DOI:
10.1039/C0SC00487A
(Edge Article)
Chem. Sci., 2011,
2, 439-445
Discrimination of flavonoids and red wine varietals by arrays of differential peptidic sensors†
Received
15th September 2010
, Accepted 18th October 2010
First published on 26th November 2010
Abstract
The chemical structures and concentrations of an organism's natural products are dependent upon its genome and environmental factors. Examples are the complex metabolite solutions resulting from plant and fermentation processes. Here, we describe sensor arrays composed of supramolecular ensembles that undergo indicator displacement and discriminate selected flavonoids and mixtures thereof: wine varietals. Changes in UV-vis absorbance upon indicator displacement in the array were analyzed using pattern recognition protocols. The flavonoids were differentiated in terms of structure and concentration, while red wines were generally classified by varietals, even from different vintners. The technique highlights the power of differential sensor arrays to classify mixtures by metabolite distribution, even when the natural products are not known.
Introduction
Complex mixtures are ubiquitous in many fields of chemistry and biology, and hence, complex mixture analysis is common in pharmaceutical sciences, medical diagnostics, the food industry, as well as environmental sciences. For instance, progress in drug discovery depends highly on the ability to effectively determine bioactive compounds,1 detect toxicity,2 determine protein targets,3,4 identify biomarkers,5 and perform metabolic profiling6–9 in complex mixtures. Sample authentication and quality assurance of medicine,10 as well as food samples such as wines,11 artificial sweeteners,12 energy drinks,13 honey,14 and even olive oil,15,16 require complex mixture analysis. Analyses in these cases employ classical analytical techniques, such as HPLC, mass spectrometry, electrochemistry, UV-vis spectrometry, as well as hyphenated modes of these analytical methods.
Various analyses of complex mixtures have also involved differential or array sensing,17–24 which is gaining interest as an alternative approach to traditional, one-dimensional chemical analysis. The technique is inspired by the senses of taste and smell, where arrays of sensors create specific patterns of signals.17 The beauty of differential sensing stems from the fact that the individual sensors of the array need not be specific, but should instead be cross reactive and able to interact variably with the analytes. Differential sensing is employed in electronic noses,19,25 which are used in the analysis of gaseous analytes or aromas, and electronic tongues,26–29 for the analysis of liquid samples. These sensor arrays employ a variety of sensor–analyte responses such as electrochemical and optical signal transduction mechanisms, depending on the type of sensor employed: conducting polymers,27 chemoresistive metal oxides, and optical fibers.28 Other types of sensor arrays employ chemosensors,30 which also have varying signal transduction mechanisms. Such arrays have been employed as physically addressable sensors in solution,31–34 in immobilized wells or pits,35–40 or as receptors mixed all together in one solution (dynamic combinatorial libraries).41–47 It is clear that the power of differential sensing derives from a combination of various types of sensors that can take advantage of a range of response mechanisms.
Wine is a complex mixture of natural products arising from grape and fermentation, giving wine its interesting organoleptic property.48 Numerous factors contribute to its chemical diversity.49 The metabolites found in wine are dictated not only by the grape's genomic properties, but also by the strain of yeast used in fermentation. Viticultural practices, the environment where the grapes are grown, and oak cooperage50 can also contribute to the compounds found in wine. One of the major components of red wines is a group of polyphenolic compounds derived mainly from the skin of the grape called tannins, which are oligomers of flavonoids and nonflavonoids.51 Their structures are primarily dictated by the grape's biochemistry, making this class of compounds diagnostic for differences among wine varietals.48 Further, because not all tannins in red wines have been characterized, wine represents an excellent test-bed to prove the principle of differential sensing in complex mixtures consisting of subtly different and unknown analyte structures, and thereby demonstrates the partial fingerprinting of an organism's metabolome.
Previous studies on discrimination and analysis of wines apply chemometric analysis of data from IR,52–54GC-MS,55 and NMR56 as well as data from analyses using electronic noses,57–60electronic tongues,61–65 and a combination of these methods.66,67 The sensor arrays used in electronic noses and tongues mostly employed sensing elements that use electrochemical methods, and involve fabrication of electrodes. Also, sample preparation such as solid phase extraction prior to the analysis of wines is required to avoid the effects of water and ethanol.60 Herein, we present how an array of peptidic, colorimetric sensors discriminates flavonoids, which are hydrolysates of tannins found in wines. We then evaluate the array of chemosensors by classifying red wine varietals and red wines of the same varietals from different vintners (not necessarily of different origins) to illustrate fingerprinting similar metabolites that stem from a common genome. We use differential sensing that employs physically addressable supramolecular ensembles of metals, indicators, and peptides as sensing elements that function viaindicator displacement in solutions under ethanolic conditions; hence, a laborious sample preparation other than filtration is also not required. Moreover, the basis of discrimination is the differential binding of analytes to arrays of sensors, rather than the analytes' structural properties as measured by spectroscopy or electrochemistry.
Results and discussion
Our group routinely uses sensors and sensing ensembles made of fully designed and combinatorially derived receptors,35–37,68–70 coupled with indicator displacement assays71–73 for discriminating various analytes. In this study, the individual sensing ensembles in the arrays were ternary complexes of commercially available colorimetric indicators, metal ions, and histidine-rich peptides of random sequence or previously known sequences (1–10, Fig. 1A).74–77 Upon addition of a flavonoid or wine to the ensemble, the indicator is displaced, resulting in a change of color.
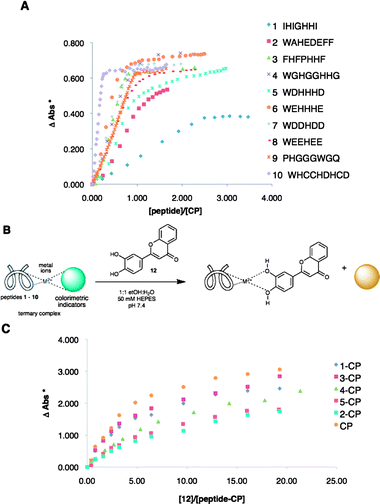 |
| Fig. 1 (A) Binding isotherms from the titration of 1 : 1 CP complex with peptides 1 to 10. (B) Indicator displacement of 12 using peptide–CP complexes. (C) Binding curves from the titration of peptide–CP complexes with 12. (* ΔAbs – change in absorbance at 444 nm). | |
Construction and optimization of sensing ensembles
The sensing ensembles were optimized individually by obtaining binding curves from the titration of a solution of the metal and the indicator with a solution of a peptide. Both solutions were buffered by HEPES at pH 7.4. For the optimization of ensembles containing Cu(II) and pyrocatechol violet (PCV) (this metal–indicator pair is referred to as CP from hereon), a 1
:
1 solution of CP was titrated with a solution of a peptide (Fig. 1A).
The peptides showed variable binding stoichiometries and affinities. From these binding curves, the compositions of the sensing ensembles were determined. Ternary complexes of peptide–metal–indicator will exist at half an equivalence of the amount of peptide that it takes to completely displace the indicator from CP. Hence, these specific ratios of peptide–metal–indicator (ESI†, Table S4) were used in the indicator displacement assays and eventually, in the array sensing of flavonoids (vide infra). The ternary complexes of peptides with CP, as well as the binary 1
:
1 CP complex alone, were used in indicator displacement assays using flavonoid 12. Saturation was seen upon addition of 12 to each of the sensing ensembles (Fig. 1C). Other flavonoids were also tested and as expected, differences in the extent of displacement were seen among the different ensembles (ESI†, Fig. S15, S16).
To evaluate the ability of arrays of sensing ensembles described to discriminate analytes, flavonoids 11 to 15 (Fig. 2A) were analyzed using an array composed of ternary complexes of peptides 3 to 10 with CP.78 The 1
:
1 complex of CP (no peptide) was also included in this array (referred to as array X). The flavonoids were evaluated at two concentrations: 0.060 and 0.12 mM (eight replicates each). The concentrations of Cu(II) and PCV were constant while that of the peptides varied according to the ratios obtained from the optimization experiments (ESI†, Table S4). Changes in the absorbance values at the λmax of PCV at pH 7.4 (444 nm) for each member of the sensor array upon addition of the flavonoids were recorded, pooled, and analyzed using principal component analysis (PCA), which gives a statistical summary of the response data.79 A PCA score plot shows groupings in the observations, and captures decreasing amounts of variance in each succeeding dimension. A loading plot expresses the relative contributions of the original variables to the model. In the analysis of our data, the observations are the flavonoids, while the variables are the individual sensing ensembles, which give different values of change in absorbance at 444 nm. The resulting score plot (Fig. 2B) showed discrimination of flavonoids tested at the two aforementioned concentrations. The first principal component, F1, separated the flavonoids of the same identity primarily according to concentration. The basis of separation along F2, on the other hand, was not definitive, although concentration also played a small part. We also observed that the substituted flavonoids 11 and 15 were classified in opposite areas along F2, while in general, underivatized flavonoids were classified in between the derivatized flavonoids along this axis. Flavonoids 12 and 14 have very similar structures and were only differentiated along F1.
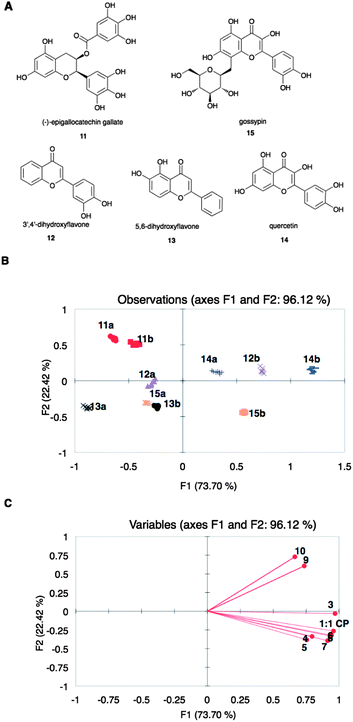 |
| Fig. 2 (A) Structures of flavonoids 11 to 15. (B) PCA score plot and (C) loading plot of the spectroscopic data from the displacement of array X by 11 to 15 at 0.06 (a) and 0.12 (b) mM. Points on the loading plot are individual members of array X, represented by peptide number. | |
According to the loading plot (Fig. 2C), the flavonoids that are found on the right side of the score plot (Fig. 2B) displaced PCV in all the sensing ensembles to a higher extent than those flavonoids found on the left side of the score plot. On the other hand, the loading plot indicated that peptides 4, 5, 6, 7, and 8 did not vary significantly in response to the flavonoids while 3 and the set of 9 and 10 were significantly different.
Discrimination of red wine varietals
The successful differentiation of the flavonoids by a statistical pattern recognition of the responses from our array of sensors encouraged us to turn our attention to wines, which are known to contain relatively high concentrations of flavonoids (about 100 mg L−1 or 0.2 mM),51 comparable to the concentrations used in the previous analysis of flavonoids. Although wines contain other types of polyphenols, and other compounds that can potentially bind to our sensing ensembles, individual examples of commercially obtained wines of different varietals were analyzed using a similar sensor array. Using peptidic ensembles of 1
:
1 CP with peptides 1 to 9 (array Y),80 different red wine varietals (Pinot Noir, Zinfandel, Beaujolais, Cabernet Sauvignon, Shiraz, and Merlot)81 were analyzed by a method similar to that used for the flavonoids. However, prior to the determination of changes in absorbance of the sensing ensembles of array Y at 444 nm upon addition of the wines, the concentration of wines to use was first determined. At pH 7.4, the anthocyanins in wine, which are responsible for its red color, essentially do not absorb at or around 444 nm.82 This was ascertained nevertheless by obtaining the absorbance spectra at pH 7.4 of the different wines in the visible range (ESI†, Fig. S17).
An indicator displacement assay was performed with a wine varietal (Merlot),83 using 1
:
1 CP as the sensing ensemble. The corresponding binding curve obtained (ESI†, Fig. S18) showed that at 1.0% (v/v) wine, there was partial displacement of PCV. The assays of different wines using array Y were then performed at 1.0% (v/v) wine concentration. At this concentration, the wine has minimal contribution to the absorbance of the mixture with the sensing ensemble. However, all absorbance readings during the assays were corrected for the absorbance of the wines. PCA of the absorbance data obtained from array Y at 444 nm with different wine varietals did not show satisfactory discrimination (ESI†, Fig. S20). Linear discriminant analysis (LDA) was then performed on this data set. Compared to PCA, LDA is a supervised pattern recognition protocol, which by virtue of the algorithm, improves clustering. Although complete classification was still not found (Fig. 3A), we used this same array to analyze different brands of one wine varietal, Shiraz.84 The data from this assay were combined with the data obtained from the analysis of different wine varietals. Scores belonging to Shiraz were found mostly in one area of the LDA plot (Fig. 3B) and therefore, even this unoptimized array showed the potential to cluster together a varietal.
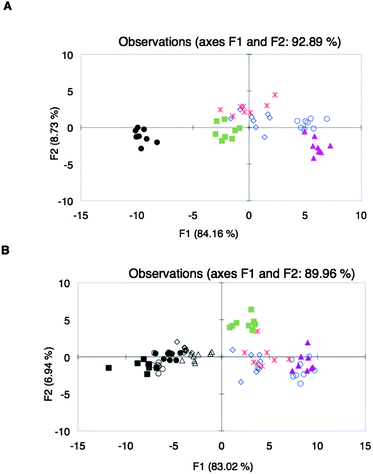 |
| Fig. 3
LDA plots of spectroscopic data from the analysis of (A) different wine varietals: Beaujolais ( ), Zinfandel ( ), Pinot Noir ( ), Shiraz (●), Merlot ( ), Cabernet Sauvignon ( ); (B) different Shiraz wines: Shiraz Ba (○), Shiraz Rw (◇), Shiraz Ma (■), Shiraz RT (△), Shiraz St (●); and other wine varietals: Beaujolais ( ), Zinfandel ( ), Pinot Noir ( ), Merlot ( ), Cabernet Sauvignon ( ), using sensor array Y. | |
The effect of other metal–indicator pairs in the array was explored in an attempt to increase the diversity of the array as a means to improve the discrimination of the wines. Metal–indicator pairs explored in the past by our group, Ni(II)–bromopyrogallol red (BPR) and Cu(II)–chromeazurol S (CAS) complexes (referred to as NB and CC, respectively), were mixed with peptides 3 and 8, which were randomly chosen among the available peptides. Optimal concentration ratios of the metal–indicator pairs with the peptides were then determined as before (ESI†, Table S4). Array Z, composed of six peptidic ensembles, was used to analyze the same set of wine varietals as those used for Array Y, but at three different wavelengths: 430, 444, and 560 nm, which are the corresponding λmax of the indicators at pH 7.4. LDA plots of the spectroscopic data showed excellent discrimination of the wine varietals (Fig. 4A). The loading plot from the analysis showed a widely differential set of sensing ensembles, as they are found in all the quadrants of the plot (Fig. 4B).
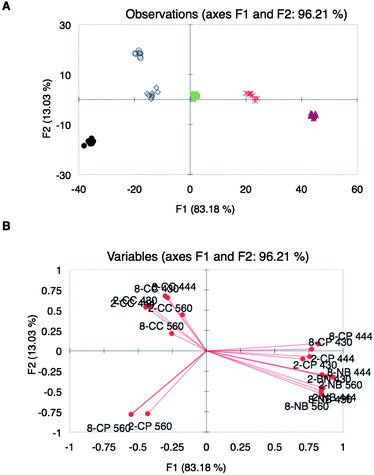 |
| Fig. 4
UV-vis response using array Z: (A) LDA score plot of UV-vis responses from different wine varietals: Beaujolais ( ), Zinfandel ( ), Pinot Noir ( ), Shiraz (●), Merlot ( ), Cabernet Sauvignon ( ). (B) Corresponding variable correlation plot: first numbers correspond to the peptide, letters are the metal–indicator pairs, and last numbers correspond to the wavelengths at which the absorbance was taken. | |
As our final test for whether our approach can classify wines of the same varietals, different brands of Zinfandel85 instead of Shiraz were analyzed together with the other five varietals. The LDA plot showed clustering of most of the Zinfandel wines, but with separation of the other wine varietals (Fig. 5). The discriminant scores of the varietals were increasingly distinct compared to the discriminant scores using array Y (Fig. 3B). The exceptions to the clustering of the Zinfandels were SV and BT (Fig. 5), although it can be seen that both of these wines were only different in either F1 or F2 relative to the discriminant scores of the other Zinfandels. We speculate that these outlying Zinfandels arise from differences in viticultural practices.
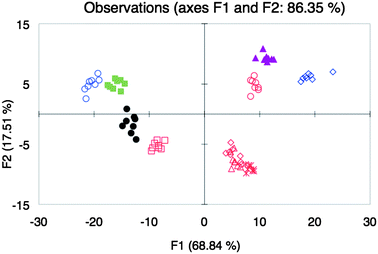 |
| Fig. 5
LDA plot of the response from different wine varietals Beaujolais ( ), Pinot Noir ( ), Shiraz (●), Merlot ( ), Cabernet Sauvignon ( ) and different brands of Zinfandel wines BS ( ), BT ( ), C ( ), R ( ), and SV ( ) with array Z. | |
Conclusions
In summary, we have shown that a simple array of peptides, metals, and indicators can fingerprint wines, and with some outliers classify them by their varietal irrespective of the vintner. Our approach targeted the various flavonoids, even though the exact structures and concentrations of the flavonoids were not known. In this manner, a single signature for the broad group of metabolites was derived, and that signature was found to generally correlate with the varietal, which in turn means it is correlated to the genome of the grape. Such an analysis of a class of metabolites bodes well for analogous metabolite fingerprinting in other complex mixtures.
Experimental
General information
All materials for Fmoc solid phase peptide synthesis such as Fmoc-L-amino acids, PyBOP or HBTU, and pre-loaded Wang resins were used without further purification. Reagent grade N,N-dimethylformamide, trifluoroacetic acid, piperidine, and N,N-diisopropylethylamine, thioanisole, triisopropyl silane, CAS (68%), PCV, and BPR were also used without purification. Gossypin, 3′,4′-dihydroxyflavone, and 5′,6′-dihydroxyflavone were purchased from Indofine while (−)-epigallocatechin gallate and quercetin dihydrate were obtained from Sigma-Aldrich. Deionized water was used in all assays. Wines were all purchased from the local grocery store, HEB. Stock solutions of wines were prepared by filtering the wines through 45 μm filter disks and dissolving the filtered wines in 50 mM HEPES in 1
:
1 ethanol–water pH 7.4 to make 6% (v/v) solutions. Statistical analyses were done using XLSTAT (PCA and LDA).
Syntheses of peptides were done using an automated peptide synthesizer (Prelude by Protein Technologies, Inc.), starting with the appropriate pre-loaded Wang resins. Coupling reactions used 5 eq. of Fmoc-L-amino acid, 5 eq. of PyBOP or HBTU, and 10 eq. of DIPEA, relative to 1 eq. of pre-loaded resin. The crude products were analyzed by reverse phase HPLC, using a Waters YMC™ ODS-AQ C18 column (4.6 × 150 mm, 5 μm) at a flow rate of 1 mL min−1, linear gradient of 5 to 90% B (A: 0.1% TFA in water; B: 0.1% TFA in acetonitrile) for 10 min (ESI†, Fig. S1–S10). A few peptides were deemed appropriate to use for the succeeding assays after HPLC analysis showed a relatively pure sample. Otherwise, the peptides were purified by solid phase extraction and preparative HPLC using a Waters Symmetry300™ C18 column (19 × 150 mm, 5 μm). Yields and MS spectra of peptides were obtained (ESI†, Table S1).
Construction of peptide–metal–indicator ensembles
Binding assays between the metal ion and the indicator were done prior to the optimization of the sensing ensemble (peptide–metal–indicator complexes). For example, for Cu(II) and PCV (ESI†, Table S1), 2.0 mL of 0.075 mM PCV in 50 mM HEPES (in 1
:
1 ethanol–water (v/v), pH 7.4) in a septum-capped glass cuvette (Starna cells) was titrated in increments of at least 5 μL, using a 10 μL microsyringe, with a solution of 0.75 mM CuSO4, and 0.075 mM PCV in the same buffer. The spectra at 380 to 750 nm of the solution were obtained after each titration. The absorbance values at the maximum wavelength of final solution in the cuvette, 624 nm, were obtained and the changes in absorbance at this wavelength were determined at each titration. A binding curve, the plot of the change in absorbance at 624 nm against the ratio of the concentrations of Cu(II) and PCV, was obtained to determine the ratio of Cu(II) to PCV to be used in the subsequent binding assays with peptides.
To determine the concentration ratios of peptides to metal–indicator pairs, binding assays with peptides were done. A solution of the metal–indicator complex in 50 mM HEPES (in 1
:
1 ethanol–water (v/v), pH 7.4) was titrated with a solution of peptide, which also contained the metal–indicator complex in the same HEPES buffer (ESI†, Table S3). The spectra of the solution were obtained at each titration. The changes in absorbance at the wavelength of maximum absorbance of the indicator at pH 7.4 (444 nm for PCV, 430 nm for CAS, and 560 nm for BPR) were obtained and plotted against the ratio of the concentration of the peptide to that of the metal–indicator complex. The ratio at half saturation point was chosen as the optimal concentration of the species in the ensemble, for use in the succeeding metal-complexing indicator displacement assays (ESI†, Table S4).
To examine the displacement of indicators from the ternary ensembles by standard flavonoids, indicator displacement assays were done, which were performed in cuvettes or well plates. While holding the concentrations of all species in the ternary ensemble (ESI†, Table S4) constant, the concentrations of the flavonoids were varied from 0 to 1.5 mM. This range was adjusted according to how strongly the flavonoids displaced the indicators from the sensing ensemble.
Discrimination of flavonoids with an array of peptidic sensing ensembles
The spectroscopic data for the discrimination of flavonoids were obtained at 0, 0.060, and 0.12 mM flavonoids, following the optimal concentrations of all components of the sensing ensemble (ESI†, Table S4). The array of sensing ensembles was composed of PCV–Cu(II) and PCV–Cu(II) complexed with peptides 3 to 10 (array X). The well plate reader was blanked with 50 mM HEPES in 1
:
1 ethanol–water (v/v), pH 7.4. The differences in absorbance values of the assay mixtures with and without flavonoids at 444 nm were calculated. Seven repetitions were done. The spectroscopic data were then analyzed by PCA.
General procedure for the assays of wines with peptidic sensor arrays Y and Z
Following the optimal composition of the sensing ensembles (ESI†, Table S4), assays with wines were done at 50 mM HEPES, pH 7.4, in 1
:
1 ethanol–water (v/v) using 96-well plates. The well plate reader was blanked with the same buffer. Absorbance values of assay mixtures were obtained at 444 nm for arrays using only PCV–Cu (array Y) and at 444, 430, and 560 nm for the arrays that included PCV–Cu(II), CAS–Cu(II) and BPR–Ni(II) (array Z). Eight repetitions were done in each assay. The differences in absorbance values between the assay mixture with 1% wine (v/v) and that of 1% wine (v/v) in 50 mM HEPES pH 7.4, in 1
:
1 ethanol–water (v/v) were calculated and the data were analyzed by PCA and LDA.
Acknowledgements
We thank the Freshman Research Initiative of the College of Natural Sciences at UT Austin, and acknowledge assistance from Jennifer Truong and Carolyne Smith during the initial optimization of sensing ensembles. We also acknowledge the National Science Foundation (Grant CHE-0716049) for the support of this work, as well as the Welch Foundation (F-1151).
Notes and references
- S.-Y. Shi, Y.-P. Zhang, X.-Y. Jiang, X.-Q. Chen, K.-L. Huang, H.-H. Zhou and X.-Y. Jiang, Trends Anal. Chem., 2009, 28, 865–877 CrossRef CAS.
- T. M. D. Ebbels, H. C. Keun, O. P. Beckonert, M. E. Bollard, J. C. Lindon, E. Holmes and J. K. Nickolson, J. Proteome Res., 2007, 6, 4407–4422 CrossRef CAS.
- G. M. West, C. L. Tucker, T. Xu, S. K. Park, X. Han, J. R. Yates and M. C. Fitzgerald, Proc. Natl. Acad. Sci. U. S. A., 2010, 107, 9078–9082 CrossRef CAS.
- M. M. Reddy and T. Kodadek, Proc. Natl. Acad. Sci. U. S. A., 2005, 102, 12672–12677 CrossRef.
- A. Sreekumar, L. M. Poisson, T. M. Ragendiran, A. P. Khan, Q. Cao, J. Yu, B. Laxman, R. Mehra, R. J. Lonigro, L. Yong, M. K. Nyati, A. Ahsan, S. Kalyana-Sundaram, B. Han, X. Cao, J. Byun, G. S. Omenn, D. Ghosh, S. Pennathur, D. C. Alexander, A. Berger, J. R. Shuster, J. T. Wei, S. Varambally, C. Beecher and A. M. Chinnaiyan, Nature, 2009, 457, 910–914 CrossRef CAS.
- R. Goodacre, Metabolomics, 2005, 1, 1–2 CrossRef CAS.
- W. J. Griffiths, T. Koal, Y. Wang, M. Kohl, D. P. Enot and H.-P. Deigner, Angew. Chem., Int. Ed., 2010, 49, 5426–5445 CrossRef CAS.
-
J. C. Lindon, J. K. Nickolson and E. Holmes, The handbook of metabonomics and metabolomics, 1st edn, Elsevier Science, Burlington, 2007 Search PubMed.
- K. K. Pasikanti, K. Esuvanranathan, P. C. Ho, R. Mahendran, R. Kamaraj, Q. H. Wu, E. Chiong and E. C. Y. Chan, J. Proteome Res., 2010, 9, 2988–2995 CrossRef CAS.
- Y. Ni, Y. Peng and S. Kokot, Anal. Chim. Acta, 2008, 616, 19–27 CrossRef CAS.
- N. Ogrinc, I. J. Kosir, J. E. Spangenberg and J. Kidric, Anal. Bioanal. Chem., 2003, 376, 424–430 CAS.
- A. Zygler, A. Wasik and J. Namlesnik, Trends Anal. Chem., 2009, 28, 1082–1102 CrossRef CAS.
- Y. B. Monakhova, S. P. Mushtakova, S. S. Kolesnikova and S. A. Astakhov, Anal. Bioanal. Chem., 2010, 397, 1297–1306 CrossRef CAS.
- D. Bertelli, M. Lolli, G. Papotti, L. Bortolotti, G. Serra and M. Plessi, J. Agric. Food Chem., 2010, 58, 8495–8501 CrossRef CAS.
- M. H. Gordon, C. Covell and N. Kirsch, J. Am. Oil Chem. Soc., 2001, 78, 621–624 CrossRef CAS.
- S. Vichi, L. Pizzale, E. Toffano, R. Bortolomeazzi and C. Lanfranco, AOAC J., 2001, 84, 1534–1541 Search PubMed.
- K. J. Albert, N. S. Lewis, C. L. Schauer, G. A. Sotzing, S. E. Stitzel, T. P. Vaid and D. R. Walt, Chem. Rev., 2000, 100, 2595–2696 CrossRef CAS.
- J. J. Lavigne and E. V. Anslyn, Angew. Chem., Int. Ed., 2001, 40, 3118–3130 CrossRef.
- K. S. Suslick, MRS Bull., 2004, 720–725 CAS.
- A. T. Wright and E. V. Anslyn, Chem. Soc. Rev., 2006, 35, 14–28 RSC.
- F. Winquist, C. Kratz-Rulcker and I. Lundstrom, MRS Bull., 2004, 726–731 CAS.
- B. E. Collins, A. T. Wright and E. V. Anslyn, Top. Curr. Chem., 2007, 277, 181–218 CAS.
- E. V. Anslyn, J. Org. Chem., 2007, 72, 687–699 CrossRef.
- G. Wittstock, Anal. Bioanal. Chem., 2002, 372, 16–17 CrossRef CAS.
- S. Capone, C. Distante, L. Francioso, D. Presicce, A. M. Taurino, P. Siciliano and M. Zuppa, Anal. Asoc. Quím. Argentina, 2005, 93, 123–135 Search PubMed.
- C. Krantz-Rulcker, F. Winquist and I. Lundström, Anal. Asoc. Quím. Argentina, 2005, 93, 105–114 Search PubMed.
- U. Lange, N. V. Roznyatovskaya and V. M. Mirsky, Anal. Chim. Acta, 2008, 614, 1–26 CrossRef CAS.
- M. J. Aernecke and D. R. Walt, Sens. Actuators, B, 2009, 142, 464–469 CrossRef.
- P. Ciosek and W. Wroblewski, Analyst, 2007, 132, 963–978 RSC.
- T. W. Bell and N. M. Hext, Chem. Soc. Rev., 2004, 33, 589–598 RSC.
- L. Baldini, A. J. Wilson, J. Hong and A. D. Hamilton, J. Am. Chem. Soc., 2004, 126, 5656–5657 CrossRef CAS.
- N. T. Green and K. D. Shimizu, J. Am. Chem. Soc., 2005, 127, 5695–5700 CrossRef CAS.
- M. A. Palacios, Z. Wang, V. A. Montes, G. V. Zyryanov and P. J. Anzenbacher, J. Am. Chem. Soc., 2008, 130, 10307–10314 CrossRef CAS.
- R. B. C. Jagt, R. F. Gomez-Biagi and M. Nitz, Angew. Chem., Int. Ed., 2009, 48, 1995–1997 CrossRef CAS.
- N. Y. Edwards, T. W. Sager, J. T. McDevitt and E. V. Anslyn, J. Am. Chem. Soc., 2007, 129, 13575–13583 CrossRef CAS.
- A. T. Wright, M. J. Griffin, Z. Zhenlin, S. C. McCleskey, E. V. Anslyn and J. T. McDevitt, Angew. Chem., Int. Ed., 2005, 44, 6375–6378 CrossRef CAS.
- A. T. Wright, E. V. Anslyn and J. T. McDevitt, J. Am. Chem. Soc., 2005, 127, 17405–17411 CrossRef CAS.
- S. C. McCleskey, P. N. Floriano, S. L. Wiskur, E. V. Anslyn and J. T. McDevitt, Tetrahedron, 2003, 59, 10089–10092 CrossRef CAS.
- A. Goodey, J. J. Lavigne, S. Savoy, M. D. Rocdriguez, T. Curey, A. Tsao, G. Simmons, J. Wright, S.-J. Yoo, Y. Sohn, E. V. Anslyn, J. B. Shear, D. Niekirk and J. T. McDevitt, J. Am. Chem. Soc., 2001, 123, 2559–2570 CrossRef CAS.
- J. J. Lavigne, S. Savoy, M. B. Clevenger, J. E. Ritchie, B. McDoniel, S.-J. Yoo, E. V. Anslyn, J. T. McDevitt, J. B. Shear and D. Niekirk, J. Am. Chem. Soc., 1998, 120, 6429–6430 CrossRef CAS.
- A. Buryak, A. Pozdnoukhov and K. Severin, Chem. Commun., 2007, 2366–2368 RSC.
- F. Zaubitzer, T. Riss-Johannessen and K. Severin, Org. Biomol. Chem., 2009, 7, 4598–4603 RSC.
- S. Rochat, J. Gao, X. Qian, F. Zaubitzer and K. Severin, Chem.–Eur. J., 2010, 16, 104–113 CrossRef CAS.
- P.-K. Muller-Graff, H. Szelke, K. Severin and R. Kramer, Org. Biomol. Chem., 2010, 8, 2327–2480 RSC.
- D. Margulies and A. D. Hamilton, Angew. Chem., Int. Ed., 2009, 48, 1771–1774 CrossRef CAS.
- A. Buryak and K. Severin, J. Comb. Chem., 2006, 8, 540–543 CrossRef CAS.
- S. L. Wiskur, P. N. Floriano, E. V. Anslyn and J. T. McDevitt, Angew. Chem., Int. Ed., 2003, 42, 2070–2072 CrossRef CAS.
-
R. S. Jackson, Wine science: principles and applications, 3rd edn, Academic Press, Burlington, 2008 Search PubMed.
- S. T. Lund and J. Bohlmann, Science, 2006, 311, 804–805 CrossRef.
- R. D. Gougeon, M. Lucio, M. Frommberger, D. Peyron, D. Chassagne, H. Alexandre, F. Feuillat, A. Voilley, P. Cayot, I. Gebefugi, N. Hertkorn and P. Schmitt-Kopplin, Proc. Natl. Acad. Sci. U. S. A., 2009, 106, 9174–9179 CrossRef CAS.
-
P. Ribereau-Gayon, Y. Glories, A. Maujean and D. Dubourdieu, The Handbook of Enology: The Chemistry of Wine: Stabilization and Treatments, 2nd edn, John Wiley & Sons, West Sussex, 2006 Search PubMed.
- Y.-l. Zhang, J.-b. Chen, Y. Lei, Q. Zhou, S.-q. Sun and I. Noda, J. Mol. Struct., 2010, 974, 144–150 CrossRef CAS.
- K. Fernandez and E. Agosin, J. Agric. Food Chem., 2007, 55, 7294–7300 CrossRef CAS.
- A. Edelmann and B. Lendl, J. Am. Chem. Soc., 2002, 124, 14741–14747 CrossRef CAS.
- R. Lopez, M. Aznar, J. Cacho and V. Ferreira, J. Chromatogr., A, 2002, 966, 167–177 CrossRef CAS.
- A. J. Weekley, P. Bruins, M. Sisto and M. P. Augustine, J. Magn. Reson., 2003, 161, 91–98 CrossRef CAS.
- S. Villanueva, A. Guadarrama, M. L. Rodriguez-Mendez and J. A. de Saja, Sens. Actuators, B, 2008, 132, 125–133 CrossRef.
- J. P. Santos, T. Arroyo, M. Aleixandre, J. Lozano, I. Sagayo, M. Garcia, M. J. Fernandez, L. Ares, J. Guitierrez, J. M. Cabellos, M. Gil and M. C. Horrillo, Sens. Actuators, B, 2004, 102, 299–307 CrossRef.
- M. Aleixandre, J. Lozano, J. Gutierrez, I. Sagayo, M. J. Fernandez and M. C. Horrillo, Sens. Actuators, B, 2008, 131, 71–76 CrossRef.
- J. P. Santos, J. Lozano, M. Aleixandre, I. Sagayo, M. J. Fernandez, L. Ares, J. Gutierrez and M. C. Horrillo, Sens. Actuators, B, 2004, 103, 98–103 CrossRef.
- J.-L. Puech, A. Prida and S. Isz, S. Afr. J. Enol. Vitic., 2007, 28, 101–106 Search PubMed.
- V. Parra, A. A. Arrieta, J. A. Fernandez-Escudero, M. Iniguez, J. A. de Saja and M. L. Rodriguez-Mendez, Anal. Chim. Acta, 2006, 563, 229–237 CrossRef CAS.
- G. Verrelli, L. Lvova, R. Paolesse and C. Di Natale, Sensors, 2007, 7, 2750–2762 CrossRef CAS.
- V. Parra, T. Hernando, M. L. Rodriguez-Mendez and J. A. de Saja, Electrochim. Acta, 2004, 49, 5177–5185 CrossRef CAS.
- M. L. Rodriguez-Mendez, V. Parra, C. Apetrei, S. Villanueva, M. Gay, N. Prieto, J. Martinez and J. A. de Saja, Microchim. Acta, 2008, 163, 23–31 CrossRef CAS.
- M. L. Rodriguez-Mendez, A. A. Arrieta, V. Parra, A. Bernal, A. Vegas, S. Villanueva, R. Guitierrez-Osuna and J. A. de Saja, IEEE Sens. J., 2004, 4, 348–354 CrossRef CAS.
- C. Di Natale, R. Paolesse, M. Burgio, E. Martinelli, G. Pennazza and A. D'Amico, Anal. Chim. Acta, 2004, 513, 49–56 CrossRef CAS.
- T. Zhang, N. Y. Edwards, M. Bonizzoni and E. V. Anslyn, J. Am. Chem. Soc., 2009, 131, 11976–11984 CrossRef CAS.
- A. T. Wright, N. Y. Edwards, E. V. Anslyn and J. T. McDevitt, Angew. Chem., Int. Ed., 2007, 46, 8212–8215 CrossRef CAS.
- S. C. McCleskey, M. J. Griffin, S. E. Schneider, J. T. McDevitt and E. V. Anslyn, J. Am. Chem. Soc., 2003, 125, 1114–1115 CrossRef CAS.
- S. L. Wiskur, H. Ait-Haddou, J. J. Lavigne and E. V. Anslyn, Acc. Chem. Res., 2001, 34, 963–972 CrossRef CAS.
- B. T. Nguyen and E. V. Anslyn, Coord. Chem. Rev., 2006, 250, 3118–3127 CrossRef CAS.
-
K. J. Wallace, B. T. Nguyen and E. V. Anslyn, Encyclopedia of Supramolecular Chemistry, Vol. 1, Taylor & Francis, 2006, pp. 1–11 Search PubMed.
- C. S. Burns, E. Aronoff-Spencer, C. M. Dunham, P. Lario, N. I. Avdievich, W. E. Antholine, M. M. Olmstead, A. Vrielink, G. J. Gerfen, J. Peisach, W. G. Scott and G. L. Millhauser, Biochemistry, 2002, 41, 3991–4001 CrossRef CAS.
- C.-W. Cheng, K.-C. Lin, F.-M. Pan, S. Sinchaikul, C.-H. Wong, W.-C. Su, C.-H. Hsu and S.-T. Chen, Bioorg. Med. Chem. Lett., 2004, 14, 1987–1990 CrossRef CAS.
- M. A. Wells, C. Jelinska, L. L. P. Hosszu, C. J. Craven, A. R. Clarke, J. Collinge, J. P. Waltho and G. S. Jackson, Biochem. J., 2006, 400, 501–510 CrossRef CAS.
- H. Yoshida, N. Matsushima, Y. Kumaki, M. Nakata and K. Hikichi, J. Biochem., 2000, 128, 271–281 CAS.
-
Peptides
3 to 10 were chosen randomly from the pool of available peptides.
-
L. Eriksson, E. Johansson, N. Kettaneh-Wold, J. Trygg, C. Wikström and S. Wold, Multi- and Megavariate Data Analysis Part I: Basic Principles and Applications, 2nd edn, Umetrics AB, Umeå, 2006 Search PubMed.
- Members of the array were randomly chosen.
- Wines used were Turning Leaf Vineyards Pinot Noir (2006), Dancing Bull Zinfandel (2005), Messina Hof Beaujolais (bought in 2008), Lindemans Cabernet Sauvignon (2007), Lindemans Shiraz (2007), and Lindemans Merlot (2007).
- R. D. Curtright, J. A. Rynearson and J. Markwell, J. Chem. Educ., 1994, 71, 682–684 CrossRef CAS.
- The wine was filtered through 0.45 μm and its pH was adjusted to 7.4.
- Shiraz tested were Ravens Wood 2006 (Shiraz Rw), Match Book JL Giguiere 2005 (Shiraz Ma), Barefoot 2009 (Shiraz Ba), Red Truck 2006 (Shiraz RT), Sterling Vintener's 2005 (Shiraz St).
- Sterling Vinters 2005 (Zinfandel SV), Cellar No. 8 2007 (Zinfandel C), Blackstone2006 (Zinfandel BS), Ravens Wood
2006 (Zinfandel R), and Bonterra Vineyards (Zinfandel BT) were used.
Footnote |
† Electronic supplementary information (ESI) available: Assay conditions, peptide synthesis and characterization, binding curves, and additional PCA and LDA plots. See DOI: 10.1039/c0sc00487a |
|
This journal is © The Royal Society of Chemistry 2011 |
Click here to see how this site uses Cookies. View our privacy policy here.