DOI:
10.1039/C5MB00577A
(Paper)
Mol. BioSyst., 2016,
12, 191-204
Identification of potential COPD genes based on multi-omics data at the functional level†
Received
26th August 2015
, Accepted 2nd November 2015
First published on 3rd November 2015
Abstract
Chronic obstructive pulmonary disease (COPD) is a complex disease, which involves dysfunctions in multi-omics. The changes in biological processes, such as adhesion junction, signaling transduction, transcriptional regulation, and cell proliferation, will lead to the occurrence of COPD. A novel systematic approach MMMG (Methylation–MicroRNA–MRNA–GO) was proposed to identify potential COPD genes by integrating function information with a methylation profile, a microRNA expression profile and an mRNA expression profile. 8 co-functional classes and 102 potential COPD genes were identified. These genes displayed a high performance in classifying COPD patients and normal samples, revealed COPD-related pathways, and have been confirmed to be associated with COPD by Matthews correlation coefficient (MCC)-values, literature, an independent data set, and pathways. The MMMG method that analyzed multi-omics data at the functional level could effectively identify potential COPD genes. These potential COPD genes would provide in-depth insights into understanding the complexity of COPD genome landscapes, improve the early diagnostics, and guide new efforts to develop therapeutics in the future.
Introduction
Chronic Obstructive Pulmonary Disease (COPD) is the fourth leading cause of deaths in the world, and estimated to become the third by the year 2020.1 COPD is an independent risk factor for lung cancer development, which can be caused by airway wall remodeling, disorders of signal transductions and occlusion of the airway lumen by mucus within the lung (emphysema).2–5 COPD is caused by aberration of multiple genes, and it is important for researchers to identify potential COPD genes.6,7 High-throughput technologies, such as Genome-Wide Association Studies (GWAS), Next-Generation Sequencing (NGS), and expression profiles (MicroArrays), have provided large quantities of experimental data.8 Systems biology requires integrative analysis methods to analyze these high-throughput data that may shed light on the potential mechanisms of complex diseases.9–11
GWAS have recently distinguished several risk sites for COPD genes.12–14 Although these studies have provided an initial look into the genetic architecture of COPD, they have been limited by the sample size, heterogeneity of disease phenotype, and potential confounders related to the amount of cigarette smoking. The emergence of NGS has allowed the identification of new types of COPD-related non-coding RNAs, and it has paved the way for the study of their functional associations.15 NGS offered unique advantage of being able to detect the simultaneous expression of thousands of functional small RNA transcripts in a single tissue under various physiological conditions.16–18 However, relevant NGS data are inadequate. Microarrays are powerful tools to investigate the expressions of thousands of genes, virtually the whole genome, simultaneously.7 The analysis of the dysfunctional genes in the lung tissues could elaborate the molecular mechanisms responsible for dysfunction in COPD and help to identify molecular targets for the development of therapeutic strategies specifically designed to improve lung function.19
In the lung tissue, DNA-methylation as one of the epigenetic regulators was an important factor for normal lung function, and several studies have recently confirmed that DNA-methylation is significantly associated with COPD susceptibility, severity, and comorbidities such as lung cancer.20–24 Recent studies have identified potentially important CpG loci associated with genetic and epigenetic pathways that may contribute to COPD.25,26 However, they have not yet clarified the role that variations in methylation play in regulating global gene expression and the biological consequences of such regulation. MicroRNAs played important roles in many different biological processes such as embryonic development, cellular proliferation, morphogenesis, and apoptosis through either degradation or translational repression of targeted mRNA.27,28In vitro studies have been widely conducted to identify differential expression microRNAs involved in the pathogenesis of COPD, asthma, and lung cancer.28–32 Although changes in microRNA expression can contribute to diseases, the mechanism is undetermined.33–36 MRNA expression levels are easy to be measured and influence the amount of proteins which are the final, functional form of genetic information.37–39 Therefore, mRNA levels were frequently used as a proxy for protein abundance. Changes in DNA-methylation, microRNA regulations, and mRNA expressions contribute to a better understanding of COPD. Researchers increasingly believed that information gained from gene expression studies in COPD would be more likely to be enhanced by the integration with other genomic data.11,29,40–46
Recent studies have highlighted the complexity of disease genome landscapes in terms of epigenetic alterations, transcriptomic changes and somatic mutation.41,47 While different levels of changes in disease cells link with cellular processes (biological processes and molecular functions) may suggest the use of sophisticated systems biology (mechanistic or probabilistic) models for data integration, their utility can be hampered by the need to learn a large number of parameters from a limited number of patient samples.48 On the other hand, it is unclear if simpler models can adequately capture key features of the data and be used to obtain biologically relevant insights. Correspondingly, relatively few methods have been proposed that can model and integrate multi-omics data. Limitations in unraveling and integration continue to be a major barrier for the exploitation in clinical applications.40,49–56 These methods were based on regression between different omics data and required each sample to have multiple level data.40,55 Currently, COPD multi-omics data for the same human sample are few in publically available databases. Although the dysfunctional genes of COPD from multi-omics data are heterogeneous, their functions are similar.44
For the study of COPD, biological processes and molecular functions enriched by aberrant genes can better explain the mechanism of pulmonary dysfunction.57 The gene HOXA5, which is inactivated by CpG island methylation, positively regulates the expression of the gene TP53 in the “embryogenesis and differentiation of adult cells” process.58,59 TP53 was reported to lead to COPD via the biological process “positive regulation of transcription, DNA-dependent”.56 Stable decreases in miR-124 expression contribute to an epigenetically reprogrammed, highly proliferative and migratory phenotype of hypertensive pulmonary adventitial fibroblasts.60 The KEGG pathways were also identified by enrichment analysis of differentially expressed genes, which could better select specific pathways involved in COPD.61 TGFBR3 was down-regulated by the let-7c expression,62 which was significantly reduced in the sputum of patients with severe COPD.63,64 In the airways, the pathological extracellular matrix of injured epithelial cells and fibroblasts is remodeled via the canonical TGFBR1-SMAD3-dependent signaling pathway which activates downstream Wnt, Notch, and NFkB signaling pathways.46,65 Functional classes and pathways enriched by abnormal genes of multi-omics data can better reflect the pathogenesis of COPD.56,57,61
In this paper, a novel and powerful approach MMMG (Methylation–MicroRNA–MRNA–GO) was proposed to identify potential COPD genes from Gene Ontology (GO) categories enriched by dysfunctional genes from a methylation profile, a microRNA expression profile, and an mRNA expression profile containing COPD and normal samples. Potential COPD genes were evaluated based on the Matthews correlation coefficient (MCC)-value, an independent data set, literature, and pathways.
Methods
The work flow of the MMMG method
The MMMG method was proposed for identifying potential COPD genes (Fig. 1).
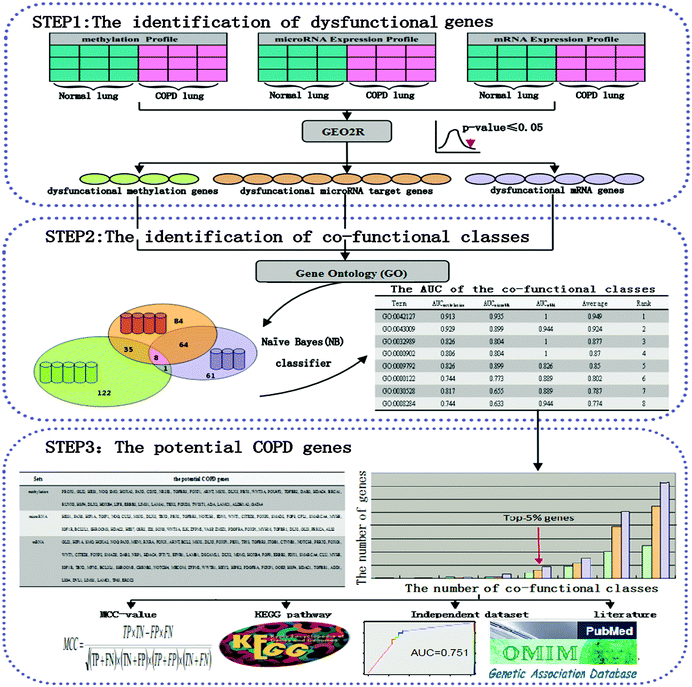 |
| Fig. 1 A schematic diagram of identifying potential COPD genes and classifying performance evaluation. Step 1: the identification of dysfunctional genes. Three groups of dysfunctional genes were selected via limma according to a methylation profile, a microRNA expression profile, and an mRNA expression profile, respectively. Step 2: the identification of co-functional classes. The co-functional classes were defined as the overlap of the GO categories of the above three groups of dysfunctional genes. The Naïve Bayes (NB) classifier was used for distinguishing COPD and normal samples through dysfunctional genes from each co-functional class. Step 3: the selection of potential COPD genes. The potential COPD genes were defined as high-frequency genes in three groups of dysfunctional genes. Eventually, potential COPD genes were validated by the following four ways: the MCC-value, KEGG pathways, an independent data set, and literature. | |
First, dysfunctional genes were selected according to a methylation profile, a microRNA expression profile, and an mRNA expression profile. Co-functional classes were obtained as GO categories enriched by dysfunctional genes. Each co-functional class was evaluated using a Naïve Bayes (NB) classifier to distinguish COPD and normal samples. The potential COPD genes were defined as high-frequency genes in three groups of dysfunctional genes. Eventually, potential COPD genes were validated by the following four ways: the MCC-value, KEGG pathways, an independent data set, and literature.
The identification of dysfunctional genes
COPD expression profiles were obtained from the Gene Expression Omnibus (GEO, http://www.ncbi.nlm.nih.gov/geo/). Here, our research was based on the methylation profile GSE55454, microRNA expression profile GSE38974, and mRNA expression profile GSE38974 (Table 1). These profiles have already been standardized.10,25
Table 1 The number of COPD and normal samples in the expression profiles
|
GSE55454 |
GSE38974 |
GSE38974 |
GSE27536 |
Omics |
Methylation |
microRNA |
mRNA |
mRNA |
GPL |
GPL8490 |
GPL7723 |
GPL4133 |
GPL570 |
COPD |
15 |
19 |
23 |
30 |
Normal |
23 |
8 |
9 |
24 |
Probes |
27 578 |
1981 |
45 220 |
54 674 |
In order to test the predictive models in this research, we used publicly available data of 54
674 probes from GEO with the accession number GSE27536 in 30 COPD patients and 24 normal samples as an independent data set, for which patients underwent a protocol of supervised endurance exercise for COPD at the Ethics Committee of the Hospital Clinic (Barcelona, Spain, Table 1).66
To identify dysfunctional genes between the COPD patients and normal samples of the three expression profiles, the LIMMA (Linear Models for Microarray Data, available at http://www.bioconductor.org/packages/release/bioc/html/limma.html) package,67,68 which provided an integrated solution for analyzing data from gene expression profiles, was used for statistical analysis. Significant differential genes or microRNAs obtained (p-value < 0.05 adjusted with Benjamini–Hochberg) were involved in dysfunctions in COPD, hence were named as methylation dysfunctional genes, mRNA dysfunctional genes, and dysfunctional microRNAs. Three dysfunctional gene groups were obtained: methylation dysfunctional genes, microRNA dysfunctional target genes of dysfunctional microRNAs, and mRNA dysfunctional genes.
Target mRNAs of dysfunctional microRNAs were identified by using the following five common and classic databases: MiRanda (http://www.microrna.org/microrna/home.do, 2011_11), which incorporated 1100 microRNAs; MirBase (http://www.ebi.ac.uk/enright-srv/microcosm/htdocs/targets/v5/, 2007_10, version: 5), which incorporated 851 microRNAs, 34
788 targets; MirTarget2 (http://mirdb.org/miRDB/download.html, 2014_09, version: 5), which incorporated 2588 microRNAs with target, 17
925 unique gene targets; TarBase (http://diana.cslab.ece.ntua.gr/tarbase/tarbase_download.php, 2008_06, version: 5), which incorporated 1331 microRNA–target interactions; and TargetScan (http://www.targetscan.org/, 2012_06), which incorporated 2
393
544 records. In order to reduce the probability of introducing false positives and/or negatives as much as possible, we selected targets that were in at least three databases. Finally, 20
162 microRNA–target gene pairs consisting of 214 microRNAs and 4301 genes were obtained.
The identification of co-functional classes
To obtain Gene Ontology (GO) categories for methylation dysfunctional genes, microRNA dysfunctional target genes and mRNA dysfunctional genes, the Database for Annotation, Visualization and Integrated Discovery v6.7 (DAVID, http://david.abcc.ncifcrf.gov/) was involved in this research.69 The p-value (Benjamini adjusted) < 0.05 was set as the criterion for this analysis.
Co-functional classes were defined as the overlap of GO categories for methylation dysfunctional genes, microRNA dysfunctional target genes and mRNA dysfunctional genes. Expression values of genes in each co-functional class were evaluated using the Naïve Bayes (NB) classifier for distinguishing COPD and normal samples.
NB classification uses probabilistic models to test samples, assuming independence among the genes, which relates with the conditional and marginal probability distributions of random events.70 The classifying result (CR) can be represented as:
where
P(COPD|Co-functional classed) and
P(Normal|Co-functional classes) represent the probability of an event COPD and Normal conditional on event co-functional classes, respectively. The NB program was implemented using weka, a JAVA tool for machine learning.
70–72
Potential COPD genes
The selection of potential COPD genes.
The frequency distributions in methylation dysfunctional genes, microRNA dysfunctional target genes, and mRNA dysfunctional genes from co-functional classes were calculated, respectively. High-frequency genes were obtained from the top-5% of all dysfunctional genes in co-functional classes.
The number of top-5% of all dysfunctional genes was counted, and then potential COPD genes were defined as the union of three groups of dysfunctional genes.
The validation of potential COPD genes.
The discriminating ability of gene groups was evaluated by the Matthews correlation coefficient (MCC), which is a balanced measurement of prediction performance that considers both sensitivity and specificity. It was calculated using the following formula:
where TP, TN, FP, and FN are the numbers of true COPD samples, true normal samples, false COPD samples, and false normal samples, respectively.
On the whole, the MCC value has three states as follows:73 MCC > 0 indicates these genes do positively contribute to distinguishing disease and normal samples, MCC-value = 0 indicates these genes do not contribute to the distinguishing, and MCC-value < 0 indicates these genes do negatively contribute.
Results
Dysfunctional genes
The limma package was used to select dysfunctional genes between the COPD patients and normal samples of the three expression profiles. Three dysfunctional gene groups were obtained (FDR < 0.05): 1854 methylation dysfunctional genes, 2589 microRNA dysfunctional target genes (83 microRNAs in 363 dysfunctional microRNAs), and 5012 mRNA dysfunctional genes.
The co-functional classes of multi-omics data
We adopted functional annotation through DAVID (p-value < 0.05 adjusted using the Benjamini method) to investigate the functional categories of the methylation dysfunctional genes, the microRNA dysfunctional target genes, and mRNA dysfunctional genes, respectively (Table S1, ESI†). The functional classes were more likely to be enriched in the biological-process.
The co-functional classes were defined as the overlap of GO categories of the above three dysfunctional gene groups (Fig. 2). 8 significant dysfunctional categories were identified and confirmed to be associated with COPD in the literature.
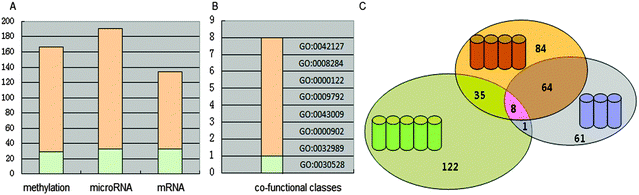 |
| Fig. 2 Dysfunctional classes. (A) The distribution of three dysfunctional categories. (B) The distribution of the co-functional classes. (C) The overlap of three dysfunctional categories. The orange bar(s) represents the number of the Biological Process category, and the green bar(s) the number of molecular function category. The circles represent the number of GO categories. The green indicates the methylation functional category, the orange indicates the microRNA functional category, and the purple indicates the mRNA functional category. The number represents how many terms in these collections. | |
“GO: 0042127 – regulation of cell proliferation” and “GO: 0008284 – positive regulation of cell proliferation” are associated with the regulation of cell proliferation. Y. Pan et al. reported that salvianolic acid A (SAA) alleviated pulmonary fibrosis by inhibiting fibroblast proliferation and inducting of apoptosis through experimental methods.74 K. Y. Lee et al. reported that NFkB activity is necessary for airway smooth muscle cell (ASMC) proliferation, and is potential to involve in airway remodeling of COPD.75 “GO: 0030528 – transcription regulator activity” and “GO: 0000122 – negative regulation of transcription from RNA polymerase II promoter” are associated with the regulation of transcription. The transcriptional repressor NFkB repressing factor (NRF) has been implicated in the basal silencing of specific NFkB targeting genes, including interferon-b, IL8/CXCL8 and inducible nitric oxide synthase via the negative regulatory elements (NREs) in the promoter. It was reported that the NRF-negative regulatory mechanism is impaired in circulating inflammatory cells from COPD patients, leading to enhanced release of IL8/CXCL8. IL8/CXCL8 has long been known to contribute to the pathogenesis of COPD through recruiting the leukocytes into the lung, regulate mucin gene expression, and inhibit lung fibroblast proliferation.75,76 “GO: 0009792 – embryonic development ending in birth or egg hatching” and “GO: 0043009 – chordate embryonic development” are associated with embryonic development. Remodeling is closely associated with embryonic development. Airway remodeling is a critical feature of chronic bronchial diseases, characterized by aberrant repair of the epithelium and accumulation of fibroblasts, which contribute to extracellular matrix (ECM) deposition resulting in fixed bronchial obstruction. Epithelial–mesenchymal transition (EMT) has been identified as a new source of fibroblasts that could contribute to the remodeling of the airways. The core transcriptional regulators of the EMT program coordinate acquisition of the mesenchymal phenotype.45,65 L. Duijts et al. suggested that changes in DNA-methylation and RNA expression patterns in early life can explain the increased risks of COPD throughout the life course.77 “GO: 0000902 – cell morphogenesis” and “GO: 0032989 – cellular component morphogenesis” are associated with cell morphogenesis. One of the features of chronic inflammatory airway diseases, including COPD, is associated with airway remodeling. The change in cell morphogenesis, namely, airway epithelial cells transfer to a mesenchymal phenotype with myofibroblast characteristics, led to airway remodeling.78 8 co-functional classes were all involved in the airway remodeling, which implied that genes in multiple function classes were vital for COPD.
Each co-functional class was used in the NB classifier to distinguish COPD and normal samples. The classification performance was evaluated by the Area Under the ROC Curve (AUC) (Table 2). It was showed that all co-functional classes could classify samples with high performance.
Table 2 The AUC of co-functional classes
Term |
AUCmethylation |
AUCmicroRNA |
AUCmRNA |
Average |
Rank |
Term is one of the co-functional classes. Definition of abbreviation: AUCmethylation = area under the ROC curve obtained from designing a classifier when only using methylation dysfunctional genes as features. AUCmicroRNA = area under the ROC curve obtained from designing a classifier when only using microRNA dysfunctional target genes as features. AUCmRNA = area under the ROC curve obtained from designing a classifier when only using mRNA dysfunctional genes as features. Rank represents the importance of each term by computing the average of AUCmethylation, AUCmicroRNA, and AUCmRNA. |
GO: 0042127 |
0.913 |
0.935 |
1.000 |
0.949 |
1 |
GO: 0043009 |
0.929 |
0.899 |
0.944 |
0.924 |
2 |
GO: 0032989 |
0.826 |
0.804 |
1.000 |
0.877 |
3 |
GO: 0000902 |
0.806 |
0.804 |
1.000 |
0.870 |
4 |
GO: 0009792 |
0.826 |
0.899 |
0.826 |
0.85 |
5 |
GO: 0000122 |
0.744 |
0.773 |
0.889 |
0.802 |
6 |
GO: 0030528 |
0.817 |
0.655 |
0.889 |
0.787 |
7 |
GO: 0008284 |
0.744 |
0.633 |
0.944 |
0.774 |
8 |
Potential COPD genes
8 functional categories were all associated with the airway remodeling of COPD, herein, high-frequency dysfunctional genes in these 8 functional classes could play crucial roles in the occurrence of COPD. The number of methylation, microRNA and mRNA dysfunctional genes in different numbers of co-functional classes was counted, respectively (Fig. 3).
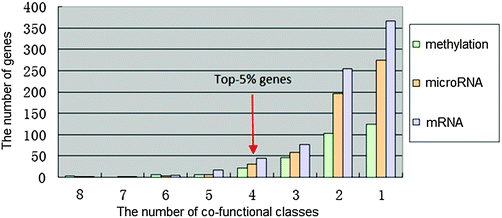 |
| Fig. 3 The frequency distribution of each dysfunctional group. The bar(s) indicates the frequency of genes in each group. The green represents the number of methylation dysfunctional genes in different numbers of co-functional classes, orange the number of microRNA dysfunctional target genes in different numbers of co-functional classes, while purple the number of mRNA dysfunctional genes in different numbers of co-functional classes. | |
The potential COPD genes were defined as the top-5% genes in three dysfunctional gene groups, i.e. the dysfunctional genes is no less than 4 co-functional classes (Fig. 4). It was showed that potential COPD genes were 36 methylation dysfunctional genes, 42 microRNA dysfunctional target genes, and 67 mRNA dysfunctional genes. Eventually, the number of potential COPD genes was 102 (Table S2, ESI†). The number of genes was 22, 23, and 36 in each two of three groups. Five genes TGFBR3, DLX5, MSX5, PBX1, and NOG co-appeared in the three groups.
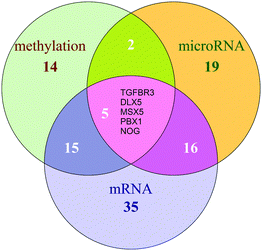 |
| Fig. 4 The Venn diagram of potential COPD genes. The green circle represents potential COPD genes from methylation dysfunctional genes, the orange for potential COPD genes from microRNA dysfunctional target genes, and the purple for those from mRNA dysfunctional genes. The overlap of three circles is TGFBR3, DLX5, MSX5, PBX1, and NOG. The number in the circle indicates how many genes in these groups. | |
The validation by the literature
We integrated the information of the known COPD genes from Online Mendelian Inheritance in Man (OMIM) and Genetic Association Database (GAD) databases. 13 known COPD genes were in our 102 genes, and 35 could be confirmed by the literature (45.098%).
TGFBR3 and DLX5 co-appeared in all of the three groups. Association analysis with COPD pedigrees revealed significant association for COPD-related traits with an intronic single nucleotide polymorphism (SNP) in transforming growth factor-b receptor-3 (TGFBR3), which is a known COPD gene susceptibility from the International COPD Genetics Network.64 DLX5, a member of the Distal-less homeobox domain protein family, is a well-known transcription factor for osteogenic differentiation. Abdul S. Qadir et al. indicated that over-expression of a miR-124 mimic decreased DLX5 expression.79 Stable decreases in miR-124 expression contribute to an epigenetically reprogrammed, highly proliferative, migratory, and inflammatory phenotype of hypertensive pulmonary adventitial fibroblasts.60
52.632% potential COPD genes appearing in two of three groups were confirmed, e.g. WNT3A, TGFB1, HEY2 and NOTCH1. W. Zou et al. showed that nicotine activated the WNT3A, which led to the translocation of β-catenin into the nucleus and activation of β-catenin transcription in the human bronchial epithelial cell (HBEC) line. Moreover, WNT3A positively regulated TGFB1 expression, which might be further enhanced in HBECs. It was the basis for future investigations into the mechanism of bronchial epithelial cell-to-mesenchymal cell differentiation, which in turn would contribute both to a better understanding of COPD and to the development of new therapeutic approaches.65 In the S. T. Gohy et al. research, COPD cultures released more TGFB1, reflecting increased epithelial TGFB1 immunostaining in the COPD lung tissue. This study also found TGFB-driven reprogramming of the bronchial epithelium, which results in impaired lung IgA immunity in patients with COPD.80 O. Boucherat et al. found that expression levels of activated NOTCH1 and the effector gene HEY2 are enhanced in patients with COPD.81
The validation by the MCC-value
The evaluation of co-functional classes.
The discriminating ability of co-functional classes was measured between COPD patients and normal samples using the MCC.
The distributions of MCC-values for genes in 8 co-functional classes in the methylation, microRNA and mRNA data set were obtained (Fig. 5A). The MCC-values of the mRNA dysfunctional genes in co-functional classes were significantly greater than those of the methylation dysfunctional genes in co-functional classes, which were significantly greater than the MCC-values of the microRNA dysfunctional target genes in co-functional classes. MCC-values > 0 indicated that genes of these co-functional classes contributed to COPD. The greater the MCC-values, the greater the contributions.
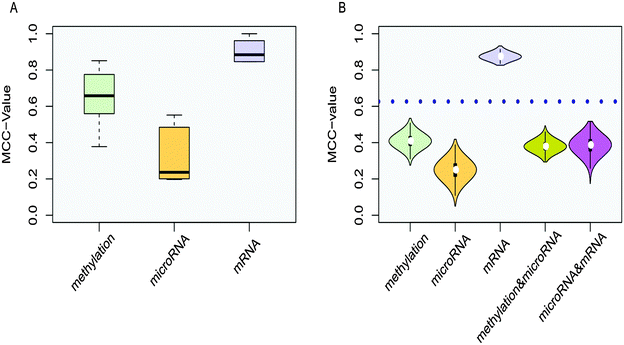 |
| Fig. 5 Co-functional classes. (A) Box plots of MCC-values. The mean MCC-values of the methylation, microRNA and mRNA dysfunctional genes in co-functional classes are 0.652, 0.324 and 0.904, respectively. The one-directional (one-sided)-greater t-test p-value for the mRNA and methylation dysfunctional genes is less than 1.4 × 10−6. The one-sided-greater t-test p-value for the methylation and microRNA dysfunctional target genes is also less than 1.2 × 10−3. (B) Violin plots of MCC-values. The horizontal axis represents five groups of dysfunctional GO categories, while the vertical axis for the MCC-value to according different colors. The dotted blue line represents the average MCC-value of 8 co-functional classes. The majority of p-values were less than 0.05 when a series of Wilcox-tests were used. | |
In addition, the MCC-value was also used to measure the classification performance for 8 GO categories from functional classes enriched by single, double, and three groups (Fig. 5B). For functional classes enriched by a single group, we randomly selected 8 GO categories from 122, 84, and 61 categories from only methylation dysfunctional categories, microRNA dysfunctional classes, and mRNA dysfunctional classes for designing classifiers each time, respectively. These genes in 8 functional classes were used as features, and each process was repeated ten thousand times. For functional classes enriched by double groups, we randomly selected 8 GO categories from 35 and 64 categories from the overlap of methylation and microRNA dysfunctional classes, and the overlap of microRNA and mRNA dysfunctional classes (only one dysfunctional category was in the overlap of methylation and mRNA dysfunctional categories and its MCC = 0.806) for designing classifiers each time, respectively. These genes in 8 functional classes were used as features for corresponding expression profiles, and the average of MCC-values was calculated for each functional class enriched by double groups, then this process was repeated ten thousand times. The Wilcox-test was used to verify that the MCC-values of the co-functional classes were significantly higher than the random ones. Violin plots showed that the distribution of MCC-values of co-functional classes was significantly higher than single and double functional random in most cases.
The validation of potential COPD genes.
Notably, the MCC-value was also used to measure the classification performance for potential COPD genes from three groups: methylation dysfunctional genes, microRNA dysfunctional target genes, and mRNA dysfunctional genes. We randomly selected genes from each group, the overlap of two and three groups, respectively. These genes were used as features for designing classifiers each time, and each process was repeated ten thousand times. Eventually, the MCC distribution was calculated (additional file 5: Fig. S1, ESI†). It was shown that the MCC-values based on the potential COPD genes were significantly higher than the random ones in most cases (one-directional-greater t-tests, p < 0.05). It was found that the MCC-values from integrating three data sources were superior to those from two kinds of data sources, and the MCC-values from integrating two data sources were higher than a single data source.
The validation by the KEGG pathway
COPD is a complex disease that involves dysregulations of multiple pathways of multi-omics. Functional annotation was adopted through DAVID (p-value < 0.05 adjusted with Benjamini) to investigate the correlation between the potential COPD genes and KEGG pathways. Eventually, these genes were enriched in 15 significant KEGG pathways (Table S3, ESI†), 3 of which were signaling pathways, 2 were adhesion-related pathways, and 9 were cancer-related (Fig. 6).
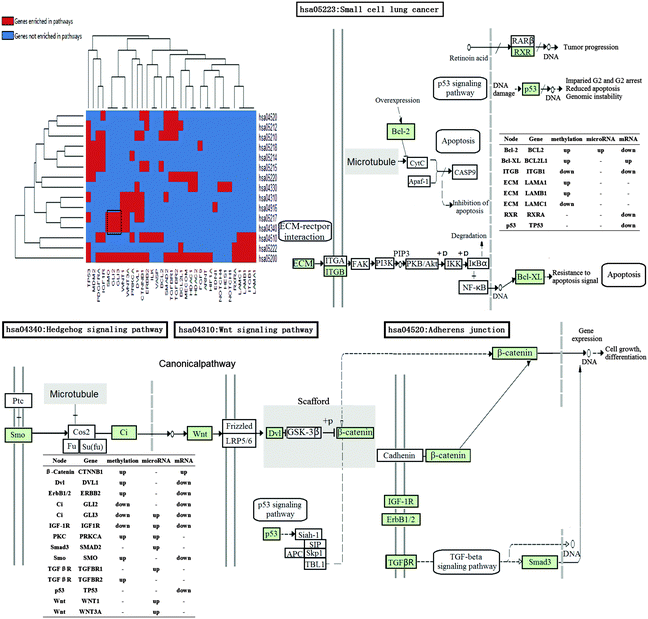 |
| Fig. 6 The enriched KEGG pathways for the potential COPD genes. The black rectangle circles out certain genes enriched in similar KEGG pathways. In the heat map, each row represents a pathway, and each column represents a gene. Red represents the genes that are enriched in pathways, while blue represents the genes that not enriched in pathways. In the whole pathways, green stands for the potential COPD genes. Tables illustrate genes' corresponding nodes in KEGG figures and their up- or down-regulation in different profiles. Some of the different pathways can be connected to each other by the potential COPD genes. | |
From the heat map of genes and KEGG pathways, we found that GLI2, GLI3, and SMO enriched in “hsa04340: Hedgehog signaling pathway” and “hsa05217: Basal cell carcinoma”. Signals of Hedgehog family proteins (SHH and IHH) were transduced through Patched family receptors and Smoothened (SMO) to GLI family transcription factors (GLI2 and GLI3), which played key roles in the development and progression of basal cell carcinoma.82 An immunohistochemical study was performed for heat shock proteins in the bronchial epithelium and in specimens with adenosquamous carcinoma (ASC), suggesting that COPD patients with smoking were more susceptible to basal cell hyperplasia (BCH).83
The close association between COPD and lung cancer has long been known. Marianna Siganaki et al. found that activating two main apoptotic pathways (the extrinsic (receptor-mediated) and the intrinsic (mitochondria-mediated) pathway) were involved in the destruction of the pulmonary tissue in COPD, which could lead to lung cancer.84 The potential COPD genes were also enriched in adhesion-related pathways, such as “hsa04510: Focal adhesion”, and “hsa04520: Adherens junction”. Epithelial–mesenchymal transition (EMT) has been identified as a new source of fibroblasts that could contribute to the remodeling of the airways. During EMT, epithelial cells lose apico-basal polarity and decrease the expression of adherens junctions (AJs). These changes lead to the disruption of adhesion of the basal epithelial layer and allow cellular penetration into an ECM, promoting enhanced ECM production and fibrosis, which was related to COPD.46 Notch, Hedgehog, and Wnt signaling pathways are responsible for progenitor cell development and pulmonary organogenesis:85 (1) based on the knowledge that Notch signaling acts to maintain “stemness” and prevent differentiation of epithelial cells, Ann E. Tilley et al. indicated that, in the setting of the ongoing epithelial injury of cigarette smoking, Notch signaling is down-regulated to permit differentiation and repair of the airway epithelium.9 (2) Wnt signaling pathway expression and activation by TGFB1 played an important role in regulating fibroblast phenotype and function in COPD.86 (3) B. Wang et al. suggested the Hedgehog signaling pathway plays an important role in lung morphogenesis and cellular responses to lung injury in their study. GWAS and integrative genomics approaches have demonstrated the associations between HHIP polymorphisms and COPD.87 Ivy Shi et al. indicated the strong association of Hedgehog signaling with non-small cell lung cancer. GLI1/GLI2 downstream genes commonly differentially expressed in the squamous cell lung cancer microarray.88
These pathways can be genetically connected directly. For instance, the Hedgehog signaling pathway and the Wnt signaling pathway are associated with each other through WNT. CI (GLI2 and GLI3) in the Hedgehog signaling pathway could initiate the canonical Wnt signaling pathway by activating downstream WNT.89 SMO played pivotal roles between the Hedgehog signaling pathway and the Wnt signaling pathway, which has been implicated in various human carcinomas.90 While the Wnt signaling pathway and the Adherens Junction pathway are cross-talk with each other via β-catenin (CTNNB1). Q. X. Liu et al. concluded that the β-catenin expression was significantly decreased in smokers with COPD, and the β-catenin level in the lungs was positively correlated with pulmonary function.91,92 In K. Kumawat et al.'s opinion, the aberrant activation of β-catenin signaling by both WNT-dependent and -independent mechanisms in asthmatic airways played a key role in remodeling the airways, including cell proliferation, differentiation, tissue repair and extracellular matrix production.93 β-catenin affects cell growth, differentiation at the level of gene expression, along with IGF1R, ERBB, TGFBR, SMAD3.45,46,64,94–96 The protein β-catenin exhibits a dual function in cells, by acting as a major structural component of cell–cell adherens junctions and as a central signaling molecule in the Wnt signaling pathway.97
The validation by an independent data set
We tested the discriminating ability of potential COPD genes in the mRNA data set and an independent data set which includes COPD and normal samples. It was shown that the potential COPD genes could differentiate the COPD from normal samples. The majority of samples were correctly classified in their actual group (AUCmRNA = 0.889, AUCindependent
data
set = 0.751) (Fig. 7). The MCC-value was used to measure the classification performance of potential COPD genes (MCCmRNA = 0.846, MCCindependent
data
set = 0.440).
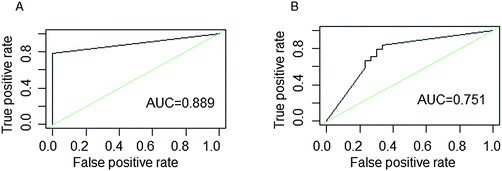 |
| Fig. 7 ROC curves of patients and normal samples with COPD. ROC curves for (A) the mRNA expression profile and (B) the independent data set. The areas under curve (AUC) are provided at the lower right of each diagram. | |
Discussion
COPD is a worldwide epidemic heterogeneous disease.98 Many changes can lead to COPD, such as methylation imbalance, microRNA regulation, and gene mutation.27,37,99–101 And all these changes are reflected in the level of function. Therefore, a systematic method, MMMG, was proposed to mine the potential COPD genes and describe the pathological mechanisms of COPD by integrating three omics data. 8 co-functional classes were obtained according to dysfunctional genes from the methylation profile, microRNA expression profile, and mRNA expression profile. The 102 potential COPD genes that appeared frequently in co-functional classes were able to effectively classify normal and disease samples, and could be confirmed by the MCC-value, pathways, an independent data set, and literature.
Multi-omics microarray data are powerful resources to investigate the expressions of thousands of genes, virtually the whole genome, simultaneously for identifying potential COPD genes.42 DNA-methylation and RNA expression patterns can explain the early development of COPD through the interactions between early environmental exposures and genetic factors.37,102 MicroRNAs, such as miR-342-3p, miR-107 and miR-124, regulate gene expressions at either the transcriptional or translational level in COPD.10,95,103–106 Gene expressions in single- or multi-omics data have heterogeneity.107,108 Efficient and replicable results could be obtained by integrative methods.109 Integrating multiple types of omics data, such as epigenetic, transcriptional, and gene expressed data, was powerful for biologically relevant studies, would enhance information, and had a great potential to uncover novel genes and pathways in COPD.44,56,70,110 In this study, 102 potential COPD genes were identified by integrating three omics data, 45.098% of which were confirmed in the literature. TGFBR3, co-appeared in all of the three groups, was confirmed as a known COPD gene in GAD and OMIM databases. Previous studies have elucidated that the hypermethylation of TGFBR3 and the reduction of TGFBR3 expression by microRNAs were associated with severe COPD.64,95,105,111 The expression changes in WNT3A, ERBB2, HEY2 and NOTCH1 in two of the three groups were reported to lead to COPD.65,80,81,94
The differentially expressed genes of COPD from multi-omics data are heterogeneous, while their enriched functions are similar.44 Co-functional classes and high-frequency dysfunctional genes in them could effectively reflect the pathogenesis of COPD.56,57,61 8 co-functional classes were all involved in the airway remodeling, which were vital for COPD.45,65,75,76,78,112 These functional classes were also enriched by known COPD genes. Seven identified potential COPD genes annotated the “GO: 0032989 – cellular component morphogenesis”, in which, two genes (HOXA2 and TGFBR3) are the known COPD genes in GAD and OMIM databases, and the rest five potential COPD genes (SMO, WNT3A, GLI2, ERBB2, and NOTCH1) are also associated with COPD by the validation of literature studies.65,81,89,90,94 High-frequency dysfunctional genes, GLI2 and HES1, were enriched in all 8 co-functional classes. GLI2 is a primary regulator in the Sonic Hedgehog (SHH) signaling pathway, which plays an important role in lung morphogenesis and cellular responses to lung injury. GWAS and integrative genomics approaches have demonstrated the associations between HHIP polymorphisms and COPD.87,89 Immunohistochemistry demonstrated several Notch ligands (DLL1), receptors (NOTCH1, 2 and 4), and downstream effector (HES1 and HEY2) genes were down-regulated in smokers with COPD than in healthy smokers.9 Functional pathways based on multi-omics data were the crucial ways to the occurrence of diseases.41,90 15 significant KEGG pathways were enriched by 102 potential COPD genes. 9 cancer-related pathways including the Small cell lung cancer (SCLC) pathway were identified. 4 (BCL2, TP53, BCL2L1, and ITGB1) of the 8 potential COPD genes in the SCLC pathway were proven associated with COPD, particularly TP53, which was a known COPD gene.72,84,113–116 The occurrence of COPD was also associated with the disorders of 3 signal transductions: the Notch signaling pathway, Hedgehog signaling, and Wnt signaling.9,87,117,118 15 of 18 potential COPD genes in these signaling pathways were proven associated with COPD.9,65,81,119–127 Cross-talks between these pathways would be effective for investigating the pathogenesis of COPD. For example, the Hedgehog signaling pathway and the Wnt signaling pathway were cross-talk with each other through WNT. The interaction between the Wnt signaling pathway and the Adhesion junction pathway via β-catenin had an important role in the pathogenesis of COPD.
Co-functional classes and potential COPD genes identified by our method had high performances for classifying normal and disease samples. MCC was used to evaluate the performances. MCC-values from integrating three data sources were superior to the MCC-values from two groups of data sources, at the same time, the MCC-values from integrating two data sources were better than a single data source (Fig. 5B). Higher MCC-values were achieved from the mRNA data than from other two omics data. Using the potential COPD genes from mRNA data as features for programming classifier, MCC-values were 0.251 and −0.127 in methylation data and microRNA data, respectively, which demonstrated that mRNA data could not classify samples efficiently. However, MCC-values were 0.388 and 0.122 in methylation data and microRNA data, respectively, when potential COPD genes from multi-omics data were used. It was shown that the potential COPD genes from three data sources could better classify samples, namely, the integration will improve the classification performance.
Our identified COPD potential genes could act as therapeutic biomarkers for the diagnosis and prognosis of COPD, while they could not be recognized in other recent studies that identified COPD-related genes mainly using the gene-association study or genome-wide association studies.128,129 ADA could be one diagnosis biomarker since mice lacking ADA developed COPD.130 One of our potential COPD genes, HDAC2, is the target of theophylline, which has been used mainly for therapy for asthma, bronchospasm, and COPD.131 β-catenin, encoded by the COPD potential gene CTNNB1, acted as a regulator and a therapeutic target for airway remodeling, which might help COPD therapy.132 With regard to the growing number of investigations of Smads family, including the gene SMAD2, Smads might be an important target for future development of new therapeutic strategies for asthma and COPD.133 Pathways these COPD potential genes enriched in could provide therapeutic targets for COPD. Activation of the Wnt signaling pathway, 7 COPD potential genes participated in, could help to develop long term treatments that induce lung tissue repair in COPD patients.134
There are several limitations to this study. First, the methylation, microRNA and mRNA data for COPD and normal were derived from different studies, which may affect the results. Second, dysfunctional genes of the microRNA profile were target genes of differentially expressed microRNAs, which were predicted by at least three of the five popular microRNA–target databases. This may affect the accuracy of our research. With different data and approaches used, only about 1/4 COPD-related genes could be recognized by at least two different studies.129 The robustness and classification performance would be improved if multi-omics data from the same platform and accurate microRNA–target prediction were available.
Conclusions
To sum up, we developed a novel systematic approach, the MMMG method, that analyzed multi-omics data at the function level, could effectively identify potential COPD genes, correctly classify samples, and reveal COPD-related pathways. 48 potential COPD genes (13 known COPD genes) were confirmed by literature studies in 102 genes. Our research would yield a systematic view of COPD, investigate the pathogenesis of COPD, and shed light on the diagnosis and prognosis of COPD.
Contributions
LC conceived, designed and supervised the overall study. WL, ZL and LC developed the idea for this manuscript and wrote the first draft. WL, JL, BC and JJ took responsibility for downloading the data in the study. ZL, RX, HH and YL led the statistical analysis. WL, RX, YH and SG participated in writing of the manuscript. All authors read and approved the final manuscript.
Conflicts of interest
The authors declare that they have no competing interests.
Acknowledgements
This work was supported in part by the National Natural Science Foundation of China (Grant No. 61272388); the Natural Science Foundation of Heilongjiang Province (Grant No. F201237); the Science & Technology Research Project of the Heilongjiang Ministry of Education (Grant No. 12541476); the Health Department Funds of Heilongjiang Province (Grant No. 2012-810); and the Master Innovation Funds of Heilongjiang Province (Grant No. YJSCX2014-18HYD).
References
- D. Moreno, J. Barroso and A. Garcia, Recent Pat. Inflammation Allergy Drug Discovery, 2015, 9, 23–30 CrossRef CAS
.
- R. M. Pascual and S. P. Peters, J. Allergy Clin. Immunol., 2005, 116, 477–486 CrossRef PubMed
; quiz 487.
- I. Cano, A. Tenyi, C. Schueller, M. Wolff, M. M. Huertas Miguelanez, D. Gomez-Cabrero, P. Antczak, J. Roca, M. Cascante, F. Falciani and D. Maier, J. Transl. Med., 2014, 12(suppl 2), S6 CrossRef PubMed
.
- G. Lin, L. Sun, R. Wang, Y. Guo and C. Xie, J. Thorac. Oncol., 2014, 9, 170–178 CrossRef CAS PubMed
.
- H. D. Seth, S. Sultan and M. H. Gotfried, J. Thorac. Dis., 2013, 5, 806–814 Search PubMed
.
- B. Li, X. Zhou, L. Chen, C. Feng and T. Li, Zhonghua Weizhongbing Jijiu Yixue, 2014, 26, 905–909 Search PubMed
.
- S. Yoo, S. Takikawa, P. Geraghty, C. Argmann, J. Campbell, L. Lin, T. Huang, Z. Tu, R. Feronjy, A. Spira, E. E. Schadt, C. A. Powell and J. Zhu, PLoS Genet., 2015, 11, e1004898 Search PubMed
.
- D. Bertrand, K. R. Chng, F. G. Sherbaf, A. Kiesel, B. K. Chia, Y. Y. Sia, S. K. Huang, D. S. Hoon, E. T. Liu, A. Hillmer and N. Nagarajan, Nucleic Acids Res., 2015, 43, e44 CrossRef PubMed
.
- A. E. Tilley, B. G. Harvey, A. Heguy, N. R. Hackett, R. Wang, T. P. O'Connor and R. G. Crystal, Am. J. Respir. Crit. Care Med., 2009, 179, 457–466 CrossRef CAS PubMed
.
- M. E. Ezzie, M. Crawford, J. H. Cho, R. Orellana, S. Zhang, R. Gelinas, K. Batte, L. Yu, G. Nuovo, D. Galas, P. Diaz, K. Wang and S. P. Nana-Sinkam, Thorax, 2012, 67, 122–131 CrossRef PubMed
.
- Z. Ammous, N. R. Hackett, M. W. Butler, T. Raman, I. Dolgalev, T. P. O'Connor, B. G. Harvey and R. G. Crystal, Chest, 2008, 133, 1344–1353 CrossRef CAS PubMed
.
- S. G. Pillai, D. Ge, G. Zhu, X. Kong, K. V. Shianna, A. C. Need, S. Feng, C. P. Hersh, P. Bakke, A. Gulsvik, A. Ruppert, K. C. Lodrup Carlsen, A. Roses, W. Anderson, S. I. Rennard, D. A. Lomas, E. K. Silverman, D. B. Goldstein and I. Investigators, PLoS Genet., 2009, 5, e1000421 Search PubMed
.
- L. Kent, L. Smyth, C. Clayton, L. Scott, T. Cook, R. Stephens, S. Fox, P. Hext, S. Farrow and D. Singh, Cytokine, 2008, 42, 205–216 CrossRef CAS PubMed
.
- B. G. Harvey, A. Heguy, P. L. Leopold, B. J. Carolan, B. Ferris and R. G. Crystal, J. Mol. Med., 2007, 85, 39–53 CrossRef CAS PubMed
.
- J. Mazieres, C. Catherinne, O. Delfour, S. Gouin, I. Rouquette, M. B. Delisle, G. Prevot, R. Escamilla, A. Didier, D. H. Persing, M. Bates and B. Michot, PLoS One, 2013, 8, e60134 CAS
.
- M. R. Jones, L. J. Quinton, M. T. Blahna, J. R. Neilson, S. Fu, A. R. Ivanov, D. A. Wolf and J. P. Mizgerd, Nat. Cell Biol., 2009, 11, 1157–1163 CrossRef CAS PubMed
.
- D. D. Jima, J. Zhang, C. Jacobs, K. L. Richards, C. H. Dunphy, W. W. Choi, W. Y. Au, G. Srivastava, M. B. Czader, D. A. Rizzieri, A. S. Lagoo, P. L. Lugar, K. P. Mann, C. R. Flowers, L. Bernal-Mizrachi, K. N. Naresh, A. M. Evens, L. I. Gordon, M. Luftig, D. R. Friedman, J. B. Weinberg, M. A. Thompson, J. I. Gill, Q. Liu, T. How, V. Grubor, Y. Gao, A. Patel, H. Wu, J. Zhu, G. C. Blobe, P. E. Lipsky, A. Chadburn, S. S. Dave and C. Hematologic Malignancies Research, Blood, 2010, 116, e118–127 CrossRef CAS PubMed
.
- P. Landgraf, M. Rusu, R. Sheridan, A. Sewer, N. Iovino, A. Aravin, S. Pfeffer, A. Rice, A. O. Kamphorst, M. Landthaler, C. Lin, N. D. Socci, L. Hermida, V. Fulci, S. Chiaretti, R. Foa, J. Schliwka, U. Fuchs, A. Novosel, R. U. Muller, B. Schermer, U. Bissels, J. Inman, Q. Phan, M. Chien, D. B. Weir, R. Choksi, G. De Vita, D. Frezzetti, H. I. Trompeter, V. Hornung, G. Teng, G. Hartmann, M. Palkovits, R. Di Lauro, P. Wernet, G. Macino, C. E. Rogler, J. W. Nagle, J. Ju, F. N. Papavasiliou, T. Benzing, P. Lichter, W. Tam, M. J. Brownstein, A. Bosio, A. Borkhardt, J. J. Russo, C. Sander, M. Zavolan and T. Tuschl, Cell, 2007, 129, 1401–1414 CrossRef CAS PubMed
.
- R. A. Rabinovich, E. Drost, J. R. Manning, D. R. Dunbar, M. Diaz-Ramos, R. Lahkdar, R. Bastos and W. MacNee, Respir. Res., 2015, 16, 1 CrossRef PubMed
.
- S. Kalari, M. Jung, K. H. Kernstine, T. Takahashi and G. P. Pfeifer, Oncogene, 2013, 32, 3559–3568 CrossRef CAS PubMed
.
- I. M. Adcock, P. Ford, K. Ito and P. J. Barnes, Respir. Res., 2006, 7, 21 CrossRef PubMed
.
- G. Egger, G. Liang, A. Aparicio and P. A. Jones, Nature, 2004, 429, 457–463 CrossRef CAS PubMed
.
- J. Lepeule, A. Baccarelli, V. Motta, L. Cantone, A. A. Litonjua, D. Sparrow, P. S. Vokonas and J. Schwartz, Epigenetics, 2012, 7, 261–269 CrossRef CAS PubMed
.
- S. A. Selamat, B. S. Chung, L. Girard, W. Zhang, Y. Zhang, M. Campan, K. D. Siegmund, M. N. Koss, J. A. Hagen, W. L. Lam, S. Lam, A. F. Gazdar and I. A. Laird-Offringa, Genome Res., 2012, 22, 1197–1211 CrossRef CAS PubMed
.
- E. A. Vucic, R. Chari, K. L. Thu, I. M. Wilson, A. M. Cotton, J. Y. Kennett, M. Zhang, K. M. Lonergan, K. Steiling, C. J. Brown, A. McWilliams, K. Ohtani, M. E. Lenburg, D. D. Sin, A. Spira, C. E. Macaulay, S. Lam and W. L. Lam, Am. J. Respir. Cell Mol. Biol., 2014, 50, 912–922 CrossRef PubMed
.
- W. Qiu, A. Baccarelli, V. J. Carey, N. Boutaoui, H. Bacherman, B. Klanderman, S. Rennard, A. Agusti, W. Anderson, D. A. Lomas and D. L. DeMeo, Am. J. Respir. Crit. Care Med., 2012, 185, 373–381 CrossRef CAS PubMed
.
- V. P. Bondanese, A. Francisco-Garcia, N. Bedke, D. E. Davies and T. Sanchez-Elsner, World J. Biol. Chem., 2014, 5, 437–456 CrossRef PubMed
.
- M. Angulo, E. Lecuona and J. I. Sznajder, Archivos de Bronconeumologia, 2012, 48, 325–330 Search PubMed
.
- S. P. Nana-Sinkam, M. G. Hunter, G. J. Nuovo, T. D. Schmittgen, R. Gelinas, D. Galas and C. B. Marsh, Am. J. Respir. Crit. Care Med., 2009, 179, 4–10 CrossRef CAS PubMed
.
- H. Rupani, T. Sanchez-Elsner and P. Howarth, Eur. Respir. J., 2013, 41, 695–705 CrossRef CAS PubMed
.
- I. K. Oglesby, N. G. McElvaney and C. M. Greene, Respir. Res., 2010, 11, 148 CrossRef PubMed
.
- M. Kupczyk and P. Kuna, Pneumonol. Alergol. Pol., 2014, 82, 183–190 CrossRef CAS PubMed
.
- S. G. Chaulk, V. J. Lattanzi, S. E. Hiemer, R. P. Fahlman and X. Varelas, J. Biol. Chem., 2014, 289, 1886–1891 CrossRef CAS PubMed
.
- H. W. Hwang, E. A. Wentzel and J. T. Mendell, Proc. Natl. Acad. Sci. U. S. A., 2009, 106, 7016–7021 CrossRef CAS PubMed
.
- W. Wagner, P. Horn, M. Castoldi, A. Diehlmann, S. Bork, R. Saffrich, V. Benes, J. Blake, S. Pfister, V. Eckstein and A. D. Ho, PLoS One, 2008, 3, e2213 Search PubMed
.
- D. N. Shelton, E. Chang, P. S. Whittier, D. Choi and W. D. Funk, Curr. Biol., 1999, 9, 939–945 CrossRef CAS PubMed
.
- C. Donovan, H. J. Seow, S. G. Royce, J. E. Bourke and R. Vlahos, Am. J. Respir. Cell Mol. Biol., 2015, 53, 471–478 CrossRef PubMed
.
- R. Almansa, L. Socias, M. Sanchez-Garcia, I. Martín-Loeches, M. del Olmo, D. Andaluz-Ojeda, F. Bobillo, L. Rico, A. Herrero, V. Roig, C. A. San-Jose, S. Rosich, J. Barbado, C. Disdier, R. O. de Lejarazu, M. C. Gallegos, V. Fernandez and J. F. Bermejo-Martin, BMC Res. Notes, 2012, 5, 401 CrossRef CAS PubMed
.
- J. Xue, S. V. Schmidt, J. Sander, A. Draffehn, W. Krebs, I. Quester, D. De Nardo, T. D. Gohel, M. Emde, L. Schmidleithner, H. Ganesan, A. Nino-Castro, M. R. Mallmann, L. Labzin, H. Theis, M. Kraut, M. Beyer, E. Latz, T. C. Freeman, T. Ulas and J. L. Schultze, Immunity, 2014, 40, 274–288 CrossRef CAS PubMed
.
- U. D. Akavia, O. Litvin, J. Kim, F. Sanchez-Garcia, D. Kotliar, H. C. Causton, P. Pochanard, E. Mozes, L. A. Garraway and D. Pe'er, Cell, 2010, 143, 1005–1017 CrossRef CAS PubMed
.
- N. Cancer Genome Atlas Research, C. Kandoth, N. Schultz, A. D. Cherniack, R. Akbani, Y. Liu, H. Shen, A. G. Robertson, I. Pashtan, R. Shen, C. C. Benz, C. Yau, P. W. Laird, L. Ding, W. Zhang, G. B. Mills, R. Kucherlapati, E. R. Mardis and D. A. Levine, Nature, 2013, 497, 67–73 CrossRef PubMed
.
- J. Y. Yang, J. Jin, Z. Zhang, L. Zhang and C. Shen, Eur. Rev. Med. Pharmacol. Sci., 2013, 17, 1923–1931 Search PubMed
.
- X. M. Wang, J. Li, M. X. Yan, L. Liu, D. S. Jia, Q. Geng, H. C. Lin, X. H. He, J. J. Li and M. Yao, PLoS One, 2013, 8, e55714 CAS
.
- B. Balliu, R. Tsonaka, S. Boehringer and J. Houwing-Duistermaat, Genet. Epidemiol., 2015, 39, 156–165 CrossRef PubMed
.
- W. Huang da, B. T. Sherman and R. A. Lempicki, Nat. Protoc., 2009, 4, 44–57 CrossRef PubMed
.
- M. Kalita, B. Tian, B. Gao, S. Choudhary, T. G. Wood, J. R. Carmical, I. Boldogh, S. Mitra, J. D. Minna and A. R. Brasier, BioMed Res. Int., 2013, 2013, 505864 Search PubMed
.
- B. S. Taylor, N. Schultz, H. Hieronymus, A. Gopalan, Y. Xiao, B. S. Carver, V. K. Arora, P. Kaushik, E. Cerami, B. Reva, Y. Antipin, N. Mitsiades, T. Landers, I. Dolgalev, J. E. Major, M. Wilson, N. D. Socci, A. E. Lash, A. Heguy, J. A. Eastham, H. I. Scher, V. E. Reuter, P. T. Scardino, C. Sander, C. L. Sawyers and W. L. Gerald, Cancer Cell, 2010, 18, 11–22 CrossRef CAS PubMed
.
- K. Erguler and M. P. Stumpf, Mol. BioSyst., 2011, 7, 1593–1602 RSC
.
- L. Chin, J. N. Andersen and P. A. Futreal, Nat. Med., 2011, 17, 297–303 CrossRef CAS PubMed
.
- A. Bashashati, G. Haffari, J. Ding, G. Ha, K. Lui, J. Rosner, D. G. Huntsman, C. Caldas, S. A. Aparicio and S. P. Shah, Genome Biol., 2012, 13, R124 CrossRef PubMed
.
- Y. A. Kim, S. Wuchty and T. M. Przytycka, PLoS Comput. Biol., 2011, 7, e1001095 CAS
.
- R. Chari, B. P. Coe, E. A. Vucic, W. W. Lockwood and W. L. Lam, BMC Syst. Biol., 2010, 4, 67 CrossRef PubMed
.
- L. R. Brunham and M. R. Hayden, Science, 2012, 336, 1112–1113 CrossRef CAS PubMed
.
- S. Ng, E. A. Collisson, A. Sokolov, T. Goldstein, A. Gonzalez-Perez, N. Lopez-Bigas, C. Benz, D. Haussler and J. M. Stuart, Bioinformatics, 2012, 28, i640–i646 CrossRef CAS PubMed
.
- J. Peng, J. Zhu, A. Bergamaschi, W. Han, D. Y. Noh, J. R. Pollack and P. Wang, Ann. Appl. Stat., 2010, 4, 53–77 Search PubMed
.
- R. Chari, B. P. Coe, C. Wedseltoft, M. Benetti, I. M. Wilson, E. A. Vucic, C. MacAulay, R. T. Ng and W. L. Lam, BMC Bioinf., 2008, 9, 422 CrossRef PubMed
.
- N. Fujino, C. Ota, T. Takahashi, T. Suzuki, S. Suzuki, M. Yamada, R. Nagatomi, T. Kondo, M. Yamaya and H. Kubo, BMJ Open, 2012, 2, e001553 CrossRef PubMed
.
- J. Garcia-Fernandez, Nat. Rev. Genet., 2005, 6, 881–892 CrossRef CAS PubMed
.
- T. Rauch, Z. Wang, X. Zhang, X. Zhong, X. Wu, S. K. Lau, K. H. Kernstine, A. D. Riggs and G. P. Pfeifer, Proc. Natl. Acad. Sci. U. S. A., 2007, 104, 5527–5532 CrossRef CAS PubMed
.
- D. Wang, H. Zhang, M. Li, M. G. Frid, A. R. Flockton, B. A. McKeon, M. E. Yeager, M. A. Fini, N. W. Morrell, S. S. Pullamsetti, S. Velegala, W. Seeger, T. A. McKinsey, C. C. Sucharov and K. R. Stenmark, Circ. Res., 2013, 114, 67–78 CrossRef PubMed
.
- H. Bi, J. Zhou, D. Wu, W. Gao, L. Li, L. Yu, F. Liu, M. Huang, I. M. Adcock, P. J. Barnes and X. Yao, Inflammation Res., 2015, 64, 119–126 CrossRef CAS PubMed
.
- M. S. Kumar, E. Armenteros-Monterroso, P. East, P. Chakravorty, N. Matthews, M. M. Winslow and J. Downward, Nature, 2013, 505, 212–217 CrossRef PubMed
.
- G. R. V. Pottelberge, P. Mestdagh, K. R. Bracke, O. Thas, Y. M. T. A. v. Durme, G. F. Joos, J. Vandesompele and G. G. Brusselle, Am. J. Respir. Crit. Care Med., 2011, 183, 898–906 CrossRef PubMed
.
- C. P. Hersh, N. N. Hansel, K. C. Barnes, D. A. Lomas, S. G. Pillai, H. O. Coxson, R. A. Mathias, N. M. Rafaels, R. A. Wise, J. E. Connett, B. J. Klanderman, F. L. Jacobson, R. Gill, A. A. Litonjua, D. Sparrow, J. J. Reilly, E. K. Silverman and I. Investigators, Am. J. Respir. Cell Mol. Biol., 2009, 41, 324–331 CrossRef CAS PubMed
.
- W. Zou, Y. Zou, Z. Zhao, B. Li and P. Ran, Am. J. Physiol.: Lung Cell. Mol. Physiol., 2013, 304, L199–209 CrossRef CAS PubMed
.
- N. Turan, S. Kalko, A. Stincone, K. Clarke, A. Sabah, K. Howlett, S. J. Curnow, D. A. Rodriguez, M. Cascante, L. O'Neill, S. Egginton, J. Roca and F. Falciani, PLoS Comput. Biol., 2011, 7, e1002129 CAS
.
- M. E. Ritchie, B. Phipson, D. Wu, Y. Hu, C. W. Law, W. Shi and G. K. Smyth, Nucleic Acids Res., 2015, 43, e47 CrossRef PubMed
.
- I. Diboun, L. Wernisch, C. A. Orengo and M. Koltzenburg, BMC Genomics, 2006, 7, 252 CrossRef PubMed
.
- X. Jiao, B. T. Sherman, W. Huang da, R. Stephens, M. W. Baseler, H. C. Lane and R. A. Lempicki, Bioinformatics, 2012, 28, 1805–1806 CrossRef CAS PubMed
.
-
M. B. Sesen, T. Kadir, R. B. Alcantara, J. Fox, M. Brady, AMIA…Annual Symposium proceedings/AMIA Symposium. AMIA Symposium, 2012, 2012, 838–847.
- L. Ma, X. Liu, L. Song, C. Zhou, X. Zhao and Y. Zhao, Comput. Med. Imaging Graph., 2015, 40, 39–48 CrossRef PubMed
.
- R. Govindan and J. Weber, Clin. Cancer Res., 2014, 20, 4419–4421 CrossRef CAS PubMed
.
- A. Rodriguez-Gonzalez, J. Torres-Nino, M. A. Mayer, G. Alor-Hernandez and M. D. Wilkinson, Comput. Math. Methods Med., 2012, 2012, 367345 Search PubMed
.
- Y. Pan, H. Fu, Q. Kong, Y. Xiao, Q. Shou, H. Chen, Y. Ke and M. Chen, J. Ethnopharmacol., 2014, 155, 1589–1596 CrossRef CAS PubMed
.
- K. Y. Lee, K. Ito, R. Hayashi, E. P. Jazrawi, P. J. Barnes and I. M. Adcock, J. Immunol., 2006, 176, 603–615 CrossRef CAS
.
- K. Y. Lee, S. C. Ho, Y. F. Chan, C. H. Wang, C. D. Huang, W. T. Liu, S. M. Lin, Y. L. Lo, Y. L. Chang, L. W. Kuo and H. P. Kuo, Eur. Respir. J., 2012, 40, 863–873 CrossRef CAS PubMed
.
- L. Duijts, I. K. Reiss, G. Brusselle and J. C. de Jongste, Eur. J. Epidemiol., 2014, 29, 871–885 CrossRef PubMed
.
- S. S. Sohal, D. Reid, A. Soltani, C. Ward, S. Weston, H. K. Muller, R. Wood-Baker and E. H. Walters, Respir. Res., 2011, 12, 130 CrossRef CAS PubMed
.
- A. S. Qadir, K. M. Woo, H.-M. Ryoo and J.-H. Baek, Exp. Cell Res., 2013, 319, 2125–2134 CrossRef CAS PubMed
.
- S. T. Gohy, B. R. Detry, M. Lecocq, C. Bouzin, B. A. Weynand, G. D. Amatngalim, Y. M. Sibille and C. Pilette, Am. J. Respir. Crit. Care Med., 2014, 190, 509–521 CrossRef CAS PubMed
.
- O. Boucherat, J. Chakir and L. Jeannotte, Biol. Open, 2012, 1, 677–691 CrossRef CAS PubMed
.
- Y. Katoh and M. Katoh, Int. J. Oncol., 2004, 25, 1875–1880 CAS
.
- F. Cappello, A. Di Stefano, S. David, F. Rappa, R. Anzalone, G. La Rocca, S. E. D'Anna, F. Magno, C. F. Donner, B. Balbi and G. Zummo, Cancer, 2006, 107, 2417–2424 CrossRef CAS PubMed
.
- M. Siganaki, A. V. Koutsopoulos, E. Neofytou, E. Vlachaki, M. Psarrou, N. Soulitzis, N. Pentilas, S. Schiza, N. M. Siafakas and E. G. Tzortzaki, Respir. Res., 2010, 11, 46 CrossRef PubMed
.
- P. Zarogoulidis, K. Zarampouka, H. Huang, K. Darwiche, Y. Huang, A. Sakkas and K. Zarogoulidis, J. Thorac. Dis., 2013, 5, 195–197 Search PubMed
.
- H. A. Baarsma, A. I. Spanjer, G. Haitsma, L. H. Engelbertink, H. Meurs, M. R. Jonker, W. Timens, D. S. Postma, H. A. Kerstjens and R. Gosens, PLoS One, 2011, 6, e25450 CAS
.
- B. Wang, H. Zhou, J. Yang, J. Xiao, B. Liang, D. Li, H. Zhou, Q. Zeng, C. Fang, Z. Rao, H. Yu, X. Ou and Y. Feng, Gene, 2013, 531, 101–105 CrossRef CAS PubMed
.
- I. Shi, N. Hashemi Sadraei, Z. H. Duan and T. Shi, Cancer Inf., 2011, 10, 273–285 CrossRef CAS PubMed
.
- S. Mishra, Cancer Inf., 2014, 13, 93–108 CrossRef PubMed
.
- T. Li, X. Liao, P. Lochhead, T. Morikawa, M. Yamauchi, R. Nishihara, K. Inamura, S. A. Kim, K. Mima, Y. Sukawa, A. Kuchiba, Y. Imamura, Y. Baba, K. Shima, J. A. Meyerhardt, A. T. Chan, C. S. Fuchs, S. Ogino and Z. R. Qian, Ann. Surg. Oncol., 2014, 21, 4164–4173 CrossRef PubMed
.
- T. Valenta, G. Hausmann and K. Basler, EMBO J., 2012, 31, 2714–2736 CrossRef CAS PubMed
.
- Q. X. Liu, X. S. Liu, W. Ni, S. X. Chen and Y. J. Xu, Chin. J. Tuberc. Respir. Dis., 2012, 35, 828–832 Search PubMed
.
- K. Kumawat, T. Koopmans and R. Gosens, Expert Opin. Ther. Targets, 2014, 18, 1023–1034 CrossRef CAS PubMed
.
- R. A. O'Donnell, A. Richter, J. Ward, G. Angco, A. Mehta, K. Rousseau, D. M. Swallow, S. T. Holgate, R. Djukanovic, D. E. Davies and S. J. Wilson, Thorax, 2004, 59, 1032–1040 CrossRef PubMed
.
- M. S. Kumar, E. Armenteros-Monterroso, P. East, P. Chakravorty, N. Matthews, M. M. Winslow and J. Downward, Nature, 2014, 505, 212–217 CrossRef CAS PubMed
.
- Y. Chen, H. Shao, H. Li, L. Han and X. Zhang, Zhongguo Feiai Zazhi, 2012, 15, 65–71 CAS
.
- X. Xu, J. E. Kim, P. L. Sun, S. B. Yoo, H. Kim, Y. Jin and J. H. Chung, Exp. Ther. Med., 2015, 9, 311–318 Search PubMed
.
- K. Tot Veres, Med. Pregl., 2012, 65, 146–151 CrossRef PubMed
.
- M. F. Sheikholeslami, J. Sadraei, P. Farnia, M. Forozandeh Moghadam and H. Emadikochak, Med. Mycol., 2015, 53, 361–368 CrossRef PubMed
.
- S. Sakao and K. Tatsumi, Respirology, 2011, 16, 1056–1063 CrossRef PubMed
.
- S. E. Stanley, J. J. Chen, J. D. Podlevsky, J. K. Alder, N. N. Hansel, R. A. Mathias, X. Qi, N. M. Rafaels, R. A. Wise, E. K. Silverman, K. C. Barnes and M. Armanios, J. Clin. Invest., 2015, 125, 563–570 Search PubMed
.
- L. Duijts, I. K. Reiss, G. Brusselle and J. C. de Jongste, Eur. J. Epidemiol., 2014, 29, 871–885 CrossRef PubMed
.
- K. L. Ellis, V. A. Cameron, R. W. Troughton, C. M. Frampton, L. J. Ellmers and A. M. Richards, Eur. J. Heart Failure, 2013, 15, 1138–1147 CrossRef CAS PubMed
.
- K. Z. Zhong, W. W. Chen, X. Y. Hu, A. L. Jiang and J. Zhao, Int. J. Clin. Exp. Pathol., 2014, 7, 4545–4551 Search PubMed
.
- G. R. Van Pottelberge, P. Mestdagh, K. R. Bracke, O. Thas, Y. M. van Durme, G. F. Joos, J. Vandesompele and G. G. Brusselle, Am. J. Respir. Crit. Care Med., 2011, 183, 898–906 CrossRef PubMed
.
- P. Leidinger, A. Keller, A. Borries, H. Huwer, M. Rohling, J. Huebers, H. P. Lenhof and E. Meese, Lung Cancer, 2011, 74, 41–47 CrossRef PubMed
.
- S. Pierrou, P. Broberg, R. A. O'Donnell, K. Pawlowski, R. Virtala, E. Lindqvist, A. Richter, S. J. Wilson, G. Angco, S. Moller, H. Bergstrand, W. Koopmann, E. Wieslander, P. E. Stromstedt, S. T. Holgate, D. E. Davies, J. Lund and R. Djukanovic, Am. J. Respir. Crit. Care Med., 2007, 175, 577–586 CrossRef CAS PubMed
.
- G. Wang, Z. Xu, R. Wang, M. Al-Hijji, J. Salit, Y. Strulovici-Barel, A. E. Tilley, J. G. Mezey and R. G. Crystal, BMC Med. Genomics, 2012, 5, 21 CrossRef CAS PubMed
.
- K. Steiling, M. van den Berge, K. Hijazi, R. Florido, J. Campbell, G. Liu, J. Xiao, X. Zhang, G. Duclos, E. Drizik, H. Si, C. Perdomo, C. Dumont, H. O. Coxson, Y. O. Alekseyev, D. Sin, P. Pare, J. C. Hogg, A. McWilliams, P. S. Hiemstra, P. J. Sterk, W. Timens, J. T. Chang, P. Sebastiani, G. T. O'Connor, A. H. Bild, D. S. Postma, S. Lam, A. Spira and M. E. Lenburg, Am. J. Respir. Crit. Care Med., 2013, 187, 933–942 CrossRef CAS PubMed
.
- M. K. Kim and D. S. Lun, Comput. Struct. Biotechnol. J., 2014, 11, 59–65 CrossRef PubMed
.
- X. Jiang, R. Liu, Z. Lei, J. You, Q. Zhou and H. Zhang, Zhongguo Feiai Zazhi, 2010, 13, 451–457 CAS
.
- S. Ying-fang, H. Jing-fang, L. Huan-zhang and Q. Hao-wen, Indian J. Med. Res., 2007, 126, 139–145 Search PubMed
.
- E. M. Mercken, G. J. Hageman, R. C. Langen, E. F. Wouters and A. M. Schols, Chest, 2011, 139, 337–346 CrossRef CAS PubMed
.
- M. C. Morissette, G. Vachon-Beaudoin, J. Parent, J. Chakir and J. Milot, Am. J. Respir. Crit. Care Med., 2008, 178, 240–247 CrossRef CAS PubMed
.
- X. M. Wang, J. Li, M. X. Yan, L. Liu, D. S. Jia, Q. Geng, H. C. Lin, X. H. He, J. J. Li and M. Yao, PLoS One, 2013, 8, e55714 CAS
.
- M. C. Kugler, A. L. Joyner, C. A. Loomis and J. S. Munger, Translational Review, 2014, 52, 1–13 Search PubMed
.
- M. Siganaki, A. V. Koutsopoulos, E. Neofytou, E. Vlachaki, M. Psarrou, N. Soulitzis, N. Pentilas, S. Schiza, N. M. Siafakas and E. G. Tzortzaki, Respir. Res., 2010, 11, 46 CrossRef PubMed
.
- L. Zheng, W. Zhang, M. Jiang, H. Zhang, F. Xiong, Y. Yu, M. Chen, J. Zhou, X. Dai, Y. Tang, M. Jiang, M. Wang, G. Cheng, J. Duan, W. Yu, B. Lin, H. Fu and X. Zhang, Evidence-Based Complementary Altern. Med., 2013, 2013, 160168 Search PubMed
.
- J. H. Xu, H. P. Yang, X. D. Zhou, H. J. Wang, L. Gong and C. L. Tang, Biomed. Environ. Sci., 2015, 28, 105–115 Search PubMed
.
- M. Xaing, X. Liu, D. Zeng, R. Wang and Y. Xu, J. Huazhong Univ. Sci. Technol., Med. Sci., 2010, 30, 159–164 CrossRef PubMed
.
- E. Puig-Vilanova, P. Ausin, J. Martinez-Llorens, J. Gea and E. Barreiro, PLoS One, 2014, 9, e102296 Search PubMed
.
- X. Y. Bai, J. Y. Lin, X. C. Zhang, Z. Xie, H. H. Yan, Z. H. Chen, C. R. Xu, S. J. An, G. M. Sheng and Y. L. Wu, Cancer Biomarkers, 2013, 13, 37–47 CAS
.
- A. Imatani and R. Callahan, Oncogene, 2000, 19, 223–231 CrossRef CAS PubMed
.
- B. You, Y. L. Yang, Z. Xu, Y. Dai, S. Liu, J. H. Mao, O. Tetsu, H. Li, D. M. Jablons and L. You, Oncotarget, 2015, 6, 4357–4368 CrossRef PubMed
.
- Y. Li, J. S. Li, W. W. Li, S. Y. Li, Y. G. Tian, X. F. Lu, S. L. Jiang and Y. Wang, BMC Complementary Altern. Med., 2014, 14, 140 CrossRef PubMed
.
- H. Sakai, M. Horiguchi, C. Ozawa, T. Akita, K. Hirota, K. Shudo, H. Terada, K. Makino, H. Kubo and C. Yamashita, J. Controlled Release, 2014, 196, 154–160 CrossRef CAS PubMed
.
- D. Malhotra, R. K. Thimmulappa, N. Mercado, K. Ito, P. Kombairaju, S. Kumar, J. Ma, D. Feller-Kopman, R. Wise, P. Barnes and S. Biswal, J. Clin. Invest., 2014, 124, 5521 CrossRef PubMed
.
- A. Berndt, A. S. Leme and S. D. Shapiro, EMBO Mol. Med., 2012, 4, 1144–1155 CrossRef CAS PubMed
.
- Y. Bosse, Int. J. Chronic Obstruct. Pulm. Dis., 2012, 7, 607–631 CrossRef CAS PubMed
.
- T. Weng, H. Karmouty-Quintana, L. J. Garcia-Morales, J. G. Molina, M. Pedroza, R. R. Bunge, B. A. Bruckner, M. Loebe, H. Seethamraju and M. R. Blackburn, FASEB J., 2013, 27, 2013–2026 CrossRef CAS PubMed
.
- B. G. Cosio, L. Tsaprouni, K. Ito, E. Jazrawi, I. M. Adcock and P. J. Barnes, J. Exp. Med., 2004, 200, 689–695 CrossRef CAS PubMed
.
- K. Kumawat, T. Koopmans and R. Gosens, Expert Opin. Ther. Targets, 2014, 18, 1023–1034 CrossRef CAS PubMed
.
- D. A. Groneberg, H. Witt, I. M. Adcock, G. Hansen and J. Springer, Exp. Lung Res., 2004, 30, 223–250 CrossRef CAS PubMed
.
- F. E. Uhl, S. Vierkotten, D. E. Wagner, G. Burgstaller, R. Costa, I. Koch, M. Lindner, S. Meiners, O. Eickelberg and M. Konigshoff, Eur. Respir. J., 2015, 46, 1150–1166 CrossRef PubMed
.
Footnotes |
† Electronic supplementary information (ESI) available. See DOI: 10.1039/c5mb00577a |
‡ These authors contributed equally to this work. |
|
This journal is © The Royal Society of Chemistry 2016 |