DOI:
10.1039/D1NR06858J
(Paper)
Nanoscale, 2022,
14, 1480-1491
Development of a high-throughput platform for screening lipid nanoparticles for mRNA delivery†
Received
17th October 2021
, Accepted 4th January 2022
First published on 5th January 2022
Abstract
mRNA lipid nanoparticles (LNPs) are at the forefront of nucleic acid intracellular delivery, as exemplified by the recent emergency approval of two mRNA LNP-based COVID-19 vaccines. The success of an LNP product largely depends on the systematic optimisation of the four lipidic components, namely the ionisable lipid, PEG lipid, structural and helper lipids. However, the in vitro screening of novel lipidic components and LNP compositions is limited by the low-throughput of LNP preparation. To address these issues, we herein present an automated high-throughput screening platform to select novel ionisable lipids and corresponding LNPs encapsulating mRNA in vitro. This high-throughput platform employs a lab-based automated liquid handling system, amenable to high-throughput (up to 384 formulations per plate and several plates per run) and allows precise mixing and reproducible mRNA LNP preparation which ensures a direct head-to-head comparison of hundreds and even thousands of novel LNPs. Most importantly, the robotic process has been successfully applied to the screening of novel LNPs encapsulating mRNA and has identified the same novel mRNA LNP leads as those from microfluidics-mixing technology, with a correlation coefficient of 0.8751. This high-throughput platform can facilitate to narrow down the number of novel ionisable lipids to be evaluated in vivo. Moreover, this platform has been integrated into a fully-automated workflow for LNP property control, physicochemical characterisation and biological evaluation. The high-throughput platform may accelerate proprietary lipid development, mRNA LNP lead optimisation and candidate selection to advance preclinical mRNA LNP development to meet urgent global needs.
Introduction
Nucleic acid-based medicines including antisense oligonucleotide (ASO), small interfering RNA (siRNA), microRNA (miRNA), messenger RNA (mRNA), self-amplifying RNA (saRNA) and DNA have emerged as game changer therapies with the potential to address previously undruggable targets and cure diseases. The first ASO drug, Fomivirsen, was approved by the U.S. Food and Drug Administration (FDA) in 1998.1 Fourteen years later, the first DNA-based medicine (Glybera) was approved by the European Medicines Agency (EMA).2 In 2018, the first siRNA drug (Onpattro) delivered by a non-viral vector (lipid nanoparticles, LNPs) was approved by the FDA3 followed by the approval of another siRNA drug (Givlaari) in 2019.4 These series of successes have evoked fierce competition for biotechnology and pharmaceutical companies to develop nucleic acid drugs.
Among a large panel of nucleic acids, mRNA is in the spotlight of recent technological innovation due to its great potential in preventing and treating disease. A key advantage of mRNA is its ability to use our own body to transiently make proteins, thus rendering better tolerability and safety profiles compared to other nucleic acid-based drugs. With the current global pandemic of COVID-19, sixteen mRNA LNP-based vaccines are at various stages of clinical development and two mRNA LNP-based vaccines have already been approved for emergency use.5 From a developability perspective, mRNA has advantages over DNA because mRNA production does not require biological systems. Through cell-free in vitro transcription, mRNA can be safely produced in large-scale at relatively low costs. From the delivery perspective, the target site of delivery for mRNA is the cytosol, whereas for DNA it is the nucleus. As a result, designing delivery systems for mRNA is a lower challenge relative to DNA.
Along with the successful clinical trials, there have been a number of failures,6 mainly attributed to safety and lack of efficient delivery. However, these problems can be circumvented by the development of safe and efficient non-viral delivery systems. Since LNP is the first non-viral delivery vector that has been successfully introduced in the clinic for the intracellular delivery of nucleic acids, many scientific efforts have been focused on LNP development. The first ever approved LNPs are composed of siRNA and four lipids: DLin-MC3-DMA (MC3), 1,2-distearoyl-sn-glycero-3-phosphocholine (DSPC), cholesterol and 1,2-dimyristoyl-rac-glycero-3-methoxypolyethylene glycol-2000 (DMG-PEG 2000).3 These lipids form stable nanoparticles with siRNA encapsulated in the core.7 Due to a high percentage of cholesterol content (38.5% molar ratio), these nanoparticles mimic endogenous low density lipoproteins particles (LDLs) and can be coated with plasma apolipoproteins following intravenous injection.8,9 Apolipoprotein E (ApoE) has been shown to be the main apolipoprotein to coat LNPs and facilitate liver targeting.10 Therefore, ApoE-coated LNPs are like a ‘Trojan Horse’ to deliver siRNA to its target tissue and intracellular compartment. One of the key features of MC3 LNPs is their pKa of 6.44,11 which prompts MC3 headgroup ionisation at low pH and the establishment of electrostatic interactions with the oppositely charged nucleic acids, leading to high encapsulation efficiency during formulation. More importantly, during endosomal acidification, the ionised MC3 forms ion pairs with the anionic endosomal lipids, which facilitates endosomal escape of nucleic acids through the formation of unstable hexagonal structures.12 Given the pivotal role of ionisable lipids in promoting endosomal release, it is not surprising that LNP development is centred around the screening of novel ionisable lipids.13–18 In fact, to advance the best in vivo performing LNPs, hundreds and even thousands of novel lipids are usually screened in vitro.13–17,19,20 Furthermore, the composition and LNP physicochemical properties often need to be optimised to achieve efficient intracellular delivery,11 which translates into a large number of combinatorial conditions to be evaluated.
LNPs are produced by lipid precipitation mainly through T-junction mixing and microfluidics-mixing techniques.21–23 The former involves controllable mixing in T-shaped tubing which is currently used as a large-scale production process, while microfluidics-mixing makes use of herringbone or baffle microstructures.22,24,25 The herringbone micromixer-based technique has been developed into commercial devices such as the NanoAssemblr from Precision NanoSystems which has been widely used to prepare LNPs to study morphology, surface property and degradability of LNPs,26–29in vitro profiling and intracellular fate of LNPs,30,31 and in vivo understanding/application of LNP formulations.32,33 However, microfluidic-mixing technology is usually chip-based and features a low-throughput preparation, which limits its application for the screening of big libraries of novel LNPs. Therefore, there is an increasing demand for a high-throughput counterpart that allows automated LNP generation, physicochemical characterisation and biological evaluation to screen novel ionisable lipids and establish structure–activity relationships (SAR) for mRNA delivery. Some effort has been made to reduce preparation volumes (e.g. NanoAssemblr Spark) or increase throughput to 24 formulations or specific for antisense oligonucleotides.24,34 However, to our knowledge there are no studies exploring the feasibility of using automations for high-throughput screening of novel ionisable lipids for mRNA delivery.
In this study, we adapted an automated liquid handling system which is commonly used in labs and optimised the mixing parameters for the purpose of mRNA LNP high-throughput preparation. To verify if this platform could be used to screen novel LNPs encapsulating mRNA, 10 new ionisable lipids were synthesised, premixed with helper lipids and formulated into LNPs using the automated platform, followed by the in vitro evaluation of their mRNA functional delivery. For head-to-head comparison, the new ionisable lipids were prepared into mRNA LNPs by standard microfluidics-mixing technology. Interestingly, we have demonstrated that this new platform has identified the same novel LNP leads in view of mRNA functional delivery as the standard microfluidics-mixing (correlation coefficient of 0.8751) which would help reduce the number of novel lipids for further evaluation. It is worthwhile to note that the nucleic acid payload applied in this automated LNP platform is a long RNA (mRNA) which is 996 nucleotides (about 60-fold longer than ASOs) and is relatively difficult to be encapsulated and delivered. Moreover, two out of the ten novel LNPs made from the high-throughput platform were out-performed in comparison with the standard-mixing method, whose mechanism will be discussed in another publication.35 Another equally attractive aspect of this platform is its high-throughput capability with up to 384 LNPs per plate and many plates per run (versus 1 LNP per run for the standard microfluidic-mixing technique). Therefore, the automated high-throughput platform renders at least 100-fold improvement in mRNA LNP preparation efficiency, cutting down the formulation timeline from weeks to hours when used in a continuous mode. Additionally, this automated process allows the preparation of lipid mixtures and mRNA dilutions, microliter-scale (60 μL) generation of mRNA LNPs and nearly full recovery of the mRNA and lipid premix and mRNA LNP formulations from preparation wells which is extremely valuable for fulfilling automation process, reducing materials and costs, and increasing preparation efficiency. This robotic process can be easily integrated into a fully-automated workflow for mRNA LNP physicochemical characterisation and biological evaluation. Combining this platform with the design of experiment (DOE) and multiparametric screening of mRNA LNP formulations would serve as a useful tool to improve the in vitro and in vivo correlation. We envision that this platform will accelerate LNP discovery for mRNA-based therapeutics and vaccines.
Results and discussion
An efficient and economical automated platform for LNP preparation
High-throughput screening with reliable reproducibility and accuracy often relies on mechanical automation. Considering the high cost of raw materials, including mRNA and novel ionisable lipids, an automated LNP platform should have the ability to generate samples at a microliter scale and meanwhile to avoid loss of starting materials or final formulations in the process. For this reason, we focused on common laboratory automated liquid handling systems, such as Hamilton or other similar instruments. These technologies can handle actions such as tip-loading, aspirating, dispensing and mixing with high-throughput and reliable precision. Therefore, the controllable and robust dispensing and mixing can be explored to prepare mRNA and lipid premix and mix mRNA aqueous and lipid-ethanolic phases to prepare LNPs (Fig. 1). Moreover, this system is compatible with either 96- or 384-well plates which allows not only high-throughput and microscale LNP preparation, but also the incorporation of modular downstream workflows for LNP quality control, physicochemical characterisation and cell-based biological evaluation (Fig. 1). The first question we sought to answer in the present work is whether the mixing produced by an automated liquid handling system is efficient enough to generate mRNA LNPs. It is well established that the formation of LNPs involves lipid precipitation (also known as anti-solvent precipitation, nanoprecipitation or phase separation) that occurs when lipid molecules are supersaturated from mixing.21,22,36 Nanoprecipitation essentially involves nucleation and nucleus growth of solutes (mRNA and lipids in the case of LNPs) when solvent compositions change. Molecular interactions define the self-assembly process of solutes and therefore the properties of the resulting particles, such as size, surface charge and internal structure. Thus, we hypothesised that an automated liquid handling system with robust mixing could potentially generate nanoprecipitation to produce mRNA LNPs.
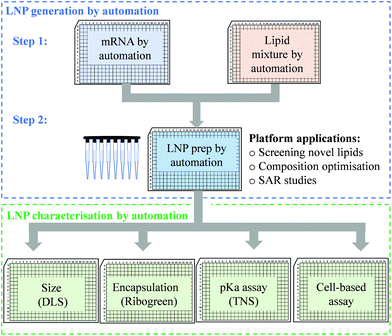 |
| Fig. 1 Diagram illustrating an example of an automated workflow for premix preparation, and LNP generation and characterisation. mRNA solution and lipid mixtures are first prepared by automation. The LNP generation steps involved in the automated preparation of LNPs include: (1) robot dispenses mRNA aqueous phase and lipid ethanolic phase into 384 or 96-well plates; (2) mRNA aqueous phase is aspirated from its original plate and dispensed into an LNP generation plate. Similar to mRNA, lipid ethanolic phase is aspirated from its original plate and dispensed into the same LNP generation plate, followed by mixing to produce LNP nanosuspensions. The aim of this automated workflow is to screen novel lipids and select lead lipids in vitro, therefore reducing the number of LNP formulations (to be prepared by microfluidic-mixing) to be tested in vivo. For downstream LNP characterisation, a robot aspirates and dispenses the LNPs into each assay plate for measurement of size (by using dynamic light scattering, DLS), mRNA encapsulation efficiency (using Ribogreen assay), pKa assay (using TNS fluorophore) and mRNA functional delivery (cell-based assays). | |
In order to determine the feasibility of this liquid handling platform to fabricate LNPs, a benchmark MC3-LNP formulation was used as a proof-of-concept. Specifically, 15 μL lipid solution was dispensed into 45 μL mRNA aqueous solution in each reaction well (96 well-plates) followed by mixing at a speed of 300 μL s−1 for ten cycles. Another 60 μL PBS was added to each well to dilute down the ethanol content and stabilise any nanoparticles that had been formed. For 384 well-plates, the volumes are 10 μL and 30 μL for lipid solution and aqueous solution respectively, followed by 40 μL PBS dilution. The resultant mixture was dialysed in PBS buffer (pH7.4) to generate LNP formulations. To assess if mRNA LNPs were formed, we used a series of characterisation methods to determine particle size and size distribution, morphology and pKa (Fig. 2). Fig. 2a shows the results of dynamic light scattering (DLS) measurement of the LNP formulation generated from the automated platform. Strikingly, a smooth correlation function was observed as a single exponential curve which suggested the presence of monodispersed particles. This was confirmed by the histogram of particle size distribution, revealing a Z-average size of 157.4 nm and a polydispersity of 0.095. To note, the large size of the LNPs is an advantage for loading more mRNA per particle.35 As expected, surface zeta potential of these nanoparticles was neutral (1.67 ± 0.34 mV) as all lipidic components are neutral at physiological pH. In addition, nanoparticle tracking analysis (NTA) further confirmed the formation of monodispersed nanoparticles (Fig. 2b). Morphology investigation using cryogenic transmission electron microscopy (cryoTEM) revealed LNPs with a spherical shape (Fig. 4e). Interestingly, these automated LNPs displayed a pKa of 6.27 (Fig. 2c), similar to the standard microfluidic-based LNPs, which represents a determinant factor for intracellular delivery. These results demonstrate that the automated liquid handling system can be used for mRNA LNP preparation, which opens up the opportunity for high-throughput mRNA LNP fabrication that can be standardised and transferrable across labs.
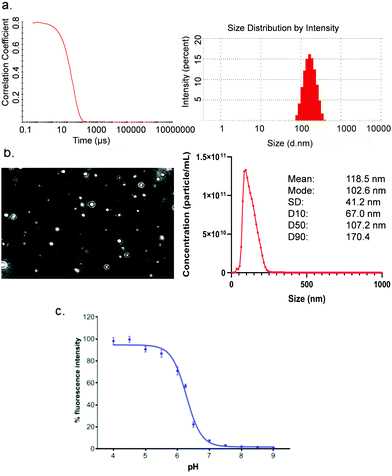 |
| Fig. 2 Physicochemical characterisation of mRNA MC3-LNPs produced with the automated platform. (a) Correlogram (left) of dynamic light scattering (DLS) measurements and size distribution of nanoparticles (right). (b) Visualized nanoparticle tracking (left) and size distribution from nanoparticle tracking analysis (NTA, right). (c) TNS assay of the automated LNPs. | |
Manipulating key automation-based parameters for LNP performance evaluation
After confirming that the automated platform could be applied to mRNA LNP preparation, it was important to understand whether these LNPs were biologically functional and able to render productive delivery of mRNA. mRNA is a long RNA and relatively harder to be loaded and delivered than small RNA such as siRNA or ASO. In parallel, the potential effect of various mixing parameters of the automated platform were investigated. Depending on the liquid handling system used, parameters such as pipetting speed and mixing volume should be optimised to ensure generation of high-performing LNPs. For the Hamilton liquid handling robot, key factors investigated included the mixing speed, mixing volume, dispensing speed, liquid tracking and tip type. When one parameter was manipulated, all other parameters were fixed to allow a controlled evaluation of the effect of that parameter on LNP generation. mRNA functional delivery of the automated LNPs was determined in H358 cells (human lung epithelial cells). Our previous studies have shown that H358 cells have slow pH change and lysosomal maturation with medium transfection capacity30 and therefore represent a suitable cell model to evaluate LNP functional delivery and rank different ionisable lipids.
Mixing speed
The mixing speed of mRNA aqueous phase and lipid ethanolic phase was first investigated. Fig. 3a shows the particle size and eGFP mRNA expression of LNPs produced at a mixing speed of 100 μL s−1 and 300 μL s−1 (while other mixing parameters were fixed, as shown in Fig. 3a). The low mixing speed is not investigated from a practical perspective because high mixing speed helps with mixing efficiency and reducing time consumed in the process. The mixing occurs with a position of 2 mm to the bottom of wells. Promisingly, all LNPs exhibited efficient eGFP mRNA expression (∼2.5 × 105) well above the background level of the untreated cells (p < 0.0001). It is worth highlighting that three batches of mRNA LNPs made at a mixing speed of 100 μL s−1 yielded the same eGFP expression (p = 0.6843), which indicates that the automated platform generates functionally reproducible mRNA LNP formulations. Next, the mixing speed was increased to 300 μL s−1 (close to the upper limit of 350 μL s−1) to test whether mixing could enhance mRNA functional delivery by generating particles with better properties. No statistical difference in eGFP expression (p = 0.1033) was obtained for LNPs prepared at 100 μL s−1 and 300 μL s−1, suggesting that the mixing speed has no effect on mRNA LNP functional delivery in the current setup. This may be because mRNA LNPs have formed in the step of dispersing the organic phase into mRNA-contained aqueous solution which is ahead of the mixing step. The effect of dispensing on mRNA LNP formation and functional delivery will be discussed later.
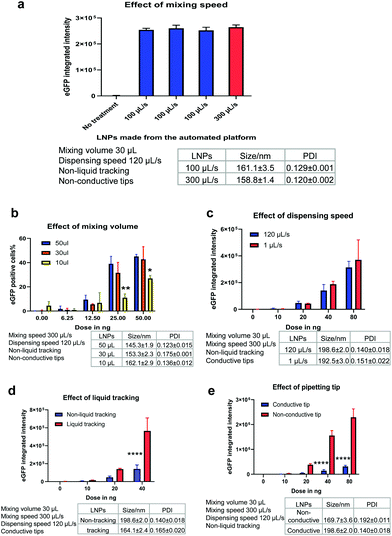 |
| Fig. 3 Key parameters of automated platform influencing LNP mRNA-eGFP functional delivery to H358 cells. (a) Mixing speed at 100 and 300 μL s−1 (mRNA 50 ng per well); (b) mixing volume of 10, 30, 50 μL; (c) dispensing speed at 1 and 120 μL s−1; (d) liquid tracking and (e) conductive and non-conductive tip types. *P < 0.05, **P < 0.01, ****P < 0.0001. Data was analysed by one-way (a) and two-way (b–e) ANOVA followed by Tukey's multiple comparisons. | |
Mixing volume
Another process parameter that was investigated was mixing volume, which is the volume of liquid that is aspirated into the pipette tip when mixing the lipid and mRNA solutions. In this experiment, three mixing volumes of 50 μL, 30 μL and 10 μL were tested (while other parameters were fixed, as shown in Fig. 3b). As expected, when the mRNA dose was increased from 6.25 to 100 ng per well, the LNPs showed increasingly higher mRNA transfection efficiencies (Fig. 3b). Interestingly, with the increase of mixing volumes from 10 μL to 30 μL, mRNA expression was improved (p < 0.01). However, when the mixing volumes increased from 30 μL to 50 μL, no statistically significant difference in the mRNA expression was observed. This indicates that a suitable mixing volume is required to produce beneficial effects for mRNA LNP functional delivery. Therefore, a mixing volume of 30 μL was used in further experiments unless otherwise specified.
Dispensing speed
In this automated process, the organic phase is dispensed into the corresponding wells where it is mixed with the mRNA aqueous phase to form LNPs. The dispensing speed is defined as the flow rate at which the lipid-containing ethanolic phase is injected to the mRNA aqueous solution. At this point, the two phases are mixed to produce LNPs and the dispensing speed resembles the mixing speed in the microfluidic mixing technology. Therefore, we investigated if the dispensing speed could have an impact on LNP formation and thus, on mRNA functional delivery. For this purpose, a slow dispensing speed of 1 μL s−1 and a median dispensing speed of 120 μL s−1 were applied to make mRNA LNPs. The dispensing height was kept at 1 mm to the bottom of wells. The particle size and eGFP expression levels of the resulting mRNA LNPs are shown in Fig. 3c. Interestingly, the slow dispensing speed did not affect the particle size nor the mRNA transfection efficiency. The slow dispensing renders a very low ethanolic phase to aqueous phase volume ratio and generates a sudden increase of polarity in the ethanolic phase which is beneficial to the formulation of nanoparticles. This could potentially suggest that in this automated platform, mRNA LNPs are formed in a shorter time scale than seconds and 1 μL s−1 has already been fast enough for the injection of 15 μL ethanolic solution to generate LNPs. It is not unreasonable to presume the LNP formation is very rapid in view that charge–charge interaction is instant and fast. Indeed, Zhigaltsev et al. have reported that small LNPs have formed within milliseconds by using a microfluidic mixing.37 120 μL s−1 dispensing speed was used hereafter to fabricate mRNA LNPs unless otherwise specified.
Liquid tracking
Liquid tracking is a mode of liquid dispensing/aspiration. In liquid tracking mode, pipette tips move up during dispensing and go down during aspiration to ensure that tips stay in the same position relative to the liquid level. It is reasonable to think that up and down movement of tips during dispensing and aspirating could render more efficient liquid mixing. In the particular case of LNP preparation, this could translate into a greater mixing of organic and aqueous phases, which could thus accelerate the change in polarity of the lipid-ethanolic phase, resulting in the nucleation and formation of LNPs. To assess whether liquid tracking mode could increase phase change and improve LNP properties, we prepared automated mRNA LNPs with and without liquid tracking. The hydrodynamic size and functional delivery of the resulting mRNA LNPs are shown in Fig. 3d. Interestingly, liquid tracking generated slightly smaller mRNA LNPs, which showed 3-fold higher eGFP expression compared to those obtained without liquid tracking (p < 0.0001 for 40 ng per well). These results show that the liquid tracking mode is important to generate efficient in vitro performing mRNA LNPs. On the other hand, without liquid tracking, pipette tips stay still at the same level relative to plate bottom when dispensing ethanolic lipid solution. This may lead to an inefficient diffusion of lipid solutions into the mRNA aqueous phase and to a supersaturated state in a local microenvironment, therefore affecting LNP particle size.38 In the subsequent experiments, liquid tracking was used to prepare LNPs unless otherwise specified.
Tip type
The pipetting tips used on the Hamilton liquid handling system can either be conductive tips (black, carbon-containing) or non-conductive tips (clear, non-carbon). These two types of tips are used to achieve dual liquid level detection (LLD) when the robot is performing liquid aspiration and dispensing. The conductive tips are used in capacitive LLD mode which detects conductive liquid. Contrarily, non-conductive tips are used in pressure LLD mode which detects all liquids, including non-conductive liquid. Since tips are in the core of a liquid handling system and given that well-performing mRNA LNPs rely on an efficient mixing, the effect of these two types of tips on the particle size and transfection of the resulting mRNA LNPs was investigated (Fig. 3e). Interestingly, non-conductive tips generated slightly smaller mRNA LNPs and with significantly greater mRNA transfection efficiency at doses of 40 ng and 80 ng (8-fold enhancement, p < 0.0001). Despite both types of tips being made of the same primary material (polypropylene), the conductive tips include carbon to improve the electrical properties. Therefore, the negative impact of the conductive tip may come from the interference of conductivity on the formation of mRNA LNPs by disturbing charge–charge interactions of lipids and mRNA. In the subsequent experiments, non-conductive tips were used to prepare LNPs unless otherwise specified.
Table 1 summarises the parameters that were evaluated and the optimised settings for mRNA LNP preparation in the following studies. The stability of the automated mRNA LNPs was also evaluated. As shown in Fig. S1,† the hydrodynamic size of the automated mRNA LNPs didn't change over 3 weeks and mRNA expression was maintained for one week when stored at 4 °C.
Table 1 Summary of the optimised parameters and the impact on mRNA functional delivery
Optimisation parameter investigated |
Tested range |
Optimised condition |
Fold-improvement |
Mixing speed |
100–300 μL s−1 |
300 μL s−1 |
None |
Mixing volume |
50–10 μL |
30 μL |
None |
Dispensing speed |
1–120 μL s−1 |
120 μL s−1 |
None |
Liquid tracking |
Yes or no |
Yes |
3× |
Tip type |
Conductive or non-conductive tip |
Non-conductive |
8× |
Head-to-head comparison of automated and standard platforms for mRNA LNP preparation
Following the establishment of the automation process for high-throughput mRNA LNP generation, we explored the feasibility of this platform to screen mRNA LNPs composed of ten novel ionisable lipids (iLipids) (Fig. 4a). To this end, a widely-used LNP fabrication technique (NanoAssemblr Benchtop) was used as a standard platform for head-to-head comparison and proof-of-concept (Fig. 4b).
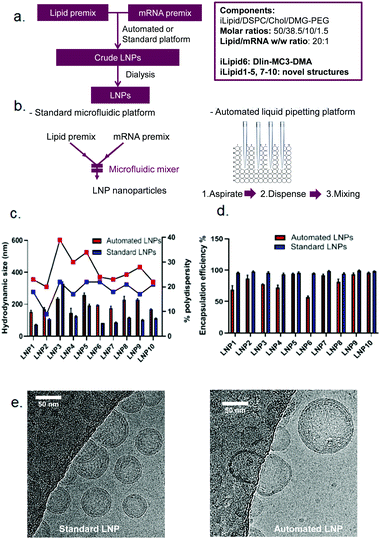 |
| Fig. 4 LNP preparation platforms and characterisation of ten novel LNPs-mRNA. (a) Formulation fabrication scheme (left) and components (right) of LNPs. (b) Key steps to fabricate mRNA LNPs using standard (left) and automated (right) platforms. Hydrodynamic size and PDI (c), mRNA encapsulation efficiency (d) and representative cryoTEM images (e) of the resulting mRNA LNPs fabricated with standard and automated platform. | |
It is worth noting that the mRNA LNPs generated from the automated and standard platforms have the same compositions and all steps in the formulation process are performed in the same way, except the mixing technique. For the automated mRNA LNPs, the mixing adopts the optimised parameters as shown in Table 1. For the standard mRNA LNPs, the mixing parameters were used as previously reported.29 All mRNA LNPs were characterised in terms of hydrodynamic size, PDI and mRNA encapsulation efficiency which have all been adapted to a high-throughput format (96 or 384-well plates) compatible with robotic automation. Here, we have shown that the techniques routinely used for mRNA LNP characterisation can equally be performed on an automated high-throughput platform, including the DLS for particle size distribution (ESI Fig. S2†), the Ribogreen assay to calculate mRNA content and encapsulation efficiency (ESI Fig. S3†), the TNS assay for pKa determination (ESI Fig. S4†) and the LNP-mediated transfection (ESI Fig. S5†). As shown in Fig. 4c, mRNA LNPs fabricated with the automated platform were generally bigger and more polydisperse compared to LNPs obtained by microfluidic-mixing. A larger size was anticipated for the automated LNPs as the mixing in this automated platform occurs in a plate without any mixing microstructures. Indeed, microstructures have been demonstrated to facilitate size reduction for LNP formulations.24,25 Kimura et al. designed a type of micromixer containing baffle microstructures for LNP generation and have shown that microstructures decreased the size of siRNA LNPs in comparison to those without microstructures.25 Chen et al. also compared their 24-channel micromixer with and without microfeatures and showed that when a microfeature is absent, the resulting LNPs were 2-fold larger.24 Interestingly, although automated LNPs were larger, they appeared to have lower mRNA encapsulation efficiency (Fig. 4d) and loading efficiency (ESI Fig. S6†). The lower mRNA encapsulation but higher mRNA expression were also observed by others which may be related with more mRNA copies per nanoparticle and increased LNP protonation in endosomal compartments.35,39 The cryoTEM images of these two types of mRNA LNPs (composed of lipid 6, MC3) are shown in Fig. 4e. In terms of particle size, the automated mRNA LNPs were about 2-fold larger than the standard mRNA LNPs, consistent with the DLS measurements. In addition, both mRNA LNPs were spherical with a dense core, although the automated mRNA LNPs appeared to be less homogeneous (Fig. 4e). From a product development perspective, pharmaceutical properties such as small particle size with narrow size distribution and a high encapsulation efficiency are preferred. However, the purpose of this high-throughput platform is to automate the process to efficiently generate a large number of mRNA LNPs with low cost and small volumes that is enough for characterisation and in vitro screenings. Therefore, we are interested in applicability of this platform in screening mRNA LNPs and selecting novel ionisable leads in vitro compared with microfluidic technology rather than improved pharmaceutical properties of the resulting mRNA LNPs. Our hypothesis is that if the automated mRNA LNPs with large size and low encapsulation efficiency works in vitro, their counterparts prepared by microfluidic-mixing would also work in vitro. The in vivo proof-of-concept of the automated mRNA LNPs is shown in a following manuscript.35
In vitro screening of novel LNPs-mRNA produced with the automated and standard platforms
As one of the biggest advantages of such an automated platform is the increase in throughput, we sought to investigate if the automated platform could identify the same leads for mRNA delivery from a library of novel LNP candidates as those identified with the standard platform. As suggested by publications, novel ionisable lipids are closely related with intracellular delivery efficiency of nucleic acid cargos and a large number of new lipid structures need to be screened for optimal efficiency and safety profile.13–17,19,20 Therefore, as mentioned in the section above, we synthesised 10 novel ionisable lipids including MC3 and formulated them into eGFP mRNA-containing LNPs by using the two platforms. The functional delivery of these novel LNPs were tested in H358 cells by measuring eGFP fluorescence intensity. As shown in Fig. 5a, the automated LNPs exhibited efficient mRNA functional delivery for all 10 new lipids. More importantly, eGFP expression produced by the automated mRNA LNPs was comparable or higher to that of the standard mRNA LNPs. Interestingly, the mRNA LNPs generated by the two platforms could be categorised in terms of mRNA functional delivery into: high expressers (lipid 6–10 including positive control MC3) and low expressers (lipid 1–5) which were defined as 100-fold difference in mRNA functional expression. This finding shows that the novel automated LNPs are not only functional but also able to be ranked into ‘good’ (high expressers) or ‘bad’ (low expressers). It is worth noting that the ranking in vitro is of great value to reduce the number of novel lipids and mRNA LNPs that will be used for next round of screening and optimisation. In the current study, 5 out 10 of the novel lipids (50%) would be selected for further evaluations. This selection is especially useful when a large library of novel lipids need to be screened in order to identify preclinical leads. It is not uncommon that hundreds or thousands of new lipids or lipidoids need to be screened for lead structures.13–17,19,20 Furthermore, the eGFP expression of the automated vs. the standard mRNA LNPs positively correlates with an R value of linear regression equal to 0.8751. Similarly, for the low expresser group (lipid 1–5), the eGFP expression obtained for the two mRNA LNPs was also correlated (circled in Fig. 5b), with a regression R value of 0.8786. The data generated supports our hypothesis that the same lipid leads are identified regardless of using the automated or standard LNP fabrication methods and shows that the automated platform is valid to differentiate novel lipids even when mRNA expression is in the lower range.
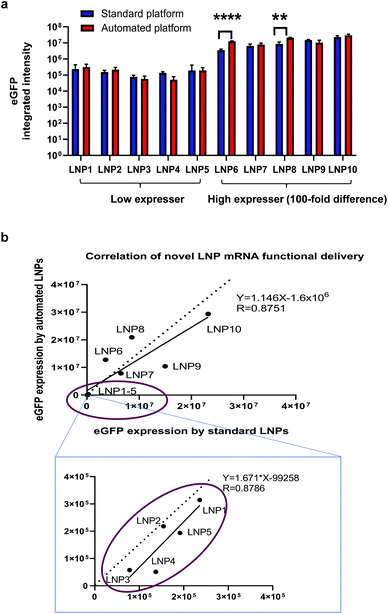 |
| Fig. 5 Functional delivery of novel LNPs-mRNA made from automated and standard platforms. (a) eGFP mRNA expression efficiency of LNPs using automated (red bars) and standard (blue bars) platforms. **P < 0.01, ****P < 0.0001 between two different groups (multiple unpaired-t test). (b) Correlation of mRNA functional delivery by automated and standard platform-fabricated LNPs (solid line). Dotted line represents a linear correlation with R = 1. Correlation of low expressor (LNP1–5) on the left graph (as in the ring) is enlarged and demonstrated on the bottom graph. | |
To this end, we have demonstrated a high-throughput automation platform for generating mRNA LNPs and screening novel lipid screening. This high-throughput platform can make hundreds and even thousands of mRNA LNPs in a cost and time-efficient way which is unachievable for the standard platform. From the high-throughput perspective, the advantage of the automation platform over the standard platform is summarised in Table 2. Lastly, it is worthwhile to note that this platform is suitable for not only lipid-based nanosystems but also polymer-based nanosystems (data not shown). Besides suitability for various delivery systems, this platform can be used for other nucleic acid modalities such as siRNA and pDNA. Despite the current platform is not intended for in vivo application, the LNPs encapsulating mRNA and pDNA generated from this platform were injected to mice. The in vivo proof-of-concept data for the automated mRNA LNPs has been shown in our mechanistic studies.35 The in vivo proof-of-concept data for the automated pDNA LNPs (pDNA encoding luciferase) is presented in ESI Fig. S7.† Interestingly, both the pDNA LNPs prepared from the high-throughput platform and the standard platform show luciferase expression in whole body and ex vivo organ images after tail vain i.v. injections (Fig. S7†). To note, the pDNA expression was observed in 48 h post injection but not early time points (6 h and 24 h), as pDNA first must cross the nucleus, be transcribed into mRNA, and trafficked back to the cytoplasm for translation. More interestingly, the LNPs generated from the high-throughput platform produced the same level of pDNA expression as these from standard platform (Fig. S7†). It is worth noting that this platform is intended for early-stage discovery of lipid nanoparticles and is a proof-of-concept of the development of a fully-automated workflow for LNP preparation, physicochemical characterisation and biological evaluation. Further optimization, such as analytical target profile and risk analysis, may be required when transitioned to late-stage development.
Table 2 A summary of the comparison of the automation platform with the standard platform for the preparation of the in vitro LNP formulations
Features |
Automated platform |
Standard platform (NanoAssemblr Benchtop) |
Minimal LNP volume |
0.06 ml, saving materials and costs |
1 ml |
Minimal mRNA amount |
∼0.006 mg, saving materials and costs |
∼0.1 mg |
Dead volume in the process |
No |
Yes |
Throughput |
High, 96 or 384 LNPs per run |
Low, 1 LNPs per run |
Time consumed (96 LNPs) |
∼40 min, high efficiency |
Days |
Process features |
Automated, integration with other functions |
Manually, no integration with other functions |
Lipid mixture preparation mRNA dilutions |
Yes |
No |
LNP characterisation |
Yes |
No |
In vitro evaluation |
Yes |
No |
Experimental
LNP preparation by standard and automated platform
LNPs containing eGFP mRNA (Trilink cleancap, 5MoU) or luciferase pDNA (made in-house) were prepared by lipid precipitation. The LNPs were composed of a ionisable cationic lipid (DLin-MC3-DMA or novel lipids (chemically synthesised)), DSPC (Avanti Polar Lipids), cholesterol (Sigma Aldrich), DMG-PEG2000 (NOF America Corporation) at 50
:
10
:
38.5
:
1.5 molar ratio. All lipids are dissolved in ethanol and mRNA is in 50 mM citrate buffer with pH 3. The mRNA concentration was kept at 0.14 mg ml−1 (lipids
:
mRNA weight ratio 20, lipids
:
pDNA weight ratio 33) in all formulations. The lipids and mRNA or pDNA solutions were mixed either in a NanoAssemblr (Precision Nanosystems) microfluidics device or in a robotic liquid handling system (Hamilton Microlab STAR). Lipid mixture and mRNA dilutions are prepared by the robotic liquid handling system for the high-throughput process and manually for the standard NanoAssemblr process. The mixing volume ratio of aqueous
:
ethanol was 3
:
1. Following the preparation process, the LNP formulations were transferred to Slide-A-Lyzer G2 dialysis cassettes (Thermo Scientific, MWCO 10 K) or 96 well microdialysis membrane (Thermo Scientific Pierce™, MWCO 10 K). Dialysis was performed in PBS for 2 h at RT, and then buffer was exchanged to fresh PBS and incubated overnight at 4 °C.
Size and zeta potential measurement in single cuvette or 384-well plates
The hydrodynamic ζ size and surface ζ-potential of LNPs was measured using a Nano ZS Zetasizer (Malvern-Panalytics) equipped with a 633 nm HeNe laser at a back scattering angle of 173 °C. Briefly, LNPs were 100-fold diluted in sterile PBS (for sizing) or deionised water (for surface ζ-potential) prior to measurement. The PBS viscosity of 0.8992 cP and refractive index of 1.331 were applied (25 °C). Samples were measured using a particle refractive index of 1.45. For novel LNPs, the hydrodynamic ζ size of LNPs was measured in 384-well plate (Greiner) using a DynaPro plate reader II (Wyatt Technology) equipped with a semiconductor laser with a wavelength of approximately 830 nm. Data analysis was performed automatically using cumulant method. Hydrodynamic particle radius (R) is obtained from diffusion coefficient D using Stokes–Einstein equation (D = kBT/6ηπR), where D is derived from the decay rate Γ (Γ = q2D) that is calculated by fitting a polynomial of third degree to the logarithm of the intensity correlation function.
mRNA encapsulation measurement by automation
The mRNA encapsulation efficiency (EE%), defined as encapsulated mRNA relative to overall mRNA including free and encapsulated mRNA, was quantified by a Quant-iT Ribogreen RNA assay kit (Invitrogen) following manufacturer's instructions and adapted to be performed on a Hamilton Microlab STAR (Hamilton) liquid handling system equipped with CO-RE tips (Hamilton). Briefly, 9 μL LNPs were diluted in 291 μL 1× TE buffer in a black clear-bottom 96-well plate (Corning). Then, 50 μL of diluted LNP were rapidly mixed and dispensed into wells containing either 1× TE buffer (for quantification of “free/unencapsulated” mRNA) or 2% (v/v) Triton X-100 (for quantification of “total” mRNA) to make 100 μL per well. Aspiration and dispensing speed was set to 150 μL s−1 with 3 mixing cycles. Similar steps were performed to generate the mRNA standard curve. After Triton X-100 addition, the plate was incubated for 10 min at 37 °C to facilitate LNP disruption and complete mRNA release. Following incubation, 100 μL of Ribogreen solution was added to each well at a speed of 120 μL s−1 followed by 5 mixing cycles. Ribogreen fluorescence was determined on an EnVision microplate reader (PerkinElmer) using an excitation wavelength of 480 nm and emission wavelength of 525 nm.
High-throughput pKa measurement
The TNS assay was used to determine the apparent pKa of LNPs. Briefly, a solution containing 10 mM HEPES, 10 mM MES, 10 mM ammonium acetate and 130 mM sodium chloride was aliquoted into different vials and the pH of each solution was manually adjusted to be in the range of 3.5–11. Next, LNPs were pipetted into an ECHO-qualified 384-well plate (Labcyte) and then dosed in triplicates into a 384-well black clear-bottom plate (Greiner) using an acoustic liquid handler (ECHO 550, Labcyte). LNP volumes (x) transferred were such that the final total lipid concentration was 10 μM. Three additional wells containing the same volume of PBS were used as a blank for background subtraction. Next, (95 − x) μL of each pH buffer solution were transferred to the 384-well plate containing LNPs using a multichannel pipette. Finally, 5 μL of a 120 μM TNS solution in DMSO were added to the wells containing LNPs, making a final TNS concentration of 6 μM. Fluorescence was determined on an EnVision microplate reader (PerkinElmer) using an excitation wavelength of 323 nm and an emission wavelength of 435 nm. The data was fitted to four-parameter logistic equation (Sigmoidal, 4PL) using GraphPad Prism v8.0.1. The apparent pKa of LNPs was determined as the pH value corresponding to half of the maximum fluorescence. This assay could potentially be adapted to a Hamilton liquid handling system to be fully-automated.
NTA measurement
NanoSight NTA 3.0 instrument (Malvern-Panalytics) was used for the determination of the size distribution of LNPs (a red laser for light scattering acquisition). LNPs were first diluted in PBS (prefiltered by 0.02 μm-pore membrane) to obtain 50–200 particles per frame in the field of view. After a suitable dilution factor was selected, LNPs were loaded to a syringe and then measured for 5 times and 60 s per time with a syringe pump infusion rate at 50 μL min−1. The camera level was set to 11 and the analysis detector threshold was 5. The data acquisition and analysis were performed using Nanosight NTA 3.0 software (Malvern-Panalytics).
cryoTEM imaging
Sample preparation and image capture was a service of FEI at the Nanoscience Centre, University of Cambridge. LNPs were blotted and flash frozen in liquid ethane using a VitrobotMKIV and quantifoil R1.2/1.3 2 mm carbon coated/copper 300 mesh grids. The grids were made hydrophilic by glow discharge in a weak vacuum in a Pelco Easiglo glow discharge unit at 0.39 mbar for 60 s at 25 mA. The Vitrobot was operated at 4 °C, 100% relative humidity, 2.5 s blot time. 2 μL LNP (containing 1 mg mL−1 mRNA) was used and the blotting was repeated twice per grid. Blot force was calibrated to give a “wedge” of thick ice on roughly 1/3 of the grid, with a gradient of ice thicknesses on the other 2/3 of the grid, corresponding to a setting of −6 on this system. Vitrified LNPs were stored in liquid nitrogen. Images were acquired on a Titan KriosTM G3i transmission electron microscope (FEI, Thermo Scientific TM) equipped with a Falcon 3 direct detector using the single particle data acquisition package EPU (1.10). Brightness and contrast were corrected in Fiji (ImageJ).
Cell culture
Human lung epithelial (H358) cells were obtained from American Type Culture Collection (ATCC). H358 cells were cultured in RPMI 1640 (Gibco, Thermo Fisher) supplemented with 10% heat-inactivated foetal bovine serum (FBS) (Gibco, Thermo Fisher), 2 mM GlutaMAX (Gibco, Thermo Fisher). Cells were maintained in a humidified incubator at 37 °C/5% CO2 for up to 10 passages post-thawing and regularly tested for mycoplasma (MycoSEQ™, Thermo Fisher).
LNP-mediated functional delivery of eGFP mRNA
One day prior transfection, 4.0 × 103 cells per well were seeded in 30 μL of complete culture media into poly-D-lysine black clear-bottom 384-well plates (Greiner) and incubated at 37 °C/5% CO2. The following day, LNPs containing 12.5–100 ng of mRNA at a working concentration of 40 ng μL−1 were added into cells using the acoustic droplet dispenser ECHO 550 (Labcyte). Immediately after LNP addition, 20 μL of complete medium was added to each well and cells were imaged using an Incucyte S3 (Essen Bioscience). The kinetic mRNA expression and phase contrast images were acquired using a 10× objective every 4 h for a total of 48 h. Image analysis was performed using the integrated Incucyte S3 2019A software. Fluorescence threshold level was adjusted to a value above the background level (non-transfected cells) in order to identify mRNA expressing cells. Fluorescence total integrated intensity and mean cell confluence were determined using segmentation masks applied over the fluorescence and phase contrast images, respectively. Statistical analysis and graphing were performed in GraphPad Prism 8 (GraphPad Software, La Jolla, CA).
Animal study
Female BALB/c mice approximately 6–8 weeks of age were obtained from Charles River UK and were housed in AstraZeneca animal facility (Babraham Research Campus). All procedures were carried out in accordance with Home Office U.K. ethical and husbandry standards, under the authority of an project licence of P8A7322E2. Randomised mice received tail injections of 100 μl of luciferase pDNA LNPs at a dose of 0.25 mg kg−1. 6, 24, 48 hours post injection, mice were injected with 0.1 ml (15 mg ml−1) XenoLight D-Luciferin (PerkinElmer) via i.p. route. Mice were anesthetized in a chamber with 3% isoflurane prior to imaging. 15 min post D-Luciferin administration, mice were imaged in an IVIS spectrum imager (PerkinElmer) including organs (liver, spleen, lungs, kidneys, heart, brain and fat tissues such as brown and white adiposes) exercised at 48 hours. Bioluminescence was quantified as average radiance (p s−1 cm−2 sr−1) and the data was analysed with GraphPad Prism v8.1.1 using unpaired t-test and two-way ANOVA followed by Tukey's multiple comparisons (significant p value < 0.05).
Conclusions
In this study, we have developed an automated high-throughput platform for mRNA LNP generation which is amenable to the modular integration of mRNA LNP physicochemical characterisation and biological evaluation. One of the advantages of this automated platform is the capability to prepare hundreds and even thousands of mRNA LNPs (microliter-scale, less materials required). The head-to-head comparison of the high-throughput platform with the standard microfluidic-mixing technology identifies the same lipid leads and shows a correlation coefficient of 0.8751 justifying the applicability of the high-throughput platform for novel ionisable lipid screening. Meanwhile, this high-throughput automation platform can narrow down the number of novel ionisable lipids and mRNA LNP formulations to be evaluated in in vivo studies. We envision that this platform can be expanded to many more applications such as DOE and multiparametric screening of mRNA LNP formulations which could serve as a useful tool to improve the in vitro and in vivo correlation and SAR relationship establishment to accelerate proprietary lipid and preclinical mRNA LNP development. The in vivo Proof-of-Concept data has been generated and will be presented with the mechanistic study of the enhanced mRNA functional delivery by the automated LNPs in a following publication.35
Author contributions
L. C., A. S. D., S. S, S. P., S. M. R., L. L. and S. van P. designed and performed the experiments. C. B. and E. B. programmed the automation. V. K. and D. U. designed and synthesised the lipids. L. C. wrote the manuscript with support from all authors and edits from S. P. All authors have given approval to the final version of the manuscript.
Conflicts of interest
L. Cui, S. Pereira, S. Sonzini, S. van Pelt, L. Liang, V. R. Krishnamurthy, E. Brannigan, C. Brankin and A. S. Desai are the employees of AstraZeneca and D. Ulkoski is the post-doc of AstraZeneca when the work is being performed.
Acknowledgements
We thank S. Puri for administrative and scientific support. We thank the editor and reviewers for their valuable comments.
References
- C. A. Stein and D. Castanotto, FDA-Approved Oligonucleotide Therapies in 2017, Mol. Ther., 2017, 25(5), 1069–1075, DOI:10.1016/j.ymthe.2017.03.023.
- N. F. Nidetz, M. C. McGee, L. V. Tse, C. Li, L. Cong, Y. Li and W. Huang, Adeno-Associated Viral Vector-Mediated Immune Responses: Understanding Barriers to Gene Delivery, Pharmacol. Ther., 2020, 207, 107453, DOI:10.1016/j.pharmthera.2019.107453.
- B. Hu, L. Zhong, Y. Weng, L. Peng, Y. Huang, Y. Zhao and X. J. Liang, Therapeutic SiRNA: State of the Art, Signal Transduction Targeted Ther., 2020, 5, 101–125, DOI:10.1038/s41392-020-0207-x.
- FDA approves first treatment for inherited rare disease https://www.fda.gov/news-events/press-announcements/fda-approves-first-treatment-inherited-rare-disease.
- The COVID-19 candidate vaccine landscape, https://www.who.int/publications/m/item/draft-landscape-of-covid-19-candidate-vaccines (accessed Jan 10, 2022).
- V. L. M. Herrera, A. H. Colby, N. Ruiz-Opazo, D. G. Coleman and M. W. Grinstaff, Nucleic Acid Nanomedicines in Phase II/III Clinical Trials: Translation of Nucleic Acid Therapies for Reprogramming Cells, Nanomedicine, 2018, 13(16), 2083–2098, DOI:10.2217/nnm-2018-0122.
- A. K. K. Leung, I. M. Hafez, S. Baoukina, N. M. Belliveau, I. V. Zhigaltsev, E. Afshinmanesh, D. P. Tieleman, C. L. Hansen, M. J. Hope and P. R. Cullis, Lipid Nanoparticles Containing SiRNA Synthesized by Microfluidic Mixing Exhibit an Electron-Dense Nanostructured Core, J. Phys. Chem. C, 2012, 116(34), 18440–18450, DOI:10.1021/jp303267y.
- G. I. Harisa and F. K. Alanazi, Low Density Lipoprotein Bionanoparticles: From Cholesterol Transport to Delivery of Anti-Cancer Drugs, Saudi Pharm. J., 2014, 22(6), 504–515, DOI:10.1016/j.jsps.2013.12.015.
- C. Zhu and Y. Xia, Biomimetics: Reconstitution of Low-Density Lipoprotein for Targeted Drug Delivery and Related Theranostic Applications, Chem. Soc. Rev., 2017, 46(24), 7668–7682, 10.1039/c7cs00492c.
- A. Akinc, W. Querbes, S. De, J. Qin, M. Frank-Kamenetsky, K. N. Jayaprakash, M. Jayaraman, K. G. Rajeev, W. L. Cantley, J. R. Dorkin, J. S. Butler, L. Qin, T. Racie, A. Sprague, E. Fava, A. Zeigerer, M. J. Hope, M. Zerial, D. W. Sah, K. Fitzgerald, M. A. Tracy, M. Manoharan, V. Koteliansky, A. De Fougerolles and M. A. Maier, Targeted Delivery of RNAi Therapeutics with Endogenous and Exogenous Ligand-Based Mechanisms, Mol. Ther., 2010, 18(7), 1357–1364, DOI:10.1038/mt.2010.85.
- M. Jayaraman, S. M. Ansell, B. L. Mui, Y. K. Tam, J. Chen, X. Du, D. Butler, L. Eltepu, S. Matsuda, J. K. Narayanannair, K. G. Rajeev, I. M. Hafez, A. Akinc, M. A. Maier, M. A. Tracy, P. R. Cullis, T. D. Madden, M. Manoharan and M. J. Hope, Maximizing the Potency of SiRNA Lipid Nanoparticles for Hepatic Gene Silencing in Vivo, Angew. Chem., Int. Ed., 2012, 51(34), 8529–8533, DOI:10.1002/anie.201203263.
- S. C. Semple, A. Akinc, J. Chen, A. P. Sandhu, B. L. Mui, C. K. Cho, D. W. Y. Sah, D. Stebbing, E. J. Crosley, E. Yaworski, I. M. Hafez, J. R. Dorkin, J. Qin, K. Lam, K. G. Rajeev, K. F. Wong, L. B. Jeffs, L. Nechev, M. L. Eisenhardt, M. Jayaraman, M. Kazem, M. A. Maier, M. Srinivasulu, M. J. Weinstein, Q. Chen, R. Alvarez, S. A. Barros, S. De, S. K. Klimuk, T. Borland, V. Kosovrasti, W. L. Cantley, Y. K. Tam, M. Manoharan, M. A. Ciufolini, M. A. Tracy, A. De Fougerolles, I. MacLachlan, P. R. Cullis, T. D. Madden and M. J. Hope, Rational Design of Cationic Lipids for SiRNA Delivery, Nat. Biotechnol., 2010, 28(2), 172–176, DOI:10.1038/nbt.1602.
- A. Akinc, A. Zumbuehl, M. Goldberg, E. S. Leshchiner, V. Busini, N. Hossain, S. A. Bacallado, D. N. Nguyen, J. Fuller, R. Alvarez, A. Borodovsky, T. Borland, R. Constien, A. de Fougerolles, J. R. Dorkin, K. Narayanannair Jayaprakash, M. Jayaraman, M. John, V. Koteliansky, M. Manoharan, L. Nechev, J. Qin, T. Racie, D. Raitcheva, K. G. Rajeev, D. W. Sah, J. Soutschek, I. Toudjarska, H. P. Vornlocher, T. S. Zimmermann, R. Langer and D. G. Anderson, A Combinatorial Library of Lipid-like Materials for Delivery of RNAi Therapeutics, Nat. Biotechnol., 2008, 26(5), 561–569, DOI:10.1038/nbt1402.
- K. A. Whitehead, J. R. Dorkin, A. J. Vegas, P. H. Chang, O. Veiseh, J. Matthews, O. S. Fenton, Y. Zhang, K. T. Olejnik, V. Yesilyurt, D. Chen, S. Barros, B. Klebanov, T. Novobrantseva, R. Langer and D. G. Anderson, Degradable Lipid Nanoparticles with Predictable in Vivo SiRNA Delivery Activity, Nat. Commun., 2014, 5(1), 4277, DOI:10.1038/ncomms5277.
- K. T. Love, K. P. Mahon, C. G. Levins, K. A. Whitehead, W. Querbes, J. R. Dorkin, J. Qin, W. Cantley, L. L. Qin, T. Racie, M. Frank-Kamenetsky, K. N. Yip, R. Alvarez, D. W. Y. Sah, D. Fougerolles, A. Fitzgerald, K. Koteliansky, V. Akinc, A. Langer, R. Anderson and D. G, Lipid-like Materials for Low-Dose, in Vivo Gene Silencing, Proc. Natl. Acad. Sci. U. S. A., 2010, 107(5), 1864–1869, DOI:10.1073/pnas.0910603106.
- M. A. Maier, M. Jayaraman, S. Matsuda, J. Liu, S. Barros, W. Querbes, Y. K. Tam, S. M. Ansell, V. Kumar, J. Qin, X. Zhang, Q. Wang, S. Panesar, R. Hutabarat, M. Carioto, J. Hettinger, P. Kandasamy, D. Butler, K. G. Rajeev, B. Pang, K. Charisse, K. Fitzgerald, B. L. Mui, X. Du, P. Cullis, T. D. Madden, M. J. Hope, M. Manoharan and A. Akinc, Biodegradable Lipids Enabling Rapidly Eliminated Lipid Nanoparticles for Systemic Delivery of RNAi Therapeutics, Mol. Ther., 2013, 21(8), 1570–1578, DOI:10.1038/mt.2013.124.
- K. P. Mahon, K. T. Love, K. A. Whitehead, J. Qin, A. Akinc, E. Leshchiner, I. Leshchiner, R. Langer and D. G. Anderson, Combinatorial Approach to Determine Functional Group Effects on Lipidoid-Mediated SiRNA Delivery, Bioconjugate Chem., 2010, 21(8), 1448–1454, DOI:10.1021/bc100041r.
- S. Sabnis, E. S. Kumarasinghe, T. Salerno, C. Mihai, T. Ketova, J. J. Senn, A. Lynn, A. Bulychev, I. McFadyen, J. Chan, Ö. Almarsson, M. G. Stanton and K. E. Benenato, A Novel Amino Lipid Series for MRNA Delivery: Improved Endosomal Escape and Sustained Pharmacology and Safety in Non-Human Primates, Mol. Ther., 2018, 26(6), 1509–1519, DOI:10.1016/j.ymthe.2018.03.010.
- L. Miao, L. Li, Y. Huang, D. Delcassian, J. Chahal, J. Han, Y. Shi, K. Sadtler, W. Gao, J. Lin, J. C. Doloff, R. Langer and D. G. Anderson, Delivery of MRNA Vaccines with Heterocyclic Lipids Increases Anti-Tumor Efficacy by STING-Mediated Immune Cell Activation, Nat. Biotechnol., 2019, 37(10), 1174–1185, DOI:10.1038/s41587-019-0247-3.
- S. Sabnis, E. S. Kumarasinghe, T. Salerno, C. Mihai, T. Ketova, J. J. Senn, A. Lynn, A. Bulychev, I. McFadyen, J. Chan, Ö. Almarsson, M. G. Stanton and K. E. Benenato, A Novel Amino Lipid Series for MRNA Delivery: Improved Endosomal Escape and Sustained Pharmacology and Safety in Non-Human Primates, Mol. Ther., 2018, 26(6), 1509–1519, DOI:10.1016/j.ymthe.2018.03.010.
- L. B. Jeffs, L. R. Palmer, E. G. Ambegia, C. Giesbrecht, S. Ewanick and I. MacLachlan, A Scalable, Extrusion-Free Method for Efficient Liposomal Encapsulation of Plasmid DNA, Pharm. Res., 2005, 22(3), 362–372, DOI:10.1007/s11095-004-1873-z.
- N. M. Belliveau, J. Huft, P. J. Lin, S. Chen, A. K. Leung, T. J. Leaver, A. W. Wild, J. B. Lee, R. J. Taylor, Y. K. Tam, C. L. Hansen and P. R. Cullis, Microfluidic Synthesis of Highly Potent Limit-Size Lipid Nanoparticles for in Vivo Delivery of SiRNA, Mol. Ther. – Nucleic Acids, 2012, 1(8), e37, DOI:10.1038/mtna.2012.28.
-
E. Samaridou, J. Heyes and P. Lutwyche, Lipid Nanoparticles for Nucleic Acid Delivery: Current Perspectives. Advanced Drug Delivery Reviews. Elsevier B.V., 2020, pp. 37–63. DOI:10.1016/j.addr.2020.06.002.
- D. Chen, K. T. Love, Y. Chen, A. A. Eltoukhy, C. Kastrup, G. Sahay, A. Jeon, Y. Dong, K. A. Whitehead and D. G. Anderson, Rapid Discovery of Potent SiRNA-Containing Lipid Nanoparticles Enabled by Controlled Microfluidic Formulation, J. Am. Chem. Soc., 2012, 134(16), 6948–6951, DOI:10.1021/ja301621z.
- N. Kimura, M. Maeki, Y. Sato, Y. Note, A. Ishida, H. Tani, H. Harashima and M. Tokeshi, Development of the ILiNP Device: Fine Tuning the Lipid Nanoparticle Size within 10 Nm for Drug Delivery, ACS Omega, 2018, 3(5), 5044–5051, DOI:10.1021/acsomega.8b00341.
- Y. Eygeris, S. Patel, A. Jozic and G. Sahay, Deconvoluting Lipid Nanoparticle Structure for Messenger RNA Delivery SI, Nano Lett., 2020, 20(6), 4543–4549, DOI:10.1021/acs.nanolett.0c01386.
- S. Patel, N. Ashwanikumar, E. Robinson, Y. Xia, C. Mihai, J. P. Griffith, S. Hou, A. A. Esposito, T. Ketova, K. Welsher, J. L. Joyal, Ö. Almarsson and G. Sahay, Naturally-Occurring Cholesterol Analogues in Lipid Nanoparticles Induce Polymorphic Shape and Enhance Intracellular Delivery of MRNA, Nat. Commun., 2020, 11, 983–995, DOI:10.1038/s41467-020-14527-2.
- H. Tanaka, T. Takahashi, M. Konishi, N. Takata, M. Gomi, D. Shirane, R. Miyama, S. Hagiwara, Y. Yamasaki, Y. Sakurai, K. Ueda, K. Higashi, K. Moribe, E. Shinsho, R. Nishida, K. Fukuzawa, E. Yonemochi, K. Okuwaki, Y. Mochizuki, Y. Nakai, K. Tange, H. Yoshioka, S. Tamagawa and H. Akita, Self–Degradable Lipid–Like Materials Based on “Hydrolysis Accelerated by the Intra–Particle Enrichment of Reactant (HyPER)” for Messenger RNA Delivery, Adv. Funct. Mater., 2020, 30(34), 1910575, DOI:10.1002/adfm.201910575.
- M. Yanez Arteta, T. Kjellman, S. Bartesaghi, S. Wallin, X. Wu, A. J. Kvist, A. Dabkowska, N. Székely, A. Radulescu, J. Bergenholtz and L. Lindfors, Successful Reprogramming of Cellular Protein Production through MRNA Delivered by Functionalized Lipid Nanoparticles, Proc. Natl. Acad. Sci. U. S. A., 2018, 115(15), E3351–E3360, DOI:10.1073/pnas.1720542115.
- E. J. Sayers, S. E. Peel, A. Schantz, R. M. England, M. Beano, S. M. Bates, A. S. Desai, S. Puri, M. B. Ashford and A. T. Jones, Endocytic Profiling of Cancer Cell Models Reveals Critical Factors Influencing LNP-Mediated MRNA Delivery and Protein Expression, Mol. Ther., 2019, 27(11), 1950–1962, DOI:10.1016/j.ymthe.2019.07.018.
- M. Maugeri, M. Nawaz, A. Papadimitriou, A. Angerfors, A. Camponeschi, M. Na, M. Hölttä, P. Skantze, S. Johansson, M. Sundqvist, J. Lindquist, T. Kjellman, I. L. Mårtensson, T. Jin, P. Sunnerhagen, S. Östman, L. Lindfors and H. Valadi, Linkage between Endosomal Escape of LNP-MRNA and Loading into EVs for Transport to Other Cells, Nat. Commun., 2019, 10, 4333, DOI:10.1038/s41467-019-12275-6.
- Q. Li, C. Chan, N. Peterson, R. N. Hanna, A. Alfaro, K. L. Allen, H. Wu, W. F. Dall'Acqua, M. J. Borrok and J. L. Santos, Engineering Caveolae-Targeted Lipid Nanoparticles To Deliver MRNA to the Lungs, ACS Chem. Biol., 2020, 15(4), 830–836, DOI:10.1021/acschembio.0c00003.
- J. D. Finn, A. R. Smith, M. C. Patel, L. Shaw, M. R. Youniss, J. van Heteren, T. Dirstine, C. Ciullo, R. Lescarbeau, J. Seitzer, R. R. Shah, A. Shah, D. Ling, J. Growe, M. Pink, E. Rohde, K. M. Wood, W. E. Salomon, W. F. Harrington, C. Dombrowski, W. R. Strapps, Y. Chang and D. V. Morrissey, A Single Administration of CRISPR/Cas9 Lipid Nanoparticles Achieves Robust and Persistent In Vivo Genome Editing, Cell Rep., 2018, 22(9), 2455–2468, DOI:10.1016/j.celrep.2018.02.014.
- Y. Fan, C. W. Yen, H. C. Lin, W. Hou, A. Estevez, A. Sarode, A. Goyon, J. Bian, J. Lin, S. G. Koenig, D. Leung, K. Nagapudi and K. Zhang, Automated High-Throughput Preparation and Characterization of Oligonucleotide-Loaded Lipid Nanoparticles, Int. J. Pharm., 2021, 599, 120392, DOI:10.1016/j.ijpharm.2021.120392.
- L. Cui, M. R. Hunter, S. Sonzini, S. Pereira, K. Liu, S. M. Romanelli, K. Liu, W. Li, L. Liang, B. Yang, N. Mahmoudi and A. S. Desai, Mechanistic Studies
of an Automated Lipid Nanoparticle Reveal Critical Pharmaceutical Properties Associated with Enhanced MRNA Functional Delivery in Vitro and in Vivo, Small, 2021 DOI:10.1002/smll.202105832.
- M. J. W. Evers, J. A. Kulkarni, R. V. D. Meel, P. R. Cullis, P. Vader and R. M. Schiffelers, State-of-the-Art Design and Rapid-Mixing Production Techniques of Lipid Nanoparticles for Nucleic Acid Delivery, Small Methods, 2018, 1700375, 1–20, DOI:10.1002/smtd.201700375.
- I. V. Zhigaltsev, N. Belliveau, I. Hafez, A. K. K. Leung, J. Huft, C. Hansen and P. R. Cullis, Bottom-up Design and Synthesis of Limit Size Lipid Nanoparticle Systems with Aqueous and Triglyceride Cores Using Millisecond Microfluidic Mixing, Langmuir, 2012, 28(7), 3633–3640, DOI:10.1021/la204833h.
- F. Li, Y. Chen, S. Liu, J. Qi, W. Wang, C. Wang, R. Zhong, Z. Chen, X. Li, Y. Guan, W. Kong and Y. Zhang, Size-Controlled Fabrication of Zein Nano/Microparticles by Modified Anti-Solvent Precipitation with/without Sodium Caseinate, Int. J. Nanomed., 2017, 12, 8197–8209, DOI:10.2147/IJN.S143733.
- M. J. Carrasco, S. Alishetty, M. Alameh, H. Said, L. Wright, M. Paige, O. Soliman, D. Weissman, T. E. C. Iv, A. Grishaev and M. D. Buschmann, Ionization and Structural Properties of MRNA Lipid Nanoparticles Influence Expression in Intramuscular and Intravascular Administration, Commun. Biol., 2021, 4, 956, DOI:10.1038/s42003-021-02441-2.
Footnote |
† Electronic supplementary information (ESI) available: Supplementary figures of stability of the mRNA LNPs generated from the high-throughput platform, high-throughput characterisation of mRNA LNPs including DLS for particle size distribution, Ribogreen assay for mRNA encapsulation, TNS assay for pKa determination and cell assay for LNP-mediated mRNA transfection, and in vivo proof-of-concept of the pDNA LNPs generated from the high-throughput platform by whole body and ex vivo tissue imaging. See DOI: 10.1039/d1nr06858j |
|
This journal is © The Royal Society of Chemistry 2022 |
Click here to see how this site uses Cookies. View our privacy policy here.