DOI:
10.1039/C4IB00137K
(Paper)
Integr. Biol., 2014,
6, 1058-1068
Elucidation of epithelial–mesenchymal transition-related pathways in a triple-negative breast cancer cell line model by multi-omics interactome analysis†
Received
13th June 2014
, Accepted 5th August 2014
First published on 7th August 2014
Abstract
In life sciences, and particularly biomedical research, linking aberrant pathways exhibiting phenotype-specific alterations to the underlying physical condition or disease is an ongoing challenge. Computationally, a key approach for pathway identification is data enrichment, combined with generation of biological networks. This allows identification of intrinsic patterns in the data and their linkage to a specific context such as cellular compartments, diseases or functions. Identification of aberrant pathways by traditional approaches is often limited to biological networks based on either gene expression, protein expression or post-translational modifications. To overcome single omics analysis, we developed a set of computational methods that allow a combined analysis of data collections from multiple omics fields utilizing hybrid interactome networks. We apply these methods to data obtained from a triple-negative breast cancer cell line model, combining data sets of gene and protein expression as well as protein phosphorylation. We focus on alterations associated with the phenotypical differences arising from epithelial–mesenchymal transition in two breast cancer cell lines exhibiting epithelial-like and mesenchymal-like morphology, respectively. Here we identified altered protein signaling activity in a complex biologically relevant network, related to focal adhesion and migration of breast cancer cells. We found dysregulated functional network modules revealing altered phosphorylation-dependent activity in concordance with the phenotypic traits and migrating potential of the tested model. In addition, we identified Ser267 on zyxin, a protein coupled to actin filament polymerization, as a potential in vivo phosphorylation target of cyclin-dependent kinase 1.
Insight, innovation, integration
This paper demonstrates a method to integrate heterogeneous datasets obtained from different biological levels reaching from genomics, over proteomics, down to the level of site-specific post translational modifications. By combining information from a human PPI-network and kinase-substrate relations, we were able to create one integrated interaction network embracing multiple omics. Data was obtained from a triple-negative breast cancer cell line model to identify functional network modules that reveal differential protein signaling activity related to EMT (epithelial–mesenchymal-transition). We show that analyzing multiple omics data in combination can provide significant biological insights into alterations of disease-related pathways.
|
1 Introduction
Omics research (genomics, proteomics, metobolomics, epigenomics, etc.) has primarily been driven by technical and technological bioanalytical advances over the last decade. These advances include high-throughput methods to conduct large-scale studies (next generation sequencing, shotgun proteomics, gene expression microarrays, etc.) and high resolution mass spectrometry-based methods that provide essential information about an organism's regulatory machinery in its efforts to maintain homeostasis in health and disease. This massive amount of diverse information has facilitated the rise of high-quality and publicly available biological databases, such as The Cancer Genome Atlas (TCGA) that integrate these various aspects to provide a disease-centric view. A fundamental computational approach to combine these various sources with interaction data is differential network mapping.1 The goal is to identify condition-distinctive, functional network modules (small impaired sub-networks) in an organism's global interaction network.2–7 Interaction networks as provided by the BioGRID database8 and the Human Protein Reference Database (HPRD)9 are compendia of pairwise protein interaction studies10,11 and protein complexes12–14 in various organisms. Methods for differential network mapping have traditionally focused on projecting aberrant patterns from gene expression microarray data onto a protein–protein interaction (PPI) network.1,15 It is widely used to overcome single gene function analysis and to identify sets of genes and their concerted disease-associated responses.16 Network modules achieve better performance because a differentially expressed sub-network is considered to be a more robust form to characterizing diseases than individual molecules.17 Additionally, uni-directional analysis based on expression data alone can suffer from low coverage and high false-positive rates or high false-negative rates.17 To alleviate these issues in traditional biomarker research, vastly more integrative approaches are needed to close the gap between computational models and their underlying biological machinery. However, since popular approaches analyze only single omics datasets,2,3,6,7,18 they fail to integrate multiple layers of information provided by different omics technology, e.g. post-translational modifications (PTMs) and protein expression. Achievements in drug design based on single gene targets such as trastuzumab19 which is based on the HER2 gene, also referred to as ErbB-2 or HER2/neu, while successful in principle, have shown overall limited response in patients and emphasize the need for a clearer classification of cancers into biologically meaningful types and subtypes based on molecular categorization.19 This can only be obtained with more complete computational models that link molecular alterations to physiology. Therefore, it is necessary to pinpoint sub-networks of differentially regulated molecular targets in the context of altered pathways due to disease-associated perturbations that allow more accurate interpretations of their interplay. The cell model behind our present study consists of two isogenic cell lines derived as single cell clones from a triple-negative ductal breast carcinoma cell line, HMT3909-S13.20 One of these cell lines (A4) has an epithelial-like morphology, hereafter referred to as HMT-E and the other cell line (G4) exhibits a mesenchymal-like morphology and is hence referred to as HMT-M. Through semi-quantitative mass spectrometry, we compared the proteome as well as the phosphoproteome of HMT-E and HMT-M to elucidate pathways that are differentially regulated between the two cell types of the same genetic background, but differ in epithelial–mesenchymal morphology. Epithelial-to-mesenchymal transition (EMT) is a process in which epithelial cancer cells lose their cell polarity and cell–cell adhesion, and gain mesenchymal features such as migratory and invasive properties. The gene regulatory programs controlling EMT are responsible for the regulation of cell adhesion and signaling pathways associated with migration and invasion.21 In triple-negative breast cancer (TNBC), EMT has been of great interest due to the correlation of EMT with enrichment of the cancer stem-like cell (CSC) subpopulation of the tumors as well as its involvement in several steps of the metastatic process.17 Our cell model provides an optimal setting to study differences in signaling network associated with the epithelial- and mesenchymal-like phenotypes. We developed computational network analysis methods that support the analysis of combined multiple omics studies and applied these methods to gene and protein expression as well as protein phosphorylation data from the cell line model described above. We present TNBC-associated networks and relate them with underlying regulatory events focusing on protein phosphorylation in combination with gene and protein expression.
2 Methods
2.1 Data collection
The present study is based on a mass spectrometry-based comparison on the isogenic human breast cancer cell lines A4 and G4, with A4 representing an epithelial-like and G4 a mesenchymal-like phenotype. A total of three omics datasets were created and analyzed in this study: gene expression, protein expression and protein phosphorylation. While gene expression was measured array-based without subfractions, phosphorylation and protein expression were measured in the membrane fraction (M) and the soluble fraction (S) consisting of non-membranous proteins separately resulting in five different datasets. Each of the non-phospho-fractions were analyzed twice, leaving a non-phospho-M1, -M2, -S1 and -S2. Overall, this lead to a total of seven datasets for bioinformatics analysis: S1, S2, M1, M2, pS, pM and gene expression (Fig. 1 and ESI,† Fig. S4–S6).
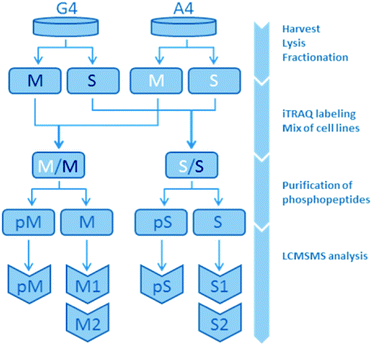 |
| Fig. 1 Organigram of the protein expression and protein phosphorylation measurements. A4 and G4 are the originating cell lines. Compartmental fractionation resulted in membrane (M) and soluble (S) fractions. Phosphopeptide purification followed by liquid chromatography tandem mass spectrometry (LC-MS/MS) finally yield three datasets per compartmental fraction: one phosphopeptide dataset (pM/pS) and two technical replicates per protein expression dataset (M1, M2, S1, S2). Gene expression is not displayed in this figure. | |
2.1.1 Cell lines.
The cell lines A4 and G4, are single cell clones of the TNBC cell line HMT3909S13, which originates from a human primary infiltrating ductal breast carcinoma. Cells were cultured in PureCol-coated (Nutacon) cell culture flasks (Cellstar) in serum-free medium MEBM (Lonza) with growth factors and supplements: SingleQuots Kit 0.1% recombinant human epidermal growth factor (rhEGF), 0.1% insulin, 0.1% Gentamicin Sulfate Amphotericin-B (GA-1000) and 0.1% hydrocortisone (Clonetics, Lonza) and 10 ng mL−1 basic fibroblast growth factor (FGF), 20 ng mL−1 epidermal growth factor (EGF) (Peprotech), 2% B27 (Gibco) and 4 g mL−1 heparin (Sigma). Cells were maintained at 37 °C, 5% CO2 and harvested with Accutase (Millipore).
2.1.2 Sample preparation and mass spectrometry.
2.1.2.1 Samples and analysis pairs.
Four samples were analysed in replicates: A4 and G4 cell line samples were divided into two fractions containing membrane proteins (M) and soluble proteins (S), respectively. These fractions were chemically labeled and corresponding fractions from each cell line were combined. Next, phosphopeptides were purified on titanium dioxide (TiO2), leaving two fractions from each mixed sample, one containing the phosphorylated peptides (pM, pS) and one non-phosphorylated peptides (M, S). Each of these fractions consisted of three biological cell samples, one A4- and two G4-samples, the latter in a biological duplicate for use as an internal control. Overall, both protein expression level and presence of phosphorylations were investigated by liquid chromatography tandem mass spectrometry (LCMSMS).
2.1.2.2 Subcellular fractionation, in solution digestion of proteins and labeling of peptides.
Cells were lysed in ice-cold Na2CO3-buffer, pH 11 (100 mM Na2CO3, 100 M Na-pervanadate, phosphatase inhibitor cocktail 1 + 2 (Sigma)) by sonication followed by incubation at 4 °C for 1 h. Lysates were subjected to ultracentrifugation (Sorvall RC M150S) for 1 hour at 100
000g to separate the samples into membrane and soluble proteins. For digestion of the membrane proteins the membrane pellet was redissolved in 6 M urea/2 M thiourea, 10 mM DTT containing 1 g lysyl endopeptidease (Wako) per 50 g protein and incubated for 3 hours at room temperature. Subsequently, 20 mM iodoacetamide was added and the solution was incubated for 20 min at room temperature in the dark. After incubation, the solution was diluted 10 times with 20 mM triethylammonium bicarbonate (TEAB), pH 7.8, containing 20 g trypsin (Promega) and left at room temperature overnight. Soluble proteins were concentrated on a 10 kDa spin filter (Millipore) to 50 L. A total of 400 L TEAB, pH 7.8, 10 mM DTT was added and the solution was concentrated again to 50 L. After centrifugation, 20 mM iodoacetamide was added and the sample was incubated at room temperature in the dark. After incubation, 50 L TEAB, pH 7.8 was added together with 20 g trypsin (Promega) and the solution was left at room temperature overnight. After digestion of the membrane samples, lipids were precipitated by addition of 2% formic acid (FA) followed by centrifugation 14
000g for 10 min. The peptide concentration of each sample was determined using amino acid composition analysis. Peptides from the four conditions were chemically labeled with iTRAQ® Reagents 4-plex (ABSCIEX, MA) according to the manufacture's protocol. Soluble and membrane samples were labeled with reagent 114–117 (115: A4, 116–117: G4, 114: not used in this study) and then mixed in a 1
:
1
:
1
:
1 ratio. The combined samples were lyophilized to 100 L.
2.1.3 Phosphopeptide enrichment.
The procedure included two rounds of TiO2 phosphopeptides purification separated by SIMAC (sequential elution from IMAC) followed by Micro HPLC HILIC.
2.1.3.1 Phosphopeptide enrichment using TiO2.
The purification of phosphorylated peptides was performed using TiO2 under strong acidic conditions to eliminate non-specific binding.22 Large scale enrichment of phosphopeptides was performed using the TiSH protocol as previously described.23
2.1.3.2 Hydrophilic interaction liquid chromatography (HILIC) – HPLC.
The phosphopeptide and non-modified peptide mixtures were resuspended in 90% ACN, 0.1% TFA and injected onto an in-house packed TSKgel Amide-80 HILIC (Tosoh, 5 m) 320 m × 170 mm HPLC column using an Agilent 1200 HPLC system. The phosphopeptides were eluted using a gradient from 90% ACN, 0.1% TFA to 60% ACN, 0.1% TFA over 35 min at a flow rate of 6 L min−1. Fractions were automatically collected at 1 min intervals after UV detection at 210 nm and combined into 10 to 12 fractions according to UV detection. All fractions were dried by vacuum centrifugation.
2.1.3.3 HPLC-MS/MS analyses.
Samples were separated using nano HPLC as described above. Phosphopeptide and non-modified peptide fractions were analyzed in duplicates by nano-LC MSMS analysis using a Dionex UltiMate 3000 nano HPLC coupled to a Thermo Scientific Orbitrap Q-Exactive mass spectrometer (Thermo Scientific, Bremen, Germany). The samples were redissolved in 10 L 0.1% TFA and loaded onto a custom made fused capillary pre-column (2 cm length, 360 m OD, 75 m ID) with a flow of 5 L per minutes for 7 minutes. Trapped peptides were eluted onto a custom made fused capillary column (20 cm length, 360 m outer diameter, 75 m inner diameter) packed with ReproSil Pur C18 3 m resin (Dr Maish, Ammerbuch-Entringen, Germany) with a flow of 300 nl per minute using a linear gradient from 95% solution A (0.1% formic acid) to 35% B (100% acetonitrile in 0.1% formic acid) over 80 min or 120 min followed by 10 min at 90% B and 14 min at 98% A. Mass spectra were acquired in positive ion mode applying automatic data-dependent switch between one Orbitrap survey MS scan in the mass range of 350 to 1200 m/z followed by HCD fragmentation and Orbitrap detection of the twelve most intense ions from the MS scan. Target value in the Orbitrap for MS scan was 1
000
000 ions at a resolution of 70
000 at m/z 200 and target value for MSMS scans was 500
000 at a resolution of 17
500 at m/z 200. Fragmentation in the HCD cell was performed at normalized collision energy of 30 eV for the iTRAQ®-labeled peptides. The isolation window was 1.5 Da and the ion selection threshold was set to 77
000 counts. Selected sequenced ions were dynamically excluded for 60 seconds. Maximum injection times were set to 100 ms for MS scans and 65 ms (120 min gradient) or 200 ms (80 min gradient) for MSMS scans.
2.1.3.4 Database searches: protein identification.
The generated peak lists (mgf files) were processed using the Proteome Discover 1.4.0.288 software integrated with the MASCOT (version 2.4) and the SEQUEST database search program. The search parameters were set to: precursor mass tolerance 5 ppm, fragment mass tolerance 0.1 Da, trypsin digestion with two missed cleavage allowed, fixed lysine and N-terminal iTRAQ®4-plex, and carbamidomethyl (C). For variable modifications methionine oxidation and deamidation (N) was chosen as well as tyrosine, serine, and threoninie phosphorylations. Tandem mass spectra were searched against the Swissprot restricted to Homo sapiens (downloaded October 25th 2012, 84
721 entries). The searches were performed with the minimum requirement of two unique peptides for protein identification and one unique peptide for phosphopeptide identification filtered to 5% peptide FDR with a decoy approach using percolator.
2.1.4 Gene expression analysis.
2.1.4.1 Cell harvest.
Upon havest, cells were rinsed with phosphate buffered saline (PBS) pH 7.4 (Gibco, Invitrogen) and detached from the culture flask by incubation with Accutase (Millipore) 10 min. according to the manufacture guideline.
2.1.4.2 Gene expression analysis.
mRNA was purified by TRIzol followed by ethanol precipitation. Each cell line in five biological replicates was analyzed on GeneChip Human Gene 1.0 ST Arrays (Affymetrix, Santa Clara, CA, USA). Microarray data were normalized and processed using the Partek Genomics Suite (false discovery rate [FDR] 0.01) (Gene Expression Omnibus [GEO] accession number GSE32455).
2.2 Data pre-processing
2.2.1 Data set.
In the case-control setting we determined the fold changes in phosphorylation and protein expression between the two cell lines A4 and G4 using a typical two-fold change threshold. Thus, a protein was considered to exhibit altered expression if the difference in expression level between A4 and G4 was at least ≤2-fold. Next, we discretized both technical replicates to a m × n binary matrix with m being the number of measured proteins (rows) and n being the number of technical replicates (columns). In this matrix a profile (row) consisting of a set of binary values is assigned to each protein with 1 indicating whether the protein was differentially expressed or phosphorylated in this replicate and a 0 otherwise. Finally, both replicates were merged into a single column. We used a contradiction solver for all possible combinations that allowed us to merge the two replicates into a m × 1 matrix. Table 1 shows the solving scheme. This pipeline of pre-processing steps is applied iteratively to both the protein expression and phosphorylation data creating one matrix per fraction (M, S, pM, pS). These matrices are called “indicator matrices”.6,24
Table 1 Resolving contradictions between replicates
Expression of protein Pn in replicate R1 |
Expression of protein Pn in replicate R2 |
Result |
Up |
Up |
Up |
Up |
0 |
Up |
Up |
Down |
0 |
0 |
Up |
Up |
0 |
0 |
0 |
0 |
Down |
Down |
Down |
Up |
0 |
Down |
0 |
Down |
Down |
Down |
Down |
2.2.2 Hybrid network construction.
The binary matrices could then be projected onto the underlying global interaction network in a network enrichment scheme. Since a regular PPI-network does not include phosphorylation events we augmented the human PPI-network from the latest version of the HPRD9 to resemble common protein–protein interactions with several public resources of the human phosphoproteome/phosphorylation network to create a heterogeneous phosphorylation/protein–protein interaction network. These resources consist of experimentally validated and predicted phosphorylation events and phosphorylation site information and include PhosphoNetworks25 which is based on the CEASAR (Connecting Enzymes And Substrates at Amino acid Resolution) method,26 PhosphoSitePlus,27 PhosphoELM28 and the phosphorylation information from the post-translational modifications (PTMs) from HPRD.29 Duplicated interactions (edges) from overlapping parts were retained so that consensus information remains available. Fig. 5a illustrates the annotation of edges reported by multiple databases. The resulting network is the union of all above-mentioned sources. Table 2 shows the edge-contributions of each of these databases.
Table 2 Complementary information presenting the edge contribution of each source database to both the extracted parent network and the full underlying hybrid network which represents the union of all sources. “EC” stands for edge contribution. The extracted parent network consists of 2636 edges/1002 nodes and the full network consists of 44
320 edges/9957 nodes in total
Source database name |
EC in parent network total/(unique) |
EC in full network total/(unique) |
HPRD |
1984/(1688) |
36 811/(34 636) |
HPRD_PTM |
247/(0) |
1808/(11) |
PhosphoNetworks |
250/(171) |
4361/(3636) |
PhosphoSitePlus |
669/(422) |
4050/(2474) |
PhosphoELM |
104/(6) |
1700/(1032) |
2.3 Differential network mapping and sub-network extraction
We determined for each node in the network whether it was aberrant or not based on its differential indication and afterwards we extracted the altered pathways. The KeyPathwayMiner scheme requires the estimation of two configurable parameters: k denotes the number of non-differential proteins (nodes) in a sub-network solution and l denotes the number of cases (biological replicates) in which a protein was allowed to be non-differential before overall being tagged as a non-differential node in the network (Fig. 2a). Whenever the profile over all replicates fulfilled the l parameter, the node was tagged as differential. This procedure was repeatedly applied to each data set with individual parameterization determined specifically for each data set while k was a global parameter only effecting the number of exception nodes in a solution. Before finally tagging a node the results were combined using logical operators (AND, OR, XOR) (Fig. 2b). The operator type as well as the order in which they are applied during the data set-linkage are user-defined. After nodes had been tagged according to the data that is projected onto the network (Fig. 2c) sub-networks that fulfilled all parameter settings were identified and extracted (Fig. 2d).
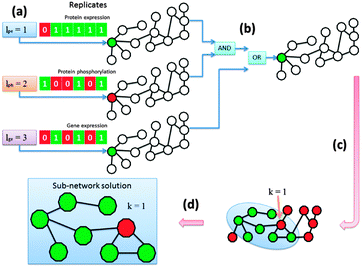 |
| Fig. 2 Schematic representation of the general workflow. All nodes in the network show either aberrant or normal characteristic in the data sets using individual parameterization. In case of multiple data sets the user can specify logical operators and their order of appliance. A network enrichment algorithm computes condition-specific key pathways in the underlying biological network. | |
2.3.1 Parameter adjustment.
We used a linear regression model to identify the optimal value for k (Fig. 3). All sub-network solutions were computed for various values of k. The biggest positive residuals represented the parameter values that maximized the size of the sub-network the most in increments of five while optimizing the trade-off between specificity and sensitivity at the same time. The regression suggested a gradient of 3.86 indicating that on the interval k = 0 to k = 100 the sub-network size increased by an average of 3.86 nodes for each non-differential node added to the network. A value of k = 46 had the highest positive residual and maximized the size of the resulting sub-network (red regression line). However, while incrementing k by one between k = 24 and k = 46 the sub-network size increased linearly (blue line). Each non-differential node added to the sub-network solution allowed to connect three differential nodes. While the sensitivity, i.e. many differential nodes in the network, increased, the specificity, i.e. few non-differential nodes in the network, decreased. Based on these findings k was set to 32 as a trade-off between sensitivity and specificity. It is important to note that this method of optimizing k can be described as adaptation and optimization to network topology for this particular set of indicator matrices and underlying network. Modifications to the matrices, e.g. due to changes in thresholds, may result in different optimal values of k. For case-exceptions previous publications of the “KeyPathwayMiner” suggested to set l to a value corresponding to a percentage of available cases. Our data consisted of two biological replicates that were finally merged, thereby allowing us to set l to 0 at all times resulting in improved comparability of the sub-network solutions.
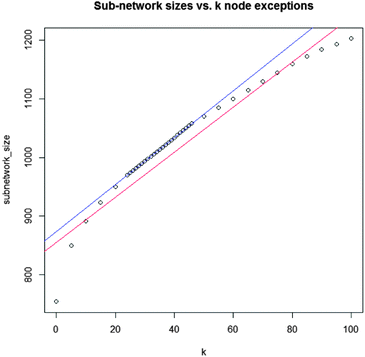 |
| Fig. 3 Regression model for k parameter adjustment. Between k = 24 and k = 46 the network size increases linearly with k. Data points are identified by computing real solution networks for each variation of k. | |
2.3.1.1 Linking omics data sets via logical operators.
The different omics data sets can be linked using common logical operators “AND”, “OR” and “XOR”. The order and combination of these operators severely impacts the way nodes are tagged throughout the enrichment step where data is projected onto the network. Accordingly, sub-network solutions vary immensely and their interpretation needs to be adapted. The investigated biological question determines the feasibility of combinations of logical links. We computed sub-networks for many different linkages, however, biological analysis is based on a sub-network generated by linking data sets with “OR” operators.
3 Results and discussion
We computed five indicator matrices for differentiation between cellular compartments and differential regulation (phosphorylation in soluble fraction, phosphorylation in membrane fraction, protein expression in soluble fraction, protein expression in membrane fraction, gene expression). Next we combined them with logical “OR” operators. We used a linear regression scheme to adjust the k parameter in a way that optimized the sub-network. We compiled a hybrid protein interaction-network that comprises the PPI network from HPRD9 complemented with phosphorylation events from PhosphoNetworks,25 PhosphoSitePlus,27 PhosphoELM28 and PTMs from HPRD.29
3.1 Phenotype-associated sub-network
The differential network enrichment identified a TNBC-associated parent sub-network for further dissection into small EMT-associated sub-sub-networks (cytoscape session file, ESI†). It shows a core interactome of proteins that are differential between A4 and G4. A GO-term enrichment was used for functional annotation of all proteins in the network and we compared this set of proteins contained in this differential network to known breast cancer-related genes obtained from the Ingenuity Pathway Analysis (IPA) database.
Testing the entire hybrid network we found that 1090 of 9957 proteins (10.9%) are breast cancer-related as classified by IPA. The coarse approach linking the different omics data sets by logical “OR” operators produced a parent network with 187 breast cancer-related proteins from a total of 1002 proteins (18.7%) displaying a higher breast cancer-related specificity. Small sub-networks were extracted from this parent network (Fig. 4–6 as well as ESI,† Fig. S1–S3 and complementary Tables S1, S3–S5). Even though the parent network allowed for 32 nodes (k = 32) which were not measured as differential in any of the omics datasets, these small networks were also contained in parent networks we computed for lower values of k up to k = 0 with a single exception of ESR1 in the Src-centric network (Fig. 4). Hence, the discussed small networks are core-structures in the parent network and robust against sub-optimal values of k. To pinpoint signaling of special interest in the conducted network we established a set of criteria to dissect extracted sub-networks focusing on hub-nodes. The protein candidates must
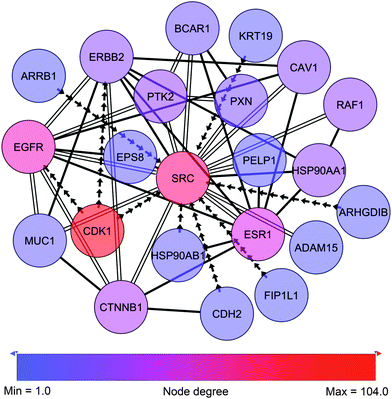 |
| Fig. 4 Src-centric network. Solid edges represent PPIs. Adjacent nodes show BRCA-related interactors as classified by IPA. Arrows represent phosphorylation and point from kinase to substrate. Double edges represent multiple edges reported by HPRD and at least one phospho-database. Node coloring represents a continuous mapping by node degree in the parent network where blue indicates a low and red indicates a high node degree. | |
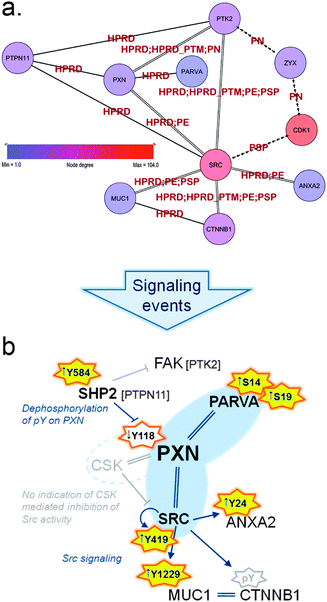 |
| Fig. 5 (a) Sub-sub-network as extracted from the TNBC-associated parent network representing signaling through paxillin and Src. Arrowed edges represent phosphorylation, double edges represent a normal protein–protein interaction as well as phosphorylation and normal edges represent protein–protein interactions only. Edge labels indicate the database that reported each particular PPI or kinase–substrate relation. Zyxin (ZYX) is displayed to illustrate the close connection to the Zyxin-centric network (Fig. 6). (b) Schematic representation of signaling in the HMT-M cell line compared to HMT-E around paxillin (PXN) and the hub-node c-Src (SRC). Orange stars indicate activating phosphorylation sites present in data, yellow fill: activation occur in HMT-M, no fill: less active in HMT-M; arrows in front of phosphosites indicate up or down regulated, respectively, in HMT-M compared to HMT-E. Grey star border: site is not present in data. Interactions are indicated as follows, arrow: activation; stump arrow: inhibition; double strand: complexes with. Nodes connected with blue interaction are both found in data; gray indications are predictions, not directly found in data. | |
• exhibit differentially expressed phosphorylation or protein/gene expression
• be a kinase
• have a high number of phosphorylation edges in the network
• show enriched interactions with breast cancer related genes (as defined by IPA)
The top 16 hub-nodes were CDK2, CDK1, PRKCA, PRKACA, YWHAG, SRC, AKT1, CSNK2A1, TP53, MAPK1, MAPK3, EGFR, CHEK1, ESR1, PRKCD, SMAD2 (ESI,† Tables S1 and S2). Applying the above-mentioned criteria we refined this list to CDK1, AKT1, SRC, CSNK2A1, TP53, MAPK1, MAPK3, EGFR and PRKCD. To further narrow the targets for closer investigation we determined the proteins that were involved in cellular movement, as this is a function closely related to EMT and focal adhesion. We adapted the inclusion criteria function provided by IPA, dividing cellular movement into three main categories: invasion, migration and a general category of cell movement. Applying these criteria, SRC together with EGFR stood out as high degree nodes with an overall total of most interacting proteins involved in cellular movement both in numbers and relative to the size of the hubs (Table 3). As a result we picked SRC for further investigation having a higher degree (75) and a higher total amount of interacting proteins that are involved in cellular movement (44 out of 75) as determined by IPA.
Table 3 Statistics for top candidates for closer examination whose interactors are enriched in phosphorylation signaling and breast cancer related proteins as classified by IPA. The total depicts how many interaction partners were categorized as being involved in cell movement, invasion and migration respectively while the percentage puts this total relative to the total number of observed interactors
Rank |
Gene name |
UniProtKB accession |
Node degree |
Cell movement total/(%) |
Invasion total/(%) |
Migration total/(%) |
1 |
CDK1 |
P06493 |
95 |
24/(25) |
20/(21) |
22/(23) |
2 |
SRC |
P12931 |
75 |
44/(59) |
28/(37) |
41/(55) |
3 |
AKT1 |
P31749 |
70 |
23/(33) |
18/(26) |
21/(30) |
4 |
CSNK2A1 |
P68400 |
68 |
22/(32) |
17/(25) |
22/(32) |
5 |
TP53 |
P04637 |
65 |
25/(38) |
15/(23) |
22/(34) |
6 |
MAPK1 |
P28482 |
62 |
27/(44) |
23/(37) |
23/(37) |
7 |
MAPK3 |
P27361 |
59 |
25/(42) |
18/(31) |
23/(39) |
8 |
EGFR |
P00533 |
58 |
37/(64) |
30/(52) |
34/(59) |
Therefore, we more closely examined the signaling surrounding this node by extracting a SRC-centric sub-sub-network to highlight phospho-interactome information around the role of the proto-oncogene tyrosine-protein kinase Src (c-Src) in cellular movement (Fig. 4 and ESI,† Fig. S1 and Tables S1 and S3). Part of this c-Src-centric network is the intracellular scaffold protein paxillin which is a central player in focal adhesion and cytoskeletal rearrangement.
During EMT, the adhesive epithelial phenotype governed by a keratin-rich network connected to adherence junctions shift towards a predominantly focal adhesion network dominated by vimentin.30 Indeed, this difference in protein expression is also present when comparing HMT-E, high in keratins, to HMT-M with a higher level of vimentin.20 Focal adhesion is the adhesion of cells to the extracellular matrix (ECM) mediated by focal adhesion complexes present at the tip of extending protrusions, such as filopodia and lamellipodia. At the site of focal adhesion, bundles of actin filaments are anchored to integrins and proteins are gathered that either link the actin cytoskeleton to these membrane receptors or function as signaling molecules in downstream integrin-mediated pathways.31 Hence, focal adhesion dynamics plays a central part in cytoskeletal rearrangement and cellular motility, including cancer cell migration and invasion.
Paxillin associates with a variety of proteins to modulate cytoskeletal reorganization during the dynamic process of cell adhesion and migration.32 Within the present sub-network we find a subset of regulated proteins associated with the paxillin scaffold complex and implicated in the dynamic process of focal adhesion (Fig. 5a). These proteins are connected through protein–protein interactions and phosphorylation events and play important roles in the complex regulation of cell motility.
Due to the complexity and level of detail of the generated network, using the proteome, as well as the phosphoproteome and integrating PPI-databases with phosphorylation site-specific databases, we identified key regulatory phosphorylation sites involved in focal adhesion and migration as well as the up-stream kinases for several of these sites.
As part of this paxillin network (Fig. 5 and ESI,† Fig. S2 and Tables S1 and S4) we identified alpha-parvin (PARVA), which has been shown to bind directly to paxillin and F-actin and to co-localize with paxillin at the leading edge of migrating cells.33 PARVA has several serine phosphorylation (pSer) sites in the N-terminal that contributes to regulation of cell spreading and migration. N-terminal pSer of PARVA have been reported to be necessary for efficient Src-matrix metalloprotease (MMP)-driven degradation of extracellular matrix (ECM) and to be elevated in triple-negative invasive breast cancer cells (MDA-MB-231) compared to normal cells (MCF10A).34 In addition, it has been reported that cells shifts towards a more mesenchymal-like morphology upon phosphorylation of PARVA N-terminal serines.35 In accordance with these findings, we found PARVA was extensively phosphorylated on two N-terminal serine residues (Ser14 and Ser19) in HMT-M compared to HMT-E.
A key player in regulation of cell migration is the tyrosine-protein phosphatase non-receptor type 11 (PTPN11) also called SHP2. SHP2 is a cytoplasmic TPTase with a narrow substrate specificity,36 responsible for tyrosine dephosphorylation of several proteins involved in migratory processes, amongst these the focal adhesion kinase (FAK) and paxillin.37 FAK is a major player in cytoskeletal rearrangement and found to complex with paxillin.32 Despite being present in the sub-network, FAK activity cannot be unambiguously determined from the present data. However, there is clear indications that paxillin phosphorylation is under the control of SHP2 in our cell lines, as the presence of active SHP2, bearing a phosphorylation at Tyr58438 in HMT-M, correlates with low levels of paxillin phosphorylation on Tyr118, a site regulating signaling downstream of paxillin.39,40 Dephosphorylation of paxillin Tyr118 is important for another key regulatory protein found in complex with paxillin, namely c-Src. c-Src activity is in part governed by its binding to paxillin, as paxillin phosphorylated on Tyr118 also recruits the inhibitor of c-Src tyrosine kinase activity, tyrosine–protein kinase CSK (CSK). Binding of CSK to paxillin brings this kinase in close proximity to its substrate c-Src, thereby enabling phosphorylation of the c-Src inhibitory phosphotyrosine, Tyr530. Phosphorylation of Tyr530 hinders c-Src autophosphorylation on its activating site (Tyr419), thus abolishing signaling downstream of c-Src.41 CSK was not present in the parent network, but high levels of c-Src autophosphorylation at Tyr419 in HMT-M, combined with the lower levels of paxillin Tyr118 phosphorylation in this cell line, suggest that the c-Src activity is high in HMT-M vs. HMT-E cells. c-Src is a member of the Src kinase family of tyrosine protein kinases, regulating intracellular signaling pathways through activated transmembrane growth factor and cytokine receptors. Consequently, Src family kinases modulated signaling cascades a wide range of which are implicated in oncogenic processes, such as cell survival, differentiation, adhesion, migration and invasion.42 In breast cancer, increased activation of Src tyrosine kinase activity is associated with the metastatic process.43 c-Src activity is known to cause phosphorylation of various proteins involved in cytoskeletal rearrangement and focal adhesion, such as FAK,44 caveolin-1 (CAV1),45 Cortactin (CTTN),46 beta-catenin,47 mucin-1 (MUC1)48 and Annexin A2 (ANXA2),49 thereby playing a central role in modulation of the adhesive phenotype of epithelial cells. All of the above-mentioned proteins are present in our sub-network and several c-Src-mediated phosphorylations were identified in our data. As further support of the notion of increased c-Src activity in HMT-M vs. HMT-E, we found higher phosphorylation of Tyr1229 on MUC1 cytoplasmic tail and Tyr24 of ANXA2 in HMT-M compared to HMT-E. ANXA2 is a calcium-regulated membrane-binding protein that binds to F-actin, one of the three major components of the cytoskeleton.50 ANXA2 forms tetramer structures upon interaction with protein S100-A10, subsequently interacting with and regulating the bundle formation of F-actin. Upon c-Src-mediated phosphorylation of Tyr24, ANXA2 completely loses its ability to bind F-actin,51 suggesting that c-Src-mediated phosphorylation of ANXA2 contributes to regulation of the cytoskeletal dynamics favoring cell motility and EMT. MUC1 is a transmembrane member of the mucin family, consisting of a highly glycosylated extracellular and membrane spanning subunit (MUC1-alpha) and a small non-covalent attached cytoplasmic tail (MUC1-beta). MUC1 is involved in adhesive as well as de-adhesive processes52,53 and is associated with the metastatic progression of breast cancer.54 Interaction of MUC1-beta with c-Src leads to c-Src-mediated cytoskeletal rearrangements and pro-migratory signaling through Ras-related C3 botulinum toxin substrate 1 (RAC1) and cell division control protein 42 homolog (CDC42), promoting an invasive phenotype in cancer cells.55 MUC1-beta interacts with a variety of proteins involved in cell adhesion.56 Two major binding partners are beta-catenin and Glycogen synthase kinase-3 beta (GSK3B). c-Src is responsible for phosphorylation of Tyr1229 on MUC1-beta at the SPYEKV motive located between the sites for GSK3B and beta-catenin binding, respectively.48 c-Src phosphorylation inhibits the interaction between MUC1 and GSK3B, enhancing the interaction between MUC1 and beta-catenin. Interaction of MUC1 with beta-catenin has been reported to compete with E-cadherin–beta-catenin complex formation found at adherent junctions57 indicating a role for MUC1 in controlling cell adhesion and when bound to beta-catenin to favor cell migration. Furthermore, co-localization of MUC1 and beta-catenin has been associated with sites of focal adhesion lamellipodia of invading cells in studies of breast cancer cell lines.58
3.2 Zyxin as a potential target for CDK1
In close proximity to the paxillin and Src-governed signaling and represented by phosphorylation events in the sub-network we find zyxin (Fig. 6, ESI,† Fig. S3 and Table S5). Like paxillin, zyxin functions as an adaptor protein at site of focal adhesion. Zyxin is a low abundant phosphoprotein that plays a role in the spatial control of actin filament assembly.59 In contrast to paxillin, zyxin is not a part of the early focal complexes in ongoing lamellipodio formation but is instead recruited to the site of these complexes only when focal adhesion is established60 – a process mediated by internal contractile forces.61 At the site of focal adhesion, zyxin takes part in the dynamic reorganization of focal adhesion through mediation of actin-polymerization.62 In cancer, expression of zyxin has been linked to cell spreading and proliferation and has been found to correlate inversely with differentiation state.63 In the present cell line model zyxin exhibits altered phosphorylation on Ser267, a site reported by PhosphoNetworks25 to be a target site for cyclin-dependent kinase 1 (CDK1). The Ser267 phosphorylation shows higher expression in the HMT-E compared to the HMT-M cells. Consistently, in our data, CDK1 shows higher expression of pTyr15 in HMT-M, indicating that the kinase is less active in these cells, as pTyr15 is a central inhibitory site for CDK1 kinase activity. Hence, the expression of phosphorylated zyxin Ser267 in the cell line exhibiting higher CDK1 activity and the lack of other potential kinases for this particular site in our data further support the notion of Ser267 being a phosphorylation target site for CDK1.
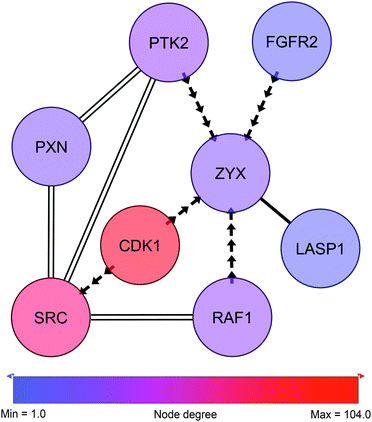 |
| Fig. 6 Zyxin-centric sub-sub-network. Adjacent nodes show breast cancer-related interactors as classified by IPA. Solid edges represent PPIs. Arrows represent phosphorylation and point from kinase to substrate. Double edges represent multiple edges reported by HPRD and at least one phospho-database. Despite not being direct neighbors, SRC and PXN are displayed to illustrate the close connection to c-Src and the paxillin network shown in Fig. 5. | |
4 Summary and conclusion
Our described analysis scheme departs from the tradition to analyze a single aspect of alteration, e.g. gene expression only, by establishing differential network mapping, analyzing multiple, concerted perturbation responses (gene expression, protein expression, protein phosphorylation including residue site information) at the same time. We introduced a method that allows combined analysis of various types of omics studies using logical operators and data set-specific parameterization. Based on extracted differential networks we compared signaling in cells from the same genetic background exhibiting epithelial-like and mesenchymal-like phenotypes, respectively. Combined with the merged multi-layer network resembling PPIs and phosphorylation, we showed that our method is fully capable of analyzing multiple data sets in combination and of identifying aberrant machinery in a general interaction network. Focusing on the differences in epithelial and mesenchymal states of triple-negative breast cancer cells, we elucidated an interconnected signaling pattern related to focal adhesion and migration. This revealed signaling through key proteins involved in migration and invasion of breast cancer cells which was clearly activated in the mesenchymal-like phenotype when compared to its epithelial-like counterpart.
The underlying global interaction network is the basis for differential network mapping and key to the success of this method. Careful and comprehensive adaptation of this network prior to the network mapping step is a necessity as it would otherwise be a limitation factor especially when combining multiple data sets. In addition to commonly known limitations such as noise (impaired true positive rate) and incompleteness, interaction scoring methods to generate high confidence PPI-networks (de-noising) may produce diverse results. Furthermore, differences in network types (e.g. directed/undirected networks vs. metabolic pathway maps) can limit their integration. We compiled a hybrid PPI-network consisting of protein interactions and protein phosphorylation events including residue site information. Additionally, we coupled compartment information, protein expression, protein phosphorylation and gene expression based on mRNA quantitation. ESI,† Tables S2–S5 highlight the importance of this integrative approach in detection-based methods with overall low coverage. While some proteins were not detected or not differential as determined by gene and protein expression they were extracted as a differentially phosphorylated node and included in the TNBC-associated parent network. As a result, a vastly more sensitive model was created that also resulted in a lower false negative rate.
Moreover, the above described findings in the generated sub-network point in the direction of a more migrating phenotype of the HMT-M compared to the HMT-E cell line as previously shown in vitro for this cell line model.20 The KeyPathwayMiner-based multi-omics approach was capable of mining and visualizing these insights into the regulation of focal adhesion and migration-related mechanisms occurring within our isogenetic breast cancer cell line model. This has enabled us to create a comprehensive view of the level of activity of the signaling cascades in question. In addition to confirming the presence of signaling to the mesenchymal phenotype of cancer cells, the network also strongly suggested that zyxin is a downstream target of CDK1. The implication of this finding for the role of zyxin in our cell line model is yet to be elucidated. Based on our results we suggest further studies to be conducted on biochemical level of the potential interaction of CDK1 and zyxin along with in vitro and in vivo studies on their influence on cellular morphology and motility.
Overall, complex interconnected signaling surrounding paxillin and c-Src was elucidated by the computational network model. Thereby, we could confirm that the model provides sufficient level of detail to discover biologically relevant alterations. Furthermore, the identification of zyx Ser267 as a target for CDK1-mediated phosphorylation strongly suggests that the integration and analysis of multiple integrated data sources can be a powerful tool for closing the gap between computational models and biological processes.
Conflicts of interest
None declared.
References
- T. Ideker and N. J. Krogan, Mol. Syst. Biol., 2012, 8, 565 CrossRef PubMed.
- T. Ideker, O. Ozier, B. Schwikowski and A. F. Siegel, Bioinformatics, 2002, 18(suppl 1), S233–S240 CrossRef PubMed.
- I. Ulitsky and R. Shamir, BMC Syst. Biol., 2007, 1, 8 CrossRef PubMed.
- T. Ideker and R. Sharan, Genome Res., 2008, 18, 644–652 CrossRef CAS PubMed.
- F. Vandin, P. Clay, E. Upfal and B. J. Raphael, Pac. Symp. Biocomput., 2012, 55–66 CAS.
- N. Alcaraz, H. Kücük, J. Weile, A. Wipat and J. Baumbach, Internet Math., 2011, 7(4), 299–313 CrossRef.
-
J. Baumbach, T. Friedrich, T. Kötzing, A. Krohmer, J. Müller and J. Pauling, Proceedings of the 14th Annual Conference on Genetic and Evolutionary Computation, 2012, pp. 169–176.
- C. Stark, B.-J. Breitkreutz, T. Reguly, L. Boucher, A. Breitkreutz and M. Tyers, Nucleic Acids Res., 2006, 34, D535–D539 CrossRef CAS PubMed.
- T. S. K. Prasad, K. Kandasamy and A. Pandey, Methods Mol. Biol., 2009, 577, 67–79 CAS.
- K. Tarassov, V. Messier, C. R. Landry, S. Radinovic, M. M. Serna Molina, I. Shames, Y. Malitskaya, J. Vogel, H. Bussey and S. W. Michnick, Science, 2008, 320, 1465–1470 CrossRef CAS PubMed.
- U. Stelzl, U. Worm, M. Lalowski, C. Haenig, F. H. Brembeck, H. Goehler, M. Stroedicke, M. Zenkner, A. Schoenherr, S. Koeppen, J. Timm, S. Mintzlaff, C. Abraham, N. Bock, S. Kietzmann, A. Goedde, E. Toksöz, A. Droege, S. Krobitsch, B. Korn, W. Birchmeier, H. Lehrach and E. E. Wanker, Cell, 2005, 122, 957–968 CrossRef CAS PubMed.
- A.-C. Gavin, P. Aloy, P. Grandi, R. Krause, M. Boesche, M. Marzioch, C. Rau, L. J. Jensen, S. Bastuck, B. Dümpelfeld, A. Edelmann, M.-A. Heurtier, V. Hoffman, C. Hoefert, K. Klein, M. Hudak, A.-M. Michon, M. Schelder, M. Schirle, M. Remor, T. Rudi, S. Hooper, A. Bauer, T. Bouwmeester, G. Casari, G. Drewes, G. Neubauer, J. M. Rick, B. Kuster, P. Bork, R. B. Russell and G. Superti-Furga, Nature, 2006, 440, 631–636 CrossRef CAS PubMed.
- N. J. Krogan, G. Cagney, H. Yu, G. Zhong, X. Guo, A. Ignatchenko, J. Li, S. Pu, N. Datta, A. P. Tikuisis, T. Punna, J. M. Peregrn-Alvarez, M. Shales, X. Zhang, M. Davey, M. D. Robinson, A. Paccanaro, J. E. Bray, A. Sheung, B. Beattie, D. P. Richards, V. Canadien, A. Lalev, F. Mena, P. Wong, A. Starostine, M. M. Canete, J. Vlasblom, S. Wu, C. Orsi, S. R. Collins, S. Chandran, R. Haw, J. J. Rilstone, K. Gandi, N. J. Thompson, G. Musso, P. St Onge, S. Ghanny, M. H. Y. Lam, G. Butland, A. M. Altaf-Ul, S. Kanaya, A. Shilatifard, E. O'Shea, J. S. Weissman, C. J. Ingles, T. R. Hughes, J. Parkinson, M. Gerstein, S. J. Wodak, A. Emili and J. F. Greenblatt, Nature, 2006, 440, 637–643 CrossRef CAS PubMed.
- M. E. Sowa, E. J. Bennett, S. P. Gygi and J. W. Harper, Cell, 2009, 138, 389–403 Search PubMed.
- O. Lavi, G. Dror and R. Shamir, J. Comput. Biol., 2012, 19, 694–709 CrossRef CAS PubMed.
- X. Wang and P. A. Ward, J. Transl. Med., 2012, 10, 240 CrossRef PubMed.
- S. Liu, Y. Cong, D. Wang, Y. Sun, L. Deng, Y. Liu, R. Martin-Trevino, L. Shang, S. P. McDermott, M. D. Landis, S. Hong, A. Adams, R. D'Angelo, C. Ginestier, E. Charafe-Jauffret, S. G. Clouthier, D. Birnbaum, S. T. Wong, M. Zhan, J. C. Chang and M. S. Wicha, Stem Cell Reports, 2014, 2, 78–91 CrossRef CAS PubMed.
- I. Ulitsky, A. Krishnamurthy, R. M. Karp and R. Shamir, PLoS One, 2010, 5, e13367 Search PubMed.
- C. A. Hudis, N. Engl. J. Med., 2007, 357, 39–51 CrossRef CAS PubMed.
- R. Leth-Larsen, M. G. Terp, A. G. Christensen, D. Elias, T. Kühlwein, O. N. Jensen, O. W. Petersen and H. J. Ditzel, Mol. Med., 2012, 18, 1109–1121 CAS.
- R. Kalluri and R. A. Weinberg, J. Clin. Invest., 2009, 119, 1420–1428 CrossRef CAS PubMed.
- M. R. Larsen, T. E. Thingholm, O. N. Jensen, P. Roepstorff and T. J. D. Jørgensen, Mol. Cell. Proteomics, 2005, 4, 873–886 CAS.
- K. Engholm-Keller, P. Birck, J. Støling, F. Pociot, T. Mandrup-Poulsen and M. R. Larsen, J. Proteomics, 2012, 75, 5749–5761 CrossRef CAS PubMed.
- N. Alcaraz, T. Friedrich, T. Kötzing, A. Krohmer, J. Müller, J. Pauling and J. Baumbach, Integr. Biol., 2012, 4, 756–764 RSC.
- J. Hu, H.-S. Rho, R. H. Newman, J. Zhang, H. Zhu and J. Qian, Bioinformatics, 2014, 30, 141–142 CrossRef CAS PubMed.
- R. H. Newman, J. Hu, H.-S. Rho, Z. Xie, C. Woodard, J. Neiswinger, C. Cooper, M. Shirley, H. M. Clark, S. Hu, W. Hwang, J. S. Jeong, G. Wu, J. Lin, X. Gao, Q. Ni, R. Goel, S. Xia, H. Ji, K. N. Dalby, M. J. Birnbaum, P. A. Cole, S. Knapp, A. G. Ryazanov, D. J. Zack, S. Blackshaw, T. Pawson, A.-C. Gingras, S. Desiderio, A. Pandey, B. E. Turk, J. Zhang, H. Zhu and J. Qian, Mol. Syst. Biol., 2013, 9, 655 CrossRef PubMed.
- P. V. Hornbeck, J. M. Kornhauser, S. Tkachev, B. Zhang, E. Skrzypek, B. Murray, V. Latham and M. Sullivan, Nucleic Acids Res., 2012, 40, D261–D270 CrossRef CAS PubMed.
- F. Diella, C. M. Gould, C. Chica, A. Via and T. J. Gibson, Nucleic Acids Res., 2008, 36, D240–D244 CrossRef CAS PubMed.
- R. Amanchy, B. Periaswamy, S. Mathivanan, R. Reddy, S. G. Tattikota and A. Pandey, Nat. Biotechnol., 2007, 25, 285–286 CrossRef CAS PubMed.
- M. I. Kokkinos, R. Wafai, M. K. Wong, D. F. Newgreen, E. W. Thompson and M. Waltham, Cells Tissues Organs, 2007, 185, 191–203 CrossRef CAS PubMed.
- V. Petit and J. P. Thiery, Biol. Cell., 2000, 92, 477–494 CrossRef CAS.
- N. O. Deakin and C. E. Turner, J. Cell Sci., 2008, 121, 2435–2444 CrossRef CAS PubMed.
- S. N. Nikolopoulos and C. E. Turner, J. Cell Biol., 2000, 151, 1435–1448 CrossRef CAS.
- J. Pignatelli, S. E. LaLonde, D. P. LaLonde, D. Clarke and C. E. Turner, J. Biol. Chem., 2012, 287, 37309–37320 CrossRef CAS PubMed.
- D. M. Clarke, M. C. Brown, D. P. LaLonde and C. E. Turner, J. Cell Biol., 2004, 166(6), 901–912 CrossRef CAS PubMed.
- B. G. Neel, H. Gu and L. Pao, Trends Biochem. Sci., 2003, 28, 284–293 CrossRef CAS.
- S. Maes, E. Mira, C. Gmez-Mouton, Z. J. Zhao, R. A. Lacalle and C. Martnez-A, Mol. Cell. Biol., 1999, 19, 3125–3135 Search PubMed.
- W. Vogel and A. Ullrich, Cell Growth Differ., 1996, 7, 1589–1597 CAS.
- C. Vindis, T. Teli, D. P. Cerretti, C. E. Turner and U. Huynh-Do, J. Biol. Chem., 2004, 279, 27965–27970 CrossRef CAS PubMed.
- T. Iwasaki, A. Nakata, M. Mukai, K. Shinkai, H. Yano, H. Sabe, E. Schaefer, M. Tatsuta, T. Tsujimura, N. Terada, E. Kakishita and H. Akedo, Int. J. Cancer, 2002, 97, 330–335 CrossRef CAS PubMed.
- Y. Ren, S. Meng, L. Mei, Z. J. Zhao, R. Jove and J. Wu, J. Biol. Chem., 2004, 279, 8497–8505 CrossRef CAS PubMed.
- M. Guarino, J. Cell. Physiol., 2010, 223, 14–26 CAS.
- E. L. Mayer and I. E. Krop, Clin. Cancer Res., 2010, 16, 3526–3532 CrossRef CAS PubMed.
- V. G. Brunton, E. Avizienyte, V. J. Fincham, B. Serrels, C. A. Metcalf 3rd, T. K. Sawyer and M. C. Frame, Cancer Res., 2005, 65, 1335–1342 CrossRef CAS PubMed.
- M. Nethe and P. L. Hordijk, Cell Adh. Migr., 2011, 5, 59–64 CrossRef.
- J. V. Evans, A. G. Ammer, J. E. Jett, C. A. Bolcato, J. C. Breaux, K. H. Martin, M. V. Culp, P. M. Gannett and S. A. Weed, J. Cell Sci., 2012, 125, 6185–6197 CrossRef CAS PubMed.
- S. Roura, S. Miravet, J. Piedra, A. Garca de Herreros and M. Duach, J. Biol. Chem., 1999, 274, 36734–36740 CrossRef CAS PubMed.
- Y. Li, H. Kuwahara, J. Ren, G. Wen and D. Kufe, J. Biol. Chem., 2001, 276, 6061–6064 CrossRef CAS PubMed.
- M. de Graauw, I. Tijdens, M. B. Smeets, P. J. Hensbergen, A. M. Deelder and B. van de Water, Mol. Cell. Biol., 2008, 28, 1029–1040 CrossRef CAS PubMed.
- U. Rescher, D. Ruhe, C. Ludwig, N. Zobiack and V. Gerke, J. Cell Sci., 2004, 117, 3473–3480 CrossRef CAS PubMed.
- I. Hubaishy, P. G. Jones, J. Bjorge, C. Bellagamba, S. Fitzpatrick, D. J. Fujita and D. M. Waisman, Biochemistry, 1995, 34, 14527–14534 CrossRef CAS.
- L. H. Regimbald, L. M. Pilarski, B. M. Longenecker, M. A. Reddish, G. Zimmermann and J. C. Hugh, Cancer Res., 1996, 56, 4244–4249 CAS.
- J. Wesseling, S. W. van der Valk, H. L. Vos, A. Sonnenberg and J. Hilkens, J. Cell Biol., 1995, 129, 255–265 CrossRef CAS.
- T. M. Horm and J. A. Schroeder, Cell Adh. Migr., 2013, 7, 187–198 CrossRef PubMed.
- Q. Shen, J. J. Rahn, J. Zhang, N. Gunasekera, X. Sun, A. R. E. Shaw, M. J. Hendzel, P. Hoffman, A. Bernier and J. C. Hugh, Mol. Cancer Res., 2008, 6, 555–567 CrossRef CAS PubMed.
- P. K. Singh and M. A. Hollingsworth, Trends Cell Biol., 2006, 16, 467–476 CrossRef CAS PubMed.
- M. Yamamoto, A. Bharti, Y. Li and D. Kufe, J. Biol. Chem., 1997, 272, 12492–12494 CrossRef CAS PubMed.
- J. A. Schroeder, M. C. Adriance, M. C. Thompson, T. D. Camenisch and S. J. Gendler, Oncogene, 2003, 22, 1324–1332 CrossRef CAS PubMed.
- M. C. Beckerle, BioEssays, 1997, 19, 949–957 CrossRef CAS PubMed.
- R. Zaidel-Bar, C. Ballestrem, Z. Kam and B. Geiger, J. Cell Sci., 2003, 116, 4605–4613 CrossRef CAS PubMed.
- H. Hirata, H. Tatsumi and M. Sokabe, J. Cell Sci., 2008, 121, 2795–2804 CrossRef CAS PubMed.
- J. Fradelizi, V. Noireaux, J. Plastino, B. Menichi, D. Louvard, C. Sykes, R. M. Golsteyn and E. Friederich, Nat. Cell Biol., 2001, 3, 699–707 CrossRef CAS PubMed.
- E. J. van der Gaag, M.-T. Leccia, S. K. Dekker, N. L. Jalbert, D. M. Amodeo and H. R. Byers, J. Invest. Dermatol., 2002, 118, 246–254 CrossRef PubMed.
Footnotes |
† Electronic supplementary information (ESI) available: Fig. S1–S6, Tables S1–S5 and cytoscape session file TNBC_session.cys. See DOI: 10.1039/c4ib00137k |
‡ These authors contributed equally to this work. |
|
This journal is © The Royal Society of Chemistry 2014 |