DOI:
10.1039/C5RA07916K
(Paper)
RSC Adv., 2015,
5, 52307-52313
Molecular basis of laccase bound to lignin: insight from comparative studies on the interaction of Trametes versicolor laccase with various lignin model compounds
Received
30th April 2015
, Accepted 1st June 2015
First published on 1st June 2015
Abstract
Laccase, a type of multicopper oxidase, is capable of efficiently degrading lignin. Until now, the molecular basis of laccase interacting with lignin is still poorly understood. Here, five lignin model compounds (2,6-dimethoxyphenol, ferulic acid, guaiacol, sinapic acid and vanillyl alcohol) were selected to demonstrate the key binding mechanisms between Trametes versicolor laccase and lignin. The results showed that the interaction energies of the lignin model compounds with laccase varied widely, which suggested the different molecular efficiencies of laccase in degrading various components of lignin. This was in full agreement with experimental reports. Hydrophobic interactions seemed to be necessary to the interaction of the lignin/lignin model compounds with laccase, while H-bonds were not essential. The molecular basis revealed by this study was helpful in designing highly efficient laccases against lignin waste to achieve environmental protection.
Introduction
Lignin is the second most abundant natural organic polymer after cellulose, constituting about 20% of lignocellulosic material.1–3 Agricultural, industrial and urban activities have accumulated huge amounts of lignin waste4 and other types of pollutants,5–8 causing serious environmental problems. Thus, lignin degradation and its efficient utilization is required for environmental protection.9 The major difficulty in exploring the molecular basis for lignin biodegradation is the high complexity of the lignin structure. Until now, there is still a lack of a complete lignin 3D structure. To address this problem, lignin model compounds were often selected as substitutes for the studies on lignin degradation.10,11 Several lignin model compounds including 2-methoxyphenol, 2,6-dimethoxyphenol, syringyl alcohol, syringic acid, p-coumaric acid, ferulic acid, coniferyl alcohol and vanillyl alcohol have been employed as the representatives for lignin degradation studies.12–14 Thus, consistent with previous studies, we also employed lignin model compounds to investigate the interaction of laccase with lignin in this study.
Laccase, one of main ligninolytic enzymes, has already been used as a biocatalyst in the fields of industry and environmental protection,15 and can efficiently degrade lignin,16 polycyclic aromatic hydrocarbons,17 and other pollutants.15 Laccases are present in microbes, plants and animals.18 Generally, laccases are composed of three domains with three copper-binding sites (T1, T2 and T3). Electron transfer occurs between them. Laccase can either transform contaminants into less toxic compounds or change the nature of the pollutants so that they can be degraded easily.19 Among all the analyzed microbial laccases, Trametes versicolor laccase has received wide attention within the past few decades. The adsorption process of T. versicolor laccase onto alkali lignin was investigated, showing that the presence of a mediator enhanced the binding affinity between laccase and lignin.20 Kolb et al.21 examined the effect of T. versicolor laccase on the variation in phenolic monomers, and found that almost all types of monomeric phenolic compounds could be removed under the proposed conditions. Another study showed that T. versicolor laccase could completely oxidise the micropollutants diclofenac, mefenamic acid, bisphenol A and triclosan.19
It was suggested that the lignin-degrading efficiency was affected by the interaction between laccase and lignin.22 Also, the structures of the lignin model compounds had an impact on the laccase’s oxidation rate.12 Thus, understanding how laccase interacts with lignin is helpful in increasing the lignin-degrading efficiency and broadening our knowledge about the laccase catalysis mechanism. The molecular basis of laccases for lignin biodegradation in relation to the interaction details between laccases and lignin has not been well-understood until now. In this regard, molecular docking and molecular dynamics (MD) simulations have proved to be reliable techniques for the exploration of protein–ligand interactions at the atomic and molecular level.15,23,24 Moreover, it was shown that MD simulations were a useful method to obtain the structural and dynamic information on Pleurotus ostreatus laccase25 and CotA laccase.26
In the present study, molecular docking and molecular dynamics simulations have been used to study and compare the interaction between various lignin model compounds (2,6-dimethoxyphenol, ferulic acid, guaiacol, sinapic acid and vanillyl alcohol) and T. versicolor laccase. Based on the interaction information from the present study, a proposed molecular basis of laccase bound to lignin has been deduced, which might be helpful in the understanding of the lignin degradation reaction.
Materials and methods
Laccase structure
The 3D structure of T. versicolor laccase created by Piontek et al.27 at 1.90 Å resolution, was retrieved from the Protein Data Bank28 (http://www.rcsb.org/pdb/home/home.do) under the code 1GYC. All ligands and water molecules were deleted.
Lignin model compounds studied
The structures of the lignin model compounds were downloaded from ChemSpider29 (http://www.chemspider.com/). A total of five lignin model compounds were selected for the present study: 2,6-dimethoxyphenol, ferulic acid, guaiacol, sinapic acid and vanillyl alcohol.
Docking
The binding site of the lignin model compounds inside laccase was identified by a grid-based cavity prediction algorithm of Molegro Virtual Docker (MVD).30 Then, the lignin model compounds were docked into the binding site. Docking analyses were performed between all analyzed lignin model compounds and laccase. The maximum population size was set to 50 individuals, and 1500 maximum interactions were allowed. The MolDock scoring function (MolDock Score) and Moldock SE algorithm were used to rank the docking poses. To avoid the appearance of the stochastic errors, five runs were carried out for each ligand. The best ranking pose was selected as the optimal binding mode between laccase and the lignin model compound. MD simulations were used to analyze the best binding mode.
Interaction analysis
The interactions of laccase with the lignin model compounds were analyzed and depicted using LigPlot+.31
MD simulations
The lignin model compounds were parameterized using Automated Topology Builder (ATB)32 (http://compbio.biosci.uq.edu.au/atb/). Complexes of laccase with 2,6-dimethoxyphenol, ferulic acid, guaiacol, sinapic acid and vanillyl alcohol were referred to as LAC–DI, LAC–FE, LAC–GU, LAC–SI and LAC–VA, respectively. Each complex was then subjected to MD simulation by the Gromacs 4.5.6 package.33 The parameters used for the protein and ligand were from the gromos53a6 force field. SPC water molecules were applied to solvate these complexes under a periodic boundary condition. The system was neutralized by adding 11 Na+. We performed energy minimization by using the steepest-decent algorithm. These minimized systems were then submitted to NVT (1.4 nm for the short-range electrostatic cutoff and van der Waals cutoff, a time step of 2 fs, 300 K for the Maxwell distribution and −1 for the generation of a random seed) and NPT (no velocity generation, LINCS algorithm34 for constraints and Parrinello–Rahman35 for pressure coupling). Finally, MD simulations were carried out for up to 10 ns using V-rescale36 for temperature coupling. Long range electrostatic interactions were calculated according to the Particle Mesh Ewald method.37
Results
Binding mode
Validation of docking accuracy. Docking analyses were performed between all analyzed lignin model compounds and laccase. We selected the best docking poses for further study. Fig. 1 shows the binding conformations of the lignin model compounds in the active site of laccase. The docking accuracy was validated using the re-docking protocol. We used the best poses of the lignin model compounds as reference ligands. Root-mean-square deviations (RMSD) between the re-docked poses and reference ligands were 0.043 Å for 2,6-dimethoxyphenol, 0.015 Å for ferulic acid, 0.009 Å for guaiacol, 0.463 Å for sinapic acid and 0.014 Å for vanillyl alcohol (Table 1). All these RMSD values were lower than 2.0 Å, which was considered as acceptable and correct,38 meaning that the present docking protocol could accurately reveal the conformation and binding mode of the lignin model compounds into the binding pocket of laccase.
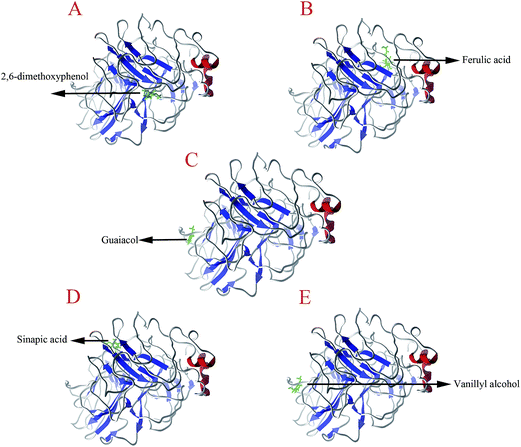 |
| Fig. 1 Binding orientation of the lignin model compounds in laccase. (A) LAC–DI; (B) LAC–FE; (C) LAC–GU, (D) LAC–SI; (E) LAC–VA. | |
Table 1 Interaction energies, docking accuracy, MolDock score and Re-Rank score for laccase with the lignin model compoundsa
Complex |
Interaction energy (kcal mol−1) |
Re-docking RMSD (Å) |
MolDock score |
Re-Rank score |
LAC–DI, complex composed of laccase and 2,6-dimethoxyphenol; LAC–FE, complex composed of laccase and ferulic acid; LAC–GU, complex composed of laccase and guaiacol; LAC–SI, complex composed of laccase and sinapic acid; LAC–VA, complex composed of laccase and vanillyl alcohol. |
LAC–DI |
−89.931 |
0.043 |
−75.925 |
−69.628 |
LAC–FE |
−111.765 |
0.015 |
−103.472 |
−90.888 |
LAC–GU |
−78.992 |
0.009 |
−67.442 |
−60.550 |
LAC–SI |
−112.174 |
0.463 |
−101.184 |
−91.124 |
LAC–VA |
−93.467 |
0.014 |
−83.321 |
−71.087 |
Binding orientation and interaction. The orientation of the ligand is important for acceptor-binding activity. Clearly, the binding orientation of the lignin model compounds inside laccase varied greatly as can be seen from Fig. 1, which suggested the performance of laccase in catalysis for the degradation of each lignin model compound was different. The interaction energies of LAC–DI, LAC–FE, LAC–GU, LAC–SI and LAC–VA were analyzed in detail (Table 1). When making comparisons between these five complexes, the most noticeable difference was the interaction energy. Their interaction energies were in a wide range. Sinapic acid showed the lowest interaction energy with laccase (−112.174 kcal mol−1), which suggested better interaction with the binding site of laccase than the other lignin model compounds. It was shown that 2,6-dimethoxyphenol interacted by H-bonding with residues Gln442 and His402 (Fig. 2). For guaiacol, we found that its interaction energy with laccase was the highest (−78.992 kcal mol−1), lacking H-bonds with laccase. Ferulic acid presented H-bonds with His111, Ala80, Leu459, Glu460, and Gly462 of laccase with an interaction energy of −111.765 kcal mol−1. We further observed that Pro394 participated in the formation of a H-bond with sinapic acid. No H-bonds were found between laccase and vanillyl alcohol. This means that H-bonds were an alternative way to determine the interaction of laccase with the lignin model compounds or lignin.
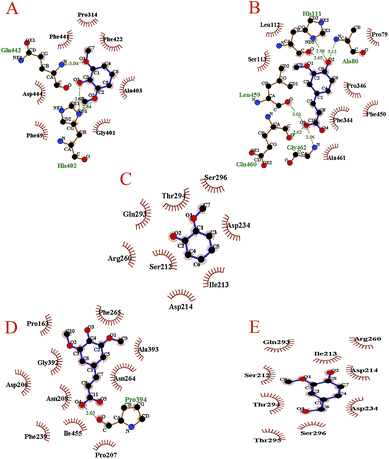 |
| Fig. 2 Interaction of laccase with (A) 2,6-dimethoxyphenol, (B) ferulic acid, (C) guaiacol, (D) sinapic acid and (E) vanillyl alcohol. | |
Hydrophobic interaction seemed to be a more important factor for the binding of laccase to the lignin model compounds than H-bonding, because all the lignin model compounds formed hydrophobic interactions with laccase (Fig. 2). We observed local differences in the types of amino acid residues participating in hydrophobic interactions. 2,6-Dimethoxyphenol presented hydrophobic interactions with seven amino acid residues of laccase: Phe441, Pro314, Phe422, Ala403, Gly401, Asp444 and Phe69. The number of involved residues for ferulic acid was the same as for 2,6-dimethoxyphenol; these included Leu112, Ser113, Pro79, Pro346, Phe344, Phe450 and Ala461. As can be seen in Fig. 2, guaiacol presented hydrophobic interactions with Asp214, Ile213, Ser212, Arg260, Asp234, Gln293, Thr294 and Ser296. In addition, ten amino acid residues took part in the hydrophobic contact with sinapic acid, including Pro163, Gly392, Asp206, Asn208, Phe239, Ile455, Pro207, Phe265, Ala393 and Asn264. In LAC–VA, residues Gln293, Arg260, Ile213, Ser212, Asp214, Thr294, Asp234, Ser296 and Thr295 participated in hydrophobic interactions with vanillyl alcohol. These results showed that hydrophobic interactions were necessary for the binding of laccase to the lignin model compounds, and thus were potentially important for lignin degradation, similar to a previous report that hydrophobic interactions were crucial for determining the binding modes between ligninolytic enzymes and lignin.22
MD simulations
MD simulations have been used for the study of laccase in previous studies.15 For example, 3D models of wild-type enzyme and mutant for Pleurotus ostreatus laccase were constructed, and then were compared using MD simulations.25 Consistent with previous studies, in the present study, MD simulations were conducted for providing insight into the dynamic behavior of the lignin model compounds inside laccase, giving new information on the molecular basis of laccase for lignin degradation.
In previous studies, root-mean-square deviation (RMSD) was often employed to measure the stability of a structure during simulation.39,40 Backbone RMSD calculations were carried out on each complex at every 10 ps during the whole simulation, and the results are shown in Fig. 3. The RMSD values of all the analyzed complexes quickly tended to reach equilibrium, with the maximum deviation being less than 2.9 Å (RMSD values: <2.6 Å for LAC–DI, <2.5 Å for LAC–FE, <2.9 Å for LAC–GU, <2.3 Å for LAC–SI and <2.8 Å for LAC–VA). This indicated that laccase could maintain stability when binding to the lignin model compounds. The RMSD for LAC–DI became stable at around 2400 ps, and remained so for the rest of the simulation time, while the RMSD for LAC–FE initially increased to about 0.18 nm, and then was at a stable level. From Fig. 3, it was found that for LAC–GU, the RMSD value was 0.21 nm at 4000 ps, and held steady until the end. The RMSD values for LAC–SI and LAC–VA became stable after 7000 ps and 3200 ps, respectively.
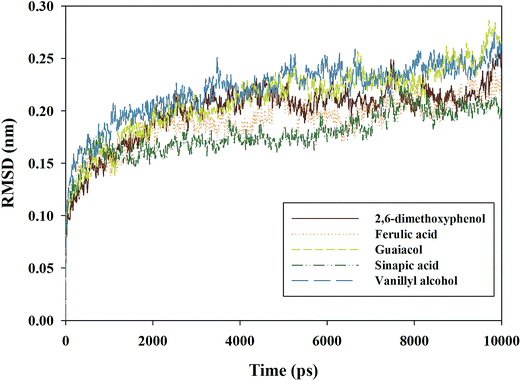 |
| Fig. 3 Backbone RMSD for LAC–DI, LAC–FE, LAC–GU, LAC–SI and LAC–VA. | |
The radius of gyration (Rg) reflects the compaction of a structure.41 The Rg of any analyzed complex did not fluctuate sharply during the whole simulation except for the initial stage which had a relatively fast increase (Fig. 4). The average Rg for LAC–DI, LAC–FE, LAC–GU, LAC–SI and LAC–VA was 2.213 nm, 2.216 nm, 2.217 nm, 2.218 nm and 2.222 nm, respectively.
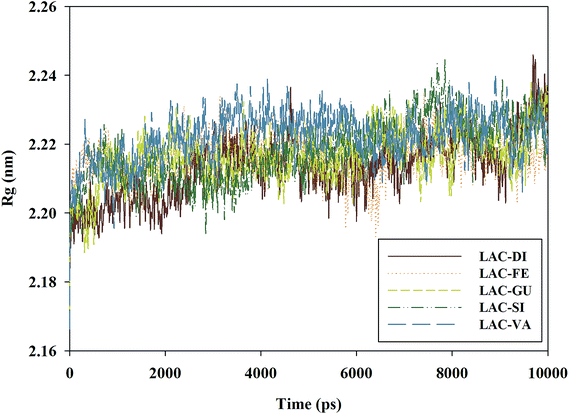 |
| Fig. 4 Radius of gyration for LAC–DI, LAC–FE, LAC–GU, LAC–SI and LAC–VA. | |
Root-mean square fluctuation (RMSF) was calculated to analyze the dynamics of Cα atoms from laccase, and was shown in Fig. 5. The Cα-RMSF value for LAC–DI was highest in residue 497. LAC–DI exhibited higher flexibility in regions composed of residues 68–78, 353–366, 424–439 and 487–500. Fluctuation in residues 171–198, 261–300, 350–370, 424–439 and 487–500 for LAC–GU was greater than in other regions. The largest fluctuation for LAC–GU was found in residues 169–186, 260–275, 425–440 and 484–500. As seen in the Cα-RMSF plot, key fluctuations for LAC–SI occurred around the residues 148–187, 427–439 and 488–500. The maximum fluctuations for LAC–VA were found in residues 463–500.
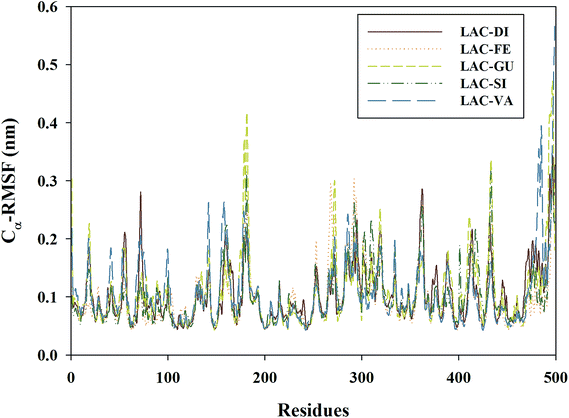 |
| Fig. 5 Cα-RMSF for LAC–DI, LAC–FE, LAC–GU, LAC–SI and LAC–VA. | |
Discussion
Biodegradation technology is becoming more and more attractive for environmental remediation due to its environmentally friendly nature.42 Laccase-based applications for lignin waste degradation are a good example.3,43,44 To increase the lignin-degrading efficiency of laccase, previous studies investigated the impact of substrate structure on the laccase-mediated oxidation rate,12 the stability of five fungal laccases in organic solvents13 and the bioconversion of substrates by Rhodococcus opacus DSM 1069 and PD630,14 using lignin model compounds. The lignin-degrading efficiency of laccase was largely related to the properties of the enzyme and substrates,12 such as their binding property.22 The stability and catalytic activities of laccase were potentially influenced by the binding modes between it and its substrates. However, the detailed interaction mechanism between laccase and lignin is still unclear, limiting the application of laccase in lignin degradation to some extent. Thus, the illustration of interaction between laccase and lignin model compounds is important. Molecular simulations such as molecular docking and MD simulations have proved to be a robust technology for the analyses of intermolecular interactions.41,45–49 In this article we performed an investigation of the molecular basis of laccases for lignin degradation, using molecular docking and molecular dynamics simulations. We showed that the present protocol was capable of giving a molecular insight into the interaction of laccase with lignin model compounds, and in this way we found several rules that may be important to lignin degradation.
It was shown that the lignin model compounds bound to laccase with a wide range of interaction energies. Guaiacol had the worst interaction with laccase, whereas sinapic acid had the best interaction with laccase, because a lower interaction energy often represents a better interaction.38
On the one hand, laccase formed H-bonds with 2,6-dimethoxyphenol, ferulic acid and sinapic acid, but not with vanillyl alcohol and guaiacol. On the other hand, laccase had hydrophobic contacts with all the analyzed lignin model compounds. Therefore, we proposed that H-bonds were not essential, but hydrophobic contacts were necessary to the interaction of laccase with lignin model compounds or lignin.
Mean backbone RMSD values for different complexes varied (LAC–DI: 0.199 nm; LAC–FE: 0.190 nm; LAC–GU: 0.211 nm; LAC–SI: 0.178 nm; LAC–VA: 0.220 nm). This not only meant the stable behavior of these complexes, but also indicated that the stability was different between various complexes. The stability of each complex was also confirmed by the Rg and Cα-RMSF values. The fluctuation of Rg was not very sharp, reflecting well the compaction of analyzed structures.23 The dominant fluctuating regions were often inconsistent between these surveyed complexes as shown in the Cα-RMSF plot. Overall, the MD simulation results showed that LAC–DI, LAC–FE, LAC–GU, LAC–PC, LAC–SI and LAC–VA were reasonably stable during the simulation.
Notably, steric hindrance might occur in the lignin-degrading process mediated by laccase when the substrate is real lignin due to the fact that lignin is bulky and a high molecular weight aromatic compound. Fortunately, it was suggested that the introduction of mediators could solve this steric hindrance problem.50 We will simulate the effect of mediators on the interaction of laccase with lignin using MD simulations in the future.
Conclusion
This study explored the molecular basis of laccase for lignin degradation by comparing the interaction of T. versicolor laccase with lignin based on five lignin model compounds, using molecular docking and MD simulations. The best poses were adopted on the basis of the MolDock score and Re-Rank score. Hydrophobic contacts were necessary, but H-bonds were not essential to the interaction of laccase with the lignin model compounds or lignin. The interaction energies varied in a wide range. RMSD, Cα-RMSF and Rg values from the MD simulation results showed that the systems composed of laccase and the lignin model compounds were highly stable. The present study provides dynamic and structural information on the interaction mechanism between laccase and lignin, which is useful to develop new laccases with high lignin-degrading ability in the field of environmental protection and industrial applications.
Conflicts of interest
Authors declare that they have no competing interests.
Acknowledgements
The study was financially supported by the National Natural Science Foundation of China (51039001, 513781906, 51408206), the Program for Changjiang Scholars and Innovative Research Team in University (IRT-13R17), the Shanghai Tongji Gao Tingyao Environmental Science & Technology Development Foundation (STGEF) and the Fundamental Research Funds for the Central Universities.
References
- T. D. Bugg, M. Ahmad, E. M. Hardiman and R. Rahmanpour, Nat. Prod. Rep., 2011, 28, 1883–1896 RSC.
- A. J. Ragauskas, G. T. Beckham, M. J. Biddy, R. Chandra, F. Chen, M. F. Davis, B. H. Davison, R. A. Dixon, P. Gilna and M. Keller, Science, 2014, 344, 1246843 CrossRef PubMed.
- S. Majumdar, T. Lukk, J. O. Solbiati, S. Bauer, S. K. Nair, J. E. Cronan and J. A. Gerlt, Biochemistry, 2014, 53, 4047–4058 CrossRef CAS PubMed.
- C. Feng, G. Zeng, D. Huang, S. Hu, M. Zhao, C. Lai, C. Huang, Z. Wei and N. Li, Process Biochem., 2011, 46, 1515–1520 CrossRef CAS PubMed.
- G. Zeng, M. Chen and Z. Zeng, Nature, 2013, 499, 154 CrossRef CAS PubMed.
- G. Zeng, M. Chen and Z. Zeng, Science, 2013, 340, 1403 CrossRef CAS PubMed.
- J.-L. Gong, B. Wang, G.-M. Zeng, C.-P. Yang, C.-G. Niu, Q.-Y. Niu, W.-J. Zhou and Y. Liang, J. Hazard. Mater., 2009, 164, 1517–1522 CrossRef CAS PubMed.
- P. Xu, G. M. Zeng, D. L. Huang, C. L. Feng, S. Hu, M. H. Zhao, C. Lai, Z. Wei, C. Huang and G. X. Xie, Sci. Total Environ., 2012, 424, 1–10 CrossRef CAS PubMed.
- V. Mendu, T. Shearin, J. E. Campbell, J. Stork, J. Jae, M. Crocker, G. Huber and S. DeBolt, Proc. Natl. Acad. Sci. U. S. A., 2012, 109, 4014–4019 CrossRef CAS PubMed.
- Q. Bu, H. Lei, A. H. Zacher, L. Wang, S. Ren, J. Liang, Y. Wei, Y. Liu, J. Tang and Q. Zhang, Bioresour. Technol., 2012, 124, 470–477 CrossRef CAS PubMed.
- P. J. Deuss and K. Barta, Coord. Chem. Rev., 2015 DOI:10.1016/j.ccr.2015.1002.1004.
- M. Lahtinen, K. Kruus, H. Boer, M. Kemell, M. Andberg, L. Viikari and J. Sipila, J. Mol. Catal. B: Enzym., 2009, 57, 204–210 CrossRef CAS PubMed.
- P. Maijala, M. L. Mattinen, P. Nousiainen, J. Kontro, J. Asikkala, J. Sipila and L. Viikari, J. Mol. Catal. B: Enzym., 2012, 76, 59–67 CrossRef CAS PubMed.
- M. Kosa and A. J. Ragauskas, Appl. Microbiol. Biotechnol., 2012, 93, 891–900 CrossRef CAS PubMed.
- N. J. Christensen and K. P. Kepp, PLoS One, 2013, 8, e61985 CAS.
- T. D. Bugg, M. Ahmad, E. M. Hardiman and R. Singh, Curr. Opin. Biotechnol., 2011, 22, 394–400 CrossRef CAS PubMed.
- A. I. Cañas, M. Alcalde, F. Plou, M. J. Martínez, Á. T. Martínez and S. Camarero, Environ. Sci. Technol., 2007, 41, 2964–2971 CrossRef.
- P. Strong and H. Claus, Crit. Rev. Environ. Sci. Technol., 2011, 41, 373–434 CrossRef PubMed.
- J. Margot, J. Maillard, L. Rossi, D. A. Barry and C. Holliger, New Biotechnol., 2013, 30, 803–813 CrossRef CAS PubMed.
- C. Lai, G. M. Zeng, D. L. Huang, M. H. Zhao, H. L. Huang, C. Huang, Z. Wei, N. J. Li, P. Xu, C. Zhang and G. X. Xie, Int. Biodeterior. Biodegrad., 2013, 82, 180–186 CrossRef CAS PubMed.
- M. Kolb, V. Sieber, M. Amann, M. Faulstich and D. Schieder, Bioresour. Technol., 2012, 104, 298–304 CrossRef CAS PubMed.
- M. Chen, G. Zeng, Z. Tan, M. Jiang, H. Li, L. Liu, Y. Zhu, Z. Yu, Z. Wei, Y. Liu and G. Xie, PLoS One, 2011, 6, e25647 CAS.
- C. B. Jalkute, S. H. Barage, M. J. Dhanavade and K. D. Sonawane, Protein J., 2013, 32, 356–364 CrossRef CAS PubMed.
- H. N. Banavath, O. P. Sharma, M. S. Kumar and R. Baskaran, Sci. Rep., 2014, 4, 6948 CrossRef PubMed.
- G. Festa, F. Autore, F. Fraternali, P. Giardina and G. Sannia, Proteins, 2008, 72, 25–34 CrossRef CAS PubMed.
- N. Gupta, F. S. Lee and E. T. Farinas, J. Mol. Catal. B: Enzym., 2010, 62, 230–234 CrossRef CAS PubMed.
- K. Piontek, M. Antorini and T. Choinowski, J. Biol. Chem., 2002, 277, 37663–37669 CrossRef CAS PubMed.
- P. W. Rose, A. Prlić, C. Bi, W. F. Bluhm, C. H. Christie, S. Dutta, R. K. Green, D. S. Goodsell, J. D. Westbrook and J. Woo, Nucleic Acids Res., 2015, 43, D345–D356 CrossRef PubMed.
- H. E. Pence and A. Williams, J. Chem. Educ., 2010, 87, 1123–1124 CrossRef CAS.
- R. Thomsen and M. H. Christensen, J. Med. Chem., 2006, 49, 3315–3321 CrossRef CAS PubMed.
- R. A. Laskowski and M. B. Swindells, J. Chem. Inf. Model., 2011, 51, 2778–2786 CrossRef CAS PubMed.
- A. K. Malde, L. Zuo, M. Breeze, M. Stroet, D. Poger, P. C. Nair, C. Oostenbrink and A. E. Mark, J. Chem. Theory Comput., 2011, 7, 4026–4037 CrossRef CAS.
- B. Hess, C. Kutzner, D. van der Spoel and E. Lindahl, J. Chem. Theory Comput., 2008, 4, 435–447 CrossRef CAS.
- B. Hess, H. Bekker, H. J. Berendsen and J. G. Fraaije, J. Comput. Chem., 1997, 18, 1463–1472 CrossRef CAS.
- M. Parrinello and A. Rahman, J. Appl. Phys., 1981, 52, 7182–7190 CrossRef CAS PubMed.
- G. Bussi, D. Donadio and M. Parrinello, J. Chem. Phys., 2007, 126, 014101 CrossRef PubMed.
- T. Darden, D. York and L. Pedersen, J. Chem. Phys., 1993, 98, 10089 CrossRef CAS PubMed.
- J. O. Giacoppo, D. T. Mancini, A. P. Guimaraes, A. S. Goncalves, E. F. da Cunha, T. C. Franca and T. C. Ramalho, Eur. J. Med. Chem., 2015, 91, 63–71 CrossRef CAS PubMed.
- X. Wang, H. Yang, X. Hu, X. Zhang, Q. Zhang, H. Jiang, W. Shi and H. Yu, Environ. Sci. Technol., 2013, 47, 11802–11809 CrossRef CAS PubMed.
- J. Jayakumar and S. Anishetty, Bioorg. Med. Chem. Lett., 2014, 24, 2098–2104 CrossRef CAS PubMed.
- C. B. Jalkute, S. H. Barage and K. D. Sonawane, RSC Adv., 2015, 5, 10488–10496 RSC.
- C. Sánchez, Biotechnol. Adv., 2009, 27, 185–194 CrossRef PubMed.
- L. Munk, A. K. Sitarz, D. C. Kalyani, J. D. Mikkelsen and A. S. Meyer, Biotechnol. Adv., 2015, 33, 13–24 CrossRef CAS PubMed.
- S. R. Couto and J. L. T. Herrera, Biotechnol. Adv., 2006, 24, 500–513 CrossRef PubMed.
- K. M. Kumar, A. Anbarasu and S. Ramaiah, Mol. Biosyst., 2014, 10, 891–900 RSC.
- M. Rajasekaran and C. P. Chen, Int. J. Biol. Macromol., 2012, 51, 305–313 CrossRef CAS PubMed.
- M. H. Fazil, S. Kumar, R. Farmer, H. P. Pandey and D. V. Singh, J. Mol. Model., 2012, 18, 1–10 CrossRef CAS PubMed.
- M. T. Cambria, D. Di Marino, M. Falconi, S. Garavaglia and A. Cambria, J. Biomol. Struct. Dyn., 2010, 27, 501–509 CAS.
- S. Kannan, A. Poulsen, H. Y. Yang, M. Ho, S. H. Ang, T. S. Eldwin, D. A. Jeyaraj, L. R. Chennamaneni, B. Liu, J. Hill, C. S. Verma and K. Nacro, Biochemistry, 2015, 54, 32–46 CrossRef CAS PubMed.
- M. A. Tadesse, A. D’Annibale, C. Galli, P. Gentili and F. Sergi, Org. Biomol. Chem., 2008, 6, 868–878 CAS.
|
This journal is © The Royal Society of Chemistry 2015 |
Click here to see how this site uses Cookies. View our privacy policy here.