Assessing the relation between language comprehension and performance in general chemistry†
Received
11th January 2013
, Accepted 10th August 2013
First published on 12th August 2013
Abstract
Few studies have focused specifically on the role that language plays in learning chemistry. We report here an investigation into the ability of language comprehension measures to predict performance in university introductory chemistry courses. This work is informed by theories of language comprehension, which posit that high-skilled comprehenders hold a cognitive advantage over the low-skilled because of a heightened ability to inhibit contextually irrelevant details and utilize prior knowledge to effectively bridge conceptual gaps when comprehending new information. Over a two-year period, data on comprehension ability, math ability, prior chemistry knowledge, and course performance were obtained in multiple general chemistry courses. Regression analyses and hierarchical linear models (HLMs) were utilized to establish relationships between predictor variables and course performance and to determine if comprehension ability could potentially compensate for low prior knowledge, a phenomenon predicted by theories of comprehension ability. Results indicate that comprehension ability correlates with general chemistry performance; it also contributes comparable information about course performance when compared to math ability and prior knowledge. In addition, we found that comprehension skill partially compensates for deficits in prior knowledge. Therefore, efforts to prepare students for success in general chemistry should include both content and the development of language comprehension skill.
Introduction
Student success in introductory university chemistry courses can be predicted by a number of variables. For example, math ability has received considerable attention in predictor studies (Kunhart et al., 1958; Schelar et al., 1963; Sieveking and Larson, 1969; Coley, 1973; Pickering, 1975; Andrews and Andrews, 1979; Ozsogomonyan and Loftus, 1979; Craney and Armstrong, 1985; Rixse and Pickering, 1985; Carmichael et al., 1986; Glover et al., 1991; Bunce and Hutchinson, 1993; Spencer, 1996; Wagner et al., 2002; Tai et al., 2005; Lewis and Lewis, 2007, 2008; Leopold and Edgar, 2008). Other work has focused on relevant prior knowledge in chemistry, as measured by either “placement tests” (Scofield, 1927; Smith and Trimble, 1929; Hovey and Krohn, 1958; Schelar et al., 1963; Sieveking and Larson, 1969; Coley, 1973; Albanese et al., 1976; Hunter, 1976; Ozsogomonyan and Loftus, 1979; Niedzielski and Walmsey, 1982; Craney and Armstrong, 1985; Russell, 1994; McFate and Olmsted, 1999; Legg et al., 2001; Wagner et al., 2002; Pienta, 2003; Bentley and Gellene, 2005; Mills and Sweeney, 2009; Seery, 2009), or high school chemistry grades (Kunhart et al., 1958; Coley, 1973; Ozsogomonyan and Loftus, 1979; Craney and Armstrong, 1985; Tai et al., 2005). While math ability and prior chemistry knowledge provide generic information concerning what factors contribute to student success in introductory chemistry courses, these constructs are not rooted in theories of learning or cognition. Thus, they are unable to suggest pedagogical strategies for remedial efforts, save “remedial courses” which have been implemented with mixed results (e.g. Bentley and Gellene, 2005; Botch et al., 2007; Schmid et al., 2012). A significant exception to these predictor studies includes work that demonstrates correlations between formal reasoning and chemistry performance (Bender and Milakofsky, 1982; Bunce and Hutchinson, 1993; Lewis and Lewis, 2007). Instructors can design classroom interventions for those students who reason at the concrete level using Piagetian theory (Jiang et al., 2010), from which the formal reasoning construct is drawn (Inhelder and Piaget, 1958). Further work with predictor variables whose theoretical bases present clear guidelines for the development of pedagogical strategies is needed in chemistry education research.
We believe that language comprehension is a key variable for predicting performance in introductory chemistry. Quantitative measures of this construct have received scant attention over the 85-year history of work with predictor variables. This may be due to a long-held bias among chemistry educators that math ability is more essential to success: “…freshmen chemistry is significantly more correlated (p < 0.001) with math SAT [than verbal SAT], a result expected, but not rigorously proven, in previous work” (Rixse and Pickering, 1985, p. 313). This comment notwithstanding, language comprehension scores have been shown to be statistically significant predictors of various chemistry learning outcomes (Carmichael et al., 1986; Glover et al., 1991; Bunce and Hutchinson, 1993; Lewis and Lewis, 2007, 2008), with reported effect sizes ranging from small to large.
Chemistry education researchers and practitioners alike have documented issues with language and learning chemistry. Like the aforementioned quantitative correlations, however, these instances are few. Seminal work by Cassels and Johnstone (1980) with pre-college students demonstrated non-technical words associated with chemistry were often a cause of alternative conceptions. At the university level, Jasien and Oberem (2008) and Jasien (2010, 2011) documented students' confusion with the terms dense, energy, neutral and strong, while Hamori and Muldrey (1984) mused on the ambiguity of the term spontaneous in the context of teaching thermodynamics. Additional work by Cassels and Johnstone (1985) recognized that language is a contributor to information overload and, consequently, limits a student's ability to solve problems. Potential issues beyond lexical ambiguity included unfamiliar/misleading vocabulary, use of “high-sounding” language, and the use of multiple negatives. Many of these language issues are further amplified in students for whom English is a foreign language (Johnstone and Selepeng, 2001; Childs and O'Farrell, 2003). Gabel (1999) echoed much of Johnstone's work by noting that the difficulties students have with chemistry might not be related to the subject matter itself but to how chemistry knowledge is linguistically expressed. Middlecamp and Kean (1988) lamented it “is not uncommon for 20 or more concepts to be introduced in the course of a one-hour lecture. Small wonder that some students perceive chemistry to be a language course!” (p. 54). Ver Beek and Louters (1991) concluded from their study, which cleverly divorced chemical language from common language and math ability, “the difficulties experienced by our beginning college chemistry students appear to be largely precipitated by a lack of chemical language skill rather than by a lack of native reasoning and/or mathematical skills.” (p. 391). Indeed, language is fundamental to the teaching and learning of chemistry, as it is to all sciences. The relationship is so profound that Bulman (1985, p. 1) proposed any school science department should work towards developing and improving language skills in its students. Toward this end, Herron (1996, pp. 161–182) devoted an entire chapter in his book The Chemistry Classroom to discussing this topic.
Background
Language comprehension as Structure Building
There are several prominent models of comprehension ability, as reviewed by McNamara and Magliano (2009). Structure Building serves as one framework for understanding the cognitive processes and mechanisms that contribute to language comprehension. This framework describes how new information, both linguistic and non-linguistic, is incorporated into one's existing knowledge base (Gernsbacher, 1990). Structure Building adopts the view that language draws on many general cognitive processes and is not a specialized skill. Thus, some of the same processes and mechanisms involved in producing and comprehending language are involved in non-linguistic tasks.
Briefly, Structure Building describes an individual's prior knowledge as having produced a foundation to which new information can be linked. As new information relevant to this foundation is encountered, a new substructure is built—a connection between new information and relevant prior knowledge. If new information irrelevant to an active substructure is encountered, the corresponding prior knowledge base is suppressed as another prior knowledge base is activated, allowing a new substructure to be built. As one substructure is suppressed in favor of building a new one, the information encoded in the suppressed substructure becomes less accessible. Students with low comprehension ability are at a distinct disadvantage as they possess inefficient suppression mechanisms and are unable to inhibit irrelevant information (Gernsbacher and Faust, 1991). As a result, poor comprehenders attempt to accommodate information that will not fit into existing substructures and regularly shift to build new substructures. Consequently, these students readily lose access to recently encoded information and build less coherent structures of new information. In the context of Cassels and Johnstone's (1980, 1985) work, inefficient suppression mechanisms may explain why less-skilled comprehenders are unable to reject contextually inappropriate meanings of ambiguous words.
The role of prior knowledge in comprehension
Deep comprehension of content is presumed to emerge from strategies that prompt the learner to generate inferences connecting what is being learned to prior knowledge (McNamara and Magliano, 2009). These strategies include asking questions, answering questions, evaluating the quality of answers to questions, generating explanations, solving problems, and reflecting on the success of such strategies (Bransford et al., 2000, p. 67; Graesser et al., 2005; McNamara, 2010). In other words, skilled comprehenders are better able to use prior knowledge to fill in the conceptual gaps encountered when attempting to comprehend new information. Low-skilled comprehenders often fail to employ strategies necessary to improve comprehension at a deeper level (Lenski and Nierstheimer, 2002).
The relationship between prior chemistry knowledge and general chemistry course performance is robust and has been well documented (vide supra). Indeed, given the abundance of research concerning the role of domain knowledge in comprehending new material (Shapiro, 2004), it is expected that, in most situations, a student of higher prior chemistry knowledge would score better than a student of lower prior chemistry knowledge on measures of general chemistry achievement (e.g. Seery, 2009). Regrettably, previous work has shown that most students rarely enter courses with an appropriate amount of background knowledge (Snow, 2002). Moreover, learning materials (e.g. high school textbooks) do not necessarily contain enough background information for new information to be easily comprehended (Beck et al., 1989). Fortunately, it has been shown that comprehension ability can partially compensate for deficits in prior knowledge (Adams et al., 1995; O'Reilly and McNamara, 2007). For example, O'Reilly and McNamara report that high school students of low prior knowledge and high comprehension ability perform similarly to students of high prior knowledge and low comprehension ability on science achievement measures. Thus, the typical achievement deficit experienced by a student of low prior knowledge was greatly offset if the student possessed high comprehension ability. It is thought that the increased ability of high-skilled comprehenders to make inferences may enable them to overcome prior knowledge deficits.
Rationale and research questions
The relationship between language comprehension and performance in university-level introductory chemistry courses has been poorly characterized. In addition, the cognitive grounding of comprehension ability affords great insight into the role this construct plays in the teaching and learning of chemistry. In this study, we investigated language comprehension as a predictor of performance in three general chemistry courses. We also compared language comprehension ability to math ability, the historically better-studied performance predictor. Last, we examined whether comprehension ability may compensate for deficits in prior chemistry knowledge. The following three research questions framed our study:
(1) How strongly does language comprehension ability correlate with performance in general chemistry courses?
(2) How does language comprehension ability compare to math ability as a predictor of general chemistry performance?
(3) To what extent can high comprehension ability compensate for low prior knowledge in general chemistry courses?
Method
Design
Assessments of general chemistry course performance were treated as dependent measures. Our predictor variables included prior chemistry knowledge, language comprehension ability, and math ability.
Participants
Participants in this study included students enrolled in a traditional two-semester general chemistry sequence (Chem A and Chem B, respectively), and in a one-semester general chemistry course for engineering majors (Chem C) at a 4-year public research university with high research activity in the northeastern United States. Participants' demographic information (academic major, class standing, ethnicity, and sex) was collected from institutional records and is presented by course in Table 1. Data regarding participants' prior chemistry knowledge, language comprehension, and math ability were collected either by assessments administered at the beginning of each course or from institutional records. In each course, students also completed an assessment of course performance at the conclusion of each semester. Data screening and descriptive statistics for each measure described herein for each course are provided in Appendix 1 (ESI†). Accompanying these descriptive statistics is an evaluation of the data with regard to assumptions of the data analysis techniques described below. Institutional Review Board (IRB) approval was sought and determined to be exempt from IRB oversight because the research design was in keeping with normal classroom practices.
Table 1 Demographics of participants
|
Chem A |
Chem B |
Chem C |
Percent of students in the course sample for whom these data were available. |
Sex |
99.3%a |
64.1% female |
97.0% |
63.9% female |
97.1% |
16.9% female |
35.2% male |
36.1% male |
83.1% male |
|
Ethnicity |
48.8% |
90.4% White |
92.3% |
90.5% White |
66.5% |
92.1% White |
2.7% Hispanic or Latino |
2.7% non-Hispanic/2 or more races |
3.6% Asian |
2.5% non-Hispanic/2 or more races |
2.5% Asian |
2.0% Hispanic or Latino |
2.2% Asian |
2.3% Hispanic or Latino |
1.5% non-Hispanic/2 or more races |
1.7% Black or African American |
1.5% Black or African American |
|
|
Class standing |
97.7% |
38.1% first-years |
96.1% |
47.4% first-years |
93.4% |
62.9% first-years |
41.5% sophomores |
36.1% sophomores |
21.9% sophomores |
15.3% juniors |
12.0% juniors |
12.7% juniors |
7.2% seniors |
4.6% seniors |
2.5% seniors |
|
Academic major |
51.7% |
11.4% Biology |
96.8% |
11.8% Biology |
51.3% |
33.3% Mechanical Engineering |
10.4% Biomedical Science: Medical and Veterinary Sciences |
10.8% Biomedical Science: Medical and Veterinary Sciences |
25.4% Civil Engineering |
9.8% undeclared |
6.3% Nutritional Sciences |
15.5% Chemical Engineering |
5.9% Zoology |
5.8% Biochemistry, Molecular and Cellular Biology |
10.2% Electrical Engineering |
5.3% Biochemistry, Molecular and Cellular Biology |
5.2% Zoology |
5.6% Environmental Engineering: Municipal Processes |
Materials
Measures of course performance. General chemistry course performance constructs such as course grades, scores on internally-constructed final examinations, or even averages of internally constructed course midterms are rarely determined consistently across institutions. Given our desire to produce generalizable information concerning how language comprehension relates to chemistry course performance, this construct was assessed using exams produced by the American Chemical Society Division of Chemical Education Examinations Institute (American Chemical Society, 2013). The Examinations Institute offers more than fifty exams for high school and university chemistry courses. The content validity of the ACS exams used in this study was established by the Examinations Institute and by the instructors of Chem A, Chem B, and Chem C. (In general, students were not responsible for completing exam items that did not align with course objectives.) The ACS First Term General Chemistry Paired Questions Exam (form 2005) and the ACS Special Exam (1997) were administered as a portion of the final examination in Chem A and Chem B, respectively. The ACS General Chemistry (Conceptual) Exam (form 2008) was administered in Chem C as a portion of the final examination. Data for all ACS exams are reported herein as the percent of total items scored as correct.While using scores on ACS exams as a measure of course performance is advantageous in that doing so may allow results to be generalized beyond the research institution, relying on a single multiple-choice measure of course performance places limitations on the study. To strengthen our approach, results obtained using ACS exams in one course were validated with an alternative measure of course performance. Accordingly, scores from four instructor-generated midterm exams were collected in Chem C during each semester of the study. Data concerning reliability (inter-exam correlations, i.e., two-tailed Pearson correlations among the four course midterms, and Cronbach's alphas, where applicable) and convergent validity (correlations of each semester's midterm exams with an ACS exam) are presented in Table 2. During each of the four semesters of data collection, the four midterm exams were highly inter-correlated and had moderate to high correlations with the ACS General Chemistry (Conceptual) Exam given at the end of the semester. While Cronbach's alphas could not be calculated for midterm exams in semesters 1 and 2 of data collection (see Table 2), midterm exams used in semesters 3 and 4 had adequate internal consistency (α = 0.73–0.85). Even though the content of midterm exams did not differ from semester to semester, the questions comprising each midterm exam did. Thus, we report herein exam scores standardized to respective semester exam means.
Table 2 Reliability and validity information for Course C instructor-generated midterm exams
Semester of data collection |
Cronbach's alphas |
Inter-exam correlations |
Correlations with ACS General Chemistry (Conceptual) Exam |
Cronbach's alphas are not available for midterm exams during these semesters, as these exams presented choices to students; therefore, not all exam items were answered by all students. |
1 |
a |
r = 0.61–0.72 |
r = 0.59–0.66 |
2 |
a |
r = 0.50–0.70 |
r = 0.49–0.52 |
3 |
0.83–0.85 |
r = 0.67–0.75 |
r = 0.48–0.58 |
4 |
0.73–0.82 |
r = 0.58–0.70 |
r = 0.53–0.65 |
Measures of prior chemistry knowledge. ACS exams were also used to assess prior chemistry knowledge. The ACS Toledo Chemistry Placement Exam (form 2009) was used for Chem A and Chem C. This exam was originally designed for placing undergraduate students into chemistry courses (Hovey and Krohn, 1963) and consists of three sections, each containing 20 multiple-choice questions. The first section contains items that assess math ability, while the second and third sections assess general and specific chemistry knowledge, respectively. For this study, the average of scores from sections two and three served as a measure of a student's prior chemistry knowledge. In our research scenario, the ACS Toledo Chemistry Placement Exam had a Kuder–Richardson Formula 20 (KR-20) of 0.68 in Chem A and 0.70 in Chem C. As only the latter two sections of the exam were used in this study, the performance of our sample on the ACS Toledo Chemistry Placement Exam could not be compared to national norms. For Chem B, the First Term subset of General Chemistry (Conceptual) (form 2008) was used to assess prior knowledge, as the Toledo Placement Exam was deemed inappropriate for assessing the prior knowledge of students enrolled in the second semester of a two-semester general chemistry sequence. The first term subset of General Chemistry (Conceptual) had a KR-20 of 0.79. At the time of publishing this report, national norms were not available for this exam. Data for all prior knowledge measures are reported herein as the percent of total items scored as correct.
Measures of language comprehension ability. Scholastic Aptitude Test Critical Reading (SAT-CR) section‡ scores were used for all three chemistry courses. The SAT is a standardized exam taken by students pursuing entrance into an undergraduate program in the United States. At the time of data collection, the SAT-CR section consisted of all multiple-choice questions that could be categorized as either passage-based reading or sentence completions (Educational Testing Service, 2013a). The passage-based reading questions drew content from the natural sciences, humanities, social sciences, and literary fiction; questions combined narrative, argumentative, and expository elements. Skills measured by the SAT-CR section included determining word meaning; understanding sentences; understanding larger sections of text; and analyzing purpose, audience, and strategies (Ewing et al., 2005). Internal consistency estimates for SAT-CR sections have been found to be high (Cronbach's alpha > 0.90) (Ewing et al., 2005). SAT-CR data are reported herein as raw scores (the maximum score was 800).As an alternative measure of comprehension ability, the Gates-MacGinitie Reading Test (Comprehension 10/12 – Form S, 4th edition) was administered to students in Chem C (MacGinitie et al., 2000a). The Gates-MacGinitie Reading Test (GMRT) consisted of 48 multiple-choice questions designed to assess student comprehension on several short text passages. All passages were taken from published books and periodicals; the content was selected to reflect the types of material that students are required to read as academic work as well as choose to read for recreation. As we were interested only in comprehension and not vocabulary scores in this study, the vocabulary section of the GMRT was not administered. The internal consistency of the GMRT used in Chem C was high (KR-20 = 0.90). Scores on the GMRT were also strongly correlated (r = 0.65, Appendix 2, ESI†) with scores on the SAT-CR section. GMRT data are reported as the percent of total items scored as correct.
Comparing the performance of the Chem C sample on the GMRT to national norms requires some discussion. The Comprehension 10/12 – Form S test was designed for a high school population; norms are published for the Fall, Winter, and Spring time points during students' final year in secondary school (MacGinitie et al., 2000b). Considering 63% of students enrolled in Chem C were first-year university students, the most reasonable norm to which we could compare this population was that for the Spring time point. Taking this into consideration, the average score on this instrument for students in Chem C (67.68%) fell at the 59th percentile of graduating high school seniors. This mean score equated to a post-high school grade level, as expected of students enrolled in a university-level chemistry course. However, approximately 22.2% of students scored below the 35th percentile of graduating high school seniors. This percentile equated to comprehension abilities below the national average score for 9th graders.
Measure of math ability. Scores on the mathematics section of the SAT were used as the measure of math ability for all three chemistry courses. At the time of data collection, the SAT-Math section consisted of multiple-choice and student-produced response questions (Educational Testing Service, 2013b). The content of questions included numbers and operations; algebra and functions; geometry and measurement; and data analysis, statistics, and probability. SAT-Math data are reported as raw scores (the maximum score was 800). Internal consistency estimates for SAT-Math sections have been found to be high (Cronbach's alpha > 0.90) (Ewing et al., 2005).
Descriptions of research settings and populations
Chem A is the first course of a two-semester “general chemistry” sequence. Topics covered in Chem A included chemical nomenclature, stoichiometry, solution chemistry, gases, thermochemistry, atomic theory, chemical bonding, and molecular structure. Course performance data (ACS First Term General Chemistry Paired Questions Exam scores) were collected from seven Chem A classes (N = 955). These seven classes were lead by three different instructors who used the same textbook. Institutional records indicate that 38.1% of the students in Chem A were in the first year at the university, 64.1% were female, and 90.4% White. Majors relating to the Life Sciences were among the most common for students enrolled in Chem A. SAT data were available for 84.5% of this sample; prior chemistry knowledge data (Toledo exam scores) were available for 91.5%. Examination of these data revealed no significant differences in ACS exam score between students that were missing SAT/Toledo exam data and those that were not; thus, missing data did not bias the Chem A sample.
Chem B is the second course in the two-semester general chemistry sequence. Topics covered in Chem B included intermolecular forces, valence bond theory, introductory organic chemistry, chemical equilibrium, acids/bases, chemical kinetics, chemical thermodynamics, and electrochemistry. Course performance data (ACS Special Exam scores) were collected from three Chem B classes (N = 567). These three classes were led by three different instructors who used the same textbook. Institutional records indicate that 47.7% of the students in Chem B were in the first year at the university, 63.9% were female, and 90.5% White. As in Chem A, majors relating to the Life Sciences were among the most common for students enrolled in Chem B. SAT data were available for 86.2% of this sample; prior chemistry knowledge data (General Chemistry (Conceptual), First Term section scores) were available for 90.0%. Examination of these data revealed no significant differences in course performance between students that were missing data and those that were not; thus, missing data did not bias the Chem B sample.
Chem C is a one-semester general chemistry course designed primarily for engineering majors. Topics covered in Chem C included atomic theory, solid state structure, chemical bonding, molecular structure, intermolecular forces, chemical reactions and stoichiometry, introductory organic chemistry, thermochemistry and thermodynamics, chemical kinetics, chemical equilibrium, acids/bases, and electrochemistry. General Chemistry (Conceptual) Exam data and internally constructed midterm exam data were collected from four Chem C classes (N = 578). These classes were lead by the same instructor whose textbook choice, topic coverage, lecture notes, and presentation aids were consistent across the four semesters of data collection. Institutional records indicate that 62.9% of the students in Chem C were in the first year at the university, 16.9% were female, and 92.1% White. Mechanical engineering and civil engineering were the most common majors for students enrolled in Chem C. SAT data were available for 91.1% of this sample; prior chemistry knowledge data (Toledo exam scores) were available for 93.7%; GMRT scores were available for 91.3%; and all four midterm scores were available for 96% of the sample. Examination of these data revealed no significant differences in course performance between students that were missing data and those that were not; thus, missing data did not bias the Chem C sample.
Data analysis and results
As stated above, three research questions guided this study. Fundamentally, each question focused on the relationship of language comprehension ability and general chemistry course performance; linear regression techniques should therefore be suitable data analysis methods. However, the data described above were collected at different levels of analysis; such data sets are “nested” and may violate assumptions of independence in linear regression techniques. For example, in Chem C, we observed repeated measures of student performance (midterm exam scores) over time; these multiple observations were not independent of one another, as each was nested within an individual student. Moreover, in all courses, we observed singular measures of performance (ACS exam scores) for students nested in classroom units. Students nested within a class often have multiple opportunities to influence the learning of one another (Saxe et al., 1999), e.g. by asking questions of the instructor, peer-to-peer discussions, or small group work. An individual student's data may therefore be dependent on peers by virtue of the social nature of any university course. Thus, the nature of our data may violate the independence of observations assumption of linear regression.
Hierarchical linear models (HLMs) are those in which nested data may be studied without violating assumptions of independence (Raudenbush and Bryk, 2002). HLMs have become an important tool for investigating nested data in discipline-centered science education research, e.g. for students nested within physics classrooms (Tai and Sadler, 2001; Lawrenz et al., 2009), or for analyzing multiple measures of chemistry performance over time (Lewis and Lewis, 2008). HLMs account for the inter-dependencies of nested data by estimating the variance associated with group (e.g. a classroom) differences in average outcome and group differences in relationships between predictors and the outcome (e.g. individual student differences in the relationship between language comprehension and performance). This is accomplished by declaring intercepts and/or slopes at higher levels to be random effects.
The extent to which assumptions of independence were violated in our nested data was evaluated by comparing the variance intrinsic to a particular level to the total variance in the data set. This ratio is known as the intraclass correlation, ρ (Raudenbush and Bryk, 2002, p. 36). High intraclass correlations imply that the assumption of independence has been violated; i.e., HLMs are more appropriate than regression for analyzing the data. In Chem C, variance parameter estimates indicated that level-2 (between-classroom) effects accounted for relatively little variance in ACS exam score as compared to between-students variance (ρ = 0.021). We concluded from this that the class itself had little effect on relationships involving ACS exam score in Chem C. Treating students nested in classrooms as independent was therefore a valid approximation (Tai et al., 2005; Lewis and Lewis, 2008). We drew similar conclusions for Chem A and Chem B data (Appendix 3, ESI†). Thus, linear regression techniques were chosen to analyze ACS exam data in all three courses. When repeated course midterm exam scores were used as the outcome variable in Chem C, however, the intraclass correlation at level-2 (between-students) was quite large (ρstudent = 0.625). Given that the variation in standardized midterm scores between classes was negligible, we employed two-level HLMs to analyze Chem C midterm data.
We organized this Data analysis and results section by research question and, for clarity, presented only the data analyses and results for Chem C as exemplars. While results from Chem A and Chem B are discussed in context, a full presentation of these data was reserved for the Appendices (ESI†). For each research question, we presented the analysis of ACS exam data first, followed by analysis of midterm exam data. Analysis of these independent data sets generates a richer understanding of the effects of language comprehension on performance than either set alone could provide. All analyses were performed using IBM SPSS Statistics, Version 21.
How strongly does language comprehension ability correlate with performance in general chemistry courses?
ACS exams. As between-classroom variance in ACS exam scores was determined to be negligible, Chem C data were pooled across semesters and Pearson correlations were calculated to establish relationships between language comprehension ability and course performance as measured by ACS exams. Pearson's r is broadly used as a measure of the strength of linear dependence between two variables. The relative magnitudes of Pearson correlations were interpreted using the qualitative guidelines described by Cohen (1988): small (0.10), medium (0.30) and large (0.50). When SAT-CR scores were used to predict ACS exam scores, r(528) = +0.45, p < 0.001. When GMRT scores were used to predict ACS exam scores, r(528) = +0.40, p < 0.001. Thus, statistically significant positive correlations with medium effect sizes were found using two different measures of language comprehension. Nearly identical results were found in Chem A and Chem B; these data are presented in Appendix 2 (ESI†).
Midterm exams. Scores from instructor-generated midterm exams were collected in Chem C and used to validate the results obtained using ACS exam data. Two-level HLMs assessed the effect of language comprehension ability on course performance. Level-1 units were examination events during which students' exam scores were collected. We viewed these multiple observations on each individual as nested within the student, i.e. as repeated measures (Tabachnick and Fidell, 2013, p. 818). Four examination events occurred at approximately equal time intervals (3–4 weeks) in each class. Level-2 units were the students comprising the four Chem C classes. A third level comprised of classroom units was not necessary, given our use of standardized midterm exam scores. In these models, time served as a fixed effect at level-1, i.e. as a predictor of midterm exam score, so as to account for the repeated measures nature of the data. Language comprehension ability was declared a fixed effect at level-2. Scores on language comprehension ability measures were standardized to facilitate comparisons between the different measures of this parameter (SAT-CR section vs. GMRT). Students were declared a random effect at level-2 to assess variability among students within classes. One of the fixed effects, time, was also declared a random effect at level-2, reflecting the possibility of individual differences in performance growth rate. Further details concerning these HLMs can be found in Appendix 4 (ESI†).Results for HLMs assessing the relationship between language comprehension and course performance as measured by midterm exam scores are presented in Tables 3 and 4, using the notation discussed in the Appendices (ESI†). In both models, the mean initial status (β00) was not statistically different than zero, as expected when using standardized exam scores as the outcome measure. For the model that predicted performance from standardized SAT-CR section scores (Table 3), a one-standard deviation increase in SAT-CR score was associated with a 0.372-standard deviation increase in exam score (β01 = 0.372, p < 0.001). This result was very comparable to that for the model which predicted performance from standardized GMRT scores (β01 = 0.370, p < 0.001, Table 4). Regardless of language comprehension measure, the mean growth rate of student performance over time was not significantly different than zero (e.g., β10 = −0.005, p = 0.679, Table 3), nor was there any significant variation in growth rate among students (e.g., r1j = 0.010, p = 0.095, Table 3). Interestingly, however, a significant negative relationship was observed between language comprehension ability and growth rate in both models (e.g., β11 = −0.024, p = 0.050, Table 3). This relationship suggested that, on average, students of low comprehension ability improved in performance over time, albeit only modestly. Thus, statistically significant relationships were found between comprehension ability and course performance as measured by course midterm exams, validating the relationship observed when ACS exams were used as the measure of course performance.
Table 3 Results of a two-level model estimating the effect of language comprehension ability (as measured by SAT-CR section scores) on Chem C course performance (as measured by course midterm exams)
Fixed effect |
Estimate |
Standard error |
Approx. df |
t |
p |
Mean initial status, β00 |
0.020 |
0.037 |
534 |
0.533 |
0.594 |
Mean growth rate, β10 |
−0.005 |
0.012 |
533 |
−0.415 |
0.679 |
SAT-CR score effect, β01 |
0.372 |
0.037 |
535 |
−1.967 |
<0.001 |
SAT-CR score by time, β11 |
−0.024 |
0.012 |
533 |
−1.97 |
0.050 |
Random effect |
Variance |
Standard error |
Wald Z |
p |
Level-1: exam scores (residual, eij) |
0.349 |
0.015 |
22.950 |
<0.001 |
Level-2: student (initial status, r0j) |
0.493 |
0.046 |
10.608 |
<0.001 |
Level-2: student (covariance) |
−0.003 |
0.013 |
−0.247 |
0.805 |
Level-2: student (growth rate, r1j) |
0.010 |
0.006 |
1.671 |
0.095 |
Table 4 Results of a two-level model estimating the effect of language comprehension ability (as measured by GMRT scores) on Chem C course performance (as measured by course midterm exams)
Fixed effect |
Estimate |
Standard error |
Approx. df |
t |
p |
Mean initial status, β00 |
0.014 |
0.037 |
538 |
0.364 |
0.716 |
Mean growth rate, β10 |
0.0002 |
0.012 |
534 |
0.014 |
0.989 |
GMRT score effect, β01 |
0.370 |
0.037 |
539 |
9.923 |
<0.001 |
GMRT score by time, β11 |
−0.036 |
0.012 |
538 |
−2.915 |
0.004 |
Random effect |
Variance |
Standard error |
Wald Z |
p |
Level-1: exam scores (residual, eij) |
0.339 |
0.015 |
22.963 |
<0.001 |
Level-2: student (initial status, r0j) |
0.504 |
0.046 |
10.857 |
<0.001 |
Level-2: student (covariance) |
−0.0008 |
0.012 |
−0.064 |
0.949 |
Level-2: student (growth rate, r1j) |
0.010 |
0.006 |
1.706 |
0.088 |
While these results provided insight into the relationship between language comprehension and course performance, they did not directly provide information concerning the strength of the relationship. We thus considered effect sizes for these models. Following guidelines outlined by Peugh (2010, p. 107), we calculated correlations between predicted midterm exam scores (using coefficients obtained from the HLMs) and observed midterm exam scores. For the model using SAT-CR scores, r(2130) = 0.333, p < 0.001; for the model using GMRT scores, r(2131) = 0.320, p < 0.001. These correlations correspond to medium effect sizes, corroborating the results obtained when using ACS exams as measures of course performance.
How does language comprehension ability compare to math ability as a predictor of general chemistry performance?
ACS exams. As stated above, language comprehension ability exhibited a positive relationship with general chemistry course performance. Historically, however, the literature on predictors of general chemistry performance tends to preference math ability, this predictor's lack of cognitive grounding notwithstanding. To examine how comprehension ability and math ability compared as predictors of general chemistry performance, course performance was regressed on comprehension ability and math ability as predictors. As presented in Appendix 2 (ESI†), comprehension ability and math ability both exhibited positive linear relationships with ACS exam score. However, correlations between comprehension ability and math ability (r = +0.41 to +0.58) did not indicate extremely high multicollinearity. Prior to performing regressions, predictor variables were centered by subtracting the sample mean. In doing this, the intercept could be interpreted as the predicted outcome (ACS exam score) for students possessing average values of the predictor variables. Both standardized (β) and unstandardized (B) regression coefficients are reported in all cases. In our presentation, however, we focus on the standardized coefficients, which are interpreted as a change in the dependent variable by β standard deviations for every one standard deviation change in the predictor variable. This was done to facilitate comparisons between the different measures of comprehension ability, as standardized coefficients ignore the independent variable's scale of units. Squared semipartial correlations (sr2) for the predictor variables used in each regression are also reported in all cases. The squared semipartial correlation represents the portion of overall variance that each predictor variable uniquely predicts; sr2 can thus be interpreted as an effect size for a particular predictor variable when the other predictors are statistically controlled. To apply Cohen's qualitative guidelines (vide supra), the semipartial correlation (sr) is used.The results of regression analyses for Chem C are provided in Tables 5 and 6. For example, Table 5 shows that students who possessed average comprehension ability (as measured by SAT-CR scores) and math ability were predicted to score 64.6% on the ACS General Chemistry (Conceptual) Exam. A one-standard deviation increase in SAT-CR score was associated with a 0.28-standard deviation increase in predicted performance on the ACS exam, β = 0.28, p < 0.001. Likewise, a one-standard deviation increase in SAT-Math score was associated with a 0.33-standard deviation increase in predicted ACS exam performance, β = 0.33, p < 0.001. This model explained 28.1% of the variance in ACS exam score in Chem C (R2 = 0.281, F(2,523) = 102.16, p < 0.001). Comparable results were obtained when GMRT scores were used as the measure of comprehension ability (Table 6). Thus, comprehension ability was significantly predictive of course performance when math ability was statistically controlled; this result was independent of language comprehension measure. Math ability was also significantly predictive of course performance when comprehension ability was statistically controlled. Additionally, the squared semipartials for comprehension ability (sr2 = 0.045–0.059) suggest small effect sizes for this predictor when math ability is statistically controlled. Likewise, the squared semipartials for math ability (sr2 = 0.081–0.123) suggest small to medium effect sizes for this predictor when comprehension ability is statistically controlled. Identical results were obtained from analyses of Chem A and Chem B data (Appendix 5, ESI†).
Table 5 Standard multiple regression of SAT-Math scores and SAT-CR scores on Chem C course performance (as measured by the ACS General Chemistry (Conceptual) Exam). N = 526
Coefficient |
B |
Standard error |
β |
t |
sr2 |
p |
R2 = 0.281, F(2,523) = 102.16, p < 0.001. |
Intercept |
64.61 |
0.483 |
|
133.76 |
|
<0.001 |
SAT-CR score |
0.050 |
0.008 |
0.278 |
6.481 |
0.058 |
<0.001 |
SAT-Math score |
0.065 |
0.008 |
0.332 |
7.737 |
0.082 |
<0.001 |
Table 6 Standard multiple regression of SAT-Math scores and GMRT scores on Chem C course performance (as measured by the ACS General Chemistry (Conceptual) Exam). N = 484
Coefficient |
B |
Standard error |
β |
t |
sr2 |
p |
R2 = 0.271, F(2,481) = 89.475, p < 0.001. |
Intercept |
64.57 |
0.517 |
|
124.95 |
|
<0.001 |
GMRT score |
0.187 |
0.036 |
0.220 |
5.159 |
0.040 |
<0.001 |
SAT-Math score |
0.078 |
0.009 |
0.390 |
9.140 |
0.127 |
<0.001 |
The squared semipartials for comprehension ability obtained from these regression models also indicate that the original zero-order correlations between comprehension ability and course performance (r = +0.40 to +0.45, vide supra) were partly (but not entirely) accounted for by math ability. These regressions indicated that the semipartials for comprehension ability were ∼50% of the Pearson correlations reported above. One possible interpretation of this outcome is that comprehension ability and math ability are partly redundant as predictors of course performance; to the extent that comprehension ability and math ability are correlated with each other, they compete to explain the same variance in course performance. This interpretation is supported by the large amount of variance in ACS exam score that can be predicted equally well by comprehension ability or math ability in each course. Since the squared semipartials for math ability and comprehension ability represent the unique variance of that predictor shared with ACS exam score, the sum of the squared semipartials can be subtracted from the overall R2 for the regression model to determine the amount of variance common to both predictors. For example, Table 5 presents a regression model that accounted for 28.1% of the total variance in the outcome measure. SAT-Math scores uniquely predicted 8.2% of the total variance explained by the model, while SAT-CR scores uniquely predicted 5.8% of the total variance. The 14.1% of variance in ACS exam score remaining must be shared equally by comprehension ability and math ability. In all regressions, including that using GMRT scores in Chem C (Table 6) and those for Chem A and Chem B (Appendix 5, ESI†), the proportion of explained variance shared by comprehension ability and math ability was consistently larger than the variance uniquely predicted by either variable. This large amount of variance in ACS exam score common to both math ability and comprehension ability may have interesting implications for using these variables to predict general chemistry achievement.
Midterm exams. To corroborate the results obtained by analyzing ACS exam data, we assessed the effects of language comprehension ability and math ability on midterm exam performance. The HLMs employed were very similar in structure to those described above for research question 1, save that standardized SAT-Math scores were added as an additional fixed effect at level-2. Results for these models are presented in Tables 7 and 8; for the sake of parsimony, only statistically significant effects are reported. A full presentation of modeling these data can be found in Appendix 6 (ESI†).
Table 7 Results of a two-level model estimating the effect of SAT-CR and SAT-Math section scores on Chem C course performance (as measured by course midterm exams)
Fixed effect |
Estimate |
Standard error |
Approx. df |
t |
p |
SAT-CR score effect, β01 |
0.191 |
0.041 |
533 |
4.686 |
<0.001 |
SAT-Math score effect, β02 |
0.350 |
0.041 |
535 |
8.556 |
<0.001 |
Random effect |
Variance |
Standard error |
Wald Z |
p |
Level-1: exam scores (residual, eij) |
0.348 |
0.015 |
22.928 |
<0.001 |
Level-2: student (initial status, r0j) |
0.405 |
0.042 |
9.825 |
<0.001 |
Table 8 Results of a two-level model estimating the effect of GMRT and SAT-Math section scores on Chem C course performance (as measured by course midterm exams)
Fixed effect |
Estimate |
Standard error |
Approx. df |
t |
p |
GMRT score effect, β01 |
0.211 |
0.041 |
490 |
5.176 |
<0.001 |
SAT-Math score effect, β02 |
0.361 |
0.041 |
492 |
8.850 |
<0.001 |
GMRT score by time, β11 |
−0.029 |
0.014 |
487 |
−2.043 |
0.042 |
Random effect |
Variance |
Standard error |
Wald Z |
p |
Level-1: exam scores (residual, eij) |
0.344 |
0.016 |
21.972 |
<0.001 |
Level-2: student (initial status, r0j) |
0.407 |
0.043 |
9.499 |
<0.001 |
As was true in the case of ACS exam scores, both math ability and comprehension ability predicted significant increases in midterm exam score when the other was statistically controlled, regardless of language comprehension measure. For the model that predicted performance from SAT-CR scores (Table 7), a one-standard deviation increase in SAT-CR score was associated with a 0.191-standard deviation increase in exam score (β01 = 0.191, p < 0.001), while a one-standard deviation increase in SAT-Math score was associated with a 0.350-standard deviation in exam score. A similar result was obtained when GMRT scores were used as the measure of comprehension ability (Table 8). Consistent with ACS exam data analyses, the mean effect of comprehension ability on midterm exam performance was less (42–47%) than the mean effect of math ability, even though both effects were statistically significant. Comprehension ability effect estimates obtained using midterm exam data were also smaller than those obtained via analysis of ACS exam data. Interestingly, the negative relationship between language comprehension ability and growth rate observed in previous HLMs remained only when GMRT scores were used as the measure of comprehension ability (β11 = −0.029, p = 0.042, Table 8). This effect of GMRT score on growth rate, roughly an order magnitude less than the main effects of comprehension ability and math ability, is likely too minor to be considered meaningful, however.
When the coefficients for language comprehension ability (β01) in Tables 3 and 4 were compared to those in Tables 7 and 8, a decrease in the coefficient was observed when math ability was added to the HLMs, also consistent with ACS exam data analyses. For example, the estimated effect of GMRT scores on midterm exam performance was 0.370 (Table 4); when math ability was added to this HLM as an additional level-2 predictor (Table 8), the effect of GMRT scores was reduced to 0.211. Overall, the mean effect of language comprehension on Chem C course performance was decreased 43–49% when math ability was taken into account. As for the regressions using ACS exam data, we concluded from these HLMs of midterm data that the relationship between language comprehension ability and course performance was partly (but not entirely) accounted for by math ability.
To what extent can high comprehension ability compensate for low prior knowledge in general chemistry courses?
ACS exams. A question that naturally arises from the theories of language comprehension is whether this cognitive variable helps students of lower prior knowledge compensate for knowledge deficits; or, alternatively, whether chemistry performance is compromised regardless of comprehension ability for students lacking sufficient prior knowledge. To help address this question, we investigated the possibility of statistical interactions arising between prior knowledge and language comprehension. Testing for such interactions involves including a prior knowledge by comprehension ability product term in regressions of prior knowledge and comprehension ability on course performance. If this interaction term was found to be a statistically significant predictor of course performance, we could conclude that prior knowledge moderates the effect of comprehension ability on course performance (and vice versa). By moderate, we mean that the effect of comprehension ability on course performance would differ across scores on the prior knowledge measure. For example, if a statistically significant prior knowledge by comprehension ability interaction were found to possess a negative slope, we would conclude that the effect of comprehension ability on course performance was greater for students of lower-than-average prior knowledge than for students of higher-than-average prior knowledge. Thus, it is very likely that the performance gap between students of low and high prior knowledge would be abated at some moderate level of comprehension ability. Thus, to address research question 3, we regressed ACS exam scores on language comprehension and prior knowledge measures. In these regressions, we included a prior knowledge by comprehension ability term to test for any moderation in these variables.Sequential regression was employed to determine if the addition of interaction terms improved predictions of ACS exam score beyond those afforded by differences in comprehension ability and prior knowledge. As presented in Appendix 2 (ESI†), comprehension ability and prior knowledge both exhibited positive linear relationships with ACS exam score. However, correlations between comprehension ability and prior knowledge (r = +0.36 to +0.53) did not indicate extremely high multicollinearity. As before, predictor variables were centered by subtracting the sample mean prior to performing regressions; comprehension ability by prior knowledge interaction terms were constructed by calculating the product of these mean-centered variables. Tables 9 and 10 display the final results of these analyses. For the case of comprehension ability measured by SAT-CR scores, after step-1, with SAT-CR and Toledo exam scores entered in the regression, R2 = 0.278, Finc(2,493) = 95.01, p < 0.001. After step-2, with the SAT-CR by Toledo exam score interaction added to the prediction of ACS exam score, R2 = 0.284, Finc(1,492) = 3.942, p = 0.048. Therefore, addition of the SAT-CR by Toledo exam interaction to the regression with SAT-CR and Toledo exam scores resulted in a significant increment in R2. This pattern of results suggested that SAT-CR and Toledo exam scores predicted over a quarter of the variability in ACS exam score; an interaction between these two variables contributed modestly to that prediction. While using SAT-CR scores as the measure of comprehension ability produced a statistically significant prior knowledge by comprehension ability interaction, using GMRT scores produced a different result. After step-1, with GMRT and Toledo exam scores entered in the regression, R2 = 0.248, Finc(2,522) = 86.276, p < 0.001. After step-2, with the GMRT by Toledo exam interaction added to the model, R2 = 0.252, Finc(1,521) = 2.445, p = 0.118. Therefore, addition of the GMRT by Toledo exam score interaction to the regression with GMRT and Toledo exam scores did not reliably improve R2. We concluded from this set of analyses that the main effect of comprehension ability remained statistically significant when prior knowledge was statistically controlled, and vice versa. Squared semi-partial correlations indicated small to medium effects for these parameters when the other was statistically controlled. By comparison, moderation of comprehension ability's main effect by prior knowledge was far less important. Regardless of statistical significance, the prior knowledge by comprehension ability interaction terms in each model explained less than one percent of variance in ACS exam score. Thus, we conclude that effect of comprehension ability on course performance is relatively the same across different levels of prior knowledge. Very similar results were found in Chem A and Chem B; these data and associated discussion are presented in Appendix 7 (ESI†).
Table 9 Sequential regression of SAT-CR and ACS Toledo exam scores on Chem C course performance (as measured by the ACS General Chemistry (Conceptual) Exam). N = 496
Coefficient |
B |
Standard error |
β |
t |
sr2 |
p |
R2 = 0.284, F(3,492) = 65.031, p < 0.001. |
Intercept |
64.53 |
0.527 |
|
121.69 |
|
<0.001 |
SAT-CR (step-1) |
0.061 |
0.007 |
0.328 |
7.997 |
0.093 |
<0.001 |
Toledo exam (step-1) |
0.320 |
0.043 |
0.299 |
7.273 |
0.077 |
<0.001 |
SAT-CR by Toledo (step-2) |
0.001 |
0.001 |
0.077 |
1.985 |
0.006 |
0.048 |
Table 10 Sequential regression of GMRT and ACS Toledo exam scores on Chem C course performance (as measured by the ACS General Chemistry (Conceptual) Exam). N = 525
Coefficient |
B |
Standard error |
β |
t |
sr2 |
p |
R2 = 0.252, F(3,521) = 58.492, p < 0.001. |
Intercept |
64.31 |
0.530 |
|
121.258 |
|
<0.001 |
GMRT (step-1) |
0.211 |
0.034 |
0.255 |
6.234 |
0.056 |
<0.001 |
Toledo exam (step-1) |
0.345 |
0.042 |
0.339 |
8.271 |
0.098 |
<0.001 |
GMRT by Toledo (step-2) |
0.004 |
0.003 |
0.059 |
1.564 |
0.003 |
0.118 |
Midterm exams. To validate the results obtained using ACS exam data, we assessed the effects of language comprehension ability, prior knowledge, as well as any potential moderation effects between these variables, on midterm exam performance. The two-level HLMs used to analyze midterm exam data were very similar to those described above for research question 2, except, rather than SAT-Math scores, standardized ACS Toledo exam scores and a prior knowledge by comprehension ability interaction term were included as fixed effects at level-2. Results for these models are presented in Tables 11 and 12; for the sake of parsimony, only statistically significant effects are reported. A full presentation of modeling these data and associated equations can be found in Appendix 8 (ESI†).
Table 11 Estimation of the effects of SAT-CR scores and Toledo exam scores on Chem C course performance (as measured by course midterm exams)
Fixed effect |
Estimate |
Standard error |
Approx. df |
t |
p |
SAT-CR score effect (β01) |
0.225 |
0.039 |
503 |
5.811 |
<0.001 |
Toledo exam score effect (β02) |
0.368 |
0.039 |
503 |
9.451 |
<0.001 |
Random effect |
Variance |
Standard error |
Wald Z |
p |
Level-1: exam scores (residual, eij) |
0.344 |
0.015 |
22.246 |
<0.001 |
Level-2: student (initial status, r0j) |
0.388 |
0.041 |
9.423 |
<0.001 |
Table 12 Estimation of the effects of GMRT scores and Toledo exam scores on Chem C course performance (as measured by course midterm exams)
Fixed effect |
Estimate |
Standard error |
Approx. df |
t |
p |
GMRT score effect (β01) |
0.237 |
0.038 |
534 |
6.272 |
<0.001 |
Toledo exam score effect (β02) |
0.344 |
0.037 |
534 |
9.214 |
<0.001 |
GMRT score by time (β10) |
−0.031 |
0.013 |
532 |
−2.369 |
0.018 |
Random effect |
Variance |
Standard error |
Wald Z |
p |
Level-1: exam scores (residual, eij) |
0.340 |
0.015 |
22.872 |
<0.001 |
Level-2: student (initial status, r0j) |
0.398 |
0.040 |
9.860 |
<0.001 |
As for the ACS exams, comprehension ability was significantly predictive of midterm exam performance when prior knowledge was statistically controlled. This result was independent of language comprehension measure. Prior knowledge was also significantly predictive of course performance when comprehension ability was statistically controlled. The mean effect of comprehension ability on course performance was consistently less than the mean affect of prior knowledge, even though both effects were statistically significant. For example, a one-standard deviation increase in SAT-CR score was associated with a 0.225-standard deviation increase in exam score (β01 = 0.225, p < 0.001), while a one-standard deviation increase in Toledo exam score was associated with a 0.368-standard deviation in exam score (β02 = 0.368, p < 0.001) (Table 11). Once again, we observed a small negative relationship between language comprehension ability and growth rate only when GMRT scores were used as the measure of comprehension ability (β11 = −0.031, p = 0.018, Table 12). Although there were consistently significant main effects for prior knowledge and comprehension ability on midterm exam performance, regardless of language comprehension ability measure, no statistically significant interactions between these variables were found. For the SAT-CR by Toledo exam score interaction, β03 = −0.003, p = 0.936, while for the GMRT by Toledo exam score interaction, β03 = −0.022, p = 0.521. Even if these interaction terms were found to be statistically significant, the corresponding effects were too small to be considered meaningful, as in both cases, these interaction terms accounted for less than one percent of the variance in midterm exam scores (Appendix 8, ESI†). These results corroborate those obtained with ACS exam scores—the main effect of comprehension ability on midterm exam scores is the same for both low- and high-prior knowledge students (i.e. there was no interaction that would cause the main effect to be moderated appreciably by level of prior knowledge).
The crux of research question 3 was whether comprehension ability would compensate for prior chemistry knowledge. If comprehension ability helps compensate for prior knowledge, then a differential effect of comprehension should be evident for lower knowledge students. This was clearly not the case, as our analyses indicated that students with high prior knowledge and high comprehension ability performed better than students with low prior knowledge and the same level of high comprehension ability. However, given the significant main effect of comprehension ability—students with low prior knowledge and high comprehension ability performed better than students with low prior knowledge and low comprehension ability. So, an alternate test of compensation would be to examine whether lower-knowledge students with high comprehension ability performed similarly to the higher-knowledge students with low comprehension ability (O'Reilly and McNamara, 2007). If the main effect of comprehension ability is large enough for students of high comprehension ability to overcome performance deficits due to low prior knowledge (i.e. if the low knowledge/high comprehension students perform as well as the high knowledge/low comprehension students), then we could conclude that comprehension ability can partially compensate for lower knowledge.
We were able to make course performance comparisons between low knowledge/high comprehension students and high knowledge/low comprehension students by constructing plots using the regression coefficients listed in Tables 9–12 (Fig. 1). These plots illustrate standardized course performance, as measured by either ACS exams (panel A) or course midterms (panel B), over a ±2-standard deviation range of language comprehension, comparing students of low and high prior knowledge. The performance gap between students of low and high levels of prior chemistry knowledge is clearly illustrated in this figure, as is the ability of comprehension ability to partially compensate for low prior knowledge. Regardless of course performance measure or comprehension ability measure, students who possessed low prior knowledge and high comprehension ability performed approximately the same as students who possessed high prior knowledge and low comprehension ability. This compensatory effect was unequivocal when ACS exams were used as the outcome (panel A), but somewhat more tenuous when course midterms were used, as the effect was realized in our research scenario only at the extreme of comprehension ability (approximately two standard deviations above the mean). Similar effects were observed in Chem A and Chem B (Appendix 7, ESI†). Thus, our analyses suggest that high comprehension ability can help students negotiate the achievement gap between those possessing low and high levels of prior chemistry knowledge.
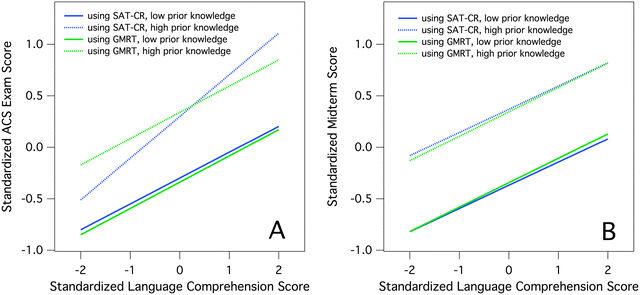 |
| Fig. 1 Plots of language comprehension ability, as measured by either SAT-CR scores (blue) or GMRT scores (green), versus course performance for Chem C students of low (solid) and high (dotted) prior knowledge. Panel A uses ACS exam scores as the measure of course performance, while panel B uses course midterm exams. Low prior knowledge students were modeled as achieving scores one standard deviation below the mean on the ACS Toledo exam, while high prior knowledge students were modeled as achieving one standard deviation above the mean on the ACS Toledo exam. | |
Conclusions and discussion
Structure Building is a cognitive model that describes language comprehension as the incorporation of new information into one's existing knowledge base. This process requires relevant prior knowledge to serve as a foundation, as well as the ability to construct a coherent mental representation of new information. Research has shown that those of high comprehension ability use prior knowledge to draw inferences and successfully comprehend new information. This study further investigated these notions in the context of university-level introductory chemistry by looking at measures of performance and their relation to prior knowledge and language comprehension. Comprehension ability was also compared to the well-studied general chemistry course predictor, math ability.
Comprehension ability correlates with general chemistry performance with medium effect sizes
Our first research question explored how strongly language comprehension ability correlated with performance in general chemistry courses. We have robustly confirmed what the chemistry education literature has only fleetingly reported: quantitative measures of language comprehension ability are significantly correlated with general chemistry performance. This result agrees with a handful of similar findings (Carmichael et al., 1986; Glover et al., 1991; Bunce and Hutchinson, 1993; Lewis and Lewis, 2007, 2008). Our study differs from this previous work in that we collected data using an alternative measure of comprehension ability (the Gates-MacGinitie Reading Test) in addition to SAT section scores. Analyses using Pearson correlations and hierarchical linear modeling demonstrated that language comprehension ability predicts course performance with statistical significance, and with medium effect sizes (r = +0.32 to +0.45). Thus, we have identified a generalizable predictive relationship between language comprehension ability and general chemistry course performance. This relationship is very likely generalizable to situations beyond our research institution, as ACS exams were used as the outcome measure in most of our analyses.
Comprehension ability and math ability are comparable predictors of general chemistry achievement
Our second research question sought to compare math ability and comprehension ability as predictors of general chemistry. Our results indicated that, regardless of language comprehension measure, performance measure, or general chemistry course, both math ability and comprehension ability predicted significant increases in course performance when the other was statistically controlled. However, the mean effect of comprehension ability on course performance was consistently less than the mean effect of math ability, even though both effects were statistically significant. Overall, these results agree with Lewis and Lewis (2007, 2008) whose regression and HLM analyses indicate that while both SAT-Math and SAT-Verbal are statistically significant predictors of scores on ACS General Chemistry Exams, the mean effect of SAT-Verbal scores is smaller than that SAT-Math scores. As this work by Lewis and Lewis was performed before the transition of the SAT-Verbal section to the SAT-Critical reading section in 2005, we are confident that the conclusions presented here are translatable to situations predating the creation of the SAT-Critical reading section.
Are commonly used measures of comprehension ability and math ability partially assessing the same construct?
An interesting consequence of investigating research question 2 was finding that math ability was partially redundant with comprehension ability as a predictor of course performance. Comparing the variance shared by comprehension ability and math ability and the total variance explained (R2) for math/comprehension regression models in each course led to the conclusion that approximately 50% of ACS exam score variance accounted for by our regression models was predicted equally well by comprehension ability or math ability. That language comprehension and math ability may be redundant predictors of course performance may seem foreign to the chemical educator. After all, the volume of previous work linking math ability to chemistry achievement is staggering in comparison to the small number of studies detailing correlations using comprehension ability as a predictor variable. However, our results are in agreement with a wealth of previous work that has documented the importance of language comprehension in math achievement (Aiken, 1971, 1972; Jerman and Rees, 1972; Larsen et al., 1978; Munro, 1979; DeCorte et al., 1985; Kintsch and Greeno, 1985; Cocking and Chipman, 1988; Mestre, 1988; Spanos et al., 1988; Rothman and Cohen, 1989; Lepik, 1990; Noonan, 1990; Garcia, 1991; Abedi et al., 1995, 1998; Orr, 1997; Abedi and Lord, 2001). For example, Abedi and Lord (2001) found that students performed better on math problems that were linguistically simple compared to those that were more complex—demonstrating that language comprehension is a key factor in being able to solve mathematical problems. Because items on instruments measuring math ability also require skill in language comprehension, it follows that these math instruments also, to an extent, measure comprehension ability. Thus, a possible interpretation of our findings is that a common measure of math ability in chemistry education research (the SAT-Math section) also measures, at least partially, comprehension ability. This interpretation may explain why measures of math ability have been popular predictors of success in chemistry—effects of math ability on chemistry course performance are artificially inflated due to the variable's strong redundancy with comprehension ability. For example, in Chem C, the Pearson r (+0.48, Appendix 2, ESI†) for math ability was ∼40% larger than the semipartial for math ability when comprehension ability (as measured by SAT-CR scores) was statistically controlled (sr = 0.29, Table 5). It is important to note that we do not dispute that possessing adequate mathematical skills is integral for the study of chemistry. Rather, we conjecture that many issues arising at the interface of math ability and chemistry performance may be precipitated by poor language comprehension skill. We encourage chemistry educators to critically discern whether learning issues are potentially linked to a true lack of mathematics knowledge or to a lack of skill in comprehending mathematics when situated in the language of chemistry.
Language comprehension partially compensates for deficits in prior chemistry knowledge
Research question 3 explored the extent to which high comprehension ability can compensate for low prior chemistry knowledge. Our results indicated that, regardless of prior knowledge measure, performance measure, or general chemistry course, a large achievement gap existed between low and high prior knowledge students. The idea that prior chemistry instruction typically leads to higher rates of success in university chemistry is not new (Chandran et al., 1987). However, chemistry educators can be encouraged by the fact that language comprehension appears to help students overcome deficits in prior knowledge.
To investigate whether high comprehension ability could eliminate the performance gap between low and high prior knowledge students, we tested for prior knowledge by comprehension ability interactions in regression and HLM analyses using prior knowledge and comprehension ability as predictors of achievement. We hypothesized that if comprehension ability truly helps compensate for low prior knowledge, the effect of comprehension ability on course performance would be greater for low prior knowledge students. However, we found that the main effect of comprehension ability on course performance was the same for both low and high prior knowledge students (i.e. there were no notable interactions that would cause the main effect of comprehension ability to be moderated appreciably by level of prior knowledge). It is of note that the statistical power in testing for interactions is known to be low in field research situations such as this (McClelland and Judd, 1993). In particular, the lack of data at the extremes of our population is unfavorable. Inspection of ACS exam scores, course midterm exam scores, SAT section scores, prior knowledge measures, and GMRT scores suggests that students participating in our study did not span the entire range of possible scores on these measures. Because moderator effects are so difficult to detect, Evans (1985) suggested that even those explaining as little as 1% of the total variance should be considered. Even with this suggestion by Evans, the prior knowledge by comprehension ability interactions investigated in this study were not particularly important, as regression analyses revealed sr2 < 0.01 in all cases.
When we applied the results of regression and HLM analyses to graphically compare the performance of low comprehension/high prior knowledge students to high comprehension/low prior knowledge students (Fig. 1 and Fig. A1, ESI†), however, we found that these two groups performed similarly on ACS exams and on Chem C course midterm exams. These findings clearly underscore the importance of both prior knowledge and language comprehension ability for success in general chemistry. While comprehension ability may not be able to completely compensate for low prior knowledge, those possessing strong comprehension ability are certainly at an advantage. Those general chemistry programs that require “placement” into introductory chemistry courses using prior knowledge assessments (such as the ACS Toledo Chemistry Placement Exam) are encouraged to also consider language comprehension ability, given its apparent compensatory effect. An ideal situation of course would be for general chemistry students to possess both a strong chemistry knowledge background as well high comprehension ability. Thus, efforts to prepare students for success in general chemistry should include both content and the development of language comprehension skill.
A final model of general chemistry course performance, as predicted by comprehension ability, math ability, and prior knowledge
Although not directly addressed by a specific research question, the results presented here comparing language comprehension ability to more commonly used performance predictors (math ability and prior knowledge) begs for the construction of a final model consisting of all three predictors. Thus, course performance (as measured by ACS exams) was regressed on comprehension ability, math ability, and prior knowledge scores in all three courses. In addition, HLMs of Course C midterm data were constructed using all three predictors. These models are presented and discussed in full in Appendices 9 and 10 (ESI†). In all cases (regardless of language comprehension measure, course, or performance measure), each parameter predicted a statistically significant increase in course performance. Thus, comprehension ability remained a statistically significant predictor of chemistry achievement even when both prior knowledge and math ability are controlled. Given this result, we find it truly surprising that so much previous research has chosen to overlook language comprehension ability and its unique variance contribution to general chemistry achievement prediction models.
What can the general chemistry instructor do to help students with low comprehension ability?
It is well recognized that reading strategy instruction improves comprehension and is one of the most effective means of helping students to overcome deficits in comprehension ability (e.g. Palinscar and Brown, 1984; Bereiter and Bird, 1985; King and Rosenshine, 1993; Ozgungor and Guthrie, 2004; McNamara, 2007). Indeed, the College Board recently acknowledged the importance of reading strategies and included a Reading Strategies strand within its English Language Arts College Board Standards for College Success. As Structure Building would predict, strategy instruction is particularly effective for students with low prior knowledge or low comprehension ability (McNamara, 2007; O'Reilly and McNamara, 2007). The main goal of most strategy instruction is for less-skilled students to learn strategies that mimic those exhibited by skilled students or that compensate for processes exhibited by skilled students (McNamara, 2009).
An example of a successful instructional technique, aligned with theories of comprehension and knowledge/skill acquisition, is Self-explanation Reading Training (SERT, McNamara, 2004). SERT was designed around self-explanation, the process of explaining in words the meaning of written text, which has been shown to improve deep-level comprehension of text (Chi et al., 1989, 1994). Unfortunately, students may need training in self-explanation as most readers self-explain poorly if prompted to do so (Chi et al., 1994). For example, a poor self-explainer may be inclined to simply restate or paraphrase a text, rather than construct a true explanation of the text's meaning. SERT combines the explicit reading technique of self-explaining with training to use active reading strategies (comprehension monitoring, paraphrasing, elaboration, using logic, prediction, and bridging inference). McNamara (2004) compared students who were prompted to self-explain to those who were provided with training to self-explain using reading strategies; those who received the additional training on reading strategies showed significantly better comprehension than those who were merely prompted to self-explain. While it is rather unreasonable to expect instructors of introductory chemistry courses to provide deliberate strategy instruction such as SERT, technology may provide assistance. An automated and interactive version of SERT, called iSTART (interactive Strategy Training for Active Reading and Thinking) has been developed (McNamara et al., 2004; Levinstein et al., 2007). A study on the effectiveness of iSTART with college students using a cell biology text produced promising results (O'Reilly et al., 2004).
Scaffolding comprehension strategies onto in-class and out-of-class learning activities may be also beneficial. Low-skilled comprehenders tend not to employ the range and frequency of strategy use of high-skilled comprehenders; thus, chemistry learning activities that promote successful reading strategies should, in theory, help to close the achievement gap between low- and high-skilled comprehenders. For example, building self-explanation into learning activities and assessments may help low-skilled comprehenders in learning concepts associated with those activities as well as increase the use of a successful comprehension strategy. The success of instructional strategies consistent with self-explanation has been reported in the chemistry education literature, notably the science writing heuristic (Greenbowe et al., 2007) and writing-to-teach (Vázquez et al., 2012); however, the ability of these techniques to differentially aid low-skilled comprehenders has not been investigated. More simple strategies (e.g. asking questions and answering questions) are also known to be hallmarks of high-skilled comprehenders (vide supra); building instructional interventions from these simple strategies may be an efficient way for a chemistry instructor to aid low-skilled comprehenders. Ozgungor and Guthrie (2004) have demonstrated the positive effects of elaborative interrogation questions, especially for students of low prior knowledge. Multiple-choice questions may also be a suitable alternative (Little et al., 2012; Pazicni, unpublished work). Intriguingly, instructional strategies like Peer-Led Guided Inquiry may be relatively ineffective at differentially aiding low-skilled comprehenders in general chemistry (Lewis and Lewis, 2008). In essence, while reading strategy instruction has received a great deal of attention in the literature, very little work has been done to design intervention strategies that specifically aid low-skilled comprehenders in real classrooms. Given the recently reported stagnation of national reading scores in the United States (Dillon, 2010), such work will be pertinent to fostering equity in general chemistry education.
Limitations of study
Although the study presented here possessed clear strengths, there are several limitations. First, this was a field study—we did not directly manipulate prior chemistry knowledge, comprehension ability, or math ability. Thus, we can only make claims concerning possible relationships between these variables; we cannot make any claims about causation. Second, the extended investigations of the three research questions using multiple performance outcomes was limited to Course C by the availability of well-regulated course data. It is possible that similar research designs in Chem A or Chem B would have revealed different results. This is especially true given the specialized nature of Chem C as a one-semester accelerated general chemistry experience. Third, the language comprehension measures used in this study may have not wholly reflected the comprehension skills needed for success in introductory college chemistry courses. Recent work has demonstrated that although university science students can comprehend narrative text, they are not proficient at comprehending expository text, such as that found in science textbooks (Caverly et al., 2000; Shanahan, 2004). Our interpretation of comprehension as Structure Building extends the university science student's inability to comprehend information-dense text to audial comprehension and comprehension of non-linguistic media (pictures/graphs). This leaves very few sources of information that a student with low expository comprehension ability can access in a university chemistry classroom setting. Standard measures of language comprehension ability, however, do not exclusively assess student competence with expository texts. The SAT Critical Reading section combines narrative, argumentative, and expository elements (Educational Testing Service, 2013a). The ETS also reports that the SAT-reading comprehension section contains passages from the natural sciences, humanities, social sciences, and literary fiction, but not the physical sciences. Rowe et al. (2006) report that the Gates-MacGinitite Reading Test for 7/9th graders contains more questions based on narrative passages than on expository passages. Though this study employed the Gates-MacGinitite Reading Test for 10/12th graders, we have little reason to believe the ratio of narrative to expository passages deviates substantially from this report. To our knowledge, no comprehension measure that utilizes solely expository text exists.
Acknowledgements
This work is supported in part by a grant from the Davis Educational Foundation. The Foundation was established by Stanton and Elisabeth Davis after Mr Davis's retirement as chairman of Shaw's Supermarkets, Inc.
References
- Abedi J. and Lord C., (2001), The language factor in mathematics tests, Appl. Meas. Educ., 14, 219–234.
- Abedi J., Lord C. and Hofstetter, C., (1998), Impact of selected background variables on students' NAEP math performance, Los Angeles: UCLA Center for the Study of Evaluation/National Center for Research on Evaluation, Standards, and Student Testing.
- Abedi J., Lord C. and Plummer J., (1995), Language background as a variable in NAEP mathematics performance. NAEP TRP Task 3d: Language background study, Los Angeles: UCLA Center for the Study of Evaluation/National Center for Research on Evaluation, Standards, and Student Testing.
- Adams B., Bell L. and Perfetti C., (1995), A trading relationship between reading skill and domain knowledge in children's text comprehension, Discourse Process., 20, 307–323.
- Aiken L. R., (1971), Verbal factors and mathematics learning: a review of research, J. Res. Math. Educ., 2, 304–313.
- Aiken L. R, (1972), Language factors in learning mathematics, Rev. Educ. Res., 42, 359–385.
- Albanese M., Brooks D. W., Day V. W., Koehler R. A., Lewis J. D., Marianelli R. S., Rack E. P. and Tomlinson-Keasey C., (1976), Piagetian criteria as predictors of success in first year courses, J. Chem. Educ., 53, 571–572.
- American Chemical Society, (2013), American Chemical Society Division of Chemical Education Examination Institute, Retrieved July, 2013, from http://chemexams.chem.iastate.edu/.
- Andrews M. H. and Andrews L., (1979), First year chemistry grades and SAT math scores, J. Chem. Educ., 56, 231–232.
- Beck I., McKeown M. and Gromoll E., (1989), Learning from social studies texts, Cognition Instruct., 6, 99–158.
- Bender D. S. and Milakofsky L., (1982), College chemistry and Piaget: the relationship of aptitude and achievement measures, J. Res. Sci. Teach., 19, 205–216.
- Bentley A. B. and Gellene G. I., (2005), A six year study of the effects of a remedial course in the chemistry curriculum, J. Chem. Educ., 82, 125–130.
- Bereiter C. and Bird M., (1985), Use of thinking aloud in identification and teaching of reading comprehension strategies, Cognition Instruct., 2, 131–156.
- Botch B., Day R., Vining W., Stewart B., Rath K., Peterfreud A. and Hart D., (2007), Effects on student achievement in general chemistry following participation in an online preparatory course, J. Chem. Educ., 84, 547–553.
- Bransford J. D., Brown A. L. and Cocking R. R., (2000), How people learn: brain, mind, experience, and school, Washington, DC: National Academy Press.
- Bulman L., (1985), Teaching language and study skills in secondary science, London: Heinemann.
- Bunce D. M. and Hutchinson K. D., (1993), The use of the GALT (Group Assessment of Logical Thinking) as a predictor of academic success in college chemistry, J. Chem. Educ., 70, 183–187.
- Carmichael J. W. J., Bauer J. S., Sevenair J. P., Hunter J. T. and Gambrell R. L., (1986), Predictors of first-year chemistry grades for Black Americans, J. Chem. Educ., 63, 333–336.
- Cassels J. R. T. and Johnstone A., (1980), Understanding of non-technical words in science, London: Chemical Society.
- Cassels J. R. T. and Johnstone A. H., (1985), Words that matter in science, London: Royal Society of Chemistry.
- Caverly D. C., Orlando V. P. and Mullen J. A. L. (2000), Textbook study reading, in Flippo R. F. and Caverly D. C. (ed.), Handbook of college reading and study strategy research, Mahwah, NJ: Lawrence Erlbaum Associates, pp. 105–147.
- Chandran S., Treagust D. F. and Tobin K. G., (1987), The role of cognitive factors in chemistry achievement, J. Res. Sci. Teach., 24, 145–160.
- Chi M. T. H., Bassok M., Lewis M. W., Reimann P. and Glaser R., (1989), Self-explanations: how students study and use examples in learning to solve problems, Cognitive Sci., 13, 145–182.
- Chi M. T. H., de Leeuw N., Chiu M. and LaVancher C., (1994), Eliciting self-explanations improves understanding, Cognitive Sci., 18, 439–477.
- Childs P. E. and O'Farrell J. O., (2003), Learning science through English: an investigation of the vocabulary skills of native and non-native English speakers in international schools, Chem. Educ. Res. Pract., 4, 233–247.
- Cocking R. R. and Chipman S., (1988), Conceptual issues related to mathematics achievement of language minority children, in Cocking R. R. and Mestre J. P. (ed.), Linguistic and cultural influences on learning mathematics, Hillsdale, NJ: Lawrence Erlbaum Associates, pp. 17–46.
- Cohen J., (1988), Statistical power analysis for the behavioral sciences, 2nd edn, Hillsdale, NJ: Lawrence Erlbaum Associates.
- Cohen J., Cohen P., West S. G. and Aiken L. S., (2003), Applied multiple regression/correlation analysis for the behavioral sciences, 3rd edn, Mahwah, NJ: Lawrence Erlbaum Associates.
- Coley N. R., (1973), Prediction of success in general chemistry in a community college, J. Chem. Educ., 50, 613–615.
- Craney C. L. and Armstrong R. W., (1985), Predictor of grades in general chemistry for allied health students, J. Chem. Educ., 62, 127–129.
- DeCorte E., Verschaffel L. and DeWin L., (1985), Influence of rewording verbal problems on children's problem representations and solutions, J. Educ. Psychol., 77, 460–470.
- Dillon S., (2010), Stagnant national reading scores lag behind math, New York Times. Retrieved June, 2013, from http://www.nytimes.com.
- Educational Testing Service, (2013a), Critical Reading Section, Retrieved January, 2013, from http://professionals.collegeboard.com/testing/sat-reasoning/about/sections/critical-reading/.
- Educational Testing Service, (2013b), Mathematics Section, Retrieved January, 2013, from http://professionals.collegeboard.com/testing/sat-reasoning/about/sections/math/.
- Evans M. G., (1985), A Monte Carlo study of the effects of correlated method variance in moderated multiple regression analysis, Organ. Behav. Hum. Dec., 36, 305–323.
- Ewing M., Huff K., Andrews M., and King K., (2005), Assessing the reliability of skills measured by the SAT®, New York: The College Board Office of Research and Analysis.
- Gabel D., (1999), Improving teaching and learning through chemistry education research: a look to the future, J. Chem. Educ., 76, 548–554.
- Garcia G. E., (1991), Factors influencing the English reading test performance of Spanish-speaking Hispanic children, Read. Res. Quart., 26, 371–391.
- Gernsbacher M. A., (1990), Language comprehension as structure building, Hillsdale, NJ: Lawrence Erlbaum Associates.
- Gernsbacher M. A. and Faust M. E., (1991), The mechanism of suppression: a component of general comprehension skill, J. Exp. Psychol. Learn., 17, 245–262.
- Glover D., Kolb D. and Taylor M., (1991), Another option for chemistry dropouts, J. Chem. Educ., 68, 762–763.
- Graesser A. C., McNamara D. S. and Van Lehn K., (2005), Scaffolding deep comprehension strategies through Point&Query, AutoTutor, and iSTART, Educ. Psychol., 40, 225–234.
- Greenbowe T. J., Rudd J. A. and Hand B. M., (2007), Using the science writing heuristic to improve students' understanding of general equilibrium, J. Chem. Educ., 84, 2007–2011.
- Hamori E. and Muldrey J. E., (1984), Use of the word eager instead of spontaneous for the description of exergonic reactions, J. Chem. Educ., 61, 710.
- Herron J. D., (1996), The chemistry classroom, Washington, DC: American Chemical Society.
- Hovey N. W. and Krohn A., (1958), Predicting failures in general chemistry, J. Chem. Educ., 35, 507–509.
- Hovey N. W. and Krohn A., (1963), An evaluation of the Toledo chemistry placement examination, J. Chem. Educ., 40, 370.
- Hunter N. W., (1976), A chemistry prep course that seems to work, J. Chem. Educ., 53, 301.
- Inhelder B. and Piaget J., (1958), The growth of logical thinking from childhood to adolescence, New York: Basic Books.
- Jasien P. G., (2010), You said “neutral”, but what do you mean? J. Chem. Educ., 87, 33–34.
- Jasien P. G., (2011), What do you mean that “strong” doesn't mean “powerful”? J. Chem. Educ., 88, 1247–1249.
- Jasien P. G. and Oberem G. E., (2008), Student contextual understanding of the terms sense and energy: a qualitative study, Chem. Educ., 13, 46–53.
- Jerman M. and Rees R., (1972), Predicting the relative difficulty of verbal arithmetic problems, Educ. Stud. Math., 13, 269–287.
- Jiang B., Xu X., Garcia A. and Lewis J., (2010), Comparing two tests of formal reasoning in a college chemistry context, J. Chem. Educ., 87, 1430–1437.
- Johnstone A. H. and Selepeng D., (2001), A language problem revisited, Chem. Educ. Res. Pract., 2, 19–29.
- King A. and Rosenshine B., (1993), Effects of guided cooperative-questioning on children's knowledge construction, J. Exp. Educ., 6, 127–148.
- Kintsch W. and Greeno J. G., (1985), Understanding and solving word arithmetic problems, Psychol. Rev., 92, 109–129.
- Kunhart W. E., Olsen L. R. and Gammons R. S., (1958), Predicting success of junior college students in introductory chemistry, J. Chem. Educ., 35, 391.
- Larsen S. C., Parker R. M. and Trenholme B., (1978), The effects of syntactic complexity upon arithmetic performance, Learn. Disability Q., 1, 80–85.
- Lawrenz F., Wood N. B., Kirchhoff A., Kim N. K. and Eisenkraft A., (2009), Variables affecting physics achievement, J. Res. Sci. Teach., 46, 961–976.
- Legg M. J., Legg J. C. and Greenbowe T. J., (2001), Analysis of success in general chemistry based on diagnostic testing using logistic regression, J. Chem. Educ., 78, 1117–1121.
- Lenski S. D. and Nierstheimer S. L., (2002), Strategy instruction from a sociocognitive perspective, Reading Psychology, 23, 127–143.
- Leopold D. G. and Edgar B., (2008), Degree of mathematics fluency and success in second-semester introductory chemistry, J. Chem. Educ., 85, 724–731.
- Lepik M., (1990), Algebraic word problems: role of linguistic and structural variables, Educ. Stud Math., 21, 83–90.
- Lewis S. E. and Lewis J. E., (2007), Predicting at-risk students in general chemistry: comparing formal thought to a general achievement measure, Chem. Educ. Res. Pract., 8, 32–51.
- Lewis S. E. and Lewis J. E., (2008), Seeking effectiveness and equity in a large college chemistry course: an HLM investigation of peer-led guided inquiry, J. Res. Sci. Teach., 45, 794–811.
- Levinstein I. B., Boonthum C., Pillarisetti S. P., Bell C. and McNamara D. S., (2007), iSTART 2: improvements for efficiency and effectiveness, Behav. Res. Methods, 39, 224–232.
- Little J. L., Bjork E. L., Bjork R. A. and Angello G., (2012), Multiple-choice tests exonerated, at least of some charges: fostering test-induced learning and avoiding test-induced forgetting, Psychol. Sci., 23, 1337–1344.
- MacGinitie W. H., MacGinitie R. K., Maria K., Dreyer L. G. and Hughes, K. E., (2000a), Gates MacGinitie Reading Test Fourth Edition Forms S and T – Paper-pencil, Chicago: Riverside.
- MacGinitie W. H., MacGinitie R. K., Maria K., Dreyer L. G. and Hughes K. E., (2000b), Gates MacGinitie Reading Tests Fourth Edition Levels 7/9 & 10/12 Forms S&T: Manual for Scoring and Interpretation, Chicago: Riverside.
- McClelland G. H. and Judd C. M., (1993), Statistical difficulties of detecting interactions and moderator effects, Psychological Bulletin, 114, 376–390.
- McFate C. and Olmsted J., (1999), Assessing student preparation through placement tests, J. Chem. Educ., 76, 562–565.
- McNamara D. S., (2004), SERT: self-explanation reading training, Discourse Process., 38, 1–30.
- McNamara D. S. (ed.), (2007), Reading comprehension strategies: theory, interventions, and technologies, Mahwah, NJ: Lawrence Erlbaum Associates.
- McNamara D. S., (2009), The importance of teaching reading strategies, Perspectives on Language and Literacy, 35, 34–40.
- McNamara D. S., (2010), Computational methods to extract meaning from text and advance theories of human cognition, Top. Cogn. Sci., 2, 1–15.
- McNamara D. S. and Magliano J., (2009), Toward a comprehensive model of comprehension, in Ross B. (ed.), The psychology of learning and motivation, Burlington: Academic Press, pp. 298–384.
- McNamara D. S., Levinstein I. B. and Boonthum C., (2004), iSTART: interactive strategy trainer for active reading and thinking, Behav. Res. Methods Instrum., Comput., 36, 222–233.
- Mestre J. P., (1988), The role of language comprehension in mathematics and problem solving, in Cocking R. R. and Mestre J. P. (ed.), Linguistic and cultural influences on learning mathematics, Hillsdale, NJ: Lawrence Erlbaum Associates, pp. 201–220.
- Middlecamp C. and Kean E., (1988), Problems and “That Other Stuff”: types of chemical content, J. Chem. Educ., 65, 53–56.
- Mills P. and Sweeney W., (2009), Using the first exam for student placement in beginning chemistry courses, J. Chem. Educ., 86, 738–743.
- Munro J., (1979), Language abilities and math performance, Read. Teach., 32, 900–915.
- Niedzielski R. J. and Walmsey F., (1982), What do incoming freshmen remember from high school chemistry? J. Chem. Educ., 59, 149–151.
- Noonan J., (1990), Readability problems presented by mathematics text, Early Child Dev.
Care, 54, 57–81.
- O'Reilly T. and McNamara D. S., (2007), The impact of science knowledge, reading skill, and reading strategy knowledge on more traditional “high stakes” measures of high school students' science achievement, Am. Educ. Res. J., 44, 161–196.
- O'Reilly T. P., Sinclair G. P. and McNamara D. S., (2004), Reading strategy training: automated versus live, in Forbus K., Gentner D. and Regier T. (ed.), Proceedings of the Twenty-Sixth Annual Conference of the Cognitive Science Society, Mahwah, NJ: Lawrence Erlbaum Associates, pp. 1059–1064.
- Orr E. W., (1997), Twice as less: Black English and the performance of Black students in mathematics and science, New York: Norton.
- Ozgungor S. and Guthrie J. T., (2004), Interactions among elaborative interrogation, knowledge, and interest in the process of constructing knowledge from text, J. Educ. Psychol., 96, 437–443.
- Ozsogomonyan A. and Loftus D., (1979), Predictors of general chemistry grades, J. Chem. Educ., 56, 173–175.
- Palinscar A. S. and Brown A. L., (1984), Reciprocal teaching of comprehension-fostering and comprehension monitoring activities, Cognition Instruct., 1, 117–175.
- Peugh J. L., (2010), A practical guide to multilevel modeling, J. School Psychol., 48, 85–112.
- Pickering M., (1975), Helping the high risk freshman chemist, J. Chem. Educ., 52, 512–514.
- Pienta N., (2003), A placement examination and mathematics tutorial for general chemistry, J. Chem. Educ., 80, 1244–1246.
- Raudenbush S. W. and Bryk A. S., (2002), Hierarchical linear models: applications and data analysis methods, 2nd edn, Thousand Oaks, CA: Sage.
- Rixse J. S. and Pickering M., (1985), Freshman chemistry as a predictor of future academic success, J. Chem. Educ., 62, 313–315.
- Rothman R. W. and Cohen J., (1989), The language of math needs to be taught, Acad. Ther., 25, 133–142.
- Rowe M., Ozuru Y. and McNamara D., (2006), An analysis of reading ability tests: what do questions actually measure? Proceedings of the 7th International conference on Learning Sciences, 627–633.
- Russell A. A., (1994), A rationally designed general chemistry diagnostic test, J. Chem. Educ., 71, 314–317.
- Saxe G. B., Gearhart M. and Seltzer M., (1999), Relations between classroom practices and student learning in the domain of fractions, Cognition Instruct., 17, 1–24.
- Schelar V. M., Cluff R. B. and Roth B., (1963), Placement in general chemistry, J. Chem. Educ., 40, 369–370.
- Schmid S., Youl D. J., George A. V. and Read J. R., (2012), Effectiveness of a short, intense bridging course for scaffolding students commencing university-level study in chemistry, Int. J. Sci. Educ., 34, 1211–1234.
- Scofield M. B., (1927), An experiment in predicting performance in general chemistry, J. Chem. Educ., 4, 1168–1175.
- Seery M. K., (2009), The role of prior knowledge and student aptitude in undergraduate performance in chemistry: a correlation-prediction study. Chem. Educ. Res. Pract., 10, 227–232.
- Shanahan C., (2004), Better textbooks, better readers and writers, in Saul E. W. (ed.), Crossing borders in literacy and science instruction, Newark, DE/Arlington, VA: National Science Teachers Association/International Reading Association, pp. 370–382.
- Shapiro A. M., (2004), How including prior knowledge as a subject variable may change outcomes of learning research, Am. Educ. Res. J., 41, 159–189.
- Sieveking N. A. and Larson G. R., (1969), Analysis of the American Chemical Society achievement test with a multivariate prediction of college chemistry achievement, J. Couns. Psychol., 16, 166–171.
- Smith O. M. and Trimble H. M., (1929), The prediction of the future performance of students from their past records, J. Chem. Educ., 6, 93–97.
- Snow C., (2002), Reading for understanding: Toward an R&D program in reading comprehension, Santa Monica, CA: RAND.
- Spanos G., Rhodes N. C., Dale T. C. and Crandall J., (1988), Linguistic features of mathematical problem solving: insights and applications, in Cocking R. R. and Mestre J. P. (ed.), Linguistic and cultural influences on learning mathematics, Hillsdale, NJ: Lawrence Erlbaum Associates, pp. 221–240.
- Spencer H. E., (1996), Mathematical SAT test scores and college chemistry grades, J. Chem. Educ., 73, 1150–1153.
- Tabachnick B. G. and Fidell L. S., (2013), Using Multivariate Statistics, 6th edn, Boston, MA: Pearson.
- Tai R. H. and Sadler P. M., (2001), Gender differences in introductory undergraduate physics performance: University physics versus college physics in the USA, Int. J. Sci. Educ., 23, 1017–1037.
- Tai R. H., Sadler P. H. and Loehr J. F., (2005), Factors influencing success in introductory college chemistry, J. Res. Sci. Teach., 42, 987–1012.
- Vázquez A. V., McLoughlin K., Sabbagh M., Runkle A. C., Simon J., Coppola B. P. and Pazicni S., (2012), Writing-to-teach: a new pedagogical approach to elicit explanative writing in undergraduate chemistry students, J. Chem. Educ., 89, 1025–1031.
- Ver Beek K. and Louters L., (1991), Chemical language skills: investigating the deficit, J. Chem. Educ., 68, 389–392.
- Wagner E. P., Sasser H. and DiBiase W. J., (2002), Predicting students at risk in general chemistry using pre-semester assessments and demographic information, J. Chem. Educ., 79, 749–755.
Footnotes |
† Electronic supplementary information (ESI) available: Discussions of data quality and screening/descriptive statistics; zero-order correlations of all dependent and independent variables used in the study; complete presentations and analyses of Chem A and Chem B data; and complete presentations of all HLMs used in the study are organized as Appendices 1-10. See DOI: 10.1039/c3rp00014a |
‡ In 2005, SAT-Verbal section was changed to the SAT-Critical reading section. |
|
This journal is © The Royal Society of Chemistry 2013 |