Improving student understanding of lipids concepts in a biochemistry course using test-enhanced learning†
Received
20th July 2015
, Accepted 5th September 2015
First published on 7th September 2015
Abstract
Test-enhanced learning has successfully been used as a means to enhance learning and promote knowledge retention in students. We have examined whether this approach could be used in a biochemistry course to enhance student learning about lipids-related concepts. Students were provided access to two optional learning modules with questions related to the lipids block of the course. The vast majority of students (98.7–100%) used the optional modules. Student performance increased significantly with increased practice attempts (mean: first attempt – 58.3%; high attempt – 89.6%; p < 0.0001). This improvement was observed across all topics for both conceptual and structure recognition questions, and question types. A subset of questions were modified and included on formal assessments, and the results were compared to students that did not have access to modules (previous year). Incorporation of the modules resulted in a significant improvement in performance on the examination (year 1: 63.4%; year 2: 78.6%, p < 0.0001), including an increase in performance on questions requiring students to discriminate between highly similar topics. Importantly, comparison of student performance on quizzes and exams suggests that module use is associated with an increase in knowledge retention compared to restudy alone within the timeframe of the course. These results suggest that test-enhanced learning can be a valuable educational tool for development of metacognitive and test-taking skills that enhance student learning and understanding of course material.
Introduction
The concepts of lipids and the integration of carbohydrate and lipid metabolism are important topics in biochemistry courses that can be difficult for students to master. In pharmacy education, a strong foundational knowledge of these concepts is critical for understanding diseases states that students will encounter such as atherosclerosis, diabetes, dyslipidemias, and inflammation, as well as the mechanisms of action for therapeutic agents that treat these disease states. The use of a block or modified block curriculum can present additional challenges to student learning of these topics due to the more rapid pace, as well as the fact that this style limits the number of exposures to material. Consequently, we sought to incorporate educational tools into the lipids block of a Cellular Biology and Metabolic Biochemistry course (modified-block curriculum) to enhance student learning and foster long-term knowledge retention since these concepts are encountered again later in the curriculum.
Test-enhanced learning or retrieval practice
Active learning has been shown to improve student performance in various disciplines, including science, technology, engineering, and mathematics (STEM) disciplines. (Freeman et al., 2014) While testing is often used in education as a means to assess student learning, research has shown that it can also be used as an active learning tool to enhance student learning and knowledge retention – a phenomenon known as the “testing effect” (Roediger and Karpicke, 2006; McDaniel et al., 2007a, 2007b; Larsen et al., 2008; Butler, 2010; Roediger et al., 2011a, 2011b). Repeated testing, or retrieval practice, has been shown to enhance performance in the short-term and to slow the forgetting process compared to repeated studying resulting in superior long-term knowledge retention (Karpicke and Roediger, 2007; Pashler et al., 2007; Butler, 2010; Rohrer and Pashler, 2010; Agarwal et al., 2012). Additionally, repeated testing has been shown to enhance the transfer of learning – the ability to apply learning from one context to another or to use learned information in a new way (McDaniel et al., 2007a, 2007b; Butler, 2010; Rohrer et al., 2010; Roediger and Butler, 2011). Test-enhanced learning has been successfully used with question formats ranging from short answer (free recall) to multiple-choice questions (MCQ) (Kang et al., 2007; McDaniel et al., 2007a, 2007b; Smith and Karpicke, 2014; Brame and Biel, 2015). Results from these studies suggest that while students benefit from retrieval practice with both question formats, the magnitude of the effect is greater with recall questions (Kang et al., 2007; McDaniel et al., 2007a, 2007b; Smith and Karpicke, 2014; Brame and Biel, 2015). Additionally, providing detailed feedback enhances the benefits of retrieval practice. Students receiving feedback on their responses, especially if incorrect, perform better on later tests than students that do not receive feedback (Pashler et al., 2007; Roediger and Butler, 2011; Wojcikowski and Kirk, 2013; Wiklund-Hornqvist et al., 2014). Feedback can also serve as an important learning opportunity following summative assessments in STEM courses, especially in light of cumulative final examinations (Schneider et al., 2014a, 2014b). Additionally, detailed feedback provides an opportunity to reinforce important concepts from the course.
Interleaving effects
Repetition is an important component of learning. Consequently, there has been extensive research on how the distribution and nature of the repetitions affect learning. Research has shown that the nature of how repetitions or practice problems are scheduled affects learning (Rohrer and Pashler, 2010; Taylor and Rohrer, 2010; Roediger III and Pyc, 2012; Carpenter, 2014). For example, if working problems of types A, B, and C, the repetitions can be carried out consecutively allowing students to master one problem type before moving on to the next, or they can be worked in a randomized or interleaved order (Fig. 1). Studies have shown that practicing problems in a random or shuffled order promotes superior knowledge retention compared to when the problems are practiced in a massed or blocked order – a finding known as the “interleaving effect” (Rohrer and Pashler, 2010; Taylor and Rohrer, 2010; Roediger III and Pyc, 2012; Carpenter, 2014). Students that practice problems in a blocked order perform better on practice problems than students that practice in an interleaved order; however, students that practice problems in an interleaved order perform better on tests compared to those that practice in blocked order (Rohrer and Taylor, 2007; Rohrer and Pashler, 2010). While interleaved practice by its nature introduces spacing, studies have shown that the benefits of interleaving are largely due to improved discrimination ability of students rather than simply a spacing effect (Rohrer and Pashler, 2007, 2010; Rohrer and Taylor, 2007; Taylor and Rohrer, 2010; Rohrer, 2012; Carvalho and Goldstone, 2014a, 2014b). In general, blocked practice tends to promote the discovery of common features, while interleaving promotes discovery of features that discriminate (Carvalho and Goldstone, 2012, 2014a, 2014b, 2015). Interestingly, students perceive better learning from blocked practice over interleaved practice likely because it is easier and yields a higher practice performance than interleaved practice (Rohrer and Pashler, 2010; Carpenter, 2014). While interleaved practice may be a more challenging way for students to learn, it is a more effective way of learning for long-term knowledge retention.
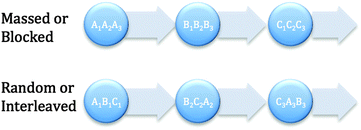 |
| Fig. 1 Different possible orders for practicing problems. | |
Spacing effect
The distribution of repetitions has also been shown to affect learning. Fig. 2 depicts a scenario where students are exposed to material twice prior to a testing event wherein the two exposures to material are separated in time with a delay, or interstudy interval (ISI), and the second exposure is separated in time from the test by a test delay, or retention interval (RI). The length of the ISI between the two exposures has been shown to influence learning and the optimal ISI is dependent on the RI (Rohrer and Pashler, 2007, 2010; Cepeda et al., 2008; Mozer et al., 2009). Studies have shown that information is better retained if the two exposures to the material are separated in time (i.e., spaced) rather than massed (i.e., ISI = 0) as indicated by test performance on both recall and recognition questions – a phenomenon known as the “spacing effect” (Pashler et al., 2007; Rohrer and Pashler, 2007; Cepeda et al., 2008; Kornell and Bjork, 2008; Roediger III and Pyc, 2012; Carpenter, 2014). Interestingly, students perceive massed learning to be more effective than spaced learning even though test results show the opposite is true (Kornell and Bjork, 2008). This may be attributed to the finding that massed learning, or cramming, can produce misleadingly high short-term results as much of the information is not retained over time (Cepeda et al., 2008). Research studies indicate that there is an optimal spacing schedule for the exposures, which is equal to ∼10–20% of the desired RI (Pashler et al., 2007; Cepeda et al., 2008; Mozer et al., 2009). Therefore, if the final testing will occur in one week, the optimal ISI is 1 day, whereas if the desired RI is 1 year, the optimal spacing interval is ∼1 month (3–4 weeks) (Pashler et al., 2007; Cepeda et al., 2008; Mozer et al., 2009). The cost of using an ISI that is too long is smaller than the cost associated with using an ISI that is too short (Rohrer and Pashler, 2007; Cepeda et al., 2008). Consequently, the spacing interval between exposures should be scheduled based on how long the material needs to be retained.
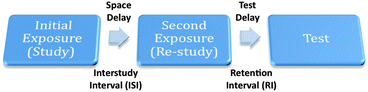 |
| Fig. 2 Effect of distribution on learning. Students are exposed to material twice separated in time by a delay (interstudy interval, ISI), and then tested on that material after a test delay (retention interval, RI) following the second exposure. | |
Metacognitive and test-taking skills
With respect to performance on formal assessments, the use of test-enhanced learning offers additional benefits, such as improvements in metacognitive and test-taking skills (Karpicke et al., 2009; Hagemeier and Mason, 2011; Roediger III et al., 2011b; Stanger-Hall et al., 2011; Tanner, 2012; Schneider et al., 2014a, 2014b; Stewart et al., 2014; Brame and Biel, 2015). Metacognitive skills include self-awareness of what one knows and does not know, the ability to plan strategies for learning, and the ability to make changes to learning processes as needed to improve learning (Karpicke et al., 2009; Hagemeier and Mason, 2011; Stanger-Hall et al., 2011; Tanner, 2012; Schneider et al., 2014a, 2014b; Stewart et al., 2014; Brame and Biel, 2015). In contrast to repeated study, the use of test-enhanced learning allows students to self-assess their understanding of the material, thereby allowing them to focus their study efforts on improving areas of weakness. This approach is similar to practice exams that have been employed in chemistry courses to enhance student metacognitive skill development (Knaus et al., 2009; Schelble et al., 2014). Test-enhanced learning also allows students to make a more accurate assessment of their understanding compared to repeated study, which often leads to overconfidence in their understanding (Roediger III et al., 2011b). Lastly, the use of repeated testing requires students to stay engaged with the course material, thereby promoting good study habits in students (Roediger III et al., 2011b).
Research questions
The Appalachian College of Pharmacy is a three-year Pharm.D. program that uses a modified-block curriculum. Students in the first year (P1) take two courses at any one time, in two three-hour blocks per day. In this format, students cover the equivalent of one week's worth of material in a traditional 3-credit college course per day, which amounts to five week's worth of material per week. The rapid pace of this style curriculum can present challenges to student learning and knowledge retention. In the year prior to introduction of the modules (year 1), students had difficulty with questions related to lipids concepts on examinations – unadjusted mean = 60.7 ± 12.7%, median = 61.4% on all lipids-related exam questions, and mean = 63.4 ± 13.4%, median = 62.1% on non-short answer questions (i.e., multiple choice questions (MCQ), matching, fill-in-the-blank (FITB)). Since students must achieve a grade of 69.5% to receive a C grade in the course, and are only allowed one D in the curriculum, the majority of the class received a score on the lipids section that is below a passing grade in the course. Consequently, we sought to introduce new educational tools into the lipids block in year 2 to improve student learning and knowledge retention. Additionally, student performance on modules should offer insights into areas of student weaknesses and identify knowledge gaps, which can be used for curricular improvements (Roediger III et al., 2011b).
Retrieval practice has been successfully used in science courses to enhance learning (Armbruster et al., 2009; Pyburn et al., 2014; Brame and Biel, 2015; Dobson and Linderholm, 2015; Hernick, in press). Therefore, we set out to use test-enhanced learning, or retrieval practice, to address these following questions: (1) Can retrieval practice be incorporated into a modified block curriculum to enhance student learning and knowledge retention in a biochemistry course? (2) Can retrieval practice and interleaving be integrated into a modified block curriculum to improve the discrimination ability of students? (3) Is student performance on modules predictive of performance on formal assessments?
Methods
Participants
This project received an exemption from the Appalachian College of Pharmacy IRB committee as it was deemed to fall within normal educational practices. Students enrolled in the P1 Cellular Biology and Metabolic Biochemistry course (6 credits) at the Appalachian College of Pharmacy over a two-year period were the participants in this study. Students enrolled in year 1 (n = 74) did not have access to the online modules, and are used as the control group for this study. Students enrolled in year 2 (n = 75) were provided access to the optional online modules through the course page in Moodle. The use of modules in year 2 was optional (voluntary) for students with an unlimited number of attempts, and was not used in the calculation of course grades. Biochemistry is not a pre-requisite for our program or most Pharm.D. programs. Therefore, students may or may not have taken a biochemistry course prior to admission at our institution. In year 1, 46% of students took a biochemistry course prior to enrolment at our institution compared to 48% of students in year 2 (see Appendix, Table S1, ESI†).
Study design
We set out to address the research questions outlined above using a test-enhanced learning approach, and developed two online modules for these purposes in year 2 – Lipids I and Lipids II (Table 1). The Lipids I module primarily tested concepts covered in the first three days of the five-day lipids block (i.e., general properties, ethanol metabolism, fatty acid metabolism and transport, hormonal regulation of carbohydrate and lipid metabolism), while the Lipids II module primarily tested concepts in the last two days of the five-day lipids block (i.e., cholesterol metabolism and transport, hormonal regulation of cholesterol/fatty acid/carbohydrate metabolism, eicosanoids, steroids). The question order in the modules was such that topics were interleaved or random, rather than sequential in nature. Questions in the modules were of varied question formats including MCQ (A-type, K-type, select-all-that-apply (SATA)), fill-in-the-blank (FITB), and matching (Table 1). An effort was made to use a significant number of SATA and K-type questions (∼45% total) since students traditionally have difficulty adjusting to these questions formats that require the students to discriminate between similar concepts.
Table 1 Module question breakdown
Module |
Topic |
Number of questionsa |
Module |
Question typeb |
Number of questionsa |
Total number of questions with number of questions containing structures in parentheses. Questions may contain more than one topic, so the sum of individual topics is greater than the total number of questions.
Question types included multiple choice (A-type, K-type, Select-all-that-apply (SATA)), matching, and fill-in-the-blank (FITB)/numerical.
|
Lipids I |
Total
|
46 (4)
|
Lipids I |
Total
|
46 (4)
|
Cholesterol metabolism & transport |
3 (0) |
A-type |
16 (2) |
Ethanol metabolism |
4 (0) |
K-type |
9 (0) |
Fatty acid metabolism & transport |
29 (0) |
SATA |
13 (0) |
General properties |
8 (4) |
Matching |
7 (2) |
Hormonal regulation |
13 (0) |
FITB/numerical |
1 (0) |
|
Lipids II |
Total
|
28 (5)
|
Lipids II |
Total
|
28 (5)
|
Cholesterol metabolism & transport |
11 (0) |
A-type |
7 (2) |
Eicosanoids |
10 (3) |
K-type |
6 (0) |
Fatty acid metabolism & transport |
3 (0) |
SATA |
5 (1) |
Hormonal regulation |
2 (0) |
Matching |
10 (2) |
Steroids |
6 (2) |
FITB/numerical |
— |
Quiz and exam questions from year 1 served as the starting point for development of the modules in year 2. Modules were created using the “Quiz” feature in Moodle, and questions were designed to provide students with immediate detailed feedback as shown in Fig. 3. The use of detailed feedback is a critical component of the modules since they are designed to be a complementary learning tool to the course lectures and active learning assignments, and not simply a means for self-assessment by students. The “General Feedback” section was used to provide explanations and reinforce the overall concept(s) being tested by the question and the rationale for the correct response(s), while feedback in the individual response fields provided an explanation as to why a specific answer was correct or incorrect.
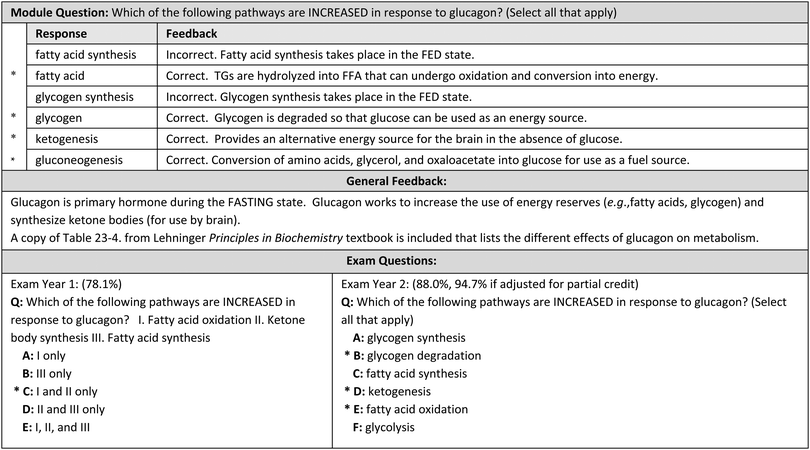 |
| Fig. 3 Sample module question. This figure depicts the two types of feedback provided to students – general and response field. Shown at the bottom are the related exam questions with student performance. | |
Student understanding of lipids concepts were formally assessed with one quiz and one examination each year. These were administered through ExamSoft with both the question order and response fields randomized. Formal assessments contained both non-essay questions similar to the questions found in the optional modules, as well as short answer questions that were more closely related to other course active learning assignments. This study will focus on non-essay questions since these are most closely related to the material covered in the modules. Select questions from the modules, including modified questions, were incorporated on formal assessments. Modifications used include: question type, response type (e.g., chemical structure vs. chemical name), distractor number and/or identity, or correct answer number and/or identity. To account for the fact that identical assessments were not used in years 1 and 2, we estimated the level of difficulty of formal assessments using the mean of the facility indices (% correct) from matched module questions for first attempts and predicted performance using the mean for all attempts (Hernick, in press). The mean for first attempts provides the best estimate of difficulty since this is the only category of attempt that excludes memorization of the correct response from a previous attempt. In the absence of modules, student performance on all attempts was previously shown to be the closest predictor of student performance on assessments (Hernick, in press).
Data analysis
Moodle provides users with statistical information on performance for both the module (i.e., collective group of all questions) and individual questions for four different categories of attempts – first attempt, last attempt, highest attempt, and all attempts. For each module, we report the mean, median, standard deviation (SD), coefficient of internal consistency (CIC), and standard error (SE) for all four attempt categories. Results for individual question performance were exported for further analysis with Excel and KaleidaGraph. Module questions were manually categorized and sorted in Excel by question type and topic(s). Statistical analysis of the facility index (% correct) and discrimination index (DI) for all questions was carried out using the Statistics and ANOVA functions (alpha = 0.05) in KaleidaGraph. Questions were categorized in ExamSoft by topic and question type, analyzed using the built-in “Longitudinal Analysis” feature, and exported for additional analysis using Excel and KaleidaGraph. All questions were scored in ExamSoft and we report the difficulty (% correct), discrimination index (DI), and Point Biserial. For the analysis in this study, no partial credit was given on SATA or matching questions except where noted. Results from ExamSoft were exported for statistical analysis in KaleidaGraph using the Statistics and ANOVA (alpha = 0.05) functions. For cohort analysis, students were sorted by final course grade and divided into three populations: top 33.3% (n = 25, 25), middle 33.3% (n = 24, 25), and bottom 33.3% (n = 25, 25).
Results
Student use of modules
To address the research questions outlined above, we created optional learning modules accessible to students through the course page on Moodle. The majority of students in year 2 accessed and attempted the modules (Lipids I: 100%, Lipids II: 98.7%) prior to formal assessments. There were a total of 287 completed attempts (100% students) on the Lipids 1 module and 246 completed attempts (91.9% students) on the Lipids II module. This equates to an average of 3.3–3.8 unique completed attempts/student. Additionally, there were 31 partial attempts on the Lipids I module, meaning that students submitted their responses before answering all of the questions, and 24 partial attempts on the Lipids II module. This is an outstanding level of participation for an optional assignment that is not directly included in course grade calculations.
Student performance on modules
To address our first research question regarding whether retrieval practice could be used in a biochemistry course to enhance student learning and knowledge retention, we examined student performance on the modules (Table 2). As expected, performance on the first attempts of the Lipids I and II modules (mean = 53.7% and 61.1%, respectively) was significantly lower than the performance on later attempts for both modules. This finding was anticipated since students use the modules as a means to study, not simply as a method for self-assessment after studying. Consequently, the first attempt can serve as a “baseline” for previous knowledge and easily understood concepts presented in lecture. Module scores improved with repeated attempts, increasing both the mean (84.8% and 86.2%, p < 0.0001) and median (90.8% and 93.1%) scores for last attempts. In general, scores on the Lipids I module are higher than the Lipids II module (1.4–7.4%). A more detailed analysis of student performance on specific topics is provided below. For both modules, the median is larger than the mean (all categories of attempts) indicating that more students score higher than the mean compared to below the mean. Consequently, the mean is brought down by a relatively smaller number of students performing below the mean. The improved performance with repeated attempts observed here is consistent with enhanced learning. Additionally, module performance on last, highest, and all attempts is higher than student scores on the exam in year 1 (unadjusted mean = 63.4%, median = 62.1% on non-short answer questions).
Table 2 Student performance on modules
Topic |
Attempt (N)a |
Mean |
Median |
SDb |
CICc |
SEd |
N = number of completed attempts.
Standard deviation (SD).
Coefficient of internal consistency (CIC).
Standard error (SE).
|
Lipids I |
First (74) |
61.1 |
64.7 |
23.0 |
94.3 |
5.52 |
Last (74) |
86.2 |
93.1 |
19.3 |
96.0 |
3.84 |
Highest (74) |
91.0 |
94.6 |
10.1 |
87.9 |
3.5 |
All (287) |
76.3 |
86.5 |
26.3 |
97.2 |
4.42 |
|
Lipids II |
First (68) |
53.7 |
54.8 |
20.9 |
89.0 |
6.9 |
Last (68) |
84.8 |
90.8 |
17.2 |
91.1 |
5.1 |
Highest (68) |
87.1 |
92.6 |
14.4 |
88.3 |
4.9 |
All (246) |
72.2 |
80.4 |
26.0 |
94.9 |
5.9 |
Student performance on questions by topic and question type
To address whether retrieval practice and interleaving could be used in a biochemistry course to improve the discrimination ability of students, we analyzed student performance on the modules by topic and question type (see Appendix, Tables S2 and S3, ESI†). Topics requiring the highest degree of discrimination ability are: hormonal regulation of metabolism, fatty acid metabolism and transport, and cholesterol metabolism and transport. The means for all seven topics on first attempts range from ∼48 to 74%. Importantly, the means for all topics increase with repeated attempts up to 85.4–94.2% on the highest attempts, consistent with increased understanding of the material. These results also reveal student difficulty with structure-based questions (mean: 61.0%) on first attempts (Table S2, ESI†).
Student performance based on question type was also analyzed (Table S3, ESI†). As stated above, an effort was made to use a significant number of SATA and K-type questions since students traditionally have difficulty adjusting to these questions formats that often require higher level of discrimination ability. Student performance on all question types improved with repeated attempts yielding the following trend for last/high/all attempts: A-type/Matching > SATA > FITB > K-type.
The DI is used as a measure of how well questions can discriminate between students of different abilities. A DI ≥ 50 indicates very good discrimination, 30–50 adequate discrimination, and 20–29 weak discrimination. Weak discrimination can be attributed to questions being either too easy or too difficult. The DI (mean) for all topics examined are >50 for both last attempts and all attempts indicating that the questions have good discrimination ability. The DI for all question types is ∼54–60% (last attempt) and >60% (all attempts) indicating that all do a good job discriminating between students with different abilities. The observed improvement for various concepts (hormonal regulation of metabolism, fatty acid metabolism and transport, and cholesterol metabolism and transport) and question types are consistent with improved discrimination ability of students with repeated testing under practice conditions.
Curricular improvements
Test-enhanced learning can also be used to provide feedback to instructors and to identify gaps in knowledge (Roediger III et al., 2011b). While traditional assessments provide instructors with similar information, the use of test-enhanced learning has the potential to identify areas of weakness that are missed on formal assessments due to constraints or limitations on the number of questions since much larger numbers of questions are used in the learning modules compared to quizzes and exams. The facility index for general properties is the highest across all attempt categories (mean: 74.4–94.2%), suggesting that it is the easiest topic for students, while ethanol metabolism has the lowest facility index mean across all attempt categories (47.9–85.4%) indicating that it is the hardest topic for students. Additional areas of concern are cholesterol metabolism and transport, eicosanoids, and hormonal regulation, which all average <73% (all attempts). Based on these results, we have updated the modules to include more questions on these topics to address these weaknesses with future classes.
Student performance on formal assessments
Students in year 1 (n = 74) did not have access to modules and are the control group for this study, while students in year 2 (n = 75) had access to modules. The GPAs and PCAT scores for students in years 1 and 2 at the time of admission were comparable (Table 3), indicating that the students in year 1 are a valid control group for these studies.
Table 3 Student admissions statistics
Statistic |
GPAa |
SGPAa |
PCATb |
Year 1 |
Year 2 |
Year 1 |
Year 2 |
Year 1 |
Year 2 |
Grade point average (GPA) and Science Grade Point Average (SGPA) are out of a 4.0 scale.
Pharmacy College Admission Test (PCAT) percentile ranking (range 1–99).
p-Values between years 1 and 2 for GPA, SGPA, and PCAT were calculated using the ANOVA function in KaleidaGraph (alpha = 0.05).
|
N
|
74 |
75 |
74 |
74 |
74 |
75 |
Mean |
2.90 |
3.00 |
2.69 |
2.81 |
51.1 |
50.6 |
Median |
2.77 |
2.93 |
2.54 |
2.66 |
50.0 |
54.0 |
Standard deviation |
0.46 |
0.40 |
0.54 |
0.54 |
15.7 |
18.1 |
p-Valuec |
0.190 |
0.170 |
0.848 |
Student scores on module questions increase with repeated attempts (combined mean: 58.3% on first attempts, 85.6% on last attempts) under practice conditions. To test whether improved scores on the modules reflected enhanced learning, we assessed student performance on formal assessments (Table 4). Overall, students performed better on all formal assessments questions in year 2 (combined mean = 75.1%) compared to year 1 (combined mean = 68.7%; p = 0.0041), including “matched” questions. Matched questions are questions that are identical or highly similar used in both years. Matched questions on all assessments (quiz + exam) include matched questions from any combination of assessments between the two years (e.g., quiz–quiz, quiz–exam, exam–quiz, exam–exam). Matched questions listed in Table 4 for quizzes and exams include only matched questions on similar assessments with similar testing intervals (i.e., quiz–quiz, exam–exam). Therefore, the total number of matched questions on all assessments is not simply the sum of the values listed for quizzes and exams as these do not include matched quiz–exam or exam–quiz questions. To determine if the improved performance on assessments in year 2 reflected a general overall class improvement or was specific for the module-related questions, we compared the average of each student on the total examination (all questions) to the average on the module-related lipids questions (Fig. 4). A positive value indicates that the student performed better on the lipids-related questions compared to the overall exam, while a negative value indicates that the student performed worse on the lipids-related questions relative to the entire exam. These results show a significant improvement in year 2 (with modules) over year 1 (no modules) with the majority of students having a positive performance indicating that the observed improvement is specific for the lipids-related topics. Interestingly, the use of modules actually improved the DI on formal assessment questions. Together these findings are consistent with enhanced learning in year 2.
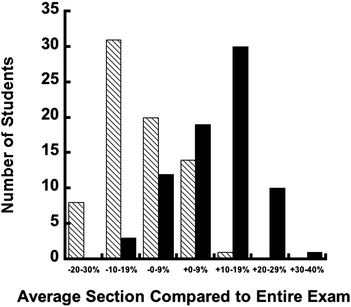 |
| Fig. 4 Student performance on module-related topics compared to entire exam in year 1 (shaded) and year 2 (solid). | |
Table 4 Student performance on formal assessments
Assessment |
Parametera |
Year 1 (Total, matched)b |
Year 2 (Total, matched)b |
p-Valuec |
Predicted difficulty reflects the mean facility index of the corresponding (similar) module questions for first attempts. Predicted performance reflects the mean facility index for the corresponding (similar) module questions for all attempts. Testing interval is the average time between the lecture(s) and assessment. Mean values were calculated using the student scores on formal assessments.
“Total” refers to the total number of questions related to module topics on the assessment(s), while “Matched” refers to similar/identical questions on formal assessments in year 1 and year 2. Matched quiz questions refers only to questions on quizzes in both years, while matched exam questions refer only to questions on exams in both years.
p-Values for the question response rates (total) between years 1 and 2 were calculated using the ANOVA function (alpha = 0.05) in KaleidaGraph.
|
All (Quiz + Exam) |
Number of questions |
45, 34 |
43, 32 |
0.0041 |
Estimated difficulty |
56.1, 55.4 |
55.8, 55.4 |
Predicted performance |
73.0, 72.8 |
73.3, 72.8 |
Mean |
68.7, 68.1 |
75.1, 73.0 |
Median |
68.9, 67.7 |
77.2, 76.1 |
Testing interval |
10.8 |
9.3 |
|
Quiz |
Number of questions |
16, 5 |
18, 5 |
0.0065 |
Estimated difficulty |
56.7, 54.0 |
55.8, 54.0 |
Predicted performance |
73.2, 69.6 |
73.3, 69.6 |
Mean |
78.0, 71.8 |
70.1, 78.1 |
Median |
81.3 |
71.1 |
Testing interval |
8.8 |
5.8 |
|
Exam |
Number of questions |
29, 14 |
25, 14 |
<0.0001 |
Estimated difficulty |
55.8, 59.0 |
55.7, 59.0 |
Predicted performance |
72.9, 74.3 |
73.3, 74.3 |
Mean |
63.4, 62.4 |
78.6, 79.6 |
Median |
62.1 |
81.5 |
Testing interval |
12.8 |
12.8 |
To address whether the use of modules had an impact on knowledge retention during the timeframe of the course, we compared student performance on quizzes and exams since these assess the same material at different testing or retrieval intervals (RI). In year 1, students performed well on quiz questions (Table 2; mean 78.0%, median 81.3%). However, when tested on the same material on the examination just four days later, student performance decreased significantly (mean 71.1%, median 69.6%, p = 0.00121). To determine if the decreased examination score could be attributed to increased question difficulty, we compared the estimated difficulty and predicted performance of both assessments (Table 4). Results from this analysis indicate that the question difficulty and predicted performance for the quiz and examination are comparable. Therefore, the decreased performance on the exam cannot be attributed to increased question difficulty. This finding suggests that the decreased exam scores may be due to a loss of knowledge over time or the “forgetting curve”.
Quiz scores in year 2 were lower than quiz scores in year 1 (mean 70.1%, median 78.0%, p = 0.00648), but comparable to the predicted performance (Table 4; mean = 73.3%). Although quiz scores were lower in year 2, students in year 2 outperformed the students in year 1 on matched quiz/quiz questions (mean 78.1% vs. 71.8%) and matched quiz/exam questions (mean 89.0% vs. 80.0%). The decrease in quiz scores in year 2 may be attributed to differences in quiz composition (year 2: Lipids I and Lipids II material vs. year 1 primarily Lipids I), and the additional study time in year 1 (average testing interval year 1–11.0 days vs. year 2–5.8 days). Additionally, 78–87% of all module attempts were made after the quiz, but before the exam. Unlike year 1, exam scores increased compared to quiz scores (mean 78.6%, p = 0.00123) in year 2. Comparison of the estimated difficulty and predicted performance (Table 4) indicates that question difficulty of the assessments is comparable, and therefore the increased performance on the exam cannot be attributed to lower question difficulty. Exam scores in year 2 are also significantly higher than exam scores in year 1 (78.6% vs. 63.4%, p < 0.0001), even though the estimated difficulty/predicted performance for the exams is comparable. Students in year 2 also outperformed students in year 1 on matched exam/exam (79.6% vs. 78.6%) and quiz/exam (69.1% vs. 63.2%) questions.
Breakdown of student performance by topic and question type
Student performance by topic and question type was also examined (see Appendix, Tables S4 and S5, ESI†). In general, students had more difficulty with Lipids II topics compared to Lipids I in both years (Table S4, ESI†), which is consistent with student performance on the modules. Importantly, there was an increase in the number of topics with passing scores (>69.5%) on exams from 4/7 topics in year 1 to 6/7 topics in year 2 with cholesterol metabolism and transport (68.4%) being the lone topic with a score below passing. In terms of question type (Table S5, ESI†), student performance for all question types improved in year 2 with free response FITB/numerical format being the most difficult question type in both years. Student performance on FITB questions on formal assessments is lower than the module performance. This is likely attributed to the fact that the FITB question used in the module was a single numerical calculation, while formal examinations in both years included FITB questions that assessed students' ability to freely recall information related to concepts practiced with MCQ or short answer questions, which is of higher difficulty.
We assessed the discrimination ability of students by examining student performance on specific topics and question types. Topics requiring the highest degree of discrimination ability are: hormonal regulation of metabolism, fatty acid metabolism and transport, and cholesterol metabolism and transport. Student performance for all of these topics is significantly increased (6.6–17.6%) in year 2. In terms of question type, K-type and SATA questions typically require the highest degree of discrimination ability. Students in year 2 performed at a high level on both K-type (83.3% in year 2 vs. 70.3% in year 1) and SATA (90.5%) questions. Although typically K-type and SATA questions are thought of as having low discrimination abilities, both question types in this study have acceptable DI and point biserials (Table S5, ESI†). These findings are consistent with improved discrimination ability of students in year 2.
Predictability
Our previous studies suggested that module performance on all attempts was the best predictor of formal assessment performance (Hernick, in press). However, due to the limited number of assessment questions used in the previous study, we sought to confirm that finding. For these purposes, we calculated the mean facility index and DI of matched module questions for all attempt categories (see Appendix, Table S6, ESI†), and compared these values to student performance on the formal assessment. Similar to what we previously observed, the assessment scores in year 1 (no modules) and the quiz in year 2 are comparable to the predictions using all attempts (within ∼5%), while the exam score in year 2 is significantly higher than the prediction using all attempts and is more closely described by last attempts. These results confirm that the modules are predictive of student performance, and further support enhanced learning with module use for the exam in year 2.
Student performance by population
We examined the effect of the modules on three populations of students based on final course grades (Table 5 and Fig. 5): bottom 33% (n = 25, 25), middle 33% (n = 24, 25), and top 33% (n = 25, 25) using the approach we previously reported that examines the average difference in exam performance (see also Fig. 4) (Hernick, in press). In this approach, a positive value (>5% increase) indicates the student performed better on the module-related questions relative to the entire exam and a negative value (>5% decrease) indicates that the student performed worse on the module-related questions relative to the entire exam. Differences of less than ±5% are considered modest or neutral, while differences of ≥±10% are considered high positive and high negative performances. In year 1, the vast majority students across all populations (63.5% overall) performed worse on the lipids-related questions relative to the rest of the exam with a large percentage of students having high negative performances (53% overall).
Table 5 Summary of average exam difference based on student population
Performancea |
Populationb |
Number of studentsc |
Mean difference (SD)d |
Year 1 |
Year 2 |
Changee |
Year 1 |
Year 2 |
Changee |
Mean difference between the student's performance on module-related questions (MCQ, matching) and all questions on the assessment. Positive indicates performance on module-related questions ≥5% higher than overall score on the assessment. Neutral reflects an average difference of −5% < x > +5%. Negative indicates performance on module-related questions ≥−5% lower than overall score on the assessment.
Students were divided into three different populations based on final course grades – top 33%, middle 33%, and bottom 33%.
Number of students in each population with indicated performance. Number of students with effect >10% change shown in parentheses.
Mean exam difference and standard deviation for students in the indicated population.
Difference year 2–year 1.
|
Overall |
Total |
74 |
75 |
+1 |
−8.2 (8.7) |
9.4 (10.1) |
+17.6 |
Positive |
Total |
4 (1) |
52 (41) |
+48 (+40) |
8.2 (1.9) |
14.7 (6.8) |
+6.5 |
Bottom |
2 (1) |
17 (14) |
+15 (+13) |
9.0 (2.7) |
16.6 (6.4) |
+7.6 |
Middle |
1 (0) |
18 (15) |
+17 (+15) |
6.6 (−) |
15.8 (7.7) |
+9.2 |
Top |
1 (0) |
17 (12) |
+16 (+12) |
8.3 (−) |
11.6 (5.0) |
+3.3 |
|
Neutral |
Total |
23 |
18 |
−5 |
−0.2 (3.0) |
−0.5 (2.7) |
−0.3 |
Bottom |
5 |
7 |
+2 |
0.6 (2.9) |
−0.5 (2.8) |
−1.1 |
Middle |
9 |
4 |
−5 |
−0.1 (3.6) |
0.2 (2.4) |
+0.3 |
Top |
9 |
7 |
−2 |
−0.8 (2.6) |
−0.8 (3.2) |
0 |
|
Negative |
Total |
47 (39) |
5 (3) |
−42 (−36) |
−13.6 (5.5) |
−9.8 (4.2) |
+3.8 |
Bottom |
18 (13) |
1 (1) |
−17 (−12) |
−9.6 (9.8) |
−12.4 (−) |
−2.8 |
Middle |
14 (14) |
3 (2) |
−11 (−12) |
−15.4 (5.3) |
−10.2 (4.9) |
+5.2 |
Top |
15 (12) |
1 (0) |
−14 (−12) |
−13.2 (5.8) |
−12.4 (−) |
+0.8 |
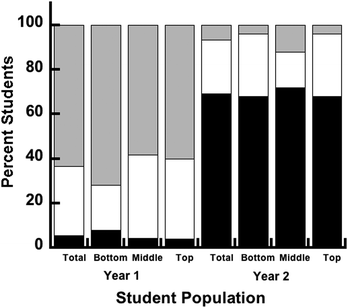 |
| Fig. 5 Student performance on module-related topics compared to entire exam based on final course grade. The percentage of students with a positive (black), neutral (white), and negative (gray) performance are shown for years 1 and 2. | |
Following the introduction of the modules in year 2, there was a significant shift in the number of positive performances (69.3% overall), including high positive performances (54.7% overall). The magnitude of the effect in this study (overall change +17.6%) is larger than what we observed in our previous study (overall change +7.9%) (Hernick, in press). There are also larger increases in the number of positive (from +26 to +48) and high positive (from +12 to +40) performances in year 2. These results suggest that all students benefit from the test-enhanced learning modules.
Discussion
Herein we report on the use of test-enhanced learning modules in a biochemistry course. Results from these studies demonstrate improved performance on all topics and question types under practice conditions, consistent with learning. Student performance on modules for all attempts was comparable to student performance on lipids-related questions on formal assessments from the previous year suggesting that student performance on modules is predictive, consistent with our previous findings (Hernick, in press). The increase in student performance on specific topics (e.g., hormonal regulation, cholesterol metabolism and transport) and question types (i.e., K-type, SATA) suggest an increased ability of students to discriminate between similar concepts. Given the importance of repetition, the modules are most beneficial when there is ample time to allow for repeated attempts over multiple days, which promotes good study habits in students.
Results from this study confirm this improved performance under exam conditions (15.2% increase over year 1). This increase in performance with module use is observed across all student populations based on final course grades. It is likely that improved metacognitive and test-taking skills contribute to the observed increase in examination performance. Additionally, these studies confirm that practice modules are predictive of student performance on formal assessments, and therefore can be used to identify areas for curricular improvements.
We previously attempted to probe knowledge retention by comparing student scores on quizzes and exams since they assess the same material; however, we were unable to conclusively demonstrate an increase in knowledge retention due to the low number of questions (5) on the quizzes in the previous study and the fact that quiz topics were different in the two years (Hernick, in press). Here, we are able to confirm that in the absence of modules (year 1), student performance decreases as the RI is increased (Fig. 6). In contrast, student performance increases as the RI is increased with module use (year 2). This finding may suggest that the use of modules could be beneficial for courses that use cumulative final examinations.
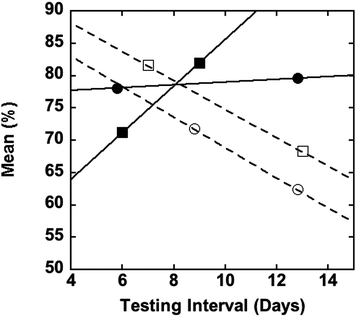 |
| Fig. 6 Student performance on formal assessments for material tested at different intervals with both a quiz and exam. Circles reflect student performance on matched lipids-related questions (described here). Squares reflect student performance on previously reported medicinal chemistry topics (Hernick, in press). Solid symbols and lines reflect performance for students that had access to modules, while open symbols and dashed lines reflect performance without access to modules. | |
Lastly, we addressed whether retrieval practice could be used to circumvent issues with discrimination ability of students due to blocked nature of the curriculum. Student performance on exam questions in year 2 (with modules) based on topic and question type is consistent with an increased ability to discriminate between similar topics. This finding is likely attributed to an interleaving effect due to the question order in the modules, and their use over a series of several days.
Together, these findings contribute to various areas of existing literature. First, these studies expand upon the disciplines that have successfully used test-enhanced learning outside of the laboratory/psychology setting to include an additional STEM course (i.e., biochemistry). Furthermore, they build upon a similar strategy that used practice exams in chemistry courses to improve the development of metacognitive skills in chemistry students (Knaus et al., 2009; Schelble et al., 2014) using a web-based platform that provides students with immediate detailed feedback. This approach can be easily adapted by other chemistry disciplines to improve student learning and promote the development of metacognitive and test-taking skills.
Limitations
While the results from this study are promising, there are limitations associated with our findings. First, this study was carried out using a sample of convenience. Additionally, analyses comparing quiz and exam scores in this study and our previous work (Hernick, in press) reveal a decrease in performance with increasing RI in the absence of modules (Fig. 6) consistent with a loss of knowledge over time. In contrast, this decrease in performance with increasing RI is not observed for students with access to the test-enhanced learning modules. While it is tempting to speculate that this finding is evidence of increased knowledge retention, this study is limited by the fact that the majority of our findings were obtained using an ISI = 1 day and maximum average RI of 12.8 days. Consequently, additional studies using longer ISIs and RIs are needed before any conclusions regarding knowledge retention can be made. One final limitation of this study is that the formal assessment questions used for the study are largely MCQ/FITB in nature. Therefore, we are working to revise and expand these modules with the goal of improving student performance on short-answer (essay) type questions.
Conclusions
In conclusion, herein we examined the use of test-enhanced learning modules to improve student understanding of material in a biochemistry course. Results from these studies indicate an improved student performance on examinations, as well as superior discrimination ability of students. These findings suggest that test-enhanced learning can be a valuable educational tool in biochemistry courses for enhancing student learning, improving student discrimination ability, and promoting the development of metacognitive and test-taking skills necessary for life-long learning.
Acknowledgements
The authors thank Veronica Keene and Kenny Blankenship for providing the admissions statistics data.
References
- Agarwal P., Bain P. and Chamberlain R., (2012), The Value of Applied Research: Retrieval Practice Improves Classroom Learning and Recommendations from a Teacher, a Principal, and a Scientist, Educ. Psychol. Rev., 24, 437–448.
- Armbruster P., Patel M., Johnson E. and Weiss M., (2009), Active Learning and Student-centered Pedagogy Improve Student Attitudes and Performance in Introductory Biology, CBE Life Sci. Educ., 8, 203–213.
- Brame C. J. and Biel R., (2015), Test-Enhanced Learning: The Potential for Testing to Promote Greater Learning in Undergraduate Science Courses, CBE Life Sci. Educ., 14, 1–12.
- Butler A. C., (2010), Repeated testing produces superior transfer of learning relative to repeated studying, J. Exp. Psychol. Learn. Mem. Cogn., 36, 1118–1133.
- Carpenter S. K., (2014), in Benassi V. A., Overson C. E. and Hakala C. M. (ed.) Applying Science of Learning in Education: Infusing Psychological Science into the Curriculum, American Psychological Association, pp. 131–141.
- Carvalho P. F. and Goldstone R. L., (2012), Category structure modulates interleaving and blocking advantage in inductive category acquisition, Sapporo, Japan.
- Carvalho P. F. and Goldstone R. L., (2014a), Effects of Interleaved and Blocked Study on Delayed Test of Category Learning Generalization, Frontiers in Psychology, 5, 936.
- Carvalho P. and Goldstone R., (2014b), Putting category learning in order: category structure and temporal arrangement affect the benefit of interleaved over blocked study, Mem. Cognit., 42, 481–495.
- Carvalho P. and Goldstone R., (2015), The benefits of interleaved and blocked study: different tasks benefit from different schedules of study, Psychon. Bull. Rev., 22, 281–288.
- Cepeda N. J., Vul E., Rohrer D., Wixted J. T. and Pashler H., (2008), Spacing Effects in Learning: A Temporal Ridgeline of Optimal Retention, Psychol. Sci., 19, 1095–1102.
- Dobson J. and Linderholm T., (2015), Self-testing promotes superior retention of anatomy and physiology information, Adv. Health. Sci. Educ., 20, 149–161.
- Freeman S., Eddy S. L., McDonough M., Smith M. K., Okoroafor N., Jordt H. and Wenderoth M. P., (2014), Active learning increases student performance in science, engineering, and mathematics, Proc. Natl. Acad. Sci. U. S. A., 111, 8410–8415.
- Hagemeier N. E. and Mason H. L., (2011), Student Pharmacists' Perceptions of Testing and Study Strategies, Am. J. Pharm. Educ., 75, 35.
- Hernick M., (2015), The Use of Test-Enhanced Learning in an Immunology and Infectious Disease Medicinal Chemistry/Pharmacology Course, Am. J. Pharm. Educ..
- Kang S. H. K., McDermott K. B. and Roediger H. L., (2007), Test format and corrective feedback modify the effect of testing on long-term retention, Eur. J. Cognit. Psychol., 19, 528–558.
- Karpicke J. D. and Roediger H. L., 3rd, (2007), Expanding retrieval practice promotes short-term retention, but equally spaced retrieval enhances long-term retention, J. Exp. Psychol. Learn. Mem. Cogn., 33, 704–719.
- Karpicke J. D., Butler A. C. and Roediger H. L., 3rd, (2009), Metacognitive strategies in student learning: Do students practise retrieval when they study on their own? Memory, 17, 471–479.
- Knaus K. J., Murphy K. L. and Holme T. A., (2009), Designing Chemistry Practice Exams for Enhanced Benefits. An Instrument for Comparing Performance and Mental Effort Measures, J. Chem. Educ., 86, 827.
- Kornell N. and Bjork R. A., (2008), Learning Concepts and Categories: Is Spacing the “Enemy of Induction”? Psychol. Sci., 19, 585–592.
- Larsen D. P., Butler A. C. and Roediger H. L., 3rd, (2008), Test-enhanced learning in medical education, Med. Educ., 42, 959–966.
- McDaniel M. A. Anderson J. L., Derbish M. H. and Morrisette N., (2007a), Testing the testing effect in the classroom, Eur. J. Cognit. Psychol., 19, 494–513.
- McDaniel M. A., Roediger H. L., 3rd and McDermott K. B., (2007b), Generalizing test-enhanced learning from the laboratory to the classroom, Psychon. Bull. Rev., 14, 200–206.
- Mozer M. C., Pashler H., Cepeda N. J., Lindsey R. and Vul E., (2009), Predicting the optimal spacing of study: a multiscale context model of memory, Adv. Neural Inform. Process. Syst., 22, 1321–1329.
- Pashler H., Rohrer D., Cepeda N. and Carpenter S., (2007), Enhancing learning and retarding forgetting: choices and consequences, Psychon. Bull. Rev., 14, 187–193.
- Pyburn D. T., Pazicni S., Benassi V. A. and Tappin E. M., (2014), The Testing Effect: An Intervention on Behalf of Low-Skilled Comprehenders in General Chemistry, J. Chem. Educ., 91, 2045–2057.
- Roediger H. L., 3rd and Butler A. C., (2011), The critical role of retrieval practice in long-term retention, Trends Cognit. Sci., 15, 20–27.
- Roediger H. L. and Karpicke J. D., (2006), Test-enhanced learning: taking memory tests improves long-term retention, Psychol Sci, 17, 249–255.
- Roediger III H. L. and Pyc M. A., (2012), Inexpensive techniques to improve education: applying cognitive psychology to enhance educational practice, J. Appl. Res. Mem. Cogn., 1, 242–248.
- Roediger H. L., Agarwal P. K., McDaniel M. A. and McDermott K. B., (2011a), Test-enhanced learning in the classroom: long-term improvements from quizzing, J. Exp. Psychol., 17, 382–395.
- Roediger III H. L., Putnam A. L. and Smith M. A., (2011b), in Psychology of Learning and Motivation, Elsevier, vol. 55, pp. 1–36.
- Rohrer D., (2012), Interleaving Helps Students Distinguish among Similar Concepts, Educ. Psychol. Rev., 24, 355–367.
- Rohrer D. and Pashler H., (2007), Increasing Retention Without Increasing Study Time, Curr. Dir. Psychol. Sci., 16, 183–186.
- Rohrer D. and Pashler H., (2010), Recent Research on Human Learning Challenges Conventional Instructional Strategies, Educ. Res., 39, 406–412.
- Rohrer D. and Taylor K., (2007), The shuffling of mathematics problems improves learning, Instr. Sci., 35, 481–498.
- Rohrer D., Taylor K. and Sholar B., (2010), Tests enhance the transfer of learning, J. Exp. Psychol. Learn. Mem. Cognit., 36, 233–239.
- Schelble S. M., Wieder M. J., Dillon D. L. and Tsai E., (2014), in Innovative Uses of Assessments for Teaching and Research, American Chemical Society, vol. 1182, ch. 5, pp. 67–92.
- Schneider E. F., Castleberry A. N., Vuk J. and Stowe C. D., (2014a), Pharmacy Students' Ability to Think About Thinking, Am. J. Pharm. Educ., 78, 148.
- Schneider J. L., Hein S. M. and Murphy K. L., (2014b), in Innovative Uses of Assessments for Teaching and Research, American Chemical Society, vol. 1182, ch. 6, pp. 93–112.
- Smith M. A. and Karpicke J. D., (2014), Retrieval practice with short-answer, multiple-choice, and hybrid tests, Memory, 22, 784–802.
- Stanger-Hall K. F., Shockley F. W. and Wilson R. E., (2011), Teaching Students How to Study: A Workshop on Information Processing and Self-Testing Helps Students Learn, CBE Life Sci. Educ., 10, 187–198.
- Stewart D., Panus P., Hagemeier N., Thigpen J. and Brooks L., (2014), Pharmacy Student Self-Testing as a Predictor of Examination Performance, Am. J. Pharm. Educ., 78, 32.
- Tanner K. D., (2012), Promoting Student Metacognition, CBE Life Sci. Educ., 11, 113–120.
- Taylor K. and Rohrer D., (2010), The effects of interleaved practice, Applied Cognitive Psychology, 24, 837–848.
- Wiklund-Hornqvist C., Jonsson B. and Nyberg L., (2014), Strengthening concept learning by repeated testing, Scand. J. Psychol., 55, 10–16.
- Wojcikowski K. and Kirk L., (2013), Immediate detailed feedback to test-enhanced learning: an effective online educational tool, Med. Teach., 35, 915–919.
Footnote |
† Electronic supplementary information (ESI) available: Tables summarizing student performance based on topic and question type, as well as the predicted performance on formal assessments are provided. See DOI: 10.1039/c5rp00133a |
|
This journal is © The Royal Society of Chemistry 2015 |
Click here to see how this site uses Cookies. View our privacy policy here.