biomarkers were annotated based on retention behavior, mass assignment, and online database query. The accurate mass and structure information of candidate metabolites were matched with those of metabolites obtained from HMDB, METLIN, and Lipid Maps. The mass tolerance between the measured m/z values and the exact mass of the components of interest was set to be within 5 mDa. Pathway analysis was performed using the MetaboAnalyst tool.
iTRAQ-based quantitative proteomic analysis
Sample preparation and protein extraction.
Plasma was fractionated using the ProteinMiner Protein Enrichment Small-Capacity kit (Bio-Rad Laboratories, Hercules, CA, USA). Protein solutions were reduced for 1 h at 56 °C with 10 mM dithiothreitol and were cysteine blocked with 55 mM iodoacetamide at room temperature for 10 min. Each sample was precipitated with four times the volume of cold acetone.
Protein digestion and peptide tagging.
Protein solutions were digested for 24 h with 10 μg L-1-(4-tosylamido)-2-phenylethyl tosylphenylalanyl chloromethyl ketone treated trypsin. Each peptide solution was labeled for 3 h at room temperature using an iTRAQ reagent (Applied Biosystems, Foster City, CA, USA). The reaction was terminated by adding MilliQ water, and the samples were labeled with tagged iTRAQ reagents.
Nano LC with tandem mass spectrometry.
Nanoflow electrospray ionization tandem mass spectrometric analysis of peptide samples was carried out using Thermo fisher Q-Exactive (Thermo Scientific, Bremen, Germany) interfaced with an Agilent's 1200 Series nanoflow LC system. The chromatographic capillary columns used were packed with Magic C18 (particle size 5 um, 100 μm × 150 mm, Michrom Bioresources, CA, USA). The peptides were eluted using a linear gradient (0.01–50 min, 0% B; 50–51 min, 0–5% B; 51–71 min, 5–30% B; 71–76 min, 30–50% B; 76–81 min, 50% B; 81–86 min, 50–100% B; 86–96 min, 100% B.) over 96 min.
Protein identification and quantitative analysis.
Peptides were quantified using the centroided reporter ion peak intensity. Intra-sample channels were normalized based on the median ratio for each channel across all proteins. Multiple isobaric tag samples were normalized by comparing the median protein ratios for the reference channel. Protein quantitative values were derived only from uniquely assigned peptides. The minimum quantitative value for each spectrum was calculated to be 5.0% of the highest peak. Protein quantitative ratios were calculated as the median of all peptide ratios. Fold-changes >1.2 or and a two-tailed p-value < 0.05 were set as cut-off values to designate significant protein expression changes. Independent component analysis (ICA) was performed to visualize the similarity of selected features between the test groups under different conditions.
Database screening and functional annotation.
Data were processed using BioTools software (Bruker Daltonics, Inc.). Gene Ontology (GO) functional classifications were analyzed with Blast2GO software, and GO enrichment analysis was performed to identify GO terms that were significantly enriched in DEPs. Identified proteins were further analysed using STRING (http://string-db.org/) for protein–protein interactions. All statistical analyses were performed using the Student's t-test.
Results
Histopathology and biochemical analysis
For histopathological analysis, liver samples were quickly obtained and fixed in a 10% neutral buffered formaldehyde solution, embedded in paraffin and sectioned. Paraffin sections were stained with H&E for routine examination, followed by Masson staining for collagen. H&E staining and Masson's staining showed the presence of hepatic fibrosis, marked fatty degeneration, and collagen accumulation in the model group (Fig. 1A). The HE staining showed that ALD was more severe in the 12W-treated group than in the 7W-treated group. Representative figures of HE-stained sections of liver tissue from the control group were evaluated as a normal histology. The liver lobules of the control group showed no pathological changes and the liver cells were normal. Compared with controls, liver cell steatosis was apparent in liver samples at 4W. Foci infiltration around fibrosis tissue can be seen. Large numbers of black and brown particles were deposited and accompanied by fibrosis tissue at 7W, and a similar pattern was evident at 12W. The collagen was shown to be green-colored in the Masson-stained section at 12W.
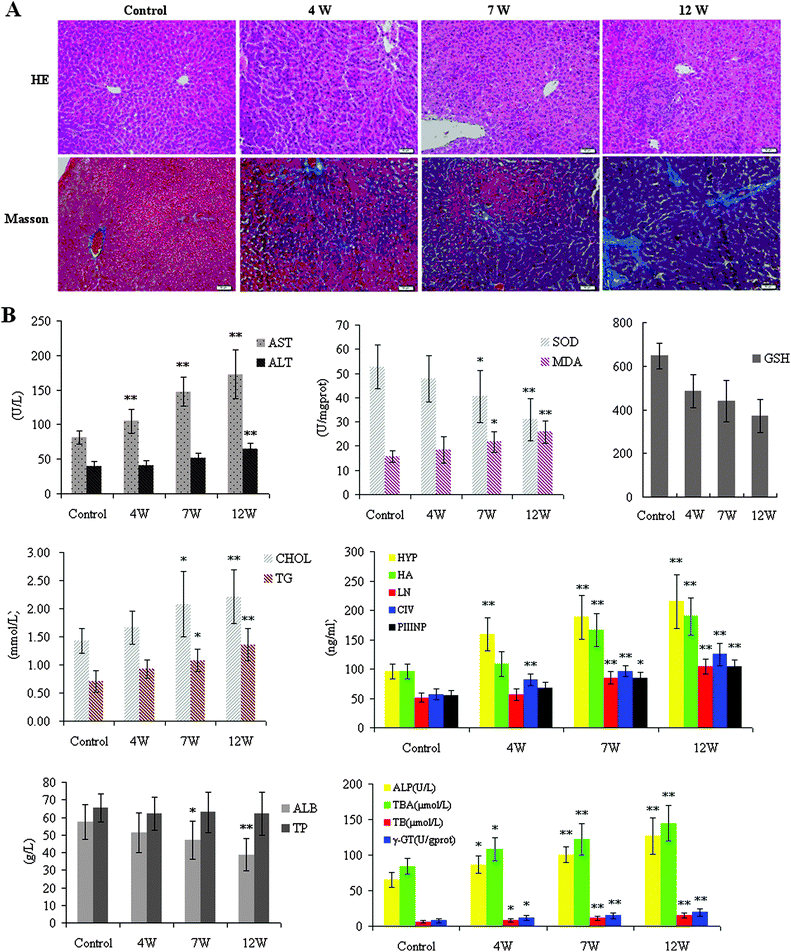 |
| Fig. 1 Representative photomicrographs of rat liver tissues with Hematoxylin and Eosin (200×) and Masson Staining (200×) in the experimental rats (A). Liver tissue from the model group showed disorderly hepatocyte cords, severe fatty degeneration, spotty or focal necrosis and infiltration of inflammatory cells; hemorrhagic necrosis with foci of lymphomonocytic infiltration around fibrosis tissue can be seen. Biochemical analysis (B). The extent of liver injury was assessed by measuring the enzymes of the liver in the mouse serum. As shown in the liver index, serum activities of ALT and AST were found to be significantly increased in the 12W-treated group when compared with the control group. The value of MDA in the serum was decreased in the 12W-treated group, but the values of the activities of SOD in the liver tissue were increased compared to the control group, and there was no significant change in either group at 4W. Both serum CHOL, TG, and the liver tissue Hyp, HA, LN, PCIIINP, CIV were markedly increased at 12W. The TP content in the 12W-treated group was markedly decreased, while the levels of ALP, T-BA, TB, and r-GT were increased, compared with that of controls. A significant increase of the liver enzymes in the serum was occurred at 12W. The values are expressed as mean ± SD. *p < 0.05 and **p < 0.01, vs. control group. | |
The extent of liver injury was assessed by measuring the enzymes of the liver in the mouse serum. As shown in the liver index, it was significantly different among the experimental groups. The liver index was decreased in rat groups compared to controls (Fig. 1B). AST and ALT concentrations in serum were used as biochemical markers to evaluate hepatic injury. Serum activities of ALT and AST were found to be significantly increased in the 12W-treated group when compared with the control group (p < 0.01). The value of MDA in the serum was decreased (p < 0.01) in the 12W-treated group, but the values of the activities of SOD in the liver tissue were increased (p < 0.01) compared to the control group, and there was no significant change in either group at 4W. Both serum CHOL, TG, and the liver tissue Hyp, HA, LN, PCIIINP, CIV were markedly increased at 12W. The TP content in the 12W-treated group was markedly decreased (p < 0.01), while the level of ALP, T-BA, TB, and r-GT was increased, compared with that of controls. A significant increase levels of the liver enzymes in the serum was occurred at 12W, suggesting that the pathological changes of ALD. Consequently, combined with the histopathological manifestation results, we successfully established ALD in animal models at 12W.
Urine metabolomic profiles
Metabolomics involves studying the processes of all metabolites in diseased samples, thereby revealing disease-related metabolic pathways. The total ion chromatograms exhibited the ideal separation result under the optimized gradient elution procedure. The urine metabolomic profile of each sample consisted of approximately 8000 peaks. Low molecular mass metabolites could be separated well in the short time of 10 min. In order to better visualize the subtle similarities and differences among these complex data sets, multiple pattern recognition methods were employed to phenotype the urine metabolome of rats.
Multivariate statistical analysis.
Urine samples were analyzed in both positive and negative ionization modes with the UPLS/MS. Typically, the trajectory analysis of PCA score plots for the alcohol treatment in the positive mode (Fig. 2A) and the negative ionization mode (Fig. 3A) can really reflect a clear separation between the model and control groups. The PCA plot of the model groups deviated from the control group on 12W reaching the maximum trend, 4W to 7W gradually away from the normal state. The tracks of the metabolic profiles at different time points also clearly demonstrate the time dependent changes in the urine metabolites, which suggest that urinary biochemical perturbation significantly happened in model groups. To maximize the differences between the groups and determine the variables that contribute to discrimination, the OPLS-DA, a supervised pattern recognition method, was further employed for metabolic data (Fig. 2B and 3B).
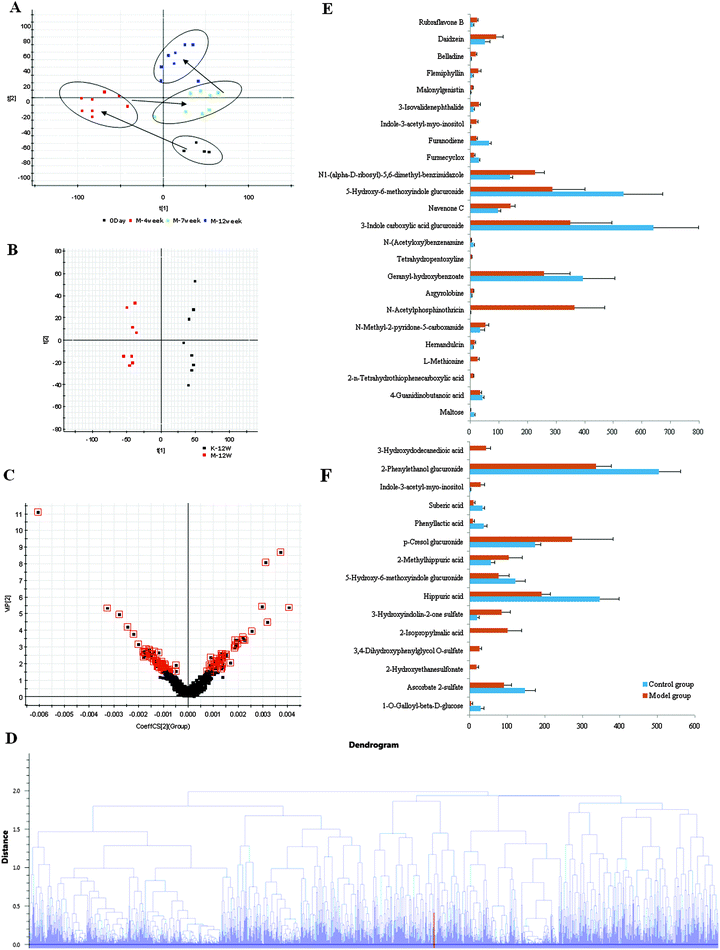 |
| Fig. 2 Metabolomic multivariate analysis of untargeted metabolomics data. Trajectory analysis of principal components analysis score plots of the alcoholic ALD at the continuous 12W in the positive mode (A); partial least-squared discriminant analysis plot of ALD in the positive mode (B); panel C shows the VIP-score plots constructed from the supervised orthogonal partial least-squared discriminant analysis of urine. Ions with the highest abundance and correlation in the model group with respect to the controls are present on the upper far right hand quadrant, whereas ions with the lowest abundance and correlation in the ALD with respect to the control group are residing in the lower far left hand quadrant. (D) Dendrogram analysis of metabolite candidates. Histogram plots show the relative signal intensities for marker metabolites for the control and model groups in the positive mode (E) and the negative mode (F). | |
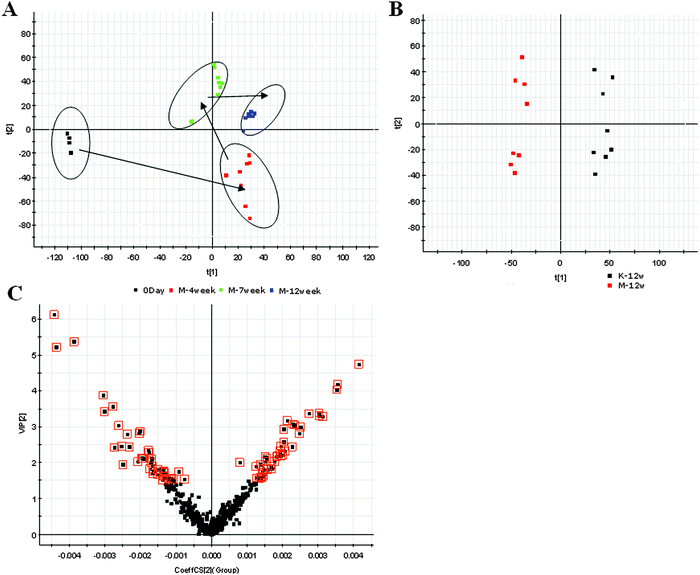 |
| Fig. 3 Metabolomic profiling of AFLD. Principal components analysis model results for the ALD group in the negative mode (A). Partial least-squared discriminant analysis plot of ALD in the negative mode (B). Panel C shows the combination of VIP-score plots constructed from the supervised orthogonal partial least-squared discriminant analysis of urine (ESI− mode). | |
Identification of metabolite candidates.
All chromatographic peaks were extracted for the discovery of metabolic biomarkers associated with ALD. For further analysis of feature ions, the VIP-plot (Fig. 2C and 3C) from the OPLS was constructed and the dendrogram analysis (Fig. 2D and 3D) of metabolite candidates was carried out to select distinct variables as potential biomarkers for distinguishing ALD from controls. From the corresponding VIP-plot, the ions furthest away from the origin may be therefore regarded as the differentiating metabolites. We generated VIP plots from the OPLS-DA with a threshold of 1.5 to identify the metabolites that significantly contribute to the clustering between groups. 21 differentially expressed small molecule metabolites considered to be strong contributors were distinguished from those of the healthy controls (p < 0.05, VIP > 1.5, 8 ions in the positive mode and 13 ions in the negative mode). Of note, it was found that, among them, 13 metabolites were upregulated and 8 metabolites were downregulated (Table 1). Histogram plots of Fig. 2E and F show relative signal intensities for 21 metabolites in the control and the ALD group.
Table 1 Urinary biomarker candidates in alcoholic liver disease rat identified by UPLC/ESI-Q/TOF-MS
No. |
Retention (time/min) |
Ion form |
Determined (mass/Da) |
Calculated (mass/Da) |
Error (mDa) |
Formula |
Identity |
VIP-value |
t-Test /p-value |
Trend |
Detected metabolites in both positive and negative modes.
|
1 |
1.05 |
[M + H]+ |
150.0581 |
150.0589 |
−0.8 |
C5H11NO2S |
L-Methionine |
2.9 |
4.65 × 10−8 |
↑ |
2 |
1.78 |
[M + H]+ |
153.0647 |
153.0664 |
−1.7 |
C7H8N2O2 |
N-Methyl-2-pyridone-5-carboxamide |
1.9 |
3.88 × 10−2 |
↑ |
3 |
1.81 |
[M + H]+ |
246.0547 |
246.0507 |
4.0 |
C7H14NO5P |
N-Acetylphosphinothricin |
11.1 |
1.19 × 10−7 |
↑ |
4 |
3.59 |
[M + H]+ |
152.0698 |
152.0712 |
−1.4 |
C8H9NO2 |
N-(Acetyloxy)benzenamine |
1.5 |
1.03 × 10−3 |
↓ |
5 |
4.03 |
[M + H]+ |
338.0883 |
338.0876 |
0.7 |
C15H15NO8 |
3-Indole carboxylic acid glucuronide |
8.7 |
1.87 × 10−3 |
↓ |
6 |
4.26 |
[M + H]+ |
340.1020 |
340.1022 |
−0.2 |
C15H17NO8 |
5-Hydroxy-6-methoxyindole glucuronide |
8.1 |
1.46 × 10−3 |
↓ |
7 |
4.61 |
[M + H]+ |
279.1328 |
279.1345 |
−1.7 |
C14H18N2O4 |
N1-(alpha-D-ribosyl)-5,6-dimethyl-benzimidazole |
5.3 |
6.08 × 10−6 |
↑ |
8 |
5.91 |
[M + H]+ |
338.1247 |
338.1240 |
0.7 |
C16H19NO7 |
Indole-3-acetyl-myo-inositol |
2.7 |
1.08 × 10−7 |
↑ |
9 |
0.70 |
[M − H]− |
377.0752 |
377.0720 |
3.2 |
C13H16O10 |
1-O-Galloyl-beta-D-glucose |
2.1 |
6.19 × 10−6 |
↓ |
10 |
0.87 |
[M − H]− |
124.9908 |
124.9909 |
−0.1 |
C2H6O4S |
2-Hydroxyethanesulfonate |
1.8 |
4.51 × 10−7 |
↑ |
11 |
0.89 |
[M − H]− |
295.0138 |
295.0124 |
1.4 |
C8H10O7S |
3,4-Dihydroxyphenylglycol O-sulfate |
2.2 |
2.63 × 10−8 |
↑ |
12 |
1.26 |
[M − H]− |
221.0656 |
221.0661 |
−0.5 |
C7H12O5 |
2-Isopropylmalic acid |
4.2 |
4.78 × 10−6 |
↑ |
13 |
1.87 |
[M − H]− |
227.9975 |
227.9967 |
0.8 |
C8H6NO5S |
3-Hydroxyindolin-2-one sulfate |
3.4 |
3.04 × 10−6 |
↑ |
14a |
3.61 |
[M − H]− |
178.0515 |
178.0504 |
1.1 |
C9H9NO3 |
Hippuric acid |
5.2 |
2.71 × 10−6 |
↓ |
15 |
4.26 |
[M − H]− |
338.0865 |
338.0876 |
−1.1 |
C15H17NO8 |
5-Hydroxy-6-methoxyindole glucuronide |
2.4 |
5.408 × 10−3 |
↓ |
16 |
4.28 |
[M − H]− |
192.0649 |
192.0661 |
−1.2 |
C10H11NO3 |
2-Methylhippuric acid |
2.6 |
3.11 × 10−3 |
↑ |
17 |
5.10 |
[M − H]− |
283.0810 |
283.0818 |
−0.8 |
C13H16O7 |
p-Cresol glucuronide |
5.5 |
2.45 × 10−6 |
↑ |
18 |
5.51 |
[M − H]− |
173.0815 |
173.0814 |
0.1 |
C8H14O4 |
Suberic acid |
2.0 |
4.17 × 10−6 |
↓ |
19 |
5.91 |
[M − H]− |
336.1071 |
336.1083 |
−1.2 |
C16H19NO7 |
Indole-3-acetyl-myo-inositol |
2.2 |
3.2 × 10−6 |
↑ |
20 |
6.77 |
[M − H]− |
297.0970 |
297.0974 |
−0.4 |
C14H18O7 |
2-Phenylethanol glucuronide |
5.4 |
1.08 × 10−5 |
↓ |
21 |
8.01 |
[M − H]− |
291.1290 |
291.1287 |
0.3 |
C12H22O5 |
3-Hydroxydodecanedioic acid |
2.8 |
7.99 × 10−8 |
↑ |
Metabolic pathways and function analysis.
To gain insight into the metabolic mechanism of ALD, metabolic pathways of the significantly altered metabolites were analyzed using the “pathway analysis” module within the MetaboAnalyst software. We identified a total of seven distinct metabolic pathways that were significantly altered in the urine (Table S1, ESI†) samples from the model group. The detailed analysis of the most relevant pathways of ALD was performed by the MetaboAnalyst's tool. A total of 7 pathways which were identified together are important for the host response to ALD (Fig. S1A, ESI†). The predominant hits were pathways involved in pentose and glucuronate interconversions, starch and sucrose metabolism, cysteine and methionine metabolism, etc. The detailed construction of the perturbed pathways of pentose and glucuronate interconversions (Fig. S1B, ESI†), starch and sucrose metabolism (Fig. S1C, ESI†), cysteine and methionine metabolism (Fig. S1D, ESI†) with a higher score had yield satisfactory results (details in Table S1, ESI†).
iTRAQ-proteomics analysis
ICA aggregates the set of proteins studied into one point per sample in the two-dimensional coordinate system spanned by the two most relevant independent components (Fig. S2, ESI†). These trajectories reveal that the proteome profiles markedly change immediately after ALD. ICA can really reflect a clear separation between the 12W and the control group.
Differentially expressed proteins.
To examine the difference in serum proteome between ALD rat models and controls, we conducted the analysis by iTRAQ. After trypsin digestion of total proteins, peptides from the model and control groups were labeled with 114 and 113, respectively. To identify the differentially expressed proteins (DEPs), the relative protein expression values were compared between groups. These proteins could provide leads for potentially useful diagnostic and prognostic biomarkers for disease progression. The confidence value for each peptide was calculated based on agreement between the experimental and theoretical fragmentation patterns. Each protein was provided with a confidence score based on confidence scores of its constituent peptides with unique spectral patterns. The threshold was set as <0.83 and >1.2 for iTRAQ labeling, 85 proteins were identified as DEPs between mouse models and controls at a 12 w time point. Of these, 33 were down-regulated (Table S2, ESI†) and 52 were up-regulated in ALD model rat. To determine the proteins with abundance changes, a histogram was used to generate the number of proteins in different abundance ratios (Fig. 4).
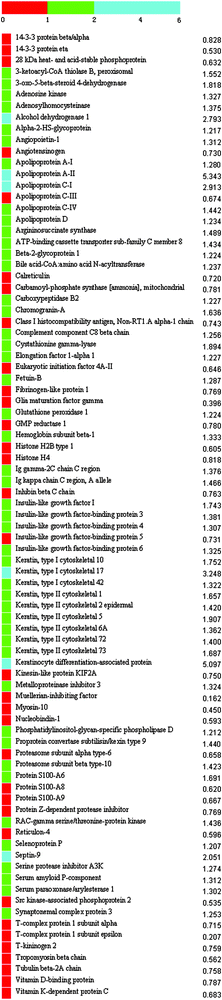 |
| Fig. 4 Heatmap of the differentially regulated proteins in biosamples. | |
Gene ontology analysis.
We subjected the DEPs to GO analysis and categorized them according to molecular function, biological processes and pathways. When we analyzed these proteins for molecular function (Fig. S3, ESI†), we found that over 20% of proteins were grouped under “metabolism” such as the macromolecule metabolic process, the primary metabolic process and the cellular metabolic process etc. The remaining DEPs were grouped under the “cellular process”, “biological regulation”, and the “multicellular organismal process”. Based on the analysis of the cellular component, 51.13% of the DEPs were annotated proteins. In the molecular function analysis of GO, most DEPs were associated with the function of binding. Classification analysis of the biological processes with the UniProt database and the KEGG online tool (http://david.abcc.ncifcrf.gov/) showed that the DEPs are mainly involved in the processes of the p53 signaling pathway, the PPAR signaling pathway, fatty acid metabolism, the TGF-beta signaling pathway, the mTOR signaling pathway, cytokine–cytokine receptor interaction, purine metabolism, natural killer cell mediated cytotoxicity, the neurotrophin signaling pathway, and the Fc epsilon RI signaling pathway, respectively (Table 2). These data suggest that interruption of these pathways may provide a means to the development of molecularly targeted therapies for ALD. Interestingly, the p53 signaling pathway (Fig. S4, ESI†) including insulin-like growth factor-binding protein 3, insulin-like growth factor I, and the Fc epsilon RI signaling pathway including the Ig gamma-2C chain C region, RAC-gamma serine/threonine-protein kinase were firstly reported in ALD. STRING analysis depicts known protein–protein interactions among genes of interest in overrepresented pathways (Fig. 5). Tumor protein p53 acts as a tumor suppressor in many tumor types; induces growth arrest or apoptosis depending on the physiological circumstances and the cell type. It is also involved in cell cycle regulation as a trans-activator that acts negatively to regulate cell division by controlling a set of genes required for this process.
Table 2 List of the pathways associated with differentially expressed proteins in the iTRAQ experiments
No. |
Pathway |
Pathway ID |
Proteins |
1 |
p53 signaling pathway |
ko04115 |
P15473, P08025 |
2 |
PPAR signaling pathway |
ko03320 |
P06759, P04639, P07871, P04638 |
3 |
Fatty acid metabolism |
ko00071 |
P07871, P06757 |
4 |
TGF-beta signaling pathway |
ko04350 |
P49000, Q9WUK5 |
5 |
mTOR signaling pathway |
ko04150 |
Q63484, P08025 |
6 |
Cytokine–cytokine receptor interaction |
ko04060 |
P49000, Q9WUK5 |
7 |
Purine metabolism |
ko00230 |
Q9Z244, Q64640 |
8 |
Natural killer cell mediated cytotoxicity |
ko04650 |
P15978, P20762 |
9 |
Neurotrophin signaling pathway |
ko04722 |
P68511, P35213, Q63484 |
10 |
Fc epsilon RI signaling pathway |
ko04664 |
P20762, Q63484 |
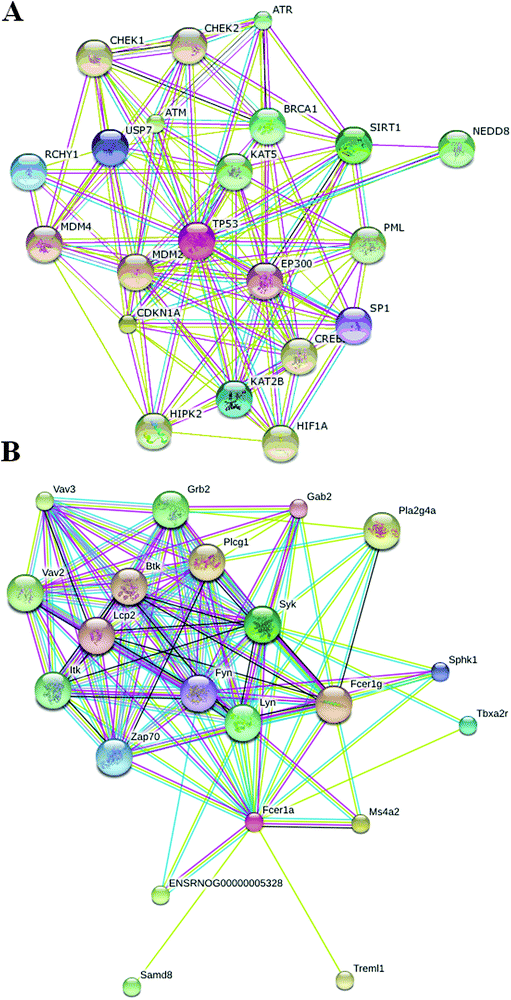 |
| Fig. 5 STRING analysis depicting known protein–protein interactions among genes of interest in overrepresented pathways. Modes of action are shown in different colors. Differentially expressed proteins are represented as pill-shaped nodes, while proteins are shown as spheres. p53 signaling pathway (A) and Fc epsilon RI signaling pathway (B). | |
Discussion
Alcohol consumption is one of the world's major risk factors for disease development. Ongoing studies show that alcohol consumption is associated with fatty liver disease in mammals. Recent advances in metabolomic technologies have enabled us to elucidate the effect of alcohol consumption on metabolism. Jaremek and co-workers have investigated the relation of alcohol intake and serum metabolite concentrations and identified potential biomarkers that could predict high levels of intake.18 It suggests that alcohol affects mostly the sphingolipid, glycerophospholipid and ether lipid metabolism. Clugston and co-workers analyzed altered hepatic lipid metabolism in mice fed with alcohol using a targeted lipidomic approach.19 They found that chronic alcohol consumption is associated with increased hepatic free fatty acid levels and decreased fatty acyl-CoA levels associated with decreased mitochondrial fatty acid oxidation and decreased fatty acyl-CoA synthesis, increased hepatic ceramide levels associated with higher levels of the precursor molecules sphingosine and sphinganine; and increased hepatic levels of the endocannabinoid anandamide associated with decreased expression of its catabolic enzyme fatty acid amide hydrolase. Kalhan et al. examined the plasma metabolomic profile in nonalcoholic fatty liver disease.20 Their results suggested markedly higher levels of glycocholate, taurocholate and glycochenodeoxycholate in subjects. A study showed that the expression of two proteins associated with alcoholic liver disease, peroxiredoxin 6 and aldehyde dehydrogenase 2, was down-regulated in ethanol fed rats.21 As far as we are aware, no metabolomics–proteomics profiling analyses of ALD rats with alcohol consumption have yet been conducted.
In the present study, special attention was paid to the time points of the 1W and 12W which are closely associated with ALD, in the experimental animal model. The results showed that histopathology and biochemical results had significant differences between the controls and 12W. Through biochemical analysis combined with the histopathological results, we successfully established ALD in animal models at 12W. Additionally, alcoholic-induced ALD rat may be a useful model for the determination of ALD. Metabolic changes are associated with a number of complex diseases. A panel of biomarkers to characterize disease could be useful for ALD diagnostics. In this paper, UPLC-MS combined with the pattern recognition analysis approach was used to simplify and quicken the identification of the metabolites of ALD. UPLC-MS based metabolomics could be an advanced tool to help us find metabolites due to its capacity of processing large datasets, and classifying of sample groups, as well as its indiscriminative nature of metabolites. By using our metabolomics platform, PCA revealed a statistically significant separation between the ALD and control samples. An OPLS model was built to find biomarker candidates of ALD and 21 statistically important variables with VIP > 1.5 were defined, many are in various stages of progress at the ALD. Differential metabolites from the urine metabolome indicate the disrupted pentose and glucuronate interconversions, starch and sucrose metabolism, cysteine and methionine metabolism pathways, etc., would be helpful for understanding the occurrence and development of ALD.
Proteomics, which measures mature proteins, could be used to closely observe biological functions in the body.22 Proteomics research is a potentially useful and effective tool for studying pathogenesis, establishing prognosis and determining treatment outcomes in a variety of diseases.23 Though proteomics is widely performed, the changes in the serum proteomes of ALD are rarely reported due to the complicated mechanism of ALD, therefore this study was also to describe the changes in protein levels in ALD using iTRAQ proteomics. The authors hypothesized that this approach had the potential to ultimately be beneficial for the identification of proteins that were important in the progression of ALD. We constructed the mouse model of the early stage of ALD using alcohol. Based on proteomics analysis, we investigated the proteomic changes and pathways leading to ALD by alcohol. For data analysis, we optimized the normalization of iTRAQ signals and quantified the expression of proteins identified. iTRAQ identified 85 different proteins that had ≥1.2-fold differences in the expression level between the ALD and the controls. Finally, the most significant pathway elucidated by bioinformatics methods was subsequently validated. Bioinformatics analysis of the DEPs illustrated the enrichment of metabolism related processes, such as the macromolecule metabolic process, the primary metabolic process and the cellular metabolic process etc. Studies have shown that the p53 signaling pathway, the PPAR signaling pathway, fatty acid metabolism, the TGF-beta signaling pathway, the mTOR signaling pathway, the cytokine–cytokine receptor interaction, purine metabolism, natural killer cell mediated cytotoxicity, the neurotrophin signaling pathway, and the Fc epsilon RI signaling pathway are critical in the pathogenesis of ALD. Interestingly, the p53 signaling pathway and the Fc epsilon RI signaling pathway were firstly reported in ALD. This study helps to achieve a better understanding of the complexity of metabolic regulations of ALD, which may shed light on metabolism to ALD. GO analysis indicated that these proteins are involved in transport, biological regulation, cellular processes, immune response, and metabolic processes. The DEPs may be involved in the host response to ALD at the molecular level and may be potential diagnostic biomarkers for ALD. It also provides insights into the molecular basis for the use of PPAR signaling agonists, which has been advocated for the treatment of ALD.
The findings yield a valuable tool that can engender new insights into the pathophysiology of ALD and advance the early diagnosis and monitor the progression of ALD, and showed that omics has the potential as a promising screening tool for exploring the essence of ALD disease. In addition, future research will be directed to the biological interpretation: which pathways were involved in the biochemical changes associated with the onset, development and progression of ALD, and whether these changes are the same during onset and progression, or if different changes of biochemistry occur at the different stages of ALD. Thus, the findings presented contribute to a better understanding of the molecular signature of ALD and may provide the biological background to pharmacological interventions in the future.
Conclusions
ALD represents a significant cause of morbidity and mortality worldwide and constitute a problem of global public health importance. Therefore, it needs more reliable metabolism information for effective treatment. We successfully reproduced ALD in animal models from liver histology combined with biochemical results. Omics is a powerful approach for the comprehensive assessment of endogenous metabolites or proteins and attempts were made to systematically identify them from biological samples. In this study, the proteomics and metabolomics improve discrimination between ALD and control. The metabolomics approach coupled with multivariate statistical methods showed 21 altered metabolic biomarkers indicating that ALD could cause more severe disturbances in pentose and glucuronate interconversions, starch and sucrose metabolism, cysteine and methionine metabolism, etc. Using iTRAQ based proteomic methods, we identified 85 differentially expression proteins related to ALD, which might provide clues to clarify novel mechanisms underlying alcohol-induced ALD. Of these, 33 were down-regulated and 52 were up-regulated in ALD. Interestingly, integrated proteomic and metabolomic profiling reveal that the p53 signaling pathway and the Fc epsilon RI signaling pathway were firstly found in ALD. These data suggest that the interruption of these pathways may provide a means to the development of molecularly targeted therapies for ALD.
Conflicts of interest
The authors declare no competing financial interests.
Acknowledgements
This work was supported by grants from the Key Program of Natural Science Foundation of State (Grant No. 81430093, 81173500, 81373930, 81302905, 81202639), National Key Subject of Drug Innovation (Grant No. 2015ZX09101043-005, 2015ZX09101043-011), Natural Science Foundation of Heilongjiang Province of China (H2015038), Youth Innovative Talent Program of Heilongjiang Province of China (UNPYSCT-2015118), Specialized Research Fund for the Doctoral Program of Higher Education (Grant No. 20122327120006), Fund Project of Heilongjiang Provincial Department of Education (Grant No. 12521498).
References
- O. George, C. Sanders, J. Freiling, E. Grigoryan, S. Vu, C. D. Allen, E. Crawford, C. D. Mandyam and G. F. Koob, Recruitment of medial prefrontal cortex neurons during alcohol withdrawal predicts cognitive impairment and excessive alcohol drinking, Proc. Natl. Acad. Sci. U. S. A., 2012, 109, 18156–18161 CrossRef CAS PubMed.
- N. A. Nasrallah, J. J. Clark, A. L. Collins, C. A. Akers, P. E. Phillips and I. L. Bernstein, Risk preference following adolescent alcohol use is associated with corrupted encoding of costs but not rewards by mesolimbic dopamine, Proc. Natl. Acad. Sci. U. S. A., 2011, 108, 5466–5471 CrossRef CAS PubMed.
- T. Neumann, A. Helander, H. Dahl, T. Holzmann, B. Neuner, E. Weiss-Gerlach, C. Müller and C. Spies, Value of ethyl glucuronide in plasma as a biomarker for recent alcohol consumption in the emergency room, Alcohol Alcohol, 2008, 43, 431–435 CrossRef CAS PubMed.
- A. Sreekumar, L. M. Poisson, T. M. Rajendiran, A. P. Khan, Q. Cao, J. Yu, B. Laxman, R. Mehra, R. J. Lonigro, Y. Li, M. K. Nyati, A. Ahsan, S. Kalyana-Sundaram, B. Han, X. Cao, J. Byun, G. S. Omenn, D. Ghosh, S. Pennathur, D. C. Alexander, A. Berger, J. R. Shuster, J. T. Wei, S. Varambally, C. Beecher and A. M. Chinnaiyan, Metabolomic profiles delineate potential role for sarcosine in prostate cancer progression, Nature, 2009, 457, 910–914 CrossRef CAS PubMed.
- N. L. Kuehnbaum, J. B. Gillen, M. J. Gibala and P. Britz-McKibbin, Personalized metabolomics for predicting glucose tolerance changes in sedentary women after high-intensity interval training, Sci. Rep., 2014, 4, 6166 CrossRef CAS PubMed.
- X. Wang, A. Zhang and H. Sun, Power of metabolomics in diagnosis and biomarker discovery of hepatocellular carcinoma, Hepatology, 2013, 57, 2072–2077 CrossRef CAS PubMed.
- E. Sabidó, O. Quehenberger, Q. Shen, C. Y. Chang, I. Shah, A. M. Armando, A. Andreyev, O. Vitek, E. A. Dennis and R. Aebersold, Targeted proteomics of the eicosanoid biosynthetic pathway completes an integrated genomics-proteomics-metabolomics picture of cellular metabolism, Mol. Cell. Proteomics, 2012, 11, M111.014746 Search PubMed.
- J. F. Kellie, R. E. Higgs, J. W. Ryder, A. Major, T. G. Beach, C. H. Adler, K. Merchant and M. D. Knierman, Quantitative measurement of intact alpha-synuclein proteoforms from post-mortem control and Parkinson's disease brain tissue by intact protein mass spectrometry, Sci. Rep., 2014, 4, 5797 CAS.
- L. Sun, M. M. Bertke, M. M. Champion, G. Zhu, P. W. Huber and N. J. Dovichi, Quantitative proteomics of Xenopus laevis embryos: expression kinetics of nearly 4000 proteins during early development, Sci. Rep., 2014, 4, 4365 Search PubMed.
- T. J. Wang, M. G. Larson, R. S. Vasan, S. Cheng, E. P. Rhee, E. McCabe, G. D. Lewis, C. S. Fox, P. F. Jacques, C. Fernandez, C. J. O'Donnell, S. A. Carr, V. K. Mootha, J. C. Florez, A. Souza, O. Melander, C. B. Clish and R. E. Gerszten, Metabolite profiles and the risk of developing diabetes, Nat. Med., 2011, 17, 448–453 CrossRef CAS PubMed.
- G. J. Patti, O. Yanes, L. P. Shriver, J. P. Courade, R. Tautenhahn, M. Manchester and G. Siuzdak, Metabolomics implicates altered sphingolipids in chronic pain of neuropathic origin, Nat. Chem. Biol., 2012, 8, 232–234 CrossRef CAS PubMed.
- Y. Chen, J. Xu, R. Zhang, G. Shen, Y. Song, J. Sun, J. He, Q. Zhan and Z. Abliz, Assessment of data pre-processing methods for LC-MS/MS-based metabolomics of uterine cervix cancer, Analyst, 2013, 138(9), 2669–2677 RSC.
- T. Pacchiarotta, R. J. Derks, E. Nevedomskaya, W. van der Starre, J. van Dissel, A. Deelder and O. A. Mayboroda, Exploratory analysis of urinary tract infection using a GC-APCI-MS platform, Analyst, 2015, 140(8), 2834–2841 RSC.
- X. Wang, A. Zhang, Y. Han, P. Wang, H. Sun, G. Song, T. Dong, Y. Yuan, X. Yuan, M. Zhang, N. Xie, H. Zhang, H. Dong and W. Dong, Urine metabolomics analysis for biomarker discovery and detection of jaundice syndrome in patients with liver disease, Mol. Cell. Proteomics, 2012, 11, 370–380 Search PubMed.
- L. Nadal-Desbarats, N. Aïdoud, P. Emond, H. Blasco, I. Filipiak, P. Sarda, F. Bonnet-Brilhault, S. Mavel and C. R. Andres, Combined 1H-NMR and 1H-13C HSQC-NMR to improve urinary screening in autism spectrum disorders, Analyst, 2014, 139(13), 3460–3468 RSC.
- H. Sun, A. Zhang, G. Yan, C. Piao, W. Li, C. Sun, X. Wu, X. Li, Y. Chen and X. Wang, Metabolomic analysis of key regulatory metabolites in hepatitis C virus-infected tree shrews, Mol. Cell. Proteomics, 2013, 12, 710–719 CAS.
- A. Zhang, H. Sun and X. Wang, Urinary metabolic profiling of rat models revealed protective function of scoparone against alcohol induced hepatotoxicity, Sci. Rep., 2014, 4, 6768 CrossRef PubMed.
- M. Jaremek, Z. Yu, M. Mangino, K. Mittelstrass, C. Prehn, P. Singmann, T. Xu, N. Dahmen, K. M. Weinberger, K. Suhre, A. Peters, A. Döring, H. Hauner, J. Adamski, T. Illig, T. D. Spector and R. Wang-Sattler, Alcohol-induced metabolomic differences in humans, Transl. Psychiatry, 2013, 3, e276 CrossRef CAS PubMed.
- R. D. Clugston, H. Jiang, M. X. Lee, R. Piantedosi, J. J. Yuen, R. Ramakrishnan, M. J. Lewis, M. E. Gottesman, L. S. Huang, I. J. Goldberg, P. D. Berk and W. S. Blaner, Altered hepatic lipid metabolism in C57BL/6 mice fed alcohol: a targeted lipidomic and gene expression study, J. Lipid Res., 2011, 52, 2021–2031 CrossRef CAS PubMed.
- S. C. Kalhan, L. Guo, J. Edmison, S. Dasarathy, A. J. McCullough, R. W. Hanson and M. Milburn, Plasma metabolomic profile in nonalcoholic fatty liver disease, Metabolism, 2011, 60, 404–413 CrossRef CAS PubMed.
- B. W. Newton, W. K. Russell, D. H. Russell, S. K. Ramaiah and A. Jayaraman, Liver proteome analysis in a rodent model of alcoholic steatosis, J. Proteome Res., 2009, 8, 1663–1671 CrossRef CAS PubMed.
- H. W. Rhee, P. Zou, N. D. Udeshi, J. D. Martell, V. K. Mootha, S. A. Carr and A. Y. Ting, Proteomic mapping of mitochondria in living cells via spatially restricted enzymatic tagging, Science, 2013, 339, 1328–1331 CrossRef CAS PubMed.
- R. Wagner, J. Li, E. Kenar, O. Kohlbacher, F. Machicao, H. U. Häring, A. Fritsche, G. Xu and R. Lehmann, Clinical and non-targeted metabolomic profiling of homozygous
carriers of Transcription Factor 7-like 2 variant rs7903146, Sci. Rep., 2014, 4, 5296 CAS.
Footnotes |
† Electronic supplementary information (ESI) available. See DOI: 10.1039/c5mb00603a |
‡ These authors contributed equally to this work. |
|
This journal is © The Royal Society of Chemistry 2016 |
Click here to see how this site uses Cookies. View our privacy policy here.