DOI:
10.1039/C3LC50625H
(Critical Review)
Lab Chip, 2014,
14, 32-44
Technologies for label-free separation of circulating tumor cells: from historical foundations to recent developments
Received
21st May 2013
, Accepted 29th July 2013
First published on 30th July 2013
Abstract
Circulating tumor cells (CTCs) are malignant cells shed into the bloodstream from a tumor that have the potential to establish metastases in different anatomical sites. The separation and subsequent characterization of these cells is emerging as an important tool for both biomarker discovery and the elucidation of mechanisms of metastasis. Established methods for separating CTCs rely on biochemical markers of epithelial cells that are known to be unreliable because of epithelial-to-mesenchymal transition, which reduces expression for epithelial markers. Emerging label-free separation methods based on the biophysical and biomechanical properties of CTCs have the potential to address this key shortcoming and present greater flexibility in the subsequent characterization of these cells. In this review we first present what is known about the biophysical and biomechanical properties of CTCs from historical studies and recent research. We then review biophysical label-free technologies that have been developed for CTC separation, including techniques based on filtration, hydrodynamic chromatography, and dielectrophoresis. Finally, we evaluate these separation methods and discuss requirements for subsequent characterization of CTCs.
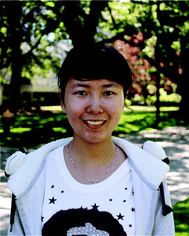 Chao Jin | Chao Jin is a MASc student at the University of British Columbia. She received her bachelor's degree in Mechatronic Engineering at Zhejiang University. She is currently pursuing her master's degree in Mechanical Engineering and her research area is the separation of circulating tumor cells from blood using microfluidic technologies. |
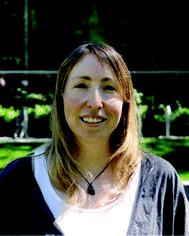 Sarah M. McFaul | Sarah McFaul received her bachelor's degree in Engineering Physics and Society at McMaster University, followed by her master's degree in Linguistics at Trinity Western University, and her master's degree in Mechanical Engineering at the University of British Columbia. She specialized in microfabrication and has done research in silicon photonics, microfluidic cell sorting, and cell deformability studies. |
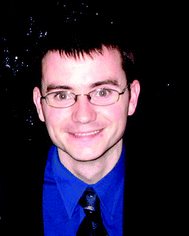 Simon P. Duffy | Simon Duffy received his PhD from the University of Victoria, Canada (2006), and pursued postdoctoral research at the Toronto Hospital for Sick Children and the University of Toronto, where he studied virus-mediated alterations of cell membrane structure and function. Dr Duffy’s current research interests involve the use of microfluidic technologies for cell separation and biomechanical characterization of the cell. |
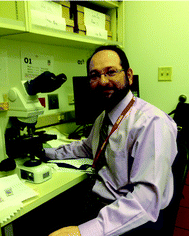 Peyman Tavassoli | Dr Tavassoli, an MD and succeeding a PhD graduate, developed cell based screening assays for agents that modulate growth, death and androgen receptor activation in prostate cancer cells. He subsequently applied his method to find more potent drugs against receptor activity. His passion about cancer cell biology led him to carry on his training in diagnostic pathology. He believes that a unique molecular profile dictates cytoskeletal character of cells. By using cytoskeletal features to sort out cancer cells, one can explore novel molecular changes at any stage of disease that may consecutively impose new treatment plans. |
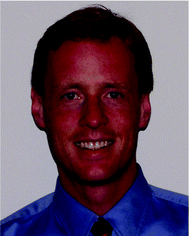 Peter C. Black | Peter Black is a Urologic Oncologist at Vancouver General Hospital, a Research Scientist at the Vancouver Prostate Centre, and an Assistant Professor in the Department of Urologic Sciences at the University of British Columbia. His clinical and research expertise lies primarily in urothelial carcinoma of the bladder and prostate cancer. He maintains a translational research program focused on developing novel therapies for bladder cancer by targeting growth factor receptors. He is also studying genomic markers for clinical decision making in both bladder and prostate cancer. |
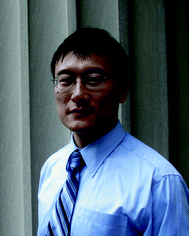 Hongshen Ma | Hongshen Ma is an Assistant Professor in the Department of Mechanical Engineering at the University of British Columbia and a Research Scientist at the Vancouver Prostate Centre. He received his bachelor’s degree in Engineering Physics at the University of British Columbia, and his master’s and PhD degrees from the Massachusetts Institute of Technology. His current research program focuses on the development of microfluidics technologies for cell biomechanics and cell separation with applications in circulating tumor cells, malaria, and blood storage. |
1. Introduction
Circulating tumor cells (CTCs) were first discovered in 1869 by Thomas Ashworth who described small numbers of cells in patient blood resembling the cells of the primary tumor.1 The significance of CTCs is derived from the belief that they may be the precursors for the formation of secondary tumors during metastasis.2 This theory is strongly supported by the demonstrated prognostic value of CTC enumeration, which correlates to progression-free survival and overall survival of patients with metastatic carcinomas.3 In addition to serving as a prognostic marker, CTCs have also been proposed as a pharmacodynamic marker to monitor treatment efficacy and to help personalize therapy.4 In recognition of the potential utility of CTCs in research and treatment of cancer, there is growing interest in developing strategies for improved capture, enumeration, and characterization of CTCs.
A key limitation in the capture and analysis of CTCs is their extreme rarity in blood relative to hematological cells, which imposes formidable technical and analytical challenges. Since CTCs can occur at rates as low as 1 in 109 erythrocytes and 1 in 107 leukocytes, the challenge in isolating CTCs is effective depletion of these cell types.5,6 Mature erythrocytes have distinct physical, chemical, and biological properties that allow them to be easily removed from blood. However, leukocytes share many properties in common with CTCs, leading to high levels of leukocyte contamination in many separation methods. Therefore, effective discrimination of CTCs from leukocytes is the key functional requirement of any separation process. Current CTC separation methods can be divided into two broad categories: biochemical methods and biophysical methods.
Biochemical CTC separation methods typically rely on a combination of affinity capture of cell surface antigens to enrich for potential target cells and fluorescent labeling of intracellular markers to identify the CTCs. Specifically, a key challenge associated with these techniques is that CTCs are inherently heterogeneous and do not universally express specific tumor markers.7 Instead, existing biochemical separation processes discriminate CTCs from hematological cells using antigens expressed in epithelial cells. Since normal epithelial cells rarely circulate in peripheral blood, cells isolated using these markers are assumed to be CTCs. An established system developed using this approach has been commercialized as the Veridex CellSearch® system (Raritan, NJ, USA), which has received FDA approval for clinical enumeration of CTCs. The CellSearch® system initially captures candidate cells using the cell surface antigen, epithelial cell adhesion molecule (EpCAM). The captured cells are positively identified as CTCs using fluorescent staining for cytokeratins (CK, a family of intracellular proteins that forms the cytoskeleton of epithelial cells), while leukocytes are excluded by staining for CD45. A fundamental flaw with the existing biochemical approach is that a subpopulation of metastatic tumor cells are likely to undergo epithelial-to-mesenchymal transition (EMT), which is associated with a loss of expression for epithelial markers, such as EpCAM and CK.8 Consequently, the most aggressive cancer cells may actually be the least likely to be captured and identified using this technique. Furthermore, questions remain whether all CK stained cells are actually CTCs and whether leukocytes can occasionally express epithelial markers when activated.9–11 Finally, affinity capture processes often result in permanent attachment of target cells to an artificial surface, which limits downstream options for the extraction and subsequent characterization of these cells.
Physical CTC separation methods rely on differences in the physical properties of CTCs compared to leukocytes including cell size, shape, deformability, density, electrical polarizability, and magnetic susceptibility.12 These methods are label-free, and are therefore able to avoid the epithelial antigen bias of existing biochemical methods. It is worth noting that although affinity capture can be considered label-free in cases where antigens are used to attach cells to a surface13 rather than to attach a label to the cell, such an approach does not avoid the problem of epithelial antigen bias. Furthermore, since CTCs are unmodified by physical separation processes, cells isolated using these methods are compatible with a wider range of analyses, including those requiring viable cells. Recently, advances in microfabrication and microfluidics provide the ability to create structures at or below the length scale of single cells,14 and therefore present potential new mechanisms for label-free separation of CTCs based on their physical properties.
Several recent reviews on CTC separation have focused on topics ranging from the methodologies of microfluidic cell separation12,14–20 to the detection and characterization of CTCs.21–25 In this review, we begin with an overview of the known physical properties of CTCs that can be exploited in label-free separation including both historical studies of the morphology of CTCs and recent studies of the biophysical and biomechanical properties of CTCs. Next, we formulate performance specifications for label-free separation methods, and then review three classes of such methods including filtration, hydrodynamic chromatography, and dielectrophoresis. Finally, we present different types of post-separation analysis that can be performed on the separated CTCs and discuss target specifications for label-free separation methods. Our aim is to provide the reader with the perspective to effectively evaluate label-free separation technologies through an understanding of the biophysical and biomechanical properties of CTCs, mechanisms and capabilities of available technologies, and the requirements of various types of post-separation analyses.
2. Biophysical and biomechanical properties of CTCs
The biophysical and biomechanical properties of CTCs are important because of the potential to use these properties to discriminate CTCs from leukocytes. This section initially reviews historical studies of the physical and morphological properties of CTCs that began to emerge in the 1950s and have yielded information on the size, shape, and internal structure of CTCs, showing them to be typically larger than leukocytes with unusual internal features such as an excess or fragmentation of chromatin. Next, we present recent microscopy studies, which also found CTCs to be typically larger than leukocytes, but with significant variability in CTC size. Finally, we discuss the appropriateness of using cultured cells as models for CTCs in order to develop and evaluate label-free separation methods, and compare known differences between CTCs and cultured cancer cells.
2.1 Historical perspectives and recent studies on the biophysical and biomechanical properties of CTCs
Historically, CTCs were found by microscopic examination of the blood from metastatic cancer patients. When they were first identified by Thomas Ashworth in 1869, he noted that they resembled the cells of the original tumor.1 In the following century there were a handful of studies which reported the observation of unusual cells in the blood of cancer patients which may have been malignant.26–29 However it was not until the Papanicolaou stain came into widespread use in the 1950s that CTCs could be identified with greater certainty. In these studies, erythrocytes were depleted either through lysis or centrifugation, while the remaining cells were fixed and stained on a slide. Identification of CTCs was done by trained cytologists who looked for cells with features such as lobulated, crenelated, or elongated nuclei, and fragmentation or granulation of the chromatin according to Papanicolaou's criteria for malignancy.30–33 In 1959 S. H. Seal observed that in density gradient centrifugation the specific gravity of CTCs appeared to be 1.065, whereas the specific gravity of leukocytes was found to be 1.056. While this difference could potentially enable separation based on this method, he also recognized the potential for significant variability in these values because of the inherent heterogeneity in both CTCs and leukocytes. He further concluded that CTCs are generally larger than other cellular elements of the blood and that they also appear to be more rigid.34 Shortly after, some studies identified other large benign cells in the blood, such as megakaryocytes, macrophages and endothelial cells which may have been mistakenly identified as CTCs in previous studies.35,36 The positive identification of CTCs involved examining cells from the primary tumor using the same staining technique and finding cells in the blood which were similar while being sufficiently different from any other benign cells to the eye of a trained cytologist.35 Additionally, several studies noted that many CTCs were found in clusters rather than individually.35–38 These historical studies demonstrated that CTCs differ from leukocytes in size, density, internal structure, as well as their tendency to form clusters – all of which could be harnessed for label-free separation.
Recently, automated microscopy and image analysis of large numbers of fluorescently stained cells has provided additional insight into the morphology of CTCs.39 In a study of CTCs from a patient with breast cancer Marrinucci et al. found CTCs to be highly heterogeneous including both high and low nuclear to cytoplasmic ratios (N/C, defined as the ratio of nuclear area and cell area with the nuclear area subtracted) and significant variation in size.40 Recent findings from our group in a study of prostate cancer patients also revealed that their CTCs have significant shape variability with many having an elongated shape.41 Examples of CTCs in clusters or with irregular shapes were also shown by Allard et al. in a study of 964 patients having different cancer types.5 Hyun et al. compared CTCs from breast cancer patients with leukocytes, and concluded that CTCs were generally larger or of similar size to leukocytes.42 Similarly, in a study of a single patient with lung adenocarcinoma, Marrinucci et al., reported that CTCs were typically larger than blood cells.43 In fact, one of the historical criteria used to identify a CTC until recently was that it be larger than leukocytes.44 However, this criterion is not uniformly true since CTCs <4 μm in diameter have been discovered using the CellSearch® system.5 Marrinucci et al. also discovered CTCs of the same size or smaller than leukocytes in a study of a small number of colorectal cancer patients.45 Our group recently measured the average diameter of CTCs from patients with castrate resistant prostate cancer and found the average size ranged from 7.05 μm to 8.94 μm across different patients.41 In comparison, recent measurements show various leukocyte types have typical average diameters ranging from 6–9 μm.46 These studies suggest that although many CTCs are larger than leukocytes, there is significant overlap in the size of CTCs and leukocytes that may hinder size-based separation efforts.
Deformability is likely to be an important property of CTCs in cell separations, however there is currently no data on the deformability of patient-derived CTCs due to the fact that isolated CTCs are typically fixed and stained in order to be identified, resulting in a loss of viability. However, deformability can be inferred from the nuclear to cytoplasmic ratio (N/C), where cells having larger N/C are likely to be less deformable. In a study of 36 breast cancer patients, Meng et al. observed that the average N/C ratio of CTCs was 4.0. In contrast, they reported the N/C ratio of leukocytes to be 1.22.44 Our recent study on prostate cancer patients found the average N/C ratio of CTCs to be 1.43 with significant variability.41 To estimate the deformability differences between CTCs and leukocytes, some studies measured the deformability of cultured cancer cells,47,48 or cells from the primary tumor as models.49,50 As expected, these cells were found to be less deformable than leukocytes, typically by an order of magnitude or more. The combination of greater N/C ratio and the decreased deformability of cultured cancer cells suggests that CTCs are likely to be significantly stiffer than leukocytes, and therefore can potentially be separated using label-free processes that discriminate these cells based on deformability.
2.2 Appropriateness of using cultured cancer cells as a model
As mentioned previously, many of the properties of CTCs are inferred from cultured cancer cells and CTC separation technologies are typically developed and evaluated using model samples generated by doping cultured cancer cells into whole blood obtained from healthy volunteers. The appropriateness of using cultured cancer cells as models for CTCs is questionable since cultured cells from epithelial cancers grow attached to the culture plate and to each other, while CTCs must be able to transit through the human circulatory system. Furthermore, cultured cancer cells are usually relatively stable and homogeneous, while CTCs in patient blood are likely to present the full morphologic spectrum of cancer cells from primary to circulating to metastatic tumors.40 Our recent study found that CTCs from castrate resistant prostate cancer patients are nearly half the diameter of cultured prostate cancer cells.41 Meng et al. compared CTCs from metastatic breast cancer patients with cultured breast cancer cells, and found both their sizes and N/C ratios to be similar.44 However Lin et al. found that cell sizes of cultured tumor cells (14–19 μm) are smaller compared with CTCs from breast cancer patients (15–30 μm).51 Another consideration is that cells from different types of cancer can have different sizes,46 and size can also vary from patient to patient.52 Additionally, while cultured cancer cells are generally less deformable than leukocytes, several studies have shown that more aggressive cancer cells are more deformable than benign cells,53–55 and that greater invasiveness is correlated with greater deformability.49,55,56 Since CTCs are generally considered a highly invasive sub-population of the primary tumor, they are presumed to be more deformable than cultured cancer cells. If CTCs are indeed smaller and more deformable than cultured cancer cells, then separation technologies that exploit size or deformability to discriminate these cells will likely over-predict their performance when cultured cancer cells are used as models for CTCs.
3. Performance metrics for label-free cell separation
The objective of a label-free separation technology is to enrich the concentration of CTCs from an input sample to an output sample (Fig. 1). Key performance metrics for evaluating these separation technologies include yield (also known as recovery rate or capture efficiency), purity, enrichment, and throughput. Yield is the fraction of captured target cells relative to the number of target cells in the original sample (eqn (1)). Yield is important for CTC enumeration where it is important to know the CTC concentration in the patient bloodstream. Purity of the output sample is the fraction of target cells relative to the total captured cells (eqn (2)). Purity can vary considerably depending on the concentration of CTCs in the patient's blood or the construction of the model samples (e.g. sample doping ratio). Purity is an important metric for certain types of post-separation analysis, such as genomic profiling, but has been poorly reported in the literature. Enrichment is the enhancement of the target cell to background cell ratio at the input of a separation system to the output (eqn (3a)). It can also be calculated from the yield and percentage of background cells removed (eqn (3b)). This parameter provides a single value, combining both yield and purity, that is indicative of the selectivity of a separation process. Throughput indicates the speed at which the system can process a sample. This parameter is typically reported as either the volumetric flow rate or the number of cells processed per time. The number of cells processed per time is more useful since it is independent of the sample concentration. |
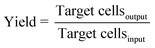 | (1) |
|
 | (2) |
|
 | (3a) |
|
 | (3b) |
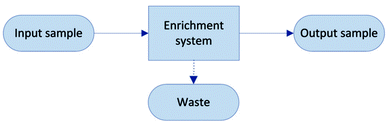 |
| Fig. 1 Conceptual schematic of a cell separation system. | |
4. Label-free separation methods
4.1 Filtration
Filtration is the process of flowing a cell sample through an array of micro-scale constrictions in order to capture target cells based on a combination of size and deformability. Filtration microstructures developed for the separation of CTCs can be categorized into three basic types: weir,57,58 pillar,59–61 and pore.51,62,63 Weir structures consist of microchannels containing a sudden decrease in the channel cross-section (Fig. 2A). Pillar structures consist of an array of micro-posts spaced appropriately to form constrictions to capture target cells (Fig. 2B). Pore structures consist of a membrane perforated with a 2D array of small holes (Fig. 2C). The key design parameters in these filter microstructures include the flow rate of the fluid and the cross-sectional opening of the constrictions. The fluid flow rate determines the force applied to each cell as it is deformed through a constriction. The cross-sectional opening determines the size and shape of the deformed cell that can be captured by the filter. The combination of the two therefore determines the threshold size and deformability of target cells that can be caught by the filter. The practical limit of this process is the maximum force that can be tolerated by the filtered cells before causing permanent damage.64 Cancer cells and other large cells in blood were captured by Seal in 1964 by pore type filtration using a perforated section of clear plastic tape.65 Vona et al. also characterized this method using pores in a polycarbonate membrane to capture cultured tumor cells spiked into peripheral blood and succeeded in detecting a single tumor cell added to 1 ml of blood.66 Recent devices developed using this principle have demonstrated the ability to capture >85% doped cultured cancer cells in work by Lin et al.,51 Lu et al.,61 Zheng et al.,63 McFaul et al.,59 and Lin et al.67 Among them, Lin et al.,51 Zheng et al.,63 and Lin et al.67 have demonstrated the ability to enrich the population of doped cancer cells by a factor of >1000. Several commercial devices utilizing the pore structure concept have also been introduced, including the Rarecells®,68 ScreenCell®,69 and ClearCell®60 devices. Studies of the effectiveness and clinical utility of these devices are currently ongoing.70–73
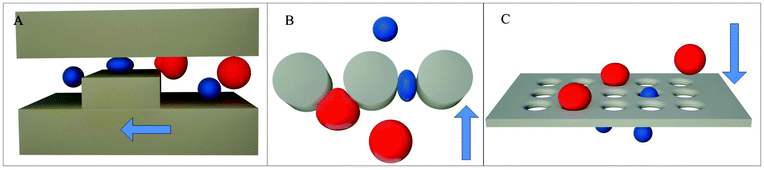 |
| Fig. 2 Filtration mechanisms: (A) weir, (B) pillar, (C) pore. | |
One of the key challenges in using mechanical constrictions to separate CTCs is the potential for clogging when large numbers of cells are processed, which can cause unpredictable variations in flow rate and consequently in the force applied to squeeze cells through each constriction. McFaul et al. addressed this shortcoming by periodically clearing the filter element with an oscillatory flow.59 Another challenge of filtration is the extraction of separated cells for further characterization, which is often hindered by the adsorption of cells onto the filter material. One solution is to simply analyze the separated cells on the filter. Lim et al. presented a system utilizing a micro-fabricated silicon micro-sieve to obtain CTC antibody staining, separation, and enumeration. They also demonstrated the feasibility of on-micro-sieve fluorescence in situ hybridization (FISH) at a single cell level using BT474 breast cancer cells.74 Zheng et al. facilitated polymerase chain reaction (PCR)-based genomic analysis by performing on-membrane electrolysis after CTCs were captured on a membrane with 16
000 filtering pores.75 This same concept can be easily extended to combine other CTC detection and characterization methods on the same chip to gain higher efficiency.
Hydrodynamic separation uses interactions between particles and obstacles in flow in order to impart different flow velocities to different particles based on differences in size, and in some cases, deformability.76 A key advantage of this approach over other microfluidic mechanisms is its relatively high throughput (up to 600 ml h−1).77 Hydrodynamic chromatography has been applied in two different ways. The first operates in the low Reynolds number (Re ≪ 1) regime where inertial effects can be ignored, while the second operates with Reynolds numbers on the order of 1 where inertial effects play an important role.78 One prominent design in the low Reynolds number regime is deterministic lateral displacement (DLD) where cells below a critical size will follow streamlines and pass through an array of posts with no net lateral displacement, while the cells above a critical size will be shifted to a different streamline when they are confronted with obstructions and are laterally displaced from the original streamline (Fig. 3).79 The lateral displacement of cells is determined by the geometry of the post array, and the shape and size of each cell. This method has been shown to be capable of separating ∼1 μm diameter microparticles with a resolution of less than 20 nm.80 Liu et al. separated spiked cancer cells from blood with >80% yield using DLD, demonstrating its potential for the separation of CTCs.81
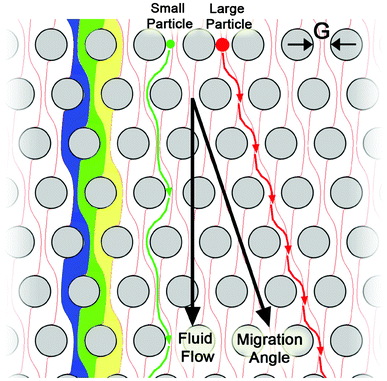 |
| Fig. 3 Principle of the deterministic lateral displacement separation method. The periodic flow patterns, or streamlines, are highlighted in blue, green, and yellow; a small particle (green) is able to follow the fluid flow, while the large particle (red) is excluded from the leftmost streamline in each gap. This difference in behaviour causes the large particle to move at a different trajectory than the bulk fluid to enable separation. Reprinted with permission from ref. 79. Copyright 2009, American Institute of Physics. | |
The second type of hydrodynamic separation is based on inertial effects.82–86,42 Recent studies have shown that in the modest Reynolds number regime between Stokes flow and inviscid flow, where both inertia and viscosity are finite, forces arising from inertial effects including drag forces from Dean flows, shear gradient lift forces, and wall-effect lift forces can be balanced against each other to enable size based separation.78 Specifically, shear gradient lift force acts along the shear gradient and reaches zero at the channel centerline. The wall-effect lift force, generated by a velocity change in the fluid as it passes through the area between the particle and the wall, act orthogonal to the wall surface. In a straight channel, inertial lift forces and drag forces act orthogonally on a particle (Fig. 4). However, in a curved channel, secondary cross-sectional flows known as Dean flows will develop due to a mismatch in velocity between the fluid in the center and near-wall regions of a channel. Dean flow will cause a particle to experience a drag force along the same axis as the shear gradient and wall-effect lift forces, all of which are orthogonal to the direction of the primary flow.87 The balance of these forces results in different equilibrium positions for particles of different sizes. In the single spiral design of Bhagat et al., these inertial forces generated by the spiral microchannel geometry cause cancer cells to occupy a single equilibrium position near the inner microchannel wall (Fig. 5).88 Smaller particles (blood cells) migrate to the outer half of the channel under the influence of Dean forces resulting in the formation of two distinct particle streams which are collected in two separate outputs.88 Hou et al. used this approach to successfully isolate CTCs from 20 lung cancer patients and showed an enrichment ratio of ∼2000 using cultured cancer cells doped into whole blood.83 Sun et al. modified the single spiral micro-channel to create a double spiral which provides better focusing behavior of small blood cells, thus increasing yield (88.5%) and throughput (2 × 109 cells h−1).82 Hur et al. demonstrated a unique phenomenon where laminar vortices act to selectively isolate particles above a critical cutoff size, and used this phenomenon for rapid size-based cell enrichment (2.7 × 1010 cells h−1).84 Hydrodynamic methods can typically achieve greater throughput than filtration methods, however enrichment is often poorer (<100 over leukocytes in many cases42,81,82,85) when compared with filtration methods because of its current inability to discriminate nucleated cells based on deformability.
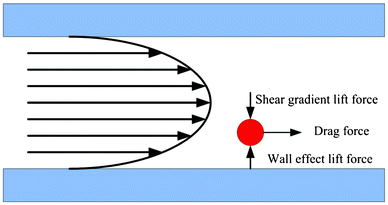 |
| Fig. 4 Forces acting on a particle in a straight microchannel. Shear gradient lift force and wall-effect lift force are orthogonal to the direction of primary flow. Drag force is in the direction of primary flow and will be zero when the particle's velocity equals the flow velocity. | |
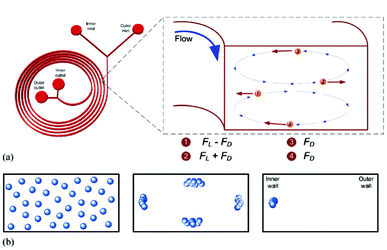 |
| Fig. 5 Principle of the inertial microfluidic separator. Sample is introduced through the inner inlet. Neutrally buoyant particles experience lift forces (FL) and Dean drag (FD), which results in differential particle migration within the microchannel. Reproduced from ref. 88. | |
4.3 Dielectrophoresis
Dielectrophoresis (DEP) is capable of discriminating between blood cells and tumor cells on the basis of cell size, nuclear morphology, and membrane morphology, all of which contribute to differences in cell membrane area and cell dielectric properties.89,90 When a cell is suspended in a medium with dielectric properties distinct from the cell and is subjected to an electric field, a dipole moment along the direction of the applied field will be generated due to charges induced at the interfaces. If the electrical polarizability of the cells exceeds that of the suspending medium, the DEP force will be along the same direction as the gradient of the electric field (Fig. 6A). In this case, cells move toward the strong electric field region, resulting in positive dielectrophoresis (pDEP) (Fig. 6B). On the other hand, when the electrical polarizability of the cells is less than that of the medium, the direction of the DEP force acts in the reverse direction (Fig. 6C) and cells move to the weak electric field region, resulting in negative dielectrophoresis (nDEP) (Fig. 6D).91 The polarizability of a cell depends strongly on its composition, morphology, and the frequency of the applied electric field.92 Therefore, cells of different phenotypes or physiological states can potentially be discriminated using this approach.89
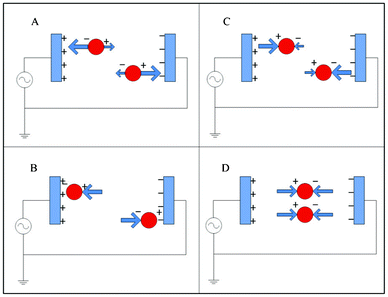 |
| Fig. 6 Dielectrophoresis: (A) positive DEP; (B) particle equilibrium point in pDEP; (C) negative DEP; (D) particle equilibrium point in nDEP. | |
Using DEP, Alazzam et al. demonstrated the separation of cultured cancer cells from blood with 96% yield.93 Gascoyne et al. used DEP to separate mixtures of three different cultured tumor cell types doped into peripheral blood with >90% yield.94 Additionally, Gascoyne et al. also found correlations between the dielectric properties of cells and their exterior morphology, which may provide a basis for predicting the DEP behavior of CTCs.46 Recently, DEP cell separation has been improved by combining DEP with other separation techniques. Shim et al. designed a system with a continuous flow microfluidic processing chamber, whereas previous microfluidic DEP methods processed the sample in batches. Similar research has been conducted by Moon et al.95 who combined multi-orifice flow fractionation (MOFF) and dielectrophoresis together to enrich the concentration of MCF-7 cells 162-fold over erythrocytes at a 7.6 ml h−1 flow rate.96 Huang et al. developed an optically-induced-dielectrophoresis system, which allowed for rapid modification of the electrode setup through the control of optical images.97 Compared to filtration and hydrodynamic methods, DEP-based methods currently lag in performance in both selectivity (enrichment over leukocytes typically <100) and throughput (typically <1 ml h−1).
4.4 Technology evaluation
Performance specifications from recent (since 2009) label-free separation devices are listed in Table 1. In general, hydrodynamic methods have the highest throughput, followed by filtration methods. DEP methods typically have lower throughput. Historically, filtration methods have had the highest yield, however recent work in hydrodynamic and DEP methods have produced yield results that are comparable with filtration. Enrichment values vary quite considerably between technologies using the same separation principle, but historically filtration has produced the highest enrichment values with comparable results recently being demonstrated by hydrodynamic methods.
Table 1 Recent research in label-free based cancer cell separation methods
Method |
Cancer cell lines used for characterization |
Yield |
Purity |
Blood cell removal |
Enrichment over RBCs |
Enrichment over WBCs |
Throughput |
Reference |
Calculated using eqn (3b). Throughput has been converted into units of ml h−1 or cells h−1. |
Filter (pore type) |
LNCaP cell line |
86.5% |
— |
— |
— |
103 |
>120 ml h−1 |
2011 Zheng et al.63 |
Filter (pore type) |
RT4, T24, J82, HT-1080, LNCaP, MCF-7, MDA-MB-231, SK-BR-3 cell line |
>90% |
— |
— |
∼107 |
— |
>225 ml h−1 |
2010 Lin et al.51 |
Filter (pillar type) |
MCF-7, MDA-MB-231 cell line |
80% |
89% |
— |
— |
— |
— |
2010 Tan et al.60 |
Filter (pillar type) |
PC-3 cell line |
>90% |
— |
99.5% WBC removal |
— |
180a |
— |
2010 Lu et al.61 |
Filter (pillar type) |
L1210 mouse lymphoma cells |
98% |
99% |
— |
— |
— |
9000 cells h−1 |
2012 McFaul et al.59 |
Filter (pillar type) |
UM-UC13 cell line |
97% |
75% |
99.7% WBC removal |
— |
3 × 103 |
>1 × 106 cells h−1 |
2013 Lin et al.67 |
Hydrodynamic (DLD) |
MCF-7 cell line |
98.7% |
— |
99.14% RBC and 97.59% WBC removal |
115 |
41 |
120 ml h−1 |
2013 Liu et al.81 |
Hydrodynamic (inertia: Dean flow) |
MCF-7, HeLa cell line |
88.5% |
— |
— |
— |
19 |
2 × 109 cells h−1 |
2012 Sun et al.82 |
Hydrodynamic (inertia: Dean flow) |
MCF-7 cell line |
>85% |
— |
— |
109 |
∼103 |
3 ml h−1 |
2013 Hou et al.83 |
Hydrodynamic (inertia: lift force) |
MCF-7, HeLa cell line |
23% (MCF-7), 10% (HeLa) |
— |
— |
— |
7.5 (MCF-7); 5.5 (HeLa) |
2.7 × 1010 cells h−1 |
2011 Hur et al.84 |
Hydrodynamic (inertia) |
MCF-7, MDA-MB-231 cell line |
81.1% |
— |
99.99969% RBC and 99.992% WBC removal |
3.25 × 105 |
1.2 × 104 |
6 × 109 cells h−1 (24 ml h−1) |
2011 Bhagat et al.86 |
Hydrodynamic (inertia: MOFF) |
MCF-7 cell line |
98.9% |
— |
70.3% WBC removal |
— |
3.3a |
8.4 ml h−1 |
2013 Moon et al.85 |
Hydrodynamic (inertia: MOFF) |
MCF-7, MDA-MB-231 cell line |
93.75% (MCF-7), 91.60% (MDA-MB-231) |
— |
90.8% WBC removal |
— |
10a |
36 ml h−1 |
2013 Hyun et al.42 |
Combination of MOFF and DEP |
MCF-7 cell line |
75.81% |
16.24% |
99.24% RBC and 94.23% WBC removal |
162 |
13a |
7.6 ml h−1 |
2011 Moon et al.95 |
DEP |
MDA-MB-435, MDA-MB-231 cell line |
70–80% |
— |
— |
— |
— |
4 × 107 cells h−1 (10 mL h−1) |
2013 Shim et al.96 |
DEP |
PC-3, OEC-M1 cell line |
76–83% (PC-3), 61–68% (OEC-M1) |
74–82% (PC-3), 64–66% (OEC-M1) |
— |
— |
— |
3 × 104 cells h−1 (0.006 ml h−1) |
2013 Huang et al.97 |
DEP |
MDA-MB-435, MDA-MB-468, MDA-MB-231 cell line |
>90% |
10% |
— |
— |
2 × 103 over mononuclear cells |
— |
2009 Gascoyne et al.94 |
DEP |
MDA-MB-231 cell line |
96% |
— |
— |
— |
— |
0.1 ml h−1 |
2011 Alazzam et al.93 |
A key problem in comparing separation technologies is the lack of standard definitions for performance metrics. For instance, some papers report enrichment of cancer cells over leukocytes,63 while others report it only over whole blood.51 Reporting of enrichment over leukocytes provides a better evaluation of the sensitivity of the system since leukocytes are the primary contaminant in CTC separations. Another difficulty is that the yield and enrichment of a size-based separation system depend on the size of cancer cell used. Gascoyne et al. analyzed a large number of cancer cell lines and found that cancer cell size varies considerably not just between different types of cancer, but also between different cell lines of the same cancer.46 Many size-based separations are characterized using only one cell line. One potential way to address this shortcoming is to validate a cell separation device using two or more different cancer cell lines encompassing a range of sizes. Lin et al. accomplish this goal by mixing together cells from 6 different cancer cell lines to test their device.51 Adopting standard definitions of performance metrics will allow label-free technologies to be more easily compared and evaluated.
5. Post-separation analysis
Post-separation analysis of CTCs includes identification, enumeration, and characterization. Currently, the widely accepted definition of CTCs involves the expression of epithelial markers (EpCAM, cytokeratins) and the absence of the leukocyte marker CD45.98 Additionally, CTCs can also be defined based on the expression of stem cell markers (e.g. CD133), mesenchymal markers (e.g. vimentin), or the shift in expression from epithelial E-cadherin to mesenchymal N-cadherin.99,100 Identification of CTCs via these markers can be performed using immunofluorescence, which is tolerant to contaminants in the output cell sample, as demonstrated in work by Nieva et al.101 and Marrinucci et al.40,102 Therefore, for the purpose of CTC enumeration, the key performance parameters for label-free separation technologies are yield and throughput, in order to capture a representative and statistically significant number of CTCs from a patient blood sample. Since CTC concentration is extremely low in the bloodstream, the enrichment of the separation system still needs to be high enough such that the target CTCs can be microscopically examined and distinguished from background cells in a reasonable amount of time.
A defining feature of CTCs is the genetic instability derived from the primary tumor. Fluorescent in situ hybridization (FISH) of labelled DNA probes can be used to assess genomic instability and has been employed in the assessment of the status of the human epidermal growth factor receptor 2 (HER2) that is commonly overexpressed in breast and prostate cancer CTCs.103,104 Similar to immunofluorescence, FISH is tolerant of cellular impurity but requires a high capture rate. Genetic instability can also be assessed by direct genomic profiling. Array-comparative genomic hybridization can assess genomic status of CTCs to infer the genomic profile of the primary tumor.105 Similarly, polymerase chain reaction (PCR)-based methods can be employed to amplify tumor-specific abnormalities present in the DNA or mRNA. Conventional PCR, nested PCR and quantitative real-time (qRT)-PCR can be used for both qualitative and quantitative measurement of the expression level of the specific target sequence. This method requires high quality nucleic acid as a template, which necessitates a nucleic acid purification step after separation. The specific and qualified primers and the appropriate control reference are of great importance for this detection method. PCR-based approaches have the advantage of being very sensitive with the lower limit of reliable detection currently at a concentration of 0.7 cells ml−1,106 but with the disadvantage of low specificity due to even minimal contamination.
Similarly, overexpression of cancer-associated genes can be assessed by analysis of the protein or expressed RNA of captured CTCs. Enzyme-linked immunosorbent assay (ELISA) can be employed to examine protein overexpression but requires a high capture efficiency and sample cell purity because ELISA has limited sensitivity. On the other hand, polymerase chain reaction (PCR)-based methods are much more widely used for detecting overexpression of cancer-associated genes. Devriese et al. use polymerase chain reaction with a panel of marker genes expressing cytokeratin 7 and 19, human epithelial glycoprotein and fibronectin 1 after immunomagnetic bead enrichment was performed. Then they use quadratic discriminant analysis to indicate CTC-positivity or CTC-negativity.4 Reverse transcriptase-polymerase chain reaction (RT-PCR) based assays detect tissue-specific mRNAs in peripheral blood, and thus their specificity for tumor cells depends on the assumption that normal tissue cells do not circulate, unless they become tumorous.66 Louha et al. showed that liver resection and needle liver biopsy will induce a release of cells from the liver into peripheral blood circulation.107 Therefore, although RT-PCR has high sensitivity, it may not be suitable for characterizing CTCs in cell preparations with a high number of contaminating non-target cells. Another detection method combines PCR and ELISA together, using PCR products as the specific probe for ELISA quantification.108 This technique has a higher sensitivity level than standard RT-PCR. PCR based approaches are sufficiently sensitive to have been proposed for characterization of circulating tumor DNA from cell-free plasma preparations.109 However, this circulating DNA would have a significant degree of DNA impurity from non-CTC cells and the capacity to capture CTCs would minimize the impact of non-target contamination.
Another approach to the characterization of CTCs is to quantify their biophysical and biomechanical properties including size, morphology, and deformability.110 Methods of characterization include visual microscopy,39,40 AFM,49,111 micropipette aspiration,47,50 electrical stretching,112,113 and optical tweezers.114,115 Since these characterization processes are typically applied on single cells, a relatively pure cell sample is required. Furthermore, since cell deformability is influenced by cell death, as well as physical and chemical damage, the capture of viable cells is a requirement for these types of studies.
In summary, the required specifications of CTC separation technologies depend critically on the desired post-separation analysis as shown in Table 2. For enumeration, yield is the most important parameter, but enrichment should also be high enough that all the cells in the output sample can be examined in a reasonable amount of time. Characterization processes such as genomic profiling require CTC purity in the output sample to be as high as possible. For example with a starting ratio of CTCs to leukocytes as low as 1
:
107 in the bloodstream,6 the initial sample will need to be enriched >2.33 × 107 to reach a sample with a CTC purity of 70%. Such demanding performance specifications suggest that multiple separation technologies will likely be needed to obtain the required purity.
Table 2 Post-separation analysis and requirements
Methods |
Applications |
Most important sample requirement |
Immunofluorescence |
Enumeration and detection |
Yield |
FISH |
Characterization and detection |
Yield |
Genomic profiling and DNA sequencing |
Characterization of genomic instability and relevant mutation |
Purity |
Gene expression and transcription profiling |
Characterization of tumor marker overexpression |
Purity |
PCR |
Characterization of tumor marker overexpression |
Purity |
ELISA |
Characterization of tumor marker overexpression |
Yield and purity |
Deformability measurement |
Biomechanical characterization |
Viability |
Size and morphology analysis |
Biomechanical characterization |
Yield |
6. Conclusions and outlook
The isolation of CTCs by affinity capture has been shown to be of tremendous value in using CTC counts to predict disease outcome, where the detection of >5 CTCs in a 7.5 ml blood sample is associated with unfavorable clinical outcomes.5 This approach, however, requires the expression of specific antigenic markers on the surface of CTCs, which in the case of established techniques is the epithelial antigen, EpCAM. A key limitation of this approach is that when CTCs lose their expression for epithelial antigens, such as when cancer cells undergo epithelial-to-mesenchymal (EMT) transition, affinity methods will fail to capture these cells.116,117 In fact, since EMT is a necessary part of metastasis, the subpopulation of CTCs that have lost or have reduced expression for epithelial markers may in fact be the most important for disease outcome.
Advances in microfabrication have enabled the development of label-free technologies with improved sensitivity and efficiency. In this review, we divided these technologies into three classes including filtration, hydrodynamic chromatography, and dielectrophoresis. These technologies enable separation based on combinations of size, deformability, and electrical polarizability. As variants of all three CTC separation strategies are currently evolving rapidly, we formulated a set of performance specifications, including yield, purity, enrichment, and throughput that could be used to evaluate and compare the capabilities of different strategies.
The potential for label-free separation methods arises from the likely physical differences between CTCs and leukocytes. Specifically, both historical literature and recent studies show that compared to leukocytes, CTCs have a greater specific gravity, larger average diameter, and distinct internal structure. Furthermore, CTCs are believed to be less deformable than leukocytes because of their greater N/C ratio and from the fact that cultured cancer cells are less deformable than leukocytes. The goal of label-free separation technologies is then to harness one or more of these physical differences to achieve selective separation. A key challenge, however, is that the inherent heterogeneity of CTCs causes significant overlap of their physical properties with leukocytes. This characteristic combined with the fact the leukocytes vastly outnumber CTCs, will likely result in significant leukocyte contamination in the output sample. The use of cultured cancer cells as models for CTCs further clouds this picture because cultured cancer cells are more physically distinct from leukocytes than CTCs, which means that separation systems tested using cultured cancer cells are likely to overestimate system performance.
The analysis of CTCs following separation will ultimately determine the suitability of a separation technology and the relative importance of various performance specifications. For example, to enumerate CTCs, the key parameter is yield, which must be as high as possible in order to determine, with statistical certainty, the concentration of these cells in patient blood. Since CTCs are identified using immunofluorescence, purity and enrichment only need to be good enough for the output cells to be imaged and distinguished in a reasonable amount of time. For genetic profiling of CTCs, such as gene expression analysis and genome sequencing, yield is of less value. Instead, sample purity is extremely important because of the need for high purity template material with minimal contamination. Finally, to propagate CTCs in culture or in xenograft models to test drug efficacy, cell viability is the most critical factor. These examples provide only some general directions. Much of the future work in this field may be related to the analysis of these very rare cells.
Ultimately, label-free separation methods are likely to provide more sensitive CTC isolation with a greater variety of analysis options. These capabilities are likely to provide researchers and clinicians with new ways to study various cancers, permit detection of cancer metastasis at earlier stages, and provide more accurate assessments of disease status to personalize treatment and evaluate drug efficacy.
Acknowledgements
This work was supported by grants from Natural Science and Engineering Research Council of Canada, Canadian Institutes of Health Research, Prostate Cancer Canada, Vancouver Prostate Centres's Translational Research Initiative for Accelerated Discovery and Development, and the Engineers-in-Scrubs training program at UBC.
References
- T. R. Ashworth, Med. J. Aust., 1869, 14, 146–149 Search PubMed.
- L. Norton and J. Massagué, Nat. Med., 2006, 12, 875–878 CrossRef CAS PubMed.
- M. Mego, S. A. Mani and M. Cristofanilli, Nat. Rev. Clin. Oncol., 2010, 7, 693–701 CrossRef CAS PubMed.
- L. A. Devriese, A. J. Bosma, M. M. van de Heuvel, W. Heemsbergen, E. E. Voest and J. H. M. Schellens, Lung Cancer, 2012, 75, 242–247 CrossRef CAS PubMed.
- W. J. Allard, J. Matera, M. C. Miller, M. Repollet, M. C. Connelly, C. Rao, A. G. J. Tibbe, J. W. Uhr and L. W. M. M. Terstappen, Clin. Cancer Res., 2004, 10, 6897–6904 CrossRef PubMed.
- M. C. Miller, G. V. Doyle and L. W. M. M. Terstappen, J. Oncol., 2010, 2010, 617421 CrossRef PubMed.
- A. L. Allan and M. Keeney, J. Oncol., 2010, 2010, 1–10 CrossRef PubMed.
- K. Polyak and R. A. Weinberg, Nat. Rev. Cancer, 2009, 9, 265–273 CrossRef CAS PubMed.
- A. C. Small, Y. Gong, W. K. Oh, S. J. Hall, C. J. M. van Rijn and M. D. Galsky, J. Urol., 2012, 188, 21–26 CrossRef PubMed.
- S. Sleijfer, J.-W. Gratama, A. M. Sieuwerts, J. Kraan, J. W. M. Martens and J. A. Foekens, Eur. J. Cancer, 2007, 43, 2645–2650 CrossRef CAS PubMed.
- R. Jung, W. Krüger, S. Hosch, M. Holweg, N. Kröger, K. Gutensohn, C. Wagener, M. Neumaier and A. R. Zander, Br. J. Cancer, 1998, 78, 1194–1198 CrossRef CAS.
- D. R. Gossett, W. M. Weaver, A. J. Mach, S. C. Hur, H. T. K. Tse, W. Lee, H. Amini and D. Di Carlo, Anal. Bioanal. Chem., 2010, 397, 3249–3267 CrossRef CAS PubMed.
- S. Nagrath, L. V. Sequist, S. Maheswaran, D. W. Bell, D. Irimia, L. Ulkus, M. R. Smith, E. L. Kwak, S. Digumarthy, A. Muzikansky, P. Ryan, U. J. Balis, R. G. Tompkins, D. A. Haber and M. Toner, Nature, 2007, 450, 1235–1239 CrossRef CAS PubMed.
- J. Autebert, B. Coudert, F.-C. Bidard, J.-Y. Pierga, S. Descroix, L. Malaquin and J.-L. Viovy, Methods, 2012, 57, 297–307 CrossRef CAS PubMed.
- E. D. Pratt, C. Huang, B. G. Hawkins, J. P. Gleghorn and B. J. Kirby, Chem. Eng. Sci., 2011, 66, 1508–1522 CrossRef CAS PubMed.
- U. Dharmasiri, M. A. Witek, A. A. Adams and S. A. Soper, Annu. Rev. Anal. Chem., 2010, 3, 409–431 CrossRef CAS PubMed.
- J. Chen, J. Li and Y. Sun, Lab Chip, 2012, 12, 1753 RSC.
- A. A. S. Bhagat, H. Bow, H. W. Hou, S. J. Tan, J. Han and C. T. Lim, Med. Biol. Eng. Comput., 2010, 48, 999–1014 Search PubMed.
- I. Cima, C. Wen Yee, F. S. Iliescu, W. Min Phyo, K. Hon Lim, C. Iliescu and M. Han Tan, Biomicrofluidics, 2013, 7, 011810–011810 CrossRef –16.
- Y. Dong, A. M. Skelley, K. D. Merdek, K. M. Sprott, C. Jiang, W. E. Pierceall, J. Lin, M. Stocum, W. P. Carney and D. A. Smirnov, J. Mol. Diagn., 2013, 15, 149–157 CrossRef PubMed.
- S. K. Arya, B. Lim and A. R. A. Rahman, Lab Chip, 2013, 13, 1995–2027 RSC.
- M. Yu, S. Stott, M. Toner, S. Maheswaran and D. A. Haber, J. Cell Biol., 2011, 192, 373–382 CrossRef CAS PubMed.
- Y.-F. Sun, X.-R. Yang, J. Zhou, S.-J. Qiu, J. Fan and Y. Xu, J. Cancer Res. Clin. Oncol., 2011, 137, 1151–1173 CrossRef PubMed.
- E. S. Lianidou and A. Markou, Clin. Chem., 2011, 57, 1242–1255 CAS.
- C. Alix-Panabières and K. Pantel, Clin. Chem., 2013, 59, 110–118 Search PubMed.
- K. Schleip, Ztschr Klin Med, 1906, 261 Search PubMed.
- G. R. Ward, The Lancet, 1913, 676–677 CrossRef.
- H. Marcus, Ztschr Krebsforsch, 1917, 217 CrossRef.
- E. H. Pool and G. R. Dunlop, Am. J. Cancer, 1934, 21, 99–102 CrossRef.
- G. Papanicolaou and H. Traut, J. Am. Med. Assoc., 1943, 123, 178–178 Search PubMed.
- W. H. Cole, D. Packard and H. W. Southwick, J. Am. Med. Assoc., 1954, 155, 1549–1553 CrossRef CAS.
- H. C. Engell, Ugeskr. Laeger, 1955, 117, 822–823 CAS.
- O. Eriksson, Cancer, 1962, 15, 171–175 CrossRef CAS.
- S. H. Seal, Cancer, 1959, 12, 590–595 CrossRef CAS.
- R. F. Alexander and A. I. Spriggs, J. Clin. Pathol., 1960, 13, 414–424 CrossRef CAS.
- T. M. Scheinin and A. P. Koivuniemi, Cancer, 1962, 15, 972–977 CrossRef CAS.
- S. Roberts, A. Watne, R. McGrath, E. McGrew and W. H. Cole, Obstet. Gynecol. Surv., 1958, 13, 745–747 Search PubMed.
- R. A. Malmgren, in Mechanisms of Invasion in Cancer, ed. P. Denoix, Springer Berlin Heidelberg, Berlin, Heidelberg, 1967, pp. 108–117 Search PubMed.
- H. B. Hsieh, D. Marrinucci, K. Bethel, D. N. Curry, M. Humphrey, R. T. Krivacic, J. Kroener, L. Kroener, A. Ladanyi, N. Lazarus, P. Kuhn, R. H. Bruce and J. Nieva, Biosens. Bioelectron., 2006, 21, 1893–1899 CrossRef CAS PubMed.
- D. Marrinucci, K. Bethel, R. H. Bruce, D. N. Curry, B. Hsieh, M. Humphrey, R. T. Krivacic, J. Kroener, L. Kroener, A. Ladanyi, N. H. Lazarus, J. Nieva and P. Kuhn, Hum. Pathol., 2007, 38, 514–519 CrossRef PubMed.
- S. Park, R. R. Ang, J. Bazov, K. N. Chi, P. C. Black and H. Ma, Submitted, 2013 Search PubMed.
- K.-A. Hyun, K. Kwon, H. Han, S.-I. Kim and H.-I. Jung, Biosens. Bioelectron., 2013, 40, 206–212 CrossRef CAS PubMed.
- D. Marrinucci, K. Bethel, M. Luttgen, R. H. Bruce, J. Nieva and P. Kuhn, Arch. Pathol. Lab. Med. Online, 2009, 133, 1468–1471 Search PubMed.
- S. Meng, D. Tripathy, E. P. Frenkel, S. Shete, E. Z. Naftalis, J. F. Huth, P. D. Beitsch, M. Leitch, S. Hoover, D. Euhus, B. Haley, L. Morrison, T. P. Fleming, D. Herlyn, L. W. M. M. Terstappen, T. Fehm, T. F. Tucker, N. Lane, J. Wang and J. W. Uhr, Clin. Cancer Res., 2004, 10, 8152–8162 CrossRef PubMed.
- D. Marrinucci, K. Bethel, D. Lazar, J. Fisher, E. Huynh, P. Clark, R. Bruce, J. Nieva and P. Kuhn, J. Oncol., 2010, 2010, 1–7 CrossRef PubMed.
- P. R. C. Gascoyne, S. Shim, J. Noshari, F. F. Becker and K. Stemke-Hale, Electrophoresis, 2013, 34, 1042–1050 CrossRef CAS PubMed.
- Q. Guo, S. Park and H. Ma, Lab Chip, 2012, 12, 2687 RSC.
- M. J. Rosenbluth, W. A. Lam and D. A. Fletcher, Biophys. J., 2006, 90, 2994–3003 CrossRef CAS PubMed.
- S. E. Cross, Y.-S. Jin, J. Rao and J. K. Gimzewski, Nat. Nanotechnol., 2007, 2, 780–783 CrossRef CAS PubMed.
- L. Weiss and G. W. Schmid-Schönbein, Cell Biophys., 1989, 14, 187–215 CAS.
- H. K. Lin, S. Zheng, A. J. Williams, M. Balic, S. Groshen, H. I. Scher, M. Fleisher, W. Stadler, R. H. Datar, Y.-C. Tai and R. J. Cote, Clin. Cancer Res., 2010, 16, 5011–5018 CrossRef CAS PubMed.
- J. G. Moreno, S. M. O'Hara, S. Gross, G. Doyle, H. Fritsche, L. G. Gomella and L. W. M. Terstappen, Urology, 2001, 58, 386–392 CrossRef CAS.
- S. Suresh, Acta Biomater., 2007, 3, 413–438 CrossRef PubMed.
- S. Suresh, Nat. Nanotechnol., 2007, 2, 748–749 CrossRef CAS PubMed.
- J. Guck, S. Schinkinger, B. Lincoln, F. Wottawah, S. Ebert, M. Romeyke, D. Lenz, H. M. Erickson, R. Ananthakrishnan, D. Mitchell, J. Käs, S. Ulvick and C. Bilby, Biophys. J., 2005, 88, 3689–3698 CrossRef CAS PubMed.
- V. Swaminathan, K. Mythreye, E. T. O'Brien, A. Berchuck, G. C. Blobe and R. Superfine, Cancer Res., 2011, 71, 5075–5080 CrossRef CAS PubMed.
- T. Gerhardt, S. Woo and H. Ma, Lab Chip, 2011, 11, 2731 RSC.
- H. M. Ji, V. Samper, Y. Chen, C. K. Heng, T. M. Lim and L. Yobas, Biomed. Microdevices, 2008, 10, 251–257 CrossRef PubMed.
- S. M. McFaul, B. K. Lin and H. Ma, Lab Chip, 2012, 12, 2369 RSC.
- S. J. Tan, R. L. Lakshmi, P. Chen, W.-T. Lim, L. Yobas and C. T. Lim, Biosens. Bioelectron., 2010, 26, 1701–1705 CrossRef CAS PubMed.
- B. Lu, T. Xu, S. Zheng, A. Goldkorn and Y.-C. Tai, in 2010 IEEE 23rd International Conference on Micro Electro Mechanical Systems (MEMS), 2010, pp. 935–938 Search PubMed.
- S. Zheng, H. Lin, J.-Q. Liu, M. Balic, R. Datar, R. J. Cote and Y.-C. Tai, J. Chromatogr., A, 2007, 1162, 154–161 CrossRef CAS PubMed.
- S. Zheng, H. K. Lin, B. Lu, A. Williams, R. Datar, R. J. Cote and Y.-C. Tai, Biomed. Microdevices, 2011, 13, 203–213 CrossRef PubMed.
- J. S. Kuo, Y. Zhao, P. G. Schiro, L. Ng, D. S. W. Lim, J. P. Shelby and D. T. Chiu, Lab Chip, 2010, 10, 837 RSC.
- S. H. Seal, Cancer, 1964, 17, 637–642 CrossRef CAS.
- G. Vona, A. Sabile, M. Louha, V. Sitruk, S. Romana, K. Schütze, F. Capron, D. Franco, M. Pazzagli, M. Vekemans, B. Lacour, C. Bréchot and P. Paterlini-Bréchot, Am. J. Pathol., 2000, 156, 57–63 CrossRef CAS.
- B. K. Lin, S. M. McFaul, C. Jin, P. C. Black and H. Ma, Biomicrofluidics, 2013, 7, 034114–034114 CrossRef –11.
- Y.-C. Ma, L. Wang and F.-L. Yu, Technol. Cancer Res. Treat., 2013, 12, 295–309 Search PubMed.
- I. Desitter, B. S. Guerrouahen, N. Benali-Furet, J. Wechsler, P. A. Jänne, Y. Kuang, M. Yanagita, L. Wang, J. A. Berkowitz, R. J. Distel and Y. E. Cayre, Anticancer Res., 2011, 31, 427–441 Search PubMed.
- E. Lim, A. Tay and A. G. Nicholson, J. Thorac. Oncol. Off. Publ. Int. Assoc. Study Lung Cancer, 2012, 7, e42–43 Search PubMed.
- V. De Giorgi, P. Pinzani, F. Salvianti, J. Panelos, M. Paglierani, A. Janowska, M. Grazzini, J. Wechsler, C. Orlando, M. Santucci, T. Lotti, M. Pazzagli and D. Massi, J. Invest. Dermatol., 2010, 130, 2440–2447 CrossRef CAS PubMed.
- P. Pinzani, C. Scatena, F. Salvianti, E. Corsini, L. Canu, G. Poli, M. Paglierani, V. Piccini, M. Pazzagli, G. Nesi, M. Mannelli and M. Luconi, J. Clin. Endocrinol. Metab., 2013 DOI:10.1210/jc.2013–1396.
- V. Hofman, C. Bonnetaud, M. I. Ilie, P. Vielh, J. M. Vignaud, J. F. Fléjou, S. Lantuejoul, E. Piaton, N. Mourad, C. Butori, E. Selva, M. Poudenx, S. Sibon, S. Kelhef, N. Vénissac, J.-P. Jais, J. Mouroux, T. J. Molina and P. Hofman, Clin. Cancer Res. Off. J. Am. Assoc. Cancer Res., 2011, 17, 827–835 CrossRef CAS PubMed.
- L. S. Lim, M. Hu, M. C. Huang, W. C. Cheong, A. T. L. Gan, X. L. Looi, S. M. Leong, E. S.-C. Koay and M.-H. Li, Lab Chip, 2012, 12, 4388 RSC.
- S. Zheng, H. Lin, J.-Q. Liu, M. Balic, R. Datar, R. J. Cote and Y.-C. Tai, J. Chromatogr., A, 2007, 1162, 154–161 CrossRef CAS PubMed.
- J. P. Beech, S. H. Holm, K. Adolfsson and J. O. Tegenfeldt, Lab Chip, 2012, 12, 1048 RSC.
- K. Loutherback, J. D'Silva, L. Liu, A. Wu, R. H. Austin and J. C. Sturm, AIP Adv., 2012, 2, 042107 CrossRef PubMed.
- D. Di Carlo, Lab Chip, 2009, 9, 3038–3046 RSC.
- D. W. Inglis, Appl. Phys. Lett., 2009, 94, 013510 CrossRef.
- L. R. Huang, E. C. Cox, R. H. Austin and J. C. Sturm, Science, 2004, 304, 987–990 CrossRef CAS PubMed.
- Z. Liu, F. Huang, J. Du, W. Shu, H. Feng, X. Xu and Y. Chen, Biomicrofluidics, 2013, 7, 011801 CrossRef.
- J. Sun, M. Li, C. Liu, Y. Zhang, D. Liu, W. Liu, G. Hu and X. Jiang, Lab Chip, 2012, 12, 3952 RSC.
- H. W. Hou, M. E. Warkiani, B. L. Khoo, Z. R. Li, R. A. Soo, D. S.-W. Tan, W.-T. Lim, J. Han, A. A. S. Bhagat and C. T. Lim, Sci. Reports, 2013, 3, 1259 CAS.
- S. C. Hur, A. J. Mach and D. Di Carlo, Biomicrofluidics, 2011, 5, 022206 Search PubMed.
- H.-S. Moon, K. Kwon, K.-A. Hyun, T. Seok Sim, J. Chan Park, J.-G. Lee and H.-I. Jung, Biomicrofluidics, 2013, 7, 014105 CrossRef.
- A. A. S. Bhagat, H. W. Hou, L. D. Li, C. T. Lim and J. Han, Lab Chip, 2011, 11, 1870 RSC.
- D. Di Carlo, D. Irimia, R. G. Tompkins and M. Toner, Proc. Natl. Acad. Sci. U. S. A., 2007, 104, 18892–18897 CrossRef CAS PubMed.
- A. A. S. Bhagat, S. S. Kuntaegowdanahalli and I. Papautsky, Lab Chip, 2008, 8, 1906–1914 RSC.
- X.-B. Wang, Y. Huang, P. R. C. Gascoyne, F. F. Becker, R. Hölzel and R. Pethig, Biochim. Biophys. Acta, Biomembr., 1994, 1193, 330–344 CrossRef CAS.
- J. Yang, Y. Huang, X. Wang, X.-B. Wang, F. F. Becker and P. R. C. Gascoyne, Biophys. J., 1999, 76, 3307–3314 CrossRef CAS.
- I. Doh and Y.-H. Cho, Sens. Actuators, A, 2005, 121, 59–65 CrossRef CAS PubMed.
- J. Yang, Y. Huang, X.-B. Wang, F. F. Becker and P. R. C. Gascoyne, Anal. Chem., 1999, 71, 911–918 CrossRef CAS.
- A. Alazzam, I. Stiharu, R. Bhat and A.-N. Meguerditchian, Electrophoresis, 2011, 32, 1327–1336 CrossRef CAS PubMed.
- P. R. C. Gascoyne, J. Noshari, T. J. Anderson and F. F. Becker, Electrophoresis, 2009, 30, 1388–1398 CrossRef CAS PubMed.
- H.-S. Moon, K. Kwon, S.-I. Kim, H. Han, J. Sohn, S. Lee and H.-I. Jung, Lab Chip, 2011, 11, 1118 RSC.
- S. Shim, K. Stemke-Hale, A. M. Tsimberidou, J. Noshari, T. E. Anderson and P. R. C. Gascoyne, Biomicrofluidics, 2013, 7, 011807–011807 CrossRef –12.
- S.-B. Huang, M.-H. Wu, Y.-H. Lin, C.-H. Hsieh, C.-L. Yang, H.-C. Lin, C.-P. Tseng and G.-B. Lee, Lab Chip, 2013, 13, 1371 RSC.
- A. J. Armstrong, M. S. Marengo, S. Oltean, G. Kemeny, R. L. Bitting, J. D. Turnbull, C. I. Herold, P. K. Marcom, D. J. George and M. A. Garcia-Blanco, Mol. Cancer Res., 2011, 9, 997–1007 CrossRef CAS PubMed.
- K. Gravdal, O. J. Halvorsen, S. A. Haukaas and L. A. Akslen, Clin. Cancer Res., 2007, 13, 7003–7011 CrossRef CAS PubMed.
- L. Zhu, P. Gibson, D. S. Currle, Y. Tong, R. J. Richardson, I. T. Bayazitov, H. Poppleton, S. Zakharenko, D. W. Ellison and R. J. Gilbertson, Nature, 2009, 457, 603–607 CrossRef CAS PubMed.
- J. Nieva, M. Wendel, M. Luttgen, D. Marrinucci, L. Bazhenova, A. Kolatkar, R. Santala, B. Whittenberger, J. Burke, M. Torrey, K. Bethel and P. Kuhn, Phys. Biol., 2012, 9, 016004 CrossRef PubMed.
- D. Marrinucci, K. Bethel, A. Kolatkar, M. S. Luttgen, M. Malchiodi, F. Baehring, K. Voigt, D. Lazar, J. Nieva, L. Bazhenova, A. H. Ko, W. M. Korn, E. Schram, M. Coward, X. Yang, T. Metzner, R. Lamy, M. Honnatti, C. Yoshioka, J. Kunken, Y. Petrova, D. Sok, D. Nelson and P. Kuhn, Phys. Biol., 2012, 9, 016003 CrossRef PubMed.
- J. A. Mayer, T. Pham, K. L. Wong, J. Scoggin, E. V. Sales, T. Clarin, T. J. Pircher, S. D. Mikolajczyk, P. D. Cotter and F. Z. Bischoff, Cancer Genet., 2011, 204, 589–595 CrossRef CAS PubMed.
- M. A. Leversha, J. Han, Z. Asgari, D. C. Danila, O. Lin, R. Gonzalez-Espinoza, A. Anand, H. Lilja, G. Heller, M. Fleisher and H. I. Scher, Clin. Cancer Res. Off. J. Am. Assoc. Cancer Res., 2009, 15, 2091–2097 CrossRef CAS PubMed.
- E. Heitzer, M. Auer, C. Gasch, M. Pichler, P. Ulz, E. M. Hoffmann, S. Lax, J. Waldispuehl-Geigl, O. Mauermann, C. Lackner, G. Höfler, F. Eisner, H. Sill, H. Samonigg, K. Pantel, S. Riethdorf, T. Bauernhofer, J. B. Geigl and M. R. Speicher, Cancer Res., 2013, 73, 2965–2975 CrossRef CAS PubMed.
- V. V. Iakovlev, R. S. Goswami, J. Vecchiarelli, N. C. R. Arneson and S. J. Done, Breast Cancer Res. Treat., 2007, 107, 145–154 CrossRef PubMed.
- M. Louha, J. Nicolet, H. Zylberberg, A. Sabile, C. Vons, G. Vona, K. Poussin, M. Tournebize, F. Capron, S. Pol, D. Franco, B. Lacour, C. Bréchot and P. Paterlini-Bréchot, Hepatology, 1999, 29, 879–882 CrossRef CAS PubMed.
- S.-M. Yie, B. Luo, N.-Y. Ye, K. Xie and S.-R. Ye, Clin. Exp. Metastasis, 2006, 23, 279–289 CrossRef CAS PubMed.
- E. A. Punnoose, S. Atwal, W. Liu, R. Raja, B. M. Fine, B. G. M. Hughes, R. J. Hicks, G. M. Hampton, L. C. Amler, A. Pirzkall and M. R. Lackner, Clin. Cancer Res., 2012, 18, 2391–2401 CrossRef CAS PubMed.
- D. Di Carlo, J. Lab. Autom., 2012, 17, 32–42 CAS.
- V. Vadillo-Rodriguez, T. J. Beveridge and J. R. Dutcher, J. Bacteriol., 2008, 190, 4225–4232 CrossRef CAS PubMed.
- H. Engelhardt, H. Gaub and E. Sackmann, Nature, 1984, 307, 378–380 CrossRef CAS.
- U. Zimmermann, U. Friedrich, H. Mussauer, P. Gessner, K. Hamel and V. Sukhorukov, IEEE Trans. Plasma Sci., 2000, 28, 72–82 CrossRef CAS.
- A. Ashkin, J. M. Dziedzic and T. Yamane, Nature, 1987, 330, 769–771 CrossRef CAS PubMed.
- T. Kaneta, J. Makihara and T. Imasaka, Anal. Chem., 2001, 73, 5791–5795 CrossRef CAS.
- P. Paterlini-Brechot and N. L. Benali, Cancer Lett., 2007, 253, 180–204 CrossRef CAS PubMed.
- B. Willipinski-Stapelfeldt, S. Riethdorf, V. Assmann, U. Woelfle, T. Rau, G. Sauter, J. Heukeshoven and K. Pantel, Clin. Cancer Res. Off. J. Am. Assoc. Cancer Res., 2005, 11, 8006–8014 CrossRef CAS PubMed.
|
This journal is © The Royal Society of Chemistry 2014 |