DOI:
10.1039/D4TC01223B
(Paper)
J. Mater. Chem. C, 2024,
12, 9217-9226
Embedded printing of graphene sponge sensors for sleep monitoring†
Received
27th March 2024
, Accepted 31st May 2024
First published on 6th June 2024
Abstract
Sleep disorders have emerged as a prevalent global issue, impacting physical and psychological health. Continuous and real-time sleep monitoring is crucial for improving sleep quality and preventing sleep-related health problems. This study presents an approach for developing sleep monitoring sensors by embedding three-dimensional graphene conductive network patterns (GCNP) onto sponges using the direct-write printing technique. The fabrication of GCNP sponge involves the precise assembly and patterning of graphene oxide (GO) onto a hydrophilic porous melamine sponge, ensuring an eco-friendly and customizable manufacturing process. This process allows the printed GO to infiltrate the sponge's inner structure, forming a strong, three-dimensional interconnected conductive network via hydrogen bonding and self-assembly. The resulting GCNP sponge exhibits satisfactory softness (Modulus = 36.2 kPa), sensitivity (206.85 kPa−1) and excellent stability (withstand 2500 compression cycles). The pressure sensors utilizing GCNP sponges have the capability to monitor sleep posture and breathing behavior, offering potential for enhancing sleep quality and addressing sleep-related disorders. This work demonstrates a promising and cost-effective method for developing comfortable, sensitive, and stable sleep monitoring sensors.
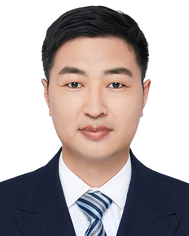
Zhandong Huang
| Zhandong Huang earned his PhD degree in 2018 from the Institute of Chemistry, Chinese Academy of Sciences. Following that, he served as a postdoctoral associate at the University of Western Ontario from 2019. Since 2022, he has held the position of associate professor at Xi’an Jiaotong University. His research focuses on investigating the interfacial interaction between fluids and solids, developing diverse methodologies for constructing structured fluid interfaces, such as structured bubbles, foams, and liquids. Based on these findings, he developed the applications in bubble acoustic metamaterials, wearable sensors, hydrogels, microfluidics and additive manufacturing. In these fields, he has published over 50 papers and applied more than 10 patents. |
1. Introduction
Sleep is an essential and vital activity in human life, playing a crucial role in maintaining human health and normal functioning.1–5 However, the fast-paced nature of modern society has led to a significant increase in sleep disorders, affecting more than half of the global population. Sleep disorders have now become a prevalent affliction worldwide, contributing to various physical and psychological conditions6–11 such as coronary heart disease,12 cardiovascular disease,13 Alzheimer's disease,14 and depression.15 Therefore, real-time and continuous sleep monitoring is essential for improving sleep quality and preventing sleep-related health problems.
With the rapid development of wearable electronic devices and the Internet of Things, flexible pressure sensors have garnered significant interest in the field of medical and health monitoring.16–22 These sensors can effectively convert external pressure stimuli into electrical signals through diverse conduction mechanisms.23,24 Due to their impressive mechanical flexibility and sensing performance, non-invasive flexible pressure sensors hold great potential for sleep monitoring applications. Currently, many studies are devoted to long-term sleep behavior monitoring by pressure detection, including the monitoring of sleep postures and physiological analysis.25,26 These studies require sensors with high sensitivity to detect small body movements during sleep. Designing pressure sensors with three-dimensional structures has proven to be an effective approach for enhancing sensor sensitivity.27,28 Three-dimensional pressure sensors can be assembled using conductive materials such as carbon nanotubes, graphene and MXenes by catalytic chemical vapor deposition or freeze-drying.29–33 Benefiting from the larger surface area of the three-dimensional structure, these sensors composed of pure conductive materials exhibit excellent conductivity and sensitivity.34–38 However, the inherent mechanical rigidity and brittleness of conductive materials limit the deformability of the sensors, making them susceptible to irreversible damage during compression. Considering that the sensor inevitably undergoes repeated compression during sleep, it is crucial to enhance its deformation capability and long-term stability while maintaining high sensitivity.
Sponges, with their high porosity and wide strain range, have emerge as candidates for fabricating large deformable pressure sensors.39 Recent studies have employed an immersion strategy to develop highly deformable 3D pressure sensors, utilizing sponges as flexible substrates to assemble conductive materials.40 However, the immersion method has drawbacks such as increased solvent and material costs and difficulties in controlling complex patterns. Furthermore, substantial loading of the conductive material by immersion leads to elevated modulus and stiffness, which may compromise the comfort of the sleep monitoring sensor. Hence, developing sleep pressure sensors with high comfort, sensitivity, and stability through economical and simple methods remains a formidable challenge.
Herein, we have developed a functional sponge with three-dimensional graphene conductive network patterns (GCNP) via embedded printing. The GCNP sponge was prepared by printing graphene oxide (GO) onto a hydrophilic melamine sponge, followed by a mild thermal reduction process. The embedded printing allows for precise control over the assembly and patterning of GO on sponges, maximizing the retention of sponge softness and realizing the construction of complex circuits. Compared to the immersion method, the direct-write printing approach represents an eco-friendly and sustainable alternative, as it reduces the excessive consumption of raw materials and solvents. Furthermore, the printed GO infiltrates the inner structure of the porous sponge via capillary action, establishing robust interactions with the hydrophilic sponge through hydrogen bonding. Simultaneously, the substantial π–π interactions among GO sheets lead to their self-assembly, culminating in forming a three-dimensional interconnected conductive network. Benefiting from the porous structure of sponges and the exceptional conductivity of graphene, the prepared GCNP sponge demonstrated remarkable softness (Modulus = 36.2 kPa), exceptional stability (withstand 2500 cycles test), and heightened sensitivity (206.85 kPa−1). Pressure sensors assembled by GCNP sponge have been successfully employed to monitor sleep posture and sleep breathing behavior, offering potential for enhancing sleep quality and mitigating sleep-related disorders.
2. Results and discussion
2.1 Preparation of GCNP sponges
Fig. 1a depicts the preparation of graphene conductive sponge using direct writing printing. Initially, an aqueous dispersion containing GO was printed onto a hydrophilic melamine sponge substrate. Due to the porous structure of the sponge, the printed GO ink further penetrated the sponge via capillary action, forming a graphene oxide composite sponge after drying. Subsequently, the composite sponge undergoes in situ reduction at 200 °C, causing the brown-colored GO within the sponge to transform into black, thereby obtaining a reduced graphene oxide (rGO) conductive sponge. During the printing process, GO permeates the interior of the sponge and undergoes self-assembly, utilizing the sponge frame as a template to form a three-dimensional structure. The abundant hydrophilic groups (e.g., hydroxyl and carboxyl groups) in the GO establish strong interconnections with the Imino groups of the melamine sponge through hydrogen bonding.41,42 Additionally, the robust π–π interactions among dispersed GO sheets promote the assembly process, resulting in the formation of a three-dimensional interconnected structure within the sponge, ultimately yielding the GCNP sponge. Fig. 1c showcases the printing of complex circuit patterns on GCNP sponge. In comparison to the previously reported immersion method,40 the direct-write printing method was more economical and more efficient in regulating GO-selective assembly on sponges for constructing intricate circuit patterns. As a result, this technology offers many advantages to fabricate functional sensing devices, enabling the development of a wide range of applications.
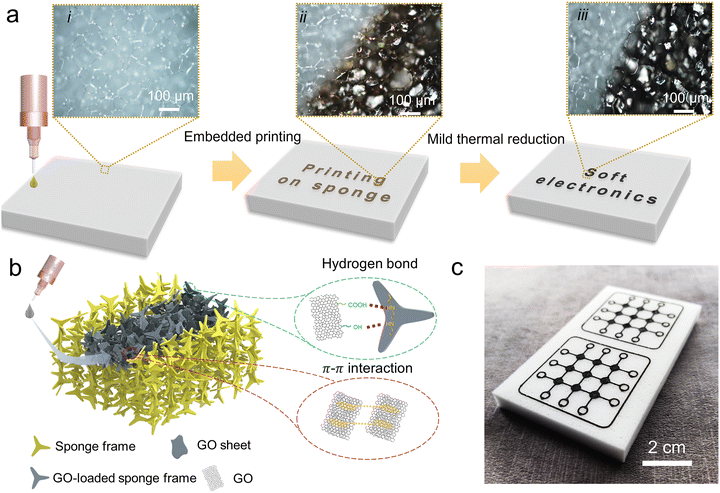 |
| Fig. 1 Preparation and patterning of GCNP sponge by embedded printing. (a) Schematic diagram of the fabrication process and the corresponding microscopic images of the internal structure of the sponge. (b) Schematic diagram of the assembly mechanism of GO within the sponge. (c) Top-view optical image of the printed GCNP sponge. | |
2.2 Embedded printing GCNP sponges
Fig. 2a and b illustrate the printing processes with low and high concentrations of GO ink, respectively, visualizing the two typical penetration characteristics. The sponge employed for the experiment was a melamine sponge with 99% porosity, featuring abundant hydrophilic groups and excellent hydrophilicity (Fig. S1 and S2, ESI†). When using a low concentration of GO, the ink will rapidly penetrate the sponge within a short period of time. Conversely, at high concentrations, the GO ink became sticky, resulting in weaker penetration and a tendency to accumulate on the sponge's surface. To better investigate the relationship between printing morphology and concentration of GO ink, we printed parallelly arranged straight lines and measured both the line width and penetration depth. As shown in Fig. 2c, the width of the printed line decreased as the GO concentration increased. This phenomenon can be attributed to the lower viscosity of the GO solution at the lower concentration, facilitating easier spreading and diffusion when printing onto the sponge, resulting in wider lines. In contrast, highly concentrated GO inks with higher viscosity are more susceptible to surface tension and adhesion during printing, forming narrower lines. By vertically cutting the samples to investigate their morphology, we measured the penetration depth (Fig. 2d and e). The penetration depth gradually decreases with the increase of GO concentration. In Fig. 2e, the cross-sectional pattern resulting from the infiltration of printed GO into the sponge exhibited a circular morphology. As the GO concentration increases, the diffusion angle of this circular pattern decreases from 138° to 90° accompanied by a decrease in area, suggesting a reduction in the diffusion and penetration of high GO concentration within the sponge. This observation can be attributed to the elevated viscosity of the GO inks, which hinders the diffusion of GO within the sponge. Additionally, the higher concentration of GO leads to a greater embedding of GO within the sponge's pore structure, consequently reducing the ability of GO penetration. These findings provide valuable insights into the relationship between GO ink concentration, printing morphology, and the penetration characteristics within the sponge. Moreover, in order to verify the bonding between GO sheets and sponge, peeling experiments were carried out on GCPN sponge with different concentrations of GO by using a tape. Only a few GO attached to the sponge surface would peel off, while the vast majority of GO penetrating into the interior would not be peeled off, indicating that there is a certain bonding force between GO sheets and sponge. This is because the sponge is super hydrophilic, ensuring that the hydrophilic GOs have favorable affinity with the sponge. In addition, the sponge structure contains abundant amino and carboxyl groups, forming hydrogen bonds with the carboxyl and hydroxyl groups on the surface of the GO, resulting in a strong interaction between the GO and the sponge.
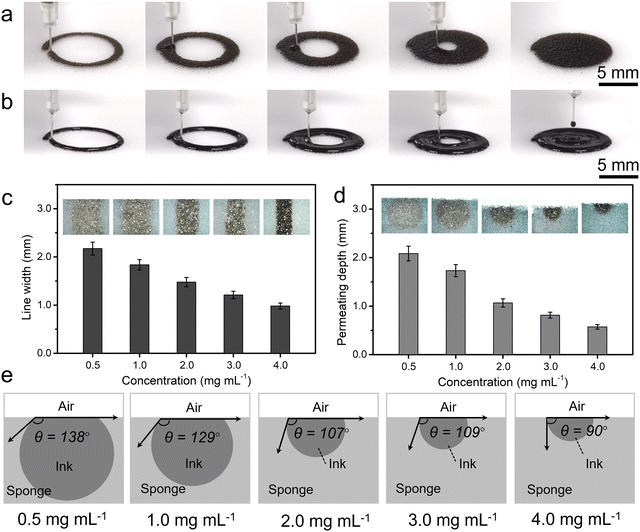 |
| Fig. 2 Direct-write printing processes and morphologies of the GCNP. (a) Printing processes of GO ink with low concentration (1 mg mL−1). (b) Printing processes using GO ink of high concentration (3 mg mL−1). (c) The relationship between the concentration of GO ink and line width of GCNP from top view. (d) Penetration depth of the GCNP in the sponge from side view. (e) Schematic representation of the penetration morphologies of GCNP with different concentrations of GO ink. | |
2.3 Microstructure of GCNP sponges
The microscopic morphology of sponges and GCNP with various GO concentrations was observed by scanning electron microscopy (SEM) in Fig. 3. Fig. 3a shows the melamine sponge with a homogeneous honeycomb porous structure, which facilitates the penetration of the GO ink and acts as a template to induce the assembly of the GO. After printing the GO on the sponge, the formation of GO with a lamellar structure inside the sponge was observed (Fig. 3b–f). At lower ink concentrations, GO flakes were found to penetrate deeper inside the sponge, indicating that higher permeability (Fig. 3b and c). As the ink concentration increased, GO gradually accumulated on the surface layer of the sponge, reflecting the weak permeability (Fig. 3d–f). Additionally, higher concentrations of GO filled more holes after printing, suggesting that increasing the GO concentration benefits its assembly. The GCNP maintains the porous structure of the sponge while incorporating highly conductive rGO, rendering it an intrinsically flexible and conductive material. The strong interaction between GO sheets and the sponge substrate enables the establishment of a three-dimensional and interconnected conductive framework, utilizing the porous sponge as a structural template. This unique structure provides the GCNP sponge with excellent conductivity and responsiveness when used as a sensing material.
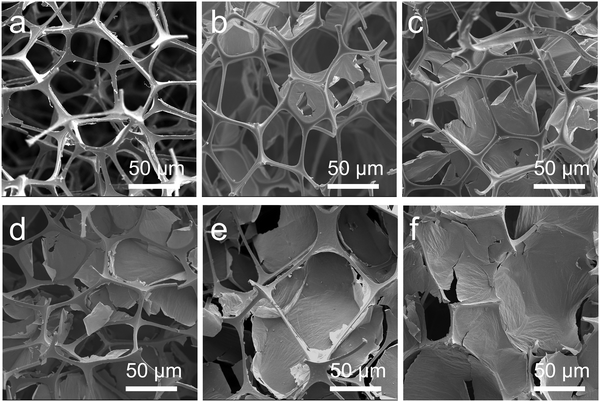 |
| Fig. 3 The microscopic morphology of GCNP sponges prepared with different GO concentrations. (a) SEM image of the melamine sponge. (b)–(f) SEM images of the GCNP with different concentrations of GO ink (0.5, 1.0, 2.0, 3.0, 4.0 mg mL−1). | |
2.4 Mechanical properties of the GCNP sponge
The comfort of the material is crucial for sleep monitoring sensors. Therefore, it is imperative to pursue the mechanical properties of the material closer to a soft sponge. To evaluate the compressive properties and energy dissipation, loading-unloading tests were employed to investigate the compressive behavior of different materials, including original sponge, GCNP sponge, and sponge graphene composite prepared by immersion method (Fig. 4a). The compression hysteresis curves of the GCNP sponge and the original sponge almost overlap, indicating that the GCNP sponge largely maintains the original softness of the sponge with a modulus of 36.2 kPa. This can be attributed to the embedded printing strategy, which effectively controls the localized assembly and patterning of graphene on the sponge, thereby preserving the original mechanical properties of the sponge. The effects of GO concentration on the compressive properties of GCNP sponge were further investigated (Fig. S4, ESI†). At lower GO content, the compressive strength and modulus of GCNP sponge are higher, indicating the improved mechanical properties. At low concentration, the rigid GO could penetrate deep into the sponge and form a three-dimensional network structure (Fig. S5, ESI†). It promoted the effective transfer of stress in the sponge network, giving GCNP sponge a high elastic modulus and compressive strength. However, as the GO content increases, the maximum compressive strength and modulus reaches a peak and then began to decrease. This was because the GO nanosheets were more easily aggregated and assembled on the sponge surface as the concentration was higher (Fig. S6, ESI†). This tended to produce stress concentration points, which was not conducive to the effective transfer of stress. Fig. 4b shows the loading-unloading curves at compressive strains of 10% to 80%. As the strain increased, the hysteresis curve of GCNP sponge exhibits an enlarged closed area, illustrating an increase in energy dissipation. Moreover, the hysteresis curves of the GCNP sponge displays the overlapping curves and areas at different rates (Fig. 4c), suggesting promising resistance and reversibility. The fatigue resistance of GCNP sponge was investigated by cyclic compression load–unload tests (Fig. 4d). After 100 cycles of compression at high strains of 80%, the hysteresis curves of GCNP sponge consistently displayed a closed and homogeneous region, demonstrating its remarkable fatigue resistance and recovery.
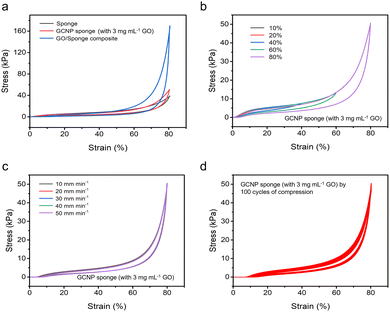 |
| Fig. 4 Mechanical properties of the GCNP sponge (with 3 mg mL−1 GO concentration). (a) Comparison of the compression behavior among the sponge, GO/Sponge composite prepared by immersion method, and GCNP sponge obtained by direct writing. (b) and (c) Compression loading-unloading curves of GCNP sponge at different compressive strains and different compression rates. (d) Cyclic loading-unloading curves of GCNP sponge at compressive strain of 80%. | |
2.5 Pressure sensing performance of the GCNP sponge sensors
When subjected to pressure, the GCNP sponge sensor undergoes deformation, altering the pathway of charge transport and resulting in a variable current. Taking advantage of this inherent characteristic, pressure sensors were fabricated by assembling GCNP sponge and PET film containing silver electrodes (Fig. 5a). To evaluate its pressure sensing performance, electrochemical tests were employed to monitor the current changes under different pressures in real time. Comparative analysis of GCNP sponge sensors with varying GO contents revealed that the sensor with 3 mg mL−1 of GO exhibited the highest sensitivity (Fig. 5b). The reason for this phenomenon was further investigated in terms of conformational relationships by SEM (Fig. S5–S7, ESI†). In the process of embedded printing, low concentration GO ink could be deeply embedded into the sponge, but it was not conducive to assembly. Conversely, high-concentration GO was easy to assemble after printing, but the penetration depth was limited, leading to most of the GO assembly being concentrated on the sponge's surface (Fig. S6, ESI†). This concentration on the surface was unfavorable for stress conduction, thereby compromising sensitivity. As the GO concentration reaches 3 mg mL−1, it not only achieved easy penetration but also facilitated assembly, thus forming a highly interconnected conductive network (Fig. S7, ESI†). This network was beneficial for the rapid conduction of pressure within the sponge. Pressure sensitivity is a key parameter in evaluating the accuracy of pressure sensors, referring to the ratio between the change in electrical signal response and the corresponding change in pressure. As shown in Fig. 5c, the sensitivity fitting curve of the GCNP sensor exhibited four partially linear fitting regions, with sensitivities of 206.85 kPa−1 for 0–65 kPa, 114.94 kPa−1 for 65–175 kPa, 71.40 kPa−1 for 175–475 kPa, and 32.73 kPa−1 for 475–1000 kPa, respectively. This sensor exhibited ultra-high sensitivity, superior to previously reported graphene-based sensors (Fig. 5d).43–52 Based on the strategy of infiltration printing, the GCNP sponge forms a porous and three-dimensional conductive structure, facilitating the rapid transfer and detection of loads in the sponge structure.
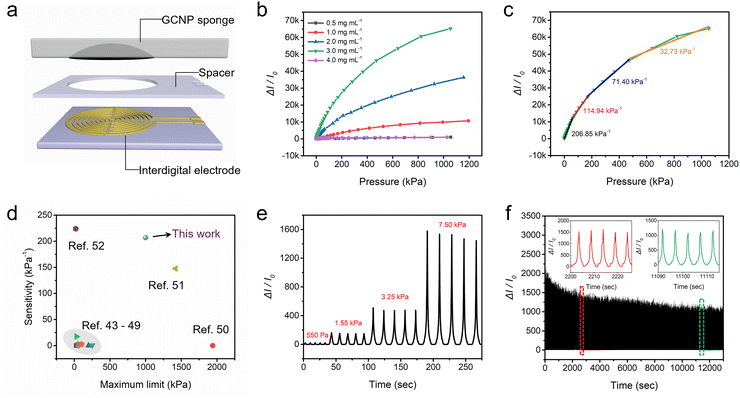 |
| Fig. 5 Performance of the pressure sensors fabricated by GCNP sponge. (a) Schematic diagram of the assembly of the GCNP sponge pressure sensor. (b) Electromechanical characterization of the sensors prepared by different concentrations of GO ink. (c) Sensitivity fitting curves of the sensor. (d) Comparison of sensitivity of this sensor with previous work. (e) Relative current change of the sensor during 5 load–unload cycles at different pressures. (f) The relative current variation curve of the sensor undergoes 2500 repeated compressions at 9.5 kPa. | |
In the GNCP sponge sensor, different layers of PET exhibited distinct functionalities. The bottom PET layer solely served as a support for the sensing element, without exerting any influence on the sensor's sensitivity. Moreover, the middle PET layer played a crucial role as a spacer, connecting the sensor to the electron collector, and significantly impacting its sensitivity. To comprehensively assess the sensor's performance, the influence of varying PET thicknesses was further examined (Fig. S8, ESI†). As the PET thickness increased, the sensitivity of the sensor decreased because increasing the thickness of the PET spacer enhanced the contact resistance between the GNCP sponge and the bottom electrode. Consequently, the optimal choice for the intermediate spacer entailed employing the thinnest PET sheet available.
Fig. S9 (ESI†) presents the response time of the sensor for the loading and unloading test at a pressure of 500 Pa. The sensor exhibited fast response to pressure loading and unloading, with a response time of 215 ms and a recovery time of 250 ms, facilitating real-time monitoring of rapid and complex pressure changes. The sensing performance at different pressures and different compression frequencies was further investigated. The GCNP sponge sensor was capable of consistently and repeatedly detecting different levels of pressure and stepwise pressure (Fig. 5e and Fig. S10, ESI†), showing a wide range of pressure response (500–7.5 kPa). Additionally, it demonstrated the ability to recognize pressure signals at different compression rates (Fig. S11, ESI†). Fig. 5f depicts the real-time curve of the current variation of the sensor under 2500 cycles of loading and unloading. During repeated compression, it could stably detect periodic current changes, proving its long-term stability and sensing reliability in practical applications. Further, the microstructural changes of GNCP sponge before and after repeated compression were analyzed (Fig. S12, ESI†). Remarkably, the structure of the GNCP sponge exhibited minimal changes even after undergoing repeated compression, with the internal GO maintaining its interconnected three-dimensional arrangement. This exceptional structural stability can be attributed to the strong bonding between the GOs and the sponge, which guarantees uniform distribution of loads, as well as the remarkable load cushioning capability of the porous structure.
2.6 Application of GCNP sponge sensors for sleep monitoring
Owing to its sponge-like softness, excellent fatigue resistance and reliable pressure sensing capability, GCNP sponge was assembled as a pressure sensor for monitoring sleep modes (Fig. 6a). A GCNP sponge pressure sensor with a 6 × 3 array was fabricated by direct writing printing and integrated into a pillow (Fig. 6b). The sensor comprises 18 channels, with each channel featuring a sensor unit composed of a sponge embedded in an rGO cylinder (15 mm diameter in cross-section) (Fig. 6c). As illustrated in Fig. 6d, the posture of the volunteer during sleep, including supine and lateral positions, was monitored in real-time using pillows equipped with GCNP sponge pressure sensors. When a volunteer transitions from supine to a side-lying position, the sensor's multiple channels detect heightened relative current changes, manifesting specific peaks and waveforms corresponding to left and right lateral lying positions. Furthermore, each sensor channel consistently and accurately monitored repetitive electrical signals even as the sleeping position was repeatedly altered, showcasing exceptional sensitivity and reliability. The relative current changes of the 18 acquired channels were then plotted to visualize the matrix distribution (Fig. 6e and f). The electrical signals were centrally distributed on the left sensor channel when the volunteer was lying on the left, and conversely on the right. The sleep distribution map indirectly reflects the pressure distribution of the human body during sleep, with the potential to enhance and rectify improper sleeping positions.
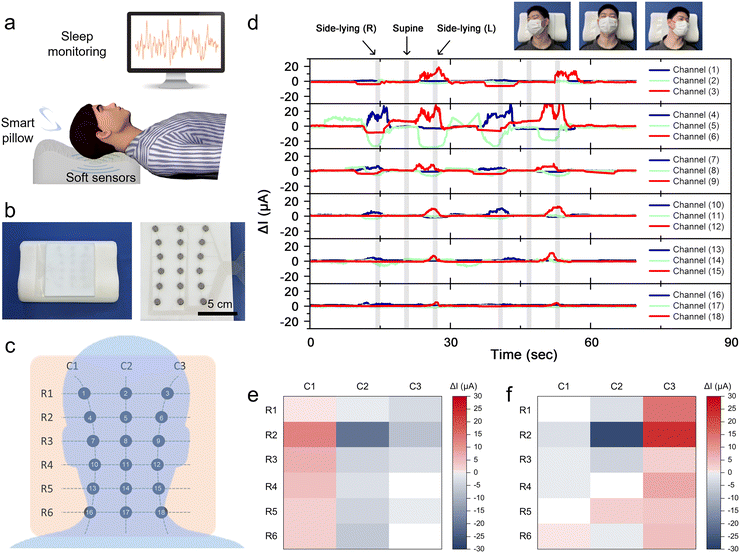 |
| Fig. 6 Application of GCNP sponge sensors for sleep monitoring. (a) Schematic diagram of a GCNP sponge sensor for sleep monitoring. (b) Integration of the GCNP sponge pressure sensor with the pillow. (c) Schematic of the array distribution of pressure sensors and the corresponding pressure distribution on the sleeper's head. (d) Relative current change profiles monitored in real time by the 18 channels of the GCNP sponge sensor for both supine and lateral positions. (e) and (f) Schematic distribution of electric signals in different lateral lying positions including right lateral lying (e) and left lateral lying (f). | |
2.7 Application of the GCNP sponge sensor to sleep breathing monitoring
Sleep breathing disorder is a prevalent sleep disorder disease that significantly impacts the quality of sleep and overall health of patients. During sleep, the pressure exerted on the pillow by the head and neck fluctuates with each breath. Consequently, by continuously monitoring the real-time pressure changes exerted by the volunteer on the pillow, the integrated pressure sensor array could effectively discern various breathing disorders, including snoring, deep sleeping, and coughing (Fig. 7a–c). The GCNP sponge sensing array accurately recorded the electrical signals when volunteers took six deep breaths in 90s, demonstrating stable and repetitive relative current changes in the multiple channels (Fig. 7a). Fig. 7d depicts a two-dimensional plot of the distribution of pressure signals during deep breathing, where red areas indicate areas of increased pressure signals and the blue areas represent attenuated pressure signals. The pressure sensing array effectively discriminated and recorded current changes at different pixel locations during deep breathing, elucidating that hindbrain pressure is mainly concentrated in the bottom region of the brain during deep breathing. Moreover, the array sensor monitored the weak electrical signals of six repeated snores within 60 s (Fig. 7b) and recognized the corresponding pressure signal distribution in Fig. 7e. The relaxation of throat muscles during snoring caused airway obstruction and diminished airflow, leading to localized pressure in the in the hindbrain and neck. Similarly, the sensor consistently detected stronger pressure signals during coughing (Fig. 7c) and identified a concentrated distribution of pressure signals within channels 5, 7, and 8 (Fig. 7f). This occurs because the body was relaxed during sleep and the head naturally tilts forward when coughing, thereby creating attenuated pressure in the triangular area above the hindbrain. Therefore, by sensing pressure changes to detect the sleep breathing process, the GCNP sponge sensor can monitor and record sleep quality and breathing, thereby assisting individuals in improving their sleep health.
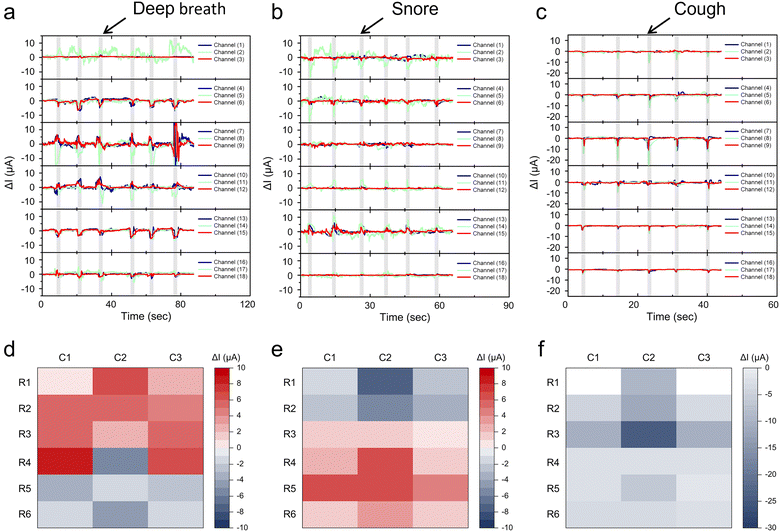 |
| Fig. 7 Application of GCNP sponge sensor for sleep breathing monitoring. (a)–(c) The sensor's 18 channels continuously monitor relative current changes during sleep in various respiratory states, including deep inspiration (a), snoring (b), and coughing (c). (d)–(f) Schematic distribution of electric signals for deep breathing, snoring and coughing. | |
3. Conclusions
In this work, a GCNP sponge sensor with satisfactory softness, excellent fatigue resistance, and high sensitivity was prepared by embedded printing. By investigating different printing conditions, the embedded assembly of GO on sponges was modulated, leading to the construction of complex circuit patterns. The embedded printing of GCNP sponge retains the porous nature of the sponge, allowing it to withstand large deformations and repeated compression, demonstrating excellent compressive and fatigue resistance. The printed GO ink formed an embedded conductive structure with three-dimensional interconnections using a hydrophilic porous sponge as a template, significantly increasing the sensitivity of the GCNP sponge sensor and enabling it to monitor small pressure changes. The obtained sensors with comfort, stability and pressure sensitivity could be easily integrated into pillows for real-time monitoring of sleep positions and sleep breathing processes. The sensor arrays could be easily prepared by printing, thus enabling simultaneous monitoring of multi-dimensional pressure changes. Further, the sensors can be integrated into the pillow, achieving real-time monitoring of sleep posture and sleep breathing process, further advancing the development of sleep medicine and health management.
4. Experimental section
4.1 Preparation of GO ink
GO was synthesized following previously reported work.53 The GO dispersion was then concentrated in a water bath at 60 °C. The solid loadings of the paste were calculated through thermogravimetric analysis. Subsequently, the paste was diluted with deionized water in varying amounts to obtain GO inks with different concentrations.
4.2 Preparation of GCNP sponge
Patterned GO composite sponges are printed using a multi-axis dispensing system (2400, EFD). A syringe loaded with GO ink was attached to a micro-nozzle (with diameter of 200 μm). By applying the appropriate pressure using an aerodynamic fluid dispenser, the GO ink was printed onto the sponge substrate following a predetermined path. The appropriate printing parameters are 7.5 psi and 10 mm s−1 in pressure and speed, respectively. The printed patterns were air-dried for 12 hours to allow the penetration and assembly of GO. Subsequently, they were vacuum-dried at 200 °C for 3 hours to obtain reduced graphene oxide (rGO) patterns.
4.3 Assembly of GCNP sponge pressure sensors
The GCNP sponge is seamlessly integrated onto a skeletonized PET (polyethylene terephthalate) sheet to expose the printed circuit pattern. The skeletonized PET sheet used as spacer was processed by laser cutting machine (Guava-LM-nps). The bottom layer was encapsulated with a PET sheet containing interdigital silver electrodes, which served as current collectors.
4.4 Characterization
The SEM images were obtained on a Phenom Nano proX system. The compressive properties of the GCNP sponge were tested by a universal testing machine (Suns Technology, UTM6103) at a speed of 50 mm min−1. The current signals were measured by a digital source meter (Keithley 2450) for the single sensor and a self-build multi-channel data acquisition system for the integrated sensing arrays. The measurements of sleep monitoring were recorded from a healthy subject (27-year-old male, 80 kg, 180 cm).
Author contributions
Wenbo Li put forward the methodology and carried out the writing. Jing Liu, Zhiyuan Sun, Jing Li, Jiawei Wang, Xintao Wu and Jiongli Li carried out the investigation, data curation and validation. Jiabing Zhang revised the paper and supplemented the experimental data. Teng Han and Meng Su provided academic guidance and experimental design suggestions. Xudong Wang carried out the project administration. Zhandong Huang carried out the supervision and formal analysis.
Conflicts of interest
There are no conflicts to declare.
Acknowledgements
This work was supported by National Natural Science Foundation of China (Grant No. 2230050342, 52222313, 52321006, T2394480, T2394484 and 52203247), the National Key R&D Pro-gram of China (Grant No. 2023YFE0111500, 2023YFC3040900, 2021YFB3200701).
References
- C. M. Barnes and N. F. Watson, Sleep Med. Rev., 2019, 47, 112–118 CrossRef PubMed.
- L. Hale, W. Troxel and D. J. Buysse, Annu. Rev. Public Health, 2020, 41, 81–99 CrossRef.
- M. R. Irwin, Nat. Rev. Immunol., 2019, 19, 702–715 CrossRef CAS PubMed.
- C. M. Swanson, W. M. Kohrt, O. M. Buxton, C. A. Everson, K. P. Wright, E. S. Orwoll and S. A. Shea, Metabolism, 2018, 84, 28–43 CrossRef CAS PubMed.
- A. M. Gordon, B. Carrillo and C. M. Barnes, Sleep Med. Rev., 2021, 57, 101428 CrossRef PubMed.
- D. Freeman, B. Sheaves, F. Waite, A. G. Harvey and P. J. Harrison, Lancet Psychiatry, 2020, 7, 628–637 CrossRef PubMed.
- X. Li, Q. Xue, M. Wang, T. Zhou, H. Ma, Y. Heianza and L. Qi, Circulation, 2021, 143, 97–99 CrossRef PubMed.
- X. Li, T. Zhou, H. Ma, T. Huang, X. Gao, J. E. Manson and L. Qi, J. Am. Coll. Cardiol., 2021, 78, 1197–1207 CrossRef PubMed.
- G. N. Nadkarni, Sci. Transl. Med., 2020, 12, eabe8124 CrossRef.
- E. Tobaldini, G. Costantino, M. Solbiati, C. Cogliati, T. Kara, L. Nobili and N. Montano, Neurosci. Biobehav. Rev., 2017, 74, 321–329 CrossRef PubMed.
- Y. Liu, Q. Yu, X. Luo, L. Ye, L. Yang and Y. Cui, Research, 2022, 2022 CAS.
- M. R. Cowie, D. Linz, S. Redline, V. K. Somers and A. K. Simonds, J. Am. Coll. Cardiol., 2021, 78, 608–624 CrossRef PubMed.
- H. A. Chami, H. E. Resnick, S. F. Quan and D. J. Gottlieb, Circulation, 2011, 123, 1280–1286 CrossRef PubMed.
- R. F. Gottesman, P. L. Lutsey, H. Benveniste, D. L. Brown, K. M. Full, J.-M. Lee, R. S. Osorio, M. P. Pase, N. S. Redeker, S. Redline and A. P. Spira, Stroke, 2024, 55, e61–e76 CrossRef.
- E. L. McGlinchey, J. A. Reyes-Portillo, J. B. Turner and L. Mufson, Child and Adolescent Mental Health, 2017, 22, 96–99 CrossRef PubMed.
- Y. Huang, X. Fan, S.-C. Chen and N. Zhao, Adv. Funct. Mater., 2019, 29, 1808509 CrossRef.
- J. Liu, W. Li, J. Li, K. Wang, Y. Wen, J. Wang, B. Zhang, J. Li, X. Wang and Z. Huang, Adv. Mater. Technol., 2024, 2302169 CrossRef CAS.
- Z. H. Guo, Z. Zhang, K. An, T. He, Z. Sun, X. Pu and C. Lee, Research, 2023, 6, 0154 CrossRef PubMed.
- R. Liu, Y. Liu, Y. Cheng, H. Liu, S. Fu, K. Jin, D. Li, Z. Fu, Y. Han, Y. Wang and Y. Tian, Adv. Funct. Mater., 2023, 33, 2308175 CrossRef CAS.
- K. Meng, X. Xiao, W. Wei, G. Chen, A. Nashalian, S. Shen, X. Xiao and J. Chen, Adv. Mater., 2022, 34, 2109357 CrossRef CAS PubMed.
- Z. Sun, Q. Ou, C. Dong, J. Zhou, H. Hu, C. Li and Z. Huang, Exploration, 2024, 20220167 CrossRef.
- F. Niu, F. Zhou, Z. Wang, L. Wei, J. Hu, L. Dong, Y. Ma, M. Wang, S. Jia, X. Chen and Z. Tong, Research, 2023, 6, 0100 CrossRef CAS PubMed.
- R. Qin, J. Nong, K. Wang, Y. Liu, S. Zhou, M. Hu, H. Zhao and G. Shan, Adv. Mater., 2024, 2312761 CrossRef PubMed.
- X. Wang, J. Yu, Y. Cui and W. Li, Sens. Actuators, A, 2021, 330, 112838 CrossRef CAS.
- H. Kou, H. Wang, R. Cheng, Y. Liao, X. Shi, J. Luo, D. Li and Z. L. Wang, ACS Appl. Mater. Interfaces, 2022, 14, 23998–24007 CrossRef CAS PubMed.
- Z. Lin, J. Yang, X. Li, Y. Wu, W. Wei, J. Liu, J. Chen and J. Yang, Adv. Funct. Mater., 2018, 28, 1704112 CrossRef.
- M. Cao, J. Su, S. Fan, H. Qiu, D. Su and L. Li, Chem. Eng. J., 2021, 406, 126777 CrossRef CAS.
- Y. Ding, T. Xu, O. Onyilagha, H. Fong and Z. Zhu, ACS Appl. Mater. Interfaces, 2019, 11, 6685–6704 CrossRef CAS.
- L. Qiu, M. Bulut Coskun, Y. Tang, J. Z. Liu, T. Alan, J. Ding, V.-T. Truong and D. Li, Adv. Mater., 2016, 28, 194–200 CrossRef CAS.
- W. Li, J. Liu, D. Liu, J. Li, J. Wang, J. Li, X. Wang, M. Su, C. Li and Y. Song, J. Mater. Chem. C, 2023, 11, 10834–10842 RSC.
- L. Qiu, J. Z. Liu, S. L. Y. Chang, Y. Wu and D. Li, Nat. Commun., 2012, 3, 1241 CrossRef PubMed.
- H. Wang, W. Lu, J. Di, D. Li, X. Zhang, M. Li, Z. Zhang, L. Zheng and Q. Li, Adv. Funct. Mater., 2017, 27, 1606220 CrossRef.
- F. Wu, P. Hu, F. Hu, Z. Tian, J. Tang, P. Zhang, L. Pan, M. W. Barsoum, L. Cai and Z. Sun, Nano-Micro Lett., 2023, 15, 194 CrossRef CAS.
- X. Chen, H. Liu, Y. Zheng, Y. Zhai, X. Liu, C. Liu, L. Mi, Z. Guo and C. Shen, ACS Appl. Mater. Interfaces, 2019, 11, 42594–42606 CrossRef CAS PubMed.
- H. Liu, X. Chen, Y. Zheng, D. Zhang, Y. Zhao, C. Wang, C. Pan, C. Liu and C. Shen, Adv. Funct. Mater., 2021, 31, 2008006 CrossRef CAS.
- W. Yang, H. Liu, H. Du, M. Zhang, C. Wang, R. Yin, C. Pan, C. Liu and C. Shen, Sci. China Mater., 2023, 66, 2829–2842 CrossRef CAS.
- M. Zhang, W. Yang, Z. Wang, H. Liu, R. Yin, C. Liu and C. Shen, Appl. Phys. Lett., 2023, 122, 043507 CrossRef CAS.
- Y. Zheng, R. Yin, Y. Zhao, H. Liu, D. Zhang, X. Shi, B. Zhang, C. Liu and C. Shen, Chem. Eng. J., 2021, 420, 127720 CrossRef CAS.
- J. Zhao, Q. Guo, X. Wang, H. Xie and Y. Chen, Colloids Surf., A, 2016, 488, 93–99 CrossRef CAS.
- C. Liu, Q. Tan, Y. Deng, P. Ye, L. Kong, X. Ma, L. Xu, Y. Xu, Q. Qiang, W. Chen, Y. Zhong, Y. A. Nikolaevich, Z. Zhao, W. Zhou and B. Liu, Compos. Commun., 2022, 32, 101178 CrossRef.
- Z. Sun, C. Dong, B. Chen, W. Li, H. Hu, J. Zhou, C. Li and Z. Huang, Small, 2023, 19, 2303612 CrossRef CAS PubMed.
- M. Wang, J. Zhu, Y. Zi and W. Huang, ACS Appl. Mater. Interfaces, 2021, 13, 47302–47312 CrossRef CAS PubMed.
- G. Ge, Y. Cai, Q. Dong, Y. Zhang, J. Shao, W. Huang and X. Dong, Nanoscale, 2018, 10, 10033–10040 RSC.
- L. Yang, Y. Liu, C. D. M. Filipe, D. Ljubic, Y. Luo, H. Zhu, J. Yan and S. Zhu, ACS Appl. Mater. Interfaces, 2019, 11, 4318–4327 CrossRef CAS PubMed.
- X. Chen, D. Zhang, H. Luan, C. Yang, W. Yan and W. Liu, ACS Appl. Mater. Interfaces, 2023, 15, 2043–2053 CrossRef CAS PubMed.
- Y. Yin, Y. Wang, H. Li, J. Xu, C. Zhang, X. Li, J. Cao, H. Feng and G. Zhu, Chem. Eng. J., 2022, 430, 133158 CrossRef CAS.
- W. Cao, Y. Luo, Y. Dai, X. Wang, K. Wu, H. Lin, K. Rui and J. Zhu, ACS Appl. Mater. Interfaces, 2023, 15, 3131–3140 CrossRef CAS PubMed.
- K. Li, W. Yang, Z. Shen, X. Zhang and M. Yi, Sens. Actuators, A, 2023, 354, 114266 CrossRef CAS.
- F. Wang, W. Zhang, Y. Song, X. Jiang and N. Sun, ACS Appl. Electron. Mater., 2023, 5, 6704–6715 CrossRef CAS.
- X. Lü, T. Yu, F. Meng and W. Bao, Adv. Mater. Technol., 2021, 6, 2100248 CrossRef.
- H. Yu, Y. Liu, G. Zhou and M. Peng, ACS Sens., 2023, 8, 4391–4401 CrossRef CAS PubMed.
- L. Li, Y. Cheng, H. Cao, Z. Liang, Z. Liu, S. Yan, L. Li, S. Jia, J. Wang and Y. Gao, Nano Energy, 2022, 95, 106986 CrossRef CAS.
- W. Li, F. Li, H. Li, M. Su, M. Gao, Y. Li, D. Su, X. Zhang and Y. Song, ACS Appl. Mater. Interfaces, 2016, 8, 12369–12376 CrossRef CAS PubMed.
|
This journal is © The Royal Society of Chemistry 2024 |
Click here to see how this site uses Cookies. View our privacy policy here.