Implications of sample treatment on characterization of riverine dissolved organic matter†
Received
1st February 2022
, Accepted 28th March 2022
First published on 4th April 2022
Abstract
High-resolution mass spectrometry techniques are widely used in the environmental sciences to characterize natural organic matter and, when utilizing these instruments, researchers must make multiple decisions regarding sample pre-treatment and the instrument ionization mode. To identify how these choices alter organic matter characterization and resulting conclusions, we analyzed a collection of 17 riverine samples from East River, CO (USA) under four PPL-based Solid Phase Extraction (SPE) treatment and electrospray ionization polarity (e.g., positive and negative) combinations: SPE (+), SPE (−), non-SPE (−), and non-SPE (+). The greatest number of formula assignments were achieved with SPE-treated samples due to the removal of compounds that could interfere with ionization. Furthermore, the SPE (−) treatment captured the most formulas across the widest chemical compound diversity. In addition to a reduced number of assigned formulas, the non-SPE datasets resulted in altered thermodynamic interpretations that could cascade into incomplete assumptions about the availability of organic matter pools for heterotrophic microbial respiration. Thus, we infer that the SPE (−) treatment is the best single method for characterizing environmental organic matter pools unless the focus is on lipid-like compounds, in which case we recommend a combination of SPE (−) and SPE (+) to adequately characterize these molecules.
Environmental significance
FTICR-MS is being increasingly utilized by the scientific community to characterize complex natural organic matter pools and how they are impacted with environmental change. When using FTICR-MS for environmental research, researchers must make many decisions regarding sample pre-treatment and instrument configuration (e.g., ionization method). Ideally, these critical decisions should be informed by data-driven patterns because they can alter results and subsequent conclusions. Here, we analyze 17 riverine water samples using four separate analysis methods (extracted vs. non-extracted, positive ionization vs. negative ionization) to provide data-driven guidance for future researchers.
|
Introduction
The development and increasing utilization of high-resolution mass spectrometry (HRMS) techniques (e.g., FTICR-MS, Orbitrap-MS, IMS-MS) has allowed scientists to characterize the molecular compounds that constitute natural organic matter1 (NOM). These approaches have been applied to samples across a diverse range of environments,2–5 and have yielded new insights into NOM processing. More recently, attempts have been made to couple these high-resolution chemical analyses with microbiome data to directly link measurements of microbial function with changes in chemical species.6–8 One of these approaches involves the analysis of dissolved organic matter (DOM) in a thermodynamic framework through which the nominal oxidation state of carbon (NOSC) can be calculated. Specifically, an empirical relationship between the NOSC and the standard molar Gibbs free energies of the oxidation half reactions of organic compounds9 allows researchers to quantify the thermodynamic favorability of DOM pools as electron donors for microbial respiration from HRMS data. One study using this approach demonstrated that aerobic respiration increased with increasing DOM thermodynamic favorability under carbon limiting conditions.6 Similarly, a study of carbon dynamics within an anoxic aquifer revealed the preservation of DOM pools that yielded insufficient energy when coupled to the reduction of sulfate.10 Other recent studies taking a similar approach have further revealed relationships between DOM thermodynamics and various ecological measurements, including biogeochemical function and microbial community assembly.7,11–13
There are many pre-treatment and instrument options to choose when utilizing HRMS techniques that could bias the resulting dataset. Environmental samples can be analyzed without enrichment or treatment but are commonly pre-treated using PPL-based solid phase extraction (SPE) to concentrate DOM or remove species that may interfere with the ionization of organic compounds14,15 (e.g., salts). During SPE, DOM is retained on a sorbent then eluted using a solvent such as methanol. Polymer-based sorbents (e.g., PPL) are commonly used during SPE due to their high extraction efficiency and representative character of retained DOM.16,17 However, DOM recovery by SPE is incomplete and studies have shown that single-sorbent SPE methods can result in the preferential loss of specific compound classes such as organo-sulfur compounds,18 aliphatic amines/amides, and tannin-like compounds with a high oxygen content.19,20 Electrospray ionization (ESI) is a common ionization technique in HRMS because it can ionize a wide range of polar, hydrophilic molecules with diverse functional groups common in DOM, results in minimal-to-no fragmentation of DOM molecules (i.e., is a ‘soft’ ionization technique), and can be used in either positive or negative mode.21 DOM can contain acidic functional groups that can be readily deprotonated in ESI (−) or basic functional groups (e.g., amines) that can be readily protonated and ionized efficiently in ESI (+). Thus, the chosen ESI mode can further govern the final HRMS DOM spectra and the chosen ESI mode has been found to result in different mass spectra for the same sample.22,23 Ohno et al. (2016)24 found that ESI (+) was better at detecting aliphatic and carbohydrate-like molecules and that the ionization of these molecules was generally suppressed in samples rich in aromatics ionized in ESI (−) mode. The selected ESI mode can further impact downstream data through the generation of unintended adducts which can confound molecular formula assignment. For example, ESI (−) can result in the formation of chloride-containing ions due to salinity interference.16 Finally, Hawkes et al. (2020)25 performed a cross-lab comparability study of HRMS data from different instruments to provide valuable metrics for future data benchmarking and further revealed patterns based upon ionization modes.
Although other studies have analyzed impacts of instrumentation, sample preparation, or ionization modes, no study has systematically examined the combined impacts of SPE and ionization mode choices or discussed changes to thermodynamic or microbial metabolic interpretations. Here, we analyze a set of 17 surface and pore water samples from the East River, CO, using four different Fourier transform ion cyclotron resonance mass spectrometry (FTICR-MS) methods (SPE or non-SPE, positive-ion or negative-ion ESI mode) with the goal of providing guidance to researchers on what method is most appropriate for their research question. While it is difficult to completely standardize these treatments (particularly regarding differences arising due to SPE), we have selected these because they represent common routes of DOM analyses in complex mixtures. We show that both sample pre-treatment and ESI mode choice influences the detection of different DOM molecules and can therefore bias the interpretation of microbial DOM processing within a given sample.
Methods
Sample collection
Surface and pore water samples (2 surface, 15 pore) were collected from the East River (CO, USA) on a 200 m reach encompassing one meander that lies within the U.S. Department of Energy-supported Lawrence Berkeley National Laboratory's Watershed Science Focus Area (Fig. S1†). This combination of 17 previously-analyzed samples were selected because they had representatives across all treatment types; see Nelson et al. (2019)26 and Saup et al. (2019)27 for more detailed information on sampling efforts and information on the remainder of the samples. Briefly, pore water samples were collected from their respective depths (Table S1†) using a 0.6 cm diameter, stainless steel pore water sipper with a screen length of 5 cm attached to a syringe (MHE Products, MI, USA). One tubing volume of approximately 30 mL was discarded before sampling and sample was immediately filtered through 0.22 μm Sterivex filters (housings made of Eastar co-polyester; Massachusetts, USA). The 20 mL aliquot for the data presented here was filtered into a pre-combusted amber glass vial and immediately placed in a cooler on ice for transport back to the lab. Dissolved organic carbon (DOC) concentrations and other relevant metadata generated from previous manuscripts (e.g., Nelson et al. (2019)26 and Saup et al. (2019)27) can be found in Table S1†.
Sample preparation and ESI-FTICR-MS data collection
Fourier Transform Ion Cyclotron Resonance Mass Spectrometry (FTICR-MS) was used to provide ultra-high resolution organic matter characterization. For the samples undergoing solid phase extraction, aqueous samples (NPOC 0.33–0.99 mg C per L) were acidified to pH 2 with 85% phosphoric acid and extracted with Bond Elut PPL cartridges (Agilent, Santa Clara, CA), following Dittmar et al. (2008).16 For those samples which were not extracted, 250 μL of sample were mixed with 500 μL of LC-MS grade MeOH. A 12 Tesla (12T) Bruker SolariX Fourier transform ion cyclotron resonance mass spectrometer (Bruker, Billerica, MA) located at the Environmental Molecular Sciences Laboratory in Richland, WA was used to collect high-resolution mass spectra of the organic matter found in each sample. Samples were directly injected into the instrument using a custom automated direct infusion cart that performed two offline blanks between each sample.28 A Bruker SolariX electrospray ionization (ESI) source was used in positive and negative modes with applied voltages of +4.4 kV and −4.2 kV, respectively. Ion accumulation time was optimized between 50 and 80 ms. One hundred and forty-four transients were co-added into a 4MWord time domain (transient length of 1.1 s) with a spectral mass window of m/z 100–900, yielding an average resolution of 265 K at m/z 400. Spectra were internally recalibrated in the mass domain using homologous series separated by 14 Da (CH2 groups) (File S1†). The mass measurement accuracy was typically within 1 ppm for singly charged ions across the mass window. Bruker Daltonics DataAnalysis (version 4.2) was used to convert mass spectra to a list of m/z values by applying the FTMS peak picking module with a signal-to-noise ratio (S/N) threshold set to 7 and absolute intensity threshold to the default value of 100%. Formularity29 was used to align peaks using a 0.5 ppm threshold, and then assign chemical formulas based on exact mass with a mass measurement error <0.5 ppm while allowing for CHONS (Formularity formula settings: O > 0 AND (N + S) < 6 AND S < 3 AND P = 0).
The R package ftmsRanalysis30,31 was used to process the report generated by Formularity and remove peaks that either were outside the desired m/z range (150–900 m/z) or had an isotopic signature, calculate nominal oxidation state of carbon (NOSC), assign putative compound classes,32 and organize the data. We have included a table that describes the number of assigned peaks included after each filtering step (Table S2†). In order to contextualize our results, data published by Hawkes et al. (2020)25 was downloaded in R from https://github.com/BarrowResearchGroup/InterLabStudy using the provided R package. The Hawkes et al. dataset consists of molecular formulas obtained by analyzing four different organic matter standards (Elliot Soil Fulvic Acid, Pony Lake Fulvic Acid, Suwannee River Fulvic Acid, Suwannee River Natural Organic Matter) with either FTICR-MS or Orbitrap-MS under varied ionization modes. NOSC values were calculated and compound classes were assigned to each molecular formula in order to evaluate whether patterns observed using data from this manuscript were broadly transferrable.
Thermodynamics calculations
We calculated the average thermodynamic potential factor (FT) for the oxidation of average DOM pools coupled to the reduction of O2 and SO42− at standard conditions.9,33 The FT was calculated as follows: | 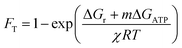 | (1) |
where ΔGr is the Gibbs energy of the reaction, m is the number of moles of ATP synthesized per formula reaction (0.15 and 2 used for aerobic respiration and SO42− reduction, respectively33), ΔGATP denotes the Gibbs energy to synthesize ATP (50 kJ mol−1 used here33), χ is the average stoichiometric number for the reaction of interest, and R and T are the universal gas constant and temperature, respectively. To determine χ for our complex DOM pools, we averaged the values used for different compounds in the analysis presented in LaRowe and Van Cappellan (2011)9 (i.e., averaging the stoichiometric numbers used for amino acids, mononucleotides, saccharides, complex organics, etc.).
KEGG mapping
In order to evaluate differences in potential biogeochemical interpretations, we assigned KEGG compound identifiers (CPD numbers) to observed molecular formulas by mapping them to the KEGG database using the provided REST API.34 In order to avoid potential duplication of CPD numbers, only exact formula matches were considered. Using these CPD numbers, we identified corresponding pathways and qualitatively compared the most represented pathways across treatments. Web scraping was performed using the R package rvest35 and the KEGG mapping scripts are available on GitHub at https://github.com/danczakre/FTICR-Methods-Comparison.
Statistics and plot generation
The statistics program R was used to perform all statistical analyses with the R package ggplot2 used to generate all plots.36,37 Comparisons across treatment groups were performed using Mann–Whitney U tests (wilcox.test) in order to identify significant differences in compound classification, elemental composition, and average NOSC. An additional Kolmogorov–Smirnov test (ks.test) was used to evaluate difference in NOSC distributions within the Hawkes et al. dataset. Multivariate differences across treatment types were investigated using a nonmetric multidimensional scaling (NMDS) graph (metaMDS, vegan package v2.5-7) paired with a permuted analysis of variation (PERMANOVA; adonis, vegan package v2.5-7). All R scripts written to perform these analyses are available on GitHub at https://github.com/danczakre/FTICR-Methods-Comparison.
Results and discussion
Molecular formula detection significantly varies based on sample preparation and ionization type
We evaluated 17 samples that were processed with or without SPE and analyzed in ESI (+) or ESI (−) mode to investigate the impact of method selection on FTICR-MS data. Initial multivariate analyses revealed clear differences in the collected data between methods (Fig. S2†). Considering the molecular formula count for each treatment, the SPE (−) treatment yielded the most assigned formulas (6218) while non-SPE (−) yielded the fewest (1257) (Table S2†). More broadly, the SPE-treated samples contained greater molecular formula counts than non-SPE samples (Fig. 1) and shared more common formulas (Table S1†). These results are expected because SPE is a common method to concentrate DOM in a sample and reduce the impact of salt during ionization.18,39. By ensuring that carbon concentrations are higher, and that salts have a smaller impact on ionization efficiency, more molecular formula will be assigned. Differences in formula count between the two ionization modes are likely impacted by differences in the types of compounds ionized; specifically, river corridor organic matter is typically acidic and often has functional groups rich in oxygen which renders it more likely to be detected using negative mode.25,40 The noted increase in molecular formula observed when comparing the non-SPE (−) vs. non-SPE (+), however, is likely the result of the SPE selecting for compounds more readily observed in negative mode. In other words, the molecular formulas observed under non-SPE conditions are more readily detectable in positive-mode or experience less ionization competition due to salinity. Additionally, the acidification of samples prior to SPE would result in more compounds readily detectable in ESI (−) relative to ESI (+) and basic modification, rather than acidification, prior to SPE may enhance the number of identifiable compounds in ESI (+).41 In these samples, conductivity (used as a proxy for salinity) ranged from 267.8–387.4 μS (Table S1†). PPL-based solid-phase extraction can also lead to compositional shifts in the detected DOM given that some compound types have a higher affinity for the column (e.g., sulfur-containing, hydrophobic, low O/C compounds).18,39 The affinity of sulfur-containing compounds for the PPL-column is apparent in our dataset; SPE retention resulted in more assigned CHONS formulas in non-SPE in both negative and positive ionization mode (7.8% and 18.1%, respectively).
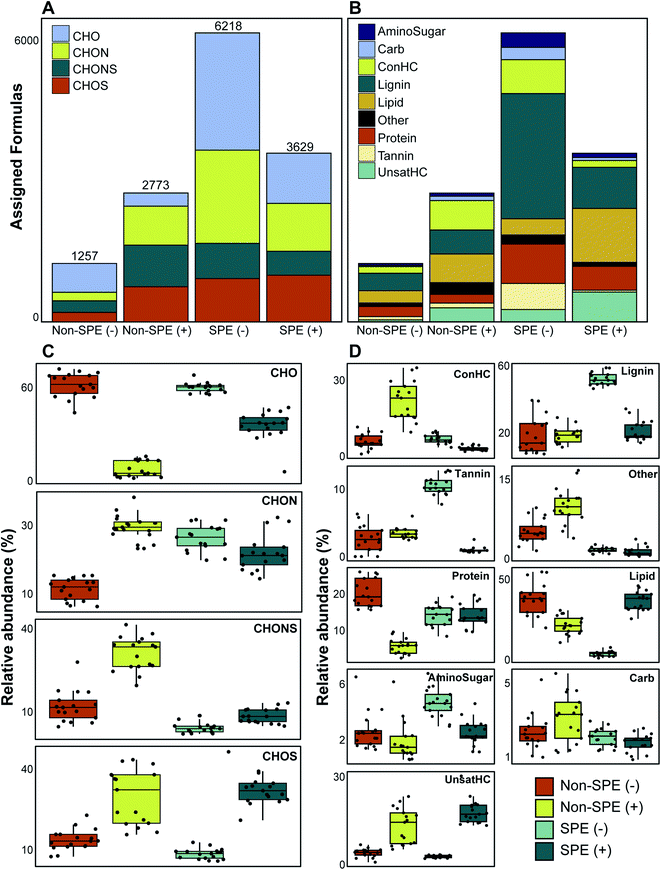 |
| Fig. 1 Classification of formulas assigned from each method by composition (A) and compound class (B). Numbers above bars (A) indicate the total number of detected and classified formulas for the sample set with each method. Trends are consistent in sample-resolved analyses of compositional (C) and compound class (D) variation between methods. | |
We observed the largest variation the proportion of formulas assigned to be CHO-only, with values ranging from 10.2% in non-SPE (+) to 49.3% in non-SPE (−) and significant differences in proportion between each condition (Mann–Whitney U p-value ≪ 0.001) (Fig. 1a). The SPE (−) samples had a similar proportion of CHO-only molecular formula as the non-SPE (−) samples (40.6% and 49.3%, respectively; Mann–Whitney U p-value: 0.392), whereas the ESI (+) samples diverged in CHO-only formula proportions (10.2% and 29.7% in non-SPE and SPE, respectively). The large proportion of CHO-only formula in ESI (−) are consistent with observations that ESI (−) is primarily used to detect O-rich molecular formula.25,40 CHON- and CHOS-containing formulas also demonstrated variability across treatment conditions overall (ranging from 14.4% to 32.2% and 14.9% to 27.7%, respectively; Fig. 1a), while sample-resolved analyses reveal more complex patterns. ESI (+) samples, regardless of extraction method, consistently contained a higher proportion of CHOS-containing formula than ESI (−) samples (Mann–Whitney U test p-value ≪ 0.001). These patterns reflect the potential for ESI (+) to capture more compositionally complex spectra than ESI (−),23 whereas ESI (−) is more ideally suited for capturing CHO-only formula. Because environmental DOM is enriched by acidic, O-rich compounds,24,40,42 the ESI (−) approach likely results in better characterization of the riverine DOM pool studied here.
Van Krevelen-based compound classes exhibited high variability across treatments as well (Fig. 1b). While the non-SPE (+) treatment yielded more molecular formulas than non-SPE (−), both non-SPE ionization modes resulted in similar proportions of compound classes when all samples are considered together. The SPE (−) treatment generated the highest proportion of lignin- and tannin-like formulas (43.3% and 9%, respectively) while the SPE (+) treatment yielded a high proportion of lipid-like compounds (31.9%; Fig. 1b). Sample-resolved analyses further showed these trends (Table 1). Non-SPE (−) samples had a higher proportion of protein-like formulas than the other treatments (Mann–Whitney U test p-value < 0.001) and non-SPE (+) had significantly greater representation of concentrated hydrocarbon-like formulas (Mann–Whitney U test p-value ≪ 0.001). Lignin-like and tannin-like formulas dominated SPE (−) samples (Mann–Whitney p-value ≪ 0.001) and both non-SPE (−) and SPE (+) had increased lipid-like efficiency (Mann–Whitney p-value < 0.001). Given that both the lignin- and tannin-like compound classes are characterized by higher O
:
C ratios (>0.28 and >0.65 respectively), we argue that these patterns reflect the enhanced capability the PPL cartridge to retain O-rich compounds.32,39 This suggests that solid phase extraction has some combinatorial effect when used in conjunction with ESI (−) due to the elevated potential for ESI (−) to enrich for O-rich compounds, as we observed above. It is also important to note that the acidification of sample prior to SPE also likely selects for compounds more readily identifiable in SPE (−).
Table 1 Results of one sample (23 A, highlighted in Fig. S1) analyzed using each of the four methods, indicating that the chosen method can result in varying conclusions and thermodynamic interpretations
Method |
Sample NOSC |
Formula count |
C : N |
Lignin : N |
F
T, coupled to O2 reduction |
F
T, coupled to SO42− reduction |
SPE (−) |
−0.28 |
1971 |
32.8 |
48.04 |
0.99 |
0.17 |
SPE (+) |
−0.98 |
492 |
29.9 |
2.6 |
0.96 |
0 |
Non-SPE (−) |
−0.49 |
182 |
15.5 |
1.9 |
0.99 |
0 |
Non-SPE (+) |
0.007 |
345 |
9.55 |
3.47 |
0.99 |
0.63 |
Thermodynamics vary substantially based upon extraction methodology and ionization mode
The NOSC for a given sample was used to evaluate the potential thermodynamic implications of the methodological differences. The NOSC metric can reveal the potential thermodynamic favorability of a carbon substrate (or bulk DOM pool favorability when averaged together), with higher NOSC values theoretically yielding a lower overall
(i.e., more favorable) when coupled to the reduction of an electron acceptor.9 We observed that each method yielded NOSC values significantly different from each other method (p < 0.001) and that compounds detected in the non-SPE (+) treatment had higher NOSC values overall while the average NOSC for detected compounds in the SPE (+) was significantly lower (Fig. 2). Interestingly, the impacts of SPE on NOSC within each ionization mode are flipped; in ESI (+) mode, the SPE samples have significantly lower NOSC values whereas, in ESI (−) mode, the non-SPE samples have significantly lower NOSC values. We hypothesize that this could be due to potentially driven by the higher average proportion of lipid-like molecular formulas in both of these treatments, which have historically had lower NOSC values.10 Thus, non-SPE (+) processing of these samples would have yielded DOM compounds considered less thermodynamically favorable in many biogeochemical analyses.6,10,43
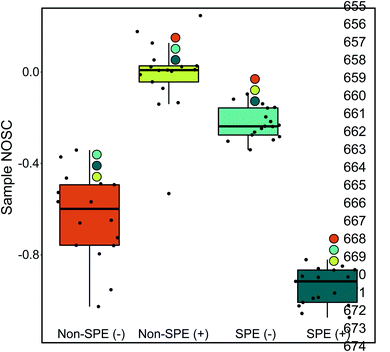 |
| Fig. 2 The distribution of sample mean NOSC values across all four FTICR-MS analysis methods. Jittered points represent the specific NOSC means for a given sample. Colored circles indicate significant differences between methods. | |
To evaluate the transferability of observations derived from our dataset, we performed similar analyses on data previously utilized in Hawkes et al. (2020).25 In brief, these data consisted of four separate organic matter standards (ESFA: Elliot Soil Fulvic Acid, PLFA: Pony Lake Fulvic Acid, SRFA: Suwannee River Fulvic Acid, SRNOM: Suwannee River Natural Organic Matter) collected across international high-resolution mass spectrometry instruments (e.g., FTICR-MS, Orbitrap-MS) in both ESI (+) and ESI (−) modes. The fulvic acid samples were prepared by isolating DOM onto XAD-8 resin and the SRDOM sample was prepared using reverse osmosis. Across each standard alone and a composite of all standards, we observed consistently lower NOSC in data collected in positive mode than in negative mode (Wilcoxon Test & Kolmogorov–Smirnov Test p values ≪ 0.001; Fig. S3†). The results help assert that the impact of ionization mode is similar across sample pre-treatment methods and sample types (e.g., ESFA is a soil standard vs. our freshwater samples).
To further explore the thermodynamic implications of the FTICR-MS methods, we calculated the average thermodynamic potential factor (FT) for the oxidation of the average DOM pools coupled to reduction of O2 and SO42− at standard conditions (Fig. 3). Briefly, FT is a dimensionless value derived from transition state theory that couples the rate of reaction to the Gibbs free energy of reaction. A value of 1 indicates that bioenergetic limitation is ignored, the reaction is more kinetically controlled, and, if there is substrate available, there will likely be a positive reaction rate until substrate is consumed.9,33 Thus, FT values are helpful for identifying differences in potential organic matter degradation rates between different environments or samples. Data resulting from the non-SPE (+) treatment consistently yielded the highest FT values for reduction of both O2 and SO42−, while the FT values calculated from SPE (+) analyses were generally the lowest of the four treatment conditions (Fig. 3). This indicates that the suite of compounds detected by non-SPE (+) would have the fewest thermodynamic constraints if utilized for sulfate reduction and that oxidation of the compounds detected by SPE (+) have the highest thermodynamic constraint when coupled to aerobic respiration. The compounds detected in SPE (−) consistently had high FT values when coupled to aerobic oxidation and had a large range for sulfate reduction (0.02–0.51; Fig. 3). As we observed with the NOSC values, we suggest that this is a result of non-SPE (−) and SPE (+) selecting for larger proportions of comparably thermodynamically unfavorable high H
:
C, low O
:
C formulas (e.g., lipid-like). As this approach is commonly coupled to FTICR-MS data,44 it is important to understand how the pre-treatment and instrument method influences final FT calculations and further biogeochemical interpretations.
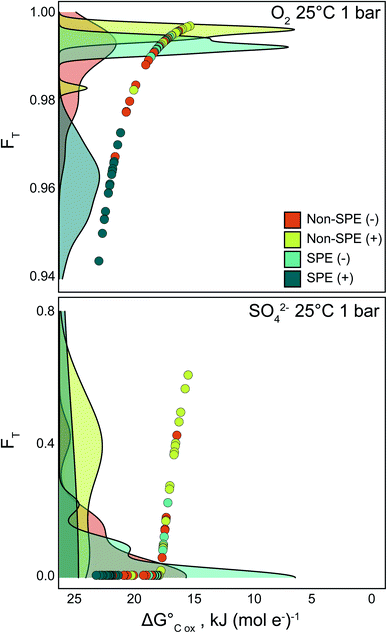 |
| Fig. 3 The Gibbs free energy of the oxidation of the average C pool plotted against the thermodynamic potential factor (FT) for the C oxidation coupled to O2 (top) or SO42− (bottom) reduction at standard conditions, colored by analysis method. Underlying colored density plots represent the distribution of FT values across each method. | |
Divergent ecosystem interpretations arise due to extraction methodology and ionization mode
We observed variability in elemental and lignin composition across ESI ± and can leverage this to further evaluate the organic matter detected by each ionization mode. For example, lignin
:
N and C
:
N ratios have been used as an index of organic matter stability and as a means to estimate decomposition rate.45,46 We calculated these widely used ratios for each of the datasets and found that the methods study here greatly impact these values (Fig. S4†). The non-SPE (+) dataset had a significantly lower C
:
N (here, organic C to organic N) than each other dataset and the SPE (−) dataset had a significantly higher lignin
:
N ratio due to the high detection of lignin-like compounds and no acidification prior to analysis (Fig. 1). This would lead to an interpretation that the DOM pool detected in non-SPE (+) has a higher residence time than those detected in the other methods. The higher lignin
:
N ratio in the SPE (−) dataset cascades into an interpretation where we would assume lower decomposition rates in this dataset relative to the others.
To identify whether the trends seen in the bulk dataset held for sample-resolved analyses, we chose a representative sample for direct comparison of selected measurements between the four methodologies (Table 1). A single representative sample was selected to focus specifically on the thermodynamic relationships across the treatment methodologies in one physical location, rather than broad scale biogeochemical processes explored elsewhere.26 Overall, there were clear differences in the formula count, with SPE (−) still resulting in the most assigned formulas (1971). Additionally, despite variability in NOSC values across the sample treatments, measured DOM pools for this sample have few to no thermodynamic restraints for the reduction of O2 (i.e., FT values close to 1 – Fig. 3a). Conversely, these same treatment-derived differences in NOSC result in greater variability in the FT parameter when oxidation of DOM is coupled to reduction of SO42−. All the DOM pools detected using the SPE (+) and non-SPE (−) treatment yielded an FT value of 0, indicating that sulfate reduction would be thermodynamically inhibited. In contrast, the non-SPE (+) produced the highest FT when coupled to SO42− reduction, indicating fewer thermodynamic constraints. Lastly, similar to the bulk dataset analysis (Fig. S4†), the ratio of lignin-like compounds to N (lignin
:
N) was significantly higher in the SPE (−) dataset due to the large number of lignin-like compounds detected (Fig. 1B) and the C
:
N ratio was still the lowest in the non-SPE (+). Thus, this example further illustrates how DOM treatment can influence our interpretation of likely redox reactions and potential decomposition rates occurring in each sample.
Given the observed patterns of unique and shared molecular formula across methodologies, extraction method and ionization mode have significant implications for biogeochemical modeling. In the case of recent multi-omics analysis, environmental metabolite data collected via high resolution MS can be incorporated into pathway models to understand carbon flux or organismal metabolism.47 Differences in the types of formula detected during data collection could have profound impacts on inferred metabolic pathways. Given the low percentage of shared molecular formula across methods (Table S3†), detected pathways may be divergent resulting in different predicted paths for carbon flux. Using the data presented within this manuscript, for example, we observe variation in the non-specific “Metabolic pathways” and “Biosynthesis of secondary metabolites” categories across the methods with SPE (−) having the highest absolute abundance and non-SPE (+) having the lowest (Fig.S6†). We also observe pathways unique to methods; for example, “Degradation of aromatic compounds” and “Flavonoid biosynthesis” were only present in SPE (−) data while we only saw “Limonene and pinene degradation” in SPE (+) (Fig. S6†). Other types of biogeochemical analyses, such as those relying on substrate-explicit modeling, will also be significantly impacted due to the shift in understood thermodynamic availability of each compound.12 Specifically, the analysis proposed by Song et al. (2020)12 relies heavily on the predicted Gibb's free energy of various carbon reactions to estimate reaction kinetics and stoichiometric coefficients of catabolic and anabolic reactions. Shifts in the average NOSC values will cascade into divergent predictions of biogeochemical rates.
Conclusions
We achieved the highest number of assigned formulas (11
072) and representation from all molecular and van Krevelen-based compound classes when combining the four datasets (Fig. S5†). Although combining methods would most holistically characterize DOM pools, experimental design clearly depends on (1) instrument availability, (2) available sample volume, and (3) funding resources. We therefore provide some of the following recommendations to help researchers prioritize their desired outputs. Our analyses first confirm that SPE treatment yields the largest number of molecular formulas from these riverine DOM samples and that ESI (−) captures the most formulas and is relatively sufficient for capturing broad chemical diversity (Fig. 1). However, if lipid-like compounds (e.g., formulas with low O
:
C and high H
:
C) are the overall target, extracted samples run with ESI (+) would be a better recommendation. If the aim is a thermodynamic analysis of the DOM pools, SPE (+) appears to detect compounds with significantly lower NOSC values (Fig. 2) than the other methods, potentially altering downstream thermodynamic predictions. We therefore recommend that SPE (−) or SPE (−) combined with SPE (+) is used for most applications. Non-SPE yields the lowest number of formulas and has the most limited applications due to salinity and other interferences. While it may be used if the laboratory has either time or funding constraints, we highlight the potential for saline interference in data capture. If samples are not treated with SPE, we recommend the use of ESI (+) instead of ESI (−) because it not only yields more formulas but also results in more formulas from each Van-Krevelen-based compound class, likely resulting in a more holistic characterization of the DOM pool (Fig. 1). However, if the study is focusing on thermodynamics, ESI (−) detects compounds more representative of the DOM pool (Fig. 2). These recommendations should be taken into consideration when performing experimental design. Furthermore, given that we observe significant differences in methodological decisions, we believe that more systematic comparisons across methods (e.g., different ionization techniques, extractions, storages, etc.) are needed to aid scientists in developing well-designed experiments utilizing FTICR-MS.
Abbreviations
SPE | Solid phase extraction |
HRMS | High-resolution mass spectrometry |
NOM | Natural organic matter |
DOM | Dissolved organic matter |
NOSC | Nominal oxidation state of carbon |
ESI | Electrospray ionization |
FTICR-MS | Fourier transform ion cyclotron resonance mass spectrometry |
NPOC | Non-purgeable organic carbon |
Data availability
All of the FTICR-MS data used throughout this study is available at the DOE's data archive ESS-DIVE at https://data.ess-dive.lbl.gov/view/doi:10.15485/1813303.38
Conflicts of interest
There are no conflicts of interest to declare.
Acknowledgements
A portion of this research was performed under the Facilities Integrating Collaborations for User Science (FICUS) program (award # 504279 to MJW) and used resources at the Environmental Molecular Sciences Laboratory (EMSL), which is a DOE Office of Science User Facility. The EMSL facility is sponsored by the Biological and Environmental Research (BER) program and operated under Contract No. DE-AC05-76RL01830. Another portion of this research was performed under DE-SC0016488 as part of the BER's Subsurface Biogeochemical Research SFA funding of MJW. Sampling efforts were funded through a grant from the Geological Society of America to ARN. This research was also supported by the U.S. DOE-BER as part of BER's River Corridor Research Program (RC). This contribution originates from the RC Scientific Focus Area (SFA) at the Pacific Northwest National Laboratory (PNNL). We would like to acknowledge the Worldwide Hydrobiogeochemical Observation Network for Dynamic River Systems (WHONDRS) for their support in data collection and analysis. We would also like to acknowledge William Kew for his assistance and guidance in the preparation in this manuscript.
References
- J. A. Hawkes, T. Dittmar, C. Patriarca, L. Tranvik and J. Bergquist, Evaluation of the Orbitrap Mass Spectrometer for the Molecular Fingerprinting Analysis of Natural Dissolved Organic Matter, Anal. Chem., 2016, 88(15), 7698–7704, DOI:10.1021/acs.analchem.6b01624
.
- R. E. Danczak, R. K. Chu, S. J. Fansler, A. E. Goldman, E. B. Graham and M. M. Tfaily,
et al., Using metacommunity ecology to understand environmental metabolomes, Nat. Commun., 2020, 11(1), 6369 CrossRef CAS PubMed
, http://www.nature.com/articles/s41467-020-19989-y.
- A. M. Kellerman, A. Arellano, D. C. Podgorski, E. E. Martin, J. B. Martin and K. M. Deuerling,
et al., Fundamental drivers of dissolved organic matter composition across an Arctic effective precipitation gradient, Limnol. Oceanogr., 2020, 65(6), 1217–1234 CrossRef CAS
.
- A. M. Kellerman, T. Dittmar, D. N. Kothawala and L. J. Tranvik, Chemodiversity of dissolved organic matter in lakes driven by climate and hydrology, Nat. Commun., 2014, 5(1), 3804 CrossRef CAS PubMed
, http://www.nature.com/articles/ncomms4804.
- M. M. Tfaily, N. J. Hess, A. Koyama and R. D. Evans, Elevated [CO2] changes soil organic matter composition and substrate diversity in an arid ecosystem, Geoderma, 2018, 1–8, DOI:10.1016/j.geoderma.2018.05.025
.
- V. A. Garayburu-Caruso, J. C. Stegen, H.-S. Song, L. Renteria, J. Wells and W. Garcia,
et al., Carbon Limitation Leads to Thermodynamic Regulation of Aerobic Metabolism, Environ. Sci. Technol. Lett., 2020, 7(7), 517–524, DOI:10.1021/acs.estlett.0c00258
.
- E. B. Graham, A. R. Crump, D. W. Kennedy, E. Arntzen, S. Fansler and S. O. Purvine,
et al., Multi ’omics comparison reveals metabolome biochemistry, not microbiome composition or gene expression, corresponds to elevated biogeochemical function in the hyporheic zone, Sci. Total Environ., 2018, 642, 742–753, DOI:10.1016/j.scitotenv.2018.05.256
.
- E. B. Graham, M. M. Tfaily, A. R. Crump, A. E. Goldman, L. M. Bramer and E. Arntzen,
et al., Carbon Inputs From Riparian Vegetation Limit Oxidation of Physically Bound Organic Carbon Via Biochemical and Thermodynamic Processes, J. Geophys. Res.: Biogeosci., 2017, 122(12), 3188–3205 CrossRef CAS
, http://www.nature.com/articles/175238c0.
- D. E. LaRowe and P. Van Cappellen, Degradation of natural organic matter: A thermodynamic analysis, Geochim. Cosmochim. Acta, 2011, 75(8), 2030–2042 CrossRef CAS
, http://www.sciencedirect.com/science/article/pii/S0016703711000378.
- K. Boye, V. Noël, M. M. Tfaily, S. E. Bone, K. H. Williams and J. R. Bargar,
et al., Thermodynamically controlled preservation of organic carbon in floodplains, Nat. Geosci., 2017, 10(6), 415–419 CrossRef CAS
.
- A. Sengupta, S. Fansler, R. Chu, R. Danczak, V. Garayburu-Caruso and L. Renteria,
et al., Disturbance Triggers Non-Linear Microbe-Environment Feedbacks, Biogeosciences, 2021, 1–42 Search PubMed
.
- H.-S. Song, J. C. Stegen, E. B. Graham, J.-Y. Lee, V. A. Garayburu-Caruso and W. C. Nelson,
et al., Representing Organic Matter Thermodynamics in Biogeochemical Reactions via Substrate-Explicit Modeling, Front. Microbiol., 2020, 1–16 Search PubMed
, https://www.frontiersin.org/articles/10.3389/fmicb.2020.531756/full.
- J. C. Stegen, T. Johnson, J. K. Fredrickson, M. J. Wilkins, W. C. Nelson and E. V. Arntzen,
et al., Influences of organic carbon speciation on hyporheic corridor biogeochemistry and microbial ecology James, Nat. Commun., 2018, 1–11, DOI:10.1038/s41467-018-02922-9
.
- N. Hertkorn, M. Harir, B. P. Koch, B. Michalke and P. Schmitt-Kopplin, High-field NMR spectroscopy and FTICR mass spectrometry: Powerful discovery tools for the molecular level characterization of marine dissolved organic
matter, Biogeosciences, 2013, 10(3), 1583–1624 CrossRef CAS
.
- R. King, R. Bonfiglio, C. Fernandez-Metzler, C. Miller-Stein and T. Olah, Mechanistic investigation of ionization suppression in electrospray ionization, J. Am. Soc. Mass Spectrom., 2000, 11(11), 942–950 CrossRef CAS PubMed
.
- T. Dittmar, B. Koch, N. Hertkorn and G. Kattner, A simple and efficient method for the solid-phase extraction of dissolved organic matter (SPE-DOM) from seawater, Limnol. Oceanogr.: Methods, 2008, 6(6), 230–235, DOI:10.4319/lom.2008.6.230
.
- Y. Li, M. Harir, M. Lucio, B. Kanawati, K. Smirnov and R. Flerus,
et al., Proposed Guidelines for Solid Phase Extraction of Suwannee River Dissolved Organic Matter, Anal. Chem., 2016, 88(13), 6680–6688 CrossRef CAS PubMed
.
- M. M. Tfaily, S. Hodgkins, D. C. Podgorski, J. P. Chanton and W. T. Cooper, Comparison of dialysis and solid-phase extraction for isolation and concentration of dissolved organic matter prior to Fourier transform ion cyclotron resonance mass spectrometry, Anal. Bioanal. Chem., 2012 Aug 15, 404(2), 447–457, DOI:10.1007/s00216-012-6120-6
.
- I. V. Perminova, I. V. Dubinenkov, A. S. Kononikhin, A. I. Konstantinov, A. Y. Zherebker and M. A. Andzhushev,
et al., Molecular Mapping of Sorbent Selectivities with Respect to Isolation of Arctic Dissolved Organic Matter as Measured by Fourier Transform Mass Spectrometry, Environ. Sci. Technol., 2014, 48(13), 7461–7468, DOI:10.1021/es5015423
.
- R. L. Sleighter and P. G. Hatcher, Molecular characterization of dissolved organic matter (DOM) along a river to ocean transect of the lower Chesapeake Bay by ultrahigh resolution electrospray ionization Fourier transform ion cyclotron resonance mass spectrometry, Mar. Chem., 2008, 110(3–4), 140–152 CrossRef CAS
, https://linkinghub.elsevier.com/retrieve/pii/S0304420308000704.
- R. L. Sleighter and P. G. Hatcher, The application of electrospray ionization coupled to ultrahigh resolution mass spectrometry for the molecular characterization of natural organic matter, J. Mass Spectrom., 2007, 42(5), 559–574, DOI:10.1002/jms.1221
.
- T. L. Brown and J. A. Rice, Effect of experimental parameters on the ESI FT-ICR mass spectrum of fulvic acid, Anal. Chem., 2000, 72(2), 384–390 CrossRef CAS PubMed
.
- C. E. Rostad and J. A. Leenheer, Factors that affect molecular weight distribution of Suwannee river fulvic acid as determined by electrospray ionization/mass spectrometry, Anal. Chim. Acta, 2004, 523(2), 269–278 CrossRef CAS
.
- T. Ohno, R. L. Sleighter and P. G. Hatcher, Comparative study of organic matter chemical characterization using negative and positive mode electrospray ionization ultrahigh-resolution mass spectrometry, Anal. Bioanal. Chem., 2016, 408(10), 2497–2504 CrossRef CAS PubMed
.
- J. A. Hawkes, J. D'Andrilli, J. N. Agar, M. P. Barrow, S. M. Berg and N. Catalán,
et al., An international laboratory comparison of dissolved organic matter composition by high resolution mass spectrometry: Are we getting the same answer?, Limnol. Oceanogr.: Methods, 2020, 18(6), 235–258, DOI:10.1002/lom3.10364
.
- A. R. Nelson, A. H. Sawyer, R. S. Gabor, C. M. Saup, S. R. Bryant and K. D. Harris,
et al., Heterogeneity in Hyporheic Flow, Pore Water Chemistry, and Microbial Community Composition in an Alpine Streambed, J. Geophys. Res.: Biogeosci., 2019, 2019JG005226, DOI:10.1029/2019JG005226
.
- C. M. Saup, S. R. Bryant, A. R. Nelson, K. D. Harris, A. H. Sawyer and J. N. Christensen,
et al., Hyporheic Zone Microbiome Assembly Is Linked to Dynamic Water Mixing Patterns in Snowmelt-Dominated Headwater Catchments, J. Geophys. Res.: Biogeosci., 2019, 124(11), 3269–3280 CrossRef
.
- D. J. Orton, M. M. Tfaily, R. J. Moore, B. L. LaMarche, X. Zheng and T. L. Fillmore,
et al., A Customizable Flow Injection System for Automated, High Throughput, and Time Sensitive Ion Mobility Spectrometry and Mass Spectrometry Measurements, Anal. Chem., 2018, 90(1), 737–744, DOI:10.1021/acs.analchem.7b02986
.
- N. Tolić, Y. Liu, A. Liyu, Y. Shen, M. M. Tfaily and E. B. Kujawinski,
et al., Formularity: Software for Automated Formula Assignment of Natural and Other Organic Matter from Ultrahigh-Resolution Mass Spectra, Anal. Chem., 2017, 89(23), 12659–12665, DOI:10.1021/acs.analchem.7b03318
.
-
L. M. Bramer and A. White, ftmsRanalysis: Analysis and Visualization Tools for FT-MS Data. R Package Version 1.0.0, 2019 Search PubMed
.
- L. M. Bramer, A. M. White, K. G. Stratton, A. M. Thompson, D. Claborne and K. Hofmockel,
et al., ftmsRanalysis: An R package for exploratory data analysis and interactive visualization of FT-MS data, PLoS Comput. Biol., 2020, 16(3), e1007654 CrossRef CAS PubMed
.
- S. Kim, R. W. Kramer and P. G. Hatcher, Graphical Method for Analysis of Ultrahigh-Resolution Broadband Mass Spectra of Natural Organic Matter, the Van Krevelen Diagram, Anal. Chem., 2003, 75(20), 5336–5344, DOI:10.1021/ac034415p
.
- Q. Jin and C. M. Bethke, Predicting the rate of microbial respiration in geochemical environments, Geochim. Cosmochim. Acta, 2005, 69(5), 1133–1143 CrossRef CAS
.
- M. Kanehisa and S. Goto, KEGG: Kyoto Encyclopedia of Genes and Genomes, Nucleic Acids Res., 2000, 28(1), 27–30, DOI:10.1093/nar/28.1.27
.
-
H. Wickham, rvest: Easily Harvest (Scrape) Web Pages, 2020, https://cran.r-project.org/package=rvest Search PubMed
.
-
R. C. Team, R: A language and environment for statistical computing, R Found Stat Comput, Vienna, Austria, 2021, https://www.r-project.org/ Search PubMed
.
-
H. Wickham, ggplot2: Elegant Graphics for Data Analysis, Springer-Verlag, New York, 2016 Search PubMed
.
-
A. R. Nelson, R. K. Chu, N. Tolic, V. Garayburu-Caruso, C. M. Saup, J. Wells, et al., East River Surface and Pore Water FTICR-MS Data Associated with “Implications of sample treatment on characterization of the riverine environmental metabolome”, Seas Control Dyn hyporheic Zo redox Biogeochem, 2021 Search PubMed
.
- J. Raeke, O. J. Lechtenfeld, M. Wagner, P. Herzsprung and T. Reemtsma, Selectivity of solid phase extraction of freshwater dissolved organic matter and its effect on ultrahigh resolution mass spectra, Environ. Sci.: Processes Impacts, 2016, 18(7), 918–927 RSC
, http://xlink.rsc.org/?DOI=C6EM00200E.
-
E. M. Perdue and J. D. Ritchie, Dissolved Organic Matter in Freshwaters, in: Treatise on Geochemistry, Elsevier, 2003, pp. 273–318, https://linkinghub.elsevier.com/retrieve/pii/B0080437516050805 Search PubMed
.
- A. Steckel and G. Schlosser, An Organic Chemist's Guide to Electrospray Mass Spectrometric Structure Elucidation, Molecules, 2019, 24(3), 611 CrossRef PubMed
, http://www.mdpi.com/1420-3049/24/3/611.
- C. E. Rostad and J. A. Leenheer, Factors that affect molecular weight distribution of Suwannee river fulvic acid as determined by electrospray ionization/mass spectrometry, Anal. Chim. Acta, 2004, 523(2), 269–278 CrossRef CAS
, https://linkinghub.elsevier.com/retrieve/pii/S0003267004008153.
- R. E. Danczak, A. E. Goldman, R. K. Chu, J. G. Toyoda, V. A. Garayburu-Caruso and N. Tolić,
et al. Ecological theory applied to environmental metabolomes reveals compositional divergence despite conserved molecular properties, Sci. Total Environ., 2021, 788, 147409 CrossRef CAS PubMed
, https://linkinghub.elsevier.com/retrieve/pii/S0048969721024803.
- M. Keiluweit, T. Wanzek, M. Kleber, P. Nico and S. Fendorf, Anaerobic microsites have an unaccounted role in soil carbon stabilization, Nat. Commun., 2017, 8(1), 1771 CrossRef PubMed
, http://www.nature.com/articles/s41467-017-01406-6.
- J. M. Melillo, J. D. Aber and J. F. Muratore, Nitrogen and lignin control of hardwood leaf litter decomposition dynamics, Ecology, 1982, 63(3), 621–626 CrossRef CAS
.
- H. Parnas, Model for decomposition of organic material by microorganisms, Soil Biol. Biochem., 1975, 7(2), 161–169 CrossRef CAS
, https://linkinghub.elsevier.com/retrieve/pii/0038071775900140.
- A. K. Kessell, H. C. McCullough, J. M. Auchtung, H. C. Bernstein and H.-S. Song, Predictive interactome modeling for precision microbiome engineering, Curr. Opin. Chem. Eng., 2020, 30, 77–85 CrossRef
, https://linkinghub.elsevier.com/retrieve/pii/S2211339820300496.
Footnote |
† Electronic supplementary information (ESI) available. See DOI: 10.1039/d2em00044j |
|
This journal is © The Royal Society of Chemistry 2022 |
Click here to see how this site uses Cookies. View our privacy policy here.