College students’ attitudes toward chemistry, conceptual knowledge and achievement: structural equation model analysis
Received
17th December 2012
, Accepted 11th January 2013
First published on 27th February 2013
Abstract
Students’ success in introductory college-level chemistry courses is important for them to continue to more advanced science courses and to progress toward science-related careers. Previous studies indicate that both cognitive and non-cognitive variables are relevant for student success. In this study, Structural Equation Modeling (SEM) was used to predict students’ achievement in chemistry from both cognitive (math ability, prior conceptual knowledge in chemistry) and non-cognitive (attitude toward chemistry) measures. The purpose of this study is to investigate which of three alternative SEM models best represents the relationships among these variables and achievement in chemistry, and what proportion of variance in chemistry achievement can be explained. Results provide support for using a SEM model with all three predictors, with 69% of the variance in chemistry achievement explained. Both prior conceptual knowledge and attitude toward chemistry contribute a significant unique portion to the prediction of chemistry achievement when controlling for math ability. The results suggest that instructors can improve students’ achievement in chemistry not only by focusing on helping them to build conceptual knowledge, but also by fostering their positive attitude toward chemistry. This study also has implications for SEM researchers, both to further replicate the study in other contexts, and to add other potentially relevant predictors. Instrument developers may also use the modeling strategy as a springboard for optimizing assessment tools.
Introduction
Introductory college-level chemistry courses are required for students to continue to advanced chemistry courses and to progress toward science-related careers. Therefore, student performance in introductory college chemistry courses remains a concern for educators. In order to guide decisions about which interventions could be effective in improving student achievement, the first step is to understand the factors that influence achievement. Many factors, such as student background and school environment, have been reported to influence students’ performance in science (e.g.Bunce and Hutchinson, 1993; Cakici et al., 2011; Seery, 2009). In chemical education, research has been done to predict students’ achievement in college chemistry courses. Mathematical ability has been important for students’ placement into specific college chemistry courses as well as for prediction of their chemistry achievement (Cooper and Pearson, 2012; Pienta, 2003; Scott, 2012). Prior conceptual knowledge has also been found to have an effect on student subsequent achievement (Seery, 2009; Wagner et al., 2002). Besides those cognitive factors, non-cognitive factors, such as attitude, are extensively studied in the chemistry education area as a potential influence on students’ achievement (Bauer, 2008; Cukrowska et al., 1999; House, 1995; Xu and Lewis, 2011).
In this study, we are interested in examining the relationships among three key factors, attitude toward chemistry, prior conceptual knowledge, and math ability, and their relationship with chemistry performance for college students enrolled in the first semester of introductory college chemistry. These three factors have been shown to be good predictors of achievement in previous studies of similar contexts (e.g., Gooding et al., 1990; House, 1995; Pienta, 2003; Xu and Lewis, 2011). In addition, these factors are of practical importance for educational practice. Effective instructional strategies can be implemented in science classrooms to influence these factors. For example, Freedman (1997) used a hands-on laboratory program to foster students’ positive attitudes toward science, while Abdullah et al. (2009) used individualized microscale experiments in chemistry to improve students’ attitudes toward chemistry practical work. Ebenezer et al. (2010) implemented interventions to promote positive changes in students’ conceptual knowledge; in the same way, Regan et al. (2011) used students’ misconceptions in chemistry to develop a course of tutorials to target those difficult areas. Finally, Pienta (2003) developed a mathematical assessment and tutorial for general chemistry students to help them with math knowledge and skills. Since different interventions have been used to improve each of these factors separately, it is reasonable to wonder whether attention to all of these factors would be likely to improve student chemistry achievement to a greater degree, and whether there are relationships between the factors that hint at the potential for synergy. This study examines these issues for a group of students enrolled in a typical first semester general chemistry course in the United States via a structural equation modeling (SEM) approach. The first step was choosing how to measure each of the relevant factors.
Student achievement in college chemistry
Course grades or final exam scores are often used to measure student achievement in the first semester general chemistry course. In the United States, course grade can be a relevant criterion for investigating student progress toward eventual careers in chemistry, because students often need a grade of C or better in the introductory course to be able to register in more advanced courses. Alternatively, more general measurement tools, especially national exams such as the chemistry exams prepared by the Examinations Institute of the American Chemical Society’s (ACS) Division of Chemical Education, can be used as a measure of student achievement (Examinations Institute, 1997). Some ACS exams have explicitly blended both traditional (algorithmic) and conceptual questions to measure students’ performance in concepts covered during college chemistry courses (Examinations Institute, 1997; Lewis and Lewis, 2008). The main advantage of using an ACS exam score over course grade, as a measure of performance or achievement in chemistry, is that the results are more readily generalizable across institutions (Examinations Institute, 1997; Lewis and Lewis, 2007).
Attitude toward chemistry and relationship with achievement
Contemporary theories of attitude define it as “a psychological tendency that is expressed by evaluating a particular entity with some degree of favor or disfavor” (Eagly and Chaiken, 1993, p. 1). The tripartite theoretical model consisting of three attitudinal dimensions, affect, behavior, and cognition, was proposed by Rosenberg and Hovland (1960, p. 3). The affective component relates to an emotional response that expresses an individual's level of preference with respect to a particular entity, such as the subject of chemistry. The cognitive component is an evaluation that comprises an individual’s beliefs and knowledge about the entity. The behavioral component corresponds to a verbal intention or behavioral tendency of an individual to act towards that entity. Attitude is not resistant to change: an individual gains most of his or her attitudes from either direct experience or observational learning from the environment (Maio and Haddock, 2010).
There are many different methods for measuring attitude. Standard attitude measures include scales such as Likert, Guttman, Thurston, and semantic differentials. In chemistry education, several extant attitude instruments are the Chemistry Expectations Survey (CHEMX) (Grove and Bretz, 2007), Colorado Learning Attitudes about Science Survey (CLASS) (Barbera et al., 2008; Heredia and Lewis, 2012), Attitude toward the Subject of Chemistry Inventory (ASCI) (Bauer, 2008; Brandriet et al., 2011; Xu and Lewis, 2011), Chemistry Attitudes and Experiences Questionnaire (CAEQ) (Coll et al., 2002), and a questionnaire to measure attitude toward chemistry learning (Berg, 2005). In its shortened version, the Attitude toward the Subject of Chemistry Inventory version 2, ASCIv2, has the most reported evidence for psychometric quality and reflects two components in the tripartite framework, cognition and affect (Brandriet et al., 2011; Xu and Lewis, 2011).
There have been many attitudinal studies where the relationship between attitude toward science and science achievement has been investigated (Cukrowska et al., 1999; House, 1995; Weinburgh, 1995). With high school students, students’ achievement has been found to be significantly related to their attitudes (Gooding et al., 1990; Tuan et al., 2005). In college chemistry, positive relationships have been found for first-year chemistry using correlation analysis (Cukrowska et al., 1999) and logistic regression analysis (House, 1995). However, these positive relationships have been found to depend on the instrument used to measure students’ attitude, making instrument choice an important issue (Xu and Lewis, 2011). Not only does ASCIv2’s psychometric information make it a reasonable choice, Xu and Lewis (2011), using multiple regression, found that one of the components of attitude measured by ASCIv2 plays a significant and unique role in predicting final achievement in a first semester general chemistry course.
Prior conceptual knowledge and relationship with achievement
Students’ prior conceptual knowledge is another factor that has been considered critical for learning chemistry in a meaningful way. Students’ incorrect ideas or alternate conceptions about important topics could hinder deeper learning of those and other relevant topics in chemistry, thereby affecting students’ final achievement in the course (Gabel, 1999; Nakhleh, 1992; Nicoll, 2001). For that reason, investigating the relationship between students’ prior knowledge and achievement is important. A recent study reported that prior knowledge is the most significant factor in predicting achievement for undergraduate chemistry (Seery, 2009). However, as Dochy (1999) pointed out, the effect of prior knowledge depends on the assessment method used.
Multiple instruments have been developed with a focus on chemistry conceptual knowledge rather than rote memorization, including the Chemistry Concept Understanding Test (CCUT) (Danipog and Ferido, 2011), Student Conceptual Understanding Test (SCUT) (Lin et al., 2005), Chemical Concept Inventory (CCI) (Mulford and Robinson, 2002), the Particulate Nature of Matter and Chemical Bonding Diagnostic Instrument (Heredia et al., 2012; Othman et al., 2008), and the Targeted Misconception Inventory (TMI) (Heredia, 2012). All of these instruments have been designed and used to probe students’ prior knowledge in chemistry and to identify students’ incorrect ideas, but none appear to have been used to predict achievement. Additionally, publicly available psychometric evidence is limited for many of these instruments. Because the TMI has been designed with a factor structure and some psychometric evidence can be obtained, it is a good candidate for measuring students’ prior conceptual knowledge and for investigating the relationship between prior knowledge and students’ achievement.
Math ability and relationship with achievement
Math ability has long been considered important for achievement in chemistry (Spencer, 1996). The SAT is the most widely used college admission test in the U.S., and it has been successfully used as a predictor of science performance in college (Ewing et al., 2005). The SAT as a whole probes students’ aptitude in different subject areas such as reading, writing, and math, with student math ability measured by the quantitative portion of the SAT, SATM. The relationship between SATM and students’ performance in college chemistry has been well documented in the research literature (Bunce and Hutchinson, 1993; Lewis and Lewis, 2007, 2008; Spencer, 1996; Wagner et al., 2002). In one study, Spencer (1996) found that SATM scores were an important factor in determining students’ grades in college-level general chemistry. In another study focused on college-level general chemistry, Lewis and Lewis (2007) found a medium-size correlation between SATM scores and ACS final exam score. While other measures of math ability exist (Cooper and Pearson, 2012; Pienta, 2003; Wagner et al., 2002), SATM has been found to be a suitable measure of students’ math ability and a good predictor of student achievement in introductory college chemistry.
Conceptual relationship among the four factors in chemistry
As the foregoing discussion indicates, these three factors and their relationship to achievement in chemistry have been examined previously, but typically in separate studies. The proposed simultaneous relationship among the different factors for the present study is shown in Fig. 1. Students’ performance in chemistry, as measured by students’ achievement in the course, is directly influenced by three predictors: students’ attitude toward chemistry, their prior conceptual knowledge and their math ability. Relationships among these variables are expected; therefore they are set to correlate with one another in this model as shown by the double arrows in Fig. 1.
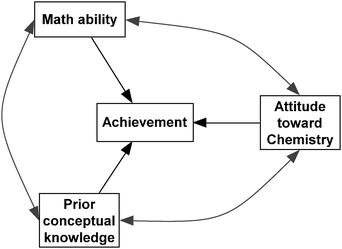 |
| Fig. 1 Conceptual model of chemistry achievement and three predictors (Model 1). | |
Structural equation modeling (SEM)
Structural equation modeling (SEM) is a multivariate data analysis approach used to study complex relationships among variables. The typical research questions that can be answered using SEM are twofold: (1) How do multiple variables interact with one another?, and (2) Which of several hypothetical models best fit empirical data?
SEM has been widely used in multiple disciplines such as economics (Goerzen and Beamish, 2005; Kaynak, 2003) and psychology (MacCallum and Austin, 2000; Wei et al., 2003). In science education, it has been employed to investigate the learning process and to improve instruction (Abbott and Berninger, 1993; Cakici et al., 2011; Hemmings et al., 2011; Schreiber et al., 2006). Recently in chemistry education, Stamovlasis et al. (2012) reported the relationships among ninth-grade junior high school Greek students’ understanding of the change of state of matter and three cognitive variables: logical thinking, field-dependence/field-independence and convergence/divergence, with the final model accounting for 85% of the variance after structural understanding had been included among the predictors. In another study, Merchant et al. (2012) investigated the relationships among variables such as spatial ability, self-efficacy, and chemistry learning achievement for college chemistry students, with the model accounting for 45% of variance in performance as measured by a chemistry test. Kim and Song (2010) examined the effects of Korean high school students’ dichotomous (intrinsic and extrinsic) attitudes towards science, interest, and conceptual understanding on school achievement with a model that explained 33% of the variance in achievement. Turning the focus to conceptual understanding, Nieswandt (2007) explored the relationships between affective variables, including attitudes toward chemistry and chemistry self-concept, and student understanding of chemistry concepts for ninth grade students in Germany and found that students’ self-concept has a positive and significant effect on conceptual understanding.
Schreiber et al. (2006) and Schreiber (2008) have recommended a set of guidelines for the use, report, and evaluation of SEM studies in social and educational areas. Standard nontechnical issues for research, such as the fact that research questions should warrant the use of the chosen analysis technique, whatever it may be, also apply to SEM studies, but using SEM adds some technical requirements as well. For example, discussion of sample size, missing data, fit indices, parameter estimates, effect sizes, explained variance, rationale for model modification, and the use of nested models are important for SEM studies (Kline, 2010; Schreiber, 2008; Schreiber et al., 2006). The recent SEM publications in chemistry education cited above abide well by these guidelines with respect to reporting fit indices and parameter estimates; however, there are some limitations in other areas. First, one study does not report explained variance (Nieswandt, 2007). Second, two studies were performed with very small sample size (Kim and Song, 2010; Nieswandt, 2007), which suggests that replication is warranted. For example, Kim and Song (2010) found no relationship between topic attitude and school achievement for 10th-graders (n = 109) and a negative relationship for 11th-graders (n = 110), which is very interesting, but needs to be verified in a larger population. Third, although all of these studies examined multiple models, they neither report effect sizes for the reported relationships, nor investigate nested models, both of which would enable more direct comparisons between models. In general, although these four studies represent a promising start, there remains a scarcity of SEM analysis in chemistry education research.
Present study
The goal of the present study is to examine the relationship of potentially relevant predictor variables such as students’ attitude toward chemistry, students’ prior conceptual knowledge of chemistry, and students’ math ability to students’ achievement in chemistry, while accounting for relationships among the predictor variables. Specifically, the goal is to use Structural Equation Modeling (SEM) to assess the hypothetical relationships among variables as shown in Fig. 1.
The four specific hypotheses for this study are:
(1) The full SEM model with all three predictors will fit the data better than a model without the inclusion of prior conceptual knowledge as a predictor.
(2) The proportion of variance in student achievement explained by the model will significantly decrease if measurement error is neglected or if prior conceptual knowledge is deleted as a predictor.
(3) Attitude and prior conceptual knowledge have direct effects on chemistry achievement when math ability is controlled.
(4) There is a significant correlation among attitude, math ability, and prior conceptual knowledge.
Method
Instruments
Attitude.
Students’ attitude toward chemistry was measured by the Attitude toward the Subject of Chemistry Inventory version 2 (ASCIv2 hereafter) (Xu and Lewis, 2011). The instrument comprises two scales: “Intellectual Accessibility”, depicting the cognitive dimension of attitude and “Emotional Satisfaction”, depicting the affective dimension. The instrument consists of eight items, four items in each scale as shown in Table 1. The scale of the instrument is a semantic differential ranging from 1–7.
Table 1 Variables in ASCIv2 instrument
Dimensions |
Variable |
Item # |
Items |
Items are shown in the orientation used in the instrument, Items 1, 4, 5, 7 are reverse scored before analysis. |
Intellectual accessibility (cognitive dimension) |
ASCI1 |
1 |
Easy |
Hard |
ASCI2 |
2 |
Complicated |
Simple |
ASCI3 |
3 |
Confusing |
Clear |
ASCI6 |
6 |
Challenging |
Not challenging |
Emotional satisfaction (affective dimension) |
ASCI4 |
4 |
Comfortable |
Uncomfortable |
ASCI5 |
5 |
Satisfying |
Frustrating |
ASCI7 |
7 |
Pleasant |
Unpleasant |
ASCI8 |
8 |
Chaotic |
Organized |
Prior conceptual knowledge.
Students’ prior conceptual knowledge in chemistry was assessed by the Targeted Misconception Inventory (TMI) (Heredia, 2012). The TMI is a two-tier multiple-choice instrument that targets three specific concepts from general chemistry: bond energy, phase changes, and ionic bonding. These basic concepts should be covered in previous chemistry courses such as high school chemistry or preparatory college chemistry and are further built upon in college general chemistry. TMI is designed to identify students’ difficulty or incorrect ideas with these basic concepts at the beginning of the first semester of college general chemistry. The test consists of 18 multiple-choice questions, which corresponds to 9 items, with each two-tiered question counted as one item. Students need to answer both tiers correctly to get 1 point for an item. Each concept in the TMI is represented by three items following a parallel structure as shown in Table 2. The possible score ranges from 0 to 9 points. Although TMI covers three basic concepts in general chemistry, it is limited in scope.
Table 2 Items in TMI instrument
Concept |
Variable |
Item # |
Phase changes |
TMI1 |
1 |
TMI5 |
5 |
TMI7 |
7 |
Bond energy |
TMI2 |
2 |
TMI4 |
4 |
TMI9 |
9 |
Ionic bonding |
TMI3 |
3 |
TMI6 |
6 |
TMI8 |
8 |
Math ability.
Students’ math ability was measured using the quantitative part of the SAT (SATM hereafter). The SAT is typically administered in the last year of high school, and it is used as a college entrance exam in the U.S. The maximum score for the SATM during this time period was 800. SATM scores have been found to have internal consistency coefficients (Cronbach’s alpha) of 0.92, which is satisfactory for a high-stakes test (Ewing et al., 2005).
Chemistry achievement.
Students’ achievement in chemistry was measured by students’ scores on the First-Term General Chemistry Blended Examination from the Examinations Institute of the American Chemical Society Division of Chemical Education (ACS exam hereafter). It consists of 40 questions divided evenly among traditional (algorithmic) and conceptual questions (Examinations Institute, 1997).
Data collection and participants
Participants are students enrolled in the first term of a general chemistry course during Fall 2010 at a large southeastern public research university. All students took the same exams at the same time. ASCIv2 and TMI were given to students during the second week of the semester as a paper and pencil test within regular exam hours, with ASCIv2 proceeding TMI. The ACS exam was given to students as the final course exam at the end of the semester.
A total of 1656 students were enrolled in the course. Among them, 1319 students had SATM scores, 1399 had ASCIv2 scores, 1427 had TMI scores, and 1259 had ACS final exam scores. Overall, 963 students had the complete set of data for SATM, ASCIv2, TMI, and the ACS exam, and were included in the SEM analysis.
Table 3 presents the demographic information for the participants in the study. There are 504 females (52.3%) and 459 males (47.7%). The decomposition for this sample is very similar to the enrolled population of 1656 students (52.1% females and 47.9% males). Regarding race/ethnicity, 53.2% are White, 18.7% Hispanic 14.4% Asian, and 10.3% Black. 54.5% students are in the second year, 24.6% are in the third year, and 12.1% in the first year. Examination of the demographic information for the missing data did not indicate suspicious patterns with respect to race/ethnicity or year of study that could bias the results based on this sample.
Table 3 Demographics: number and percentage of students by sex and race/ethnicity (n = 963)
|
No. of students |
Percentage |
Sex |
Female |
504 |
52.3 |
Male |
459 |
47.7 |
Race/Ethnicity |
White (Not of Hispanic Origin) |
512 |
53.2 |
Hispanic or Latina |
180 |
18.7 |
Asian |
139 |
14.4 |
Black (Not of Hispanic Origin) |
99 |
10.3 |
Non-Resident Alien |
15 |
1.6 |
American Indian or Alaskan Native |
5 |
0.5 |
Ethnicity unspecified |
9 |
0.9 |
Native Hawaiian or Other Pacific Islanders |
4 |
0.4 |
Model specification for SEM analysis
Three SEM models were specified and tested in this study. The first model is represented in Fig. 1. This model assumes no measurement error in each variable; therefore, it includes only measured variables. This model is a simple path analysis model. Achievement as measured directly by the ACS final exam is predicted by math ability as measured by the SATM, attitude as measured by the total score on ASCIv2, and prior conceptual knowledge as measured by the total score on the TMI.
Fig. 2 and 3 present two SEM models included in the analysis. Both of these models include two parts, the path or structural model and the measurement model, in the analysis. Fig. 2 shows the same relationship among variables as in the path model (Fig. 1) for the student variables, achievement, math ability, prior conceptual knowledge, and attitude toward chemistry. Note that, in this more complex model, three variables are included as latent variables, which are measured by indicators such as SATM, ASCI1, and TMI1. All three models, as illustrated in Fig. 1 through 3, posited correlations among the predictors.
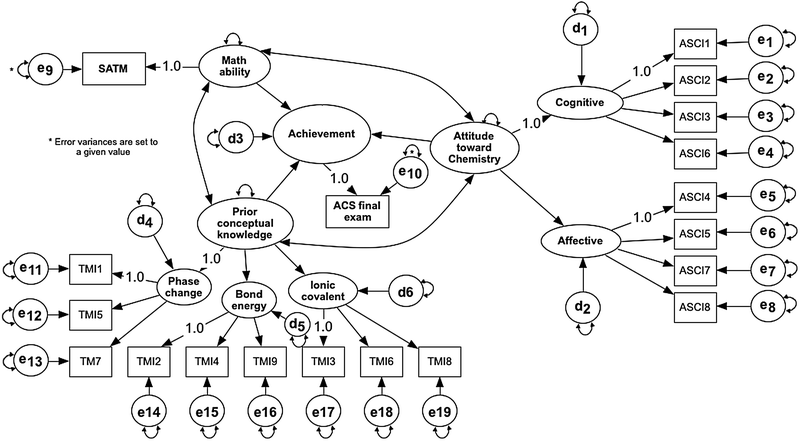 |
| Fig. 2 SEM Model 2 with three predictors. | |
 |
| Fig. 3 SEM Model 3 with two predictors. | |
The TMI, which is a measure of prior conceptual knowledge in this study, is only measuring three basic concepts from all of chemistry; therefore, it might be argued that the TMI is not a relevant predictor of achievement as measured by ACS exam scores. In order to address this concern, TMI was removed from the prediction for Model 3 as represented in Fig. 3. Accordingly, Model 3 examines the prediction of achievement with one less predictor than does Model 2. Except for the difference of one path coefficient, both of these models include the same measured variables, latent variables, and relationships among variables, thus a comparison of chi-square change between the two nested models will provide information about which model better fits the data. This comparison will also allow us to determine whether prior conceptual knowledge as measured by the TMI is a relevant predictor of achievement for this data.
Both SEM models have a total of nine latent variables (represented with ovals) and 19 measured variables (represented as rectangles), and 23 error variances (reflected in circles). Each SEM model has 190 pieces of information in the covariance matrix. Model 2 estimates 47 parameters, with degrees of freedom at 143. Model 3 estimates 66 parameters, with degrees of freedom at 144.
For model identification purposes, the loading for the first item on its corresponding latent variable was set to 1. The error variances for two measured variables, ACS and SATM, were calculated from the Cronbach’s alpha coefficient for each one according to the procedure recommended by Valiente and co-workers, because they are proprietary exams and no further information was available (Valiente et al., 2007). The ACS final exam had a Cronbach’s alpha of 0.85 for the 40 items for this sample; therefore the value of the error variance was set to 15% of the total variance obtained (47.1), resulting in an error value set to 7.09. For the SATM, previous reports indicated the quantitative SAT had an alpha of 0.92; therefore the error variance was set to 482, which is 8% of the total variance for the variable (6026).
Data analysis
Descriptive statistics and assumption checking for SEM.
Descriptive statistics for each measured variable were obtained using SAS 9.3. Univariate and multivariate normality and outliers were examined.
Structural equation model (SEM).
SEM was performed using the CALIS procedure in SAS 9.3 to investigate both model fitness and parameter estimates. Fit indices such as Chi-Square (χ2), Comparative Fit Index (CFI), and the Standardized Root Mean Square Residual (SRMR) were examined to assess the fitness of the model to the data. The criteria of CFI value at least 0.95 and SRMR value less than 0.08 are used to indicate a good model fit (Hu and Bentler, 1999). SEM analysis parameters such as model specification, standardized parameter estimates and effect sizes, and predicted variance indicated by R2, were reported in compliance with the guidelines proposed by Kline (2010).
Results
Descriptive statistics and assumption checking for SEM
The analysis was performed using 963 complete sets of data for the variables included in the model. Descriptive statistics for the sample were examined to observe the general differences in the variables and to examine the univariate normality of each variable. As shown in Table 4, the mean for the ASCIv2 variables ranged from 2.75 to 4.56. Four of the variables (ASCI1, ASCI4, ASCI5, ASCI7) were reverse coded for interpretation; therefore, higher scores mean students feel chemistry is intellectually accessible and emotionally satisfying. For the TMI, items 2, 3, and 9 are apparently quite difficult, because less than 20% of the students got these items correct.
Table 4 Descriptive statistics for each measured variable (n = 963)
|
M |
SD |
Skewness |
Kurtosis |
Min |
Max |
Note: The relatively large kurtosis values for the three difficult TMI items are indicated in bold. |
ASCI1 |
3.45 |
1.42 |
0.31 |
−0.33 |
1 |
7 |
ASCI2 |
3.33 |
1.42 |
0.37 |
−0.40 |
1 |
7 |
ASCI3 |
3.81 |
1.47 |
0.05 |
−0.56 |
1 |
7 |
ASCI6 |
2.75 |
1.38 |
0.80 |
0.39 |
1 |
7 |
ASCI4 |
4.10 |
1.48 |
−0.06 |
−0.45 |
1 |
7 |
ASCI5 |
4.04 |
1.68 |
0.04 |
−0.76 |
1 |
7 |
ASCI7 |
3.95 |
1.46 |
0.04 |
−0.18 |
1 |
7 |
ASCI8 |
4.56 |
1.54 |
−0.35 |
−0.51 |
1 |
7 |
TMI1 |
0.34 |
0.47 |
0.67 |
−1.55 |
0 |
1 |
TMI5 |
0.30 |
0.46 |
0.88 |
−1.22 |
0 |
1 |
TMI7 |
0.58 |
0.49 |
−0.34 |
−1.89 |
0 |
1 |
TMI2 |
0.12 |
0.33 |
2.31 |
3.32
|
0 |
1 |
TMI4 |
0.20 |
0.40 |
1.51 |
0.27 |
0 |
1 |
TMI9 |
0.09 |
0.29 |
2.82 |
5.96
|
0 |
1 |
TMI3 |
0.11 |
0.32 |
2.46 |
4.07
|
0 |
1 |
TMI6 |
0.28 |
0.45 |
0.96 |
−1.09 |
0 |
1 |
TMI8 |
0.22 |
0.41 |
1.37 |
−0.13 |
0 |
1 |
SATM (α = 0.92) |
596.00 |
77.63 |
−0.11 |
0.02 |
370 |
800 |
ASCIv2 (α = 0.88) |
3.75 |
1.09 |
−0.10 |
0.21 |
1 |
7 |
TMI (α = 0.45) |
2.25 |
1.61 |
0.90 |
0.99 |
0 |
9 |
ACS Exam (α = 0.85) |
26.20 |
6.86 |
−0.20 |
−0.51 |
7 |
40 |
Univariate normality was first assessed via examination of the skewness and kurtosis for each variable. According to Table 4, all the variables for ASCIv2 (ASCI1–ASCI8), SATM, and ACS Exam have absolute skewness and kurtosis values less than one; however, for the TMI, items 2, 3 and 9 have kurtosis values beyond two, which may be cause for concern (Curran et al., 1996). These items were difficult for students in our sample; therefore, these items may need to be revised for future administrations. A SEM model using only the composite (sum) score for the nine TMI items was run, since the skewness and kurtosis for the composite TMI score are both less than one (Table 4). The model fit and parameter estimates for the model did not change, suggesting that the model is robust, and indicating relative univariate normality. Therefore, the SEM model with the individual TMI was used for the analysis and interpretation.
Outlier assessment testing revealed a single outlier with the largest Mahalanobis distance of 59.4 away from the sample mean. The SEM analysis was re-run after removing the outlier to examine the consequence of the outlier. The values of the fit indices (χ2, CFI, SRMR) did not change significantly, suggesting that the fitness of the data to the model remains the same. The path coefficients also did not change for the analysis without the outlier. For these reasons, the original SEM with the complete data set was used for the analysis and interpretation. Since relative univariate normality was obtained, the outlier assessment did not reveal any consequential outliers, and the sample size is large, Maximum Likelihood (ML) estimate was used as the estimation method for the proposed SEM models (Finney and DiStefano, 2006).
Structural equation model analysis
Model fit.
For Model 1, model fit is not assessed and fit indices are not reported since it is a path analysis, e.g., its degrees of freedom are zero and there is no measurement error (Raykov and Marcoulides, 2006). The overall fit for SEM Models 2 and 3 is reported as shown in Table 5. Although the Chi-square (χ2) test is statistically significant (p < 0.001) for both models, suggesting that the models did not fit the data well, the large value of χ2 arises from the large sample size (Brown, 2006, p. 81). Other fit indices, insensitive to sample size, indicate that both SEM models have good fit (Hu and Bentler, 1999). For Model 2, the CFI value (0.95) meets the suggested criterion of at least 0.95 and the SRMR value (0.04) meets the suggested criterion of smaller than 0.08. For Model 3, the CFI value (0.94) is close to the suggested criterion, and the SRMR value (0.04) remains less than 0.08. Since Model 3 is a nested model within Model 2, the change in χ2 for the two model fits can be used to compare the models (West et al., 2012, p. 211). The change in χ2 is 71, with a change in degrees of freedom of 1, which indicates that the data fits Model 2 significantly better than Model 3.
Table 5 Fit Indices and predicted variance for models (N = 963)
SEM Model |
χ
2 (df) |
p-Value |
SRMR |
CFI |
Δ df |
Δχ2 |
Predicted variance |
1 |
— |
— |
— |
— |
— |
— |
46% |
2 |
395 (143) |
<0.001 |
0.04 |
0.95 |
1 |
71 (s.s.) |
69% |
3 |
466 (144) |
<0.001 |
0.04 |
0.94 |
57% |
As shown in Fig. 6, Model 3 has one less predictor for achievement, which allows examination of how removing prior content knowledge from the model might influence the prediction accuracy. The prediction accuracy was assessed using the percentage of variance in achievement that is accounted for the sets of predictors. Model 1 has the lowest prediction accuracy with 46% of the variance explained as shown in Table 5. For Model 3, 59% of the variance in the achievement variable is explained by the set of predictors for this model while for Model 2, 69% of the variance is explained.
The following formula, for determining the F-value, was used to examine whether adding the variable of prior conceptual knowledge contributed significantly to the prediction in Model 3 (Pedhazur, 1997).
where
R2 is the proportion of variance explained by the model
k is “number of predictors”
N is total sample size
Results from this test, F(1,959) = 371.2, p < 0.001, indicate that the unique contribution of prior conceptual knowledge is significant for the prediction.
Based on the change in χ2 and the explained variance, Model 2 can better explain the relationships among the variables than Model 3. Therefore, Model 2 is the better model to represent the relationships among the studied variables. Below the relationships for the three models are explained in more detail.
Decomposition of relationships.
The standardized parameter estimates resulting from the three models are presented in Fig. 4 through 6, respectively.
 |
| Fig. 4 Standardized parameters for the path analysis Model 1. | |
Measurement model for ASCIv2.
The first set of coefficients estimated in both SEM Models 2 and 3 represent the attitude toward chemistry variable as measured by ASCIv2. This attitude variable has two components: the cognitive (“Intellectual Accessibility”) and the affective (“Emotional Satisfaction”), indicated as “Cognitive” and “Affective” in the diagrams. As can be seen from the high positive (0.57–0.80) path coefficients for the cognitive component in the path diagram,“Intellectual Accessibility” has a strong influence on all of the measured variables. Similarly, as shown by the positive (0.53–0.79) values of the path coefficient for the affective component “Emotional Satisfaction” has a strong influence on its measured variables. The second order of this attitude test structure is the relationship between these two latent variables with overall “Attitude toward Chemistry.” The path coefficient from attitude toward chemistry to cognitive is 0.89 and from attitude toward chemistry to affective is 0.93, which provides strong validity evidence based on the internal structure of ASCIv2 that items are measuring two components of attitude toward the subject of chemistry. The variance in attitude toward chemistry explained by the cognitive component is 78%, and by the attitude component is 86%. These results are the same for both SEM models and consistent with literature (Brandriet et al., 2011; Xu and Lewis, 2011).
Measurement model for TMI.
TMI addresses three basic concepts, bond energy, phase changes, and ionic/covalent bonds. Each concept is measured using three items. As shown in Fig. 5 and 6, the test items loaded well on their respective scales. However, the scale of bond energy does not load well under the general construct of chemistry conceptual knowledge, since its loadings are −0.06 and −0.04 for Model 2 and 3, respectively. For the other two concepts, the loadings are higher, 0.85 and 0.84 for phase changes in Model 2 and 3, respectively and for ionic/covalent bond the loadings are 0.71 and 0.72 for Model 2 and Model 3. This situation indicates that the bond energy concept, as measured by the TMI, does not really function well with the other two concepts as an indicator of overall understanding.
 |
| Fig. 5 Path diagram with the standardized parameter coefficients for Model 2. | |
 |
| Fig. 6 Path diagram with the standardized parameter coefficients for Model 3. | |
Relationships among the three predictors.
Table 6 presents the relationship among the three predictors as measured by correlation coefficients for the three models. For Model 2, the correlation between math ability and prior conceptual knowledge is 0.61, between math ability and attitude toward chemistry is 0.29, and between attitude toward chemistry and conceptual knowledge 0.48. These correlation values are considered moderate, and these results are consistent with literature related to math ability and conceptual understanding (Holme and Murphy, 2011; Nicoll and Francisco, 2001), math ability and attitude toward chemistry (Brandriet et al., 2011; Xu and Lewis, 2011), and attitude toward chemistry and conceptual understanding (Kim and Song, 2010; Nieswandt, 2007).
Table 6 Standardized correlation coefficients
|
Model 1 |
Model 2 |
Model 3 |
Note: r = 0.1, 0.3, 0.5 for weak, medium and strong effect size (Cohen, 1988, p. 78). |
Math ability – prior conceptual knowledge |
0.37 |
0.61 |
0.64 |
Prior conceptual knowledge – attitude |
0.28 |
0.48 |
0.52 |
Math ability – attitude |
0.25 |
0.29 |
0.29 |
The correlation coefficients from Model 2 are comparable with those of Model 3. However, since Model 1 did not account for measurement error, the correlation for Model 1 is underestimated. For example, math ability is moderately correlated to prior conceptual knowledge with r at 0.37 for Model 1, but a much higher correlation is observed for Models 2 and 3 with the estimates for r at 0.61 and 0.64, respectively.
Relationships with student achievement in college chemistry.
The next part of the model concerns the relationships between achievement and the predictors. Standardized path coefficients and effect sizes from the three models are shown in Table 7. All path coefficients achieved significance. For Model 1, the path coefficient from attitude to achievement is 0.19, which indicates a positive, though small (f
2 = 0.04), effect on achievement. The path between math ability and achievement is 0.49, which indicates a strong positive effect (f
2 = 0.32). The path coefficient between the third predictor, prior conceptual knowledge and achievement is 0.20, which again indicates a small (f
2 = 0.04) positive effect of the conceptual knowledge on achievement.
Table 7 Standardized path coefficient and effect size for the regression on achievement
|
Model 1 |
Model 2 |
Model 3 |
Parameter estimate |
Path coefficient |
Effect size f 2 |
Path coefficient |
Effect size f 2 |
Path coefficient |
Effect size f 2 |
Note: f 2 = 0.02, 0.15, and 0.35 for small, medium and large effect size (Cohen, 1988, p. 413). |
Math ability |
0.49 |
0.32 |
0.36 |
0.15 |
0.62 |
0.62 |
Attitude |
0.19 |
0.04 |
0.16 |
0.03 |
0.28 |
0.09 |
Prior concept |
0.20 |
0.04 |
0.50 |
0.33 |
— |
— |
Model 2 assessed the relationship of achievement with three predictors. First, the path coefficient between math ability and achievement is 0.50, which indicates a strong (f
2 = 0.33) positive effect. The path coefficient from attitude to achievement is 0.16, which indicates a small (f
2 = 0.03) positive effect on achievement. The path coefficient between prior conceptual knowledge and achievement is 0.36, which indicates a moderate (f
2 = 0.15) positive effect of the conceptual knowledge on achievement. For Model 3, the path coefficient from math ability to achievement increases to 0.62, a much larger (f
2 = 0.62) effect, and from attitude to 0.28, a slightly larger but still small (f
2 = 0.09) effect. In other words, when the prior conceptual knowledge is not included in the model, an overestimation of the path coefficients for math ability and attitude is observed. This overestimation is most obvious for math ability.
Discussion and implications
This study examined three alternate models to predict student achievement in introductory college chemistry. All three proposed models have reasonable fit to account for variance/covariance in the observed variables. We found that Model 2 can best represent the relationships among these achievement-relevant variables, based on the model fit, chi-square change when removing one predictor, and the proportion of variance accounted for student achievement in chemistry.
Each hypothesis was addressed in this study. First, the model results suggest a significant effect for each predictor on the performance of college chemistry. Based on Model 2, each predictor contributed a unique portion for prediction. Students’ achievement as measured by an ACS exam at the end of the semester can be mostly predicted by the combination of predictors at as early as the second week of the semester. This means, besides student math ability, both prior conceptual knowledge and attitude toward chemistry exert a significant unique effect on student achievement. This finding has a very important implication for instructors, since they can improve students’ achievement in chemistry not only by focusing on helping students to build conceptual knowledge, but also by fostering their positive attitude toward chemistry.
Second, the model suggests significant relationships among the predictors of attitude, math ability, and prior chemistry conceptual knowledge as shown in Fig. 5 and 6. These results imply that instructors may emphasize the interaction of multiple aspects in the classroom as a way to help students’ performance in the course.
Third, the comparison of the nested models suggests that Model 2 fits the data better than Model 3. Although math ability is often used as a proxy for cognitive factors to predict chemistry achievement in the literature, the importance of student conceptual knowledge should not be diminished. Leaving out prior conceptual knowledge resulted in significant over-estimation of the importance of math ability. This clear result should encourage instructors to re-think simplistic math-focused instructional interventions, which are likely to be insufficient to maximize improvement in students’ potential for achievement in college-level general chemistry.
Fourth, the amount of explained variance in students’ achievement significantly increased with a SEM model (Model 2 and 3) as opposed to a path analysis model (Model 1). Model 1 predicts only 46% of the variance in achievement, since the measurement tools used are assumed to be perfect and the prediction effect tends to be underestimated. Model 2 predicts 69% of the variance in student achievement by taking into account the measurement error associated with each observed variable. Model 3 predicts less than Model 2 due to missing one predictor, prior conceptual knowledge. Therefore, the accuracy of predicting achievement can significantly decrease if measurement error is neglected, and the same is true if important predictors are absent.
Some limitations are present in this study. First, the sample was drawn from a unique population, a single semester of a general chemistry course at a particular institution; therefore, the model may work for this unique context but not apply to other situations. Second, an appropriate assessment that covers a broader range of concepts that students need to know when they enter college chemistry course needs to be developed. In this study, the prior knowledge exam is based on three important concepts, but there is evidence that it does not represent the broad range of chemistry conceptual knowledge. If the measure is improved, we expect to have better prediction of achievement and model fit. Third, only three predictors were added to the model. Additional predictors were not explored, nor were alternative measurement strategies.
This study has several implications for chemistry education researchers with respect to further studies. In one hand, the obtained model needs to be replicated in another context. On the other hand, there are multiple ways to optimize the model. Due to the scarcity of SEM studies in chemistry education, multiple perspectives on aspects of SEM modeling, such as model specification, predictor choices, and operationalization and measurement of the variables, have yet to be explored.
Considering that there is 31% of variance that is not explained by the model in the study, future studies can explore what other factors could explain the rest of the achievement. For example, adding more appropriate predictors or mediating variables can help better explain the relationships and improve prediction. Other factors such as spatial ability (Carter et al., 1987; Merchant et al., 2012; Pribyl and Bodner, 1987), self-efficacy (Kan and Akbas, 2006; Merchant et al., 2012; Nieswandt, 2007; Uzuntiryaki and Aydin, 2009), or instructional strategy (Merchant et al., 2012; Schroeder et al., 2007) are reported as factors influencing student achievement in previous studies. More work is needed to capture the broad range of factors that are potentially important to understand chemistry learning. Moreover, the operationalization and measurement of key variables can be refined to better reflect the learning theory and empirical data. In sum, whether the necessary predictors are included and how well they are measured will affect the model fit and change the magnitude of path coefficients. By trying multiple combinations of models in multiple samples, over time researchers can achieve a better understanding of interactions among factors influencing student achievement in chemistry, help to build discipline-based learning theory, and provide information to support curriculum innovation.
References
- Abbott R. D. and Berninger V. W., (1993), Structural equation modeling of relationships among developmental skills and writing skills in primary-grade and intermediate-grade writers. J. Educ. Psychol.,85(3), 478–508.
- Abdullah M., Mohamed N. and Ismail Z. H., (2009), The effect of an individualized laboratory approach through microscale chemistry experimentation on students' understanding of chemistry concepts, motivation and attitudes. Chem. Educ. Res. Pract.,10(1), 53–61.
- Barbera J., Adams W. K., Wieman C. E. and Perkins K. K., (2008), Modifying and validating the Colorado Learning Attitudes about Science Survey for use in chemistry. J. Chem. Educ.,85(10), 1435–1439.
- Bauer C., (2008), Attitude towards chemistry: A semantic differential instrument for assessing curriculum impacts. J. Chem. Educ.,85(10), 1440–1445.
- Berg C. A. R., (2005), Factors related to observed attitude change toward learning chemistry among university students. Chem. Educ. Res. Pract.,6(1), 1–18.
- Brandriet A. R., Xu X., Bretz S. L. and Lewis J. E., (2011), Diagnosing changes in attitude in first-year college chemistry students with a shortened version of Bauer's semantic differential. Chem. Educ. Res. Pract.,12(2), 271–278.
- Brown T. A., (2006), Confirmatory factor analysis for applied research. New York: Guilford Press.
- Bunce D. M. and Hutchinson K. D., (1993), The use of the GALT (group assessment of logical thinking) as a predictor of academic-success in college chemistry. J. Chem. Educ.,70(3), 183–187.
- Cakici Y., Aricak O. T. and Ilgaz G., (2011), Can ‘attitudes toward biology course' and ‘learning strategies' simultaneously predict achievement in biology? Egit. Arast.,11(45), 31–48.
- Carter C. S., Larussa M. A. and Bodner G. M., (1987), A study of two measures of spatial ability as predictors of success in different levels of general chemistry. J. Res. Sci. Teach.,24(7), 645–657.
- Cohen J., (1988), Statistical power analysis for the behavioral sciences (2nd ed.). Hillsdale, NJ: Erlbaum.
- Coll R., Dalgety J. and Salter D., (2002), The development of the chemistry attitudes and experiences questionnaire (CAEQ). Chem. Educ. Res. Pract.,3(1), 19–32.
- Cooper C. and Pearson P., (2012), A genetically optimized predictive system for success in general chemistry using a diagnostic algebra test. J. Sci. Educ. Technol.,21(1), 197–205.
- Cukrowska E., Staskun M. G. and Schoeman H. S., (1999), Attitudes towards chemistry and their relationship to student achievement in introductory chemistry courses. South Afr. J. Chem.-Suid-Afr. Tydskr. Chem.,52(1), 8–14.
- Curran P. J., West S. G. and Finch J. F., (1996), The robustness of test statistics to nonnormality and specification error in confirmatory factor analysis. Psychol. Methods,1(1), 16.
- Danipog D. L. and Ferido M. B., (2011), Using art-based chemistry activities to improve students' conceptual understanding in chemistry. J. Chem. Educ.,88(12), 1610–1615.
- Dochy F., Segers M. and Buehl M. M., (1999), The relation between assessment practices and outcomes of studies: The case of research on prior knowledge. Rev. Educ. Res.,69(2), 145–186.
- Eagly A. H. and Chaiken S., (1993), The psychology of attitudes. Fort Worth, TX: Harcourt Brace Jovanovich.
- Ebenezer J., Chacko S., Kaya O. N., Koya S. K. and Ebenezer D. L., (2010), The effects of Common Knowledge Construction Model sequence of lessons on science achievement and relational conceptual change. J. Res. Sci. Teach.,47(1), 25–46.
- Ewing M., Huff K. Andrews M. and King K., (2005), Assessing the reliability of skills measured by the SAT® (Office of Research and Analysis): The College Board.
- Examinations Institute, (1997), The American Chemical Society Division of Chemical Education, First Term General Chemistry Special Examination (Form 1997): Clemson University: Clemson, SC.
- Finney S. J. and DiStefano C., (2006), Non-normal and categorical data in structural equation modeling. In G. R. Hancock and R. O. Muller (Eds.), Structural Equation Modeling: A Second Course (pp. 269–314). Greenwich, CN: Information Age Publishing.
- Freedman M. P., (1997), Relationship among laboratory instruction, attitude toward science, and achievement in science knowledge. J. Res. Sci. Teach.,34(4), 343–357.
- Gabel D., (1999), Improving teaching and learning through Chemistry Education Research: A look to the future. J. Chem. Educ.,76(4), 548–554.
- Goerzen A. and Beamish P. W., (2005), The effect of alliance network diversity on multinational enterprise performance. Strategic Manage. J.,26(4), 333–354.
- Gooding C. T., Swift J. N., Schell R. E., Swift P. R. and McCroskery J. H., (1990), A causal-analysis relating previous achievement, attitudes, discourse, and intervention to achievement in biology and chemistry. J. Res. Sci. Teach.,27(8), 789–801.
- Grove N. and Bretz S. L., (2007), CHEMX: An instrument to assess students' cognitive expectations for learning chemistry. J. Chem. Educ.,84(9), 1524–1929.
- Hemmings B., Grootenboer P. and Kay R., (2011), Predicting mathematics achievement: the influence of prior achievement and attitudes. Int. J. Sci. Math. Educ.,9(3), 691–705.
- Heredia K., (2012), Refinement, application, and evaluation of chemistry measures for college students. Unpublished work, Department of Chemistry, University of South Florida.
- Heredia K. and Lewis J. E., (2012), A psychometric evaluation of the Colorado Learning Attitudes about Science Survey for use in chemistry. J. Chem. Educ.,89(4), 436–441.
- Heredia K., Xu X. Y. and Lewis J. E., (2012), The application and evaluation of a two-concept diagnostic instrument with students entering college general chemistry. Chem. Educ. Res. Pract.,13(1), 30–38.
- Holme T. and Murphy K., (2011), Assessing conceptual and algorithmic knowledge in general chemistry with acs exams. J. Chem. Educ., 88(9), 1217–1222.
- House D. J., (1995), Noncognitive predictors of achievement in introductory college chemistry. Res. Higher Educ.,36(4), 473–490.
- Hu L. and Bentler P. M., (1999), Cutoff criteria for fit indexes in covariance structure analysis: Conventional criteria versus new alternatives. Struct. Equation Model.,6, 1–55.
- Kan A. and Akbas A., (2006), Affective factors that influence chemistry achievement (attitude and self efficacy) and the power of these factors to predict chemistry achievement-1. J. Turk. Sci. Educ.,3(1), 76–85.
- Kaynak H., (2003), The relationship between total quality management practices and their effects on firm performance. J. Oper. Manage.,21(4), 405–435.
- Kim M. and Song J., (2010), A confirmatory structural equation model of achievement estimated by dichotomous attitudes, interest, and conceptual understanding. Eurasia J. Math. Sci. Technol.,6(4), 271–285.
- Kline R. B., (2010), Principles and Practice of Structural Equation
Modeling (3rd ed.). New York: Guilford Press.
- Lewis S. E. and Lewis J. E., (2007), Predicting at-risk students in general chemistry: comparing formal thought to a general achievement measure. Chem. Educ. Res. Pract.,8(1), 32–51.
- Lewis S. E. and Lewis J. E., (2008), Seeking effectiveness and equity in a large college chemistry course: An HLM investigation of Peer-Led Guided Inquiry. J. Res. Sci. Teach.,45(7), 794–811.
- Lin H. S., Lee S. T. and Treagust D., (2005), Chemistry teachers' estimations of their students' learning achievement. J. Chem. Educ.,82(10), 1565–1569.
- MacCallum R. C. and Austin J. T., (2000), Applications of structural equation modeling in psychological research. Annu. Rev. Psychol.,51, 201–226.
- Maio G. and Haddock G., (2010), The psychology of attitudes and attitude change. Thousand Oaks: Sage Publications.
- Merchant Z., Goetz E. T., Keeney-Kennicutt W., Kwok O. M., Cifuentes L. and Davis T. J., (2012), The learner characteristics, features of desktop 3D virtual reality environments, and college chemistry instruction: A structural equation modeling analysis. Comput. Educ.,59(2), 551–568.
- Mulford D. R. and Robinson W. R., (2002), An inventory for alternate conceptions among first-semester general chemistry students. J. Chem. Educ.,79(6), 739–744.
- Nakhleh M. B., (1992), Why some students don't learn chemistry: Chemical misconceptions. J. Chem. Educ.,69(3), 191–196.
- Nicoll G., (2001), A report of undergraduates' bonding misconceptions. Int. J. Sci. Educ.,23(7), 707–730.
- Nicoll G. and Francisco J. S., (2001), An investigation of the factors influencing student performance in physical chemistry. J. Chem. Educ.,78(1), 99.
- Nieswandt M., (2007), Student affect and conceptual understanding in learning chemistry. J. Res. Sci. Teach.,44(7), 908.
- Othman J., Treagust D. F. and Chandrasegaran A. L., (2008), An investigation into the relationship between students' conceptions of the particulate nature of matter and their understanding of chemical bonding. Int. J. Sci. Educ.,30(11), 1531–1550.
- Pedhazur E. J., (1997), Multiple regression in behavioral research (3rd ed.). Fort Worth: Harcourt Brace.
- Pienta N. J., (2003), A placement examination and mathematics tutorial for general chemistry. J. Chem. Educ.,80(11), 1244.
- Pribyl J. R. and Bodner G. M., (1987), Spatial Ability and its Role in Organic Chemistry: a Study of Four Organic Courses. J. Res. Sci. Teach.,24, 229.
- Raykov T. and Marcoulides G., (2006), A first course in Structural Equation Modeling (2nd ed.). New York: Psychology Press: Taylor & Francis Group.
- Regan A., Childs P. and Hayes S., (2011), The use of an intervention programme to improve undergraduate students' chemical knowledge and address their misconceptions. Chem. Educ. Res. Pract.,12(2), 219–227.
- Rosenberg M. J. and Hovland C. I., (1960), Cognitive, affective & behavioral components of attitudes. In C. I. Hovland and M. J. Rosenberg (Eds.), Attitude Organization & Change: an analysis of consistency among attitude components (p. 3). New Haven, CT: Yale University Press.
- Schreiber J. B., (2008), Core reporting practices in structural equation modeling. Res. Soc. Administrative Pharm.,4(2), 83–97.
- Schreiber J. B., Nora A., Stage F. K., Barlow E. A. and King J., (2006), Reporting structural equation modeling and confirmatory factor analysis results: A review. J. Educ. Res.,99(6), 323–337.
- Schroeder C. M., Scott T. P., Tolson H., Huang T. Y. and Lee Y. H., (2007), A meta-analysis of national research: Effects of teaching strategies on student achievement in science in the United States. J. Res. Sci. Teach.,44(10), 1436–1460.
- Scott F. J., (2012), Is mathematics to blame? An investigation into high school students' difficulty in performing calculations in chemistry. Chem. Educ. Res. Pract.,13(3), 330–336.
- Seery M. K., (2009), The role of prior knowledge and student aptitude in undergraduate performance in chemistry: a correlation-prediction study. Chem. Educ. Res. Pract.,10(3), 227–232.
- Spencer H. E., (1996), Mathematical SAT test scores and college chemistry grades. J. Chem. Educ.,73(12), 1150–1153.
- Stamovlasis D., Tsitsipis G. and Papageorgiou G., (2012), Structural equation modeling in assessing students' understanding of the state changes of matter. Chem. Educ. Res. Pract.,13(3), 357–368.
- Tuan H. L., Chin C. C. and Shieh S. H., (2005), The development of a questionnaire to measure students' motivation towards science learning. Int. J. Sci. Educ.,27(6), 639–654.
- Uzuntiryaki E. and Aydin Y. C., (2009), Development and validation of chemistry self-efficacy scale for college students. Res. Sci. Educ.,39(4), 539–551.
- Valiente C., Lemery-Chalfant K. and Reiser M., (2007), Pathways to problem behaviors: Chaotic homes, parent and child effortful control and parenting. Soc. Dev.,16(2), 249–267.
- Wagner E. P., Sasser H. and DiBiase W. J., (2002), Predicting students at risk in general chemistry using pre-semester assessments and demographic information. J. Chem. Educ.,79(6), 749–755.
- Wei M. F., Heppner P. P. and Mallinckrodt B., (2003), Perceived coping as a mediator between attachment and psychological distress: A structural equation modeling approach. J. Counseling Psychol.,50(4), 438–447.
- Weinburgh M., (1995), Gender differences in student attitudes toward science—a metaanalysis of the literature from 1970 to 1991. J. Res. Sci. Teach.,32(4), 387–398.
- West S. G., Taylor A. B. and Wu. W., (2012), Model fit and model selection in structual equation modeling. In R. H. Hoyle (Ed.), Handbook of structural equation modeling (p. 211). New York: The Guilford Press.
- Xu X. and Lewis J. E., (2011), Refinement of a chemistry attitude measure for college students. J. Chem. Educ.,88(5), 561–568.
|
This journal is © The Royal Society of Chemistry 2013 |