DOI:
10.1039/C5RA02531A
(Paper)
RSC Adv., 2015,
5, 38939-38947
Experimental design for simultaneous analysis of malachite green and methylene blue; derivative spectrophotometry and principal component-artificial neural network
Received
9th February 2015
, Accepted 22nd April 2015
First published on 23rd April 2015
Abstract
In this study, oxidized multiwalled carbon nanotubes (MWCNT) with sizes in the range of 10–30 nm were efficiently applied for simultaneous and competitive adsorption of malachite green (MG) and methylene blue (MB). The competitive adsorption of MB and MG was studied following accurate and repeatable monitoring in binary mixtures via second order derivative spectrophotometry (SODS) and a principal component–artificial neural network model (PCA–ANN). The MWCNTs were characterized by different techniques such as TEM, XPS and FTIR. The dyes removal percentages as responses were modeled and optimized versus the variables by central composite design (CCD) under response surface methodology and PCA–ANN. The optimum values of initial MG and MB concentrations, MWCNT mass (g) and sonication time (min) were found to be 14.6 and 10 mg L−1, 0.025 g and 2.6 min, respectively, at which the removal percentages of the dyes were maximum. The advantage of this process is its high removal performance in a very short time using a small amount of MWCNT. Various isotherm models were applied and their suitability for describing the experimental equilibrium data was investigated. It was shown that the Langmuir model is able to predict the real behavior of both dyes in the equilibrium mode with removal percentages of more than 95% and adsorption capacities of 102.3 and 57.6 mg g−1 for MB and MG, respectively.
1. Introduction
Nanostructures are of high importance and have exhaustive applications in versatile fields of science and technology.1 Nanomaterials are applied in wastewater treatment. Dyes and their photo and/or chemical induced degradation products can seriously affect the kidneys, reproductive system, liver, brain, and central nervous system.2 Cationic toxic dyes of methylene blue (MB, thiazine cationic)3 and malachite green (MG)4 (Fig. 1), as reported previously, have numerous medicinal, industrial and biological activities.4–8 The carcinogenic, genotoxic, mutagenic and teratogenic properties6–8 of these dyes, which may be related to the presence of nitrogen5 and positive charge on their structures, were tested on animals as a case study.
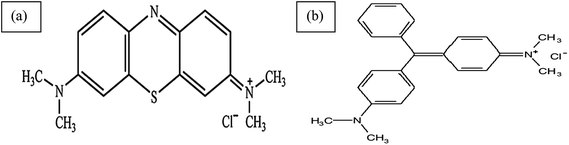 |
| Fig. 1 (a) Chemical structure of MB. (b) Chemical structure of MG. | |
Environmental-oriented limiting laws have made it necessary to develop novel and non-toxic materials in a cost-effective and efficient pathway to provide safe and high quality ecosystem.9 Among various techniques, adsorption process using nanoscale adsorbents could be applied as a promising one to achieve the above-mentioned goals.10 MWCNTs with high surface area as well as large and wide dimension pores can be a good candidate for wastewater treatment. Therefore, in this work, MWCNTs were used as high performance adsorbent. One of the challenges and requirements in wastewater treatment is the development of novel methods for simultaneous determination and removal of multi-component pollutions from real systems. In such systems, seriously overlapped spectra corresponding to different dyes in their mixtures would cause the lack of accuracy and low repeatability in simultaneous determination of dyes content in the real samples.11,12
Therefore, it is necessary to resolve the spectra overlap and overcome these difficulties by using simple spectrophotometric method such as derivative spectrophotometry applied in this study.13–16 Central composite design (CCD) was applied under response surface methodology (RSM) to make a predictive model for the simultaneous determination and adsorption of toxic dyes of MG and MB on MWCNTs.
Various isotherm models were applied and their suitability for describing the experimental equilibrium data was investigated.
2. Experimental
2.1. Instruments and reagents
All chemicals including HCl, NaOH, MG, MB and MWCNTs were purchased from Merck, Darmstadt, Germany. The stock solution of each dye (100 mg L−1) was prepared by dissolving 25 mg of the dye in 250 mL double distilled water. It was used as working solution after suitable dilution. The pH/ion meter (model-827, Metrohm, Swiss), Biochrom UV-Vis spectrophotometer (UK), centrifuge (model T4, Iran), and ultrasonic bath (model Tecna 3, Italy) were used. MWCNT was characterized by transmission electron microscopy (JEM-100CX TEM) and X-ray photoelectron spectroscopy (XPS).
2.2. Central composite design (CCD)
Experimental design is efficiently used for the optimization of responses against variables involved to improve the process performance and minimize error17–19 while running least number of experiments. The significance of parameters including sonication time, amount of MWCNT as adsorbent, MG and MB concentration was investigated by applying the five-level CCD (Table 1) under response surface methodology (RSM) using the Design-Expert software version 7 (Stat-Ease, Minneapolis, USA). In general, the following model may apply to predict any response:20 |
 | (1) |
where Y is the predicted response; Xi's are the independent variables. The parameter β0 is the model constant; βi's are the linear coefficients; βij and βijk's are the coefficients of interaction terms and βii's are the quadratic coefficients.
Table 1 Experimental factors and levels in the central composite design
Factors |
Level |
Star point α = 2 |
Low (−1) |
Central (0) |
High (+1) |
−α |
+α |
X1 (time) |
0.5 |
2.5 |
4.5 |
1.5 |
3.5 |
X2 (adsorbent) |
0.005 |
0.02 |
0.035 |
0.125 |
0.275 |
X3 (MG concentration) |
5 |
10 |
15 |
7.5 |
12.5 |
X4 (MB concentration) |
5 |
10 |
15 |
7.5 |
12.5 |
2.3. Definition of the derivative spectrophotometric (DS)
Seriously overlapped spectra can be resolved by using derivative spectrophotometry.21,22 Subsequently, calibration curve with high accuracy and repeatability can be obtained. The desired order of derivative is selected and calibration curve for each component is constructed at a wavelength at which the contribution of that component is significant while others are negligible.
2.4. Definition of the PCA–ANN model
PCA and multi-layer ANN perceptron (one hidden layer) are set for the main ANN approach. Also the PCA technique is used to eliminate correlations between rows of the input data and to diminish the input data. In this work, a set of 54 by 341 data was converted to 54 by 4 matrix through PCA to obtain maximum variance in new space according to normalized value [0.1–1] intervals. MLP-AAN training was achieved by Levenberg–Marquardt with high speed convergence; while the ANN was configured by 0–30 neurons. The mean square error (MSE) is set as a criterion for the ANN performance.
Also, the normalized equation for mapping input data into [0.1–1] interval is obtained as:
|
 | (2) |
where matrix
X is input data; min(
X) and max(
X) denote minimum and maximum value of each input data columns, respectively. Then, the ANN outputs are transferred back into origin intervals by renormalizing through the following equation:
|
 | (3) |
After that, weights and biases as the ANN parameters are taken to predict the resultant dyes of experiment with no target. Finally, the removal percentage is available from the results. The ANN procedure is illustrated in Fig. 2.22
 |
| Fig. 2 The PCA–ANN procedure. | |
3. Results and discussion
3.1. Characterization of adsorbent
MWCNTs (10–20 nm) were oxidized and characterized by TEM, XPS and FTIR. TEM image (Fig. 3) confirms the external and internal diameters of MWCNTs to be 10–20 and 10.5 nm, respectively. IR spectrum of MWCNT shows some important characteristic vibrational frequencies at 3415.31 and 1573.63 corresponding to functional groups of OH and COOH, respectively (Fig. 4) confirmed by C 1s XPS spectrum of the nanotubes (Fig. 5).
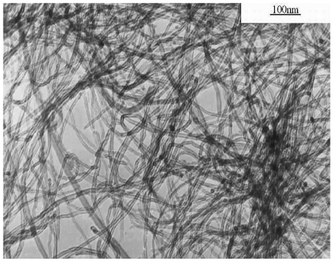 |
| Fig. 3 TEM image of MWCNTs. | |
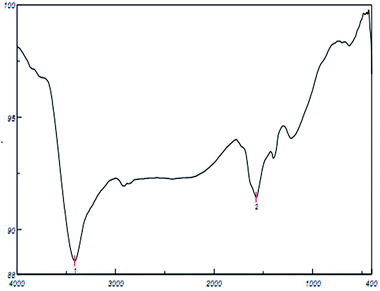 |
| Fig. 4 FTIR spectrum of MWCNTs. | |
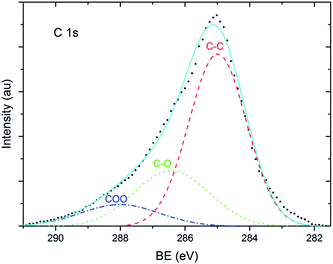 |
| Fig. 5 C 1s XPS spectrum of MWCNTs. | |
3.2. Optimization of pH
The effect of pH on simultaneous adsorption of MB and MG was studied in the pH range of 3.0–8.0. The maximum removal percentages of dyes corresponding to optimum adsorption capacity were obtained at pH 6 (Fig. 6). As known, the occurrence of optimum dyes removal at natural pH which corresponds to working solution of dyes permits to run experiments without any time consuming pH adjustment stage. This fact is promising and could make a great opportunity for the technology of dye removal from natural aqueous dye solutions.
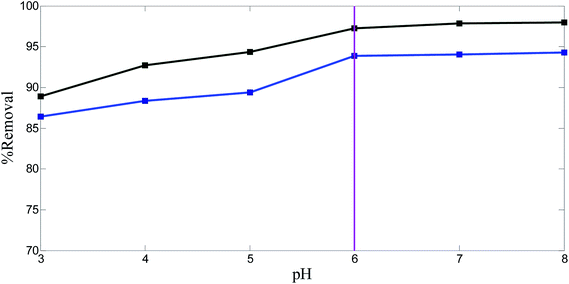 |
| Fig. 6 Effect of pH on the removal of MG and MB MWCNTs, contact time of 4.5 min, adsorbent dosage of 0.02 g in 50 mL and dye concentration of 20 mg L−1 for each dye. | |
3.3. The simultaneous analysis of MG and MB in binary mixtures by derivative spectrophotometric method
Fig. 7 shows the addition of absorbance spectra of single solutions of MG and MB (with a concentration of 7.5 mg L−1 for each dye) which is not fitted to the absorbance spectrum of their binary solution prepared from 7.5 mg L−1 of each dye. Therefore, their accurate determination in binary mixture is difficult and has low figures of merit. The derivatives of the spectra (e.g. see Fig. 8a and b for the first and second order derivatives, respectively) were obtained and the wavelength, at which one compound has zero absorbance and the other one contributes in the spectra was found. Thus the absorbance is attributed and proportional to the concentration of other species.23 The construction of calibration at such wavelength regarding criteria such as determination coefficient and recovery test can judge about suitability of each wavelength. After each differentiation step, a Savitzky–Golay smoothing procedure was applied to obtain a good level of signal-to-noise. Note that, the selected wavelength for MG and MB were 506.1 nm and 602.5 nm, respectively, in the second order derivative spectra (Fig. 8c and d).
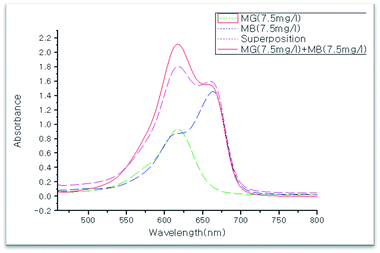 |
| Fig. 7 Zero order absorption spectra of MG and MB in single and binary solutions (initial dye concentration of 7.5 mg L−1 for each dye). | |
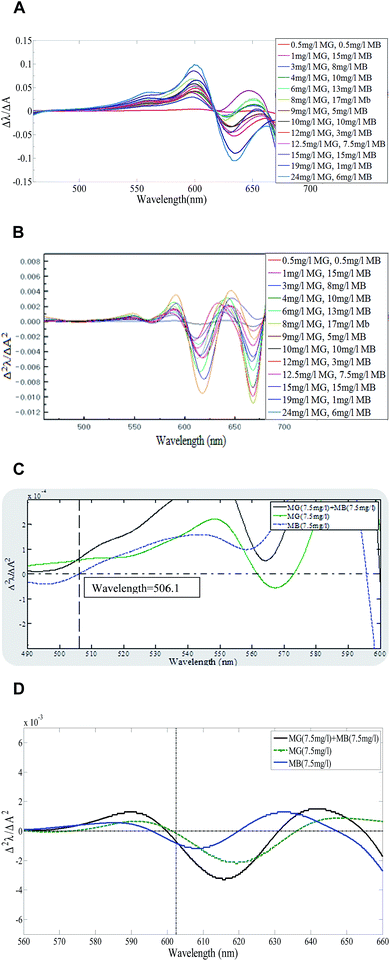 |
| Fig. 8 (a) First order derivative spectra of MG and MB in binary solution. (b) Second order derivative spectra of MG and MB in binary solution. (c) Second order derivative spectra of MG and MB in single and binary solutions (initial dye concentration of 7.5 mg L−1). (d) Second order derivative spectra of MG and MB in single and binary solutions (initial dye concentration of 7.5 mg L−1). | |
The amount of each dye was analyzed via the corresponding calibration curve (RMG2 = 0.9928 andRMB2 = 0.9934) at the above-mentioned wavelengths. The recoveries (%) and errors (%) between the theoretical (Ct) and measured (Cm) concentrations were calculated using eqn (4) and (5), respectively.
|
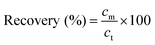 | (4) |
|
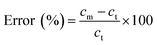 | (5) |
As seen, reasonably high recovery and low error show the applicability of this method for accurate analysis and determination of both dyes contents in their binary mixtures (see Table 2).
Table 2 Recovery and error percentages values for MG and MB in binary mixture obtained by SODS method
Theoretical (mg L−1) |
Measurement (mg L−1) |
Recovery (%) |
Error (%) |
CMG |
CMB |
CMG |
CMB |
MG |
MB |
MG |
MB |
7.5 |
12.5 |
7.94 |
12.9 |
105.86 |
102.64 |
5.87 |
3.2 |
10 |
10 |
8.89 |
10.01 |
98.9 |
102.1 |
−11.1 |
0.1 |
10 |
10 |
9.73 |
8.97 |
97.3 |
96.8 |
−2.7 |
−10.3 |
7.5 |
7.5 |
6.93 |
6.73 |
105.74 |
89.74 |
−7.6 |
−8.27 |
10 |
5 |
9.08 |
5.09 |
96.4 |
97.8 |
−9.9 |
1.8 |
7.5 |
7.5 |
7.67 |
6.99 |
94.27 |
103.87 |
2.27 |
−6.8 |
12.5 |
7.5 |
12.69 |
7.78 |
96 |
94.4 |
1.52 |
3.74 |
7.5 |
12.5 |
7.95 |
14.48 |
100.7 |
104.64 |
6 |
15.84 |
12.5 |
12.5 |
12.4 |
12.07 |
104.8 |
88.56 |
−0.8 |
−3.44 |
10 |
15 |
10.42 |
16.9 |
104.2 |
106 |
4.2 |
12.7 |
7.5 |
12.5 |
6.9 |
11.99 |
103.6 |
102.96 |
−8 |
−4.08 |
10 |
10 |
10.05 |
10.63 |
91.3 |
102.3 |
0.5 |
6.3 |
7.5 |
12.5 |
8.36 |
12.31 |
94.14 |
96.32 |
11.47 |
−1.52 |
7.5 |
7.5 |
7.74 |
6.52 |
105.07 |
89.87 |
3.2 |
−13.06 |
12.5 |
12.5 |
13.02 |
12.85 |
102.72 |
98.8 |
4.16 |
2.8 |
5 |
10 |
4.83 |
10.03 |
102.6 |
100.3 |
−3.4 |
0.3 |
10 |
10 |
9.63 |
9.01 |
106.3 |
103.4 |
−3.7 |
−9.9 |
10 |
10 |
10.33 |
10.02 |
102.3 |
90.2 |
3.3 |
0.2 |
12.5 |
12.5 |
12.69 |
12.97 |
104.24 |
95.76 |
1.52 |
3.76 |
10 |
10 |
9.49 |
10.18 |
99.7 |
101.8 |
−5.1 |
1.8 |
12.5 |
7.5 |
12.79 |
7.37 |
98.4 |
105.6 |
2.32 |
−1.7 |
10 |
10 |
10.14 |
10.09 |
96.4 |
91 |
1.4 |
0.9 |
10 |
10 |
10.65 |
9.75 |
98.5 |
101.5 |
6.5 |
−2.5 |
10 |
10 |
9.03 |
10.98 |
96.8 |
97.8 |
−9.7 |
9.8 |
12.5 |
7.5 |
12.3 |
7.69 |
102.64 |
94.94 |
−1.6 |
2.54 |
12.5 |
12.5 |
13.19 |
12.06 |
103.84 |
98.08 |
5.52 |
−3.52 |
15 |
10 |
14.89 |
1037 |
106.47 |
97.9 |
−0.7 |
3.7 |
10 |
10 |
9.44 |
10.32 |
98.8 |
93.2 |
−5.6 |
2.2 |
12.5 |
7.5 |
13.01 |
7.31 |
96 |
100.14 |
4.8 |
2.54 |
7.5 |
5 |
7.69 |
7.93 |
105.6 |
104.7 |
2.54 |
5.74 |
3.4. Central composite design (CCD)
Four independent variables ((X1(time), X2(dsorbent), X3(MG concentration), X4 (MB concentration)) were included in CCD with low, basal and high levels with respective coded values of −1, 0, +1 and the star points of +2 and −2 for +α and −α, respectively. The values of factors and responses are listed in Table 3.
Table 3 Central composite design (CCD)
Run |
Time (min) |
Adsorbent (g) |
Concentration of MG (mg L−1) |
Concentration of MB (mg L−1) |
Removal of MG (%) |
Removal of MB (%) |
1 |
1.5 |
0.0275 |
7.5 |
12.5 |
96.20 |
92.19 |
2 |
2.5 |
0.02 |
10 |
10 |
80.66 |
91.00 |
3 |
2.5 |
0.005 |
10 |
10 |
65.22 |
72.06 |
4 |
1.5 |
0.0125 |
7.5 |
7.5 |
79.57 |
87.26 |
5 |
2.5 |
0.02 |
10 |
5 |
98.45 |
79.85 |
6 |
1.5 |
0.0275 |
7.5 |
7.5 |
96.91 |
86.73 |
7 |
3.5 |
0.0275 |
12.5 |
7.5 |
95.62 |
87.04 |
8 |
3.5 |
0.0275 |
7.5 |
12.5 |
96.38 |
92.03 |
9 |
3.5 |
0.0125 |
12.5 |
12.5 |
11.52 |
68.98 |
10 |
2.5 |
0.02 |
10 |
15 |
74.83 |
90.82 |
11 |
1.5 |
0.0125 |
7.5 |
12.5 |
70.64 |
86.30 |
12 |
2.5 |
0.02 |
10 |
10 |
81.77 |
90.69 |
13 |
3.5 |
0.0125 |
7.5 |
12.5 |
74.15 |
89.65 |
14 |
3.5 |
0.0125 |
7.5 |
7.5 |
79.56 |
87.41 |
15 |
1.5 |
0.0125 |
12.5 |
12.5 |
10.84 |
33.33 |
16 |
2.5 |
0.02 |
5 |
10 |
89.74 |
89.95 |
17 |
2.5 |
0.02 |
10 |
10 |
13.90 |
90.61 |
18 |
2.5 |
0.02 |
10 |
10 |
88.59 |
90.30 |
19 |
3.5 |
0.0275 |
12.5 |
12.5 |
82.90 |
92.02 |
20 |
2.5 |
0.02 |
10 |
10 |
81.82 |
90.52 |
21 |
3.5 |
0.0125 |
12.5 |
7.5 |
67.78 |
89.29 |
22 |
0.5 |
0.02 |
10 |
10 |
59.81 |
90.03 |
23 |
2.5 |
0.02 |
10 |
10 |
89.08 |
90.04 |
24 |
2.5 |
0.035 |
10 |
10 |
97.16 |
89.93 |
25 |
1.5 |
0.0125 |
12.5 |
7.5 |
77.95 |
89.58 |
26 |
1.5 |
0.0275 |
12.5 |
12.5 |
82.19 |
92.39 |
27 |
2.5 |
0.02 |
15 |
10 |
87.09 |
91.12 |
28 |
4.5 |
0.02 |
10 |
10 |
88.48 |
90.57 |
29 |
1.5 |
0.0275 |
12.5 |
7.5 |
97.56 |
87.01 |
30 |
3.5 |
0.0275 |
7.5 |
7.5 |
98.52 |
86.71 |
Analysis of variance (ANOVA) was applied for the removal of MG (Table 4) and MB (Table 5). The ANOVA makes it possible to predict the individual, interaction and quadratic effects of all variables on the responses. A p-value less than 0.05 in the ANOVA indicates the statistical significance of a variable at 95% confidence level. After considering the significant terms in eqn (1), it reduces to the following predictive model for removal percentage (R%) of MG and MB, respectively.
|
RMG% = 87.74 + 7.99X2 − 5.9X4 + 6.45X2X3 + 7.85X2X4 − 8.58X3X4 + 6.41X2X3X4 + 8.97X12X2 − 8.51X12X3
| (6) |
|
RMB% = 86.18 + 5X2 + 4.39X2X3 + 6.03X2X4 − 4.89X3X4
| (7) |
Table 4 Analysis of variance for removal of MG
Source |
Sum of squares |
df |
Mean square |
F value |
p-Value Prob. > F |
|
Model |
12218.8 |
12 |
1018.233 |
31.47069 |
< 0.0001 |
|
A-time |
30.76458 |
1 |
30.76458 |
0.950846 |
0.3432 |
|
B-adsorbent |
510.085 |
1 |
510.085 |
15.76528 |
0.0010 |
|
C-MG |
3.496349 |
1 |
3.496349 |
0.108062 |
0.7464 |
|
D-MB |
278.8854 |
1 |
278.8854 |
8.619553 |
0.0092 |
|
BC |
664.7292 |
1 |
664.7292 |
20.54489 |
0.0003 |
|
BD |
985.5686 |
1 |
985.5686 |
30.46112 |
<0.0001 |
|
CD |
1176.899 |
1 |
1176.899 |
36.3746 |
<0.0001 |
|
B2 |
123.1256 |
1 |
123.1256 |
3.805462 |
0.0678 |
|
BCD |
657.7444 |
1 |
657.7444 |
20.32901 |
0.0003 |
|
A2B |
429.2194 |
1 |
429.2194 |
13.26595 |
0.0020 |
|
A2C |
386.4311 |
1 |
386.4311 |
11.94349 |
0.0030 |
|
A2D |
124.0369 |
1 |
124.0369 |
3.833629 |
0.0669 |
|
Residual |
550.0345 |
17 |
32.35497 |
|
|
|
Lack of fit |
461.7341 |
12 |
38.47785 |
2.178806 |
0.2007 |
Not significant |
Pure error |
88.30032 |
5 |
17.66006 |
|
|
|
Cor total |
12768.83 |
29 |
|
|
|
|
Table 5 Analysis of variance for removal of MB
Source |
Sum of squares |
df |
Mean square |
F value |
p-Value prob. > F |
|
Model |
2163.634 |
7 |
309.0905 |
4.337868 |
0.0037 |
|
A-Time |
64.72473 |
1 |
64.72473 |
0.908366 |
0.3509 |
|
B-Adsorbent |
600.4141 |
1 |
600.4141 |
8.426389 |
0.0083 |
|
C-MG |
183.129 |
1 |
183.129 |
2.570086 |
0.1232 |
|
D-MB |
43.23022 |
1 |
43.23022 |
0.606706 |
0.4443 |
|
BC |
308.2424 |
1 |
308.2424 |
4.325965 |
0.0494 |
|
BD |
581.1627 |
1 |
581.1627 |
8.15621 |
0.0092 |
|
CD |
382.7306 |
1 |
382.7306 |
5.371355 |
0.0302 |
|
Residual |
1567.588 |
22 |
71.25402 |
|
|
|
Lack of fit |
1567.038 |
17 |
92.17868 |
836.6602 |
<0.0001 |
Significant |
Pure error |
0.550873 |
5 |
0.110175 |
|
|
|
Cor total |
3731.222 |
29 |
|
|
|
|
P-values for lack of fit (LOF) for MG and MB are 0.2007 and 0.0001, respectively. The optimized values of sonication time, adsorbent mass, MG and MB concentration were found to be 2.6 min, 0.025 g, 14.6 and 10 mg L−1, respectively. At this condition, the removal percentages for MG and MB were predicted to be 93.85% and 90.03%, respectively, with desirability 1.00. The LOF for the model predicting the removal percentage of MB is significant which may imply the failure of the model. However, to test the validity of the predictions, three experiments were repeated at optimal conditions and the removal percentages for MG and MB were obtained to be 91.75 and 90.12, respectively, indicating good agreement with the predicted. Therefore, both models were validated.
3.5. The simultaneous analysis of MG and MB by PCA–ANN
The simultaneous analysis of MG and MB is performed by PCA–ANN model. It was seen (Table 6) that the use of 8 neurons leads to best final outcomes. By dividing a MIMO†–ANN into some MISO‡–ANN, the networks are trained to provide desired performance. So the parameters Wi1, bi1,Wi2 and bi2 (i = 1, 2) were set as Table 6 and 7. Finally, the overall removal percentages of MG and MB are calculated from predicting their concentrations.
Table 6 Tuned weight and bias for hidden and output layer for MB
W1 |
W2 |
b1 |
b2 |
−18.29552 |
12.43589 |
−3.77786 |
18.43532 |
6.23529 |
0.7253 |
−16.12921 |
9.98686 |
−3.06175 |
36.10166 |
5.97259 |
−13.69073 |
7.27125 |
−2.30438 |
17.82432 |
5.68168 |
−36.18205 |
130.52836 |
68.59978 |
0.03371 |
9.91369 |
|
5.15683 |
−2.37396 |
−7.73911 |
−1.25341 |
4.66208 |
1.84681 |
2.98850 |
−10.76078 |
0.98113 |
5.46931 |
−10.67629 |
7.39008 |
−7.15177 |
−0.12935 |
4.8884 |
−58.29830 |
−16.48420 |
85.01243 |
0.06106 |
24.31207 |
Table 7 Tuned weight and bias for hidden and output layer for MG
W1 |
W2 |
b1 |
b2 |
14.04157 |
−19.07305 |
−1.67141 |
32.30820 |
4.01607 |
0.11555 |
−2.99004 |
23.43434 |
0.0272 |
51.48711 |
−10.9942 |
3.85957 |
4.23926 |
0.43602 |
0.93353 |
−4.66861 |
2.80566 |
23.01649 |
−0.04299 |
52.3291 |
10.875 |
|
14.16208 |
−19.24329 |
−1.68804 |
−31.8603 |
4.05448 |
326.14317 |
−34.70597 |
47.21624 |
0.19873 |
−166.2270 |
−181.6341 |
171.98565 |
−179.2723 |
0.02758 |
65.75084 |
−29.6628 |
−9.92741 |
22.29310 |
−0.12011 |
−2.12567 |
As stated before, 54 experiments are considered to train ANN including 70 percent as training data and the remaining as test data. Also, the train and test data are selected randomly. Two activation functions for hidden and output layers are considered as hyperbolic tangent sigmoid and linear, respectively. The number of epochs is set to 1000 iterations. The networks parameters including the coefficients of determination and mean square error (R2 and MSE) generally give useful knowledge about the applicability of network for repeatable and accurate prediction of real behavior of system:
|
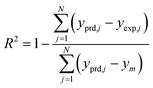 | (8) |
|
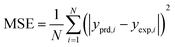 | (9) |
where,
N is the number of experiment,
yprd,i is the output of PCA–ANN and
yexp,i is the output of experiment.
The optimal neuron is obtained through try and error by applying various neurons to the ANN and investigating R2 and MSE. The R2 and MSE values imply that the use of 8 neurons is the best. According to the designed ANNs (Fig. 9 and 10), the R2 values of 0.9946 and 0.9996 are obtained for MG and MB training, respectively, while the MSE values of 2.29 × 10−04 and 1.6162 are obtained for MG and MB, respectively.
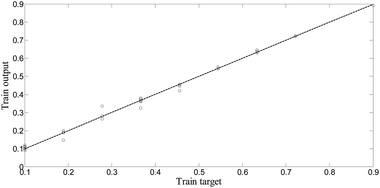 |
| Fig. 9 Performance of the MISO PCA–ANNs for MG. | |
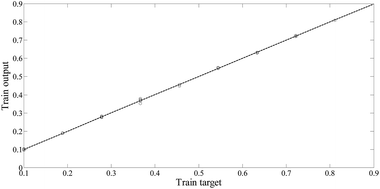 |
| Fig. 10 Performance of the MISO PCA–ANNs for MB. | |
According to R2 and MSE values, the ANN performance is good enough to use it for predicting the designed experiments by considering four factors, namely initial MG concentration, initial MB concentration, adsorbent dosage (g) and sonication time (min). Table 8 is presented to show the predicted values of two MISO ANNs.
Table 8 The individual and total adsorption equilibrium uptake and equilibrium concentration for MG and MB in binary mixture obtained by PCA–ANN model
Run |
Time (min) |
Adsorbent (g) |
Concentration of MG (mg L−1) |
Concentration of MB (mg L−1) |
Removal of MG (%) |
Removal of MB (%) |
1 |
1.5 |
0.028 |
7.5 |
12.5 |
93.02 |
90.44 |
2 |
2.5 |
0.02 |
10 |
10 |
73.69 |
86.83 |
3 |
2.5 |
0.005 |
10 |
10 |
99.49 |
92.87 |
4 |
1.5 |
0.013 |
7.5 |
7.5 |
89.82 |
91.39 |
5 |
2.5 |
0.02 |
10 |
5 |
98.19 |
85.24 |
6 |
1.5 |
0.028 |
7.5 |
7.5 |
97.59 |
90.16 |
7 |
3.5 |
0.028 |
12.5 |
7.5 |
95.81 |
84.07 |
8 |
3.5 |
0.028 |
7.5 |
12.5 |
93.02 |
90.44 |
9 |
3.5 |
0.013 |
12.5 |
12.5 |
92.42 |
94.29 |
10 |
2.5 |
0.02 |
10 |
15 |
73.69 |
95.69 |
11 |
1.5 |
0.013 |
7.5 |
12.5 |
64.93 |
94.83 |
12 |
2.5 |
0.02 |
10 |
10 |
73.69 |
93.56 |
13 |
3.5 |
0.013 |
7.5 |
12.5 |
64.93 |
94.83 |
14 |
3.5 |
0.013 |
7.5 |
7.5 |
89.46 |
91.39 |
15 |
1.5 |
0.013 |
12.5 |
12.5 |
88.70 |
94.29 |
16 |
2.5 |
0.02 |
5 |
10 |
89.54 |
88.05 |
17 |
2.5 |
0.02 |
10 |
10 |
73.69 |
86.83 |
18 |
2.5 |
0.02 |
10 |
10 |
73.69 |
86.83 |
19 |
3.5 |
0.028 |
12.5 |
12.5 |
98.99 |
94.83 |
20 |
2.5 |
0.02 |
10 |
10 |
73.69 |
93.54 |
21 |
3.5 |
0.013 |
12.5 |
7.5 |
78.95 |
91.39 |
22 |
0.5 |
0.02 |
10 |
10 |
73.69 |
93.54 |
23 |
2.5 |
0.02 |
10 |
10 |
73.69 |
93.56 |
24 |
2.5 |
0.035 |
10 |
10 |
98.74 |
92.62 |
25 |
1.5 |
0.013 |
12.5 |
7.5 |
78.95 |
91.39 |
26 |
1.5 |
0.028 |
12.5 |
12.5 |
78.95 |
94.83 |
27 |
2.5 |
0.02 |
15 |
10 |
94.88 |
93.54 |
28 |
4.5 |
0.02 |
10 |
10 |
92.36 |
86.83 |
29 |
1.5 |
0.028 |
12.5 |
7.5 |
93.89 |
82.44 |
30 |
3.5 |
0.028 |
7.5 |
7.5 |
97.59 |
90.16 |
3.6. Application of multi-component adsorption modeling of equilibrium data
Common isotherm models such as Langmuir, Freundlich and Temkin are generally applied to discuss the equilibrium characteristics of the adsorption process.24 The adsorptions of MG and MB onto MWCNTs in their binary systems were studied at different initial MG and MB concentrations in the range of 8.6 to 16.6 mg L−1 and 4 to 12 mg L−1, respectively, at two different amounts of adsorbent at optimal time (2.6 min). The constant parameters of different isotherms investigated for this adsorption process and the correlation coefficient (R2) are summarized in Table 9.
Table 9 Isotherm parameters for the MG and MB adsorption onto MWCNTs at optimal conditions
Isotherm |
Parameters |
Value of parameters |
0.015 |
0.025 |
MG |
MB |
MG |
MB |
Langmuir |
Qm (mg g−1) |
16.29 |
32.79 |
15.94 |
16.08 |
Ka (L mg−1) |
1.26 |
2.52 |
21.05 |
7.07 |
R2 |
0.9713 |
0.9612 |
0.9925 |
0.9858 |
Freundlich |
1/n |
0.1536 |
0.2976 |
0.1309 |
0.0155 |
KF (L mg−1) |
26.37 |
22.30 |
17.94 |
13.29 |
R2 |
0.7095 |
0.4894 |
0.9246 |
0.0025 |
Temkin |
Bl |
3.2861 |
6.2127 |
2.5943 |
0.0375 |
KT (L mg−1) |
2921.9 |
43.38 |
9992.27 |
370.77 |
R2 |
0.7646 |
0.5673 |
0.9164 |
1 × 10−4 |
Based on the linear form of Langmuir isotherm model (according to Table 9), the values of Ka (the Langmuir adsorption constant (L mg−1)) and Qm (theoretical maximum monolayer adsorption capacity (mg g−1) were obtained from the intercept and slope of the plot of Ce/qe versus Ce, respectively.
The Freundlich isotherm model can be expressed in linear form (Table 9). The values of KF (L mg−1) and 1/n are determined from the intercept and slope of linear plot of log(qe) versus log(Ce). The high correlation coefficient of Langmuir model at various conditions shows its applicability for the interpretation of experimental equilibrium data.25 The heat of the adsorption and the adsorbent–adsorbate interaction were evaluated by using Temkin isotherm model.26 B is the Temkin constant related to heat of the adsorption (J mol−1), T is the absolute temperature (K), R is the universal gas constant (8.314 J mol−1 K−1), KT is the equilibrium binding constant (L mg−1).
4. Conclusion
In this study, multiwalled carbon nanotubes (MWCNT) were oxidized and used for simultaneous adsorption of malachite green (MG) and methylene blue (MB). The adsorption of MG and MB dyes in their binary mixtures was investigated at batch system at optimized pH (6.0). The influences of experimental variables on the removal percentages of the dyes were investigated by experimental design methodology. First, the competitive adsorption of MB and MG was studied following evaluation of both dyes content using second order derivative spectrophotometry (SODS). The optimum values of initial MG and MB concentrations, MWCNT mass (g) and sonication time (min) were found to be 14.6 and 10 mg L−1, 0.025 g and 2.6 min, respectively, at which the removal percentages of the dyes were maximum.
In the second part, PCA–ANN model as an effective method was applied for the simultaneous prediction of the MG and MB concentrations. The suitability and applicability of the Langmuir model was presented by fitting the experimental equilibrium data to various isotherm models.
The advantage of the process is its high removal performance in very short time using small amount of MWCNT.
Acknowledgements
The authors express their appreciation to the Graduate School and Research Council of the University of Yasouj for financial support of this work.
References
- M. Rahim Nami, M. Janghorban and M. Damadam, Aerosp. Sci. Technol., 2015, 41, 7–15 CrossRef PubMed.
- M. Ghaedi, M. Pakniat, Z. Mahmoudi, S. Hajati, R. Sahraei and A. Daneshfar, Spectrochim. Acta, Part A, 2014, 123, 402 CrossRef CAS PubMed.
- M. Roosta, M. Ghaedi, A. Daneshfar, R. Sahraei and A. Asghari, Ultrason. Sonochem., 2014, 21, 242 CrossRef CAS PubMed.
- S. Hajati, M. Ghaedi and S. Yaghoubi, J. Ind. Eng. Chem., 2015, 21, 760–767 CrossRef CAS PubMed.
- V. K. Gupta, A. Mittal, L. Krishnan and V. Gajbe, Sep. Purif. Technol., 2004, 40, 87–96 CrossRef CAS PubMed.
- S. J. Culp, L. R. Blankenship, D. F. Kusewitt, D. R. Doerge, L. T. Mulligan and F. A. Beland, Chem. Biol. Interact., 1999, 122, 153–170 CrossRef CAS.
- L. Papinutti, N. Mouso and F. Forchiassin, Enzyme Microb. Technol., 2006, 39, 848–853 CrossRef CAS PubMed.
- S. D. Khattri and M. K. Singh, Adsorpt. Sci. Technol., 1999, 17, 269–282 CAS.
- X. Chen and J. Y. Hu, Water, Air, Soil Pollut., 2010, 206, 251 CrossRef CAS.
- Y. Li, K. Sui, R. Liu, X. Zhaoa, Y. Zhang, H. Liang and Y. Xia, Energy Procedia, 2012, 16, 863 CrossRef CAS PubMed.
- S. Hajati, M. Ghaedi, B. Barazesh, F. Karimi, R. Sahraei, A. Daneshfar and A. Asghari, J. Ind. Eng. Chem., 2013, 19, 227–233 CrossRef PubMed.
- S. Hajati, M. Ghaedi, F. Karimi, B. Barazesh, R. Sahraei and A. Daneshfar, J. Ind. Eng. Chem., 2014, 20, 564–571 CrossRef CAS PubMed.
- S. Hajati, M. Ghaedi, B. Barazesh, F. Karimi, R. Sahraei, A. Daneshfar and A. Asghari, J. Ind. Eng. Chem., 2014, 20, 2421–2427 CrossRef CAS PubMed.
- C. B. Ojeda and F. S. Rojas, Anal. Chim. Acta, 2004, 518, 1 CrossRef PubMed.
- S. Sayar and Y. Ozdemir, Food Chem., 1998, 61, 367 CrossRef CAS.
- J. Karpinska, Talanta, 2004, 64, 801 CrossRef CAS PubMed.
- M. Ghaedi, H. Mazaheri, S. Khodadoust, S. Hajati and M. K. Purkait, Spectrochim. Acta, Part A, 2015, 135, 479–490 CrossRef CAS PubMed.
- M. Ghaedi, A. Shahamiri, B. Mirtamizdoust, S. Hajati and F. Taghizadeh, Spectrochim. Acta, Part A, 2015, 138, 878–884 CrossRef CAS PubMed.
- A. Asfaram, M. Ghaedi, S. Hajati, A. Goudarzi and A. A. Bazrafshan, Spectrochim. Acta, Part A, 2015, 145, 203–212 CrossRef CAS PubMed.
- M. Ghaedi, S. Hajjati, Z. Mahmudi, I. Tyagi, S. Agarwal, A. Maity and V. K. Gupta, Chem. Eng. J., 2015, 268, 28–37 CrossRef CAS PubMed.
- M. Turabik, J. Hazard. Mater., 2008, 158, 52 CrossRef CAS PubMed.
- N. Zeinali, M. Ghaedi and G. Shafie, J. Ind. Eng. Chem., 2014, 20, 3550–3558 CrossRef CAS PubMed.
- M. Turabik, J. Hazard. Mater., 2008, 158, 52–64 CrossRef CAS PubMed.
- M. Ghaedi, S. Zamani Amirabad, F. Marahel, S. Nasiri Kokhdan, R. Sahraei and A. Daneshfar, Spectrochim. Acta, Part A, 2011, 83, 46–51 CrossRef CAS PubMed.
- G. McKay, Chem. Eng. J., 1983, 27, 187 CrossRef CAS.
- M. R. Samarghandi, M. Hadi, S. Moayedi and F. Barjasteh Askari, Iran. J. Environ. Health Sci. Eng., 2009, 6, 285–294 CAS.
Footnotes |
† Multi input multi output. |
‡ Multi input single output. |
|
This journal is © The Royal Society of Chemistry 2015 |
Click here to see how this site uses Cookies. View our privacy policy here.