DOI:
10.1039/C1MB05231D
(Paper)
Mol. BioSyst., 2012,
8, 268-281
Attributes of short linear motifs†‡
Received
9th June 2011
, Accepted 8th August 2011
First published on 12th September 2011
Abstract
Traditionally, protein–protein interactions were thought to be mediated by large, structured domains. However, it has become clear that the interactome comprises a wide range of binding interfaces with varying degrees of flexibility, ranging from rigid globular domains to disordered regions that natively lack structure. Enrichment for disorder in highly connected hub proteins and its correlation with organism complexity hint at the functional importance of disordered regions. Nevertheless, they have not yet been extensively characterised. Shifting the attention from globular domains to disordered regions of the proteome might bring us closer to elucidating the dense and complex connectivity of the interactome. An important class of disordered interfaces are the compact mono-partite, short linear motifs (SLiMs, or eukaryotic linear motifs (ELMs)). They are evolutionarily plastic and interact with relatively low affinity due to the limited number of residues that make direct contact with the binding partner. These features confer to SLiMs the ability to evolve convergently and mediate transient interactions, which is imperative to network evolution and to maintain robust cell signalling, respectively. The ability to discriminate biologically relevant SLiMs by means of different attributes will improve our understanding of the complexity of the interactome and aid development of bioinformatics tools for motif discovery. In this paper, the curated instances currently available in the Eukaryotic Linear Motif (ELM) database are analysed to provide a clear overview of the defining attributes of SLiMs. These analyses suggest that functional SLiMs have higher levels of conservation than their surrounding residues, frequently evolve convergently, preferentially occur in disordered regions and often form a secondary structure when bound to their interaction partner. These results advocate searching for small groupings of residues in disordered regions with higher relative conservation and a propensity to form the secondary structure. Finally, the most interesting conclusions are examined in regard to their functional consequences.
Introduction
Folded, globular domains were once seen as the sole mediators of protein–protein interactions, however, accumulating evidence has revealed a continuous spectrum of binding interfaces, showing various degrees of flexibility.1 Globular domains mediate the bulk of interactions characterised to date, but an ever-growing proportion of known cellular interactions involves an interface that lacks a well-defined tertiary structure under native conditions.2,3 The vast majority of unstructured interfaces adapt to a template provided by their structured interaction partner, where following initial binding by conformational selection, optimisation of intermolecular interactions often leads to induction of the secondary structure.4 However, exceptions exist where neither partner provides a rigid template, e.g. the tetramerisation domains of p53.5 Though disordered interaction modules encompass a continuous range of binding interfaces, they can be approximately split into three major classes of autonomous functional interfaces:6 large serpentine disordered domains (e.g. CREB-binding protein (CBP) TAZ domain-binding modules in Hypoxia-inducible factor 1α7), multi-partite disordered interfaces (e.g. Inhibitor-2 binding to Protein phosphatase 18), and compact mono-partite, short linear motifs (SLiMs) (e.g. the canonical SH3 domain-binding PxxP motif9).
Compact SLiMs, often referred to as linear motifs (LMs), miniMotifs or eukaryotic linear motifs (ELMs) (for reviews see ref. 10–12), differ from the large induced fit interface classes on two major attributes, both a direct result of the limited number of residues directly contacting the binding partner. Firstly, as the genesis of a rudimentary functional motif necessitates only a handful of mutations, SLiMs have a greater propensity to evolve convergently.13 This inherent evolutionary plasticity facilitates SLiM proliferation, as evidenced by their ubiquitous presence in higher eukaryotes,3 for instance, regulatory motifs involved in facilitating protein localisation14,15 or synchronising protein degradation.16,17 Accordingly, convergent motif evolution has been hypothesised to expedite interactome adaptability by rapidly adding functional modules to proteins, thereby rewiring networks.11 Secondly, SLiMs have lower affinity for their binding partners (generally in the 1–150 μM range),10 allowing them to engage in reversible and transient interactions.18 Consequently, many dynamic networks, such as those regulating cell signalling, where large multi-protein complexes rapidly assemble and disassemble, depend on reversible yet high avidity SLiM-mediated interactions (e.g. the LAT-GRB2-SOS complex in T-cell receptor signalling19,20). Furthermore, SLiMs can mediate molecular switching by means of overlapping interfaces, altered functional properties conferred by post-translational modifications, or a combination of both, and they allow concentration of upstream signals through high functional interface density (e.g. the extensive disordered regions of p5321). These inherent abilities render SLiMs appropriate interfaces to facilitate co-operative decision-making, a quality central to the robustness of signalling networks.20
Knowledge of the discriminatory attributes of biologically relevant SLiMs is vital to expand our understanding of the complex wiring of the interactome and further the development of bioinformatics tools for motif discovery. To date, several studies have revealed defining attributes of SLiMs.22–25 Certainly, current data suggest that SLiMs predominantly occur in disordered regions,22 although multiple examples in the disordered loops of structured regions are available.26 Additionally, SLiMs are more conserved than their flanking non-functional residues25 and non-functional instances of the same motifs,23,24 pointing to functional constraints that result in strong selection of amino acid residues within the motif. Structural and conservation studies showed that the motif context, the residues surrounding those residues making direct contact with the binding partner, also performs a vital role, often in facilitating the secondary structure induced upon binding.27,28 In this paper, the curated instances of the Eukaryotic Linear Motif (ELM) database3 are analysed to provide a comprehensive overview of the defining attributes of SLiMs, updating previous analyses in light of more extensive data and analysing novel attributes, which, by empirical observation, appear to have discriminatory power for functional motif instances. The defining attributes, physicochemical properties, evolution and structural constraints of motifs are analysed and discussed, focusing on the implications for the motif use by the cell. All data used in the analysis are available in the ESI.‡
Dataset
Eukaryotic Linear Motif (ELM) database
The SLiMs used in this analysis were retrieved from the ELM database (April 2011),3 the only freely available database of experimentally validated eukaryotic motifs (Table S1, ESI‡). The ELM data are hierarchically organised into type, class and instance (see Fig. 1 in study of Gould et al.3). Functionally validated SLiMs are split into 4 types based on the function of the motif: ligand sites (LIG), which cover interaction motifs that mediate binding of the ligand protein to its interaction partner, post-translational modification (PTM) sites (MOD), proteolytic cleavage and processing sites (CLV) and subcellular targeting sites (TRG). These types are further subdivided into 160 classes based on binding partner, modifying enzyme, acting peptidase and targeted subcellular localisation, respectively. Of these, 129 classes possess one or more instances (Table 1) that define a particular motif that has been experimentally validated in a particular protein (e.g. LIG_SH3_1 at positions 88 to 94 in P85A_HUMAN). Due to the small sample size the CLV type was not considered in the analysis.
Table 1 ELM classes and ELM instances categorised (before and after orthologous clustering) by motif type
ELM type |
Number (%) of ELM classes |
Number (%) of ELM instances |
Number (%) of ELM instances post-clustering |
CLV |
8 (5.0%) |
14 (1.0%) |
7 (0.6%) |
LIG |
101 (63.1%) |
925 (64.6%) |
747 (62.0%) |
MOD |
30 (18.8%) |
336 (23.5%) |
313 (26.0%) |
TRG |
21 (13.1%) |
157 (10.9%) |
137 (11.4%) |
Annotation of ELM instances is based on manual annotation of the relevant literature, with a preference for instances whose functionality is thoroughly supported by multiple sources of complimentary experimental evidence. Each ELM class is described by a regular expression (RegEx), defining the residues important for the affinity and specificity of the motif, derived from the collected instances for that class. Several sources of evidence contribute to the definition of a SLiM RegEx. Firstly, data inferred from mutational studies indicate the functionally important residues of a motif. Secondly, aligning regions surrounding collected instances with each other and similar homologous regions in orthologous proteins determines the evolutionarily constrained residues of a motif. Additionally, if available, structural data define residues making direct contact with the interaction partners and suggest residues that may cause sterical hindrance.
The ELM database uses regular expressions to annotate ELM classes as they allow flexible length wildcards, are conducive to simple statistical analysis and are easily understandable to human annotators and investigators.3 The positions within an ELM RegEx can be divided into different categories based on the degree of degeneracy that is allowed at a particular position. Fixed positions are defined as positions that cannot tolerate an amino acid substitution. Degenerate positions are positions that can tolerate a limited number of amino acid substitutions that usually share some physicochemical or structural property. Both fixed and degenerate positions are defined positions in the RegEx. Undefined positions are either wildcard positions, in which any amino acid is allowed, or prohibited positions, in which all but a limited set of amino acids is allowed.
Based on the relevant literature, taxonomic range and cellular compartment of an ELM class are manually assigned by the annotators (Table S2, ESI‡). Taxonomic range also exploits further evidence based on analysis of the distribution of conservation of known functional instances of the ELM class and, if possible, the globular domain that interacts with the ELM class. Some functional ELM instances encoded by viral or bacterial pathogens interact with globular domains encoded by the genome of their eukaryotic host. As these interactions occur in the context of the eukaryotic host's cellular machinery, these instances are considered and annotated as associated with the host/interacting-domain-encoding organism.
Biases
Curation of the ELM database is subject to an acquisition bias that results from the criteria by which motifs are selected for experimental validation and annotation. In the absence of unbiased genome-scale screening for functional motifs, the database composition tends towards the interest of biologists, usually with a preference for proteins of strong therapeutic interest, and the simplicity of discovery, both experimentally and computationally. However, this tendency is not restricted to this analysis, but rather it is inherent to biological focus in general and therefore, this should not invalidate the major trends that are apparent from the analyses described in this paper.
Many motifs do not have enough sequence specificity to define a consensus (e.g., O-linked glycosylation, unlike N-linked glycosylation, does not have a consensus sequence29). Since ELM performs only RegEx pattern searches, such motif instances cannot currently be integrated into the ELM resource, biasing data towards classes with definable sequence specificities. In our experience, LIG sites often have stronger sequence specificities than other ELM types. As a result, 101 LIG classes are available, while only 38 CLV and MOD classes have been annotated (despite extensive data for such sites, for example, ∼25
000 known phosphorylation sites in the human proteome30 and over 300 different post-translational modifications are known31).
Removing redundancy
A non-redundant set of ELM instances was created to remove the bias introduced by closely related, divergently evolved ELM instances. ELM-containing proteins were clustered into groups of orthologous proteins using eggNOG 2.0.32 Metazoan proteins were grouped based on eggNOG metazoan orthologous groups. Eukaryotic instances outside Metazoa were grouped based on eggNOG eukaryote orthologous groups and viral proteins were grouped based on detectable BLAST hits (e-value of 10−4).33 The proteins of each orthologous cluster were aligned with MUSCLE.34 Instances were mapped onto each multiple sequence alignment and instances of the same ELM class falling in the same column of the alignment in different proteins were considered evolutionarily divergent and clustered in homologous instance clusters (HIC) (Table S3, ESI‡). A representative instance for each HIC was chosen, based on the evolutionary distance from Homo sapiens or, in the case where two or more instances were of equal distance from Homo sapiens (e.g. instances in paralogues), based on the amount of annotated experimental evidence. Post-processing, 1204 HICs in 799 proteins remained (CLV: 7, MOD: 313, TRG: 137, LIG: 747) (Table 1, HIC data are available in Table S4, ESI‡).
A small proportion of motif instances (141, 11.7%) are contained within extracellular regions of proteins (Table S2, ESI‡). Biases can be caused by the differing properties of extracellular and intracellular regions, for example intrinsic disorder is depleted in extracellular regions,35 and the uneven distribution of instances across ELM types (of 141 extracellular ELM instances, 126 are modification sites, 10 ligand sites and 5 cleavage sites). In the majority of analyses this bias is negligible, however, on occasions where the results of the analysis of extracellular and intracellular ELM instances deviate from the analysis of grouped ELM instances these differences will be discussed.
Control dataset
Experimentally validated negative instances are rare, therefore a control dataset of putative false positives (FPs) was constructed by taking instances in the 799 ELM-containing proteins that match the ELM RegExs but are not yet present in the ELM database (142
226 instances, Table S5, ESI‡). The majority of FP instances are likely to be non-functional, however, as the coverage of known SLiMs in the ELM database is incomplete, this set will undoubtedly also contain functional instances. For the analysis of motif contexts, the regions surrounding matches in both TP and FP sets were split into: adjacent positions, undefined positions within a motif and positions within 3 residues from the first and last defined position of the motif, and flanking positions, positions located between 3 and 10 residues from the first and last defined position of the motif.
Motif attributes
General features of SLiMs were extracted using the RegExs from 160 ELM classes (Table 2, and Table S6 and Fig. S1, ESI‡). ELMs have an average length of approximately 6 (6.3) residues and range in length from 1 residue (the N-terminal LIG_BIR_II_1 class) to 23 residues (the flexible length MOD_TYR_ITAM class). 125 (78.1%) ELM classes have a length between 3 and 8 amino acid residues, while 143 classes (89.4%) consist of 3 to 11 residues (Fig. 1A). On average, the annotated ELMs contain 3.7 defined positions, 1.9 of which are fixed positions, and 1.8 of which are degenerate positions (Fig. 1B). On average 2.6 of the positions of an ELM are undefined, 2.1 of which are wildcard positions, and 0.5 of which are prohibited positions. Furthermore, 139 (86.9%) of the annotated ELM classes contain between 0 and 3 wildcard positions, while 35 (21.9%) of them have one or more prohibited positions in which certain amino acids are not allowed. 25 ELM classes (15.6%) contain at least one variable length gap, which is defined as a flexible number of wildcard positions between two defined residues in a RegEx. While the majority of ELM classes describe motifs that have no positional preference within the protein (137 classes, 85.6%), 23 (14.4%) are located at the protein termini, with 8 (34.8% of the cases; 5.0% of total) located directly at the N-terminal of the protein, and 15 (65.2% of the cases; 9.4% of total) located directly at the C-terminal of the protein (Table 3).
Table 2 The average number of positions in an ELM RegEx for each of the different position categories as defined in the text
Position |
Average positions |
Total length |
6.3 |
Defined |
3.7 |
-Fixed |
1.9
|
-Degenerate |
1.8
|
Undefined |
2.6 |
-Wildcard |
2.1
|
-Prohibited |
0.5
|
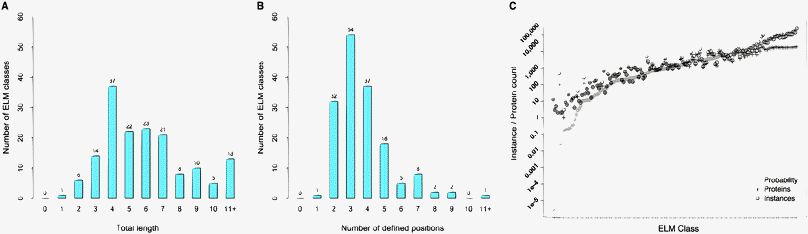 |
| Fig. 1 For 160 ELM classes, (A) the distribution of the motif lengths, (B) the distribution of the total number of defined positions in a motif and (C) occurrences of a RegEx match within the human proteome (circles), number of proteins containing an occurrence of a RegEx match (plus symbol) and the expected number of occurrences of a RegEx match in the human proteome (diamonds). ELM classes are ordered according to the expected number of occurrences. | |
Table 3 ELM classes and ELM instances categorised (after orthologous clustering) based on their position within a protein sequence (All classes) and the requirement of post-translational modifications for binding (LIG classes only)
Feature |
Number (%) of ELM classes |
Number (%) of ELM instances post-clustering |
N-terminal |
8 (5.0%) |
29 (2.4%) |
C-terminal |
15 (9.4%) |
104 (8.6%) |
Internal |
137 (85.6%) |
1071 (89.0%) |
Pre-modified (1×) |
18 (11.3%) |
83 (5.8%) |
Pre-modified (2×) |
2 (1.3%) |
20 (1.4%) |
Methods
The level of degeneracy of a SLiM correlates with the stochastically occurring motif count in a proteome, therefore expectation of random occurrence is not equal for all ELM classes. To illustrate this, a proteome-wide scan for matches to ELM RegExs was performed using 20
225 human sequences (excluding isoforms) from Uniprot.36 For each ELM class, the number of instances, and of proteins containing at least one instance, was noted. Additionally, the number of instances expected to appear at random in the human proteome was calculated (as described by Edwards et al.37) based on amino acid frequencies of disordered residues in the human proteome (residues in reviewed UniProt human proteins with IUPred60 scores greater than or equal to 0.4,22 Fig. S2 and Table S7, ESI‡).
Results
Fig. 1C shows the number of RegEx matches, expected number of RegEx matches and the number of proteins containing one or more RegEx match for 160 ELM classes in the human proteome (Table S7, ESI‡). The counts vary across several orders of magnitude, reflecting the significant difference in the level of degeneracy. Motifs with stronger sequence specificities tend to occur rarely by chance (left side of Fig. 1C), for example, the ELM class “LIG_Clathr_ClatBox_2” is only found in two human proteins (AMPH_HUMAN and BIN1_HUMAN), which are also the only annotated instances for this class (amino acid positions 381 to 385 and 416 to 420, respectively), indicating that the pattern definition of this ELM class might be too strict. Conversely, ELM classes that are more degenerate are found in a higher number of protein sequences and often more than once per protein (e.g. MOD_GlcNHglycan and MOD_GSK3_1 with instances found in 18
739 and 19
317 proteins, respectively).
Biological implications
The defining feature of SLiMs is their ability to encode a functional and specific interaction interface with a limited number of residues. However, this degeneracy renders stochastically occurring non-functional motifs quite common. Consequently, regions resembling known functional SLiMs are ubiquitous. Yet, the majority of these will be non-functional (an observation that strongly advocates caution when considering putative motifs based on RegEx matches). This raises the question, how does the cell discriminate between biologically significant and randomly occurring instances?
An important aspect is that under normal cellular concentrations, biologically relevant motifs will generally outcompete lower affinity off-target instances38 (the implications for motif definitions based purely on binding assays are clear and advocate functional assays as the minimum criteria for experimental validation of a motif39). Moreover, many false positive instances will not co-occur with their corresponding binding partner due to restricted protein expression as a result of cell compartmentalisation,40 cell cycle phase41 or tissue specificity.42 These spatial and temporal boundaries decrease the likelihood of many possible off-target binding events. Preclusion of direct interactions between a large portion of proteins by eukaryotic cell compartmentalisation is reflected by many SLiMs having distinct functional localisation, with 43% of the annotated ELM classes functioning in the nucleus, 61% in the cytosol and only 18% in both. Furthermore, even when proteins come into direct contact, additional layers of regulation can inhibit binding outside specific localisations, for example, the AP2μ binding site for the endocytic signal Yxxφ (TRG_ENDOCYTIC_2 class) only becomes accessible, in a phosphorylation-dependent manner, upon co-localisation with clathrin at the cell membrane.43
Residues directly bordering the motif play an important role in the recognition process, and thus in the distinction between functional and non-functional instances. To reflect this, many ELM class RegExs (∼22%) contain prohibited positions that disallow certain amino acids. The majority of these positions disrupt binding by sterical hindrance (for example, the helical nuclear receptor box (LIG_NRBOX class) motif disallows helix-breaking prolines in certain positions), however, several examples also relate to physicochemical incompatibility with the interaction partner's binding site. For example, SxIP motifs (LIG_SxIP_EBH_1 class) disallow flanking acidic residues as a result of incompatibility with their binding pocket on EBH domains of microtubule end-binding proteins.44 Interestingly, certain EBH domain-binding SxIP instances take advantage of this to modulate binding by altering their physicochemical properties in a phosphorylation-dependent manner.44 Such modification of adjacent residues to affect motif binding by altering affinity appears to be a common mechanism for regulating motif binding, either negatively (e.g. cyclin/CDK inhibition of HPV E1 cytoplasmic localisation by phosphorylation of nuclear export signal flanking regions45) or positively (e.g. the phosphorylation-dependent rheostat modulation of p53 affinity for CBP46), compounding the importance of the flanking regions of SLiMs.
Co-operative attributes
Modification
Nearly a quarter of ELM classes are post-translationally modified (38 classes, 23.8%), 30 (79.0%, 18.8% of total) of which are modified by addition of a post-translational moiety, and 8 (21.1%, 5.0% of total) of which are sites for proteolytic cleavage. Notably, 20 (12.5%) ligand ELM classes require post-translational modification in order to be functional. The modification involved is a phosphorylation of one (17 classes, 85.0% of the cases; 10.6% of total) or two (2 classes, 10.0% of the cases; 1.3% of total) residues, or a prolyl hydroxylation (1 class, 5.0% of the cases; 0.6% of total) (Table 3).
Overlap
Of all ELM instances, 66 (5.4%) overlap with at least one other annotated instance from any ELM class. A further 177 instances (14.7%) occur within 10 residues of another ELM instance (Table S8, ESI‡).
Repeats
Approximately one-third of motif instances (420 instances, 34.9%) in 44 (27.5%) ELM classes occur with at least one additional copy of the same ELM class in the same protein (135 clusters, see Table S9, ESI‡). Several classes have multiple examples of tandem motifs, for example, 15 proteins (54 instances) and 5 proteins (45 instances) of the canonical EH domain-binding NPF motif (LIG_EH_1 class) and the adaptor protein AP-2 alpha-subunit-binding DPW motif (LIG_AP2alpha_2 class), respectively, contain clusters that contain two instances or more. For 12 (7.5%) ELM classes, instances occur more frequently in proteins with an additional instance of the same motif class than with only one instance.
Biological implications
PTMs are an important cellular control mechanism that not only modifies the physicochemical properties of the individual amino acids but also alters protein activity, folding, distribution, stability, and consequently, function.47 Unsurprisingly, 12.5% of ligand-binding motifs in ELM are regulated by PTMs, their functionality having the prerequisite of enzyme recruitment and modification, which enables these proteins to exhibit switch-like behavior. For example, DNA damage-induced phosphorylation of a serine residue on SxxF motifs in the CTiP complex is required for binding to the BRCT domain of BRCA1.48 Moreover, modification “switches” do not always follow the on–off light switch analogy. Modified residues can also shift the specificity of a motif from one domain to another, for example, the endocytic signal Yxxφ of CLTA-4, typically recognised by the clathrin-coated vesicle adaptor protein AP2μ, binds the SH2 domain of Syp upon tyrosine phosphorylation.49 Modification sites can themselves also be regulated by modification. Candidate glycogen synthase kinase 3 (GSK-3) sites can be found in almost every human protein sequence, reflecting their weak sequence specificity (p[ST]xxx[ST]), however, this is offset by the requirement of a priming phosphorylation prior to GSK3 phosphorylation.50
Overlapping SLiMs can also act as molecular switches by requiring mutually exclusive binding of interacting partners, thereby enabling the transmission of robust and discrete signals. For example, Ataxin-1 contains overlapping 14-3-3, NLS and ULM motifs, so that phosphorylation switches specificity to 14-3-3-binding, thereby inhibiting the recruitment of splicesomal components via the UHM motif.51 Overlapping motifs also allow efficient use of space by allowing multiple sites for different interaction partners within a small region, an extreme example of the general property of disordered regions to encode interfaces in a compact fashion,52 and an attribute that is particularly useful for general functionality such as localisation and degradation signals (e.g. the nuclear localisation signal of retinoblastoma overlaps with a cyclin/CDK-binding motif53,54). Relatively few overlapping motifs are annotated in the ELM database (66 of the 1204 instances in this analysis overlap with one or more instances), however, approximately 15% of ELM instances occur within 10 residues of another motif, suggesting some level of co-operativity. Nevertheless, we expect this to be a common feature.
Many motifs can act multivalently, coordinating the use of multiple interaction interfaces simultaneously (such as the tandem SH2-binding motifs referred to as ITAMs55). Consequently, the observation that nearly 35% of ELM instances co-occur with additional copies of the same ELM class is not unexpected. Multivalency allows SLiMs to initiate multiple low affinity interactions, varying the number of repeats to tune affinity, collectively resulting in high avidity interactions with a strength that is comparable to globular–globular interactions (homomultimerisation also allows avidity effects even when the motifs do not occur in tandem56). Avidity effects allow proteins to assess binding partner density, binding transiently until sufficient density has been reached to achieve a high avidity interaction, a paradigm of co-operative decision-making.20 Furthermore, tandem repeats make excellent platforms to recruit and scaffold proteins into large multi-component complexes. Endocytosis, in particular the motif-rich clathrin coat recruitment,57 provides several excellent examples of tandem motif use, for instance, the extensive repeats of AP2-binding DPW motifs in multiple endocytic proteins58 (EPS15 alone contains 15 DPW instances59).
Physicochemical properties
Methods.
Amino acid preferences of defined, fixed and degenerate residues (grouped as all (discussed below), extracellular and intracellular) for 1204 ELM instances were assessed and compared against the properties of their adjacent and flanking residues, as well as those residues contained within disordered regions (defined as regions with IUPred scores greater than or equal to 0.422,60). The amino acid frequencies were calculated for each instance within an ELM class and averaged across the class to ensure no bias towards classes with large numbers of annotated instances. Fold change relative to the amino acid frequencies of disordered regions was calculated for the amino acid frequencies and amino acids were grouped by physicochemical properties61 (Table S10, ESI‡). The mean charge (isoelectric point (pl)) and hydropathy (hydrophobic moments (HM)62) were also calculated for each instance within an ELM class and averaged across the class63 (Table S11, ESI‡).
Results.
Several strong physicochemical preferences were shown in all ELM types (Fig. 2), their flanking and adjacent regions (Fig. S3A, ESI‡). In the defined positions, aromatic and hydrophobic amino acids are enriched (1.92- and 1.61-fold increase, respectively) compared to disordered regions in general. Hydrophobic residues are also enriched within the degenerate positions (LIG: 1.73-, MOD: 1.47-, TRG: 1.58-fold increase). Conversely, small, tiny, and C-beta branching residues are under-represented (1.31-, 2.43-, 1.45-fold decrease, respectively) in the defined positions. Tryptophan is depleted within the adjacent regions of all motifs (LIG: 1.54-, MOD: 2.67-, TRG: 2.28-fold decrease).
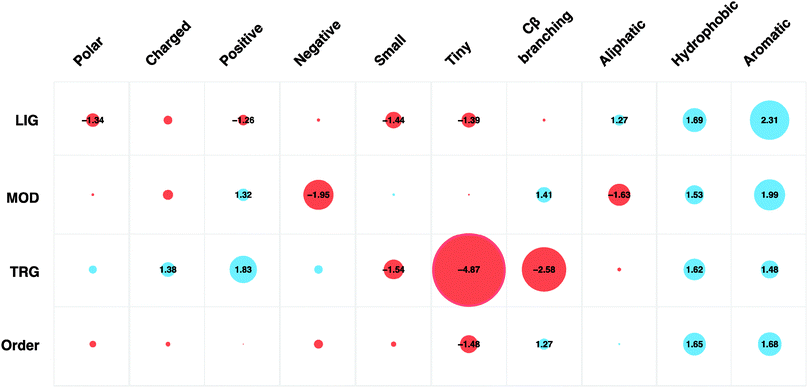 |
| Fig. 2 Amino acid preferences (grouped by physicochemical properties) of ELM instances split by motif type (LIG, MOD, TRG) and predicted ordered regions (IUPred < 0.4). Circle sizes are proportional to fold change preference compared to disordered regions in general (IUPred ≥ 0.4). Blue circles indicate amino acids of a given physicochemical property are over-represented compared to disordered regions whilst the red circles indicate depletion of those amino acids. Scores within the circles denote fold change compared to the amino acid preferences of disordered regions. | |
Amino acid propensity was not consistent across each ELM type. Positive amino acids are strongly favoured by modification and targeting motifs (1.32- and 1.83-fold increase, respectively). Interestingly, for modification sites, arginine (1.77-fold increase) is preferred to the physicochemically similar lysine (1.34-fold decrease). Aromatic amino acids are strongly favoured in the fixed positions of ligand (2.75-fold increase) and modification (3.93-fold increase) motifs. Cysteine (15.87-fold increase) and tryptophan (8.16-fold increase) are often observed in the fixed positions of modification motifs. Ligand motifs disfavour polar residues and amino acids with positive charge, with a 1.34- and 1.26-fold decrease, respectively. They instead have a preference for aliphatic, aromatic and hydrophobic residues (1.27-, 2.31- and 1.69-fold increase, respectively). In the fixed positions of ligand motifs, proline (2.21-fold increase) and tyrosine (4.77-fold increase) residues are favoured. Targeting motifs also have a particularly strong aversion to small, tiny and C-beta branching residues (1.54-, 4.87- and 2.58-fold decrease, respectively).
Adjacent and flanking amino acids also differ by motif type (Fig. S3A, ESI‡). The adjacent residues of targeting motifs are enriched for positively charged amino acids (1.33-fold increase) but have an aversion for aromatic residues (1.67-fold decrease), whilst the flanking regions are ambivalent to aromatic residues (no fold change) but developed a tendency for negative residues (1.19-fold increase). The flanking and adjacent amino acids of modification sites, in general, resemble more the features of ordered regions, whilst the flanking regions of targeting and ligand motifs most closely resemble the disordered regions, an observation that reflects the structural context of each ELM type. The effect of splitting the data into extracellular and intracellular groups was negligible, propensities resembled those of modification instances (which are enriched in annotated extracellular instances, 126 out of 141 extracellular instances are modification sites) with the exception of a preference for small residues (intracellular: −1.85-fold, extracellular: 2.12-fold) and increased aversion for aliphatic residues (intracellular: −1.4-fold, extracellular: −6.04-fold) (Fig. S3B, ESI‡).
Hydrophobicity versus charge
All ELM types differ strongly from the generic properties of disordered regions (charge: 6.05pl; hydrophobicity: −1.01HM, see Fig. S4, ESI‡). The fixed positions of targeting motifs display a high charge level (6.70pl) with low hydrophobicity (−1.69HM), however their degenerate positions resemble disordered regions (charge: 6.02pl; hydrophobicity: −0.98HM). Ligand motif instances exhibit a higher level of hydrophobicity than both ordered (−0.56HM) and disordered regions (−1.01HM) in both their fixed (−0.46HM) and degenerate (−0.11HM) positions. The fixed positions within modification motifs have a low hydrophobicity (−1.49HM) and are slightly more charged (6.20pl) than disordered regions (6.05pl), whilst their degenerate positions share very similar charge and hydrophobicity properties to ordered regions. The flanking and adjacent residues of the targeting and ligand motifs all clustered around disordered region values, however the undefined positions of modification residues were slightly more hydrophobic (adjacent: −0.84HM and flanking: −0.92HM).
Biological implications
Natively unstructured interfaces have previously been shown to have a strong preference for hydrophobic residues.64 This makes them distinct from domain–domain binding interfaces, which are enriched for charged and polar residues and depleted in hydrophobic amino acids,64,65 and from disordered regions in general, which also contain a high proportion of charged and polar residues.66 Analyses of ligand motifs corroborate these findings and additionally suggest that aromatic residues are of particular importance. For some observations, the residue preferences of SLiMs reflect some underlying biological significance, related to thermodynamics or kinetics. For instance, the rigidity of polyproline stretches results in minimal loss of conformational entropy upon binding,67 and as a result they are strongly enriched in some ligand motifs (e.g. the proline-rich SH3, WW, GYF, EVH1 domain-binding motifs). Conversely, tiny, non-polar residues (alanine, glycine) are disfavoured, reflecting their non-reactivity and tendency to disfavour secondary structure formation that is induced in many motifs (∼60%) upon binding. Some preferences have simple functional implications. The huge enrichment for cysteine in PTM sites is likely due to the reactive thiol group of cysteine. Certain preferences, however, are more puzzling, such as the preference for positively charged residues in targeting motifs. This may suggest two separate types of interaction interfaces, one favouring hydrophobic interactions (enriched in ligand and modification motifs) and the other favouring electrostatic interactions (enriched in targeting and possibly cleavage motifs), however the biological explanation remains to be revealed.
Evolution
Orthologue alignment construction
Single-protein-per-species orthologue alignments were created for each ELM-containing protein as described by Davey et al.25 Homologous sequences for each protein were identified using a BLAST search33 against a non-redundant database of EnsEMBL proteomes (release 5968). For each protein-coding gene in each EnsEMBL metazoan (plus yeast) genome, the longest isoform was chosen (except if the sequence differs from the main UniProt isoform (SwissProt or TrEMBL), in which case the sequence was replaced by the UniProt sequence36). Orthologues for each ELM-containing protein were predicted using the GOPHER algorithm with default parameters.69 Multiple sequence alignments were generated with MUSCLE.34 Long branches were pruned as described by Chica et al.24 and 79 alignments with less than 4 species remaining were not considered (including 33 viral, 33 fungal and 2 bacterial alignments). The remaining alignments contained 1098 ELM instances in 126 classes.
Conservation
Methods.
TP and FP instance datasets were scored using two complementary conservation metrics: the Conservation Score (CS), a column-based, tree-weighted information content score measuring the raw conservation of a motif,24 and the Relative Local Conservation (RLC), measuring the conservation of a motif relative to their neighbouring regions.25 CSs are weighted based on the divergence of organisms within the alignment from the query sequence and range between 0 and 1 (with 0 denoting no conservation, and 1 denoting conservation across all species). RLC extends the CS metric, calculating the conservation of each residue relative to amino acids within 25 residues (scores are distributed around 0, with values above 0 more conserved than their bordering regions). The flanking, adjacent and defined residues of TP and FP ELM instances (see Dataset for definition) were scored for CS and RLC. A mean conservation score (MCS) of defined, adjacent and flanking residues of each ELM instance was calculated using the CS and the RLC scoring scheme (Table S12, ESI‡).
Results.
Defined residues within TP ELM instances were significantly more conserved than defined residues within FP instances (Mann–Whitney–Wilcoxon test, one-sided, both p = < 2.2 × 10−16). The average MCS of defined residues of TP instances are higher (RLC: 0.592, CS: 0.667) than MCSs of defined positions of FP instances (RLC: −0.011, CS: 0.596). Furthermore, the defined positions of TP instances are clearly more conserved than their surrounding residues (RLC: 0.592, see black peak around the ELM instances in Fig. 3C) and also have higher MCS than either their adjacent or flanking residues (RLC adjacent: 0.039, RLC flanking: −0.196 and CS adjacent: 0.539, CS flanking: 0.487). Adjacent residues of TPs have higher MCSs than flanking residues, however, corresponding regions of FPs have comparable MCSs (RLC adjacent: −0.065, RLC flanking: −0.086 and CS adjacent: 0.560, CS flanking: 0.557) (Fig. S5, ESI‡). The slight increase in conservation scores of defined positions of FP instances suggests that several FP instances may be functional but have not yet been defined as such. Further literature searches corroborated this assumption, revealing a handful of well conserved experimentally validated, but unannotated, instances in the FP dataset. The MCS for CSs of flanking residues of TPs (CS flanking: 0.487) are lower than corresponding regions of FPs (CS flanking: 0.557). This observation may be explained by the increased proportion of FPs in ordered regions, which have higher conservation levels due to structural constraints. Clear differences are detectable between the average MCSs of different ELM types: motifs of the LIG type (mean RLC: 0.751, mean CS: 0.704) are more conserved than both MOD (mean RLC: 0.307, mean CS: 0.621) and TRG (mean RLC: 0.383, mean CS: 0.638) motifs (Fig. 3B). Defined positions of TP instances (CS: 0.667) are more conserved than predicted disordered regions (CS: 0.505; IUPred cutoff greater than or equal to 0.4) and residues within Pfam (version 25.079) domains (CS: 0.640) (Fig. 3A), however, when split by type, only LIG instances are more conserved than domains.
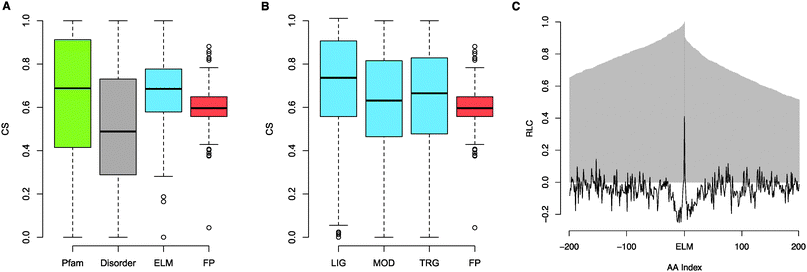 |
| Fig. 3 (A) Boxplot of CSs of residues annotated as within Pfam domains (green), CSs of residues predicted to be within disordered regions (IUPred scores ≥ 0.4) (grey), mean CSs for annotated ELM instances (blue) and mean CSs for FP instances (compression of boxplot is due to larger sample size decreasing variance) (red). (B) Boxplot of mean CSs for annotated ELM instances split by motif type (LIG, MOD, TRG) (blue) and mean CSs for FP instances (red). (C) RLC scores of the 200 residues (AAindex −200 to 200) either side of annotated ELM instances. The proportion of proteins with data at a given distance (AA index) from the motif is plotted in grey. | |
Convergent evolution
Methods.
Clustering of instances into homologous instance clusters, as described previously in Dataset, removes all obvious gene duplication- and speciation-related divergently evolved instances and can therefore be considered an upper limit to the number of convergently evolved instances (i.e. there are at most 1204 convergently evolved instances in the ELM database). However, not all remaining instances are unquestionably convergently evolved, since other evolutionary processes can generate novel but divergent motif instances. For example, gene duplication followed by a gene fusion event may give the appearance of convergence. Alternatively, intra-gene duplications caused by tandem exon duplications70 or repeat expansion71 may result in divergent tandem motifs. To calculate a high confidence lower limit of the number of convergently evolved SLiMs, for each ELM class, the proteins containing each experimentally validated instance were sorted into “Unrelated Protein Clusters” (UPCs).37 UPCs are non-divergent protein clusters, i.e. proteins in separate clusters cannot share a BLAST-detectable homology (e-value of 10−4 and complexity filtering),33 however, not all protein pairs within a UPC have a BLAST-detectable homology as two proteins can have BLAST-detectable homology with a third protein but not with each other. UPC counts can be considered an overly strict lower bounds estimate of the number of convergently evolved instances for an ELM class.
Results.
Of the 1204 ELM instances in the filtered dataset, a minimum of 628 are highly likely to be convergently evolved instances, occurring in proteins with no BLAST-detectable similarity (Table S13, ESI‡). Over half of the ELM classes (92 classes, 57.5%) have an example of convergent evolution (i.e. having instances occurring in 2 or more UPCs), and 18 classes (14%) possess at least 10 convergently evolved instances. For example, both the APC Cdh1 subunit-binding KEN box (LIG_APCC_KENbox_2 class) and the KDEL endoplasmic reticulum retrieval signal (TRG_ER_KDEL_1 class) have evolved convergently on at least 10 occasions. The retinoblastoma-binding motif LxCxE (LIG_Rb_LxCxE_1 class) has 22 convergently evolved instances, including multiple examples in viral proteins.
Taxonomic range
The annotated taxonomic distribution of ELM classes specifies the taxonomic groups in which the ELM class is believed to be functional, and in some cases also the taxonomic groups in which it is believed not to function (although only 4 ELM classes are excluded from a taxonomic group) (Table S14, ESI‡). The distribution of the taxonomic range of ELM classes is presumably strongly influenced by the tendency of the ELM resource (and biological research in general) to focus on understanding organisms more closely related to humans compared to more distantly related organisms. Therefore, it would be irresponsible to take any of the trends seen in ELM taxonomic data as being representative of SLiMs in general, nevertheless, some interesting observations may still be made. For example, one ELM class, LIG_PCNA is annotated as present in Archaea, Bacteria and Eukaryota. All other ELM classes are annotated as restricted to either Eukaryota (81 classes) or to subsets of this taxonomic group. The second-largest group of ELM classes (41 classes) is annotated as restricted to Metazoa, and the third-largest group is restricted to Vertebrata (14 classes). Several ELM classes fall into interesting taxonomic subsets, for example, Caenorhabditis elegans appears to have lost a secondary peroxisomal targeting signal (TRG_PTS2 class), and the polypyrimidine tract-binding protein (PTB) RRM2 domain-binding PRI motif (LIG_RRM_PRI_1 class) appears to be chordate-specific (though the taxonomic range of PTB is more extensive).
Methods.
Each ELM instance was mapped onto their corresponding orthologue alignment and the species that matched the ELM class RegEx at the same position as the validated ELM instance were noted (Table S14, ESI‡). Several factors will bias the discovery of motif instances across orthologues, including poor quality of alignment, divergence of motif sequence specificity and badly constructed genomes, however, the analysis will provide a conservative estimate of the taxonomic range of the conservation of motif instances.
Results.
983 ELM instances were experimentally validated in human (823) or in another species but conserved in human. Of these 983 human instances, 894 (90.9%) were conserved in Mus musculus, 479 (48.7%) in Danio rerio, 146 (14.9%) in Drosophila melanogaster, 76 (7.7%) in Caenorhabditis elegans and 35 (3.6%) in Saccharomyces cerevisiae (Fig. 4). Motifs conserved from yeast to human included a D-box (LIG_APCC_Dbox_1 class)72 and MAD2-binding motif (LIG_MAD2 class)73 in APC/C activator protein CDC20 and a PIP box (LIG_PCNA class) in DNA excision repair protein ERCC-5 (RAD2 in yeast)74 (Table 14, ESI‡). Targeting motifs have the largest taxonomic range with 34 (33.3%) TRG instances conserved between human and fly. Modification sites are, in general, conserved over shorter evolutionary distances than other ELM types. Only 19 (6.6%) MOD instances are conserved between human and fly compared to 93 (15.7%) ligand motifs (Table S14, ESI‡). The disparity between TRG conservation scores and their extensive taxonomic range relates to the highly degenerate nature of several targeting motifs, which retain functionality while losing much of their sequence similarity, for example the peroxisomal targeting signal 1 (TRG_PTS1 class defined as (.[SAPTC][KRH][LMFI]$) or ([KRH][SAPTC][NTS][LMFI]$)).
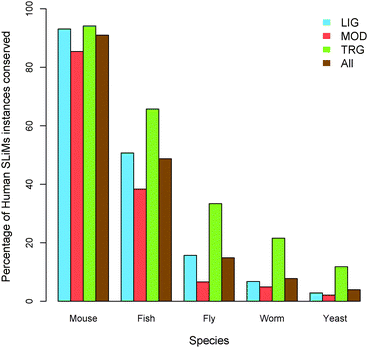 |
| Fig. 4 Taxonomic range Homo sapiens ELM instances, split by the motif type, based on their conservation in several model organisms. | |
Biological implications
SLiM use has been observed in all phylogenetic kingdoms. However, only one known example is present in Archaea, Bacteria and Eukaryota, the PCNA-binding PIP box motif, responsible for mediating interactions with components of the DNA replication and repair apparatus common to all cellular organisms. Though only half (81) of the ELM classes are annotated as occurring across all Eukaryota, many instances are conserved across large evolutionary distances, for example at least 15% of ELM instances are shared between fly and human. Accordingly, we found functional SLiMs are relatively highly conserved, more so than both their flanking non-functional residues and corresponding non-functional instances, corroborating previous studies.23–25 However, different ELM types display different patterns of conservation. While ligand-binding motifs are strongly conserved (comparable with globular domains) and targeting motifs retained function though not sequence over great evolutionary distances, modification sites are relatively poorly conserved, possibly reflecting functional redundancy of modification sites75 or relating to their simpler genesis, leading to migration of motifs within a protein.
SLiMs also possess great evolutionary plasticity, however, to date, this observation has been supported solely by anecdotal evidence. This analysis has shown that at least 57% of ELM classes possess annotated convergently evolved instances, extending observations from viral proteins where convergently evolved instances are known for 30% of the ELM classes.13 Furthermore, many targeting motifs, such as the NLS (nuclear localisation signal) and NES (nuclear export signal),15 and modification sites76 also have numerous unannotated convergently evolved instances. Remarkably, whole classes of motifs can be gained and lost in specific lineages, emphasising the plasticity of these modules, exemplified by the apparently chordate-specific use of PTB RRM-binding PRI motifs and the loss of the secondary peroxisomal import system in C. elegans. These observations add weight to the hypothesis that the use of unstructured, compact and evolutionarily adaptable interfaces might be nature's way to accelerate network evolution and increase network connectivity, rendering them central to the evolution of the increased levels of regulation that is necessary to support complex biological systems and organisms.11 Ultimately, many evolutionary questions concerning motifs remain to be answered, particularly regarding motif genesis where, outside of evidence suggesting that non-functional instances that match a known functional motif but adversely affect the function of a biologically relevant instance will be selected against,77 little is known.
Structural context
Bound secondary structure
Methods.
154 PDB structures of ELM instances in complex with their binding partner were parsed to reveal 250 motif-containing chains for 68 classes. The secondary structure of the regions spanning the annotated motif in each chain of solved motif-containing structures was assigned using DSSP78 and the secondary structure content of an ELM class was calculated as the mean secondary structure proportions across all chains for that class (Table S15, ESI‡). Each ELM class was classified as having one of six possible secondary structures based on their secondary structure content (Helical (>50% of residues in 310 helix, α helix or π helix), strand (>50% of residues in β sheet or β bridge), partially helical or β strand (>25% but <50% of residues being helical or β strand), turn (high curvature or turn) and unstructured (no structure having more than 25% of any secondary structural element)).
Results.
40 (59%) ELM classes solved in complex with an interaction partner contain secondary structural elements in their bound conformation (Fig. 5A). Less than a quarter of classes (15 classes (22%)) are induced to form the secondary structure over the majority of their interface, 11 (16%) of which are mostly helical and 4 (6%) of which are mostly β strand, binding by β augmentation. A further 25 classes (37%) are partially structured when bound (motifs may have greater than a quarter of their residues with 2 different secondary structures, e.g. the LIG_PTS2 class contains both strand and helix), 12 (17%) are partially strand, 5 (7%) are partially helical and 11 (16%) form a turn when bound. Finally, 28 (41%) do not adopt a recognisable secondary structure when bound.
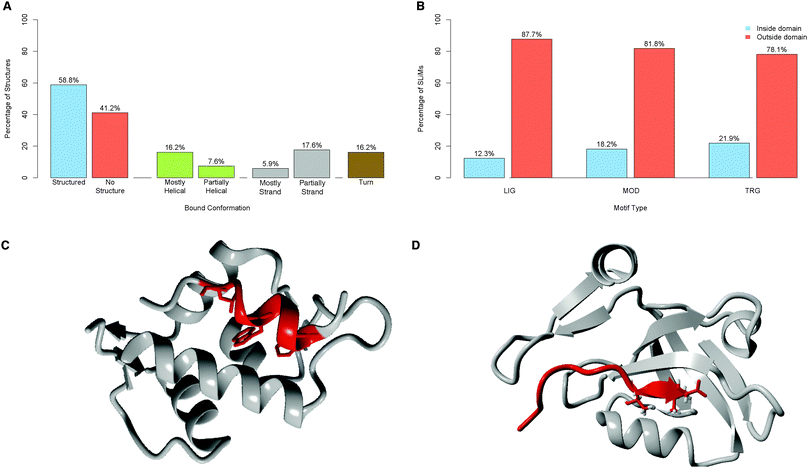 |
| Fig. 5 (A) Secondary structure features of 68 ELM classes solved in complex with their binding partner. “Structured” denotes classes where greater than 25% of the residues spanning the motif are contained within a helix, strand or turn, the remainder are defined as “No structure”. Other categories are as defined in the text. (B) Classification of ELM instances, split by motif type, according to their position relative to Pfam domains. (C) Example of a helical motif, MDM2-binding motif of p53 (red) bound to MDM2 (grey)104 (PDB:1YCR). (D) Example of a strand motif, the PDZ-binding motif of Usher syndrome type-1G protein binding (red) to the PDZ domain of Harmonin (grey)105 (PDB: 3K1R). | |
Disorder and domains
Methods.
The 799 ELM-containing proteins contain 2297 globular (Pfam domains with a mean IUPred disorder value below 0.4 were considered60,79) and transmembrane (as annotated by UniProt36) domains. ELM instances were split into 2 categories based on their positioning with respect to domains: (i) ELM instances located completely or partially within the boundaries of domains, and (ii) ELM instances located outside the boundaries of domains, or found in proteins that do not contain a domain. Furthermore, for each ELM instance, the distance to the nearest domain was measured as the number of residues between the boundaries of the ELM and the domain. The intrinsic disorder propensity of the 799 ELM-containing proteins was calculated using IUPred.60 The disorder attributes of 1204 manually annotated ELM instances, as well as FP hits matching ELM class RegExs in proteins of the ELM dataset, and their bordering regions were analysed. The mean IUPred disorder score (MIDS) for the defined positions of ELM instances was calculated and averaged across all instances of a class. The IUPred disorder scores for each residue in the neighbouring 200 residues, upstream and downstream of the motif, were calculated and averaged for each ELM class.
Results.
257 (21%) ELM instances fall within globular domains and proportions vary with the ELM type. Modification sites (126 instances, 40.3% of MOD instances) occur more commonly in domains than ligand (97 instances, 12.9% of LIG instances) and targeting (30 instances, 21.9% of TRG instances) sites (Fig. S6A, ESI‡). However, this observation is largely due to the enrichment of MOD ELM instances in extracellular regions of proteins (of 141 extracellular ELM instances, 126 are modification sites, 10 ligand sites and 5 cleavage sites), where disordered regions are depleted.35 When only intracellular instances are considered the proportions are comparable (LIG: 87.7%; MOD: 81.8%, TRG: 78.1% occurring outside domains) (Fig. 5B). The average distance from an ELM instance to the nearest known globular domain is 154 residues, but there are distinctive differences between the different ELM types (LIG: 184; MOD: 97; TRG: 70) (Table S16, ESI‡).
As reported by Fuxreiter et al.,22 SLiMs are enriched in disordered regions (71.1% of annotated instances have MIDSs greater than or equal to 0.4, see Fig. 6C), with an average MIDS of 0.54 (Fig. 6A). FP instances show no preference for disordered regions (average MIDS of 0.45) (Fig. 6B). Defined residues within TP ELM instances were significantly more disordered than defined residues within FP instances (Mann–Whitney–Wilcoxon test, one-sided, p = < 2.2 × 1016, Table S16, ESI‡). Furthermore, only 28.9% of annotated functional motifs have a mean IUPred score below 0.4, compared to 45.7% of FP matches. The propensity to occur in disordered regions also varies depending on whether extracellular or intracellular regions are considered, corroborating the finding of the Pfam analysis (Fig. S7, ESI‡). Intracellular ELM instances are enriched in predicted disordered regions (127 (68%) MOD, 592 (80%) LIG and 104 (76%) TRG instances occur in predicted unstructured protein regions with an MIDS ≥ 0.4). Conversely, extracellular MOD instances often occur in predicted structured regions (103 (82% of extracellular MOD instances) occur with an MIDS > 0.4) (Fig. S6B, ESI‡).
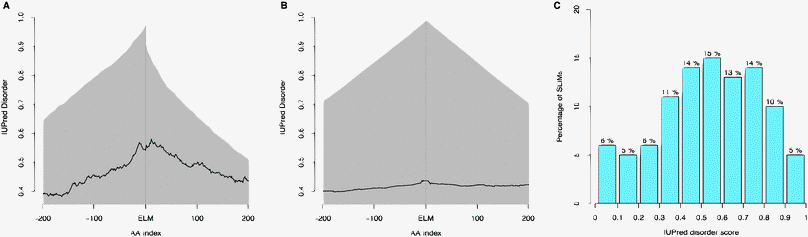 |
| Fig. 6 (A) IUPred disorder scores of the 200 residues either side of annotated ELM instances. The proportion of proteins with data at a given distance (AA index) from the motif is plotted in grey. (B) IUPred disorder scores of the 200 residues either side of FP instances. The proportion of proteins with data at a given distance (AA index) from the motif is plotted in grey. (C) Binned distribution of mean IUPred disorder scores for defined residues of 1204 annotated motifs. | |
Biological implications
The principal tenet of motif biology is that SLiMs occur most frequently in unstructured regions22 (an observation once again verified in this study), however, the biological significance of the tendency to occur in disordered regions is unclear, though several hypotheses can be suggested. Conditional accessibility is often used as a regulatory mechanism by proteins (e.g. the NES of MAP kinase activated protein kinase 2 (MAPKAPK2)80), and clearly the accessibility inherent to disordered regions is important for motif binding. However, we have also shown that the majority of known ELM classes (∼60%) solved in the complex form secondary structure over at least part of their length upon binding (mirroring observations in larger disordered domains18), suggesting that the flexibility of disordered regions to allow unstructured interfaces to adapt to their interaction partner upon binding is a significant factor. Furthermore, the faster evolutionary rates that are inherent to disordered regions81 increase the likelihood of convergent evolution of a SLiM, likely causing further enrichment in these regions. Fundamentally, it is likely that each hypothesis plays an important role in the preference to occur in intrinsically disordered regions.
Conclusion
Large proportions of the human proteome are intrinsically disordered82,83 and proteome-wide analyses have revealed that disordered regions are enriched in highly connected hub proteins.84–86 Such observations hint at extensive use of compact, natively unstructured interfaces, promoting high functional density and denser wiring of the interactome. As 80% of identified protein–protein interactions are estimated to occur through unknown interfaces87 and two-thirds of the approximately 12
000 domain families lack a known binding partner,88 it is likely that a sizeable proportion of interactions will be mediated by interfaces in disordered regions. Given the prominence of intrinsically disordered proteins in many important facets of cell regulation,89,90 the tight regulation of disordered proteins to insure correct dosage38,91 and the unfortunate outcomes of aberrant functionality,92,93 it is surprising that the molecular details of the mechanisms by which the majority of disordered regions function still remain largely elusive. Despite numerous examples of disordered domains, few have been extensively functionally characterised.46,94 Furthermore, only a small fraction of the estimated cellular SLiM complement has been discovered.95 Many canonical biochemical assays were created to aid the characterisation of classical globular proteins and, despite giant steps in the past decade, experimental methods to interrogate disordered interfaces have only recently “come of age”.6 However, the biological functionality of a motif cannot be elucidated from a single experiment and therefore we advocate the use of multiple complementary techniques in order to define a motif. For example, structural and mutational analysis of motif binding interfaces should, for completeness, be coupled to functional studies.
Computational approaches, which should lead and focus experimental discovery, are in many ways lagging behind the advances of the experimentalists. Though bioinformatics prediction of intrinsically disordered regions should be seen as a mature field with a number of complementary and accurate methods,96 the bioinformatics approaches to discover disordered interfaces (ELM,3 Minimotif,97 Dilimot,95 SLiMFinder,37 Alpha-mOrf,98 Anchor,99 SLiMSearch100) have yet to reveal the expected multitude of novel motif classes and instances. Successes are sporadic, however several analyses have discovered putative functional motifs (SH3-binding,101 EH1-binding102 and KEPE103 motifs). Nevertheless, it is clear that many of the defining attributes of SLiMs have yet to be used to their full potential, specifically the simultaneous utilisation of multiple discriminatory properties. Hopefully, this comprehensive overview of motif properties (a synopsis of results is available in Table S17, ESI‡) will be beneficial to those designing the next generation of bioinformatics tools and will aid in the computational discovery of novel motif instances and classes. More importantly, basic knowledge of the functional and evolutionary properties of SLiMs will undoubtedly improve our comprehension of motif biology and consequently our understanding of the dynamics of protein–protein interactions in general.
References
- H. J. Dyson and P. E. Wright, Nat. Rev. Mol. Cell Biol., 2005, 6, 197 CrossRef CAS.
- P. Tompa, M. Fuxreiter, C. J. Oldfield, I. Simon, A. K. Dunker and V. N. Uversky, BioEssays, 2009, 31, 328 CrossRef CAS.
- C. M. Gould, F. Diella, A. Via, P. Puntervoll, C. Gemund, S. Chabanis-Davidson, S. Michael, A. Sayadi, J. C. Bryne, C. Chica, M. Seiler, N. E. Davey, N. Haslam, R. J. Weatheritt, A. Budd, T. Hughes, J. Pas, L. Rychlewski, G. Trave, R. Aasland, M. Helmer-Citterich, R. Linding and T. J. Gibson, Nucleic Acids Res., 2010, 38, D167 CrossRef CAS.
- D. D. Boehr, R. Nussinov and P. E. Wright, Nat. Chem. Biol., 2009, 5, 789 CrossRef CAS.
- P. D. Jeffrey, S. Gorina and N. P. Pavletich, Science, 1995, 267, 1498 CrossRef CAS.
- P. Tompa, Curr. Opin. Struct. Biol., 2011, 21, 419 CrossRef CAS.
- S. A. Dames, M. Martinez-Yamout, R. N. De Guzman, H. J. Dyson and P. E. Wright, Proc. Natl. Acad. Sci. U. S. A., 2002, 99, 5271 CrossRef CAS.
- T. D. Hurley, J. Yang, L. Zhang, K. D. Goodwin, Q. Zou, M. Cortese, A. K. Dunker and A. A. DePaoli-Roach, J. Biol. Chem., 2007, 282, 28874 CrossRef CAS.
- B. J. Mayer, J. Cell Sci., 2001, 114, 1253 CAS.
- F. Diella, N. Haslam, C. Chica, A. Budd, S. Michael, N. P. Brown, G. Trave and T. J. Gibson, Front. Biosci., 2008, 13, 6580 Search PubMed.
- V. Neduva and R. B. Russell, FEBS Lett., 2005, 579, 3342 CrossRef CAS.
- V. Neduva and R. B. Russell, Curr. Opin. Biotechnol., 2006, 17, 465 CrossRef CAS.
- N. E. Davey, G. Trave and T. J. Gibson, Trends Biochem. Sci., 2011, 36, 159 CrossRef CAS.
- B. T. Kelly and D. J. Owen, Curr. Opin. Cell Biol, 2011, 23(4), 404–412 Search PubMed.
- M. Fabbro and B. R. Henderson, Exp. Cell Res., 2003, 282, 59 Search PubMed.
- A. Castro, C. Bernis, S. Vigneron, J. C. Labbe and T. Lorca, Oncogene, 2005, 24, 314 Search PubMed.
- S. Y. Fuchs, V. S. Spiegelman and K. G. Kumar, Oncogene, 2004, 23, 2028 Search PubMed.
- P. E. Wright and H. J. Dyson, Curr. Opin. Struct. Biol., 2009, 19, 31 CrossRef CAS.
- J. C. Houtman, H. Yamaguchi, M. Barda-Saad, A. Braiman, B. Bowden, E. Appella, P. Schuck and L. E. Samelson, Nat. Struct. Mol. Biol., 2006, 13, 798 Search PubMed.
- T. J. Gibson, Trends Biochem. Sci., 2009, 34, 471 CrossRef CAS.
- V. N. Uversky and A. K. Dunker, Biochim. Biophys. Acta, 2010, 1804, 1231 CAS.
- M. Fuxreiter, P. Tompa and I. Simon, Bioinformatics, 2007, 23, 950 CrossRef CAS.
- H. Dinkel and H. Sticht, Bioinformatics, 2007, 23(24), 3297–3303 CrossRef CAS.
- C. Chica, A. Labarga, C. M. Gould, R. Lopez and T. J. Gibson, BMC Bioinf., 2008, 9, 229 CrossRef.
- N. E. Davey, D. C. Shields and R. J. Edwards, Bioinformatics, 2009, 25, 443 CrossRef CAS.
- A. Via, C. M. Gould, C. Gemund, T. J. Gibson and M. Helmer-Citterich, BMC Bioinf., 2009, 10, 351 Search PubMed.
- C. Chica, F. Diella and T. J. Gibson, PLoS One, 2009, 4, e6052 Search PubMed.
- A. Stein and P. Aloy, PLoS One, 2008, 3, e2524 Search PubMed.
- H. C. Hang, C. Yu, D. L. Kato and C. R. Bertozzi, Proc. Natl. Acad. Sci. U. S. A., 2003, 100, 14846 CrossRef CAS.
- S. Lemeer and A. J. Heck, Curr. Opin. Chem. Biol., 2009, 13, 414 CrossRef CAS.
- S. P. Mirza and M. Olivier, Physiol. Genomics, 2008, 33, 3 Search PubMed.
- J. Muller, D. Szklarczyk, P. Julien, I. Letunic, A. Roth, M. Kuhn, S. Powell, C. von Mering, T. Doerks, L. J. Jensen and P. Bork, Nucleic Acids Res., 2010, 38, D190 Search PubMed.
- S. F. Altschul, W. Gish, W. Miller, E. W. Myers and D. J. Lipman, J. Mol. Biol., 1990, 215, 403 CrossRef CAS.
- R. C. Edgar, BMC Bioinf., 2004, 5, 113 CrossRef.
- A. De Biasio, C. Guarnaccia, M. Popovic, V. N. Uversky, A. Pintar and S. Pongor, J. Proteome Res., 2008, 7, 2496 CrossRef CAS.
- E. Jain, A. Bairoch, S. Duvaud, I. Phan, N. Redaschi, B. E. Suzek, M. J. Martin, P. McGarvey and E. Gasteiger, BMC Bioinf., 2009, 10, 136 CrossRef.
- R. J. Edwards, N. E. Davey and D. C. Shields, PLoS One, 2007, 2, e967 CrossRef.
- T. Vavouri, J. I. Semple, R. Garcia-Verdugo and B. Lehner, Cell, 2009, 138, 198 CrossRef CAS.
- R. B. Jones, A. Gordus, J. A. Krall and G. MacBeath, Nature, 2006, 439, 168 CrossRef CAS.
- J. D. Scott and T. Pawson, Science, 2009, 326, 1220 CrossRef CAS.
- M. Rowicka, A. Kudlicki, B. P. Tu and Z. Otwinowski, Proc. Natl. Acad. Sci. U. S. A., 2007, 104, 16892 Search PubMed.
- A. Bossi and B. Lehner, Mol. Syst. Biol., 2009, 5, 260.
- A. P. Jackson, A. Flett, C. Smythe, L. Hufton, F. R. Wettey and E. Smythe, J. Cell Biol., 2003, 163, 231 Search PubMed.
- S. Honnappa, S. M. Gouveia, A. Weisbrich, F. F. Damberger, N. S. Bhavesh, H. Jawhari, I. Grigoriev, F. J. van Rijssel, R. M. Buey, A. Lawera, I. Jelesarov, F. K. Winkler, K. Wuthrich, A. Akhmanova and M. O. Steinmetz, Cell, 2009, 138, 366 Search PubMed.
- W. Deng, B. Y. Lin, G. Jin, C. G. Wheeler, T. Ma, J. W. Harper, T. R. Broker and L. T. Chow, J. Virol., 2004, 78, 13954 Search PubMed.
- C. W. Lee, J. C. Ferreon, A. C. Ferreon, M. Arai and P. E. Wright, Proc. Natl. Acad. Sci. U. S. A., 2010, 107, 19290 Search PubMed.
- T. Pawson and J. D. Scott, Trends Biochem. Sci., 2005, 30, 286 CrossRef CAS.
- A. K. Varma, R. S. Brown, G. Birrane and J. A. Ladias, Biochemistry, 2005, 44, 10941 Search PubMed.
- T. Shiratori, S. Miyatake, H. Ohno, C. Nakaseko, K. Isono, J. S. Bonifacino and T. Saito, Immunity, 1997, 6, 583 Search PubMed.
- B. Chu, F. Soncin, B. D. Price, M. A. Stevenson and S. K. Calderwood, J. Biol. Chem., 1996, 271, 30847 Search PubMed.
- C. de Chiara, R. P. Menon, M. Strom, T. J. Gibson and A. Pastore, PLoS One, 2009, 4, e8372 Search PubMed.
- K. Gunasekaran, C. J. Tsai, S. Kumar, D. Zanuy and R. Nussinov, Trends Biochem. Sci., 2003, 28, 81 CrossRef CAS.
- P. D. Adams, X. Li, W. R. Sellers, K. B. Baker, X. Leng, J. W. Harper, Y. Taya and W. G. J. Kaelin, Mol. Cell Biol., 1999, 19, 1068 Search PubMed.
- E. Zacksenhaus, R. Bremner, R. A. Phillips and B. L. Gallie, Mol. Cell Biol., 1993, 13, 4588 Search PubMed.
- D. M. Underhill and H. S. Goodridge, Trends Immunol., 2007, 28, 66 Search PubMed.
- L. Radnai, P. Rapali, Z. Hodi, D. Suveges, T. Molnar, B. Kiss, B. Becsi, F. Erdodi, L. Buday, J. Kardos, M. Kovacs and L. Nyitray, J. Biol. Chem., 2010, 285, 38649 Search PubMed.
- H. T. McMahon and I. G. Mills, Curr. Opin. Cell Biol., 2004, 16, 379 Search PubMed.
- D. J. Owen, Y. Vallis, M. E. Noble, J. B. Hunter, T. R. Dafforn, P. R. Evans and H. T. McMahon, Cell, 1999, 97, 805 Search PubMed.
- T. J. Brett, L. M. Traub and D. H. Fremont, Structure, 2002, 10, 797 Search PubMed.
- Z. Dosztanyi, V. Csizmok, P. Tompa and I. Simon, J. Mol. Biol., 2005, 347, 827 CrossRef CAS.
-
M. J. Betts and R. B. Russell, in Bioinformatics for Geneticists, ed. M. R. Barnes and I. C. Gray, John Wiley & Sons Ltd., Chichester, 1st edn, 2003, ch. 14, pp. 289–316 Search PubMed.
- J. C. Biro, Theor. Biol. Med. Model., 2006, 3, 15 Search PubMed.
- V. N. Uversky, J. R. Gillespie and A. L. Fink, Proteins, 2000, 41, 415 CrossRef CAS.
- K. Gunasekaran, C. J. Tsai and R. Nussinov, J. Mol. Biol., 2004, 341, 1327 CrossRef CAS.
- B. Meszaros, P. Tompa, I. Simon and Z. Dosztanyi, J. Mol. Biol., 2007, 372, 549 CrossRef CAS.
- S. Vucetic, C. J. Brown, A. K. Dunker and Z. Obradovic, Proteins, 2003, 52, 573 CrossRef CAS.
- B. K. Kay, M. P. Williamson and M. Sudol, FASEB J., 2000, 14, 231 CAS.
- P. Flicek, M. R. Amode, D. Barrell, K. Beal, S. Brent, Y. Chen, P. Clapham, G. Coates, S. Fairley, S. Fitzgerald, L. Gordon, M. Hendrix, T. Hourlier, N. Johnson, A. Kahari, D. Keefe, S. Keenan, R. Kinsella, F. Kokocinski, E. Kulesha, P. Larsson, I. Longden, W. McLaren, B. Overduin, B. Pritchard, H. S. Riat, D. Rios, G. R. Ritchie, M. Ruffier, M. Schuster, D. Sobral, G. Spudich, Y. A. Tang, S. Trevanion, J. Vandrovcova, A. J. Vilella, S. White, S. P. Wilder, A. Zadissa, J. Zamora, B. L. Aken, E. Birney, F. Cunningham, I. Dunham, R. Durbin, X. M. Fernandez-Suarez, J. Herrero, T. J. Hubbard, A. Parker, G. Proctor, J. Vogel and S. M. Searle, Nucleic Acids Res., 2011, 39, D800 Search PubMed.
- N. E. Davey, R. J. Edwards and D. C. Shields, Nucleic Acids Res., 2007, 35, W455 CrossRef.
- I. Letunic, R. R. Copley and P. Bork, Hum. Mol. Genet., 2002, 11, 1561 Search PubMed.
- P. Tompa, BioEssays, 2003, 25, 847 Search PubMed.
- S. Prinz, E. S. Hwang, R. Visintin and A. Amon, Curr. Biol., 1998, 8, 750 CrossRef CAS.
- Y. Zhang and E. Lees, Mol. Cell Biol., 2001, 21, 5190 Search PubMed.
- R. Gary, D. L. Ludwig, H. L. Cornelius, M. A. MacInnes and M. S. Park, J. Biol. Chem., 1997, 272, 24522 Search PubMed.
- C. S. Tan, C. Jorgensen and R. Linding, Cell Cycle, 2010, 9, 1276 Search PubMed.
- F. Diella, S. Cameron, C. Gemund, R. Linding, A. via, B. Kuster, T. Sicheritz-Ponten, N. Blom and T. J. Gibson, BMC Bioinf., 2008, 5, 79 Search PubMed.
- A. Via, P. F. Gherardini, E. Ferraro, G. Ausiello, G. Scalia Tomba and M. Helmer-Citterich, BMC Bioinf., 2007, 8, 68 Search PubMed.
- W. Kabsch and C. Sander, Biopolymers, 1983, 22, 2577 CrossRef CAS.
- R. D. Finn, J. Mistry, J. Tate, P. Coggill, A. Heger, J. E. Pollington, O. L. Gavin, P. Gunasekaran, G. Ceric, K. Forslund, L. Holm, E. L. Sonnhammer, S. R. Eddy and A. Bateman, Nucleic Acids Res., 2010, 38, D211–D222 CrossRef CAS.
- W. Meng, L. L. Swenson, M. J. Fitzgibbon, K. Hayakawa, E. Ter Haar, A. E. Behrens, J. R. Fulghum and J. A. Lippke, J. Biol. Chem., 2002, 277, 37401 Search PubMed.
- C. J. Brown, S. Takayama, A. M. Campen, P. Vise, T. W. Marshall, C. J. Oldfield, C. J. Williams and A. K. Dunker, J. Mol. Evol., 2002, 55, 104 CrossRef CAS.
- J. J. Ward, J. S. Sodhi, L. J. McGuffin, B. F. Buxton and D. T. Jones, J. Mol. Biol., 2004, 337, 635 CrossRef CAS.
- M. Sickmeier, J. A. Hamilton, T. LeGall, V. Vacic, M. S. Cortese, A. Tantos, B. Szabo, P. Tompa, J. Chen, V. N. Uversky, Z. Obradovic and A. K. Dunker, Nucleic Acids Res., 2007, 35, D786 CrossRef CAS.
- A. K. Dunker, M. S. Cortese, P. Romero, L. M. Iakoucheva and V. N. Uversky, FEBS J., 2005, 272, 5129 CrossRef CAS.
- Z. Dosztanyi, J. Chen, A. K. Dunker, I. Simon and P. Tompa, J. Proteome Res., 2006, 5, 2985 CrossRef CAS.
- C. Haynes, C. J. Oldfield, F. Ji, N. Klitgord, M. E. Cusick, P. Radivojac, V. N. Uversky, M. Vidal and L. M. Iakoucheva, PLoS Comput. Biol., 2006, 2, e100 CrossRef.
- B. Schuster-Bockler and A. Bateman, BMC Bioinf., 2007, 8, 259 CrossRef.
- A. Stein, A. Ceol and P. Aloy, Nucleic Acids Res., 2011, 39, D718 Search PubMed.
- L. M. Iakoucheva, P. Radivojac, C. J. Brown, T. R. O'Connor, J. G. Sikes, Z. Obradovic and A. K. Dunker, Nucleic Acids Res., 2004, 32, 1037 CrossRef CAS.
- M. Fuxreiter, P. Tompa, I. Simon, V. N. Uversky, J. C. Hansen and F. J. Asturias, Nat. Chem. Biol., 2008, 4, 728 CrossRef CAS.
- J. Gsponer, M. E. Futschik, S. A. Teichmann and M. M. Babu, Science, 2008, 322, 1365 CrossRef CAS.
- M. M. Babu, R. van der Lee, N. S. de Groot and J. Gsponer, Curr. Opin. Struct. Biol., 2011, 21, 432 CrossRef CAS.
- K. Kadaveru, J. Vyas and M. R. Schiller, Front. Biosci., 2008, 13, 6455 Search PubMed.
- C. A. Galea, Y. Wang, S. G. Sivakolundu and R. W. Kriwacki, Biochemistry, 2008, 47, 7598 CrossRef CAS.
- V. Neduva, R. Linding, I. Su-Angrand, A. Stark, F. d. D. Masi, T. J. J. Gibson, J. Lewis, L. Serrano and R. B. B. Russell, PLoS Biol., 2005, 3, e405 CrossRef.
- B. He, K. Wang, Y. Liu, B. Xue, V. N. Uversky and A. K. Dunker, Cell Res., 2009, 19, 929 CrossRef CAS.
- S. Rajasekaran, S. Balla, P. Gradie, M. R. Gryk, K. Kadaveru, V. Kundeti, M. W. Maciejewski, T. Mi, N. Rubino, J. Vyas and M. R. Schiller, Nucleic Acids Res., 2009, 37, D185 CrossRef CAS.
- Y. Cheng, C. J. Oldfield, J. Meng, P. Romero, V. N. Uversky and A. K. Dunker, Biochemistry, 2007, 46, 13468 CrossRef CAS.
- B. Meszaros, I. Simon and Z. Dosztanyi, PLoS Comput. Biol., 2009, 5, e1000376 CrossRef.
- N. E. Davey, N. J. Haslam, D. C. Shields and R. J. Edwards, Nucleic Acids Res., 2011, 39, W56 CrossRef CAS.
- P. Beltrao and L. Serrano, PLoS Comput. Biol., 2005, 1, e26 Search PubMed.
- R. R. Copley, BMC Genomics, 2005, 6, 169 Search PubMed.
- F. Diella, S. Chabanis, K. Luck, C. Chica, C. Ramu, C. Nerlov and T. J. Gibson, Bioinformatics, 2009, 25, 1 Search PubMed.
- P. H. Kussie, S. Gorina, V. Marechal, B. Elenbaas, J. Moreau, A. J. Levine and N. P. Pavletich, Science, 1996, 274, 948 CrossRef CAS.
- J. Yan, L. Pan, X. Chen, L. Wu and M. Zhang, Proc. Natl. Acad. Sci. U. S. A., 2010, 107, 4040 Search PubMed.
Footnotes |
† Published as part of a Molecular BioSystems themed issue on Intrinsically Disordered Proteins: Guest Editor M. Madan Babu. |
‡ Electronic supplementary information (ESI) available. See DOI: 10.1039/c1mb05231d |
|
This journal is © The Royal Society of Chemistry 2012 |
Click here to see how this site uses Cookies. View our privacy policy here.