DOI:
10.1039/C5IB00019J
(Paper)
Integr. Biol., 2015,
7, 513-524
High-throughput protease activity cytometry reveals dose-dependent heterogeneity in PMA-mediated ADAM17 activation†
Received
23rd January 2015
, Accepted 19th March 2015
First published on 23rd March 2015
Abstract
As key components of autocrine signaling, pericellular proteases, a disintegrin and metalloproteinases (ADAMs) in particular, are known to impact the microenvironment of individual cells and have significant implications in various pathological situations including cancer, inflammatory and vascular diseases. There is great incentive to develop a high-throughput platform for single-cell measurement of pericellular protease activity, as it is essential for studying the heterogeneity of protease response and the corresponding cell behavioral consequences. In this work, we developed a microfluidic platform to simultaneously monitor protease activity of many single cells in a time-dependent manner. This platform isolates individual microwells rapidly on demand and thus allows single-cell activity measurement of both cell-surface and secreted proteases by confining individual cells with diffusive FRET-based substrates. With this platform, we observed dose-dependent heterogeneous protease activation of HepG2 cells treated with phorbol 12-myristate 13-acetate (PMA). To study the temporal behavior of PMA-induced protease response, we monitored the pericellular protease activity of the same single cells during three different time periods and revealed the diversity in the dynamic patterns of single-cell protease activity profile upon PMA stimulation. The unique temporal information of single-cell protease response can help unveil the complicated functional role of pericellular proteases.
Insight, innovation, integration
Pericellular proteases, including both the membrane-bound ADAMs and the secretory MMPs, are important regulators of cell–microenvironment interactions. Cell-to-cell variation in protease activities could lead to diverse cellular behavior in response to other stimuli, highlighting the need in studying the heterogeneity of protease response and the corresponding cell behavioral consequences. In this work, we present a high-throughput microfluidic platform with capability to monitor single-cell pericellular protease activity. We demonstrate that single-cell protease activation upon drug stimulation could be heterogeneous not only in terms of activity magnitude but more surprisingly with respect to temporal profile – with cells exhibiting transient activity increase asynchronously. Our platform hence offers prospect for studying the roles of pericellular protease activities in governing cell behaviors in a context-dependent manner.
|
Introduction
Single-cell analysis has recently received wide attention as the importance of cellular heterogeneity has been increasingly recognized in many biological processes. While the population-based analysis has the advantage in averaging out the stochastic noises to highlight the causal interaction, it also masks the signals from functionally important subpopulations. The presence of those subpopulations is especially evident in cases of leukemia and solid tumors, where rare subpopulation, termed as “cancer stem cells”, with different proliferative and differentiative capacities from the bulk cancer cells have been identified and shown to play an important role in tumorigenesis and response to cancer therapy.4–7 Moreover, recent studies of genetically homogenous populations revealed that non-genetic variability, such as fluctuations of intracellular biochemical reaction, could lead to significantly diverse responsiveness to drugs and stimuli,8–12 highlighting the need to study the context-dependent cell fate decision process at the resolution of individual cells.
A disintegrin and metalloproteinases (ADAMs), a family of transmembrane proteins with peptide cleavage activities, have been shown to be the principal mediators of protein ectodomain shedding on the cell surface.13 Together with the closely-related matrix metalloproteinases (MMPs), ADAMs process and cleave hundreds of proteins including cytokines, receptors, growth factors and adhesion molecules and hence regulate many key cell signaling pathways via the modulation of the cellular microenvironment.14–16 There is increasing evidence to support the significant contributions of ADAMs in many physiological and pathological processes, ranging from multi-cellular organism development, wound healing to tumorigenesis, and thus ADAMs have been recognized as potential therapeutic targets in various diseases.1–3 The most established role of ADAMs, especially ADAM17 and ADAM10, is in cancer formation and progression,17 where the ADAM-mediated shedding of EGF family members is associated with increased cell proliferation, migration and survival.13 A recent study revealed that the autocrine signaling via protease-mediated EGF ligand shedding could stimulate the directed migration of individual human mammary epithelial cells (HMECs) without affecting their close neighborhood.18 The studies suggested that variability in single-cell protease activity could lead to diverse intracellular kinase activation profiles or cell migration patterns in response to the same stimuli, which might contribute to the resistance development against cancer therapies. Indeed, on one hand, the active ADAMs on the cell surface are generally considered to promote malignancy since they activate the growth factor ligands via proteolysis, and selective inhibitors against ADAM17 in particular have been shown to restore the sensitivity of gefitinib resistant non-small cell lung cancer (NSCLC).19 On the other hand, ADAMs could also shed the growth factor receptors from the cell surface and researchers have found that the receptor accumulation resulting from ADAM inhibition could enhance the activation of compensatory signaling pathway involved in the drug resistance development of endometriosis.20 Therefore, there is an emerging need in studying the heterogeneity of protease response of individual cancer cells and its cell behavioral consequences. The first step towards any relevant biological study is to develop an appropriate technique for single-cell measurement of pericellular protease functional characteristics.
Unlike MMPs, ADAMs are primarily located at the cell membrane and function in the pericellular space. Beside the multiple post-translational modifications and other intracellular regulatory mechanisms mediating the proteolytic activity of ADAMs, the local balance between active ADAMs and their physiological inhibitors in the extracellular environment also determines the actual function of those enzymes.21–23 Moreover, one recent study on endometriosis discovered a counter-intuitive decrease in both MMP-2 and ADAM-9 protease activities in the presence of reduced concentration of TIMP-4 protease inhibitor,24 further suggesting the catalytic activity to be a better surrogate marker for the ADAM functionality than the protein expression. Pericellular activity measurement of proteases primarily relied on various fluorescent reporter systems that generated fluorescence upon reaction with proteases, and the key issue of single-cell measurement is to constrain the readout to individual cells. Many existing methodologies utilize the translocation of fluorescence generating systems into the cytoplasm to enable single cell detection25–28 and significant throughput has been achieved when conjugated with flow cytometry. But caution must be taken as the translocation of extensive amount of exogenous molecules into the cytoplasm could potentially interfere with the intracellular singling events. Another big category of single-cell protease activity measurement in pericellular space is based on dye quenched (DQ) extracellular matrix (ECM) proteins.29,30 Coupled with advanced live-cell imaging system, DQ-ECM substrates have been shown to be useful in monitoring the spatio-temporal proteolysis events associated with cell migration and cell–cell interaction.30,31 Despite the benefits of DQ-ECM systems, an important drawback is that the DQ-ECM approach for single-cell pericellular protease measurement not only requires prior nuclear labeling that is known to interfere with cellular processes,32 but also imposes high demands on the imaging setup in many aspects including cell tracking, data memory, and spatial and temporal resolution of measurements. Nevertheless, the signaling response associated with protease-mediated shedding could be very rapid and dynamic.33 For example, as a typical downstream signaling process of cell surface receptors' binding to their ligands, early responses like calcium flux could happen within several seconds to minutes, while the subsequent intracellular kinase reaction cascade and transcriptional changes might take minutes to hours to occur. Therefore, to reveal the underlying regulation mechanisms associated with pericellular protease function, it is valuable to develop a novel methodology for single-cell protease activity measurement. An ideal measurement method would not only work in higher time resolution at considerable throughput, but also be compatible with conventional activity/concentration measurements and cell culture techniques, with minimal preparation and perturbation. This is especially important considering that the major goal of single-cell assays is to compare and contrast the signals at the bulk and single-cell levels, to elucidate both the role of critical subpopulations and any emergent population behaviors.
Microfluidics systems, which have been increasingly recognized as a useful tool in biological studies, offer the tremendous advantages in single-cell analysis.34,35 Until now, many different kinds of microfluidic chips have been developed to allow the manipulation and analysis of cells within the miniaturized devices in a controlled and reproducible way. Diverse single-cell assays against various molecular properties such as cellular transcriptome and secretory profile, or biophysical properties such as deformability and density, have also been realized in microfluidic platforms.36,37 Particularly, isolating individual cells with microwell arrays or discrete microchambers has emerged as a popular and robust approach for microfluidic single-cell platform. With appropriate engineering, microwell-based systems hold generic applicability to versatile biological problems. Both mammalian cell culture38,39 and ELISA-like measurement of secretary molecules40,41 have been successfully demonstrated with microwell-based systems for high-throughput single-cell study.
In this work, we developed a flexible yet robust microfluidic approach of multiwell confinement for single-cell measurement of pericellular protease activity. We took advantage of a microfluidic platform with valving function42 to control the molecular transport of individual microwells and also to confine the fluorescence readout signal from each cells for higher detection sensitivity. Since our platform has very little requirement on sensing substrates, various commercially available FRET-based substrates with high specificity against certain protease(s) could be used in the same manner as corresponding bulk assays. Potential multiplexed protease activity profiling is possible when assayed with panels of moderately specific FRET-based protease substrates with different fluorescence spectra.43 As a proof-of-concept, we demonstrated the capability of single-cell protease activity measurement using adherent human hepatocellular carcinoma cell line, HepG2, and studied its ADAM17 protease response mediated by PMA, a potent inducer of inflammation. Furthermore, since the microfluidic platform allows us to replace the medium within microwells easily and rapidly, we are able to monitor the temporal evolution of pericellular protease response of the same single cell. Results derived from our temporal protease activity profiling reveal that the extracellular protease activity profile could have diverse temporal dynamic patterns at the single-cell level. Moreover, the data indicates that the typical analogue dose–response relationship observed at the bulk level might have root in the dose-dependent effect of stimulant on single cells' signaling dynamics. Although the physiological significance of the asynchronous single-cell protease response is unclear, our results open up the possibility that heterogeneous protease response may have an impact on the dynamic interaction of the protease-mediated autocrine-signaling network, which may result in diverse cell fate decisions.
Results and discussion
Device design
Many conventional bulk cell measurements and some in vivo protease activity image techniques utilized synthetic FRET-based substrates that have high specificity against certain protease or protease class. Inspired by the popular microwell-based approach in microfluidic single-cell study, we established a multiwell platform to confine individual cells in discrete compartments during protease activity measurement with small molecule substrates with high diffusivity. Due to the enhanced signal readout via the confinement of the excessive diffusible substrates within microwells, the signal generated by pericellular protease-mediated reaction dominates the signal resulting from intracellular substrate cleavage events.
Compared to the existing methods, our approach has little requirement on substrate design and could be used for study of single-cell pericellular protease response in a time-dependent manner, as replenishing fresh substrate into the system allows for interrogation of same cells for multiple times. The additional temporal information of protease response might have an impact on cellular outcomes since the dynamics of downstream kinase signaling network has been shown to be an important component of the cell fate decision process.44,45 Moreover, our platform is compatible with other single-cell study technologies, including various live-cell reporter systems for intracellular signaling events, micro-engraving for molecular secretory profile40 and FISH assay for single-cell chromosome analysis.
Cell culture is performed with the bottom PDMS piece patterned with microwell array structure at the central region (Fig. 1A). We observed healthy HepG2 morphology and cell proliferation on the microwell array over a 1-week tissue culture (ESI,† Fig. S1). Compared to a closed system, cells grown in those microwells would experience similar oxygen gradient and nutrient condition as conventional tissue culture, which is important for comparison between single-cell assay results with those from conventional bulk assays performed on cells grown on standard tissue culture plate. While the flow chamber used in this work is a simple straight channel with branched microfluidic connection to reservoirs for synchronized delivery of fluid across channel width (Fig. 1B), the flow chamber could be modified to compose of a microfluidic generator46 when on-chip stimulation with a spatial gradient of drug is desired.
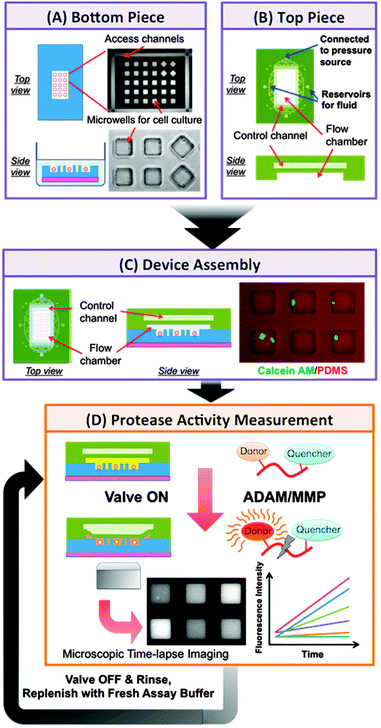 |
| Fig. 1 Schematics of microfluidic platform for single-cell protease activity measurement and the assay procedure. The platform is composed of two PDMS pieces, a bottom piece with microwell array pattern for cell culture (A) and a top piece with a 2-layer structure (B). Upon device assembly (C), the flow chamber is formed between the two pieces and is designed for injection of drug-containing buffer or reaction mixture with FRET-based protease substrate to the microwells. The top chamber of valve control layer allows the pneumatically actuation of flow channel ceiling to control the closing and opening of microwells. (D) After the introduction of assay buffer and the closing of microwells, the protease activity measurements were conducted via microscopic time-lapse imaging of fluorescence generating from the protease-specific substrate cleavage. For repeated measurements on the same cells, the microwell array was rinsed by introducing of fresh assay buffer without substrate at the end of each run. The rinsed cell-loaded array would then be used in the subsequent run of protease measurement after replenishing with fresh substrate-containing assay buffer. | |
Device characterizations
We first characterized the device performance with different amounts of ADAM17 recombinant protease mixed with FRET-based protease substrate. While current available substrates usually have cross-reactivity against closely related proteases, it is possible to distinguish between the proteases when the cleavage of multiple substrates by the same sample has been monitored.43 In this work, we only used one kind of substrate in all the measurements and the substrate chosen has been demonstrated to have very high catalytic efficiency against ADAM17 over others.43 As shown in Fig. 2, we observed a positive correlation between the concentration of recombinant ADAM17 in the system and the measured microwell activity index (AI), defined as the increasing rate of fluorescence intensity resulting from the substrate cleavage. Meanwhile, the background AI values derived from the fluorescence of plateau regions around microwells remained low for all the conditions tested. Furthermore, we also noticed that as a consequence of light scattering and non-uniform illumination within the observation window of microscope, the distributed range of microwell AI values increased with the average AI value under same condition, resulting in a coefficient of variation (CV) of 15–20%. Thus, we considered more than 30% deviation from the original AI value to be the real change in protease activity measured by our platform.
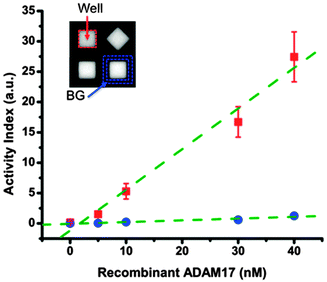 |
| Fig. 2 Device characterization with recombinant ADAM17. Sample mixture containing different concentrations of recombinant human ADAM17 protease and 10 μM FRET-based substrate was injected to the flow chamber of the assembled device. Fluorescence intensity was monitored for 36 min after the flow chamber was closed to isolated individual microwells. The activity index (AI), defined as the increasing rate of normalized fluorescence intensity was calculated (see ESI,† Fig. S2) and is shown here for an individual microwell area (marked by red dash line in the inset) or plateau region around the microwell (marked by blue dash line in the inset). One can observe a positive correlation between recombinant protease concentrations in the system and the AI values for microwells. Red squares: microwell area (Well); blue circles: plateau region around the microwell (BG); green dash lines: linear fit of AI vs. ADAM17 concentration. Error bars indicate standard deviations of individual microwell AI values from duplicate assays using 2 different devices (n > 1800 per condition). | |
PMA-induced protease activity of single HepG2 cells
As one of the most common causes of cancer death, liver cancer results in the death of around 598
000 people yearly due to the poor prognosis.47 Mounting evidence supports the association of poor prognosis with upregulation of many pro-inflammatory signals that can be cleaved and activated by ADAM17 protease, such as EGFR ligands and tumor necrosis factor alpha (TNF-α).48 Besides, the increased expression level of ADAM17 has also been observed in liver injury and liver cancer development.49 Given the importance of protease study in liver cancer, we tested the functionality of our platform with human hepatoma HepG2 cells challenged with PMA, a potent inducer of inflammation that has been shown to activate the ADAM17-dependent shedding of multiple substrates.13 As shown in Fig. 3, cell-containing microwells in DMSO control case displayed low normalized AI values similar to those of empty wells, suggesting few active ADAM17 present at the cell surface under basal condition. Meanwhile, PMA challenge resulted in a long tailed distribution of single cells' normalized AI values with a large fraction of single cells exhibiting high protease activity level (5–28% at [PMA] ≥ 0.2 μM). Notice that the threshold for high activity microwells defined in this work was chosen based on the normalized AI values of 0-cell wells and has been set to be normalized AI = 2, which was beyond 2 standard deviations away from the average value of 0-cell wells' normalized AI values. Moreover, we observed a dose-dependent relationship between the percentage of single cells with high protease activity and the PMA concentration (Fig. 3B). These results indicate that PMA treatment could increase the cell surface ADAM17 activity of HepG2 cells and this is consistent with previous findings where PMA is known to massively stimulate the shedding of several ADAM17 protease substrates, such as TNF-α and c-Met, in HepG2 cells.50 Interestingly, even under PMA concentration as high as 1 μM, there was still ∼72% of single cells displaying a very low, baseline protease activity, at least during the assay time (i.e. PMA treatment tine, tPMA = 15–51 min). The presence of those ‘non–responding’ cells reveals the inherent heterogeneity in protease response at the single-cell level and suggests that the escalating protease activity of a minority of fast-responding cells is the primary contributor of PMA-induced ADAM17 protease activation observed during short-term assay at the bulk scale.
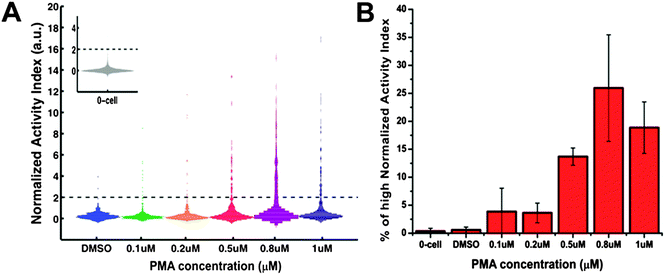 |
| Fig. 3 Heterogeneous protease response for HepG2 cells treated with DMSO or different concentrations of PMA (0.1, 0.2, 0.5, 0.8, 1 μM). The normalized activity index (AI) is shown for microwells containing single HepG2 cells. (A) Histogram of the normalized AI values for 1-cell wells (n > 300 per condition). The inset shows the histogram of the normalized AI values for 0-cell wells. Dark dash lines mark the place where normalized AI value = 2. (B) Percentage of 1-cell wells with high normalized AI values (>2). Error bars represent standard deviation of a triplicate using three different arrays. | |
Signaling components involved in PMA-mediated ADAM17 protease response
There are several distinct, potential mechanisms that could modulate the ADAM protease-mediated substrate cleavage. Cells' protease activity can be affected via the regulations on enzyme proteins' expression, maturation, trafficking to the cell surface and post-translational modifications that could prime the protease activity via the induction of protein conformational changes. Alternatively, modifications on the substrate proteins (i.e. cell surface receptors that are targets of ADAM-induced shedding) could also modulate the cleavage event without affecting the catalytic activity of the responsible protease. Currently, the exact mechanism of PMA-induced ADAM-dependent shedding in HepG2 cell system is still not fully clarified. PMA is a strong and pleiotropic stimulus.51–53 It is known to regulate the accessibility of the catalytic site of ADAM17 on the cell surface of mouse embryonic fibroblasts.54 PMA is also a potent activator of PKC kinases55 and Jurkat cells with PKC knockdown have been shown to be incapable of altering some substrate shedding in response to PMA challenging.56 Moreover, the activation of PKC could lead to the activation of ERK cascade.57 In HeLa cells, ERK activation is known to induce phosphorylation of the cytoplasmic domain of ADAM17 protein and accelerate its transportation to cell surface,58 where the active ADAM protease plays an important role in autocrine signaling. However, contradictory evidence also exists regarding the role of ERK kinases in PMA-mediated ADAM17 activation. In mouse monocytic cells59 and fibroblast cells,60 researchers observed no altered transport of ADAM17 to cell surface upon PMA challenging and found that the cytoplasmic domain of ADAM17 was not even required for PMA-induced ADAM17-dependent EGFR ligand shedding.
To investigate the mechanism of PMA-induced protease activation in HepG2 cells and also to show that the increase in protease activity observed by our assay was indeed a result of authentic biological event rather than artifacts, we pre-treated the cells with inhibitors against different components of signaling pathway and observed the corresponding changes in cell surface protease activity. As shown in Fig. 4, TAPI2, a broad-spectrum inhibitor against MMP proteases along with ADAM17, reduced the PMA-induced protease activation to the level of empty wells, confirming that the responsible protease in this case belonged to MMP/ADAM family. Additionally, PKC inhibition (Gö6983) clearly suppressed the single-cell protease activity to the level of the DMSO control case, suggesting a causal role of PKC kinases in the signaling network of PMA-mediated ADAM17 protease response. On the contrary, ERK inhibitor only slightly dampened the protease activation (p-value = 0.19, insignificant) and thus ERK kinases were unlikely to be essential for ADAM17 activation in PMA-treated HepG2 cells. Therefore, we confirmed that our system was capable of detecting changes in the extracellular protease activity of single cells upon drug challenge and there were inside-out signaling events through PKC kinases involved in the PMA-induced protease response for HepG2 cells.
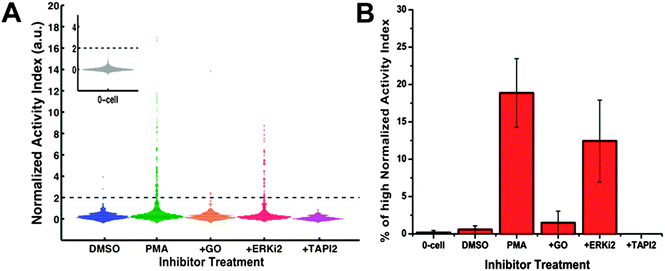 |
| Fig. 4 Inhibitors against different components of signaling pathway suppressed the PMA-induced protease activity increase in HepG2 cells. Serum-starved HepG2 cells were first incubated with different inhibitors and then subjected to protease activity measurement in the presence of both 1 μM PMA and inhibitors. For PKC kinase inhibition, HepG2 cells were pre-treated with 1 μM Gö6983 for 1.5 h. For ERK 1/2 kinase inhibition, HepG2 cells were exposed to 10 μM Erk inhibitor II FR108204 (ERKi2) for 4 h before protease assay. For protease inhibition, HepG2 cells were incubated with 20 μM TAPI2, a broad-spectrum inhibitor against several MMPs and ADAM17, for 4 h before measurement. (A) Histogram of the normalized AI values for 1-cell wells (n > 300 per condition). The inset shows the histogram of the normalized AI values for 0-cell wells. Dark dash lines mark the place where normalized AI value = 2. (B) Percentage of 1-cell wells with high normalized AI values (>2). Data for 0-cell wells with high normalized AI values is also shown. DMSO: control vehicle; PMA: stimulation with 1 μM PMA alone; +GO: PKC kinase inhibition along with 1 μM PMA challenge; +ERKi2: Erk kinase inhibition along with 1 μM PMA challenge; +TAPI2: broad-spectrum protease inhibition along with 1 μM PMA stimulation. Error bars represent standard deviation of a triplicate using three different arrays. | |
Snapshots for temporal response of PMA-mediated protease activation
Since it has been long recognized that cells make their decision based on not only the magnitude but also the dynamics of signaling events, we studied the temporal behavior of HepG2 protease response upon PMA challenging. To get the snapshots of protease response, we first performed a single run of protease activity measurement for cells in different microwell arrays with various PMA exposure time. On one hand, for treatment of 0.5 μM PMA (Fig. 5A and B), we observed a small upward shift in the distribution of 1-cell wells' normalized AI values over time. But the percentage of single cells with very high protease activity (i.e. normalized AI > 2) remained relative constant at ∼14% for PMA exposure time ranging from 0.25 h to 4 h. On the other hand, in the case of 1 μM PMA treatment (Fig. 5C and D), protease activation seemed extend to the majority of single cells as the PMA exposure time increased. We observed that the percentage of 1-cell wells with high AI values varied with the pre-treatment duration. The percentage of responding cells peaked around ∼50% when the pre-treatment time was 1.5 h, and decreased to ∼26% at pre-treatment time of 4 h. Comparing the results under these two different PMA concentrations, we found that stimulant dosage exerted an impact on the response properties of the single-cell population in terms of protease activation dynamics. That is, the lower stimulant dosage ([PMA] = 0.5 μM) generated a low but relatively constant level of protease activity over time, whereas the higher dosage ([PMA] = 1 μM) produced a varying but high protease activity signal among the single-cell population. Reasons for this dose-dependent impact on single-cell population might lie in the time-dependent behavior of individual single cells and thus require tracking of protease response for individual cells over time.
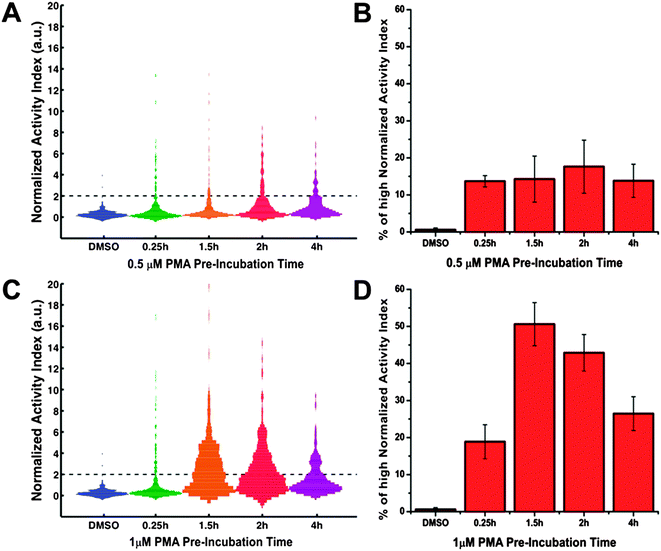 |
| Fig. 5 Temporal response of the PMA-mediated single-cell protease activity measured in 1-run assay. HepG2 cells were treated with either 0.5 μM (A, B) or 1 μM PMA (C, D) for various durations (0.25 h, 1.5 h, 2 h, 4 h) before subjected to protease activity measurement. Each cell-load arrays were assayed only once. (A, C) Histogram of the normalized AI values for 1-cell wells (n > 300 per condition). Dark dash lines mark the place where normalized AI value = 2. (B, D) Percentage of 1-cell wells with high normalized AI values (>2). Error bars represent standard deviation of a triplicate using three different arrays. | |
Tracking the protease temporal response of single cells
Many cell-signaling and transcriptional process show pulsatile, or even oscillatory, behaviors.33 Although the physiological significance of such oscillations in these systems is not fully understood, it could be another regulatory layer in which biological information can be encoded. Therefore, we further investigated the temporal behavior of individual cells by performing 3 sequential runs of measurements on the same cell-seeded array. As shown in Fig. 6, we observed various temporal patterns of the protease response for individual cells. In DMSO control case, the majority (∼95%) of the cell-containing microwells displayed low level of protease activity during the entire assay period of 3.2 hours (Fig. 6A) and there were little changes between runs (Fig. 6C and E). About 4% of DMSO-treated single cells displayed high protease activity in the 3rd run (Fig. 6B), potentially as a result of the cellular response to the stress induced by repeated measurements. In both PMA stimulation case of 0.5 μM and 1 μM, the fraction of the non-responding cells reduced to ∼60 ± 2% (no. 8 in Fig. 6E), while 15–20% of single cells exhibited a pulsatile activity profile (initial increase followed by decrease, no. 3 in Fig. 6E). There were also 8–10% of PMA-treated single cells with a delayed protease response (no. 7 in Fig. 6E), as they started to show high protease activity signal in the 3rd run of measurement, which corresponded to PMA treatment time: tPMA = 2.5–3.2 h. The impact of PMA dosage was most evident in the early protease response of single cells. As shown in Fig. 6B, higher dosage of PMA treatment resulted in more single cells with high activity in the 1st run of measurement. Meanwhile, Fig. 6E shows that most of these fast-responding cells experienced a decrease in protease activity at 2nd run of measurement and thus fell into the category of dynamic pattern no. 5 (3.8% for [PMA] = 0.5 μM vs. 10.2% for [PMA] = 1 μM; p-value = 0.21, insignificant).
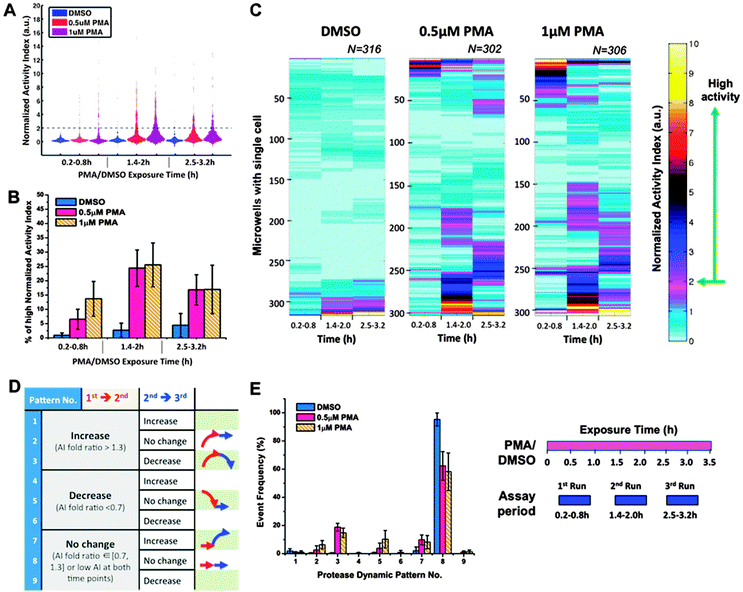 |
| Fig. 6 Heterogeneous temporal behavior of single-cell protease activity upon PMA challenging. Three sequential runs of protease activity measurement were performed with the same cell-loaded arrays for DMSO control case and PMA stimulation case (0.5 μM or 1 μM PMA), respectively. As illustrated in the experiment schedule shown on the lower right, the DMSO or PMA drug (magenta bar) was applied to cells at constant concentration for the entire experiment. The protease measurements (blue blocks) were conducted in sequential manners and thus corresponded to different drug exposure times. (A) Histogram of the normalized AI values for 1-cell wells (n > 300 per condition). Dark dash lines mark the place where normalized AI value = 2. (B) Percentage of 1-cell wells with high normalized AI values (>2). (C) Clustered heat maps for 1-cell wells' normalized AI under different conditions. In each heat map, there are three columns corresponding to the three different time periods of protease measurement and each row represents the protease activity of individual single cells (n > 300 per condition). (E) Distribution of the single-cell protease activity profile over different dynamic patterns. (D) Table for description of each dynamic pattern. Schematic arrowed profiles were also shown for the patterns with more than 5% single cells in at least one of the drug conditions. If the normalized AI values of single cells are less than 2 at both time points or changes in their AI values are less than 30% of the original AI values, we define them as “no change” in protease activity over time. Meanwhile, an “increase” or “decrease” in protease activity is defined for the rest of single cells depending on whether their AI values at the latter time point are 30% larger or smaller than the corresponding AI values at the earlier time point. Error bars represent standard deviation of a triplicate using three different arrays. | |
Although our assay provided limited time points for each single cell, we could gain a glimpse of how individual cells' protease response might change over time. Firstly, results from both 1-run and 3-run assays support asynchronous yet transient protease response at the single-cell level – that is, each single cell follows an increase-then-decrease protease activity profile upon PMA challenging. Secondly, higher stimulant concentration seems to favor more cells with earlier onset of protease activation, instead of modulating the maximum level of individual cells' protease activity. A similar behavior has been observed in the well-studied case of single cells' gene expression,61,62 where DNA enhancers had been demonstrated to augment the activation probability of single cell without affecting the strength of cellular activation at the individual cell level. Lastly, inspired by the insight that analogue dose–response at the population level could be a result of digital behavior of many individual players, we hypothesized that PMA could increase the probability of single cell being turned ON to go through the transient protease activation profile in a dose-dependent manner. Under this assumption, we came up with an explanation for the distinct dynamic properties of single-cell population observed in the 1-run assays. In the case of lower PMA concentration, the probability of single cells being turned ON might be low and thus there would always be enough cells for turning ON as most of the cells haven't gone through the protease activation process. As a result, one could observe a low but relative constant level of protease activity at the population level of single cells, since at any given time point of PMA treatment, there might be a small but relatively constant number of cells being initiated for protease activation. However, in the case of higher PMA concentration, most of the cells could be turned ON at the early stage due to the increased activation probability endowed by the high PMA concentration. This would result in a more synchronous, higher-strength but pulsatile protease activity at the population level. Obviously, higher time resolution measurements are needed to validate our hypothesis. Nevertheless, our microfluidic platform is able to provide unique temporal information of a large number of single cells utilizing the same biosensing modalities and without involving complex intracellular sensor engineering.25 Thus, the platform could serve as a generic drug-screening step for primary cells from individual patients.
Conclusions
Here we have developed a microfluidic platform for single-cell study of pericellular protease activity. In contrast to existing methodologies for single-cell pericellular protease measurement, we constrained the fluorescence readout of protease activity to individual cells by physical isolation of cells within each microwells using a pneumatically actuated lid. Due to the simplicity of our design, our platform works with various small molecule FRET-based protease substrates and is able to measure single-cell protease activity with meaningful time resolution (∼1.2 h). With the developed platform, we studied the PMA-induced single-cell protease response of HepG2 cells and showed, for the first time, that the extracellular protease activity of individual cells displayed diverse dynamic patterns despite the overall trend of population cell response during the same period. Moreover, our platform is compatible with many existing single-cell analysis methods probing other molecular events of single cells. A typical example would be to combine our platform for protease activity measurement with the micro-engraving method for molecule shedding from the cell surface. This combination of techniques is particularly valued in the study of protease-mediated cancer resistance development. Our platform therefore has the potential for studying the context-dependent role of pericellular protease activities in governing cell behaviors.
Experimental
Device fabrication
For the bottom piece of the microfluidic platform, arrays of microwells were made of polydimethylsiloxane polymer (PDMS, Sylard 184 Silicone Elastomer Kit, Dow Corning, USA) using standard soft lithography techniques from a SU8-patterned silicon master. Individual microwell was cuboid in shape with a volume of 1.89 nL (area of 100 × 100 μm2 with a depth of 189 μm), arranging into 5 × 6 blocks with well-to-well interval of 190 μm. The microwell dimensions described were empirically established for easy monitoring of cellular morphology and locomotion of adherent mammalian cells with 15–20 μm in diameter. The access channels between each block had the same depth and width as that of microwells, and were designed to facilitate the liquid convection across the microwell array during operation. Each microwell array containing 4 × 8 blocks of microwells (960 microwells per array) was patterned on the center area of a 1 mm-thick PDMS slab laying facing up on top of a 1.5′′ × 1.5′′ glass slide (VWR® Plan Micro Slides, VWR International LLC, USA), and used for cell culture.
The top piece of the microfluidic platform was also made of PDMS polymer and comprised of a flow chamber layer and a valve control layer. The flow chamber layer contained an 8 mm × 15 mm × 100 μm (width × length × depth) straight channel, both ends of which were connected to the inlet and outlet reservoirs via branching channels. The valve control layer is positioned directly on top of the flow chamber layer and formed a 15 mm × 8 mm × 200 μm straight channel via irreversible plasma bonding. This is where the pressure would be applied to control the closing and opening of the underneath flow chamber (Fig. 1).
Preparation of microwell arrays and cell culture
The PDMS microwell array on a glass slide was placed within a 35 mm × 10 mm tissue culture dish and treated with oxygen plasma (Harrick Plasma Cleaner/Sterilizer, Harrick Plasma, Inc., USA) for 1 min to sterilize the surface. To promote cell adhesion, plasma-treated microwell array was first incubated with 0.02 N sterile acetic acid containing 40 μg mL−1 rat collagen I (A1048301, Invitrogen, USA) at room temperature for 1 h, followed by washing with 1× PBS three times to remove unbound collagen molecules and residual acid. Then, suspensions of HepG2 cells (HB-8065, ATCC, USA) were deposited onto the surface of the microwell array at 1.2 × 105 cells per mL concentration in complete medium, which consists of Eagle's minimum essential medium (EMEM; 30-2003, ATCC, USA), 10% fetal bovine serum (16000, Invitrogen, USA), 50 U mL−1 of penicillin and 50 μg mL−1 of streptomycin (15070-063, Invitrogen, USA). Cells were allowed to settle down for 5–6 min before the surface of microwell array was gently washed with medium from the side to remove the cells in the access channels or outside microwells. The microwell array seeded with cells would then be submerged in complete medium and incubated at 37 °C with 5% CO2 for 6–8 h before being subjected to overnight serum starvation and assayed on the second day. Visual inspection of the cell-loaded microwell array by microscopy suggested that the sedimentation of cells into microwells followed a Poisson distribution and thus on average ∼25% of total microwells contained single cells.
Device assembly and operation
Prior to protease measurement, the top control piece of device would first be exposed to a 30 s plasma treatment for sterilization and then be aligned manually onto the bottom cell-loaded piece that is covered by serum-free medium. Then the two pieces would be clamped together under light compression between plates of a homemade assembly chamber and the entire assembly would then be mounted within a stage top incubator (Tokai Hit Co., Ltd, Japan) onto an inverted epifluorescence microscope (Olympus IX71, Olympus Inc., USA) equipped with a motorized stage (H117 ProScan™ motorized stage, Prior Scientific Inc., USA) and a 12-bit CCD camera (SensiCam QE, PCO, Germany). The whole assembly process takes 5 minutes and the humidified incubator was kept at 37 °C with 5% CO2 supply for live-cell imaging. Sterile assay buffer or washing buffer was loaded within a syringe and pumped through the flow chamber formed between the top and bottom piece at varying flow rates using a syringe pump (Harvard Apparatus PHD 2000, Harvard Apparatus Inc., USA). The valve control chamber of the top piece was filled with deionized water and its inlet reservoir was connected to a gas controller. With no external pressure applied to valve control chamber, the flow chamber was connected to reservoirs for buffer introduction and all microwells in the same array were exposed to the same fluidic environment. The flow chamber can be closed by applying 16 kPa to the valve control layer, and thus the valve membrane of control layer isolates the microwells underneath from each other to form discrete and closed compartments for protease activity measurement of individual microwells.
Device characterization with recombinant protease
Different concentrations of recombinant human ADAM17 protease (930-ADB-010, R&D Systems, Inc., USA) were mixed with serum-free medium (EMEM) containing 10 μM ADAM17-specific FRET-based substrate (PEPDAB010, BioZyme, Inc., USA). Immediately after the mixing, sample mixture was injected to the flow chamber of the assembled device at 200 μL min−1 for 1 min followed by 40 μL min−1 for 10 min. Subsequently, 16 kPa pressure was applied to valve control chamber to isolate the microwells underneath. Fluorescence intensity of each closed microwell was recorded at 2.5 min intervals for 15 frames using a 10× objective lens with the help of a motorized stage. The starting time for time-lapse fluorescence intensity recording was t = 14 ± 1 min after the mixing of substrates and recombinant enzyme.
Single live-cell protease activity assays
HepG2 cells seeded in collagen I-coated microwell arrays were cultured in serum-free medium (EMEM) overnight before assayed for protease activity. For some assays, cells were pre-treated with various inhibitors, including Gö6983 (G1918, Sigma-Aldrich, USA), ERK inhibitor II FR180204 (328010, EMD Millipore, Germany) and TAPI2 (SML0420, Sigma-Aldrich, USA). For all assays, cells were pre-loaded with viability dye, 2 μM CellTrace™ calcein violet AM (C34858, Invitrogen, USA), for 30 min prior the device assembly. Following the device assembly and mounting onto a stage top incubator (37 °C, 5% CO2, humidified), cells were imaged briefly for the localization and viability under microscope at multiply positions along z direction. Then, assay buffer consisting of 10 μM ADAM17-specific FRET-based substrate, PEPDAB010, along with either different concentrations of phorbol 12-myristate 13-acetate (PMA; P1585, Sigma-Aldrich, USA) or DMSO control in serum-free medium would be injected into the flow chamber at 200 μL min−1 for 1 min followed by 40 μL min−1 for 10 min. Then, cells in each microwells would be isolated by pressurizing the valve control chamber. The fluorescent intensity would be recorded at 2.5 min intervals for 36 min. At the end of the 1st assay, the cells in microwells were rinsed at 40 μL min−1 for 15 min using fresh washing buffer, which had the same composition as the assay buffer used in the 1st run of measurement but contained no FRET-based protease substrates. The rinsed cell-loaded array can then be used in subsequent run of protease measurement if applicable. Once all the runs of protease measurement were done and the residual substrates were washed away, serum-free medium containing 3 μM propidium iodide (P3566, Invitrogen, USA) and 0.8 μM Hoechst 33342 (H1399, Invitrogen, USA) were injected into the flow chamber at 40 μL min−1 for 10 min to stain the cells post-measurement. Fluorescent images under different wavelength channels were taken for cells within microwells at multiple depth positions and would be used for subsequent data analysis of cell number and post-assay survival. Then, the device would be disassembled and the bottom piece loaded with stained cells in microwells were rinsed with fresh medium and kept within tissue culture dish with complete medium in cell culture incubator for further culture. On the day following single-cell protease measurements, cells would be stained with 2 μM calcein AM (354216, BD Biosciences, USA) for 20 min before imaging with microscope for the day 2 survival.
Data analysis
Fluorescent images taken post-assay for cells stained with Hoechst 33342 and propidium iodide were scrutinized manually to count the cell number within individual microwells and to identify the location of each cells in the depth direction of microwell array. Images taken prior to the assay and on day 2 were also analyzed for cell survival across the entire assay. We excluded data from microwells with non-cell objects (dirt, impurity in coating solution, etc.) or cells dead by day 2 from further analysis. For each microwell array, we could get valid data from more than 180 1-cell wells and 100 2-cell wells. Moreover, to avoid confounding factors relevant to the varying depth locations of individual cells, we only considered the single cells remaining at the bottom of each microwells during the entire assay period into the final protease activity analysis, unless otherwise stated. Usually, we got 60–70% of single cells located at the bottom of microwells by the time of protease measurement.
We have developed a procedure for signal processing and normalization of the protease measurement. As illustrated in ESI,† Fig. S2, (i) the time-lapse raw images for the substrate cleavage were captured by fluorescence inverted microscope and then stabilized using Image Stabilizer plugin of Image J® software. Given the non-uniform illumination within observation window and the well-to-well interference due to light scattering, a normalization method was applied. (ii) Briefly, two templates were generated based on the raw images with the aid of MATLAB and defined the regions within each microwells and the background regions around the wells, respectively. (iii) The pixel intensity within each region was further sorted in ascending order and the extreme values at both ends would be discarded. The average value of the central ±20% pixel intensity was then considered as the intensity indicator for that region. In this manner, one can reduce the variance introduced by inaccurate microwell border identification and the presence of bright objects within microwell. (iv) For background regions, the average value became the normalized intensity. For microwell regions, the average value of each well region was further subtracted by the normalized intensity of its surrounding background region. Then, one can obtain time-lapse profile of normalized intensity for individual microwells. (v) We modeled the protease-mediated substrate cleavage as the classical Michaelis–Menten model, where the initial rate of cleavage is proportional to the concentration of active enzyme in the system with excessive substrate of nearly constant concentration. Therefore, we defined the protease activity index (AI) as the increasing rate (i.e. slope) of normalized fluorescence intensity and extracted the AI value for each region via robust linear least-squares fitting of the time-lapse normalized intensity profile. (vi) When dealing with different cell-loaded microwell arrays, we used the median AI value of empty microwells from each array as the reference value, to account for the spontaneous substrate cleavage occurred in the absent of cells within that particular array. We further calculated the normalized AI value by subtracting each microwell AI value with the reference value of the same array and used the resultant normalized AI value to evaluate the protease response of cells within different microwell arrays. Notice that all the histograms shown in this manuscript have been smoothened using MATLAB function ksdensity(
).m where the density estimation was based on a normal kernel function and the locations of kernel smoothing windows were robustly estimated via function histogram(
).m. Based on the normalized AI values of 0-cell wells, we also defined a threshold for high activity microwells to be normalized AI = 2, which was beyond 2 standard deviations away from the average value of 0-cell wells' normalized AI values. Percentage of high activity microwells derived from a given protease assay then provides an indicator to quantify the overall protease response of all the single cells measured during that particular assay. Any p-values shown were calculated based on Welch's t-test.
Abbreviations
ADAM | A disintegrin and metalloproteinase |
AI | Activity index |
CV | Coefficient of variation |
DMSO | Dimethyl sulfoxide |
DQ | Dye quenched |
ECM | Extracellular matrix |
EGFR | Epidermal growth factor receptor |
EMEM | Eagle's minimal essential medium |
ERK | Extracellular signal-regulated kinase |
FISH | Fluorescence in situ hybridization |
FRET | Fluorescence resonance energy transfer |
HMEC | Human mammary epithelial cell |
MMP | Matrix metalloproteinase |
NAI | Normalized activity index |
NSCLC | Non-small cell lung cancer |
TIMP | Tissue inhibitor of metalloproteinase |
TNF-α | Tumor necrosis factor alpha |
PDMS | Polydimethylsiloxane |
PKC | Protein kinase C |
PMA | Phorbol 12-myristate 13-acetate |
Acknowledgements
This work is supported by the Singapore-MIT Alliance for Research and Technology (SMART) Centre (BioSym IRG). This work is also supported by NIH Grant R01-CA096504.
References
- J. Arribas and C. Esselens, Curr. Pharm. Des., 2009, 15, 2319–2335 CrossRef CAS.
- M. J. Duffy, E. McKiernan, N. O'Donovan and P. M. McGowan, Clin. Chim. Acta, 2009, 403, 31–36 CrossRef CAS PubMed.
- M. J. Duffy, M. Mullooly, N. O'Donovan, S. Sukor, J. Crown, A. Pierce and P. M. McGowan, Clin. Proteomics, 2011, 8, 9 CrossRef CAS PubMed.
- D. Bonnet and J. E. Dick, Nat. Med., 1997, 3, 730–737 CrossRef CAS PubMed.
- S. K. Singh, I. D. Clarke, M. Terasaki, V. E. Bonn, C. Hawkins, J. Squire and P. B. Dirks, Cancer Res., 2003, 63, 5821–5828 CAS.
- C. A. O'Brien, A. Pollett, S. Gallinger and J. E. Dick, Nature, 2007, 445, 106–110 CrossRef PubMed.
- A. D. Boiko, O. V. Razorenova, M. van de Rijn, S. M. Swetter, D. L. Johnson, D. P. Ly, P. D. Butler, G. P. Yang, B. Joshua, M. J. Kaplan, M. T. Longaker and I. L. Weissman, Nature, 2010, 466, 133–137 CrossRef CAS PubMed.
- J. G. Albeck, J. M. Burke, B. B. Aldridge, M. Zhang, D. A. Lauffenburger and P. K. Sorger, Mol. Cell, 2008, 30, 11–25 CrossRef CAS PubMed.
- K. E. Gascoigne and S. S. Taylor, Cancer Cell, 2008, 14, 111–122 CrossRef CAS PubMed.
- S. L. Spencer, S. Gaudet, J. G. Albeck, J. M. Burke and P. K. Sorger, Nature, 2009, 459, 428–432 CrossRef CAS PubMed.
- J. D. Orth, Y. Tang, J. Shi, C. T. Loy, C. Amendt, C. Wilm, F. T. Zenke and T. J. Mitchison, Mol. Cancer Ther., 2008, 7, 3480–3489 CrossRef CAS PubMed.
- O. Feinerman, J. Veiga, J. R. Dorfman, R. N. Germain and G. Altan-Bonnet, Science, 2008, 321, 1081–1084 CrossRef CAS PubMed.
- C. P. Blobel, Nat. Rev. Mol. Cell Biol., 2005, 6, 32–43 CrossRef CAS PubMed.
- K. Kessenbrock, V. Plaks and Z. Werb, Cell, 2010, 141, 52–67 CrossRef CAS PubMed.
- M. Gooz, Crit. Rev. Biochem. Mol. Biol., 2010, 45, 146–169 CrossRef CAS PubMed.
- G. Murphy, Nat. Rev. Cancer, 2008, 8, 932–941 CrossRef PubMed.
- P. M. McGowan, B. M. Ryan, A. D. K. Hill, E. McDermott, N. O'Higgins and M. J. Duffy, Clin. Cancer Res., 2007, 13, 2335–2343 CrossRef CAS PubMed.
- G. Maheshwari, H. S. Wiley and D. A. Lauffenburger, J. Cell Biol., 2001, 155, 1123–1128 CrossRef CAS PubMed.
- B.-B. S. Zhou, M. Peyton, B. He, C. Liu, L. Girard, E. Caudler, Y. Lo, F. Baribaud, I. Mikami, N. Reguart, G. Yang, Y. Li, W. Yao, K. Vaddi, A. F. Gazdar, S. M. Friedman, D. M. Jablons, R. C. Newton, J. S. Fridman, J. D. Minna and P. A. Scherle, Cancer Cell, 2006, 10, 39–50 CrossRef CAS PubMed.
- M. A. Miller, A. S. Meyer, M. T. Beste, Z. Lasisi, S. Reddy, K. W. Jeng, C.-H. Chen, J. Han, K. Isaacson, L. G. Griffith and D. A. Lauffenburger, Proc. Natl. Acad. Sci. U. S. A., 2013, 110, E2074–E2083 CrossRef CAS PubMed.
- K. Horiuchi, S. Le Gall, M. Schulte, T. Yamaguchi, K. Reiss, G. Murphy, Y. Toyama, D. Hartmann, P. Saftig and C. P. Blobel, Mol. Biol. Cell, 2007, 18, 176–188 CrossRef CAS PubMed.
- M. H. Pillinger, N. Marjanovic, S.-Y. Kim, J. U. Scher, P. Izmirly, S. Tolani, V. Dinsell, Y.-C. Lee, M. J. Blaser and S. B. Abramson, J. Biol. Chem., 2005, 280, 9973–9979 CrossRef CAS PubMed.
- P. Xu and R. Derynck, Mol. Cell, 2010, 37, 551–566 CrossRef CAS PubMed.
- C.-H. Chen, M. A. Miller, A. Sarkar, M. T. Beste, K. B. Isaacson, D. A. Lauffenburger, L. G. Griffith and J. Han, J. Am. Chem. Soc., 2012, 135, 1645–1648 CrossRef PubMed.
-
J. P. Hobson, S. Liu, S. Leppla and T. Bugge, in Proteases and Cancer, ed. T. H. Bugge and T. M. Antalis, Humana Press, 2009, vol. 539, pp. 115–129 Search PubMed.
- J. O. McIntyre and L. M. Matrisian, Methods Mol. Biol., 2009, 539, 155–174 CAS.
- J. O. McIntyre, B. Fingleton, K. S. Wells, D. W. Piston, C. C. Lynch, S. Gautam and L. M. Matrisian, Biochem. J., 2004, 377, 617–628 CrossRef CAS PubMed.
- T. Jiang, E. S. Olson, Q. T. Nguyen, M. Roy, P. A. Jennings and R. Y. Tsien, Proc. Natl. Acad. Sci. U. S. A., 2004, 101, 17867–17872 CrossRef CAS PubMed.
- B. F. Sloane, M. Sameni, I. Podgorski, D. Cavallo-Medved and K. Moin, Annu. Rev. Pharmacol. Toxicol., 2006, 46, 301–315 CrossRef CAS PubMed.
-
K. Moin, M. Sameni, B. C. Victor, J. M. Rothberg, R. R. Mattingly and B. F. Sloane, in Methods Enzymol., ed. P. M. Conn, Academic Press, 2012, vol. 506, pp. 175–194 Search PubMed.
- K. Wolf and P. Friedl, Biochimie, 2005, 87, 315–320 CrossRef CAS PubMed.
- R. M. Martin, H. Leonhardt and M. C. Cardoso, Cytometry, Part A, 2005, 67A, 45–52 CrossRef CAS PubMed.
- D. G. Spiller, C. D. Wood, D. A. Rand and M. R. H. White, Nature, 2010, 465, 736–745 CrossRef CAS PubMed.
- E. Primiceri, M. S. Chiriaco, R. Rinaldi and G. Maruccio, Lab Chip, 2013, 13, 3789–3802 RSC.
- I. Meyvantsson and D. J. Beebe, Annu. Rev. Anal. Chem., 2008, 1, 423–449 CrossRef CAS PubMed.
- H. Yin and D. Marshall, Curr. Opin. Biotechnol., 2012, 23, 110–119 CrossRef CAS PubMed.
- Y. Zheng, J. Nguyen, Y. Wei and Y. Sun, Lab Chip, 2013, 13, 2464–2483 RSC.
- M. Ochsner, M. R. Dusseiller, H. M. Grandin, S. Luna-Morris, M. Textor, V. Vogel and M. L. Smith, Lab Chip, 2007, 7, 1074–1077 RSC.
- M. Charnley, M. Textor, A. Khademhosseini and M. P. Lutolf, Integr. Biol., 2009, 1, 625–634 RSC.
- J. C. Love, J. L. Ronan, G. M. Grotenbreg, A. G. van der Veen and H. L. Ploegh, Nat. Biotechnol., 2006, 24, 703–707 CrossRef CAS PubMed.
- C. Ma, R. Fan, H. Ahmad, Q. Shi, B. Comin-Anduix, T. Chodon, R. C. Koya, C.-C. Liu, G. A. Kwong, C. G. Radu, A. Ribas and J. R. Heath, Nat. Med., 2011, 17, 738–743 CrossRef CAS PubMed.
- M. A. Unger, H.-P. Chou, T. Thorsen, A. Scherer and S. R. Quake, Science, 2000, 288, 113–116 CrossRef CAS.
- M. A. Miller, L. Barkal, K. Jeng, A. Herrlich, M. Moss, L. G. Griffith and D. A. Lauffenburger, Integr. Biol., 2011, 3, 422–438 RSC.
- C. J. Marshall, Cell, 1995, 80, 179–185 CrossRef CAS.
- R. M. Shymko, P. De Meyts and R. Thomas, Biochem. J., 1997, 326, 463–469 CAS.
- S. K. W. Dertinger, D. T. Chiu, N. L. Jeon and G. M. Whitesides, Anal. Chem., 2001, 73, 1240–1246 CrossRef CAS.
- D. M. Parkin, F. Bray, J. Ferlay and P. Pisani, Ca-Cancer J. Clin., 2005, 55, 74–108 CrossRef.
- Y. Hoshida, A. Villanueva, M. Kobayashi, J. Peix, D. Y. Chiang, A. Camargo, S. Gupta, J. Moore, M. J. Wrobel, J. Lerner, M. Reich, J. A. Chan, J. N. Glickman, K. Ikeda, M. Hashimoto, G. Watanabe, M. G. Daidone, S. Roayaie, M. Schwartz, S. Thung, H. B. Salvesen, S. Gabriel, V. Mazzaferro, J. Bruix, S. L. Friedman, H. Kumada, J. M. Llovet and T. R. Golub, N. Engl. J. Med., 2008, 359, 1995–2004 CrossRef CAS PubMed.
- C. Berasain, A. Nicou, O. Garcia-Irigoyen, M. U. Latasa, R. Urtasun, M. Elizalde, F. Salis, M. J. Perugorría, J. Prieto, J. A. Recio, F. J. Corrales and M. A. Avila, Dig. Dis., 2012, 30, 524–531 CrossRef PubMed.
- K. Chalupský, I. Kanchev, O. Žbodáková, H. Buryová, M. Jiroušková, V. Kořínek, M. Gregor and R. Sedláček, Folia Biol., 2013, 59, 76–86 Search PubMed.
- L. E. Gentry, K. E. Chaffin, M. Shoyab and A. F. Purchio, Mol. Cell. Biol., 1986, 6, 735–738 CAS.
- S. Jacobs, N. E. Sahyoun, A. R. Saltiel and P. Cuatrecasas, Proc. Natl. Acad. Sci. U. S. A., 1983, 80, 6211–6213 CrossRef CAS.
- P. E. Driedger and P. M. Blumberg, Proc. Natl. Acad. Sci. U. S. A., 1980, 77, 567–571 CrossRef CAS.
- S. M. Le Gall, T. Maretzky, P. D. A. Issuree, X.-D. Niu, K. Reiss, P. Saftig, R. Khokha, D. Lundell and C. P. Blobel, J. Cell Sci., 2010, 123, 3913–3922 CrossRef CAS PubMed.
- P. M. Blumberg, Cancer Res., 1988, 48, 1–8 CAS.
- M. Dang, N. Armbruster, M. A. Miller, E. Cermeno, M. Hartmann, G. W. Bell, D. E. Root, D. A. Lauffenburger, H. F. Lodish and A. Herrlich, Proc. Natl. Acad. Sci. U. S. A., 2013, 110, 9776–9781 CrossRef CAS PubMed.
- L. G. Puente, J.-S. He and H. L. Ostergaard, Eur. J. Immunol., 2006, 36, 1009–1018 CrossRef CAS PubMed.
- S. M. Soond, B. Everson, D. W. H. Riches and G. Murphy, J. Cell Sci., 2005, 118, 2371–2380 CrossRef CAS PubMed.
- J. R. Doedens, R. M. Mahimkar and R. A. Black, Biochem. Biophys. Res. Commun., 2003, 308, 331–338 CrossRef CAS.
- P. Reddy, J. L. Slack, R. Davis, D. P. Cerretti, C. J. Kozlosky, R. A. Blanton, D. Shows, J. J. Peschon and R. A. Black, J. Biol. Chem., 2000, 275, 14608–14614 CrossRef CAS PubMed.
- D. R. Larson, C. Fritzsch, L. Sun, X. Meng, D. S. Lawrence and R. H. Singer, eLife, 2013, 2, e00750 Search PubMed.
- M. C. Walters, S. Fiering, J. Eidemiller, W. Magis, M. Groudine and D. I. Martin, Proc. Natl. Acad. Sci. U. S. A., 1995, 92, 7125–7129 CrossRef CAS.
Footnote |
† Electronic supplementary information (ESI) available. See DOI: 10.1039/c5ib00019j |
|
This journal is © The Royal Society of Chemistry 2015 |