Health impact assessment of exposure to fine particulate matter based on satellite and meteorological information†
Received
8th July 2013
, Accepted 5th November 2013
First published on 5th November 2013
Abstract
Air pollution in China, especially in the Pearl River Delta (PRD) region, has drastically increased in recent years. We modelled annual mean ground-level PM2.5 concentrations based on worldwide satellite information and meteorological data from 40 cities outside the PRD. The model of PM2.5 concentration (R = 0.845) was best explained by aerosol optical thickness (43.8%). We validated the spatial–temporal dimensions of the model and estimated that the annual mean PM2.5 concentration in PRD ranged between 22 and 65 μg m−3. Then we used meta-analysis to obtain the pooled excess risks of mortality in China and assessed the health impacts. We found an inverse association between short-term excess risks of mortality and annual mean PM2.5 concentrations. Based on the above models and analyses, the associated excess deaths for all-cause and cardiopulmonary diseases were 3386 and 2639 respectively. The corresponding risk-standardized excess death rates were 2006 and 1069 per million people.
Environmental impact
Particulate air pollution has surged over densely populated cities in China in recent years and has recently received international attention due to the toxicity of the fine portion of particulate matter and the associated adverse health consequences to local residents as well as to tourists and investors. The environmental impact could be assessed based on the community health burden attributable to exposure to the ambient concentration in the Pearl River Delta which represents Chinese regions with rapid economic growth where energy production mainly depends on fossil fuels with air pollution generated from power plants, motor vehicles and industrial emissions. Worldwide satellite and meteorological information could be applied for exposure assessment and model validation.
|
Introduction
Long-term exposure to fine particulate matter PM2.5 (aerodynamic diameter ≤ 2.5 μm) has been linearly associated with all-cause, cardiovascular and lung cancer mortality with detectable effects down to 8 μg m−3,1 which is very close to the World Health Organization (WHO) Air Quality Guidelines (AQG) for PM2.5 of 10 μg m−3.2 Urban air pollution in Asian countries contributed to two thirds of the global burden of disease due to poor air quality3 and the highest levels were often observed in Chinese cities.4 China's rapid economic growth relying on vast consumption of fossil fuels5 for power generation, transportation and manufacturing industries has drastically increased the emissions in recent decades.6 The Pearl River Delta (PRD) in south mainland China is one of the most polluted regions where PM2.5 monitoring is still at an initial stage.7
Recent epidemiological studies have used correlations between satellite data of aerosol optical thickness (AOT) and PM2.5 to estimate the spatial variations of exposure to particulate pollution4,8–11 and the associated adverse health impacts.12 We aimed to estimate the PM2.5 concentration in the PRD region based on worldwide satellite and meteorological data, to pool the excess risks of mortality from the worldwide literature, and to assess the associated excess mortality in the PRD population.
Materials and methods
PM2.5 data
We collected PM2.5 annual mean concentration (μg m−3) for 40 cities in 25 countries (Fig. 1) corresponding to the years 2008 (n = 31) and 2009 (n = 9) from the World Health Organization (WHO) Urban Outdoor Air Pollution Database13 and from the Hong Kong SAR Government.14 The latter was the only city in the PRD with accessible data of PM2.5 recorded by a fixed-site monitoring network. We also obtained PM2.5 data from Beijing, Guangzhou, Shanghai and Tokyo by contacting the authors of relevant publications.15,16
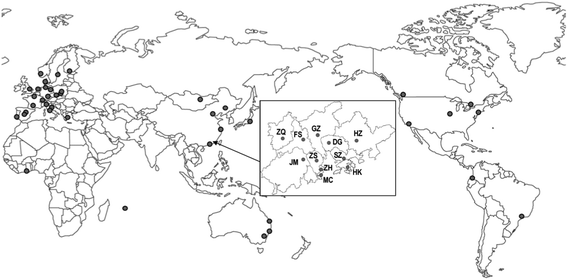 |
| Fig. 1 Forty cities for modeling and eleven cities in the Pearl River Delta region in China for health impact assessment. Note: Large dots represent 40 cities in the world for modeling. Small dots inside the box represent 11 cities in the Pearl River Delta region in China for health impact assessment. ZQ = Zhaoqing, FS = Foshan, GZ = Guangzhou, DG = Dongguan, HZ = Huizhou, JM = Jiangmen, ZS = Zhongshan, SZ = Shenzhen, ZH = Zhuhai, MC = Macau, and HK = Hong Kong. 4 cities in China (Beijing, Shanghai, Shenyang, and Hong Kong) were part of the 40 cities in the world for modeling. | |
Satellite and meteorological data
We retrieved the databases from National Aeronautics and Space Administration (NASA)'s Moderate-resolution Imaging Spectroradiometer (MODIS) for monthly averages of AOT,17,18 cloud optical thickness (COT), carbon monoxide (CO) level (ppbv), land surface temperature (LST) (°C), and vegetation index (VI) in the 40 world cities as well as 11 Chinese cities in the PRD region (Fig. 1). We assessed the satellite information in 2–4 grids of fixed coordinate size (0.11 × 0.11) in the central area of each city (covering 272 to 547 km2). The annual mean value for air temperature (°C), dew point (°C), relative humidity (%), visibility (km), wind speed (km h−1), and cloud cover (okta) data of the base year were retrieved from a publicly accessible database.19 Missing data of monthly AOT (26%) were replaced by the data of the same month in the closest year (±1 to 3 years) multiplied by the year-to-year percentage changes of the available monthly data. The remaining missing data of AOT (13%) as well as some missing data of COT and CO (1.5% and 7.3%, respectively) were replaced by a multiple imputation method.20 AOT and visibility data from all cities were right-skewed and ln-transformed.
Modeling
We used cluster analysis to select independent variables with low multicollinearity and then fitted multiple regression models to estimate PM2.5 annual mean concentrations for all combinations of these selected variables (Suppl 1†).21 We assessed models' temporal sensitivity by using years different from 2008 and 2009 in Hong Kong, Los Angeles, Rome, Shenyang and Sydney which were cities with non-missing data. We also assessed models' spatial sensitivity by using cities other than the 40 cities. We identified the best model as having the smallest average discrepancy in both temporal and spatial sensitivity for prediction of PM2.5 annual mean (Suppl 2†). We ranked influential predictors based on the percentage variance explained (partial eta square η2) by each variable in the model and then visually inspected the linear associations between PM2.5 and the influential predictors after adjusting for covariates (Suppl 2†).22
Systematic review
We searched the MEDLINE database using structured keyword terms, (“fine particulate”[tiab] or “fine particulates”[tiab] or “fine particle”[tiab] or “fine particles”[tiab] or “PM2.5”[tiab] or “PM(2.5)”[tiab]) and (“health”) and (mortality[tiab]), and retrieved 390 abstracts on 1st June 2012. We selected 35 literature articles using the following inclusion criteria (Fig. 2a): (i) all epidemiological studies on the adverse health effects of PM2.5 as the main research question; (ii) the health outcomes were related to deaths; (iii) the subjects were not high risk groups (e.g. patients or smokers) nor sensitive age subgroups (e.g. children or elders); (iv) exposures to ambient levels (not indoor, occupational or accidental exposures); (v) the health risk estimates were expressed in terms of unit change in pollutant mass concentration; and (vi) reported in English. We included non-duplicated publications on short-term effects only. We excluded the literature on long-term effects of mortality due to the insufficient number of studies that followed the above-mentioned criteria (n = 5). We included studies on the single-pollutant model with lags ranging within day 0 and 1 since these findings were mostly reported. Finally, we selected the latest study only in each of the population to avoid over-representation by any single population (Fig. 2b).
 |
| Fig. 2 (a) Literature selection. (b) Selected literature' records of PM2.5 concentrations. Note: Only the annual mean concentrations of the latest exposure year in each city are shown. Three-letter country codes: Australia (AUS), Canada (CAN), China (CHN), Czech Republic (CZE), Germany (GER), Japan (JPN), Korea (KOR), Mexico (MEX), Spain (ESP), Sweden (SWE), United Kingdom (GBR), and United States (USA). The dotted line represents the WHO annual AQG of 10 μg m−3 for PM2.5. | |
Meta-analysis
We used meta-analysis to pool risk estimates of PM2.5 on mortality for every 10 μg m−3 increase in pollutant concentration.23 We pooled excess risks (ER) in random effects if I2 statistics for heterogeneity was >25% or otherwise in a fixed effect. We used the influence plot of the conditional square root q2 statistics against square root w weight to diagnose the overall heterogeneity due to each study; and funnel plots with the Egger test on asymmetry at alpha level 0.1 to assess publication bias. We assumed the overall heterogeneity as statistically significant under normal distribution when the square root of q2 statistic lay outside −1.96 and 1.96.24,25
Meta-regression
Recent reviews of the health effects of PM2.5 in the American,26 European27 and Asian countries28 have consistently indicated evidence of increased deaths when exposure is elevated, but meta-analyses with meta-regression of these estimates across regions were rare. In this study, we pooled the short-term effect estimates of the excess risk of mortality from time-series studies on ambient PM2.5 in different global regions and conducted a meta-regression analysis of these estimates for improving our understanding of the differences in effect sizes across regions.
Health impact assessment
We obtained the annual total number of deaths (N) in 2008 from the Guangzhou Health Statistical Yearbook,29 Department of Health Statistical yearbook in Macau,30 and Hospital Authority clinical database in Hong Kong.31 The number of deaths in prefectures other than Guangzhou in PRD was not publicly available and we estimated by proportionality using the population ratios between these prefectures and Guangzhou (the capital city of Guangdong). We calculated the health impact by multiplying the pooled ER with N and the estimated annual mean concentrations of PM2.5. We assumed no threshold level for PM2.5 based on the hypothesis of both linear32–35 and nonlinear concentration response relationships.36,37 For comparison with the global health burden assessment study of excess deaths due to PM2.5,38 we calculated risk-standardized excess death rates, which was defined as the excess death rates per one million population multiplied by 10% (per 10 μg m−3 of PM2.5) as the fixed excess risk of death.
Results
Table 1 shows the selected best model (R = 0.85) that PM2.5 concentration was positively associated with ln-transformed aerosol optical thickness (β = 0.613 [95%CI: 0.367, 0.859]) but negatively associated with ln-transformed visibility (β = −0.987 [−1.587, −0.388]). These associations were consistent in other models. The largest variance of PM2.5 was explained by ln-transformed AOT (44%, p < 0.001) and multi-collinearity (variance inflation factors: 1.1 to 1.8) which were low for the rest of the covariates.
Table 1 Selected best model of annual mean PM2.5 concentration (μg m−3) for health impact assessmenta
Model |
Unit |
β
|
95% CI |
p-values |
VIF |
η
2 (%) |
Note: A = aerosol optical thickness, V = visibility, C = cloud optical thickness, W = wind speed, L = land surface temperature, and cc = cloud cover. VIF = variance inflation factor, in which VIF less than 2.5 is indicative of non-collinearity. η2 = partial eta square, which indicates the percentage variance explained by the variable in the model. Model selection (see M8 in Suppl 2, ESI).
|
Constant |
|
8.097 |
6.427, 9.767 |
<0.001 |
|
|
ln A |
0–1 |
0.613 |
0.367, 0.859 |
<0.001 |
1.5 |
43.8 |
In V |
km |
−0.987 |
−1.587, −0.388 |
0.002 |
1.3 |
25.4 |
C
|
0–50 |
−0.048 |
−0.095, −0.001 |
0.045 |
1.7 |
11.7 |
W
|
km h−1 |
−0.037 |
−0.075, 0.002 |
0.063 |
1.1 |
10.1 |
L
|
°C |
−0.020 |
−0.049, 0.009 |
0.165 |
1.8 |
5.7 |
cc |
okta |
−0.068 |
−0.233, 0.097 |
0.406 |
1.4 |
2.1 |
The pooled ER of mortality due to all-cause (ICD-10 A00-R99), cardiovascular (ICD-10 I00–I99) and respiratory (ICD-10 J00-J99) diseases for all the reviewed studies were 0.69% [0.45, 0.94], 0.60% [0.39, 0.80] and 0.83% [0.50, 1.17] respectively (Suppl 3 and 4†).63–93 The highest pooled ER by region in America (1.01% [0.79, 1.22]) followed by Europe 0.88% [0.34, 1.42] and Asia-Pacific 0.50% [0.19, 0.81] while the pooled ER for all of the six Chinese cities was 0.51% [0.14, 0.89] (Suppl 3†). Some publication bias was found (Egger test: p = 0.080) but it was not evident (Egger test: p = 0.460) after excluding influential studies defined by significant overall heterogeneity (Suppl 5†). Results of meta-regression analysis indicated that the ER of mortality in the literature were inversely related to the annual PM2.5 concentrations in a slightly decaying pattern (Fig. 3).
 |
| Fig. 3 Meta-regression analysis of annual mean PM2.5 concentration and the non-negative excess risk (%) of mortality for all natural causes (n = 22) and for cardiovascular (n = 19) and respiratory causes (n = 13) in different studies. | |
In PRD regions in 2008–2009, the model predicted annual mean PM2.5 concentrations were 45 μg m−3, which was 4.5 times of the WHOAQG of 10 μg m−3. The lowest predicted annual mean was in Zhuhai (22 μg m−3) and the highest one was in Foshan (65 μg m−3). In the region, the mean estimated PM2.5 annual concentration based on the ratio of PM2.5/PM10 was 50 μg m−3, with the lowest annual mean in Hong Kong (33 μg m−3) and the highest one in Foshan (81 μg m−3) (Table 2). The predicted values of the PM2.5 in PRD regions were relatively higher than the rest of the other cities in the world (Fig. 4).
Table 2 Health impact assessment – excess deaths due to PM2.5 in the PRD regionsa
|
HK |
MC |
DG |
FS |
GZ |
HZ |
JM |
SZ |
ZH |
ZQ |
ZS |
PRD |
Note: PM2.5 in Hong Kong was the real data from the Hong Kong Environmental Protection Department. HK = Hong Kong, MC = Macau, DG = Dongguan, FS = Foshan, GZ = Guangzhou, HZ = Huizhou, JM = Jiangmen, SZ = Shenzhen, ZH = Zhuhai, ZQ = Zhaoqing, ZS = Zhongshan, and PRD = Pearl River Delta. ER = excess risks per 10 μg m−3 derived from meta-regression models (see Fig. 3). ED = excess deaths. The risk-standardized ED rate was calculated using 10% as the excess risks per 10 μg m−3 of PM2.5. The ratio of PM2.5 to PM10 annual mean has been very stable at 0.7 over the years in Hong Kong, which is the only city in PRD with a fixed site monitoring of PM2.5.
|
Annual mean (μ gm
−3
)
|
|
|
|
|
|
|
|
|
|
|
|
Mean
|
Monitored PM2.5 |
33 |
|
|
|
|
|
|
|
|
|
|
45.2 |
Modeled PM2.5 |
|
28 |
58 |
65 |
61 |
43 |
32 |
47 |
22 |
63 |
47 |
Estimated PM2.5 (70% of monitored PM10, for validation purpose) |
33 |
39 |
56 |
81 |
50 |
55 |
48 |
38 |
39 |
62 |
49 |
50.0 |
|
|
|
|
|
|
|
|
|
|
|
|
Total
|
Population (million)
|
7.0 |
0.5 |
1.7 |
3.6 |
7.8 |
3.2 |
3.9 |
2.3 |
1.0 |
4.1 |
1.5 |
36.7 |
Death rate per 1000 |
6.00 |
3.20 |
4.54 |
5.49 |
5.55 |
4.90 |
7.49 |
0.98 |
2.79 |
5.25 |
6.01 |
|
|
No. of deaths (1000)
|
|
|
|
|
|
|
|
|
|
|
|
Total
|
All-cause |
39.8 |
1.8 |
7.9 |
20.0 |
43.5 |
15.6 |
28.9 |
2.3 |
2.8 |
21.5 |
8.8 |
192.9 |
Cardiovascular |
11.4 |
0.5 |
2.8 |
7.1 |
15.5 |
5.6 |
10.3 |
0.8 |
1.0 |
7.7 |
3.1 |
65.9 |
Respiratory |
8.2 |
0.2 |
1.4 |
3.6 |
7.9 |
2.8 |
5.3 |
0.4 |
0.5 |
3.9 |
1.6 |
36.0 |
|
ER derived from meta-regressions
|
|
|
|
|
|
|
|
|
|
|
|
Mean
|
All-cause |
0.56 |
0.60 |
0.43 |
0.41 |
0.42 |
0.50 |
0.57 |
0.48 |
0.64 |
0.42 |
0.48 |
0.49 |
Cardiovascular |
0.66 |
0.69 |
0.55 |
0.53 |
0.54 |
0.61 |
0.67 |
0.60 |
0.72 |
0.53 |
0.59 |
0.60 |
Respiratory |
1.02 |
1.06 |
0.83 |
0.79 |
0.81 |
0.93 |
1.02 |
0.90 |
1.12 |
0.80 |
0.90 |
0.91 |
|
ED
|
|
|
|
|
|
|
|
|
|
|
|
Total
|
All-cause |
505 |
19 |
165 |
447 |
937 |
259 |
362 |
40 |
22 |
472 |
157 |
3386 |
Cardiovascular |
170 |
6 |
75 |
206 |
429 |
113 |
152 |
18 |
9 |
217 |
70 |
1463 |
Respiratory |
187 |
4 |
57 |
157 |
326 |
87 |
119 |
14 |
7 |
165 |
53 |
1176 |
|
ED rate (per million)
|
|
|
|
|
|
|
|
|
|
|
|
|
All-cause |
72 |
34 |
94 |
123 |
120 |
81 |
94 |
17 |
22 |
115 |
108 |
92 |
Cardiovascular |
24 |
11 |
43 |
57 |
55 |
35 |
39 |
8 |
9 |
53 |
47 |
40 |
Respiratory |
27 |
8 |
33 |
43 |
42 |
27 |
31 |
6 |
7 |
40 |
37 |
32 |
|
Risk-standardized ED rate (per million)
|
|
|
|
|
|
|
|
|
|
|
|
|
All-cause |
1282 |
562 |
2175 |
3002 |
2835 |
1616 |
1658 |
359 |
339 |
2772 |
2238 |
2006 |
Cardiovascular |
366 |
155 |
777 |
1072 |
1012 |
577 |
592 |
128 |
121 |
990 |
799 |
698 |
Respiratory |
263 |
77 |
396 |
547 |
517 |
294 |
302 |
65 |
62 |
505 |
408 |
371 |
 |
| Fig. 4 Modeled and monitored annual mean PM2.5 concentrations (μg m−3) in all cities from 2008–2009. | |
Based on model predicted PM2.5 levels, the annual total number of deaths due to all natural causes ranged from 1756 in Macau to 43
521 in Guangzhou, with a total of 192
911 deaths per year in the whole region (Table 2). The annual cardiovascular deaths were the lowest in Macau (485) and the highest in Guangzhou (15
543); but the highest respiratory deaths were observed in Hong Kong (8160).
The annual excess deaths due to model-predicted PM2.5 for all causes were the highest in Guangzhou (937) and the lowest in Macau (19). The same trends followed in excess deaths due to cardiovascular and respiratory diseases. The total excess deaths due to all-cause, cardiovascular and respiratory diseases were 3386, 1463, and 1176 respectively in the whole PRD region in 2008–2009. The corresponding risk-standardized excess death rates per one million people were 2006, 698 and 371 respectively (Table 2).
Discussion
Many studies have examined the relationship between ground-level monitored PM2.5 concentration and satellite AOT on a local or countrywide scale10,11,39–50 as well as on a global scale.4,51 We based our study on the established approach of predicting ground-level PM2.5 annual mean concentration in the PRD region where public accessibility to the monitoring data was not yet fully available.
Our model utilized both the vertical column aerosol, as measured by AOT, and the horizontal ground-level aerosol, as measured by visibility, together with cloud optical thickness and other satellite and meteorological information as co-predictors of the ground-level annual mean of PM2.5 concentration. Visibility is a well-known indicator of PM2.5.52,53 It is measured horizontally at a height that is relevant to the ground level monitoring of PM2.5 and provides information to explain the variance of PM2.5 in addition to that measured vertically by AOT. Besides, visibility data are readily obtainable in many cities for worldwide comparisons as they are standard measurements in airports as well as in weather observatories. Cloud optical thickness is a satellite measure of light extinction due to the amount and thickness of a cloud, which is related to humidity and pressure in the atmosphere. This variable together with cloud cover in our model shared the variance of relative humidity as a common covariate.10 Although the satellite detected carbon monoxide was identified as a covariate of AOT, it was not included in our selected model. Further studies on applying the carbon monoxide variable should be warranted since it is publicly available and is related to fossil fuel burning and traffic emissions, and we found the best predictive performance in the temporal sensitivity assessment.
It is interesting that the annual mean concentrations in the Chinese cities were relatively higher than all other studies, but the magnitude of pooled ER of all natural mortalitities for these six Chinese cities was only a half of that in the American region. The APHENA investigators have also tested whether the concentration–response curve was actually nonlinear and whether statistical methods were appropriate though conclusive evidence was not explicit.54 A distributed lag nonlinear model was also proposed to detect the nonlinear concentration–response curve and delayed effects.55 In meta-regression analysis we found some evidence of an inverse linear relationship between ER of mortality and the annual PM2.5 concentration. This pattern appears to be leveling off in the downward direction rather than purely linear.36 A similar pattern of inverse association has been observed in a cross-sectional study of lung function and exposure to indoor PM2.5 concentration.37 This inverse relationship could be related to a saturation mechanism occurring at lower exposure levels56 where both irreversible and reversible processes may simultaneously exist. This has been hypothesized that, as indicated by structural changes in airways,57,58 particulates that penetrate to the deepest part of the lung and cause alveolar epithelial injury are associated with both acute reversible inflammatory responses and cumulative irreversible pulmonary damage.37 An explanation by the saturation hypothesis for cardiovascular diseases is also warranted.36 Our findings suggest that there is a need for further study to assess the effects of longer-term exposure on the short-term mortality risks. In addition, difference in climate, population structures, gene susceptibility, health status, occupational exposures, long-term exposure history and composition in PM2.5 may play a role in explaining the potential differences in short-term effects by region. There were some reviews on the epidemiological studies on the impact of fine particulate air pollution on mortality,26,59,60 while reviews on the cause for specific mortality are rare. The present review provided a meta-regression between the short-term effects and the annual mean concentrations and is the first to report an inverse relationship with a slightly decaying pattern.
For health impact assessment in the PRD region, we reviewed six Chinese studies and pooled the excess risks of mortalities based on physician-diagnosis, which were once-in-a-lifetime clinical records and so could provide more reliable health effect information than physiological and bio-microscopic measurements. Our health impact assessment results were well supported by the latest global estimation of health burden due to PM2.5 concentration38 since our estimation of the risk-standardized excess death rate of 1069 per million people for cardiorespiratory causes was very close to that in Asia of 1104 per million per 10% excess risk (i.e. 2584 thousand × 1 million/1.8 billion × 10 excess risk%/13 reported excess risk%) in that report. Non-risk standardized estimates of disease burden varies between places and the type of study design. For instance, the short-term excess death rates calculated in the current study were smaller by an order of magnitude than those estimated in long-term studies.34,59 However when we standardized the excess risk to 10%, comparison with other studies was more meaningful.
There were several limitations in this study. First, the number of cities involved in modeling was based on availability of satellite AOT data and ground-level fixed site monitored records of PM2.5 concentrations as compiled by WHO.5 Also some cities might have been overlooked, but the approximate normal distributions of our collected data have indicated that the cities selected in our sample are representative of respective regions so that selection bias might have been minimum and therefore not affecting overall representativeness of the sample. Second, our assumption of PM2.5 concentration as 70% of PM10 concentration in the PRD region might have ignored the heterogeneity of PM2.5/PM10 ratio across the region. However a similar ratio derived from a recent study in Guangzhou has indicated that it is in good agreement with the ratio we used.61 Third, the generalization of our best model for prediction in cities outside China has not been assessed despite that our spatial validation was based on ten cities in the PRD region only. Nevertheless, our temporal validation that was based on cities in different continents has demonstrated to have good performance for prediction among the forty cities that were included in the modeling. Fourth, the variation in risks due to differences in anthropogenic versus natural dust components of PM was not addressed.62 Our results should be interpreted with caution especially for international comparison.
Conclusions
The exposure to particulate pollutants in the Pearl River Delta regions in China is much higher than many other cities in the world. This study shows that the variation in PM2.5 annual concentrations may be inversely related to magnitude of short-term effects on mortality across geographic regions. The rapid growth of the economy in the region undoubtedly has contributed to the impact of air pollution on the population which is estimated to be responsible for 1069 excess deaths per million people. Nowadays, satellite and meteorological information is readily accessible so that public health impacts due to particulate air pollution in locations lacking monitoring can also be assessed.
Acknowledgements
This work was supported by the Wellcome Trust [#094330/Z10/Z].
Notes and references
- J. Lepeule, F. Laden, D. Dockery and J. Schwartz, Environ. Health Perspect., 2012, 120, 165–170 CrossRef PubMed.
-
World Health Organization, Air Quality Guidelines Global Update 2005: particulate matter, ozone, nitrogen dioxide and sulfur dioxide. WHO Regional Office for Europe, Copenhagen, 2006 Search PubMed.
- M. Krzyzanowski and A. Cohen, Air Qual., Atmos. Health, 2008, 1, 7–13 CrossRef.
- A. van Donkelaar, R. V. Martin, M. Brauer, R. Kahn, R. Levy, C. Verduzco and P. J. Villeneuve, Environ. Health Perspect., 2010, 118, 847–855 CrossRef CAS PubMed.
- J. N. Wang, Y. Lei, J. T. Yang and G. Yan, Environ. Sci. Technol., 2012, 46, 4263–4264 CrossRef CAS PubMed.
- B. H. Chen, H. D. Kan, R. J. Chen, S. H. Jiang and C. J. Hong, J. Air Waste Manage. Assoc., 2011, 61, 1292–1299 CAS.
- Y. Yuan, S. S. Liu, R. Castro and X. B. Pan, Environ. Sci. Technol., 2012, 46, 3627–3628 CrossRef CAS PubMed.
- J. Wang and S. A. Christopher, Geophys. Res. Lett., 2003, 30 Search PubMed.
- J. A. Engel-Cox, R. M. Hoff and A. D. J. Haymet, J. Air Waste Manage. Assoc., 2004, 54, 1360–1371 Search PubMed.
- Y. Liu, J. A. Sarnat, A. Kilaru, D. J. Jacob and P. Koutrakis, Environ. Sci. Technol., 2005, 39, 3269–3278 CrossRef CAS.
- Y. Liu, C. J. Paciorek and P. Koutrakis, Environ Health Persp, 2009, 117, 886–892 CrossRef PubMed.
- D. L. Crouse, P. A. Peters, A. van Donkelaar, M. S. Goldberg, P. J. Villeneuve, O. Brion, S. Khan, D. O. Atari, M. Jerrett, C. A. Pope, M. Brauer, J. R. Brook, R. V. Martin, D. Stieb and R. T. Burnett, Environ. Health Perspect., 2012, 120, 708–714 CrossRef PubMed.
- World Health Organization, Urban Outdoor Air Pollution Database, WHO, Geneva, 2011. http://www.who.int/phe/health_topics/outdoorair/databases/en/index.html Last accessed 13 June 2012.
- Hong Kong Environmental Protection Department. Past air monitoring station data, 2008–2009. http://epic.epd.gov.hk/ca/uid/airdata/p/1 Last accessed 21 May 2012.
- R. Chen, Y. Li, Y. Ma, G. Pan, G. Zeng, X. Xu, B. Chen and H. Kan, Sci. Total Environ., 2011, 409, 4934–4938 CrossRef CAS PubMed.
- T. Yorifuji, I. Kawachi, M. Kaneda, S. Takao, S. Kashima and H. Doi, Sci. Total Environ., 2011, 409, 3620–3627 CrossRef CAS PubMed.
- H. K. Lai, S. Y. Ho, C. M. Wong, K. K. Mak, W. S. Lo and T. H. Lam, Int. J. Environ. Health Res., 2010, 20, 219–230 CrossRef PubMed.
- Y. Liu, M. Franklin, R. Kahn and P. Koutrakis, Remote Sensing of Environment, 2007, 107, 33–44 CrossRef PubMed.
- Weather underground. Weather History for cities in 2008 and 2009. http://www.wunderground.com, 2013 Weather Underground, Inc, Last accessed 1 May 2012.
- J. L. Schafer and J. W. Graham, Psychological Methods, 2002, 7, 147–177 CrossRef.
- H. K. Lai, L. Bayer-Oglesby, R. Colvile, T. Gotschi, M. J. Jantunen, N. Künzli, E. Kulinskaya, C. Schweizer and M. J. Nieuwenhuijsen, Atmos. Environ., 2006, 40, 1299–1313 CrossRef CAS PubMed.
- W. A. Larsen and S. J. McCleary, Technometrics, 1972, 14, 781–790 CrossRef.
- H. K. Lai, H. Tsang and C. M. Wong, BMC Public Health, 2013, 13, 360 CrossRef PubMed.
-
M. Woodward. Meta-analysis (Ch.12). Epidemiology: study design and data analysis, 2nd edn, Fla: Chapman & Hall/CRC, Boca Raton, 2005 Search PubMed.
- M. Egger, S. G. Davey and M. C. Schneider, BMJ, 1997, 315, 629–634 CrossRef CAS.
- J. I. Levy, D. Diez, Y. Dou, C. D. Barr and F. Dominici, Am. J. Epidemiol., 2012, 175, 1091–1099 CrossRef PubMed.
-
H. R. Anderson, R. W. Atkinson, J. L. Peacock, L. Marston and K. Konstantinou. Meta-analysis of time-series studies and panel studies of particulate matter (PM) and ozone (O3): report of a WHO task group, World Health Organization, Copenhagen, 2004 Search PubMed.
- H. J. Jahn, A. Schneider, S. Breitner, R. Eissner, M. Wendisch and A. Kramer,
et al.
, Int. J. Hyg. Environ. Health, 2011, 214, 281–295 CrossRef CAS PubMed.
- Bureau of Health of Guangzhou Municipality, 2010.
- Macao Department of Health, Boletim Estatistico, 2008. http://www.ssm.gov.mo/design/statistic/e_statistic_fs.htm, Last accessed 2 February 2012.
- Hong Kong Hospital Authority. Hospital Authority Statistical Report, 2009, http://www.ha.org.hk/upload/publication_15/281.pdf, Last accessed 2 February 2012.
- M. J. Daniels, F. Dominici, J. M. Samet and S. L. Zeger, American journal of epidemiology, 2000, 152, 397–406 CrossRef CAS PubMed.
- E. Samoli, A. Analitis, G. Touloumi, J. Schwartz, H. R. Anderson, J. Sunyer, L. Bisanti, D. Zmirou, J. M. Vonk, J. Pekkanen, P. Goodman, A. Paldy, C. Schindler and K. Katsouyanni, Environ. Health Perspect., 2005, 113, 88–95 CrossRef CAS.
- C. A. Pope 3rd and D. W. Dockery, J. Air Waste Manage. Assoc., 2006, 56, 709–742 Search PubMed.
- C. M. Wong, N. Vichit-Vadakan, H. Kan and Z. Qian, Environ. Health Perspect., 2008, 116, 1195–1202 CrossRef CAS PubMed.
- K. R. Smith and J. L. Peel, Environ Health Persp, 2010, 118, 1643–1645 CrossRef CAS PubMed.
- H. K. Lai, A. J. Hedley, J. Repace, C. So, Q. Y. Lu, S. M. McGhee, R. Fielding and C. M. Wong, Thorax, 2011, 66, 615–623 CrossRef PubMed.
- S. C. Anenberg, L. W. Horowitz, D. Q. Tong and J. J. West, Environ Health Persp, 2010, 118, 1189–1195 CrossRef CAS PubMed.
- A. van Donkelaar, R. V. Martin and R. J. Park, J. Geophys. Res.: Atmos., 2006, 111 Search PubMed.
- N. Kumar, A. Chu and A. Foster, Atmos. Environ., 2007, 41, 4492–4503 CrossRef CAS PubMed.
- Z. F. Wang, L. F. Chen, J. H. Tao, Y. Zhang and L. Su, Remote Sensing of Environment, 2010, 114, 50–63 CrossRef PubMed.
- P. Glantz, A. Kokhanovsky, W. von Hoyningen-Huene and C. Johansson, Atmos. Environ., 2009, 43, 5838–5846 CrossRef CAS PubMed.
- C. J. Paciorek and Y. Liu, Environ. Health Perspect., 2009, 117, 904–909 CrossRef PubMed.
- Y. Liu, P. Koutrakis and R. Kahn, J. Air Waste Manage. Assoc., 2007, 57, 1351–1359 Search PubMed.
- Y. Liu, P. Koutrakis, R. Kahn, S. Turquety and R. M. Yantosca, J. Air Waste Manage. Assoc., 2007, 57, 1360–1369 CAS.
- C. J. Paciorek, Y. Liu, H. Moreno-Macias and S. Kondragunta, Environ. Sci. Technol., 2008, 42, 5800–5806 CrossRef CAS.
- J. Tian and D. M. Chen, Remote Sensing of Environment, 2010, 114, 221–229 CrossRef PubMed.
- M. Green, S. Kondragunta, P. Ciren and C. Xu, J. Air Waste Manage. Assoc., 2009, 59, 1082–1091 CAS.
- C. Li, N. C. Hsu and S. C. Tsay, Atmos. Environ., 2011, 45, 3663–3675 CrossRef CAS PubMed.
- W. Di Nicolantonio and A. Cacciari, Ital. J. Remote Sens., 2011, 43, 97–109 Search PubMed.
- P. Gupta, S. A. Christopher, J. Wang, R. Gehrig, Y. Lee and N. Kumar, Atmos. Environ., 2006, 40, 5880–5892 CrossRef CAS PubMed.
- J. L. Wang, Y. H. Zhang, M. Shao, X. L. Liu, L. M. Zeng, C. L. Cheng and X. F. Xu, J. Environ. Sci., 2006, 18, 475–481 CAS.
- D. E. Abbey, B. E. Ostro, G. Fraser, T. Vancuren and R. J. Burchette, J. Exposure Sci. Environ. Epidemiol., 1995, 5, 161–180 CAS.
- K. Katsouyanni, J. M. Samet, H. R. Anderson, R. Atkinson, A. Le Tertre and S. Medina,
et al.
, Res Rep Health Eff Inst, 2009, 5–90 CAS.
- A. Gasparrini, B. Armstrong and M. G. Kenward, Stat. Med., 2010, 29, 2224–2234 CrossRef CAS PubMed.
- G. Liistro, C. Vanwelde, W. Vincken, J. Vandevoorde, G. Verleden and J. Buffels, Chest, 2006, 130, 657–665 CrossRef PubMed.
- D. W. Dockery, F. E. Speizer, B. G. Ferris, J. H. Ware, T. A. Louis and A. Spiro, Am. Rev. Respir. Dis., 1988, 137, 286–292 CrossRef CAS PubMed.
- A. D. Flouris, G. S. Metsios, A. E. Carrillo, A. Z. Jamurtas, K. Gourgoulianis, T. Kiropoulos, M. N. Tzatzarakis, A. M. Tsatsakis and Y. Koutedakis, Am. J. Respir. Crit. Care Med., 2009, 179, 1029–1033 CrossRef PubMed.
- N. Künzli, S. Medina, R. Kaiser, P. Quenel, F. Horak Jr and M. Studnicka, Am. J. Epidemiol., 2001, 153, 1050–1055 CrossRef PubMed.
- M. L. Bell, J. M. Samet and F. Dominici, Annu. Rev. Public Health, 2004, 25, 247–328 CrossRef PubMed.
- C. Yang, X. Peng, W. Huang, R. Chen, Z. Xu, B. Chen and H. Kan, Int Arch Occup Environ Health, 2012, 85, 579–585 CrossRef CAS PubMed.
- J. Evans, A. van Donkelaar, R. V. Martin, R. Burnett, D. G. Rainham, N. J. Birkett and D. Krewski, Environ. Res., 2013, 120, 33–42 CrossRef CAS PubMed.
- R. T. Burnett, J. Brook, T. Dann, C. Delocla, O. Philips and S. Cakmak, Inhal Toxicol, 2000, 12, 15–39 CrossRef CAS.
- V. H. Borja-Aburto, M. Castillejos, D. R. Gold, S. Bierzwinski and D. Loomis, Environ. Health Perspect., 1998, 106, 849–855 CrossRef CAS.
- A. Zanobetti and J. Schwartz, Environ. Health Perspect., 2009, 117, 898–903 CrossRef PubMed.
- B. D. Ostro, R. Broadwin and M. J. Lipsett, J. Exposure Anal. Environ. Epidemiol., 2000, 10, 412–419 CAS.
- J. Schwartz, D. W. Dockery and L. M. Neas, J. Air Waste Manage. Assoc., 1996, 46, 927–939 CAS.
- A. Peters, J. Skorkovsky, F. Kotesovec, J. Brynda, C. Spix and H. E. Wichmann,
et al.
, Environ. Health Perspect., 2000, 108, 283–287 CrossRef CAS.
- M. Branis, J. Vyskovska, M. Maly and J. Hovorka, Inhalation Toxicol., 2010, 22(2), 21–28 CrossRef CAS PubMed.
- A. Peters, S. Breitner, J. Cyrys, M. Stolzel, M. Pitz and G. Wolke,
et al.
, Res Rep Health Eff Inst, 2009, 5–77, 79–90 Search PubMed , discussion.
- B. Ostro, A. Tobias, X. Querol, A. Alastuey, F. Amato and J. Pey,
et al.
, Environ. Health Perspect., 2011, 119, 1781–1787 CrossRef CAS PubMed.
- A. Tobias, L. Perez, J. Diaz, C. Linares, J. Pey and A. Alastruey,
et al.
, Sci. Total Environ., 2011, 412-413, 386–389 CrossRef CAS PubMed.
- K. Meister, C. Johansson and B. Forsberg, Environ. Health Perspect., 2012, 120, 431–436 CrossRef CAS PubMed.
- R. W. Atkinson, G. W. Fuller, H. R. Anderson, R. M. Harrison and B. Armstrong, Epidemiology, 2010, 21, 501–511 CrossRef PubMed.
- H. R. Anderson, S. A. Bremner, R. W. Atkinson, R. M. Harrison and S. Walters, ccup. Environ. Med., 2001, 58, 504–510 CrossRef CAS.
- R. Simpson, G. Williams, A. Petroeschevsky, T. Best, G. Morgan and L. Denison,
et al.
, Aust N Z J Public Health, 2005, 29, 205–212 CrossRef.
- R. Chen, Y. Li, Y. Ma, G. Pan, G. Zeng, X. Xu, B. Chen and H. Kan, Sci. Total Environ., 2011, 409, 4934–4938 CrossRef CAS PubMed.
- S. A. Venners, B. Wang, Z. Xu, Y. Schlatter, L. Wang and X. Xu, Environ. Health Perspect., 2003, 111, 562–567 CrossRef CAS.
- B. H. Chen, H. D. Kan, R. J. Chen, S. H. Jiang and C. J. Hong, J. Air Waste Manage. Assoc., 2011, 61, 1292–1299 CAS.
- Y. Ma, R. Chen, G. Pan, X. Xu, W. Song and B. Chen,
et al.
, Sci. Total Environ., 2011, 409, 2473–2477 CrossRef CAS PubMed.
- J. Cao, H. Xu, Q. Xu, B. Chen and H. Kan, Environ. Health Perspect., 2012, 120, 373–378 CrossRef CAS PubMed.
- J. Y. Son, J. T. Lee, K. H. Kim, K. Jung and M. L. Bell, Environ. Health Perspect., 2012, 120, 872–878 CrossRef PubMed.
- R. Simpson, L. Denison, A. Petroeschevsky, L. Thalib and G. Williams, J. Exposure Anal. Environ. Epidemiol., 2000, 10, 488–496 CAS.
- G. E. Morgan, S. Corbett, J. Wlodarczyk and P. Lewis, Am. J. Public Health, 1998, 88, 759–764 CrossRef CAS.
- M. S. Goldberg, R. T. Burnett, J. C. Bailar, J. Brook, Y. Bonvalot and R. Tamblyn,
et al.
, Environ. Res., 2001, 86, 26–36 CrossRef CAS PubMed.
- B. Ostro, W. Y. Feng, R. Broadwin, S. Green and M. Lipsett, Environ. Health Perspect., 2007, 115, 13–19 CrossRef CAS.
- W. E. Wilson, T. F. Mar and J. Q. Koenig, J. Exposure Anal. Environ. Epidemiol., 2007, 17(2), S11–S19 Search PubMed.
- J. C. Slaughter, E. Kim, L. Sheppard, J. H. Sullivan, T. V. Larson and C. Claiborn, J. Exposure Anal. Environ. Epidemiol., 2005, 15, 153–159 CrossRef CAS PubMed.
- M. Lippmann, K. Ito, A. Nadas and R. T. Burnett, Res Rep Health Eff Inst, 2000, 5–72, 73–82 Search PubMed , discussion.
- M. S. Goldberg, J. C. Bailar, R. T. Burnett, J. R. Brook, R. Tamblyn and Y. Bonvalot,
et al.
, Res Rep Health Eff Inst, 2000, 7–113, 115–120 Search PubMed , discussion.
- B. Ostro, R. Broadwin, S. Green, W. Y. Feng and M. Lipsett, Environ. Health Perspect., 2006, 114, 29–33 CrossRef.
- K. Ueda, H. Nitta and M. Ono, Circulation, 2009, 73, 1248–1254 CrossRef CAS PubMed.
- M. Stolzel, S. Breitner, J. Cyrys, M. Pitz, G. Wolke and W. Kreyling,
et al.
, J. Exposure Anal. Environ. Epidemiol., 2007, 17, 458–467 CrossRef PubMed.
Footnote |
† Electronic supplementary information (ESI) available: Supplementary I: cluster analysis for selection of independent variables in multiple regressions. Supplementary II: regression models of PM2.5 and sensitivity of the model predictions; visual inspection of the major independent variables: ln-transformed data of PM2.5versus AOT and visibility in 40 cities. Supplementary III: literature review – detection of heterogeneity and publication bias. Supplementary IV: summary of excess risks of different causes of mortality in all age groups due to PM2.5. See DOI: 10.1039/c3em00357d |
|
This journal is © The Royal Society of Chemistry 2014 |
Click here to see how this site uses Cookies. View our privacy policy here.