DOI:
10.1039/D3NR06672J
(Review Article)
Nanoscale, 2024,
16, 10880-10900
Dynamic 3D in vitro lung models: applications of inorganic nanoparticles for model development and characterization
Received
30th December 2023
, Accepted 6th May 2024
First published on 13th May 2024
Abstract
Being a vital organ exposed to the external environment, the lung is susceptible to a plethora of pathogens and pollutants. This is reflected in high incidences of chronic respiratory diseases, which remain a leading cause of mortality world-wide and pose a persistent global burden. It is thus of paramount importance to improve our understanding of these pathologies and provide better therapeutic options. This necessitates the development of representative and physiologically relevant in vitro models. Advances in bioengineering have enabled the development of sophisticated models that not only capture the three-dimensional architecture of the cellular environment but also incorporate the dynamics of local biophysical stimuli. However, such complex models also require novel approaches that provide reliable characterization. Within this review we explore how 3D bioprinting and nanoparticles can serve as multifaceted tools to develop such dynamic 4D printed in vitro lung models and facilitate their characterization in the context of pulmonary fibrosis and breast cancer lung metastasis.
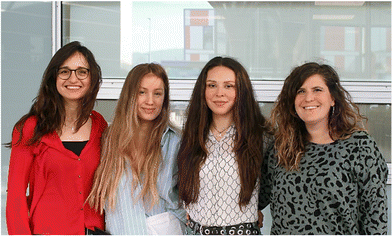 From left to right in the image: Amaia Cipitria, Laura Fallert, Ane Urigoitia-Asua, Dorleta Jimenez de Aberasturi |
Dorleta Jimenez de Aberasturi is an Ikerbasque Research Fellow leading the Hybrid Biofunctional Materials group at CIC biomaGUNE. She received a joint PhD from Philipps University of Marburg and University of the Basque Country. She has broad expertise in hybrid nanostructured materials for bio-applications. Recently she focused her interests on biofabrication of 3D in vitro models. Amaia Cipitria is an Ikerbasque Research Associate at IIS Biogipuzkoa and leads the group Bioengineering in Regeneration and Cancer. Her group aims to understand how biophysical and biochemical properties of native extracellular matrix and synthetic biomaterials guide cell response in tissue regeneration, cancer dormancy and bone metastasis. Amaia did her PhD at the University of Cambridge, was a postdoc at QUT Brisbane and Charité University Hospital Berlin, and an Emmy Noether group leader at the Max Planck Institute of Colloids and Interfaces. Ane Urigoitia-Asua is a PhD student working on the development and characterization of 4D-printed in vitro alveolar models. Laura Fallert is a PhD student working on 3D printed breast cancer models to monitor drug response and investigate lung metastatic events, aiming to develop a platform for personalized therapy. |
Introduction
The lung represents a vital organ, exposed to the external environment and is responsible for the uptake of oxygen and elimination of carbon dioxide from the bloodstream, while simultaneously also providing a functional barrier which prevents the crossing of bacteria, viruses and airborne environmental pollutants. Being in continuous contact with an array of pathogens, makes lungs susceptible to a variety of pathologies referred to as chronic respiratory diseases. These include among others pulmonary fibrosis, lung cancer, asthma as well as chronic obstructive pulmonary diseases, which combined represent the third leading cause of death worldwide.1 Considering the essential functions lungs exert, it is imperative to improve our understanding of these pathologies to preserve and regenerate healthy lung tissue and to develop efficient treatments for unresolved lung diseases. This, in turn, necessitates the development of advanced physiologically relevant in vitro models. 3D in vitro cell models are increasingly becoming the standard, as opposed to traditional 2D cultures, and their ever-increasing complexity is finally allowing them to be valuable tools for preclinical studies. Whilst significant advances have been made to represent various diseases affecting distinct organs, organs that display high levels of complexity require further scrutiny to develop realistic models that faithfully represent the in vivo environment. This particularly applies the modelling of lungs, which due to their intricate architecture remain a rather underrepresented organ.2 Given the prevalence and severity of pulmonary diseases, biomimetic 3D lung models hold promise to pave the way to investigate their etiology, as well as to accelerate drug design and discovery.3 This notion is supported by the high attrition rates of drugs in clinical trials due to a lack of efficacy and safety, indicating the poor prediction value of conventional preclinical models.3–6 In addition, an ever-increasing body of evidence further highlights the enhanced prediction value of 3D over 2D models.7,8 Even if important advances have already been achieved,9 modelling anatomically accurate regions of human lungs and lung diseases in vitro in 3D poses a plethora of challenges. One of these challenges is the choice of suitable biomimetic materials to capture the mechanical and biochemical properties of native lung tissue. Likewise, the scarcity of available lung tissue and invasive nature of obtaining cells from human donors, further narrow down the tools to develop representative lung models. However, alternatives such as lung cells derived from human pluripotent stem cells (hPSCs) hold great potential to address this issue.10 In addition, as the influence of biophysical forces on cell behaviour has become more and more evident, it is imperative to acknowledge and incorporate the physical stimuli to which cells within the lung are exposed to.11,12 In this regard, the concept of four dimensional (4D) in vitro modelling has emerged, which accounts for responses to a given stimuli in the context of a 3D model setup, resulting in a change over time, thereby representing a fourth dimension.13 Progress has been made in this field not only via bioprinting but also by means of bioreactors, including perfusion bioreactors, rotating vessels, orbital shakers as well as microfluidic chips.11,14 However, these constructs are often still not standardized, which in turn can result in variations in the experimental configurations, translating into poor reproducibility and throughput.15 Related to this, it is important to note that a significant challenge involves the characterization of 3D lung models, as common assays developed for the traditional 2D culture systems are not readily applicable to 3D. On that note, it should also be mentioned that conventional techniques such as confocal microscopy or histology, pose certain drawbacks, including limited penetration depth, destructive procedures, spectral overlap as well as low sensitivity and specificity, which become more pronounced in 3D systems.16,17 While these remain undeniably important and valuable techniques, additional characterization methods are required to potentiate information retention from 3D models. It is important to establish consensus on treating 3D models as biological tissue, which would benefit from in vivo modalities tailored to an in vitro scale to be properly characterized. Numerous endeavours are underway to develop multimodal 3D imaging techniques combined with machine learning to improve 3D model characterization,18 which nonetheless still falls short of being a flawless solution. In addition, non-invasive and real-time monitoring is often limited when combining 3D models with the aforementioned bioreactors. The incorporation of high-resolution imaging modalities with continuous flow bioreactor-based cultures, is not straightforward, where gathering information often requires the disruption of the culture at a given timepoint. While microfluidic devices are generally compatible with high-resolution microscopy, readouts heavily rely on end-point assays, with certain assays necessitating the de-construction of the devices.19 Hence, while bioreactors generally offer numerous advantages, in certain instances they can potentially complicate and narrow down the variability of assays or read-outs that can be performed and obtained within such a setting. Thus, developing alternative imaging methods is of high interest. In face of these observations, it becomes clear that tackling the above-mentioned challenges remains an essential chore to gain insight into underlying disease mechanisms and pivot the development of more effective therapeutic strategies.
Nanoparticles (NPs) embody a promising versatile tool to address some of these challenges. Based on their composition, they are broadly categorized into organic NPs, which are mainly carbon based, such as carbohydrates, proteins, lipids, polymers, etc. and inorganic NPs, including metals, ceramics and semiconductors.20 Opposed to their non-nano counterparts, NPs display unique physical and chemical properties which find broad applications within the field of biomedicine.21,22 In the context of lung models, NPs have primarily gained increasing popularity as drug delivery agents or for toxicity studies.23–25 However, they harbor an unprecedented potential to advance the development of 3D in vitro models. In this context, emerging evidence demonstrates how incorporating inorganic NPs within a given biomaterial can enhance the biocompatibility and provide means of tuning the mechanical properties of such biomaterial.21 Moreover, depending on their composition, their magnetic (magnetic NPs),26 photothermal conversion (plasmonic NPs),27 thermal28 or electroconductive29 properties can be exploited to introduce biophysical stimuli, thereby circumventing the need of complicated bioreactor setups.30–33 For instance, NP-based photothermal conversion within a thermo-responsive polymer can yield expansion or contraction of the biomaterial upon irradiation with an incident laser.34 Finally, they can serve as versatile non-invasive characterization agents with a broad range of analytes that can be identified with high specificity and sensitivity.35–38
This review provides an overview of established 3D in vitro lung models, with a focus on pulmonary fibrosis and breast cancer lung metastasis to elaborate on recent progress and outline perspectives on future models. Within this framework we will discuss how inorganic NPs can be exploited to advance the development of dynamic biomimetic 3D in vitro lung models. Lastly, we will elaborate on the use of NP-based characterization techniques of such models and highlight their benefits in comparison to conventional techniques. We have summarized in Fig. 1 the main sections and subsections discussed within this review.
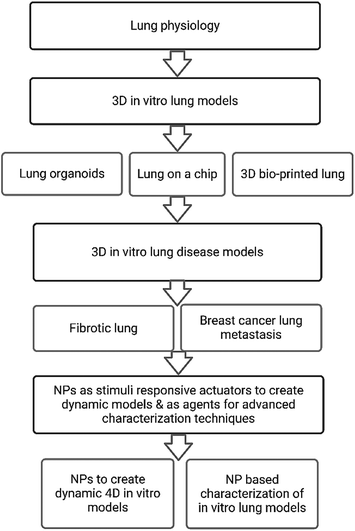 |
| Fig. 1 Overview of discussed topics and subsections within this review. | |
Lung physiology
The lung is a complex organ composed of different cell types such as epithelial, endothelial, mesenchymal, smooth muscle, and immune cells as well as fibroblasts (Fig. 2).39 Generally, one distinguishes between proximal upper airways, which include the trachea as well as bronchi, and distal lower airways, which include the bronchioles and alveoli.40,41 The alveoli are surrounded by a dense network of capillaries, separated by a thin basement membrane. Together this constitutes the barrier where gas exchange occurs and is referred to as the air–liquid interface (ALI).8,42 The intricacy of this region is of vital importance, not only to facilitate the uptake of oxygen and elimination of carbon dioxide, but also to prevent the breaching of inhaled pollutants or pathogens. Hence, this area is specifically susceptible to various chronic lung diseases such as idiopathic pulmonary fibrosis, cystic fibrosis or lung emphysema, and represents the target site of lung cancer and metastasis.43–45 Thus, within this review we will focus primarily on the alveolar region and ALI of the lung. The alveoli are air cavities separated by a thin honeycomb-shaped epithelium, whose diameter range from 150–200 μm in healthy and young individuals, up to 250–500 μm in older individuals.41,46,47 They occupy a large area in the lungs (over 100 m2 in adults) in order to obtain an efficient gas exchange.42,48 The alveolar epithelial lining consists of type I (ATI) and type II (ATII) pneumocytes,49 which form tight junctions and thereby constitute a vital component of the barrier within the air–liquid interface.50,51 ATI cells are squamous, cover more than 90% of the alveolar surface and they partake in the gas exchange process.52,53 ATII cells are cuboidal, produce pulmonary surfactant, metabolize drugs, repair the epithelial layer by proliferating and differentiating into ATI cells, and are critical in the immune response against pathogens.49,54,55 The alveoli are surrounded by the basement membrane, a thin (<2 μm), specialized extracellular matrix (ECM) composed of fibres of type IV collagen, laminin and proteoglycans.56,57 Capillaries are in close contact with the alveoli on the other side of the basement membrane (BM),58 allowing the diffusion of small gas molecules (O2, CO2) while avoiding the entrance of bigger molecules or microorganisms, due to the selective permeability of the epithelium and BM.56,57 Apart from the BM, an additional type of ECM surrounds the inter-alveolar spaces. The primary constituents of this ECM are collagen fibres (collagen type I, II, III, V and IX) and elastin, which provide elasticity and mechanical strength.59–61 Other constituents include fibronectin, proteoglycans and glycosaminoglycans, which further maintain structural support, cell adhesion and local water homeostasis.60,62 In terms of cellular constituents, this ECM hosts fibroblasts which maintain and remodel the surrounding ECM including the BM. In addition, local immune cells, mainly alveolar macrophages, are located here to provide a first line of defence against potential pathogens.63 As already indicated, the ECM acts as a support for the tissue, by aiding cell adhesion, proliferation, and guiding migration, while also providing elasticity and mechanical strength to the tissue. The maintenance of these mechanical properties is essential, as breathing requires the alveoli and surrounding ECM to stretch in order to accommodate the extra air volume.64 This in turn requires a certain degree of elasticity of the tissue, with a reported Young's modulus of 1–5 kPa.61,65,66 Importantly, breathing-induced mechanical stretching has been demonstrated to be a vital stimulus for the correct functioning of the lung, as it initiates the synthesis and release of pulmonary surfactant by ATII cells.67 Together this emphasizes the importance of taking the above discussed physiological characteristics of the lung into account, including an ALI as well as dynamic stretching, when aiming to develop physiologically relevant in vitro models. In the following sections we will elaborate how, and to which extent available studies facilitate the incorporation of various of these characteristics.
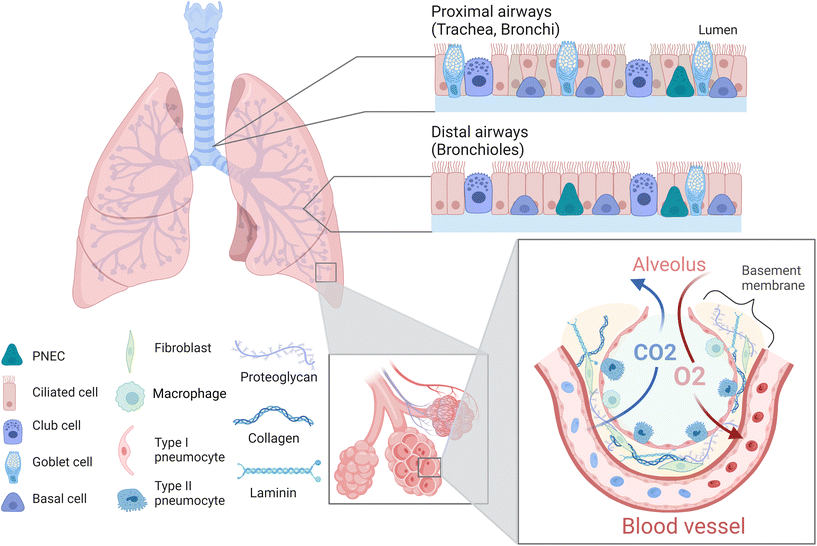 |
| Fig. 2 The proximal airways (trachea, bronchi) bifurcate into the distal airways (bronchioles) and are lined by columnar ciliated cells, mucus-secreting goblet cells, homeostasis-maintaining club cells, pulmonary neuroendocrine cells and regenerative basal cells. The alveoli are lined by type I and type II pneumocytes and also host immune cells such as macrophages. They are surrounded by a dense network of capillaries, separated by a thin basement membrane composed of collagens, proteoglycans and laminins. Together this represents the air-liquid interface, where carbon dioxide is eliminated, and oxygen is taken up by the bloodstream. PNEC, pulmonary neuroendocrine cells; CO2, carbon dioxide; O2, oxygen. This illustration was created with Biorender adapted from “Respiratory Epithelium” and “Alveolar Capillary Barrier” by Biorender 2023. | |
3D in vitro lung models
In an effort to bridge 2D in vitro and animal-based in vivo models, increasing attention has been dedicated towards the establishment of human in vitro 3D lung models to study chronic lung diseases. Not only is the use of animal models inherently tied to considerable ethical concerns but also suffers from interspecies differences and hence provide a poor prediction of human responses.71,76 As for in vitro models, it is well acknowledged that, opposed to 3D, 2D cultures oversimplify the in vivo environment due to the lack of representative cell–cell and cell–ECM interactions present within native tissue.77 As mentioned before, within the native lung tissue, cells are exposed to mechanical and physical cues such as compression or shear stress, and are arranged in a specific geometric and dimensional order.78–80 Such stimuli are involved in cell motility, proliferation as well as differentiation, and are vastly absent in 2D culture models. This highlights the need for shifting from conventional 2D culture towards the standardization of 3D culture models, aiming to capture such complex tissue characteristics.76,81 Specifically, in the context of modelling in vitro alveoli and an air–liquid interface (ALI), several factors need to be considered: (i) mimicking the mechanical properties (stiffness, elasticity) of the tissue is essential to achieve a mature, in vivo-like tissue, as mechanical cues evidently determine cell behavior.79,80,82 The selection of biomaterials to generate the models is crucial for that purpose. (ii) imitating the morphology of the native tissue is important. Apart from the arrangement between the alveolus and capillaries, the model should be incubated between air (epithelial side) and liquid (endothelial side), as the correct functioning of the ALI depends on this environmental arrangement.49,83 (iii) the dynamism of the model, imitating breathing motions is also sought, in order to obtain a fully functioning in vitro model with pulmonary surfactant production.67 Several methods have been used to generate 3D in vitro lung models, including organoids, Lung-on-a-Chip (LoC) systems and 3D-bioprinted models, with each of them offering various benefits but also displaying certain drawbacks. A summary is presented in Table 1. Among them, human organoids are self-organized 3D culture systems generally derived from stem cells that mimic the architecture and function of real organs, which have emerged as a powerful tool.84,85 The development of organoid technology is still in its infancy with challenges to overcome.86 To date, the most studied models incorporating dynamic features and ALI conformation are mostly based on Lung-on-a-chip (LoC) devices.11 More recently, 3D bioprinting techniques have also gained importance, demonstrating significant utility in this direction by achieving organized, layered models (Fig. 3). Certainly, more combinations of all techniques will likely begin to emerge. LoCs generally consist of a top and a bottom chamber separated by a thin, porous membrane acting as a basement membrane, with endothelial cells seeded within the bottom chamber and alveolar epithelial cells in the top chamber.87,88 The bottom chamber is perfused with medium whereas the top chamber is ventilated to achieve an ALI. Additionally, they include two side chambers through which a cyclic vacuum can be applied, stretching the central chamber, and providing dynamism. Using this approach, Huh et al.87 created a LoC model by seeding alveolar epithelial cells on the top and human pulmonary microvascular endothelial cells at the bottom of a ECM-coated (fibronectin or collagen) 10 μm thick PDMS membrane (Fig. 3A). They observed that culturing the chips in ALI, opposed to liquid culture, increased the electrical resistance (TEER) and decreased the transport of albumin molecules across the membrane, indicating a better barrier permeability similar to in vivo (Fig. 3B). The authors also demonstrated the influence of breathing motions on the transport of 20 nm fluorescent NPs across the ALI, where applying 10% cyclic strain at 0.2 Hz significantly increased the diffusion/transport of the NPs from the air to the liquid phase, which did not occur neither in the static model nor in a transwell system (Fig. 3C). The cyclic mechanical strain also led to an increased production of pulmonary surfactant.89 Varone et al.88 developed a similar chip where the ALI was separated by a 50 μm PDMS membrane with 7 μm diameter pores, and fibroblasts embedded in a 200 μm thick type I collagen hydrogel between the endothelial and the epithelial layers (Fig. 3D). The constructs were cultured on a liquid–liquid interface (LLI) until the alveolar cells created a monolayer and were then subsequently cultured in an ALI. They concluded that, apart from the ALI culture, the cyclic strain (15% strain, 0.2 Hz) significantly increased the production of pulmonary surfactant (Fig. 3E). Additionally, Zamprogno et al.47 developed a LoC system which recreated the appearance and organization of the in vivo alveolus by adding a stretchable elastin-collagen (EC) coating to a honeycomb-shaped gold mesh, where alveolar and endothelial cells were seeded on top and the bottom of the EC membrane respectively (Fig. 3F). They demonstrated that this membrane was more flexible than the widely used PDMS membranes. The chip allowed the permeability for smaller hydrophilic molecules (FITC-sodium, 0.4 kDa) but not for bigger molecules (RITC-dextran, 70 kDa). The same phenomenon was observed by Stucki et al.73 in a dynamic chip, where bronchial epithelial cells (16HBEo-14) and endothelial cells (pHUVEC) were seeded in a 10 μm thin PDMS membrane with 8 μm diameter pores. Moreover, they compared the permeability of the system with or without stretching (10% strain, 0.2 Hz), concluding that the permeability of bigger molecules (RITC-dextran) was not affected by the strain, while smaller molecules (FITC-sodium) exhibited relative increase in permeability of 46% (Fig. 3G). This indicated the importance of breathing forces for the transport of small molecules across the alveolar barrier. While LoC systems are able to mimic breathing motions and provide means to assess alveolar barrier permeability, they fail to fully embody the complexity necessary to study the mechanisms occurring within the lung tissue, beyond barrier interactions.75 Given that these constructs primarily consist of cell monolayers seeded on a membrane, they generally lack the ECM. As already mentioned, the alveolar ECM provides mechanical cues that determine cell behavior.79,80,82 Thus, the inclusion of ECM in such models is important especially in the context of disease models, as tissue stiffness as well as certain ECM components are known to induce the diseased phenotype.90,91 In light of this lack of complexity, LoC systems have been described as 2.5D cultures, rather than 3D cultures.92
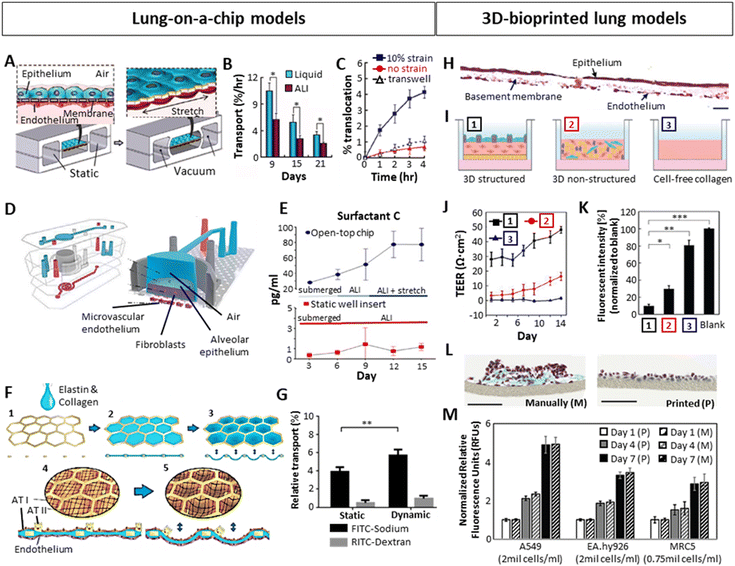 |
| Fig. 3 Importance of ALI, dynamic stretching and layered organization on the development of 3D in vitro lung with focus on the alveolar region, tested in Lung-on-a-chip (A–G) or bioprinted (H–M) models. (A) Schematic example of a LoC with the epithelial and endothelial layers separated by a thin membrane, cultured in ALI and stretched via vacuum through its side chambers.87 (B) Improved barrier function of ALI culture over liquid culture, showing a lower albumin transport through the epithelial-endothelial layers. (C) Increased barrier permeability for 20 nm fluorescent particles after applying 10% cyclic strain on the chip. (D) A LoC including lung epithelial cells, fibroblasts in a collagen type I hydrogel and endothelial cells in ALI culture.88 (E) Increased production of lung surfactant after introducing 15% cyclic strain. (F) LoC approach mimicking the native alveolar structure using a honeycomb-shaped gold mesh with a collagen-elastin coating, where cyclic strain could be applied via vacuum at the bottom part of the model.47 (G) Low barrier permeability of big molecules (dextran) and higher permeability of small molecules (sodium), which was significantly higher under 10% cyclic strain.73 (H) Cross-section of a 3D-inkjet printed alveolar model stained with Hematoxylin–Eosin, containing endothelial cells (bottom), fibroblasts embedded in collagen I (middle, basement membrane), and alveolar epithelium (top). Scale bar: 20 μm.8 (I) Schematic representation of the structured model shown in H [1], a non-structured model consisting of a blend of fibroblasts, epithelial and endothelial cells embedded in collagen [2], and a cell-free collagen layer [3]. (J and K) Comparison of TEER (J) and barrier permeability (K) between the 3 models schematically represented above, showing a better barrier function in the 3D organized structure. (L) Comparison by Horvath et al.93 between manual pipetting vs inkjet-printing of a multilayered Matrigel – endothelial cell – Matrigel – epithelial cell system, 3 days after seeding. Masson Goldner trichrome staining of a cross-section is shown. Scale bars: 100 μm. (M) Effect of 3D printing on cell viability compared to manual pipetting.94 (A–C) Reproduced with permission from ref. 87 Copyright 2010 Science (D and E) Reproduced with permission from ref. 88 Copyright 2021 Elsevier (F) Reproduced with permission from ref. 47 Copyright 2021 Springer Nature (G) Reproduced from ref. 73 with permission from the Royal Society of Chemistry (H–K) Reproduced with permission from ref. 8 Copyright 2021 Advanced Science published by Wiley-VCH (L) reproduced with permission from ref. 93 Copyright 2015 Springer Nature (M) Reproduced with permission from ref. 94 Copyright 2021 Ng, et al. | |
Table 1 Features of organoids, LoC and 3D-bioprinted lung models. The table is focused on the degree of complexity of each model, and the three desired features mentioned when modelling ALI: similar mechanical properties as the native tissue, arrangement of the model in an air–liquid environment, and dynamism, imitating breathing motions
|
Organoids |
Lung-on-a-Chip (LoC) |
3D-bioprinting |
Preparation strategy |
Culture of stem, primary or tumoral cells in specific differentiation conditions to develop into different cell types from the lung |
Growth of epithelial (air) and endothelial (liquid) cells on opposite sides of a membrane in a microfluidic chip cultured in ALI |
Precise and controlled deposition of either cell droplets or cells embedded in a biomaterial, achieving a layered heterogeneous structure |
Heterogeneity |
✗ |
✓ |
✓ |
Clusters of cell types similar to in vivo |
A simplified version of the in vivo organ |
Printing of different cell types mimicking in vivo |
Complexity |
✗ |
✗ |
✓ |
Lack of vasculature and immune cells |
Use of microfluidics monitoring only certain features |
Print different cell types and biomaterials arranged in a complex manner |
Mechanical properties |
✗ |
✗ |
✓ |
Lack of supporting materials |
Cells without surrounding ECM |
Selection of supporting biomaterials imitating native properties |
Air–Liquid Interface |
✗ |
✓ |
✓ |
Lack of endothelium, they only mimic the “air” part |
Lung epithelial monolayer in an air chamber; endothelial monolayer in a perfused chamber; separated by a thin “basement” membrane |
Print epithelial cells on top in contact with air, rest of the cells below in contact with media |
Dynamism |
✗ |
✓ |
✗ |
Static models |
Stretchable “basement” membrane by vacuum |
In general, static models. Dynamism achievable printing stimuli-responsive materials |
Applications |
Disease modelling, drug screening or personalized medicine |
Disease modelling, studying cell–cell interactions or ALI barrier properties |
Complex organ modelling, drug screening |
References |
68–71
|
47, 72 and 73 |
8, 74 and 75 |
In order to achieve more complex models, 3D printing emerged as a promising tool for lung 3D modelling, enabling the spatial separation of the alveolar key compartments: endothelial (liquid) compartment, basement membrane and epithelial (air) compartment. A correct 3D organization has been demonstrated to improve the barrier function when compared to a lack of organization. Based on this strategy, Kang et al.8 inkjet-printed a three-layer alveolar barrier model to investigate the influence of membrane organization on barrier properties, with epithelium, a collagen basement membrane and endothelium. They achieved models of a thickness of 10 μm, containing alveolar type I and II cells (NCI-H1703 and NCI-H441 respectively), lung endothelial cells (HULEC-5a) and lung fibroblasts (MRC5) embedded in collagen I with a defined structure (Fig. 3H). Comparison of structured to unstructured models (all cell types co-encapsulated in collagen) or collagen alone (Fig. 3I), demonstrated a superior barrier function (TEER and FITC-dextran transport) of the structured models (Fig. 3J and K). This 3D organization can be more easily achieved via 3D printing. Horvath et al.93 demonstrated the use of inkjet 3D printing to achieve thin, reproducible layers as opposed to manual pipetting (Fig. 3L). Ng et al.94 compared the cell viability and proliferation of three main cell types in alveolar models (A549 lung epithelial cells, EA.hy926 endothelial cells and MRC5 lung fibroblasts) after inkjet printing and manual pipetting, reporting no significant differences (Fig. 3M).95 Taken together, an increasing body of evidence demonstrates the utility of LoC systems as well as 3D printing as attractive tools to develop sophisticated lung models. While LoC studies emphasized the relevance of ALI and dynamic culture conditions, they still oversimplify the dimensionality of the in vivo complexity. 3D printing on the other hand, can address these shortcomings and embodies a promising technique to develop physiologically relevant models. Ideally, by combining the advantages of both techniques and introducing human organoids, we may be able to overcome current limitations and create more realistic models.
3D in vitro lung disease models
While diseases affecting the lung can be of distinct etiologies, they often manifest in similar clinicopathological profiles, namely dyspnea (difficulty breathing), chest pain, chronic non-productive cough and hypoxia-induced fatigue.43,96 As already indicated within the framework of this review, emphasis is placed on pulmonary fibrosis and lung metastasis, representing two heterogenous and very distinct, yet highly prevalent diseases affecting millions of individuals world-wide and compromising lung function, specifically within the alveolar region.
3D in vitro models of fibrotic lung
Fibrosis in the lung occurs as a consequence of a variety of chronic respiratory diseases (CRDs) such as chronic obstructive pulmonary disease (COPD), cystic fibrosis (CF) or interstitial lung diseases (ILDs, a family of over 100 diseases of unknown etiology among which is idiopathic pulmonary fibrosis (IPF)).96 Some of these diseases have a high prevalence (COPD, around 10% of the population),97 whereas others progress rapidly (IPF, with a survival median of 3–5 years since diagnosis).98 Symptoms of CRDs vary depending on the disease, but generally include dyspnea, chronic cough, declining lung function and fatigue due to hypoxia.43,95,96 The cause of the diseases varies between genetic (CF), unknown (ILD) or age- and smoking-related (COPD).44,98,99
The development of realistic 3D models representing fibrotic lung is challenging but important to better understand the underlying mechanisms of it and find possible treatments. Fig. 4 summarizes some examples found in literature. Efforts have been made in this direction by creating organoids that represent fibrotic lung, for example, Surolia et al. generated 3D multicellular pulmospheres derived from healthy or IPF donors.100 These pulmospheres contained ATII cells, macrophages, endothelial cells and myofibroblasts, where the IPF phenotype was distinguished by increased α-SMA (a marker for myofibroblasts), collagen type I and IV (Fig. 4A). The authors measured the zone of invasion (% ZOI) of fibroblasts on the pulmospheres by subtracting the inner core area from the total (core + invaded) area. The ZOI was more prominent in the fibrotic models, especially in the organoids derived from end-stage patients, suggesting that they could reflect the in vivo condition. Moreover, the addition of transforming growth factor beta 1 (TGFβ1), a well-established fibrosis-inducing growth factor, induced fibroblast invasion (% ZOI) on healthy spheres (Fig. 4B), whereas the addition of the anti-fibrotic drug nintedanib (commonly used for pulmonary fibrosis treatments) reduced the invasion (% ZOI) on IPF spheres (Fig. 4C).
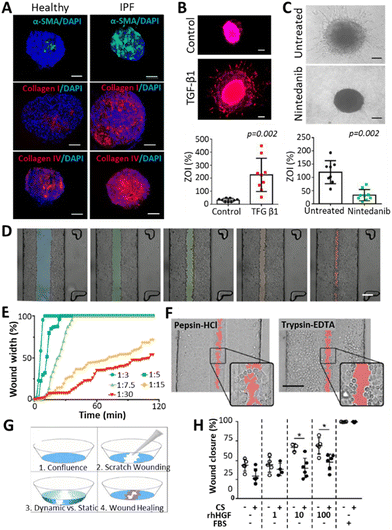 |
| Fig. 4 Representation of 3D models of fibrosis/wounding. (A) Organoids derived from healthy or IPF donors, presenting increased levels of fibrotic markers (α-SMA, Col I, Col IV) on the IPF pulmospheres. Scale bars: 250 μm.100 (B) Increased invasion of fibroblasts (measured by % zone of invasion or ZOI) on healthy spheres after treatment with a fibrosis inducer TGFβ1. Scale bars: 250 μm. (C) Decreased invasion of fibroblasts (% ZOI) on IPF spheres after treatment with an anti-fibrotic drug Nintedanib. Scale bars: 250 μm. (D and E) Wound formation observed by Felder et al.102 on A549 cell monolayers after applying different central:sheath flow rates of a 0.5% trypsin-EDTA solution. Differences were observed in wound size (D, scale bar: 200 μm.) and time for wound formation (E), where lower flow rates derived in smaller and slower wounding patterns. (F) Comparison of wounding patterns between a 2.5 mg ml−1 pepsin-HCl solution (left) and a 0.5% trypsin-EDTA solution. While trypsin-EDTA detached the cells from the surface, pepsin-HCl activated cell migration away from the solution. Scale bar: 200 μm.104 (G) Wound healing experiment after scratching A549 cell monolayers and applying different stimuli such as cyclic strain (10%, 0.2 Hz), presence of human hepatic growth factors (rhHGF, 0, 1, 10 or 100 ng ml−1) and 10% FBS as a positive control. (H) Impaired wound healing was observed with the cyclic strain (CS), and improved healing with the addition of rhHGF. | |
Lung-on-a-chip (LoC) devices have also been employed for modelling fibrosis. Mejías et al. seeded small airway epithelial cells (SAECs) in a central channel located between two side channels consisting of an endothelial/fibroblast cell mixture seeded on top of a fibrin gel.101 Here, the authors observed the interaction between endothelial cells/fibroblasts and lung epithelial cells, with an upregulation of fibrosis-promoting mechanisms upon adding TGFβ to the chip. However, the model failed to recapitulate a reduction of fibrosis when treated with pirfenidone, indicating that the chip required modifications to better represent the fibrotic phenotype. Felder et al.102 developed a microfluidic chip to study the epithelial wound formation (Fig. 4D–F) and healing (Fig. 4G and H) in the alveolar region. They evaluated the wound formation by directing a 0.5% trypsin-EDTA solution through a confluent monolayer of A549 cells, where the width of the wound and the time for its formation was dependent on the central-to-sheath flow rate of the solution (Fig. 4D and E). Afterwards, the effect of 2.5 mg ml−1 pepsin-HCl was compared to the effect of 0.5% trypsin-EDTA in the wound formation (Fig. 4F). Pepsin-HCl was used as an example of gastric contents, as the gastro-oesophageal reflux disease (GERD), a condition occurring when there is a retrograde flow of stomach contents, which can on some occasions be inhaled, is said to be correlated to the alveolar microinjuries deriving in fibrosis.103 The authors claimed that there were differences in the wounding mechanism of the two solutions: cells detached from the monolayer due to the trypsin solution, whereas they migrated away from the pepsin-HCL solution, as indicated by the cell protrusions that were not found in the trypsin-EDTA solution. Within the framework of a follow-up study, Felder et al. used a breathing LoC model to assess wound healing.104 In brief, wounds were introduced in the alveolar microfluidic models by scratching monolayers of A549 cells with a pipette tip to observe healing (Fig. 4G) under different conditions: the presence or absence of 10%, 0.2 Hz cyclic strain (CS), the addition of human hepatic growth factors (rhHGF) (0, 1, 10, 100 ng ml−1), and the addition of 10% FBS as a positive control. They demonstrated that the administration of rhHGF slightly improved the condition, whereas the mechanical strain significantly impaired wound healing (Fig. 4H). Considering that fibrosis can occur upon poor healing of microinjuries in the alveolar epithelium,105 and taking into account that breathing motions hinder the healing process (Fig. 4H), this suggests that motion might play a pivotal role during fibrosis initiation and progression in the lung. Thus, incorporating dynamism in alveolar models of fibrosis could be a key factor to study disease progression. Moreover, as the ECM plays an important role in the activation and progression of the disease, encapsulating cells in hydrogels imitating the ECM of healthy and fibrotic lung has been researched.77 Given that one of the key features of fibrosis is tissue stiffening, some researchers have modulated the hydrogel stiffness to mimic either healthy or fibrotic tissue.106 Moreover, the use of decellularized porcine lung extracellular matrix (lung dECM) offers the opportunity to mimic mechanical and functional properties of the native lung. Importantly, this approach is compatible with exogenous pulmonary cells107,108 and enables the generation of alveolar epithelium (with ATII cells differentiating into ATI cells).109 Moreover, hydrogels representing the components of healthy or fibrotic lung have been obtained by employing such lung dECM derived from healthy or fibrotic tissues.110 Unfortunately, while these hydrogels with encapsulated cells provide the necessary mechanical cues, they do not provide an ALI arrangement or dynamism, two other necessary factors for achieving effective in vitro lung models. Interestingly, these dECM hydrogels arranged in an ALI manner have been explored for healthy lung models by 3D printing,8,111 yet there are no such models available for fibrotic lung. One should also note that dECM based approaches are prone to suffer from batch-to-batch variability and provide limited control over their mechanical properties. Thus, chemically functionalizing dECM or combining dECM with tuneable biomaterials could potentiate their properties, which has been demonstrated across a wide range of dECM from distinct tissue sources.112 Lastly, the lack in dynamism of the above-described 3D-printed models should be taken into consideration. This challenge could be addressed via the ever-evolving technology of 4D printing, which contemplates the modification of 3D printed objects when an external stimulus is applied, resulting in controlled changes over time.
3D in vitro models of breast cancer lung metastasis
Breast cancer (BC) remains the leading cause of cancer related mortality in female's worldwide, with women primarily succumbing to metastatic outgrowth.113 Next to bones, lungs represent the second most common site of BC metastasis.114 Once BC cells start to colonize and actively grow within the lung, lung function progressively declines but whether or not and when active outgrowth occurs is highly variable across subtypes.115–117 Interestingly, recent evidence also indicates how not only subtype but also changes in lung ECM drive the activation and outgrowth of metastasized BC cells.118 While this remains a largely underexplored territory, it provides a rationale to capture the 3D context in which BC cells are embedded, to gain a comprehensive understanding of metastatic growth. In the past, BC lung metastasis has primarily been studied with in vivo models or reductionist in vitro 2D co-culture models, but lately more complex 3D bioengineered models have emerged, and hold promise to address the unmet need of identifying effective therapeutic strategies.118–124
Recently, Kundu and colleagues developed a sophisticated approach to model BC lung metastasis, based on a synthetic biomimetic PEG-hydrogel, functionalized with a peptide mixture to improve adhesion and cell-mediated ECM remodelling.125 For this purpose, the authors initially identified core adhesion and cleavable peptides present in healthy human lung ECM samples (Fig. 5A). A well-defined selection of these peptides was synthesized and incorporated in a tuneable PEG-based hydrogel. As a result, the authors presented a 10 wt% four-arm 10 kDa PEG-maleimide hydrogel with a stiffness of 1.5 ± 0.4 kPa, which matches the stiffness of previously measured porcine lungs (Fig. 5B and C). Initially, the function of the adhesion peptides was validated using human lung fibroblasts and BC cells. Later, the synthetic lung ECM served as a soil to investigate the crosstalk between these two cell types, identifying the synergistic activating potential they exert on each other, with Tenasin-C emerging as a relevant driver within this activation loop (Fig. 5D). Unfortunately, this was not investigated based on co-encapsulated cells, but rather based on a single encapsulated cell type exposed to conditioned media from another cell type. Thus, it would be of interest to extend the complexity of this model, evident on a biomaterial level, to a cellular level, by co-encapsulating multiple relevant cell types. Nonetheless, this work sets a great pipeline to establish a versatile lung inspired biomaterial, which could be adapted in future work. To understand the effect of ECM composition on dormant BC cells, Ovadia and colleagues established 3D human cultures of distinct metastatic niches.126 To mimic the different niches, namely bone marrow, basement membrane and lung, the authors also relied on tuneable synthetic bioactive PEG-based hydrogels, capturing main characteristics of the respective local environments. Specifically, to mimic the lung niche, a 10 wt% four-arm PEG-thiol with degradable collagen I derived bis-alkene peptide crosslinker, was functionalized with collagen peptide sequences, which serve as adhesion sites for cells (Fig. 5E). This yielded hydrogels with a stiffness of 4.21 ± 0.47 kPa, which, according to the authors, matches the elastic modulus of lung tissue. Within these hydrogels, different BC cell lines reflecting different levels of aggressiveness were encapsulated and maintained a high viability throughout culture periods of 40 days (Fig. 5F). It was then demonstrated that, next to BC subtype, niche-based hydrogel stiffness dictated whether BC cells would enter a state of dormancy or display further growth (Fig. 5G and H). It is important to emphasize that relying solely on tissue stiffness and a single peptide sequence to define or limit these niches might not accurately reflect cell behavior within their native niche. Especially given that stiffness and composition within a given niche might be subject to variation across individuals and over the course of disease.66,127 Regardless, these studies have provided valuable insight into the effect that distinct tissue environments, based on peptides and mechanical cues, infer on cell behaviour and disease progression. In line with this, recognizing the relevance of the dynamic forces BC cells are facing in different microenvironments, Novak et al. established a pleural effusion model that exposes cells within hydrogels to shear stress (Fig. 5I).14 It should be noted that pleural effusion of BC occurs as a result of the spread of BC cells to the pleural space and is considered to be a severe complication.128 In order to investigate this, various BC cell lines were encapsulated in 3% w/v agarose and rat tail collagen type I based hydrogels, displaying elastic moduli of 10.36 ± 0.08 kPa to mimic the pleural effusion environment. The hydrogels were subsequently placed in a bioreactor, exposing cells to an input fluid velocity of 3.83 mm s−1, yielding a maximum shear stress of 5.41 dynes cm−2 experienced by the cells. Intriguingly, under shear stress conditions, BC cells exhibited a changed morphology (Fig. 5J–L), enhanced proliferation and chemoresistance. These observations undeniably accentuate the importance of implementing dynamic stimuli in 3D models.14 However, to further amplify their translational value, such models would benefit from incorporating more advanced bioinspired materials to provide cells with a more physiological environment.
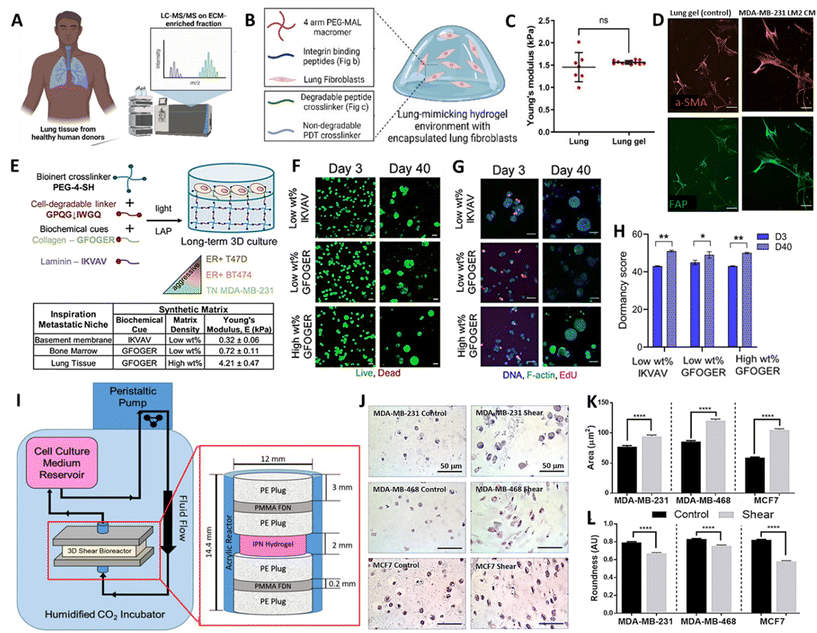 |
| Fig. 5 (A) Schematic representation of proteomic analysis of healthy human lung, (B) Composition of synthetic lung-mimicking hydrogel and (C) mechanical characterization of porcine lungs and lung mimicking gels. (D) Fluorescence images of fibroblasts stained with alpha smooth muscle actin (a-SMA) and fibroblast activation protein (FAP) encapsulated in lung-mimicking hydrogels exposed to conditioned media (CM) of BC cells (E) Schematic representation of synthetic basement membrane, bone marrow and lung-mimicking hydrogel for breast cancer cell culture. (F) Fluorescent images of viability (G) growth and (H) quantification of dormancy score of BC cells after 40 days of culture in synthetic metastatic niches. (I) Schematic representation and images of the bioreactor to expose breast cancer cells to shear stress mimicking the pleural effusion environment. (J–L) Images and quantification of morphological changes upon exposure of BC cell lines to shear stress (A–D) Reproduced with permission from ref. 125 Copyright 2023 John Wiley and Sons (E–H) Reproduced with permission from ref. 126 Copyright 2020 John Wiley and Sons (I–K) Reproduced with permission from ref. 14 Copyright 2019 John Wiley and Sons. | |
In summary, the above discussed 3D models of BC lung metastasis have implemented tuneable bioactive materials that mimic the ECM of the lung, thereby providing the backbone and great tools to take on the development of physiologically more relevant models. While most of these studies made efforts to create niche specific environments, common shortcomings remain the lack of cellular complexity and a discrepancy about the niche's local mechanical properties. This was reflected in the rather big differences in stiffness ranging from 1–10 kPa across these studies, possibly due to distinct measurement methods and specimen preparation. This aspect requires careful attention, especially since these and other studies demonstrated that a difference in hydrogel stiffness can elicit substantially distinct cellular behaviour profiles.126,128,129 Hence, if the in vitro values do not match the in vivo values, one might obtain misleading readouts, stressing the need to accurately characterize the mechanics of the desired in vivo niches. Likewise, it would also be beneficial, yet challenging, to include vascular channels in such 3D models to also recapitulate the shear forces cells experience due to local fluid flow. Another important consideration is the inclusion of the cyclic stretch, which cells situated in the lung experience due to frequent breathing motions. As already discussed, such dynamics evidently influence cell behaviour and advanced BC lung metastasis models should aim to capture these aspects.11,12 Finally, the characterization of the above-discussed 3D models is primarily limited to conventional optical imaging techniques, which pose several constraints. Thus, in the following sections, we will explore how inorganic NPs can contribute to the advancement of dynamic 3D lung models, keeping in mind that the goal is to develop models that closely resemble reality. Additionally, we will highlight the advantages offered by NP-based characterization techniques over conventional approaches.
Nanoparticles as stimuli responsive actuators to create dynamic models and as agents for advanced characterization techniques
Within the field of bioengineering, NPs have recently gained increasing attraction as tools to introduce another layer of complexity into 3D models. Specifically, in response to an external stimulus, NPs can induce a change in the surrounding matrix over time, thereby adding the aforementioned fourth dimension to a given model. To date, various types of inorganic NPs have been explored in order to obtain mechanical motions34,132,151 that could potentially be extrapolated to emulate breathing motions in lung models and will be further discussed. Importantly, next to providing stimuli responses, NPs can also be pivoted to serve as contrast or bio-sensing agents to characterize such models. This makes NPs particularly relevant for 3D and 4D in vitro modelling, facilitating capturing the complexity of 3D models.152 The following subsections explore the existing work in this direction, highlighting the most commonly used NPs. Herein, different types of in vitro models are discussed, as still few in vitro lung models have been done.
Nanoparticles to create dynamic 4D in vitro models
In light of the necessity to incorporate dynamism into different in vitro models, novel biocompatible materials that exhibit rapid responsiveness to diverse stimuli have arisen. In this regard, the combination of stimuli-responsive (bio)materials (polymers, proteins etc.)153,154 with inorganic NPs that can act as actuators has emerged, opening up a whole new avenue of possibilities.155–157 Among the most common NPs, plasmonic, magnetic, electric or piezoelectric NPs can be found. The desired NP response can be triggered by applying an external stimulus according to the NP nature. For example, plasmonic NPs are activated upon irradiation with an incident light,158 whereas magnetic159,160 or piezoelectric NPs161,162 are activated in the presence of a magnetic or electric field, respectively. When these NPs are embedded in a matrix they can modify its properties in terms of shape, size or mechanics among others.163–165 The organic matrix, in turn, can also respond to certain stimuli such as pH changes166 or temperature,167 among others, which in combination can provide the desired effect. In fact, these kind of hybrid biocompatible polymers combined with NPs have been extensively used for the creation of drug delivery systems,168 and are now starting to be utilized for generating stimuli-responsive movement/shape changes,132,169,170 which can be employed to incorporate dynamism into in vitro models. Table 2 summarises the features of the three most common inorganic NPs incorporated in hybrid materials and in in vitro models, compatible with different imaging techniques.
Table 2 Features of the most common used NPs: magnetic, piezoelectric and plasmonic NPs
Nanoparticles |
Magnetic |
Piezoelectric |
Plasmonic |
Materials |
Iron Oxide NPs(Fe2O3/Fe3O4) |
Barium titanate, boron nitride nanotubes, lead zirconate titanate, zinc oxide NPs |
Gold NPs (AuNPs) |
Properties |
Response to magnetic fields |
Activation under electrical and/or mechanical stimuli |
Localized surface plasmon resonance (LSPR), excitation upon incidence with a given wavelength light source |
Effect |
• Mechanical response (movement, folding) in presence of magnetic field. |
Generation of electric response upon mechanic stimulation or vice versa |
• Generation of localized heating-upon light irradiation (photothermia) |
• Heat: hyperthermia |
• Controllable contraction-expansion movements |
|
• High electrical conductivity. |
|
• Ultrasensitive sensors |
Characterization function |
Contrast agents in Magnetic Resonance Imaging (MRI) and Magnetic Particle Imaging (MPI) |
Contrast agents in ultrasound imaging |
Contrast agents in Surface Enhanced Raman Spectroscopy (SERS) and Computer Tomography (CT) imaging |
Applications |
Cancer treatment (magnetic hyperthermia), osteogenic differentiation, drug carriers, MRI and MPI imaging |
Neuromodulation, cardiac tissue maturation, cancer treatment, ultrasound imaging |
Cancer treatment (photothermal therapy), dynamic in vitro tissue models, SERS imaging and detection of metabolites |
Advantages |
Relatively cheap material |
Wireless electric activation (ultrasound) |
Highly efficient |
Good biocompatibility |
Very localized heating |
|
Fast responses |
Disadvantages |
High concentrations of NPs are required |
Optimization of NP is required |
A laser is needed to activate them |
Difficulties optimizing heating efficiency |
Generally combined with piezoelectric polymers to achieve good efficiency |
Adjustment of the plasmon band and incident light wavelength |
|
|
Heating control required to either surpass or avoid exceeding the physiological temperature |
References |
30, 33 and 130–136 |
137–142
|
30, 34 and 143–150 |
Along those lines of research, the concept of 4D printing has also started to gain more significance and has triggered studies dedicated to the design of smart bioinks implementing such NPs. Up until now, most examples of these 4D printed materials have appeared in the field of electronics or soft robotics. For instance, Jang et al.131 3D-printed biocompatible and biodegradable gripper-shaped hydrogels composed of chitosan and citric acid-coated superparamagnetic iron oxide NPs (SPIONs). Upon subjecting the magneto- and electro-active hydrogels to an electric field, the grippers closed (Fig. 6A and B), and re-opened upon applying the contrary electric field. The speed of opening and closing motions varied by applying different electric fields (Fig. 6C). Additionally, they could be transported under the influence of a magnetic field, thereby providing a proof-of-concept for gripping and transporting drugs or other molecules of interest. Hybrid 4D materials including NPs have also been employed for stimulating cell differentiation or tissue healing.171 In this context, magnetic130 and piezoelectric137,140,141 NPs have primarily been used. As the latter can trigger a mechanical response upon electrical stimulation and vice versa, ultrasound (US) is a widely used mechanical stimulus to activate them, generating an electrical response.137 For instance, Li et al.141 developed silk fibroin (SF) hydrogels with polydopamine (PDA)-coated barium titanium oxide (BaTiO3) NPs for spinal cord injury (SCI) regeneration. The materials with or without NPs were biocompatible, with mechanical properties matching the in vivo properties of the spinal cord (0.9–3 kPa).172 Strikingly, in hydrogels with NPs, but not in hydrogels without NPs, the application of US triggered an increase in markers implicated in the differentiation of neural stem cells. In line with this, implanting these materials in vivo in a SCI rat model, demonstrated that the combination of piezoelectric NPs with US stimulation yields a superior neural regeneration.
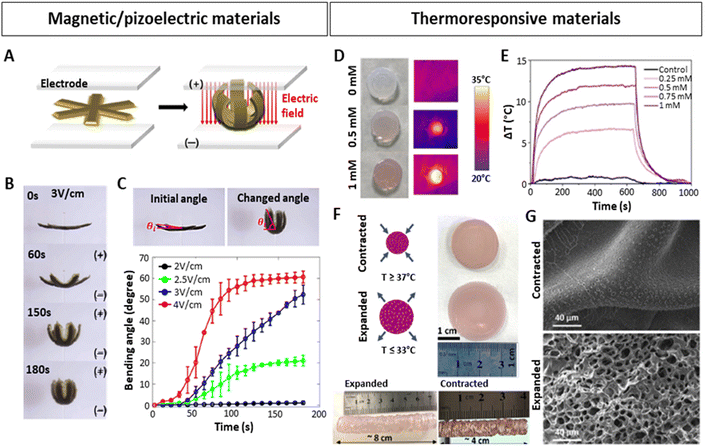 |
| Fig. 6 Stimuli-responsive hydrogels with embedded NPs. (A–C) 4D-printed, magnetically and electrically responsive, gripper-shaped chitosan hydrogels with citric acid-coated SPIONs. (A) Schematic representation of gripping motion under an electric field. (B–C) Bending of the hydrogel over time after applying different electric fields. (D–G) 4D-printed, thermoresponsive PNIPAM-PEGDA hydrogels with plasmonic AuNRs. (D and E) Heating of hydrogel discs upon 808 nm laser irradiation at 1 W/cm2, dependent on the concentration of AuNRs (measured as [Au0] concentration by Uv-vis). (F) Contracted and expanded states of hydrogel discs and extrusion-printed cylinders at temperatures above and below the LCST. (G) SEM images of contracted and expanded hydrogels. Scale bars: 40 μm. (A–C) Reproduced with permission from ref. 151 Copyright 2023 Elsevier (D–G) Reproduced with permission from ref. 34 Copyright 2023 Royal Society of Chemistry. | |
Interesting studies outside the scope of lung modelling have laid down additional NP-based strategies that could potentially be adapted to introduce dynamism into lung models. In this regard, the combination of plasmonic NPs158 with thermoresponsive materials167,173 poses a feasible approach, as evidenced in numerous investigations.34,146,147,174 Plasmonic NPs, such as commonly used silver (Ag) or gold (Au) NPs, can be excited by applying a resonant light irradiation, generating an extremely rapid and localized heating and cooling,74,175 resulting in changes within the matrices they are embedded in, as long as they are thermosensitive. Anisotropic AuNPs presenting localized surface plasmon resonances (LSPRs) in the first biological window of the electromagnetic spectrum, are excellent candidates to be used as such bio-nanoheaters, as they allow the use of non-cytotoxic near-infrared (NIR) light sources with high penetrative abilities in tissues.152,176 In fact, the LSPR of AuNPs can be modified by tuning the shape, size or surface, which, apart from heating capabilities, can also improve conductivity in in vitro models,143 or serve as in situ sensors.144,145 With the aim of generating contractions and expansions similar to those of the heartbeat, Aizarna-Lopetegui et al.34 generated a 4D-printed stimuli-responsive cylindrical artery model via extrusion printing (Fig. 6D–G), combining thermoresponsive materials and AuNPs. They incorporated plasmonic gold nanorods (AuNRs) in a biocompatible polymer mixture containing poly(N-isopropylamide) (pNIPAM) and polyethylene diacrylate (PEGDA) to fabricate the external layer of the artery. Being a thermoresponsive polymer, pNIPAM possesses a lower critical solution temperature (LCST) around 32 °C and can be tuned to more physiological conditions (37–38 °C).177 Importantly, below the LCST, pNIPAM is hydrophilic and swollen, but when transitioning the temperature above the LCST, pNIPAM becomes hydrophobic, releases the entrapped water and contracts. When the temperature decreases, it re-gains hydrophilicity and swells. The authors irradiated gel mixtures with different AuNRs concentrations (0.25, 0.5, 0.75 and 1 mM [Au0]) with a NIR (808 nm) laser at 1 W cm−2, monitoring the temperature change (ΔT) (Fig. 6D and E), selecting 0.5 mM [Au0] as the best candidate, and subsequently studied its expansion/contraction capability (Fig. 6F). Differences between the expanded and contracted state of the hydrogel were also visible by SEM microscopy (Fig. 6G). The authors then proceeded to demonstrate as a proof of concept that due to the incorporation of AuNRs, which can be rapidly irradiated using a cyclic laser, the pulsatile motions of an artery could be mimicked. An inner layer of the artery was also 3D-printed, consisting of smooth muscle cells (SMCs) embedded in Matrigel with a monolayer of endothelial cells (ECs) on top. Afterwards the biocompatibility of the model for both cell types was validated and more importantly, it was shown that AuNRs-NIR-induced pulsed irradiation led to cyclic contraction, generating the expression of mechanoresponsive mesenchymal activation gene signatures associated with microenvironmental physical cues.
To summarize, NP-based 4D printing for in vitro tissue modelling is still in its infancy, and to our knowledge, currently no studies have been conducted in the field related to 3D lung in vitro models. Nonetheless, the herein discussed evidence suggests that by developing stimuli responsive materials which can respond to stimuli generated by NPs, different dynamic motions and responses can be produced, which could be translated to lung in vitro models.
Nanoparticle-based characterization of in vitro lung models
As previously mentioned, the majority of research regarding NPs in the context of in vitro lung models is in relation to their toxicity or potential as therapeutics.178–181 While the use of NPs as contrast agents is quite common in in vivo studies,182–184 few studies recognize their utility as agents to characterize and functionalize lung in vitro 3D models. Characterizing these models and evaluating the effect of different therapeutics is particularly challenging given their complex architecture, which can pose penetration depth limits and hamper the diffusion of labels, antibodies or dyes, and the ability to follow their evolution over time. In addition, these strategies often involve invasive procedures. Commonly used fluorescence-based methods can suffer from photo-bleaching, limited multiplexing capacity and auto-fluorescence.152 Thus, to overcome these issues, NPs can be exploited for instance as optical probes with better photo-stability and low toxicity, thereby making them attractive tools compatible with live cell imaging.185–187 Especially plasmonic AuNPs find popularity as optical enhancing agents, given their easy surface modification, chemical stability as well as biocompatibility, and have found applications across numerous in vitro lung studies.188–191 They provide contrast, in the context of surface enhanced Raman spectroscopy (SERS) imaging, a sensitive, label-free, non-invasive characterization technique,192 as well as in computer tomography (CT).184 Importantly, they can be combined with fluorescent imaging193 or additional techniques. Fluorescent NPs that avoid spectral overlap, such as quantum dots194,195 or carbon dots196 harbor great potential and are compatible with magnetic resonance imaging (MRI) techniques, when doped with specific elements such Gd(III).197 Alternatively, magnetic NPs can be used as contrast agents, offering non-invasive deep imaging with low background signal generation not only for MRI198 but also for magnetic particle imaging (MPI).199 Moreover, they can be combined with gold131,200 or with nuclear imaging agents to be analysed with positron emission tomography (PET)199 or photon emission computed tomography (SPECT).201 The possibility to perform multimodal imaging is of high relevance to obtain complementary information. While these techniques are already standardized for lung in vivo studies,136,202 they start to appear in in vitro 3D studies,131,203 and are expected to gain more presence in the near future.
Along those lines of evidence, within the scope of lung in vitro studies NPs have been implemented as biochemical sensors to study different lung diseases and track a variety of cellular analytes. This can be achieved by functionalizing NPs with molecules that are for instance sensitive to a desired metabolite, enabling non-invasive monitoring of metabolic changes as evidenced in the following 3D lung models. Based on this concept, and as performed before for an in vitro breast cancer model,144,204 Eom et al. have developed multifunctional alveolar scaffolds coated with AuNPs, to monitor real time alveolar cell responses.188 The backbone of the scaffolds was based on nickel foam with integrated electroactive metal–organic framework crystals and AuNPs, functionalized with hyaluronic acid and seeded with A549 cells (alveolar epithelial cells) (Fig. 7A). The AuNP coating enabled the monitoring of distinct cellular responses to different toxicants based on changes in cellular components resulting in different Raman spectra (Fig. 7B and C). More specifically, secreted molecules (i.e., DNA bases, tryptophan, phenylalanine) from cells exposed to a given toxicant were reflected in specific Raman intensity changes across the spectra. Based on that, the authors could identify and distinguish phases and forms of cell death. This approach evidently emphasized the compelling capacity of SERS to assess dynamic changes in a complex 3D lung in vitro model. Likewise, within the framework of an airway organoid study, Skinner and colleagues utilized SERS probes as microsensors to track pH changes within the organoid lumen and ECM (Fig. 7D–G).189 These microsensors were composed of solid polymer cores with surface immobilized AuNPs, functionalized with 4-meraptobenzoic acid, which served as pH sensitive reporters (Fig. 7D). In order to track luminal pH in a non-invasive manner, these SERS probes were added prior to organoid assembly, resulting in their incorporation into the organoid lumen (Fig. 7E). This enabled the tracking of pH differences inside and outside of the organoid lumen, further demonstrating the broad spectrum of analytes that NPs can reliably detect with high sensitivity (Fig. 7F and G). Yet another study, albeit not based on 3D models, demonstrated the utility of SERS probes as tools to distinguish the segregation of lung resident mesenchymal stem cells (LR-MSC) into different subtypes.190 More specifically, to accomplish this the authors used intracellular TAT-peptide conjugated Au Nanostar (AuNS) SERS probes (Fig. 7H–J). Beyond the distinction of undifferentiated and differentiated LR-MSC, these probes also enabled the monitoring of changes occurring during the differentiation process itself (Fig. 7H and I). In light of the inherent difficulty of standard techniques discriminating closely related cellular phenotypes, these results provide a powerful rational for NPs as a highly beneficial tool in stem-cell research. In a subsequent study, the authors used the same TAT-functionalized AuNS probes to characterize the epithelial to mesenchymal transition (EMT) of alveolar type II cells (Fig. 7J).191 Given the EMT process is known to be involved in fibrosis as well as cancer metastasis, this provides an attractive strategy to adapt this technique to 3D lung models.178,180
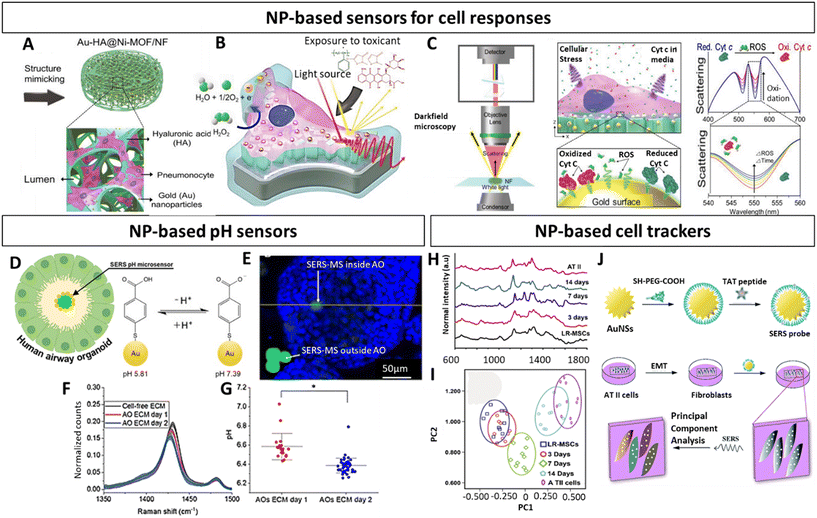 |
| Fig. 7 Nanoparticle based characterization of in vitro lung models (A) Schematic representation of multifunctional Au-HA@Ni-MOF 3D alveolar scaffolds. (B) Illustration of scaffold exposure to toxicants and (C) monitoring of oxidative stress responses of pneumocytes. Left panel displays the spectral monitoring setup, the middle panel illustrates the optical ROS monitoring, and the right panel depicts the expected spectral changes in response to ROS release. (D) Schematic representation of airway organoid and SERS pH sensor. (E) Fluorescence image of SERS pH-sensor (green) outside and inside of airway organoid (blue epithelial cell nuclei). (F) Spectra illustrating the decrease of v(COO) in ECM of airway organoids and (G) pH-values based on SERS spectra at different timepoints. (H) Raman spectra and (I) principal component analysis of differentiating LR-MSCs into ATII cells (J) Representation of AuNPs functionalized with TAT-peptides and their application to identify the EMT process of ATII cells. Au-HA@Ni-MOF, gold hyaluronic acid nickel metal organic frawork; ROS, reactive oxygen species; SERS, surface enhanced Raman spectroscopy; AO, airway organoid; gold nanostars (AuNS); ATII, type II pneumocytes; LR-MSCs, lung resident-mesenchymal stem cells. (A–C) Reproduced with permission from ref. 188 Copyright 2023 Wiley-VCH GmbH (open access) (D–G) Reproduced with permission from ref. 189 Copyright 2023 Royal Society of Chemistry (H) Reproduced with permission from ref. 191 Copyright 2016 Royal Society of Chemistry (open access) (I and J) Reproduced with permission from ref. 190. Copyright 2015 Elsevier. | |
To summarize, NPs harbor an underappreciated potential to greatly advance the characterization of complex 3D lung models. The above discussed studies provide evidence that NP-based assays can tackle challenging concepts such as probing biochemical analytes of complex cellular structures, but also provide an accurate and sensitive tool to facilitate cell tracking. Thus, NPs embody a highly adaptable and attractive tool to assess a broad range of analytes in a non-invasive, sensitive, and rapid manner. Moreover, emerging evidence beyond the scope of lung models highlights the potential of clinically relevant NPs-based contrast agents for multimodal imaging techniques, with implacable safety and imaging profiles. Nonetheless, it should be acknowledged that this requires highly specialized expertise and toolsets, which are not necessarily accessible within standard laboratories.
Conclusion and perspectives
The persisting need to gain a better understanding and develop improved therapies for pulmonary diseases has triggered researchers to take on novel approaches to establish physiologically relevant in vitro models. An ever-growing body of evidence emphasizes the relevance of incorporating physiological features, such as an ALI, mechanical forces that mimic breathing motions, the spatial cellular organization and the implementation of niche like ECM in 3D models of the lung. In this context, LoC as well as 3D printing have been shown to be suitable strategies to accomplish this. While LoC models have demonstrated how mimicking the ALI and including mechanical forces evidently change cell behaviour, 3D printing approaches established an in vivo like cellular architecture within lung inspired ECMs. These findings indicate the importance of combining different approaches to obtain functional 3D in vitro lung models. Importantly, bioinspired materials that reflect tissue composition on a cellular and extracellular level should be at the core of such translational 3D models. However, it remains challenging to establish consensus on certain tissue characteristics, such as stiffness and composition since this requires standardized measurement procedures. Especially within the lung such readouts can vary based on methodology as well as anatomical locations, which reflect distinct functions and hence distinct compositions.205,206 This in turn requires a certain toolset as well as expertise and specimens to obtain this information, all of which are not easily accessible. Thus, public tissue data bases should be expanded and developed to serve as a reliable source of information that can be implemented in the model design. While advances in single cell sequencing and proteomics enabled the establishment of cellular atlases39,207 as well as the elucidation of ECM composition of human lung,206,208 equivalent resources on relevant tissue biophysical characteristics are required to further fill this gap.
The above-discussed literature highlights the multiple levels at which NPs can and should be implemented to accelerate dynamic 3D and 4D modelling of respiratory diseases. Currently dynamic in vitro models of lung heavily rely on external stimulation based on bioreactor set-ups.14,73,87,88,104 As already described, such set-ups can complicate and limit readouts and monitoring. In this regard it has been demonstrated how NPs can generate local biophysical forces, which could in future studies facilitate the recapitulation of the in vivo breathing-induced cyclic stretch via the combination of stimuli responsive (bio-)materials combined with plasmonic, magnetic, conductive or additional NPs that can act as multi-responsive actuators. Still research should be done in terms of NPs concentration, generated response, adequation of speed and mechanic motions, biocompatibility with stem cells, among others. Nonetheless, promising results obtained so far indicate that it could be a favourable approach.
In addition, NPs have been demonstrated to serve as multimodal agents to characterize such complex model setups. In this context, NP-based bioimaging and biosensing has found versatile applications across a broad spectrum of analytes, which circumvent shortcomings of conventional modalities in 2D as well as 3D models. Along those lines of evidence, they have been proven to accurately report pH changes, informative spectra of real time cell responses, distinct cell states as well as to track and distinguish between closely related cell populations in a highly sensitive manner. However, despite this large body of evidence, their potential remains underappreciated in the context of in vitro lung models, possibly due to fact that these modalities are still in their infancy and require a certain level of expertise. Regardless, even more defined 3D lung in vitro models should be pursued, combining multiple of the herein discussed physiological features in order to amplify their clinical value and to study unresolved medical questions related to diseases affecting a significant portion of the population, such a pulmonary fibrosis or breast cancer lung metastasis, among others. For this purpose, we highly encourage future studies to consider the implementation of NPs to complement the development of such dynamic in vitro lung complex models.
Author contributions
The manuscript was written through contributions of all authors. All authors have given approval to the final version of the manuscript.
Conflicts of interest
There are no conflicts to declare.
Acknowledgements
Financial support is acknowledged through grants #PID2022-143248OB-I00, #PID2021-123013OB-I00 and #CNS2022-135941 from the MCIN/AEI/10.13039/501100011033 and "ERDF A way of making Europe" and by “European Union NextGeneration EU/PRTR”. A. C. thanks to the Spanish Association Against Cancer Scientific Foundation (grant #LABAE223466CIPI) and to the Basque Government (grant #2023333027). A. U. A thanks the Gipuzkoa Provincial Council (grant #2022-CIEN-000086-01). L. F thanks the Investigo Program, NextGeneration EU and the Collaborative PhD studentship CICbiomaGUNE program 2022 (#CICBMG_PhD_03_2022).
References
- G. A. Roth, G. A. Mensah, C. O. Johnson, G. Addolorato, E. Ammirati, L. M. Baddour, N. C. Barengo, A. Z. Beaton, E. J. Benjamin, C. P. Benziger, A. Bonny, M. Brauer, M. Brodmann, T. J. Cahill, J. Carapetis, A. L. Catapano, S. S. Chugh, L. T. Cooper, J. Coresh, M. Criqui, N. DeCleene, K. A. Eagle, S. Emmons-Bell, V. L. Feigin, J. Fernández-Solà, G. Fowkes, E. Gakidou, S. M. Grundy, F. J. He, G. Howard, F. Hu, L. Inker, G. Karthikeyan, N. Kassebaum, W. Koroshetz, C. Lavie, D. Lloyd-Jones, H. S. Lu, A. Mirijello, A. M. Temesgen, A. Mokdad, A. E. Moran, P. Muntner, J. Narula, B. Neal, M. Ntsekhe, G. Morales de Oliveira, C. Otto, M. Owolabi, M. Pratt, S. Rajagopalan, M. Reitsma, A. L. P. Ribeiro, N. Rigotti, A. Rodgers, C. Sable, S. Shakil, K. Sliwa-Hahnle, B. Stark, J. Sundström, P. Timpel, I. M. Tleijeh, M. Valgimigli, T. Vos, P. K. Whelton, M. Yacoub, L. Zuhlke, C. Murray and V. Fuster, J. Am. Coll. Cardiol., 2020, 76, 2982–3021 CrossRef PubMed
.
- L. Karamchand, D. Makeiff, Y. Gao, K. Ayzat, M. J. Serpe and M. Kulka, Bioprinting, 2023, 29, e00255 CrossRef
.
- S. A. Langhans, Front. Pharmacol., 2018, 9, 1–14 CrossRef PubMed
.
- P. J. Barnes, S. Bonini, W. Seeger, M. G. Belvisi, B. Ward and A. Holmes, Eur. Respir. J., 2015, 45, 1197–1207 CrossRef PubMed
.
- J. Arrowsmith and P. Miller, Nat. Rev. Drug Discovery, 2013, 12, 569–569 CrossRef CAS PubMed
.
- V. Jentzsch, L. Osipenko, J. W. Scannell and J. A. Hickman, JAMA Netw. Open, 2023, 6, e2324977 CrossRef PubMed
.
- Z. N. Abbas, A. Z. Al-Saffar, S. M. Jasim and G. M. Sulaiman, Sci. Rep., 2023, 13, 18380 CrossRef CAS PubMed
.
- D. Kang, J. A. Park, W. Kim, H. R. Lee, W. J. Kim, J. Y. Yoo and S. Jung, Adv. Sci., 2021, 8, 2004990 CrossRef CAS PubMed
.
- A. Doryab and J. Groll, Adv. Mater., 2023, 35, 2210519 CrossRef CAS PubMed
.
- J. C. Nawroth, R. Barrile, D. Conegliano, S. van Riet, P. S. Hiemstra and R. Villenave, Adv. Drug Delivery Rev., 2019, 140, 12 CrossRef CAS PubMed
.
- B. A. Hassell, G. Goyal, E. Lee, A. Sontheimer-Phelps, O. Levy, C. S. Chen and D. E. Ingber, Cell Rep., 2017, 21, 508–516 CrossRef CAS PubMed
.
- C. Shen, H. Yang, W. She and Q. Meng, Biotechnol. Bioeng., 2023, 120, 2027–2038 CrossRef CAS PubMed
.
- B. Gao, Q. Yang, X. Zhao, Y. Ma and F. Xu, Trends Biotechnol., 2016, 34, 746–756 CrossRef CAS PubMed
.
- C. M. Novak, E. N. Horst, C. C. Taylor, C. Z. Liu and G. Mehta, Biotechnol. Bioeng., 2019, 116, 3084–3097 CrossRef CAS PubMed
.
- X. Huang, Z. Huang, W. Gao, W. Gao, R. He, Y. Li, R. Crawford, Y. Zhou, L. Xiao and Y. Xiao, Gels, 2022, 8, 829–848 CrossRef CAS PubMed
.
- T. Riss and O. J. Trask, In Vitro Cell. Dev. Biol.: Anim., 2021, 57, 238–256 CrossRef CAS PubMed
.
- N. Carragher, F. Piccinini, A. Tesei, J. Trask Jr., M. Bickle and P. Horvath, Nat. Rev. Drug Discovery, 2018, 17, 606–606 CrossRef CAS PubMed
.
- T. I. Anderson, B. Vega and A. R. Kovscek, Comput. Geosci., 2020, 145, 104593 CrossRef
.
- C. M. Leung, P. de Haan, K. Ronaldson-Bouchard, G. A. Kim, J. Ko, H. Z. Rho, Z. Chen, P. Habibovic, N. L. Jeon, S. Takayama, M. L. Shuler, G. Vunjak-Novakovic, O. Frey, E. Verpoorte and Y. C. Toh, Nat. Rev. Methods Primers, 2022, 2, 33–61 CrossRef CAS
.
- N. Joudeh and D. Linke, J. Nanobiotechnol., 2022, 20, 262–290 CrossRef PubMed
.
- A. Hasan, M. Morshed, A. Memic, S. Hassan, T. J. Webster and H. Marei, Int. J. Nanomed., 2018, 13, 5637–5655 CrossRef CAS PubMed
.
- R. Khursheed, K. Dua, S. Vishwas, M. Gulati, N. K. Jha, G. M. Aldhafeeri, F. G. Alanazi, B. H. Goh, G. Gupta, K. R. Paudel, P. M. Hansbro, D. K. Chellappan and S. K. Singh, Biomed. Pharmacother., 2022, 150, 112951 CrossRef CAS PubMed
.
- M. P. Lokugamage, D. Vanover, J. Beyersdorf, M. Z. C. Hatit, L. Rotolo, E. S. Echeverri, H. E. Peck, H. Ni, J. K. Yoon, Y. Kim, P. J. Santangelo and J. E. Dahlman, Nat. Biomed. Eng., 2021, 5, 1059–1068 CrossRef CAS PubMed
.
- M. J. Mitchell, M. M. Billingsley, R. M. Haley, M. E. Wechsler, N. A. Peppas and R. Langer, Nat. Rev. Drug Discovery, 2021, 20, 101–124 CrossRef CAS PubMed
.
- J. W. Card, D. C. Zeldin, J. C. Bonner and E. R. Nestmann, Am. J. Physiol. Lung Cell. Mol. Physiol., 2008, 295, L400–L411 CrossRef CAS PubMed
.
- T. Kuhnt, S. Camarero-Espinosa, M. T. Ghahfarokhi, M. Arreguín, R. Cabassi, F. Albertini, D. Nieto, M. B. Baker and L. Moroni, Adv. Funct. Mater., 2022, 32, 2202539 CrossRef CAS
.
- L. Wang, M. H. Kafshgari and M. Meunier, Adv. Funct. Mater., 2020, 30, 2005400 CrossRef CAS
.
- R. Zhang, S. Qing, X. Zhang, Z. Luo and Y. Liu, Nanotechnol. Rev., 2023, 12, 20220562 CrossRef CAS
.
- E. Mostafavi, D. Medina-Cruz, K. Kalantari, A. Taymoori, P. Soltantabar and T. J. Webster, Bioelectricity, 2020, 2, 120–149 CrossRef PubMed
.
- S. Rittikulsittichai, A. G. Kolhatkar, S. Sarangi, M. A. Vorontsova, P. G. Vekilov, A. Brazdeikis and T. R. Lee, Nanoscale, 2016, 8, 11851–11861 RSC
.
- H. L. Tan, S. Y. Teow and J. Pushpamalar, Bioengineering, 2019, 6, 17 CrossRef CAS PubMed
.
- Q. Shi, H. Liu, D. Tang, Y. Li, X. Li and F. Xu, NPG Asia Mater., 2019, 11, 64 CrossRef CAS
.
- F. Xu, C. M. Wu, V. Rengarajan, T. D. Finley, H. O. Keles, Y. Sung, B. Li, U. A. Gurkan and U. Demirci, Adv. Mater., 2011, 23, 4254–4260 CrossRef CAS PubMed
.
- U. Aizarna-Lopetegui, C. García-Astrain, C. Renero-Lecuna, P. González-Callejo, I. Villaluenga, M. A. del Pozo, M. Sánchez-Álvarez, M. Henriksen-Lacey and D. Jimenez de Aberasturi, J. Mater. Chem. B, 2023, 11, 9431 RSC
.
- K. Bando, Z. Zhang, D. Graham, K. Faulds, K. Fujita and S. Kawata, Analyst, 2020, 145, 5768–5775 RSC
.
- Y. Wang, K. Zhang, T. Tian, W. Shan, L. Qiao and B. Liu, ACS Appl. Mater. Interfaces, 2021, 13, 4886–4893 CrossRef CAS PubMed
.
- B. Zhang, M. Xie, L. Bruschweiler-Li and R. Brüschweiler, Metabolites, 2018, 8, 21 CrossRef PubMed
.
- D. Yeo, C. Wiraja, R. C. Y. Jin, G. Yu and C. Xu, Sci. Rep., 2015, 5, 14768 CrossRef CAS PubMed
.
- K. J. Travaglini, A. N. Nabhan, L. Penland, R. Sinha, A. Gillich, R. V. Sit, S. Chang, S. D. Conley, Y. Mori, J. Seita, G. J. Berry, J. B. Shrager, R. J. Metzger, C. S. Kuo, N. Neff, I. L. Weissman, S. R. Quake and M. A. Krasnow, Nature, 2020, 587, 619–625 CrossRef CAS PubMed
.
- M. Z. Nikolić, D. Sun and E. L. Rawlins, Development, 2018, 145, dev163485 CrossRef PubMed
.
- J. L. Schneider, J. H. Rowe, C. Garcia-de-Alba, C. F. Kim, A. H. Sharpe and M. C. Haigis, Cell, 2021, 184, 1990–2019 CrossRef CAS PubMed
.
- J. Bastacky, C. Y. C. Lee, J. Goerke, H. Koushafar, D. Yager, L. Kenaga, T. P. Speed, Y. Chen and J. A. Clemets, J. Appl. Physiol., 1995, 79, 1615–1628 CrossRef CAS PubMed
.
- T. E. King, A. Pardo and M. Selman, Lancet, 2011, 378, 1949–1961 CrossRef PubMed
.
- M. R. Knowles and P. R. Durie, N. Engl. J. Med., 2002, 347, 439–442 CrossRef PubMed
.
- P. L. Shah, F. J. Herth, W. H. van Geffen, G. Deslee and D. J. Slebos, Lancet Respir. Med., 2017, 5, 147–156 CrossRef PubMed
.
- J. P. Janssens, J. C. Pache and L. P. Nicod, Eur. Respir. J., 1999, 13, 197–205 CAS
.
- P. Zamprogno, S. Wüthrich, S. Achenbach, G. Thoma, J. D. Stucki, N. Hobi, N. Schneider-Daum, C. M. Lehr, H. Huwer, T. Geiser, R. A. Schmid and O. T. Guenat, Commun. Biol., 2021, 4, 168–177 CrossRef CAS PubMed
.
- P. Gehr, M. Bachofen and E. R. Weibel, Respir. Physiol., 1978, 32, 121–140 CrossRef CAS PubMed
.
- T. Do, L. Synan, G. Ali and H. Gappa-Fahlenkamp, Stem Cell Res. Ther., 2022, 13, 464–476 CrossRef PubMed
.
- M. Strengert and U. G. Knaus, Methods Mol. Biol., 2011, 763, 195–206 CrossRef CAS PubMed
.
- E. D. Crandall and M. A. Matthay, Am. J. Respir. Crit. Care Med., 2001, 162, 1021–1029 CrossRef PubMed
.
- L. G. Dobbs, M. D. Johnson, J. Vanderbilt, L. Allen and R. Gonzalez, Cell. Physiol. Biochem., 2010, 25, 55–62 CrossRef CAS PubMed
.
- M. D. Johnson, J. H. Widdicombe, L. Allen, P. Barbry and L. G. Dobbs, Proc. Natl. Acad. Sci. U. S. A., 2002, 99, 1966–1971 CrossRef CAS PubMed
.
- O. D. Chuquimia, D. H. Petursdottir, M. J. Rahman, K. Hartl, M. Singh and C. Fernández, PLoS One, 2012, 7, e32125 CrossRef CAS PubMed
.
- M. C. Williams, Annu. Rev. Physiol., 2003, 65, 669–695 CrossRef CAS PubMed
.
- K. Jandl, A. C. Mutgan, K. Eller, L. Schaefer and G. Kwapiszewska, Matrix Biol., 2022, 105, 31–52 CrossRef CAS PubMed
.
- P. Jain, A. Nishiguchi, G. Linz, M. Wessling, A. Ludwig, R. Roissant, M. Möller and S. Singh, Adv. Biol., 2021, 5, 2000427 CrossRef CAS PubMed
.
- A. Furuyama, K. Kimata and K. Mochitate, Cell Struct. Funct., 1997, 22, 603–614 CrossRef CAS PubMed
.
- E. Thunnissen, N. Motoi, Y. Minami, D. Matsubara, W. Timens, Y. Nakatani, Y. Ishikawa, X. Baez-Navarro, T. Radonic, H. Blaauwgeers, A. C. Borczuk and M. Noguchi, Histopathology, 2022, 80, 457–467 CrossRef PubMed
.
- P. C. Wijsman, L. H. van Smoorenburg, D. M. de Bruin, J. T. Annema, H. A. M. Kerstjens, O. M. Mets, M. van der Berge, P. I. Bonta and J. K. Burgess, Curr. Opin. Physiol., 2021, 22, 100444 CrossRef CAS
.
- B. Suki, S. Ito, D. Stamenovic, K. R. Lutchen and E. P. Ingenito, J. Appl. Physiol., 2005, 98, 1892–1899 CrossRef PubMed
.
- I. A. Savin, M. A. Zenkova and A. V. Sen'kova, Int. J. Mol. Sci., 2022, 23, 14959 CrossRef CAS PubMed
.
- Z. Abassi, Y. Knaney, T. Karram and S. N. Heyman, Front. Immunol., 2020, 11, 1312 CrossRef CAS PubMed
.
- E. Namati, J. Thiesse, J. De Ryk and G. McLennan, Am. J. Respir. Cell Mol. Biol., 2008, 38, 572–578 CrossRef CAS PubMed
.
- B. Hinz, Proc. Am. Thorac. Soc., 2012, 9, 137–147 CrossRef CAS PubMed
.
- D. Sicard, A. J. Haak, K. M. Choi, A. R. Craig, L. E. Fredenburgh and D. J. Tschumperlin, Am. J. Physiol. Lung Cell. Mol. Physiol., 2018, 314, L946–L955 CrossRef CAS PubMed
.
- Y. S. Edwards, Comp. Biochem. Physiol., Part A: Mol. Integr. Physiol., 2001, 129, 245–260 CrossRef CAS PubMed
.
- M. A. Lancaster and J. A. Knoblich, Science, 2014, 345, 1247125 CrossRef PubMed
.
- N. Sachs, A. Papaspyropoulos, D. D. Zomer-van Ommen, I. Heo, L. Böttinger, D. Klay, F. Weeber, G. Huelsz-Prince, N. Iakobachvili, G. D. Amatngalim, J. de Light, A. van Hoeck, N. Proost, M. C. Viveen, A. Lyubimova, L. Teeven, S. Derakhshan, J. Korving, H. Begthel, J. F. Dekkers, K. Kumawat, E. Ramos, M. F. M. van Oosterhout, G. J. Offerhaus, D. J. Wiener, E. P. Olimpio, K. K. Dijkstra, E. F. Smit, M. van der Linden, S. Jaksani, M. van de Ven, J. Jonkers, A. C. Rios, E. E. Voest, C. H. M. van Moorsel, C. K. van der Ent, E. Cuppen, A. van Oudenaarden, F. E. Coenjaerts, L. Meyaard, L. J. Bont, P. J. Peters, S. J. Tans, J. S. van Zon, S. F. Boj, R. G. Vries, J. M. Beekman and H. Clevers, EMBO J., 2019, 38, e100300 CrossRef PubMed
.
- D. C. Wilkinson, J. A. Alva-Ornelas, J. M. S. Sucre, P. Vijayaraj, A. Durra, W. Richardson, S. J. Jonas, M. K. Paul, S. Karumbayaram, B. Dunn and B. N. Gomperts, Stem Cells Transl. Med., 2017, 6, 622–633 CrossRef PubMed
.
- J. Shrestha, S. R. Bazaz, H. Aboulkheyr, D. Y. Azari, M. E. Warkiani and M. Ghadiri, Crit. Rev. Biotechnol., 2020, 40, 213–230 CrossRef CAS PubMed
.
- S. N. Bhatia and D. E. Ingber, Nat. Biotechnol., 2014, 32, 760–772 CrossRef CAS PubMed
.
- A. O. Stucki, J. D. Stucki, S. R. R. Hall, M. Felder, Y. Mermoud, R. A. Schmid, T. Geiser and O. T. Guenat, Lab Chip, 2015, 15, 1302–1310 RSC
.
- M. Kim, J. H. Lee and J. M. Nam, Adv. Sci., 2019, 6, 1900471 CrossRef PubMed
.
- W. Kim, Y. Lee, D. Kang, T. Kwak, H. R. Lee and S. Jung, ACS Biomater. Sci. Eng., 2023, 9, 2806–2815 CrossRef CAS PubMed
.
- J. J. Pippin, South Tex. Law Rev., 2012, 54, 469–511 Search PubMed
.
- A. I. Vazquez-Armendariz, M. M. Barroso, E. El Agha and S. Herold, Cells, 2022, 11, 1526 CrossRef CAS PubMed
.
- D. E. Ingber, Ann. Med., 2003, 35, 564–577 CrossRef PubMed
.
- S. J. Mousavi and M. H. Doweidar, PLoS One, 2015, 10, 0124529 Search PubMed
.
- E. K. F. Yim and K. W. Leong, Nanomedicine, 2005, 1, 10–21 CrossRef CAS PubMed
.
- E. Maestri, BioTech, 2021, 10, 1–11 Search PubMed
.
- S. B. Han, J. K. Kim, G. Lee and D. H. Kim, Adv. Biosyst., 2020, 4, 2000247 CrossRef PubMed
.
- A. J. Ghio, L. A. Dailey, J. M. Soukup, J. Stonehuerner, J. H. Richards and R. B. Devlin, Part. Fibre Toxicol., 2013, 10, 25 CrossRef CAS PubMed
.
- Z. Zhao, X. Chen, A. M. Dowbaj, A. Sljukic, K. Bratlie, L. Lin, E. L. S. Fong, G. M. Balachander, Z. Chen, A. Soragni, M. Huch, Y. A. Zeng, Q. Wang and H. Yu, Nat. Rev. Methods Primers, 2022, 2, 1–21 CrossRef
.
- B. Cunniff, J. E. Druso and J. L. van der Velden, Histochem. Cell Biol., 2021, 155, 301–308 CrossRef CAS PubMed
.
- J. Kim, B. K. Koo and J. A. Knoblich, Nat. Rev. Mol. Cell Biol., 2020, 21, 571–584 CrossRef CAS PubMed
.
- D. Huh, B. D. Matthews, A. Mammoto, M. Montoya-Zavala, H. Y. Hsin and D. E. Ingber, Science, 2010, 328, 1662–1668 CrossRef CAS PubMed
.
- A. Varone, J. K. Nguyen, L. Leng, R. Barrile, J. Sliz, C. Lucchesi, N. Wen, A. Gravanis, G. A. Hamilton, K. Karalis and C. D. Hinojosa, Biomaterials, 2021, 275, 120957 CrossRef CAS PubMed
.
- S. H. Han and R. K. Mallampalli, Ann. Am. Thorac. Soc., 2015, 12, 765–774 CrossRef PubMed
.
- J. Herrera, C. A. Henke and P. B. Bitterman, J. Clin. Invest., 2018, 128, 45–53 CrossRef PubMed
.
- M. W. Parker, D. Rossi, M. Peterson, K. Smith, K. Sikström, E. S. White, J. E. Connett, C. A. Henke, O. Larsson and P. B. Bitterman, J. Clin. Invest., 2014, 124, 1622–1635 CrossRef CAS PubMed
.
- A. Sundarakrishnan, Y. Chen, L. D. Black, B. B. Aldridge and D. L. Kaplan, Adv. Drug Delivery Rev., 2018, 129, 78–94 CrossRef CAS PubMed
.
- L. Horvath, Y. Umehara, C. Jud, F. Blank, A. Petri-Fink and B. Rothen-Rutishauser, Sci. Rep., 2015, 5, 7974 CrossRef CAS PubMed
.
- W. L. Ng, T. C. Ayi, Y. C. Liu, S. L. Sing, W. Y. Yeong and B. H. Tan, Int. J. Bioprint., 2021, 7, 1–15 CrossRef PubMed
.
- T. Yanagihara, S. G. Chong, M. Vierhout, J. A. Hirota, K. Ask and M. Kolb, Expert Opin. Drug Discovery, 2020, 15, 931–941 CrossRef CAS PubMed
.
-
S. D. Shukla, K. S. Vanka, A. Chavelier, M. D. Shastri, M. M. Tambuwala, H. A. Bakshi, K. Pabreja, M. Q. Mahmood and R. F. O'Toole, in Targeting Chronic Inflammatory Lung Diseases Using Advanced Drug Delivery Systems, Elsevier, 2020, pp. 1–31 Search PubMed
.
- A. Agustí, C. Vogelmeier and R. Faner, Am. J. Physiol. Lung Cell. Mol. Physiol., 2020, 319, L879–L883 CrossRef PubMed
.
- R. B. Hopkins, N. Burke, C. Fell, G. Dion and M. Kolb, Eur. Respir. J., 2016, 48, 187–195 CrossRef PubMed
.
- C. Raherison and P. O. Girodet, Eur. Respir. Rev., 2009, 18, 213–221 CrossRef CAS PubMed
.
- R. Surolia, F. J. Li, Z. Wang, H. Li, G. Liu, Y. Zhou, T. Luckhardt, S. Bae, R. M. Liu, S. Rangarajan, J. de Andrade, V. J. Thannickal and V. B. Antony, JCI Insight, 2017, 2, 91377 CrossRef PubMed
.
- J. C. Mejías, M. R. Nelson, O. Liseth and K. Roy, Lab Chip, 2020, 20, 3601–3611 RSC
.
- M. Felder, A. O. Stucki, J. D. Stucki, T. Geiser and O. T. Guenat, Integr. Biol., 2014, 6, 1132–1140 CrossRef CAS PubMed
.
- B. Ruaro, R. Pozzan, P. Confalonieri, S. Tavano, M. Hughes, M. M. Cerinic, E. Baratella, E. Zanatta, S. Lerda, P. Geri, M. Confalonieri and F. Salton, Pharmaceuticals, 2022, 15, 1033 CrossRef CAS PubMed
.
- M. Felder, B. Trueeb, A. O. Stucki, S. Borcard, J. D. Stucki, B. Schnyder, T. Geiser and O. T. Guenat, Front. Bioeng. Biotechnol., 2019, 7, 1–5 CrossRef PubMed
.
- B. Wu, L. Tang and M. Kapoor, Semin. Arthritis Rheum., 2021, 51, 310–317 CrossRef CAS PubMed
.
- J. R. Tse and A. J. Engler, Curr. Protoc. Cell Biol., 2010, 10, 1–16 Search PubMed
.
- J. L. Balestrini, A. L. Gard, A. Liu, K. L. Leiby, J. Schwan, B. Kunkemoeller, E. A. Calle, A. Sivaraptna, T. Lin, S. Dimitrievska, S. G. Campbell and L. E. Niklason, Integr. Biol., 2015, 7, 1598–1610 CrossRef CAS PubMed
.
- J. D. O’Neill, R. Anfang, A. Anandappa, J. Costa, J. J. Javidfar, H. M. Wobma, G. Singh, D. O. Freytes, M. D Bacchetta, J. R. Sonett and G. Vunjak-Novakovic, Ann. Thorac. Surg., 2013, 96, 1046–1056 CrossRef PubMed
.
- M. Ghaedi, E. A. Calle, J. J. Mendez, A. L. Gard, J. Balestrini, A. Booth, P. F. Bove, L. Gui, E. S. White and L. E. Niklason, J. Clin. Invest., 2013, 123, 4950–4962 CrossRef CAS PubMed
.
- A. J. Booth, R. Hadley, A. M. Cornett, A. A. Dreffs, S. A. Matthes, J. L. Tsui, K. Weiss, J. C. Horowitz, V. F. Fiore, T. H. Barker, B. B. Moore, F. J. Martinez, L. E. Nikkalson and E. S. White, Am. J. Respir. Crit. Care Med., 2012, 186, 866–876 CrossRef CAS PubMed
.
- B. Grigoryan, S. J. Paulsen, D. C. Corbett, D. W. Sazer, C. L. Fortin, A. J. Zaita, P. T. Greenfield, N. J. Calafat, J. P. Gounley, A. H. Ta, F. Johansson, A. Randles, J. E. Rosenkrantz, J. D. Louis-Rosenberg, P. A. Galie, K. R. Stevens and J. S. Miller, Science, 2019, 364, 458–464 CrossRef CAS PubMed
.
- B. Wang, T. Qinglai, Q. Yang, M. Li, S. Zeng, X. Yang, Z. Xiao, X. Tong, L. Lei and S. Li, Mater. Today Bio, 2023, 18, 100530 CrossRef CAS PubMed
.
- R. L. Siegel, K. D. Miller, H. E. Fuchs and A. Jemal, CA Cancer J. Clin., 2022, 72, 7–33 CrossRef PubMed
.
- A. Soni, Z. Ren, O. Hameed, D. Chanda, C. J. Morgan, G. P. Siegal and S. Wei, Am. J. Clin. Pathol., 2015, 143, 471–478 CrossRef PubMed
.
- A. I. Riggio, K. E. Varley and A. L. Welm, Br. J. Cancer, 2021, 124, 13–26 CrossRef PubMed
.
- H. Pan, R. Gray, J. Braybrooke, C. Davies, C. Taylor, P. McGale, R. Peto, K. I. Pritchard, J. Bergh, M. Dowsett and D. F. Hayes, N. Engl. J. Med., 2017, 377, 1836–1846 CrossRef PubMed
.
- E. Copson, B. Eccles, T. Maishman, S. Getty, L. Stanton, R. I. Cutress, D. G. Altman, L. Durcan, P. Simmonds, G. Lawrence, L. Jones, J. Bliss and D. Eccles, J. Natl. Cancer Inst., 2013, 105, 978–988 CrossRef CAS PubMed
.
- F. K. Turrell, R. Orha, N. J. Guppy, A. Gillespie, M. Guelbert, C. Starling, S. Haider and C. M. Isacke, Nat. Cancer, 2023, 4, 468–484 CrossRef CAS PubMed
.
- M. Montagner, R. Bhome, S. Hooper, P. Chakravarty, X. Qin, J. Sufi, A. Bhargava, C. D. H. Ratcliffe, Y. Naito, A. Pocaterra, C. J. Tape and E. Sahai, Nat. Cell Biol., 2020, 22, 289–296 CrossRef CAS PubMed
.
- J. Wang, R. Ocadiz-Ruiz, M. S. Hall, G. G. Bushnell, S. M. Orbach, J. Y. Decker, R. M. Raghani, Y. Zhang, A. H. Morris, J. S. Jeruss and L. D. Shea, Nat. Commun., 2023, 14, 4790 CrossRef CAS PubMed
.
- M. Furukawa, S. Wheeler, A. M. Clark and A. Wells, PLoS One, 2015, 10, e0118060 CrossRef PubMed
.
- T. Bui, Y. Gu, F. Ancot, V. Sanguin-Gendreau, D. Zuo and W. J. Muller, Oncogene, 2022, 41, 527–537 CrossRef CAS PubMed
.
- J. B. Xu, J. Cao, J. Xia, Y. Zhu, Y. He, M. G. Cao, B. M. Fang, J. P. Thiery and W. Zhou, Breast Cancer Res., 2023, 25, 59 CrossRef CAS PubMed
.
- R. Jiang, J. Huang, X. Sun, X. Chu, F. Wang, J. Zhou, Q. Fan and L. Pang, BMC Cancer, 2022, 22, 1–9 CrossRef PubMed
.
- A. N. Kundu, C. E. Dougan, S. Mahmoud, A. Kilic, A. Panagiotou, N. R. Richbourg, N. Irakoze and S. R. Peyton, Adv. Mater., 2023, 35, 2301493 CrossRef CAS PubMed
.
- E. M. Ovadia, L. Pradhan, L. A. Sawicki, J. E. Cowart, R. E. Huber, S. W. Polson, C. Chen, K. L. van Golen, K. E. Ross, C. H. Wu and A. M. Kloxin, Adv. Biosyst., 2020, 4, e2000119 CrossRef PubMed
.
- J. H. Kim, N. Schaible, J. K. Hall, E. Bartolák-Suki, Y. Deng, J. Herrmann, A. Sonnenberg, H. P. Behrsing, K. R. Lutchen, R. Krishnan and B. Suki, Sci. Adv., 2023, 9, eadf2535 CrossRef PubMed
.
- Y. Wang, T. Zhou, S. Zhao, N. Li, S. Sun and M. Li, Cancer Manage. Res., 2023, 15, 409–422 CrossRef PubMed
.
- S. Asano, S. Ito, K. Takahashi, K. Furuya, M. Kondo, M. Sokabe and Y. Hasegawa, Physiol. Rep., 2017, 5, e13281 CrossRef PubMed
.
- J. Thévenot, H. Oliveira, O. Sandre and S. Lecommandoux, Chem. Soc. Rev., 2013, 42, 7099–7116 RSC
.
- S. Jang and S. Park, Sens. Actuators, B, 2023, 384, 133654 CrossRef CAS
.
- E. Lenzi, D. Jimenez de Aberasturi and L. M. Liz-Marzán, ACS Sens., 2019, 4, 1126–1137 CrossRef CAS PubMed
.
- Z. Yuan, K. Memarzadeh, A. S. Stephen, R. P. Allaker, R. A. Brown and J. Huang, Sci. Rep., 2018, 8, 16270 CrossRef PubMed
.
- C. De La Encarnación, E. Lenzi, M. Henriksen-Lacey, B. Molina, K. Jenkinson, A. Herrero, L. Colás, P. Ramos-Cabrer, J. Toro-Mendoza, I. Orue, J. Langer, S. Bals, D. Jimenez de Aberasturi and L. M. Liz-Marzán, J. Phys. Chem. C, 2022, 126, 19519–19531 CrossRef
.
- S. Leal-Marin, G. Gallaway, K. Höltje, A. Lopera-Sepulveda, B. Glasmacher and O. Gryshkov, Curr. Dir. Biomed. Eng., 2021, 7, 460–463 CrossRef
.
- G. Thomas, J. Boudon, L. Maurizi, M. Moreau, P. Walker, I. Severin, A. Oudot, C. Goze, S. Poty, J. M. Vrigneaud, F. Demoisson, F. Denat, F. Brunotte and N. Millot, ACS Omega, 2019, 4, 2637–2648 CrossRef CAS PubMed
.
- L. Beola, L. Asín, R. M. Fratila, V. Herrero, J. M. de la Fuente, V. Grazú and L. Gutiérrez, ACS Appl. Mater. Interfaces, 2018, 10, 44301–44313 CrossRef CAS PubMed
.
- J. Pellico, J. Ruiz-Cabello and F. Herranz, ACS Appl. Nano Mater., 2023, 6, 20523–20538 CrossRef CAS
.
- Y. Du, W. Du, D. Lin, M. Ai, S. Li and L. Zhang, Micromachines, 2023, 14, 167 CrossRef PubMed
.
- A. Marino, J. Barsotti, G. De Vito, C. Filippeschi, B. Mazzolai, V. Piazza, M. Labardi, V. Mattoli and G. Ciofani, ACS Appl. Mater. Interfaces, 2015, 7, 25574–25579 CrossRef CAS PubMed
.
- Z. H. Li, G. L. Li, X. Y. Wang and Z. Zhao, J. Mater. Sci. Technol., 2024, 172, 228–239 CrossRef
.
- H. GhaedRahmati, M. Frounchi and S. Dadbin, Mater. Sci. Eng. B, 2022, 276, 115535 CrossRef CAS
.
- S. J. Mousavi and M. H. Doweidar, Comput. Mech., 2019, 63, 471–489 CrossRef
.
- A. Cafarelli, A. Marino, L. Vannozzi, J. Puigmartí-Luis, S. Pané, G. Ciofani and L. Ricotti, ACS Nano, 2021, 15, 11066–11086 CrossRef CAS PubMed
.
- M. Shevach, S. Fleischer, A. Shapira and T. Dvir, Nano Lett., 2014, 14, 5792–5796 CrossRef CAS PubMed
.
- C. García-Astrain, E. Lenzi, D. Jimenez de Aberasturi, M. Henriksen-Lacey, M. R. Binelli and L. M. Liz-Marzán, Adv. Funct. Mater., 2020, 30, 2005407 CrossRef
.
- F. Diehl, S. Hageneder, S. Fossati, S. K. Auer, J. Dostalek and U. Jonas, Chem. Soc. Rev., 2022, 51, 3926–3963 RSC
.
- M. Malki, S. Fleischer, A. Shapira and T. Dvir, Nano Lett., 2018, 18, 4069–4073 CrossRef CAS PubMed
.
- Y. Wang, E. S. Keneth, A. Kamyshny, G. Scalet, F. Auricchio and S. Magdassi, Adv. Mater. Technol., 2022, 7, 2101058 CrossRef CAS
.
- A. Espinosa, J. Kolosnjaj-Tabi, A. Abou-Hassan, A. P. Sangnier, A. Curcio, A. K. A. Silva, Riccardo Di Corato, S. Neveu, T. Pellegrino, L. M. Liz-Marzán and C. Wilhelm, Adv. Funct. Mater., 2018, 28, 1803660 CrossRef
.
- S. Chortarea, K. Fytianos, L. Rodriguez-Lorenzo, A. Petri-Fink and B. Rothen-Rutishauser, Nanomedicine, 2018, 13, 1169–1185 CrossRef CAS PubMed
.
- L. C. Kennedy, L. R. Bickford, N. A. Lewinski, A. J. Coughlin, Y. Hu, E. S. Day, J. L. West and R. A. Drezek, Small, 2011, 7, 169–183 CrossRef CAS PubMed
.
- N. Du, F. Ye, J. Sun and K. Liu, ChemBioChem, 2022, 23, e202100416 CrossRef CAS PubMed
.
- M. Bril, S. Fredrich and N. A. Kurniawan, Smart Mater. Med., 2022, 3, 257–273 CrossRef
.
- W. Di Cianni, M. de la Mata, F. J. Delgado, J. Hernández-Saz, M. Herrera, S. I. Molina, M. Giocondo and A. Sanz de León, Polym. Test., 2023, 117, 107869 CrossRef CAS
.
- N. Löwa, J. M. Fabert, D. Gutkelch, H. Paysen, O. Kosch and F. Wiekhorst, J. Magn. Magn. Mater., 2019, 469, 456–460 CrossRef
.
- S. S. Athukorala, T. S. Tran, R. Balu, V. K. Truong, J. Chapman, N. K. Dutta and N. R. Choudhury, Polymers, 2021, 13, 1–21 CrossRef PubMed
.
- D. Jimenez de Aberasturi, A. B. Serrano-Montes and L. M. Liz-Marzán, Adv. Opt. Mater., 2015, 3, 602–617 CrossRef CAS
.
- J. Martínez-Ramírez, M. Toldos-Torres, E. Benayas, N. Villar-Gómez, L. Fernández-Méndez, F. M. Espinosa, R. García, S. Veintemillas-Verdaguer, M. del P. Morales and M. C. Serrano, Acta Biomater., 2024, 176, 156–172 CrossRef PubMed
.
- M. Colombo, S. Carregal-Romero, M. F. Casula, L. Gutiérrez, M. P. Morales, I. B. Böhm, J. T. Heverhagen, D. Prosperi and W. J. Parak, Chem. Soc. Rev., 2012, 41, 4306–4334 RSC
.
- C.-T. Pan, K. Dutt, C.-K. Yen, A. Kumar, A. C. Kaushik, D.-Q. Wei, A. Kumar, Z.-H. Wen, W.-H. Hsu and Y.-L. Shiue, Comb. Chem. High Throughput Screening, 2022, 25, 720–729 CrossRef CAS PubMed
.
- Y. Zhao, Q. Liao, G. Zhang, Z. Zhang, Q. Liang, X. Liao and Y. Zhang, Nano Energy, 2015, 11, 719–727 CrossRef CAS
.
- F. Momeni, S. M. M. Hassani, X. Liu and J. Ni, Mater. Des., 2017, 122, 42–79 CrossRef CAS
.
- Y. Zhang, X. Y. Yin, M. Zheng, C. Moorlag, J. Yang and Z. L. Wang, J. Mater. Chem. A, 2019, 7, 6972–6984 RSC
.
- C. Ou, A. L. Sangle, A. Datta, Q. Jing, T. Busolo, T. Chalklen, V. Narayan and S. Kar-Narayan, ACS Appl. Mater. Interfaces, 2018, 10, 19580–19587 CrossRef CAS PubMed
.
- G. Kocak, C. Tuncer and V. Bütün, Polym. Chem., 2016, 8, 144–176 RSC
.
- F. Doberenz, K. Zeng, C. Willems, K. Zhang and T. Groth, J. Mater. Chem. B, 2020, 8, 607–628 RSC
.
- R. Jia, L. Teng, L. Gao, T. Su, L. Fu, Z. Qiu and Y. Bi, Int. J. Nanomed., 2021, 16, 1525–1551 CrossRef PubMed
.
- Q. L. Zhu, C. Du, Y. Dai, M. Daab, M. Matejdes, J. Breu, W. Hong, Q. Zheng and Z. L. Wu, Nat. Commun., 2020, 11, 5166 CrossRef CAS PubMed
.
- Y. Dong, J. Wang, X. Guo, S. Yang, M. O. Ozen, P. Chen, X. Liu, W. Du, F. Xiao, U. Demirci and B. F. Liu, Nat. Commun., 2019, 10, 4087 CrossRef PubMed
.
- C. Wang, X. Liu, V. Wulf, M. Vázquez-González, M. Fadeev and I. Willner, ACS Nano, 2019, 13, 3424–3433 CrossRef CAS PubMed
.
- Y. Luo, L. Fan, C. Liu, H. Wen, S. Wang, P. Guan, D. Chen, C. Ning, L. Zhou and G. Tan, Bioact. Mater., 2022, 7, 98–111 CAS
.
- T. R. Yeazel and M. L. Becker, Biomacromolecules, 2020, 21, 3957–3965 CrossRef CAS PubMed
.
- S. Yu, N. Sadaba, E. Sanchez-Rexach, S. L. Hilburg, L. D. Pozzo, G. Altin-Yavuzarslan, L. M. Liz-Marzán, D. Jimenez de Aberasturi, H. Sardon and A. Nelson, Adv. Funct. Mater., 2023, 2311209 Search PubMed
.
- G. Baffou and R. Quidant, Laser Photonics Rev., 2013, 7, 171–187 CrossRef CAS
.
- J. Reguera, J. Langer, D. Jimenez de Aberasturi and L. M. Liz-Marzán, Chem. Soc. Rev., 2017, 46, 3866–3885 RSC
.
- X. Xu, Y. Liu, W. Fu, M. Yao, Z. Ding, J. Xuan, D. Li, S. Wang, Y. Xia and M. Cao, Polymers, 2020, 12, 580–601 CrossRef CAS PubMed
.
- Y. Zhang, J. Liang, N. Cao, J. Gao, L. Song and X. Tang, Cell Death Discovery, 2022, 8, 500 CrossRef CAS PubMed
.
- Q. Wan, X. Zhang, D. Zhou, R. Xie, Y. Cai, K. Zhang and X. Sun, J. Nanobiotechnol., 2023, 21, 215 CrossRef CAS PubMed
.
- M. Skibba, A. Drelich, M. Poellmann, S. Hong and A. R. Brasier, Front. Pharmacol., 2020, 11, 607680 Search PubMed
.
- A. Raj, R. K. Thomas, L. Vidya, V. M. Aparna, S. Neelima and C. Sudarsanakumar, Sci. Rep., 2023, 13, 9045 CrossRef CAS PubMed
.
- S. Carregal-Romero, H. Groult, O. Cañadas, N. A. Gonzalez, A. V. Lechuga-Vieco, B. García-Fojeda, F. Herranz, J. Pellico, A. Hidalgo, C. Casals and J. Ruiz-Cabello, Biomater. Adv., 2022, 134, 112551 CrossRef CAS PubMed
.
- J. Roller, M. W. Laschke, T. Tschernig, R. Schramm, N. T. Veith, H. Thorlacius and M. D. Menger, Nanomedicine, 2011, 7, 753–762 CrossRef CAS PubMed
.
- J. Kim, P. Chhour, J. Hsu, H. I. Litt, V. A. Ferrari, R. Popovtzer and D. P. Cormode, Bioconjugate Chem., 2017, 28, 1581–1597 CrossRef CAS PubMed
.
- H. Paysen, N. Loewa, A. Stach, J. Wells, O. Kosch, S. Twamley, M. R. Makowski, T. Schaeffter, A. Ludwig and F. Wiekhorst, Sci. Rep., 2020, 10, 1922 CrossRef CAS PubMed
.
- B. Yan, S. T. Kim, C. S. Kim, K. Saha, D. F. Moyano, Y. Xing, Y. Jiang, A. L. Roberts, F. S. Alfonso, V. M. Rotello and R. W. Vachet, J. Am. Chem. Soc., 2013, 135, 12564–12567 CrossRef CAS PubMed
.
- X. Y. Zhou, K. E. Jeffris, E. Y. Yu, B. Zheng, P. W. Goodwill, P. Nahid and S. M. Conolly, Phys. Med. Biol., 2017, 62, 3510–3522 CrossRef CAS PubMed
.
- S. Eom, S. Y. Lee, J. T. Park and I. Choi, Adv. Sci., 2023, 10, e2301395 CrossRef PubMed
.
- W. H. Skinner, N. Robinson, G. R. Hardisty, H. Fleming, A. Geddis, M. Bradley, R. D. Gray and C. J. Campbell, Chem. Commun., 2023, 59, 3249–3252 RSC
.
- C. Shi, X. Cao, X. Chen, Z. Sun, Z. Xiang, H. Zhao, W. Qian and X. Han, Biomaterials, 2015, 58, 10–25 CrossRef CAS PubMed
.
- X. Cao, X. Chen, C. Shi, M. Zhang, W. Lu, L. Li, J. Dong, X. Han and W. Qian, RSC Adv., 2016, 6, 14321–14328 RSC
.
- D. Jimenez de Aberasturi, M. Henriksen-Lacey, L. Litti, J. Langer and L. M. Liz-Marzán, Adv. Funct. Mater., 2020, 30, 1909655 CrossRef CAS
.
- E. Lenzi, D. Jimenez de Aberasturi, M. Henriksen-Lacey, P. Piñeiro, A. J. Muniz, J. Lahann and L. M. Liz-Marzán, ACS Appl. Mater. Interfaces, 2022, 14, 20708–20719 CrossRef CAS PubMed
.
-
N. Thondavada, R. Chokkareddy, N. V. Naidu and G. G. Redhi, in Nanomaterials in Diagnostic Tools and Devices, Elsevier, 2020, pp. 417–437 Search PubMed
.
- Y. Xu, X. Wang, W. L. Zhang, F. Lv and S. Guo, Chem. Soc. Rev., 2018, 47, 586–625 RSC
.
- S. Y. Lim, W. Shen and Z. Gao, Chem. Soc. Rev., 2014, 44, 362–381 RSC
.
- L. Cardo, L. Martínez-Parra, M. Cesco, B. M. Echeverría-Beistegui, M. Martínez-Moro, N. Herrero-Álvarez, M. Beraza-Cabrerizo, S. Carregal-Romero, P. Ramos-Cabrer, J. Ruiz-Cabello and M. Prato, Small, 2023, 19, 2206442 CrossRef CAS PubMed
.
- A. Avasthi, C. Caro, E. Pozo-Torres, M. P. Leal and M. L. García-Martín, Top. Curr. Chem., 2020, 378, 40 CrossRef CAS PubMed
.
- Z. W. Tay, P. Chandrasekharan, X. Y. Zhou, E. Yu, B. Zheng and S. Conolly, Theranostics, 2018, 8, 3676–3687 CrossRef CAS PubMed
.
- N. Lee and T. Hyeon, Chem. Soc. Rev., 2012, 41, 2575–2589 RSC
.
- M. A. Karageorgou, P. Bouziotis, E. Stiliaris and D. Stamopoulos, Nanomaterials, 2023, 13, 503 CrossRef CAS PubMed
.
- J. R. Ashton, E. B. Gottlin, E. F. Patz, J. L. West and C. T. Badea, PLoS One, 2018, 13, e0206950 CrossRef PubMed
.
- A. Doctor, V. Seifert, M. Ullrich, S. Hauser and J. Pietzsch, Cancers, 2020, 12, 1–28 CrossRef PubMed
.
- P. S. Valera, J. Plou, I. García, I. Astobiza, C. Viera, A. M. Aransay, J. E. Martin, I. R. Sasselli, A. Carracedo and L. M. Liz-Marzán, Proc. Natl. Acad. Sci. U. S. A., 2023, 120, e2311674120 CrossRef CAS PubMed
.
- S. R. Polio, A. N. Kundu, C. E. Dougan, N. P. Birch, D. E. Aurian-Blajeni, J. D. Schiffman, A. J. Crosby and S. R. Peyton, PLoS One, 2018, 13, e0204765 CrossRef PubMed
.
- E. T. Hoffman, F. E. Uhl, L. Asarian, B. Deng, C. Becker, J. J. Uriarte, I. Downs, B. Young and D. J. Weiss, Biomaterials, 2023, 293, 121960 CrossRef CAS PubMed
.
- L. Sikkema, C. Ramírez-Suástegui, D. C. Strobl, T. E. Gillett, L. Zappia, E. Madissoon, N. S. Markov, L. E. Zaragosi, Y. Ji, M. Ansari, M. J. Arguel, L. Apperloo, M. Banchero, C. Bécavin, M. Berg, E. Chichelnitsky, M. Chung, A. Collin, A. C. A. Gay, J. Gote-Scniering, B. H. Kashani, K. Inecik, M. Jain, T. S. Kapellos, C. J. Kole, S. Leroy, C. H. Mayr, A. J. Oliver, M. von Papen, L. Peter, C. J. Taylor, T. Waltzthoeni, Chuan Xu, L. T. Bui, C. De Donno, L. Dony, A. Faiz, M. Guo, A. J. Gutierrez, L. Heumos, L. Huang, I. L. Ibarra, N. L. Jackson, P. K. L. Murty, M. Lotfollahi, T. Tabib, C. Talavera-López, K. J. Travaglini, A. Wilbrey-Clark, K. B. Worlock, M. Yoshida, M. van der Berge, J. Bossé, T. J. Desai, O. Eickelberg, N. Kaminski, M. A. Krasnow, R. Lafyatis, M. Z. Nikolic, J. E. Powell, J. Rajagopal, M. Rojas, O. Rozenblatt-Rosen, M. A. Seibold, D. Sheppard, D. P. Shepherd, D. D. Sin, W. Timens, A. M. Tsankov, J. Whitsett, Y. Xu, N. E. Banovich, P. Barbry, T. E. Duong, C. S. Falk, K. B. Meyer, J. A. Kropski, D. Pe’er, H. B. Schiller, P. R. Tata, J. L. Schultze, S. A. Teichmann, A. V. Misharin, M. C. Nawijn, M. D. Luecken and F. J. Theis, Nat. Med., 2023, 29, 1563–1577 CrossRef CAS PubMed
.
- O. Rosmark, E. Åhrman, C. Müller, L. E. Rendin, L. Eriksson, A. Malmström, O. Hallgren, A. K. Larsson-Callerfelt, G. Westergren-Thorsson and J. Malmström, Sci. Rep., 2018, 8, 5409 CrossRef PubMed
.
Footnote |
† Both authors contributed equally to this manuscript. |
|
This journal is © The Royal Society of Chemistry 2024 |