DOI:
10.1039/D3LC00909B
(Tutorial Review)
Lab Chip, 2024,
24, 1419-1440
AI-enhanced biomedical micro/nanorobots in microfluidics
Received
22nd October 2023
, Accepted 11th December 2023
First published on 4th January 2024
Abstract
Human beings encompass sophisticated microcirculation and microenvironments, incorporating a broad spectrum of microfluidic systems that adopt fundamental roles in orchestrating physiological mechanisms. In vitro recapitulation of human microenvironments based on lab-on-a-chip technology represents a critical paradigm to better understand the intricate mechanisms. Moreover, the advent of micro/nanorobotics provides brand new perspectives and dynamic tools for elucidating the complex process in microfluidics. Currently, artificial intelligence (AI) has endowed micro/nanorobots (MNRs) with unprecedented benefits, such as material synthesis, optimal design, fabrication, and swarm behavior. Using advanced AI algorithms, the motion control, environment perception, and swarm intelligence of MNRs in microfluidics are significantly enhanced. This emerging interdisciplinary research trend holds great potential to propel biomedical research to the forefront and make valuable contributions to human health. Herein, we initially introduce the AI algorithms integral to the development of MNRs. We briefly revisit the components, designs, and fabrication techniques adopted by robots in microfluidics with an emphasis on the application of AI. Then, we review the latest research pertinent to AI-enhanced MNRs, focusing on their motion control, sensing abilities, and intricate collective behavior in microfluidics. Furthermore, we spotlight biomedical domains that are already witnessing or will undergo game-changing evolution based on AI-enhanced MNRs. Finally, we identify the current challenges that hinder the practical use of the pioneering interdisciplinary technology.
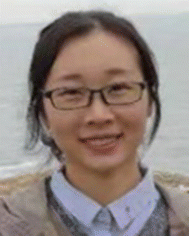 Hui Dong | Dong Hui is currently a Professor at the School of Mechanical Engineering and Automation at the Fuzhou University, and also the ‘Shenzhou Scholar’ Distinguished Professor at the State Key Laboratory of Robotics and Systems, Harbin Institute of Technology, where she received her Ph.D. degree in 2015. From 2012 to 2013, she was a Visiting Scholar at the School of Mechatronics, Johns Hopkins University, Maryland, US. Her principal research interests include deep learning, machine vision and robotics with an emphasis on developing intelligent systems for biomedical research. |
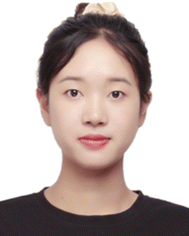 Jiawen Lin | Jiawen Lin received her Bachelor's degree in Engineering from the Huaqiao University, Xiamen, China, in 2022. She is currently pursuing a Master's degree in Prof. Hui Dong's group at the School of Mechanical Engineering and Automation, Fuzhou University, China. Her research focuses on microfluidics and robotics. |
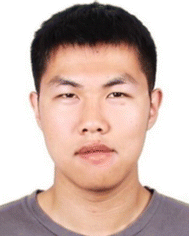 Yihui Tao | Yihui Tao received a B.Eng. degree from the Harbin Institute of Technology in 2015. He received his Ph.D. degree at the University of Sheffield from the Department of Automation Control. His research primarily focuses on data modelling and Fuzzy Logic systems. |
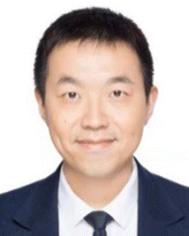 Yuan Jia | Yuan Jia received M.S. and Ph.D. degrees in Mechanical Engineering from Columbia University, NY, USA, in 2012 and 2017, respectively. He currently is an Assistant Professor at Shenzhen Technology University, Shenzhen, China. His research interests include the development of flexible MEMS temperature sensors, MEMS calorimetric sensors for biomedical applications, and point-of-care microfluidics platforms for environmental and biomedical applications. |
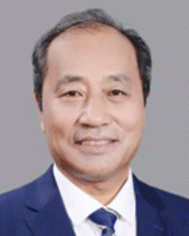 Lining Sun | Lining Sun received M.S. and Ph.D. degrees in Mechanical and Electrical Engineering from the Robotics Institute, Harbin Institute of Technology, Harbin, China, in 1988 and 1993, respectively. He is currently the Director of the Robotics and Microsystems Center and the Dean of the School of Mechanical and Electrical Engineering, Soochow University, Suzhou, China. He is the Deputy Director of the State Key Laboratory of Robot Technology and System, Harbin Institute of Technology. He has over 300 published academic articles and over 40 invention patents. His current research interests include surgical and rehabilitation robots, micro–nano operational robot and equipment, industrial and service robots and advanced robot and control. He is a Foreign Full Member and an Academician of the Russian Academy of Engineering. He gained the China National Funds for Distinguished Young Scientists. He is the Distinguished Professor of Changjiang Scholar. He received the Science and Technology Achievement Award by the Ho Leung Ho Lee Foundation. |
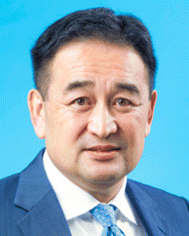 Wen Jung Li | Wen Jung Li is the Vice President (Talent and International Strategy) and Chair Professor (Dept. of Mechanical Engineering) of the City University of Hong Kong (CityU). Prior to joining CityU in 2011, he was affiliated with the Dept. of Mechanical and Automation Engineering of The Chinese University of Hong Kong (CUHK) from 1997 to 2011, where he also served as the Director of the Centre for Micro and Nano Systems from 2002 to 2011. His academic honors include IEEE Fellow, ASME Fellow, AIAA (Asia-Pacific AI Association) Fellow and 100 Talents of the Chinese Academy of Sciences. He served as the President of the IEEE Nanotechnology Council in 2016/2017 and as the founding Editor-in-Chief of the IEEE Open Journal on Nanotechnology (2019–2022) and the IEEE Nanotechnology Magazine (2007–2013). His research areas are tied by diverse engineering disciplines, including electrical engineering, mechanical engineering, biomedical engineering and artificial intelligence. |
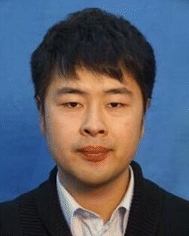 Hao Sun | Hao Sun is a Professor at the School of Mechanical Engineering and Automation at the Fuzhou University, Fujian China. He completed his Ph.D. at Harbin Institute of Technology in 2015. From 2012 to 2014, he was a joint training doctoral scholar at Columbia University, NY, USA. His research focuses on developing integrated microfluidic devices. These devices aim to allow sensitive and accurate characterization of biomolecules in micro-scale environments, thereby enabling new insights into fundamental research as well as innovative capabilities for practical applications. |
1. Introduction
The human body comprises a myriad of sophisticated microcirculation networks that play significant roles in managing physiological mechanisms to maintain systemic balance and optimal health.1 Networks of arterioles, capillaries, venules and interstitial space, which function as innate microfluidic systems, are integral to the microcirculation.2 The transportation of nutrients, hormones and oxygen and the simultaneous removal of metabolic waste through the microfluidic systems are essential to meeting metabolic demands, maintaining body fluid balance, and mediating immune responses.3 Lab-on-a-chip technology, derived from the miniaturization and integration of chemical and biological processes onto chips, enables the precise control and manipulation of fluids that are geometrically constrained to a small scale.4–6 As a subset of microfluidics, organ-on-a-chip (OOC) technology has been developed to recapitulate in vitro human microphysiological systems, including organ level structures, physiological features and mechanical cues.7 This technology offers a sophisticated tool for studying cell-to-cell interactions, signal transmission and essential cell functions.8 Within these microengineered biomimetic systems, cells can be cultured in an dynamic environment that closely mimics their in vivo conditions, enabling researchers to perform the precise and controlled investigations of complex cellular dynamics.9 By linking together multiple OOCs through fluidic interfaces, ‘body-on-chips’ can be built and systemic responses of the human body can be studied.10 Beyond the original progress in OOC development focused on recapitulating healthy organ environments, OOC disease models have been recently created for more in-depth investigations.11,12 These models have served as predictive tools of human responses to pathogens, medicines and chemicals.13
Following Richard Feynman's groundbreaking 1959 discourse “There is Plenty of Room at the Bottom”,14 continuing advancements in the conception and manufacture of active miniaturized components have prompted an array of explorations within the micro, nano, and quantum dimensions.15,16 Nowadays, micro/nanorobots (MNRs) have extended our ability to observe, exploit and control the microscopic world.17,18 MNRs refer to robotic agents with dimensions from the millimeter to nanometer range that are capable of transforming energy to movement and forces. They became reified owing to the microelectromechanical system (MEMS) technology that emerged in the late 1980s.19 Thereafter, various microrobotic devices (such as microgripper,20 microgears,21 micromotors22 and microdrillers23) were successfully developed for multiple applications. With a size similar to or larger than the small organisms (e.g., bacteria and viruses), microrobots can interact with the microscale world in ways that are not possible for macroscopic robots.24 Meanwhile, nanorobots are typically developed with the intent of interacting with the molecular components of living organisms, such as DNA and proteins.25 Microfluidics offers a robust foundation to faithfully reveal the characteristics of MNRs in the human body.26 In microfluidics, interactions of MNRs with small organisms can be precisely monitored and analyzed, and therefore enable refinement of the robots' functionalities.27,28 Manipulation of MNRs (like a microswimmer or vehicle) in microfluidics is essential for practical use. During the past decade, the microscale or nanoscale objects made from soft or solid materials have been positioned, oriented, and controlled by external magnetic,29 acoustic,30 optical31 or electric32 fields. Theranostic payloads for targeted treatment can also be maneuvered, controlled, and delivered by tethered or untethered MNRs.33,34 The microscopic robot MNRs have also been utilized to manipulate, capture, sort and deliver cells.35 Moreover, minimally invasive surgeries have benefited from MNRs aids based on precise motion and advanced imaging.36
Artificial Intelligence (AI) has established groundbreaking benchmarks across many industries and academic realms, and revolutionized virtually all aspects of human life. As an integral part of AI, machine learning equips computers with the ability to learn from data without the requirement of explicit programming.37 Numerous algorithms of machine learning (such as decision tree, random forest, support vector machine (SVM), k-nearest neighbors (k-NN), convolution neural network (CNN), recurrent neural network (RNN), Transformers, graph neural network (GNN), reinforcement learning (RL), and their variants) have made remarkable accomplishments in diverse domains.38–42 These include computer vision, natural language processing, facial recognition, robotic process automation and bioinformatics. For instance, in the field of computer vision, AI algorithms have improved the ability to interpret and understand visual data, which have a profound impact on areas like image analysis.43 AI has also reshaped the micro/nanorobotics study by providing a unique prowess in motion measurement and autonomous control of MNRs.44,45 Firstly, by leveraging AI-based data processing techniques, the robotic systems can increase the accuracy and reliability of motion measurement.46 These algorithms can extract meaningful information to facilitate robust tracking and localization of MNRs. In parallel, by AI-based control strategies, MNRs can precisely navigate and autonomously respond to environmental obstacles with improved adaptability.47 Multiple complex tasks such as sorting, filtering and transporting cells or substances within a microfluidic environment can be accomplished.48 Furthermore, accompanied by the prevalence trend of MNRs in the medical field, it can be foreseen that massive amounts of image or video data,49 time-series data50 or multimodal sensing data51,52 will be generated in the future, either experimentally or clinically. The capability to proficiently investigate vast quantities of data is indeed the primary strength of AI. Therefore, it can be anticipated that AI and micro–nanorobotics will intersect more closely, and the convergence will be projected to reciprocally stimulate progress in their respective fields.
Although initially developed independently, MNRs, microfluidics and AI have become more intertwined in the past few years (Fig. 1). Following Richard Feynman's conceptual trope, there should also be significant advances in intelligence consequently endowed by the interdisciplinary technologies. The cross-disciplinary research integrating AI, MNRs and microfluidics is rapidly growing, which may make substantial contributions to the paradigm shift in the field of biomedical research. In this review, we initially illustrate the useful AI algorithms for developing MNRs. A concise examination of the components, design, and assembly of robots in microfluidics is also presented. Subsequently, we provide an overview of the most recent research relating to AI-enhanced MNRs with an emphasis on their motion control, sensory capabilities, and group dynamics. Moreover, various biomedical sectors which are experiencing change or poised for transformations resulting from the integration of AI and MNRs are discussed. Such sectors include drug delivery, cell maneuvering, organ-on-chip systems, biopsy and precision surgery, and implantation techniques. Finally, we profile the predominant barriers that pose as constraints to the practical application of this groundbreaking interdisciplinary technology.
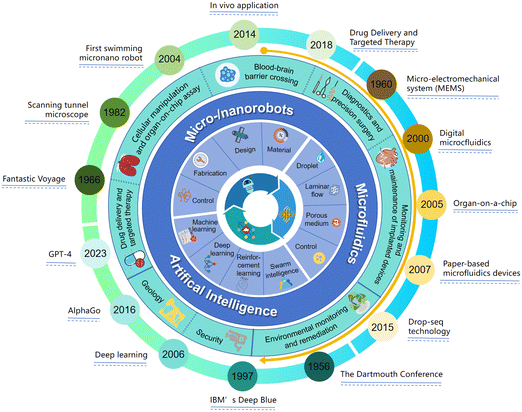 |
| Fig. 1 Artificial intelligence-enhanced robots and their applications in microfluidics. | |
2. AI and micro/nanorobots
2.1 Algorithms
Since the landmark Dartmouth Conference in 1956,53 AI has experienced several phases of refinement. Numerous algorithms have been continuously developed, ranging from elementary rule-based algorithms to advanced machine learning and deep learning algorithms that dominate the landscape today. This section of review selectively presents a collection of classic algorithms that are relevant to microfluidics. A concise introduction is provided for algorithms without delving excessively into technical details.
2.1.1 Traditional machine learning.
Traditional machine learning refers to a class of algorithms that mainly utilize statistical techniques to extract patterns and make predictions from data. These algorithms can be supervised (learning from labeled data), unsupervised (learning from unlabeled data), or semi-supervised (a mix of the two). Naive Bayes, linear regression, logistic regression, SVM, decision trees, random forests, hierarchical clustering, Gaussian mixture models, self-organizing maps, Apriori algorithm and k-NN are among the mainstream traditional algorithms. Traditional machine learning methods have many advantages. They excel in handling large datasets, extracting meaningful features that may lead to accurate predictions for labeled data. The algorithms are usually well-established with a solid theoretical foundation and wide understanding. Their effectiveness in processing structured data also enhances their usability.54 However, traditional methods have some limitations. They often require extensive manual feature engineering, making them time-consuming processes. The algorithms may struggle with generalizing complex and high dimensional data well, and can be sensitive to outliers and noise, which potentially impact the robustness.55 Nevertheless, on-chip study, cell classification,56 biomarker detection,57 optimization of chemical reactions58 and fluid or droplet control42,59 have been successfully improved by machine learning technologies.
2.1.2 Deep learning.
Deep learning (DL), a key subset of machine learning, is a computational approach that emulates the human brain's neural networks to process intricate patterns and extract meaningful representations from datasets. By employing multiple layers of interconnected nodes, DL models enable hierarchical feature extraction and data transformation. DL algorithms can also be supervised, unsupervised, or semi-supervised. Convolution neural networks (CNNs), deep residual networks, sequence-to-sequence models, recurrent neural networks (RNNs), long short-term memory networks (LSTMs), transformer networks (including BERT, GPT, etc.), graph neural networks (GNN), and generative adversarial networks (GANs) are among the most famous DL algorithms. DL offers several notable advantages over traditional methods.60 The deep neural networks are proficient in autonomously handling high dimensional and unstructured data, such as images, text, and audio independent of domain knowledge. The need for manual feature extraction required in conventional machine learning can be avoided. DL also thrives with a larger dataset and higher computational power, which make it especially effective in dealing with massive data. Despite noteworthy advancements, DL is constricted by certain limitations.61 The process of training a DL model typically calls for thousands or potentially millions of datasets. This requirement often presents a significant challenge due to the difficulties intrinsic to gathering such extensive data in every context. DL models also suffer from a lack of interpretability, which causes challenges to understanding how they have arrived at a particular decision through “black boxes”. Recently, interpretable and trustworthy deep learning which aim at producing dependable and comprehensible models has evolved into a topic of significant interest.62,63 By these approaches, the transparency, robustness, fairness and privacy of models can be enhanced. On-chip cell classification,64,65 drug design66 and delivery,67 target recognition,68 and nucleic acid amplification prediction69 have been successfully assisted by DL models (Fig. 2).
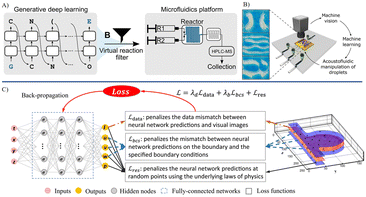 |
| Fig. 2 Exemplary AI algorithms developed for microfluidics. A) A generative deep learning model based on a long-short term memory network for on-chip synthesis for drug design.66 B) machine learning-guided ultrasonic method for versatile two-dimensional droplet manipulation in programmable microfluidic devices.59 C) Schematic illustrating an artificial-intelligence velocimetry model based on PINN. A fully connected neural network is employed to approximate the solutions for the desired output parameters.76 Reproduced with permission from ref. 66. Copyright 2021, Science Advances, ref. 59. Copyright 2022, ACS Publications, ref. 76. Copyright 2021, Proceedings of the National Academy of Sciences. | |
2.1.3 Physics-based deep learning.
Physics-based deep learning (PBDL) is an emerging interdisciplinary field that integrates principles of physics into DL to enhance model interpretability and training efficiency.70 These physical laws, commonly articulated as ordinary differential equations (ODEs) or partial differential equations (PDEs), support DL models with innovated structures. The models transcend the rudimentary dependencies on data-driven correlations, and offer a more sophisticated learning paradigm.71 Current representative methods under this discipline include physics-informed neural networks (PINNs) and deep hidden physics models (DHPMs). PINNs mold the deep learning procedure by supplementing the loss function with ODEs or PDEs (i.e., governing equations, initial and boundary conditions), which orient the functioning of the neural network towards compliance with physical laws.72 From a different perspective, DHPMs learn the time evolution of nonlinear systems to discover unknown equations governing the system dynamics, followed by reasonably forecasting future states.73 It is noteworthy that PBDL methods do not undermine the conventional data-driven learning. Instead, they enrich AI methodologies by layering on the systematic knowledge derived from physical laws. The intersection of physics and deep learning leads to more robust and interpretable paradigms. On-chip concentration gradient generator,74 droplet formation,75 and blood flow analysis76 have been empowered by PBDL models.
2.1.4 Reinforcement learning.
Reinforcement learning (RL) is a facet of AI that employs a method of learning via interaction with the environment. The concept revolves around an agent making decisions based on a set of actions with the aim of maximizing the cumulative reward. RL does not necessitate the presence of prelabeled data as a prerequisite for the initiation of the learning process. Instead, it learns through a system of trial and error through continuous observation of its actions and the results.77 One major advantage of RL is the capacity to robotically improve the performance through repeated learning patterns. However, the learning process by RL may be inevitably time-consuming and computationally expensive, and struggle in situations where the reward system or environment are unpredictable. Specifically, in the field of robotics, the impact of RL is monumental. Algorithms based on the RL framework have enabled robots to learn a plethora of complex tasks independently and adapt to new environments. On-chip experiments, including flow network construction,78 pump control79 and microbead manipulation,80 were elevated by RL algorithms.
2.1.5 Swarm intelligence.
Swarm intelligence (SI) represents an innovative distributed intelligent paradigm for problem-solving systems inspired by the collective behavior of social insects and other animal societies.81 The power of SI arises from the process of self-organization, a set of individuals interacting with one another in a decentralized manner to achieve global behaviors. It significantly embodies the Aristotle's axiom, “the whole is greater than the sum of the parts”. Ant colony optimization (ACO) and particle swarm optimization (PSO) are the main SI algorithms used, as well as bacterial foraging optimization, artificial shepherd algorithm, artificial bee swarm, and fish school search.82 The fusion of SI and ML can potentially overcome their individual limitations and fortify their strengths. For instance, ACO and PSO can be used to optimize the hyperparameters of ML models efficiently, which eliminates the need for trial-and-error in hyperparameter tuning.83 Conversely, RL can enhance the learning and adaptation abilities of SI systems, making them more intelligent and capable of solving a wider range of problems.84 On-chip fault localization and residue removal,85 single-particle micropatterning,86 and droplet sorting87 were refined by ACO and PSO algorithms.
2.2 Material
MNRs are typically made from solid materials (like metals, ceramics, polymers, and composites) or non-solid materials (such as gels, bubbles and droplets) that are suitable for miniaturization. These materials are selected for their mechanical strength, biocompatibility, and responsiveness to external stimuli, such as light, acoustic and magnetic fields or chemicals. Biocompatibility is a critical requirement for materials used in MNRs. The materials should be non-toxic and non-immunogenic, resistant to corrosion, and capable of peacefully coexisting within a biological environment without causing adverse reactions. AI promotes material science by accelerating the discovery and development of new materials. These advanced algorithms also provide unique advantages in the aspect of material and composition of MNRs.88 In order to discover new materials, machine learning algorithms have been harnessed to process massive databases of the existing materials. These algorithms can extract features and predict the properties of potential materials, and thus expedite the materials discovery process.89 To optimize material synthesis, AI collaborates the parameters and conditions with specific qualities. Deep learning models predicted the resultant material properties based on different protocols, which may improve the manufacturing efficiency. Furthermore, AI can forecast the relationships between the MNR's structures and their resulting biological or physical functions.90 Interesting, AI tools, particularly inverse design approaches, are being utilized to engineer materials with bespoke functions.91 By providing the desired functionality, AI algorithms backtrack to determine the optimal composition. The unique contributions of AI in MNRs-related material research are expected to offer invaluable opportunities for future advancements.
2.3 Design
For AI-enhanced material selection, the configuration design of MNRs can be rendered flexible, but still nontrivial. First, the miniaturization of robots should incorporate scalability, which enable them to retain functionality at reduced scales. The actuation mechanisms based on light, acoustic and magnetic fields or chemicals methods should also be harmonized with design strategy. Moreover, it is imperative to consider the mechanical properties and dynamic response characteristics in the configuration design phase. Evaluating these factors is crucial to the successful execution of the micro/nano robotic systems. The contribution of AI to the optimized design process in macro mechanical design has a similar applicability in the domain of MNRs. Machine learning enables the exploration of numerous design possibilities and predicts performance outcomes.92 For the driving or energy transducing units, AI can analyze variables such as electrostatic, electromagnetic, and electrothermal forces followed by evaluating the driving performance and regulating energy consumption.93 For actuators or end-effect manipulators, AI is able to facilitate the design with emphasis on high precision and responsiveness.94 On the other hand, considering the mature application of numerical simulation such as multi-physics coupled simulation in design optimization, the integration of multi-physics simulation with PBDL can provide additional insights for optimizing the design of MNRs.95
Biomimetics offers a wealth of inspirational concepts for improving micro and nanorobot design. Learning from natural structures and physiological systems, bionic MNRs can imitate cells and microorganisms. Biological motors like kinesins,96 sperm,97 and bacteria98 presented potential for ecologically benign self-propulsion biomedical applications. The synergistic interaction between natural cells such as red blood cells,99 macrophages,100 and intelligent MNRs enhanced biocompatibility and autonomous movement. AI-enhanced bionic carriers, termed “smart nanocarriers” like pollen101 and microalgae,102 also offered invisibility to the immune system, enhancing targeting precision and minimizing toxicity.
2.4 Fabrication
The main fabrication methods for MNRs include photolithography, chemical vapor deposition electron beam lithography, and additive manufacturing, which utilize the optical transfer of patterns, chemical reactions, focused electron beams and printing technique, respectively.103 They offer customized structures with high precision at a micro/nanoscale. Encapsulation technologies for MNRs primarily involve self-assembly, DNA origami, and sol–gel chemistry. Moreover, coating methods such as layer-by-layer assembly can be employed to encapsulate the robots. Each of these technologies contributes different attributes towards the mechanical strength and biochemical stability. The adoption of environmentally sustainable materials and methods to minimize hazardous waste is highly favored in the manufacturing process. For medical utilization, it is crucial that the manufactured devices are nontoxic, biocompatible, and ultimately recyclable or biodegradable. The contributions of AI to the manufacturing of MNRs are versatile. In the stages prior to and during manufacturing, machine learning can be used to perform predictive analysis and optimization (Fig. 3).104 By studying the key manufacturing parameters and possible outcomes, machine learning was employed to predict the potential flaws or errors. In addition, the intermediate products of micro/nano manufacturing can be identified by AI.105 In the stage of post-manufacturing, by collaborating with microscopic morphological characterization or material composition analysis, the fabricated robot products can be assessed quickly through automated morphological analysis based on AI.106
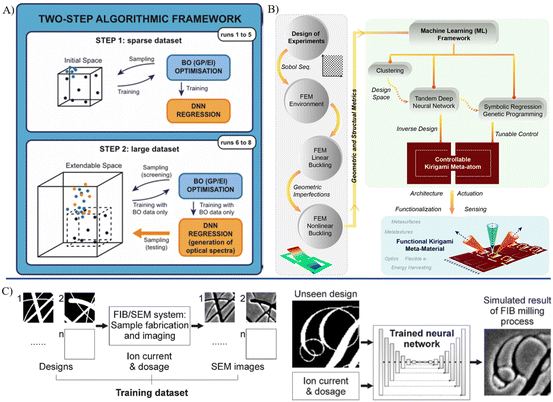 |
| Fig. 3 AI-enhanced material synthesis, device design and fabrication. A) Algorithmic framework for a high-throughput experimental loop to assist the synthesis of metal nanoparticles.91 B) A comprehensive structure for the machine learning-aided design of Kirigami meta-atoms and metamaterials.94 C) A neural network trained on binary design patterns and SEM images from focused ion beam milling, enabling the accurate prediction of postfabrication appearances for unseen designs.104 Reproduced with permission from ref. 91. Copyright 2022, Nature Portfolio, ref. 94. Copyright 2022, Nature Portfolio, ref. 104. Copyright 2022, ACS Publications. | |
3. Intelligent robotics in microfluidics
3.1 Propulsion
The simple transition of traditional power sources by instrumental miniaturization is often not suitable for MNRs. Activating these tiny robots in a biological microfluidic environment such as blood vessels and interstitial space within tissues can be difficult. As device dimensions decrease to a few micrometers/nanometers, the ratio of inertial to viscous forces becomes significantly small. Thus, inertial forces become virtually negligible for MNRs movement. In these highly viscous, low Reynolds number environments, continuous power provision for propelling these devices becomes important.107 Prior to clinical applications, it is imperative to conduct a certain amount of tests on MNRs in microfluidic environments. In microfluidics, the motion of these microscopic robots can be either self-propelled or remotely stimulated.108–110 Self-propulsion uses chemical reactions on the MNRs surface to convert chemical energy into kinetic energy. Such strategies can be divided into two categories: chemical propulsion, which typically requires a range of chemical fuels; and propulsive forces based on microorganisms, such as enzymes or motile cells.111 For instance, the tiny mechanized entities has employed temperature fluctuations for efficient propelling.112
Computational capabilities and machine learning algorithms have enabled an achievement of desirable energy for activating MNRs with optimal velocities.113 Rohr et al. presented a probabilistic learning strategy rooted in the concepts of Gaussian processes and Bayesian optimization for activating soft microrobots through light. This data-driven methodology led to a propelling procedure with optimized gait under a restrictive trials budget. The method illustrated a significant amplification of locomotive performance of microrobots by 115%.114 For gait optimization, recent studies using RL and genetic algorithms for swimming strategy optimization in different microswimmers have been explored.115–117 Hartl et al. employed the NEAT (NeuroEvolution of Augmenting Topologies) genetic algorithm and artificial neural networks to control the motion of microswimmers. This method obtained inspiration from biologically relevant chemotactic sensing strategies.118 Huang et al. developed a closed-loop method for propelling swimming microrobots along arbitrary three-dimensional (3-D) trajectories at low Reynolds numbers using visual servoing. The trajectory was segmented into a sequence of linear parts within this approach. The microrobots were activated to follow intricate 3-D paths without requirement for inputting parametric equations.119 Janiak et al. delineated a discernment and depiction of the microbubbles' behavior within a limited aperture between the glass confines in an acoustic field. With discontinuous activation, transformation occurred from spherical to ellipsoidal forms, facilitating entrapment within the interstice. Meanwhile, persistent activation propelled alterations in the ellipsoidal microbubbles' shape and volume modes.120 The ongoing research and evolution in AI-enhanced propulsion methodologies will be instrumental in supporting and revolutionizing the capabilities of MNR technologies.
3.2 Sensing
By virtue of their reduced size, these robots have the capability to infiltrate and effectively operate in confined spaces. This characteristic is particularly advantageous for applications wherein the mobile entity is required to actively engage with microenvironments inaccessible to human operators. Microfluidic technology is a vital platform for assessing the sensory capabilities of MNRs. It is also a key tool for analyzing the robot-environment interactions and optimizing the choice of biomarkers. Meanwhile, AI can be utilized for tasks of data mining, feature extraction, specimen classification, and intelligent forecasting tasks.121,122 Generally, these tasks represent a unidirectional flow from the physical domain to the information domain. In the context of micro-electromechanical engineering, MNRs, which possess the capacity for energy transformation and move according to predetermined human inputs or machine intelligence, can perceive the environment for themselves. The sensing feedback from their environment subsequently provides dynamic information essential for targeted positioning, navigation, and control of these bots. Within this framework, the data will be bidirectionally communicated between the physical and information domains. With the grown data scale, AI is delegated with a broader range of responsibilities.123
Dai et al. developed microswimmers capable of sensing the external light source illumination direction and adjusting its orientation accordingly. The robot comprised a Janus nanotree with a nanostructured photocathode and photoanode at opposite ends. Chemical alterations allowed control over the photoanode's zeta potential, which facilitated the programmable positive or negative phototaxis.124 Li et al. presented a cutting-edge AI microrobot capable of responding to environmental changes without an onboard energy supply and transmitted real-time signals wirelessly. Controlled by an external magnetic field, the microbot can move accurately, which could enable minimally invasive in situ monitoring, especially in potential disease areas. The study highlighted the feasibility of self-sensing AI microbots for in situ diagnostic or even therapeutic development.125 Li et al. proposed swarming photonic nanorobots capable of mapping diverse physicochemical conditions on-the-fly and guiding localized photothermal therapy. These responsive photonic nanorobots were composed of a photonic nanochain of periodically assembled encapsulated Fe3O4 nanoparticles. The color-responsive mapping of physiological anomalies enables the targeted irradiation for localized photothermal treatment.126 AI-enhanced micro/nanorobotic perception technology has achieved significant advancements, such as more precise environment mapping and improved target localization. Existing challenges in this field include constraints in computational capacity and the requirement for enhanced algorithms to adapt complex environments affected by factors, such as pH, temperature, and glucose levels.
3.3 Navigation
For guiding a robot from the current location to a designated destination, it often requires the intricate interplay of two fundamental factors: localization and path planning. Accurate localization is performed either relative to an environmental landmark or based on various sensor data. Path planning should be realistically feasible, meaning it should respect the robot's kinematic and dynamic constraints and ensure safety by avoiding obstacles. Navigation of MNRs represents a distinctive subclass characterized by unique demands arising from scale-specific properties.127 One of the main challenges at this scale is the influence of Brownian motion, which tends to produce random and unpredictable movements of MNRs. The stochastic movement can lead to imprecise navigation and cause significant deviations from planned routes. Another issue encountered by MNRs is the highly nonlinear and non-Newtonian characteristics of the microfluidic flow, as well as sensitivity to friction and viscosity.128,129 Friction and viscosity forces dramatically increase as the size of the objects decreases, which may impede the movements of MNRs and cause them to slow down or even stop. Unanticipated changes in the environment's friction and viscosity can lead to unplanned movements and decreased navigational accuracy of MNRs. Microfluidics can serve as a major observational instrument for assessing the navigational accuracy and response speed of MNRs, and acts as a principal platform for dynamically optimizing real-time trajectories.
AI has significantly promoted the development of MNRs navigation.130 Advanced algorithms have provided contributions in localization, path optimization and adaptive responses to environmental changes. Cai et al. proposed an innovative control strategy that relies on deep reinforcement learning (DRL) to maneuver a soft magnetic microrobot (SMMR). A neural network-based simulator was constructed to establish the association between the induced magnetic field and the movement states of the robot. With scant prior knowledge relating to the surrounding environment, a DRL algorithm grounded on a gated recurrent unit (GRU) was formulated. The analysis indicated the promising capabilities of DRL in navigating soft magnetic robots in microfluidic environments.131 Botros et al. introduced a microrobot ultrasound dataset composed of 40k frames. The dataset was examined by four deep learning detectors (named as Faster R-CNN, Yolo, Mask-R-CNN, TPH-Yolo) and four corresponding trackers (named as STARK, DiMP, Tomp, Pr-Dimp). By this means, the enhanced navigation of a greater quantity of microrobots can be potentially obtained.132 Liu et al. investigated the management of small-scale, electromagnetically actuated swimmers. To establish a navigation system for these magnetically propelled microswimmers, an informed optimal random exploring tree (informed RRT*) global planning algorithm was used. A closed-loop control algorithm was also proposed to track various reference paths using visual feedback. Specifically, a single hidden layer feedforward neural network approximated the mapping between the magnetic self-rotation direction and actual movement.133 In dealing with complex and unknown environments, AI plays a crucial role for microswimmer navigation, especially through RL methods.134–137 AI will potentially foster the development of MNRs navigation by facilitating more autonomous capabilities and proactive adaptability.
3.4 Control
Pose and motion control underpin the functionality of robotics by enabling precise operation regulation, accurate execution, and interaction with environments. When it comes to MNRs in microfluidics, the robotic control encounters challenges due to the reduced scale and extreme operating conditions. Miniature robots are often utilized in environments characterized by high dynamism, nonlinearity, and prevalent uncertainty.138 High dynamism implies swift and recurring alterations in the environment, to which robots should adapt quickly. This necessitates real-time identification, interpretation, and processing of environmental transformations. Nonlinearity indicates complex spatial configurations through which robots must navigate in real-time, requiring intricate movement controls. Moreover, the inherent uncertainty refers to unpredictable elements and situations that can disrupt robot operations, and demands advanced predictive and reactive mechanisms. In parallel, the multimodal fusion technologies which processes data based on the integration of information from multiple sensor types have proved the capacity for generating holistic interpretation. The fusion method helps surpass individual sensor limitations with improved robustness and reliability, and can act as a vital facilitator in the motion control of MNRs.139
Leveraging AI technology, the control strategies for MNRs will become enriched and augmented. To date, there has been a big scope for the utilization of AI for MNRs control. For controlling the pose of MNRs, Zhang et al. Illustrated a sim-to-real method to estimate the 3D pose of micro/nano objects. Based on a GAN model, limited experimental data were enhanced through simulated data generation. The method enabled advancement of closed-loop control in micro/nanorobotic systems to handle complex shaped micro/nano-objects.140 Khiyati et al. presented optimal control strategies for thin deformable microswimmers in viscous fluids. The approach addressed complex scenarios, in which the effects of non-homogeneous flow, limited configuration information, robot motion, and decision-making were intertwined. Using multiple independent Q-learning simulations, a set of viable policies was generated, which allowed profound efficiency and robustness for robotic control.141 Chen et al. proposed a magnetic untethered peanut-like millirobot (MUPM) with rolling and crablike motion modes. The tiny robot was manipulated under a rotating magnetic field created by a magnetic driving navigation system. An algorithm named as tracking-learning-detection was adopted for facilitating robotic control. The MUPM demonstrated maneuverability in both modes, and had potential applications in intraluminal drug delivery.142 Xu et al. developed a control policy using the broad learning system (BLS), and applied it to a microrobotic system. Advantages of the BLS included its simple structure, and no need for retraining when new demonstration data were provided. The Lyapunov theory was combined with a complex learning algorithm to establish constraints for the controller parameters. The effectiveness of the strategy was confirmed through simulation and tests by a microswimmer trajectory tracking system.143 Neghab et al. demonstrated a stochastic model predictive controller (MPC) based on a neural network for regulating the motion of the microrobots. The system identification was firstly performed to obtain the model's state spaces for constructing the MPC. A nonlinear neural network was utilized to predict the dynamic model of the physical systems. The controller successfully tracked the reference trajectory without error and reduced noise.144 As indicated by Reynolds et al., the creation of microscopic robots capable of processing internal information rather than external controls would establish the groundwork for the emergence of “next-generation intelligent micromachines”, which may become a burgeoning field.145
3.5 Collective behavior
MNRs are more pragmatically meaningful when they operate in swarm mode. From the viewpoint of flow-field control, microfluidic technology provides a controllable variable for manipulating swarmed MNRs. Swarm intelligence, as a bio-inspired computational paradigm, provides a robust framework for collective behaviors. The collective behavior provides scalability, reconfigurability, self-organization, and optimizes resource utilization.146 Firstly, the expansion or reduction of the swarm scale can be modulated by recruiting more members or releasing surplus members. This flexibility allows a swarm to operate efficiently under fluctuating conditions and requirements, and thereby enhances resilience. Reconfigurability entails the ability to rearrange components to suit new conditions. Hence, it permits the potential to evolve dynamically as per environmental stimuli. Self-organization endows MNRs with adaptability in response to environmental changes or internal feedback, without explicit external control. This extends to an efficient utilization of system resources, driving towards an optimal state of operation. Moreover, the intelligent collective behavior ensures resource utilization to maximize the output from the available resources. The resource allocation with minimized redundancy upholds the systematic efficiency and sustainability.147
AI-enhanced swarm intelligence promotes continuous improvement in coordinating and collaborating MNRs. By leveraging AI, adaptive decision-making ability based on environment dynamics requirements can be obtained. The continuous learning capabilities of AI permit enhancement of efficacy, precision, and autonomy.148 Wang et al. presented a novel approach for real-time navigation of a nanoparticle microswarm in active endovascular delivery by employing ultrasound Doppler imaging guidance. A magnetic microswarm was generated and guided near the vessel boundaries. The strategy demonstrated a promising link between swarm control and real-time imaging of microrobotic swarms.149 Shahrokhi et al. conducted a study to find the important factors in manipulating particles based on a collection of online games, in which players steer swarms of up to 500 particles. By analyzing data from over 10
000 players, control techniques that relied solely on the mean and variance of the swarm were investigated. Practicability of the controllers was verified by manipulating 100 kilobots controlled by a global light source direction.150 Ceraso et al. described a swarm intelligence-based control mechanism for medical nanorobots, which function as artificial platelets to locate wounds in the human body. A coloured perceptive particle swarm algorithm was adopted to regulate the movement of nanorobots through self-assembly. The study basically verified that swarm intelligence algorithms can benefit from Compute Unified Device Architecture (CUDA)-based implementations due to their inherent parallel structure.151 Recently, more studies have emerged on the adaptive behaviors of microrobots via RL.152,153 However, given the intricacy of microrobot swarms coupled with their potential for reconfiguration, the progress in learning-based intelligent navigation has yet to reach a mature stage.
3.6 Bio-hybrid behavior
The conceptual exploration of biomimetic engineering based on gaining insights from nature has formed a crucial junction of contemporary research pursuits. The forthcoming frontier in robotics is anticipated to be spearheaded by biohybrids.154 Bio-hybridization of MNRs relies on combining biological (e.g., DNA-, enzyme- or cytomembrane-based) with artificial elements, which are often achieved in microfluidics. In contrast to the counterparts without living organisms, the bio-hybrid MNRs offer notable advantages, such as higher functional versatility, naturally enhanced adaptability, and responsiveness and compatibility.155 Firstly, the biological components offer specificity and sensitivity, while the artificial module brings resilience. Next, biological constituents in bio-hybrid MNRs possess inherent capability of biological organisms to acclimate autonomously to altering environments or stimuli. Lastly, the presence of biological elements suggests a higher degree of biocompatibility, which is vital in scenarios like medical interventions. Reduced chances of immunological rejection and increased prospects for integration with biological tissues can reduce operating complexity. Within microfluidic systems, the characteristics and behaviors of these bio-hybrid MNRs can be more proficiently observed, controlled, and optimized.
AI greatly assists in advancing hybrid MNRs by providing capabilities, ranging from propulsion to collective behaviors, as mentioned in the above sections. Wang et al. developed a data-processing pipeline based on deep neural networks to facilitate the rapid characterization of dynamic DNA devices. Tasks of particle detection and pose estimation were achieved by the YOLOv5 and Resnet50 network architectures. The results by the neural network exhibited fine performance and consistency with experimental distributions. The generalization of the pipeline was verified by diverse nanodevices (Fig. 4).156 Mirzaiebadizi et al. designed an intelligent nanorobot dependent on a DNA framework using molecular programming and logic gate operation. To examine the applicability, DNA nanorobots were applied as capping agents on silica nanoparticles pores.157 Chen et al. presented a biohybrid microrobot with integrated thermal, magnetic and hypoxia sensitivities, and a dual-reporter fluorescent protein for targeted cancer therapy. Probiotic E. coli Nissle1917 were loaded with nanoparticles and NDH-2 enzymes. The microrobot effectively targets the tumor area by combining magnetothermal ablation and NDH-2-induced ROS damage, demonstrating its potential utility in AI-based perception and targeted therapy.158 Although bio-hybrid MNRs have not yet been used in clinical trials, the novel convergence of biology and engineering may offer unprecedented opportunities for advancements in biomedical applications.
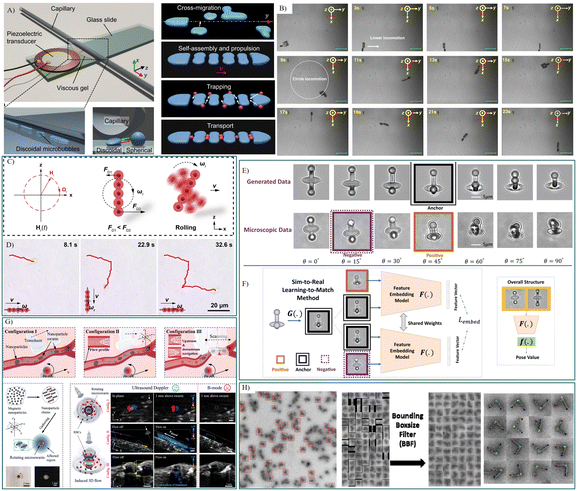 |
| Fig. 4 Intelligent biomedical robots tested in microfluidics for drug delivery and cell manipulation. A) Schematic of the acoustically propelled, train-like microbubble assembly.104 B) Time-resolved optical images of self-sensing intelligent microrobots for noninvasive and wireless monitoring.125 C) Schematic illustration of a pH-responsive photonic nanorobots moving in a ‘rolling’ mode, and D) time-lapse microscopic images depicting ‘rolling’ nanorobots moving in a predesigned trajectory (red curves) when navigated by a magnetic field.126 E) Illustrative instances of the data procured through CycleGAN-based sim-to-real transfer, and F) conceptual exposition of the learning-to-match approach and the comprehensive architecture of the pose estimation model.140 G) Schematic elucidation of the swarm origination and navigation within vascular channels driven by ultrasound Doppler imaging. Doppler signals in proximity to the microswarm were observed within the blood environment, allowing for real-time tracking and navigation of the microswarm via Doppler feedback.149 H) Dynamic DNA origami devices characterized by deep neural networks.156 Reproduced with permission from ref. 104. Copyright 2023, Nature Portfolio, ref. 125. Copyright 2023, Nature Portfolio, ref. 126. Copyright 2023, Springer Link, ref. 140. Copyright 2022, Nature Portfolio, ref. 149. Copyright 2021, Science Advances, ref. 156. Copyright 2023, Biorxiv. | |
4. Game-changing applications
MNRs offer unique and unparalleled applications in the biomedical field. Particularly, by integrating AI algorithms, these intelligent agents demonstrate the proficiency to process information, signal, sense, actuate, communicate, and execute biological tasks at cellular levels. Such capabilities lead to an enhancement in the efficacy of traditional therapeutics, while also abating the associated side effects. We selected various applications, including drug delivery, cell manipulation, organ-on-chip deployment, precision surgery, and implanted device maintenance, to illustrate the revolutionary impact of integrating AI, MNRs, and microfluidics within these fields.
4.1 Drug delivery and targeted therapy
Traditional drug delivery methods rely on systemic administration of medicines, which are distributed throughout the body via the bloodstream. It is difficult to control the timing, dosage, and release rate of drugs, which may lead to fluctuations in drug concentration and compromise the efficacy of the treatment.159 MNRs are revolutionizing the way that medications are administered by delivering drugs directly to the targeted cells or tissues in the body with reduced toxic side effects.160 Yu et al. presented magnetic photonic crystal microrobots that can visually detect pH changes and enable self-regulated drug delivery. The development of this technology supported the progression of intelligent MNRs for active, targeted tumor diagnosis and treatment.161 In light of the rising prevalence of neurological disorders and the need for innovative drug delivery, there has been a notable emphasis on expediting brain research in recent years.162 When diseases occur in the brain, the blood–brain barrier (BBB) may block drugs and hinder their therapeutic effects.163 The traditional experimental validation of theranostic agents that successfully cross the BBB to investigate their permeability and targeted drug development in the central nervous system is a time-consuming process. It often spans over a decade with a relatively low success rate upon completion. Nanorobots offer significant advantages in the field of drug delivery to the brain. Their miniature size and precise control enable them to navigate through the complex pathways of the central nervous system. Nanorobots can also be programmed to specifically target diseased cells or regions, thereby increasing the therapeutic efficiency and reducing off-target effects. Furthermore, nanorobots can overcome the BBB, allowing for the transportation of therapeutic agents to targeted areas. Several studies have identified numerous benefits associated with the utilization of nanoscale materials in brain research.164 To evaluate various nanobots, the development of prescreening tools is of utmost importance. AI algorithms can analyze large volumes of data and extract patterns to accurately predict the efficacy and safety of nanorobots in crossing the BBB. This enables the identification of optimal nanorobot designs and drug formulations for enhanced brain-targeted drug delivery. Machine learning can also adapt and learn from ongoing experimental data, and improve the predictive capabilities. Alsenan et al. presented a deep learning approach utilizing the RNN model for predicting the BBB permeability.165 Singh et al. employed the correlation function as a machine learning algorithm to accurately predict cellular and nuclear shapes, as well as polarity functions. Studies have been performed on the distinctive interactions among nanoparticles (which may vary in comparison to their individual interactions) that govern their diffusion, aggregation, and transportation from the cell culture medium to the cell surfaces.166
AI algorithms are able to further endow MNRs with higher automation, adaptability, and modulated immune response that deliver precise doses in the target area, rather than relying on the release of ordinary drug doses. Current challenges that limit MNRs from laboratory settings to clinical applications primarily involve entry barriers, such as susceptibility to immune system attacks. For example, the deficiency of highly effective non-viral systems with limited cellular toxicity continues to pose a significant hurdle. The employment of AI holds immense potential to unearth novel paradigms to resolve this issue.167 Remarkable evidence suggested that AI may hold significant promise in projecting potential immune responses towards not only viral vectors, but also non-viral gene delivery vehicles. This can be achieved through the establishment of a surrogate assay for genotoxicity evaluation that is proficient in predicting the genotoxicity associated with viral and retroviral vectors employed for stem cell gene therapy. Such predictive capabilities are largely attributed to machine learning methodologies.168 In parallel, when operating the MNRs for drug delivery, one main issue is the aggregation properties of micro or nanovehicles. The surface charge strongly influences the agglomeration phenomena of nanoparticles, which in turn, increases their overall size and triggers a heightened immune response.
This challenge may potentially be mitigated by leveraging AI-based workflows, such as linear regression models, capable of accurately predicting the zeta potential and surface charge of nanoparticles. Additional methodologies encompass deep learning techniques incorporating scanning electron microscopy and a CNN-supported interactive learning and segmentation tool. These methods are proven to facilitate excellent predictive and evaluative capacity in terms of MNRs interactions and their distributions.169 Moreover, AI has been employed for forecasting and enhancing the functionality of nanorobots embedded with biosensors and transducers. Such an approach could have momentous implications in cancer therapy, and in mitigating adverse drug reactions. Furthermore, some illustrations entailed the utilization of a CNN algorithm combined with Surface Enhanced Raman Spectroscopy (SERS). The combination could be leveraged for the unequivocal analysis of extensive spectral data sets, and quantification of analyte concentrations at the single molecule level. The methods facilitated metabolomic profiling and fundamental investigations into serum and blood components, which interfere with MNRs and impede them from reaching their target cells.170,171 To meet the needs of precision medicine and individualized healthcare, AI can be used to analyze patient-specific data to optimize the performance of MNRs. An individual patient's drug dosages based on features such as body weight, age and medical history can be analyzed by AI, which help to reduce the risk of overdosing or underdoing and improving treatment outcomes.172 Although AI-based MNRs drug delivery and targeted therapy lead to adaptability and precision, it may often require more computational resources. Additional research is still required to optimize and standardize AI-based MNRs in drug delivery and targeted therapy.
4.2 Cell manipulation
Traditional cell manipulation methods consist of micropipette aspiration, optical tweezers, and magnetic tweezers, as well as dielectrophoresis, acoustophoresis, and optoelectronic methods. These techniques are frequently constrained by additional factors that could hinder cell viability and proliferation abilities, such as excessive volume and high power requirements. In contrast, MNRs offer exceptional control and adaptability, which enable the precise manipulation of cells with fewer concerns about damaging cell structures.173 Cell health and integrity due to minimal invasiveness could be ensured.174 Also, these tiny agents can often be scaled for simultaneous manipulation of multiple cells, and thus increase the efficiency of processes. However, conventional micro/nanorobots usually require direct control from an operator for specific tasks. This manual control can be insufficient for tasks requiring high repeatability and adaptability to dynamic cellular environments due to limitations in human response and decision-making speed. Integrated with AI, MNRs can utilize advanced algorithms to learn from and adapt to their environment autonomously with higher speed. Therefore, the intelligent MNRs have the potential to revolutionize cell manipulation, leading to breakthroughs in fields, such as genetic or tissue engineering and regenerative medicine.
Grammatikopoulou et al. presented a supervised CNN-LSTM method to estimate the transparent microrobots' depth. The model used monocular grayscale images as input, and achieved submicron accuracy in depth estimation. Validation data confirmed the efficacy of the pretrained regression model under various illumination conditions and orientations for a specific geometrical model. This specific design can be applied for actuation purposes in a microfluidic chip or for the manipulation of cells.175 Most recently, Schrage et al. applied a RL control strategy to navigate ultrasound activated microrobots, enabling efficient transport of a single swarm of microrobots to a user-defined target point. The utilization of a RL strategy can be extended to the manipulation of living cells utilizing a stationary acoustic wavefield. Specifically, when multiple piezo elements are simultaneously activated, a standing acoustic wavefield is generated. As a consequence of the positive contrast factor exhibited by cells in a surrounding microfluidic medium, they can be effectively trapped at the pressure nodes within the wavefield (Fig. 5).176 As reported by Wang et al., the gap between integrated systems and in vivo applications still needs more technical and scientific innovations.177
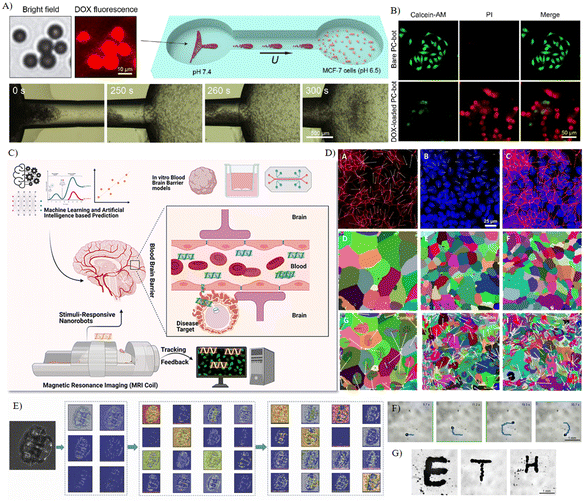 |
| Fig. 5 Drug delivery and cell manipulation by AI-enhanced robots in microfluidics. A) Self-regulated drug delivery by swarming photonic-crystal microrobots, and B) fluorescence images of MCF-7 cells after being incubated with the microrobots and DOX-loaded microrobots for 24 hours.161 C) The possibility to predict and design the next generation of nanorobots that can interact and deliver substances across the blood–brain barrier by combining artificial intelligence with robotics.163 D) Machine learning used to profile and quantify the effects of nanomaterials on cell shape.166 E) Example-learned feature maps obtained from the first, second, and third pair of convolutional and max-pooling layers of the trained network for a input image.175 F) Real-time images demonstrating the manipulation of the swarm, and G) the utilization of both global and local dynamics in swarm manipulation to spell “ETH”.176 Reproduced with permission from ref. 161. Copyright 2023, Wiley, ref. 163. Copyright 2021, ACS Publications, ref. 166. Copyright 2020, ACS Publications, ref. 175. Copyright 2018, IEEE, ref. 176. Copyright 2023, Wiley. | |
4.3 Organ-on-chip deployment
OOC typically consists of microfluidic channels lined with living cells, which closely resemble the physiological environment of the targeted organ.178 This technology offers several advantages over traditional in vitro models and animal testing. The simulated in vivo-like conditions of OOC, together with the precise manipulation provided by MNRs, make it possible to perform experiments that closely mimic in vivo scenarios, providing more reliable and physiologically relevant results.179 Singh et al. presented a bacteria-propelled microswimmer utilizing the proactive locomotion and sensory characteristics of bacteria, which facilitated the active transportation and delivery of various cargoes, such as imaging constituents, genetic material, and therapeutics, to living cells. The method also may carry therapeutic gene components to live tissues in in vitro disease model systems (e.g., OOC devices).180 Jeon et al. illustrated that MNRs hold the capability to transfer colorectal carcinoma cells to microtissue tumors in a liver-tumor micro-organ-on-chip under in vitro conditions. Similarly, it was demonstrated that nanorobots possess the ability to traverse through mesenchymal stem cells located in an intraperitoneal cavity of a nude mouse brain, and in a blood vessel of a rat brain under in vivo conditions.181 Sun et al. have introduced a caterpillar-styled soft robotic entity in a heart-on-a-chip paradigm, which is driven by cardiomyocytes and derives structural color alteration as a result of employing an inverse opal flexible substrate, aiming to serve as a platform for drug discovery.182 Raman et al. developed a biohybrid robot by seeding genetically modified myoblasts onto a patterned substrate or a microfluidic chip integrated with elastic cantilevers. The activities of the microrobot were modulated via electrical stimulation control and motor neuron innervation through the neuromuscular junction.183
The integration of OOC and AI technologies is a burgeoning research area with considerable prospects for advancing drug development, disease modeling, and personalized medicine.184 AI-powered OOCs are expected to originate innovative paradigms in cellular network modeling.185 As presented by Jordan and Mitchell, machine learning algorithms can readily be leveraged to detect on-chip recurring patterns among diverse cells and/or cellular clusters once cellular activities were quantified into numerical features. This usage facilitated the comprehension of biological behaviors in response to various external factors encompassing contaminants and chemical stimulants. Comes et al. introduced an innovative hybrid-imaging paradigm that hinged on the assimilation of organs-on-chip/time-lapse microscopy (OOC/TLM) with a multi-scale generative adversarial network. The objective was to generate interleaved video frames to yield high-throughput videos. The predictive aptitude of GAN was tested on synthetic videos and on actual OOC experiments. This proposed methodology grants the option to obtain a lower quantity of high-caliber TLM images.186 Reported by Pérez-Aliacar et al., a CNN framework was formulated to pinpoint the critical parameters of tumor cell behavior from fluorescence images within a glioblastoma OOC setup.187 Chong et al. presented a microfluidic multicellular co-culture array (MCA) combined with machine learning for the appraisal of skin sensitivity to drugs. The predictive efficacy of the MCA and the SVM classification algorithm embodied a model precision of 87.5%, specificity of 75%, and a sensitivity of 100% in foretelling the skin's adverse drug responses.188 Theoretically, OOCs provide ideal platforms for expanding the capabilities of machine learning-based microrobot control. The synergy of AI, OOC and MNRs may empower the amalgamation of advanced computational methodologies with bioengineered models and nanoscale devices, and is expected to enable breakthroughs in precision medicine and healthcare technology in the future.
4.4 Biopsy and precision surgery
Traditional tissue biopsy procedures typically involve invasive methods that may cause patient discomfort and possible complications. Biopsies-leveraging MNRs can be executed with a higher degree of precision and work in a minimally or non-invasive manner, owing to their minuscule size and superior maneuverability. These attributes make micro–nano robotic biopsies more efficient and have lower associated side effects. Also, the capacity for target specific sampling significantly enhances the accuracy of diagnostics. Gultepe et al. elucidated the application of microgrippers, which distinctly feature a smaller scale compared with biopsy forceps or robotic grasping apparatus. Their operations hinged on the mechanical energy derived from residual stress-fueled microactuators.189 Actis et al. devised a single-cell nanobiopsy platform that depended on scanning ion conductance microscopy tailored for the continuous sampling of the intracellular content from individual cells. This nanobiopsy platform harnesses electrowetting within a nanopipette to sequester cellular material from living cells, while minimally disrupting the cellular environment.190
Traditional surgical procedures usually require large or multiple incisions, invasive manipulation, and a relatively long recovery period. Surgeries based on MNRs introduce a new dimension to minimally invasive surgery with higher precision and unprecedented dexterity.191 Unique capabilities such as navigability across intricate anatomical structures, accurate targeting with reduced trauma to surrounding tissues can be endowed by MNRs. Then, they help to reduce the risk of postoperative complications, wound infection, and pain, while accelerating patient recovery.192 In particular, compared with their larger robotic equivalents, MNRs are able to accomplish procedures at the cellular level. In the last decade, micro/nanorobotic instruments (including nanodrillers, microgrippers, and microbullets) have shown distinct capabilities for minimally invasive surgical procedures when utilized in an untethered manner.193 Go et al. presented microrobots, which can be magnetically directed towards the tumor feeding vessels to perform transcatheter chemoembolization in the liver in vivo.194 Cao et al. introduced phototactic/phototherapeutic nanomotors incorporating biodegradable block copolymers adorned with aggregation-induced emission motifs capable of transmuting radiant energy into movement. The potential applicability of these nanomotors in photodynamic therapy was substantiated in vitro.195 Lin et al. documented the deployment of a bubble-propelled Ga/Zn Janus micromotor with strong biocompatibility and biodegradability, which can be employed for the active management of bacterial infections. The motility of Ga/Zn micromotors was amplified by the Ga–Zn galvanic effect, and then the antibacterial efficiency against H. pylori were significantly elevated.196
AI grants significant advantages in the sphere of micro and nanoscale robotics concerning targeted biopsies and precision surgery. Through machine learning algorithms, MNRs can be continuously self-improved to enhance their performance over time. The ability to integrate and synthesize data from various sources by AI could also promote surgical procedure or biopsy, following the patient's individual needs. Recently, implementation of computer vision and RL algorithms has suggested potential avenues for further exploration in this domain. Zhang et al. introduced a data-driven approach for estimating pose and depth in a system of optically manipulated microrobots. The utilization of focus measurement provided features for Gaussian process regression (GPR) to facilitate depth estimation. A deep residual neural network fused with prior knowledge encoded in GPR was employed by the microrobots to exhibit various poses. The study demonstrated novel microrobotics for potential surgery application.197 Xie et al. demonstrated algorithms premised on visual data to discern the cell from image plane orientation angles, which leveraged two-dimensional image slices garnered via microscope. Subsequently, a feedback controller was presented to accomplish the out-of-plane cellular rotation. Cell nucleus extraction surgeries were conducted to validate the efficacy of the proposed approach.198 Yang et al. reported a model-independent deep RL algorithm that instructed colloidal robots to navigate proficiently amidst unfamiliar terrains. The robot agents acquired the capability to execute navigation decisions based on circumventing obstacles and reducing travel time. The employment of this robot and training methodology may potentially contribute to the advancement of precision surgery.199 Biopsy and precision surgical interventions powered by AI-enhanced MNRs are in their initial stages of evolution. However, the amalgamation of these technologies offers promising potential to radically transform the conventional paradigm and improve patient-centered care execution.
4.5 Maintenance of implanted devices
Traditional methods for the maintenance of implantable devices usually consist of external monitoring systems, routine medical check-ups, and invasive procedures. Routine medical check-ups can detect device malfunctions, but the interval between each check-up might leave potential issues for prolonged periods. Invasive procedures for the maintenance, repair, or replacement pose inherent surgical risks. MNRs for the monitoring and maintenance of implantable devices provide several unique advantages, which mainly stem from overcoming the limitations of traditional methods.200 MNRs are able to directly interface with the device and surrounding tissues at a micro/nano level. This allows for an unprecedented level of detail in data acquisition, and facilitate far superior accuracy in monitoring. The high frequency of data acquisition allows for the real-time visibility of device function and condition. Moreover, the precision, small size and excellent maneuverability of MNRs make them ideal candidates for highly targeted maintenance or repair tasks without the need for risky procedures.
Titanium miniplates, which are essential in oral and maxillofacial surgery, often entail removal due to biofilm-induced complications. Ussia et al. exploited the potential of light-activated nanorobots to control bacterial biofilms on these implants. By motion analysis, various responses to different wavelengths were disclosed. The results suggested that these nanorobots could enhance the treatment of biofilm-infected metallic miniplates.201 Mayorga-Martinez et al. effectively eliminated dental biofilm on titanium implants using swarming magnetic microrobots constructed from ferromagnetic and photoactive components. These microrobots derive their propulsion from a rotating magnetic field, while utilizing reactive oxygen species for biofilm eradication. This demonstrates their potential application in precision medicine (Fig. 6).202 The team also developed magnetic microrobots composed of halloysite nanotubes and iron oxide nanoparticles, which were capable of carrying ampicillin. These microrobots demonstrate multimodal motion, transitioning between various forms of movement.203 Dong et al. developed a microswarm controlled by a rotating magnetic field. Remote actuation, high cargo capacity, and strong localized convections had been realized. The swarm efficiently eliminated biofilms by generating bactericidal free radicals and physically disrupting the biofilm. This platform holds promise for treating biofilm occlusions in medical and industrial settings.204 Interestingly, in some scenarios, the MNRs themselves can serve as wireless controlled implantable devices. Chatzipirpiridis et al. presented miniaturized devices exhibiting potential applications in ophthalmology, specifically in targeted drug release and minimally invasive surgery. Manipulation of the microimplants in biological settings was facilitated by a 5-degree-of-freedom magnetic manipulation system. The tubular shape maximized the size of the magnetic materials, and enabled seamless injection into the eye.205
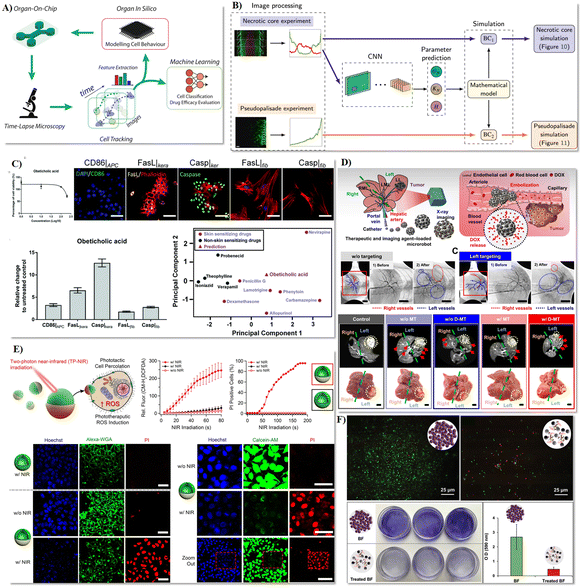 |
| Fig. 6 Intelligent biomedical robots tested in microfluidics for organ-on-a-chip assay, biopsy and precision surgery, and maintenance of implanted devices. A) Concept of an AI-enhanced high-throughput platform for comprehensive investigation and replication of the intricate characteristics of the tumor microenvironment.185 B) Deep learning-based prediction of cell behaviour parameters from glioblastoma using on-chip images.187 C) Fusion of a microfluidic multicellular coculture array coupled with machine learning to forecast the skin sensitizing capability of obeticholic acid.188 D) Assessment of tumor therapy utilizing microrobots.194 E) Investigation of the biological efficacy of phototherapeutic nanomotors on cancer cells.195 F) Assessment of the antibacterial activity of microrobots on a planar substrate.202 Reproduced with permission from ref. 185. Copyright 2019, Frontiers Media S.A., ref. 187. Copyright 2021, Elsevier, ref. 188. Copyright 2022, Royal Society of Chemistry, ref. 194. Copyright 2022, Science Advances, ref. 195. Copyright 2021, Nature Portfolio, ref. 202. Copyright 2023, Wiley. | |
Despite the joint studies into AI, MNRs and implantable devices are few so far. The recent application of nanorobots in the dentistry field has provided potential opportunities for this integration.206 A dentifrice containing configured nanorobots was developed for calculus debridement. These nanorobots were capable of identifying pathogenic bacteria present within the plaque that cause halitosis and subsequently eliminating them. To ensure safety, they were preprogrammed with an automatic disablement feature if inadvertently ingested.207 A nanorobotic dentifrice which could survey all supragingival and subgingival surfaces for undertaking constant calculus removal was also introduced. The nanorobots would be programmed to achieve enhanced teeth cleaning outcomes. With the development in material science, fabrication processes, and algorithms, a new era beckons in the integrated research of AI, MNRs, and implantable technologies.
5. Challenges and perspectives
Intelligent biomedical robots in microfluidics have brought about cutting-edge and promising possibilities within the biomedical field. Nevertheless, the amalgamation of these revolutionary technologies also poses some significant challenges that warrant investigation.
5.1 Design, fabrication and integration
One of the foremost obstacles encountered in the field of MNRs pertains to the difficulty in ensuring the cohesive functionality of constituent components. The primary factor underpinning this trial can be linked to the scale-dependent sensitivity of these robotic systems. In essence, as the dimensions of the robot decrease, an exponential rise in its sensitivity toward environmental changes is experienced. This necessitates that the design components of MNRs not merely function independently, but that they also operate collectively in an intricate, microscopically coordinated manner dedicated to precision.
Also, the gradual reduction in size of these robotic systems poses specific issues with respect to their fabrication. Traditional manufacturing methods, with their broad scopes and larger scales of operation, often find themselves inept at addressing the nuanced needs of MNR production. Therefore, the development of precise, scalable, and specialized fabrication techniques capable of producing these minuscule robotic systems remains an essential and central challenge.208
Additionally, the inherent features of MNRs create further complications. The drastically reduced physical dimensions of micro and nanorobots correlate directly with the limited sensor, actuator, and power-source capacity, thereby catalyzing the demand for advanced miniaturized and integrated technologies that facilitate efficient in situ operations. This shifts the focus from an external field dependency toward developing internal capacities, further reinforcing the complexity in the operational design of MNRs.
In addition to the intricate operational design, miniaturized sensor technology development, and precise fabrication techniques, the issues of functionality optimisation (while ensuring cost-effectiveness) also pose significant challenges. Increased sensitivity to environmental changes, integration at higher densities, and robust operation under dynamic conditions also constitute key hurdles in the successful implementation and deployment of MNRs that researchers strive to address. This industry's evolution thus depends heavily on technological advancements, fabrication prowess, and interdisciplinary synergy of materials science, robotics, and control engineering, among others.
5.2 Control strategy and algorithm
The paramount algorithmic challenges in the evolving realms of autonomous micro-/nano robotic systems principally encompass the development of real-time decision-making mechanisms, formulating effective coordination algorithms for decentralized control in multi-agent systems, and enhancing machine learning techniques to accommodate fluctuating conditions and environmental uncertainties.
Micro-/nano robotic navigation in intricate environments represents a notable challenge due to the minuscule sizes and distinct navigational constraints of these technologies. Therefore, real-time decision-making tools are indispensable to enhance the dynamism of these systems. Advancements in this area require innovative algorithms that can instantaneously analyze high volumes of data from sensors to calculate an optimal pathway, while simultaneously circumventing obstacles. These algorithms, due to the sensitivity of micro-/nano robotic systems, need to accommodate a variety of uncertainties associated with varying environmental conditions. The task of articulating such sophisticated algorithms requires an in-depth understanding of the micro-/nano scale dynamics, making such ventures intricate and demanding.
Multi-agent systems, characterized by multiple interactive entities or agents working together towards a collective outcome, introduce another significant challenge: devising efficient coordination algorithms for decentralized control. Such systems, given their inherent decentralized structure and collaborative orientation, necessitate the operators (individual agents) to not only make independent decisions, but also synchronize their actions harmoniously with others. The complexities arise from including the individual agents' unique capabilities and inter-agent dynamics, while designing these algorithms, culminating in a multifaceted challenge that involves several areas of research from control theory to computer science.
Moreover, the dynamic nature of micro-/nano environments and the inherent uncertainties implicate the need for refining machine learning techniques for these systems. Machine learning algorithms need to consistently adapt and learn from new data, a challenge that intensifies in environments prone to sudden changes. Consequently, the focus lies in developing algorithms that are dexterous in navigating the thin line between adapting to new information (plasticity) and retaining learnt knowledge from historical data (stability), a conundrum that is widely thought of as the plasticity-stability dilemma in the field of machine learning.
Given the intertwined complexity of the algorithmic challenges, the approach to devise solutions should be interdisciplinary, involving expertise from the fields of computer science, artificial intelligence, nanotechnology, robotics, and data analytics. Liquidating these ostensible bottlenecks would yield revolutionary advancements in micro-/nano robotic systems, making them more robust, efficient, and adaptable than ever.
5.3 Safety and ethical considerations
AI-enhanced MNRs, owing to their miniature sizes, can infiltrate human systems where any unplanned disturbances could potentially lead to detrimental consequences. This introduces the pivotal importance of the development of detailed error-correction and fault-mitigation strategies that can prevent any unexpected system malfunction or erroneous behavior.
In parallel, the associated system or equipment of these MNRs may potentially collect and monitor personal data without explicit knowledge or consent. The nature of invasive technologies sometimes makes it challenging to preserve the sanctity of private information. In this digital age characterized by massive data creation and storage, questions about data privacy and security have become more pressing. Analogue tracking features must be adequately defended against potential misuse, warranting the encryption of personal health data and ensuring necessary control measures are in place to handle data breaches. This leads to significant ethical implications, such as the transparency in decision-making processes and impact on human life. These MNRs, while contributing immensely to the advancement of science and technology, are effectively making decisions autonomously – a characteristic that may consequently lead to potential ethical quandaries, especially in incidences of error or crisis. It becomes crucial to address these ethical conundrums by striking a delicate balance between encouraging advancements in algorithmic development and ensuring ethical considerations are not compromised.
Precisely, a proactive regulatory framework needs to be devised that governs the operational principles of MNRs, thereby defining a boundary for their decision-making capacity, while ensuring the indispensable accountability. Such a framework would not only free humans from being unreasonably subjected to automated decisions, but also authenticate that the accountability for decisions is not entirely relinquished to AI. The whole exploration of AI-enhanced MNRs thereby stands as a paramount stride towards scientific growth, warranting an equally evolved safety, ethical and regulatory framework.
5.4 Perspectives
Artificial general intelligence (AGI) focuses on developing systems that can learn and perform any intellectual task that a human can. One of the most significant advances in AGI is the development of RL, which can be used to train MNRs to navigate the human body, to deliver drugs to specific cells, or to repair damaged tissue. If AGI is ever achieved, it will have a profound impact on MNRs, which are more intelligent, more autonomous, and more capable than what is currently possible. AGI could also be used to develop new ways to control and manipulate MNRs. Furthermore, it is exciting that given the promising prospects of compute-in- memory chip or in situ learning technology, a superior integration of AI-enhanced MNRs in microfluidics may be achieved. Unencumbered by conscious intervention and devoid of bulky external devices, these robots are poised to conquer much more intricate tasks intelligently and autonomously.
In parallel, with the continuous development of lab-on-a-chip technology, an increasing amount of massive data in formats of time series, image, and video is generated. AI provides an unprecedented ability to integrate these diverse data types, and consequently enhances the performances of decision making and task execution. The attainment of success relied on the standardization of data collected from MNRs across various patient populations. Collaborations among experts in AI, big data, medicine, bioinformatics, MNRs and microfluidics will expedite the interdisciplinary field towards more avant-garde development.
Currently, technological revolutions are spurred by transformative advances in 3D printing nanofabrication, and the fusion of biological and information technologies. These encompass the internet of things (IoT), robotics, artificial intelligence, and nanomedicine. Progressions in 3D technology have given birth to mobile microsystems, capable of diverse in vitro and in vivo functionalities. Micro-robotics presents a wealth of opportunities to address a myriad of challenges related to minimally invasive diagnostics, targeted drug delivery, gene engineering, organ-on-a-chip phenomena, among others. These burgeoning technologies could play a seminal role in the commercialization of concept-validated micro-robots as clinical devices. In this light, several domains are expected to emerge as future foci: 1) incorporation of artificial intelligence algorithms within MNRs. 2) Optimization of payload efficiency through intelligent, collaborative operation of MNR swarms. 3) Imbuing sensing, actuation, and learning/decision-making capabilities into MNRs using smart materials.
6. Conclusions
Human health is determined by microscopic physiological environments and processes within the body. The integration of artificial intelligence with micro/-nano robots has revolutionized their design, fabrication, and functionality. In particular, motion control, sensing abilities, and intricate collective behavior in microfluidics can be significantly enhanced by artificial intelligence. This pioneering technology has the potential to transform biomedical fields and further our understanding of complex physiological mechanisms, despite current challenges that need to be addressed for its practical implementation.
Author contributions
Hui Dong, Jiawen Lin and Yihui Tao: investigation, visualization, and writing – original draft. Yuan Jia: writing – review and editing. Lining Sun and Wen Jung Li: manuscript editing and project supervision. Hao Sun: funding acquisition, investigation, supervision; manuscript draft, review, and editing.
Conflicts of interest
There are no conflicts to declare.
Acknowledgements
This work was supported by the National Natural Science Foundation of China [grant numbers: 62173093, 61604042], and the National Key Research and Development Program of China [grant number: 2022YFB3606502].
Notes and references
- D. D. Gutterman, D. S. Chabowski, A. O. Kadlec, M. J. Durand, J. K. Freed, K. Ait-Aissa and A. M. Beyer, Circ. Res., 2016, 118, 157–172 CrossRef CAS PubMed.
- H. Luan, Q. Zhang, T.-L. Liu, X. Wang, S. Zhao, H. Wang, S. Yao, Y. Xue, J. W. Kwak and W. Bai, Sci. Adv., 2021, 7, eabj3686 CrossRef CAS PubMed.
- D. R. Myers and W. A. Lam, Annu. Rev. Biomed. Eng., 2021, 23, 407–432 CrossRef CAS PubMed.
- A. Manz, N. Graber and H. á. Widmer, Sens. Actuators, B, 1990, 1, 244–248 CrossRef CAS.
- M. A. Unger, H.-P. Chou, T. Thorsen, A. Scherer and S. R. Quake, Science, 2000, 288, 113–116 CrossRef CAS PubMed.
- G. M. Whitesides, Nature, 2006, 442, 368–373 CrossRef CAS PubMed.
- D. Huh, B. D. Matthews, A. Mammoto, M. Montoya-Zavala, H. Y. Hsin and D. E. Ingber, Science, 2010, 328, 1662–1668 CrossRef CAS PubMed.
- H. Sun, Y. Jia, H. Dong, D. Dong and J. Zheng, Curr. Opin. Chem. Eng., 2020, 28, 1–9 CrossRef.
- B. Zhang, A. Korolj, B. F. L. Lai and M. Radisic, Nat. Rev. Mater., 2018, 3, 257–278 CrossRef.
- S. N. Bhatia and D. E. Ingber, Nat. Biotechnol., 2014, 32, 760–772 CrossRef CAS PubMed.
- A. Loewa, J. J. Feng and S. Hedtrich, Nat. Rev. Bioeng., 2023, 1–15 Search PubMed.
- L. A. Low, C. Mummery, B. R. Berridge, C. P. Austin and D. A. Tagle, Nat. Rev. Drug Discovery, 2021, 20, 345–361 CrossRef CAS PubMed.
- P. Vulto and J. Joore, Nat. Rev. Drug Discovery, 2021, 20, 961–962 CrossRef CAS PubMed.
- R. P. Feynman, Eng. Sci., 1959, 23 Search PubMed.
- P. Guo, Nat. Nanotechnol., 2010, 5, 833–842 CrossRef CAS PubMed.
- S. Li, C. Li, M. I. Khan, J. Liu, Z. Shi, D. Gao, B. Qiu and W. Ding, Microsyst. Nanoeng., 2023, 9, 75 CrossRef CAS PubMed.
- B. J. Nelson, I. K. Kaliakatsos and J. J. Abbott, Annu. Rev. Biomed. Eng., 2010, 12, 55–85 CrossRef CAS PubMed.
- B. Wang, K. Kostarelos, B. J. Nelson and L. Zhang, Adv. Mater., 2021, 33, 2002047 CrossRef CAS PubMed.
- M. Sitti, H. Ceylan, W. Hu, J. Giltinan, M. Turan, S. Yim and E. Diller, Proc. IEEE, 2015, 103, 205–224 CAS.
- S. Baghbani Kordmahale, J. Qu, A. Muliana and J. Kameoka, Sci. Rep., 2022, 12, 21403 CrossRef CAS PubMed.
- C. Wang, S. Chandra, X. Tan and S. Tor, J. Micromech. Mol. Phys., 2021, 6, 2141002 CrossRef CAS.
- Y. Wu, S. Yakov, A. Fu and G. Yossifon, Adv. Sci., 2023, 10, 2204931 CrossRef CAS PubMed.
- T. Evens, L. Van Hileghem, F. Dal Dosso, J. Lammertyn, O. Malek, S. Castagne, D. Seveno and A. Van Bael, J. Micro Nano-Manuf., 2021, 9, 030902 CrossRef CAS.
- Z. H. Shah, M. Sokolich, S. Mallick, D. Rivas and S. Das, J. Mater. Chem. B, 2023, 11, 8926–8932 RSC.
- W. Siti, H. L. Too, T. Anderson, X. R. Liu, I. Y. Loh and Z. Wang, Sci. Adv., 2023, 9, eadi8444 CrossRef CAS PubMed.
- E. Diller, J. Giltinan and M. Sitti, Int. J. Rob. Res., 2013, 32, 614–631 CrossRef.
- G. Hwang, I. A. Ivan, J. Agnus, H. Salmon, S. Alvo, N. Chaillet, S. Régnier and A.-M. Haghiri-Gosnet, Sens. Actuators, B, 2014, 215, 56–64 CrossRef CAS.
- H. Choi, B. Kim, S. H. Jeong, T. Y. Kim, D. P. Kim, Y. K. Oh and S. K. Hahn, Small, 2023, 19, 2204617 CrossRef CAS PubMed.
- H. Zhou, C. C. Mayorga-Martinez, S. Pané, L. Zhang and M. Pumera, Chem. Rev., 2021, 121, 4999–5041 CrossRef CAS PubMed.
- Y. Deng, A. Paskert, Z. Zhang, R. Wittkowski and D. Ahmed, Sci. Adv., 2023, 9, eadh5260 CrossRef CAS PubMed.
- W. Yang, X. Wang, Z. Wang, W. Liang and Z. Ge, Opt. Lasers Eng., 2023, 161, 107380 CrossRef.
- S. R. Dabbagh, M. R. Sarabi, M. T. Birtek, S. Seyfi, M. Sitti and S. Tasoglu, Nat. Commun., 2022, 13, 5875 CrossRef CAS PubMed.
- D. A. LaVan, D. M. Lynn and R. Langer, Nat. Rev. Drug Discovery, 2002, 1, 77–84 CrossRef CAS PubMed.
- S. S. Andhari, R. D. Wavhale, K. D. Dhobale, B. V. Tawade, G. P. Chate, Y. N. Patil, J. J. Khandare and S. S. Banerjee, Sci. Rep., 2020, 10, 4703 CrossRef CAS PubMed.
- R. D. Wavhale, K. D. Dhobale, C. S. Rahane, G. P. Chate, B. V. Tawade, Y. N. Patil, S. S. Gawade and S. S. Banerjee, Commun. Chem., 2021, 4, 159 CrossRef CAS PubMed.
- M. Li, N. Xi, Y. Wang and L. Liu, IEEE Trans. Biomed. Eng., 2020, 68, 130–147 Search PubMed.
- B. J. Heil, M. M. Hoffman, F. Markowetz, S.-I. Lee, C. S. Greene and S. C. Hicks, Nat. Methods, 2021, 18, 1132–1135 CrossRef CAS PubMed.
- H. Sun, W. Xie, Y. Huang, J. Mo, H. Dong, X. Chen, Z. Zhang and J. Shang, Talanta, 2023, 258, 124470 CrossRef CAS PubMed.
- L. Liu, M. Bi, Y. Wang, J. Liu, X. Jiang, Z. Xu and X. Zhang, Nanoscale, 2021, 13, 19352–19366 RSC.
- Y. Zhuang, S. Cheng, N. Kovalchuk, M. Simmons, O. K. Matar, Y.-K. Guo and R. Arcucci, Lab Chip, 2022, 22, 3187–3202 RSC.
- H. Sun, W. Xie, J. Mo, Y. Huang and H. Dong, Front. Bioeng. Biotechnol., 2023, 11, 1208648 CrossRef PubMed.
- Z. Ao, H. Cai, Z. Wu, L. Hu, A. Nunez, Z. Zhou, H. Liu, M. Bondesson, X. Lu and X. Lu, Proc. Natl. Acad. Sci. U. S. A., 2022, 119, e2214569119 CrossRef CAS PubMed.
- E. A. Holm, R. Cohn, N. Gao, A. R. Kitahara, T. P. Matson, B. Lei and S. R. Yarasi, Metall. Mater. Trans. A, 2020, 51, 5985–5999 CrossRef CAS.
- L. Huang, Y. Pan, M. Wang and L. Ren, Eng. Regen., 2023, 4, 411–426 Search PubMed.
- Q. Wang and L. Zhang, ACS Nano, 2021, 15, 149–174 CrossRef CAS PubMed.
- M. Guo, Z. Lin, C. Zhai, Y. Chen, X. Zhou, Z. Chai, T. Guo and C. Hu, Opt. Express, 2023, 31, 12397–12409 CrossRef CAS PubMed.
- S. Yu, Y. Cai, Z. Wu and Q. He, View, 2021, 2, 20200113 CrossRef.
- Y. Zhou, M. Ye, C. Hu, H. Qian, B. J. Nelson and X. Wang, ACS Nano, 2023, 17, 15254–15276 CrossRef CAS PubMed.
- K. A. Brown, S. Brittman, N. Maccaferri, D. Jariwala and U. Celano, Nano Lett., 2019, 20, 2–10 CrossRef PubMed.
- H. Sun, L. Xiong, Y. Huang, X. Chen, Y. Yu, S. Ye, H. Dong, Y. Jia and W. Zhang, Fundam. Res., 2022, 2, 476–486 CrossRef CAS.
- Z. Wang, Y. Wu, B. Zhu, Q. Chen, L. Wang, Y. Zhao, D. Sun, J. Zheng and D. Wu, Addit. Manuf., 2023, 61, 103320 CAS.
- J. E. Huddy, M. S. Rahman, A. B. Hamlin, Y. Ye and W. J. Scheideler, Cell Rep. Phys. Sci., 2022, 3, 100786 CrossRef CAS.
- J. Moor, AI Mag., 2006, 27, 87–87 Search PubMed.
- R. ElShawi, Y. Sherif, M. Al-Mallah and S. Sakr, Comput. Intell., 2021, 37, 1633–1650 CrossRef.
- M. Hammad, R. N. Kandala, A. Abdelatey, M. Abdar, M. Zomorodi-Moghadam, R. San Tan, U. R. Acharya, J. Pławiak, R. Tadeusiewicz and V. Makarenkov, Inf. Sci., 2021, 571, 580–604 CrossRef.
- A. Mavropoulos, C. Johnson, V. Lu, J. Nieto, E. C. Schneider, K. Saini, M. L. Phelan, L. X. Hsie, M. J. Wang and J. Cruz, Mod. Pathol., 2023, 36, 100195 CrossRef PubMed.
- J. Zheng, T. Cole, Y. Zhang, J. Kim and S.-Y. Tang, Biosens. Bioelectron., 2021, 194, 113666 CrossRef CAS PubMed.
- Z. Fu, X. Li, Z. Wang, Z. Li, X. Liu, X. Wu, J. Zhao, X. Ding, X. Wan and F. Zhong, Org. Chem. Front., 2020, 7, 2269–2277 RSC.
- K. Yiannacou, V. Sharma and V. Sariola, Langmuir, 2022, 38, 11557–11564 CrossRef CAS PubMed.
- A. Korotcov, V. Tkachenko, D. P. Russo and S. Ekins, Mol. Pharmaceutics, 2017, 14, 4462–4475 CrossRef CAS PubMed.
- G. Stiglic, P. Kocbek, N. Fijacko, M. Zitnik, K. Verbert and L. Cilar, WIREs Data Min. Knowl., 2020, 10, e1379 CrossRef.
- C. Rudin, Nat. Mach. Intell., 2019, 1, 206–215 CrossRef PubMed.
- F. Oviedo, J. L. Ferres, T. Buonassisi and K. T. Butler, Acc. Mater. Res., 2022, 3, 597–607 CrossRef CAS.
- M. Rubin, O. Stein, N. A. Turko, Y. Nygate, D. Roitshtain, L. Karako, I. Barnea, R. Giryes and N. T. Shaked, Med. Image Anal., 2019, 57, 176–185 CrossRef PubMed.
- A. Ahmad, F. Sala, P. Paiè, A. Candeo, S. d'Annunzio, A. Zippo, C. Frindel, R. Osellame, F. Bragheri and A. Bassi, Lab Chip, 2022, 22, 3453–3463 RSC.
- F. Grisoni, B. J. Huisman, A. L. Button, M. Moret, K. Atz, D. Merk and G. Schneider, Sci. Adv., 2021, 7, eabg3338 CrossRef CAS PubMed.
- C. A. Patino, P. Mukherjee, E. J. Berns, E. H. Moully, L. Stan, M. Mrksich and H. D. Espinosa, ACS Nano, 2022, 16, 7937–7946 CrossRef CAS PubMed.
- M. Durve, F. Bonaccorso, A. Montessori, M. Lauricella, A. Tiribocchi and S. Succi, Philos. Trans. R. Soc., A, 2021, 379, 20200400 CrossRef CAS PubMed.
- H. Sun, Q. Jiang, Y. Huang, J. Mo, W. Xie, H. Dong and Y. Jia, Biomed. Signal Process. Control, 2023, 83, 104721 CrossRef.
- L. Zdeborová, Nat. Phys., 2020, 16, 602–604 Search PubMed.
- K. E. Willcox, O. Ghattas and P. Heimbach, Nat. Comput. Sci., 2021, 1, 166–168 Search PubMed.
- G. E. Karniadakis, I. G. Kevrekidis, L. Lu, P. Perdikaris, S. Wang and L. Yang, Nat. Rev. Phys., 2021, 3, 422–440 Search PubMed.
- M. Raissi, J. Mach. Learn. Res., 2018, 19, 932–955 Search PubMed.
- S. H. Hong, H. Yang and Y. Wang, Microfluid. Nanofluid., 2020, 24, 1–20 CrossRef.
- J. X. Wang, W. Yu, Z. Wu, X. Liu and Y. Chen, Appl. Phys. Lett., 2022, 120, 204101 CrossRef CAS.
- S. Cai, H. Li, F. Zheng, F. Kong, M. Dao, G. E. Karniadakis and S. Suresh, Proc. Natl. Acad. Sci. U. S. A., 2021, 118, e2100697118 CrossRef CAS PubMed.
- L. P. Kaelbling, M. L. Littman and A. W. Moore, J. Artif. Intell. Res., 1996, 4, 237–285 CrossRef.
- M. Shahab and R. Rengaswamy, Comput. Chem. Eng., 2022, 161, 107787 CrossRef CAS.
- T. Abe, S. Oh-Hara and Y. Ukita, Biomicrofluidics, 2021, 15, 034101 CrossRef PubMed.
- T. Abe, S. Ohhara and Y. Ukita, Sens. Actuators, B, 2023, 134636 CrossRef CAS.
- J. Kennedy, Swarm Intell, 2006, 187–219 Search PubMed.
- J. Tang, G. Liu and Q. Pan, IEEE/CAA Journal of Automatica Sinica, 2021, 8, 1627–1643 Search PubMed.
- Q. T. Bui, Q.-H. Nguyen, X. L. Nguyen, V. D. Pham, H. D. Nguyen and V.-M. Pham, J. Hydrol., 2020, 581, 124379 CrossRef.
- M. Matta, G. C. Cardarilli, L. Di Nunzio, R. Fazzolari, D. Giardino, M. Re, F. Silvestri and S. Spanò, Electron. Lett., 2019, 55, 589–591 CrossRef.
- S. Mukherjee, I. Pan and T. Samanta, Appl. Soft Comput., 2019, 85, 105839 CrossRef.
- K. Huang, Z. Cui, J. Lai, B. Lu and H. K. Chu, IEEE Trans. Autom. Sci. Eng., 2021, 19, 818–832 Search PubMed.
- E. Arun Sankar, M. Shahab and R. Rengaswamy, Ind. Eng. Chem. Res., 2021, 60, 1699–1708 CrossRef CAS.
- H. Tao, T. Wu, M. Aldeghi, T. C. Wu, A. Aspuru-Guzik and E. Kumacheva, Nat. Rev. Mater., 2021, 6, 701–716 CrossRef.
- Y. Jia, X. Hou, Z. Wang and X. Hu, ACS Sustainable Chem. Eng., 2021, 9, 6130–6147 CrossRef CAS.
- P. H. Thike, Z. Zhao, P. Shi and Y. Jin, Bull. Mater. Sci., 2020, 43, 1–22 CrossRef.
- X. Chen and H. Lv, NPG Asia Mater., 2022, 14, 69 CrossRef.
- M. Zeng, S. Yuan, D. Huang and Z. Cheng, ACS Appl. Mater. Interfaces, 2019, 11, 40099–40106 CrossRef CAS PubMed.
- P. Jiao, Nano Energy, 2021, 88, 106227 CrossRef CAS.
- N. A. Alderete, N. Pathak and H. D. Espinosa, npj Comput. Mater., 2022, 8, 191 CrossRef.
- Y. Liu, Y. Feng, M. An, M. T. Sarwar and H. Yang, Adv. Intell. Syst. Comput., 2023, 2200466 CrossRef.
- A. Pena-Francesch, J. Giltinan and M. Sitti, Nat. Commun., 2019, 10, 3188 CrossRef PubMed.
- H. Xu, M. Medina-Sánchez, M. F. Maitz, C. Werner and O. G. Schmidt, ACS Nano, 2020, 14, 2982–2993 CrossRef CAS PubMed.
- M. M. Stanton, B.-W. Park, D. Vilela, K. Bente, D. Faivre, M. Sitti and S. Sánchez, ACS Nano, 2017, 11, 9968–9978 CrossRef CAS PubMed.
- J. Guo, J. O. Agola, R. Serda, S. Franco, Q. Lei, L. Wang, J. Minster, J. G. Croissant, K. S. Butler and W. Zhu, ACS Nano, 2020, 14, 7847–7859 CrossRef CAS PubMed.
- I. C. Yasa, H. Ceylan, U. Bozuyuk, A.-M. Wild and M. Sitti, Sci. Robot., 2020, 5, eaaz3867 CrossRef PubMed.
- M. Sun, X. Fan, X. Meng, J. Song, W. Chen, L. Sun and H. Xie, Nanoscale, 2019, 11, 18382–18392 RSC.
- L. Xie, X. Pang, X. Yan, Q. Dai, H. Lin, J. Ye, Y. Cheng, Q. Zhao, X. Ma and X. Zhang, ACS Nano, 2020, 14, 2880–2893 CrossRef CAS PubMed.
- J. Li and M. Pumera, Chem. Soc. Rev., 2021, 50, 2794–2838 RSC.
- O. Buchnev, J. A. Grant-Jacob, R. W. Eason, N. I. Zheludev, B. Mills and K. F. MacDonald, Nano Lett., 2022, 22, 2734–2739 CrossRef CAS PubMed.
- N. Theobald, D. Ledvina, K. Kukula, S. Maines, K. Hasz, M. Raschke, J. Crawford, J. Jessing and Y. Li, IEEE Sens. J., 2023, 23, 7910–7916 CAS.
- Z. Sun, J. Shi, J. Wang, M. Jiang, Z. Wang, X. Bai and X. Wang, Nanoscale, 2022, 14, 10761–10772 RSC.
- A. Halder and Y. Sun, Biosens. Bioelectron., 2019, 139, 111334 CrossRef CAS PubMed.
- J. Jiang, Z. Yang, A. Ferreira and L. Zhang, Adv. Intell. Syst., 2022, 4, 2100279 CrossRef.
- M. Li, A. Pal, A. Aghakhani, A. Pena-Francesch and M. Sitti, Nat. Rev. Mater., 2022, 7, 235–249 CrossRef PubMed.
- P. Sharan, A. Nsamela, S. C. Lesher-Pérez and J. Simmchen, Small, 2021, 17, 2007403 CrossRef CAS PubMed.
- H. Xu, M. Medina-Sánchez, V. Magdanz, L. Schwarz, F. Hebenstreit and O. G. Schmidt, ACS Nano, 2018, 12, 327–337 CrossRef CAS PubMed.
- A. P. Bregulla, H. Yang and F. Cichos, ACS Nano, 2014, 8, 6542–6550 CrossRef CAS PubMed.
- A. I. Bunea, D. Martella, S. Nocentini, C. Parmeggiani, R. Taboryski and D. S. Wiersma, Adv. Intell. Syst., 2021, 3, 2000256 CrossRef.
-
A. von Rohr, S. Trimpe, A. Marco, P. Fischer and S. Palagi, Gait Learning for Soft Microrobots Controlled by Light Fields, 2018 IEEE/RSJ International Conference on Intelligent Robots and Systems (IROS), Madrid, Spain, 2018, pp. 6199–6206, DOI:10.1109/IROS.2018.8594092.
- A. C. H. Tsang, P. W. Tong, S. Nallan and O. S. Pak, Phys. Rev. Fluids, 2020, 5, 074101 CrossRef.
- Z. Zou, Y. Liu, Y.-N. Young, O. S. Pak and A. C. Tsang, Commun. Phys., 2022, 5, 158 CrossRef.
- M. R. Behrens and W. C. Ruder, Adv. Intell. Syst., 2022, 4, 2200023 CrossRef.
- B. Hartl, M. Hübl, G. Kahl and A. Zöttl, Proc. Natl. Acad. Sci. U. S. A., 2021, 118, e2019683118 CrossRef CAS PubMed.
- C. Huang, T. Xu, J. Liu, L. Manamanchaiyaporn and X. Wu, IEEE Robot. Autom. Lett., 2019, 4, 4185–4191 Search PubMed.
- J. Janiak, Y. Li, Y. Ferry, A. A. Doinikov and D. Ahmed, Nat. Commun., 2023, 14, 4705 CrossRef CAS PubMed.
- S. Shi, N. Sharifi, U. K. Cheang and Y. Chen, IEEE Trans. NanoBiosci., 2019, 19, 267–269 Search PubMed.
- L. Zheng, N. Hart and Y. Zeng, Lab Chip, 2023, 23, 3741–3767 RSC.
- H. Liu, L. Nan, F. Chen, Y. Zhao and Y. Zhao, Lab Chip, 2023, 23, 2497–2513 RSC.
- B. Dai, J. Wang, Z. Xiong, X. Zhan, W. Dai, C.-C. Li, S.-P. Feng and J. Tang, Nat. Nanotechnol., 2016, 11, 1087–1092 CrossRef CAS PubMed.
- Z. Li, K. Wang, C. Hou, C. Li, F. Zhang, W. Ren, L. Dong and J. Zhao, Microsyst. Nanoeng., 2023, 9, 102 CrossRef PubMed.
- L. Li, Z. Yu, J. Liu, M. Yang, G. Shi, Z. Feng, W. Luo, H. Ma, J. Guan and F. Mou, Nano-Micro Lett., 2023, 15, 141 CrossRef PubMed.
- K. Xu and R. Su, Microsyst. Technol., 2022, 28, 2393–2401 CrossRef.
- S. E. Spagnolie and P. T. Underhill, Annu. Rev. Condens. Matter Phys., 2023, 14, 381–415 CrossRef.
- G. Li, E. Lauga and A. M. Ardekani, J. Non-Newtonian Fluid Mech., 2021, 297, 104655 CrossRef CAS.
- L. Yang, J. Jiang, X. Gao, Q. Wang, Q. Dou and L. Zhang, Nat. Mach. Intell., 2022, 4, 480–493 CrossRef.
- M. Cai, Q. Wang, Z. Qi, D. Jin, X. Wu, T. Xu and L. Zhang, IEEE Trans. Cybern., 2022, 53, 7699–7711 Search PubMed.
- K. Botros, M. Alkhatib, D. Folio and A. Ferreira, IEEE Robot. Autom. Lett., 2023, 8, 3254–3261 Search PubMed.
- J. Liu, T. Xu, S. X. Yang and X. Wu, IEEE Trans. Industr. Inform., 2019, 16, 477–487 Search PubMed.
- S. Colabrese, K. Gustavsson, A. Celani and L. Biferale, Phys. Rev. Lett., 2017, 118, 158004 CrossRef PubMed.
- Y. Yang, M. A. Bevan and B. Li, Adv. Theory Simul., 2020, 3, 2000034 CrossRef CAS.
- L. Amoudruz and P. Koumoutsakos, Adv. Intell. Syst., 2022, 4, 2100183 CrossRef.
- M. Putzke and H. Stark, Eur. Phys. J. E: Soft Matter Biol. Phys., 2023, 46, 48 CrossRef CAS PubMed.
- L. Yang and L. Zhang, Annu. Rev. Control Robot. Auton. Syst., 2021, 4, 509–534 CrossRef.
- L. Yang, M. Zhang, Z. Yang and L. Zhang, IEEE ASME Trans. Mechatron., 2023, 28, 1662–1673 Search PubMed.
- D. Zhang, A. Barbot, F. Seichepine, F. P.-W. Lo, W. Bai, G.-Z. Yang and B. Lo, Commun. Phys., 2022, 5, 80 CrossRef.
- Z. El Khiyati, R. Chesneaux, L. Giraldi and J. Bec, Eur. Phys. J. E: Soft Matter Biol. Phys., 2023, 46, 43 CrossRef CAS PubMed.
- W. Chen, X. Chen, M. Yang, X. Fan, C. Tian, H. Zhang and H. Xie, IEEE ASME Trans. Mechatron., 2023, 28, 1638–1648 Search PubMed.
- S. Xu, J. Liu, C. Yang, X. Wu and T. Xu, IEEE Trans. Cybern., 2021, 52, 13727–13737 Search PubMed.
- H. Keshmiri Neghab, M. Jamshidi and H. Keshmiri Neghab, Information, 2022, 13, 321 CrossRef.
- M. F. Reynolds, A. J. Cortese, Q. Liu, Z. Zheng, W. Wang, S. L. Norris, S. Lee, M. Z. Miskin, A. C. Molnar and I. Cohen, Sci. Robot., 2022, 7, eabq2296 CrossRef PubMed.
- H. Duan, M. Huo and Y. Fan, Natl. Sci. Rev., 2023, 10, nwad040 CrossRef PubMed.
- K. Hou, Y. Yang, X. Yang and J. Lai, Control Theory Technol., 2020, 18, 114–134 CrossRef.
- F. Cichos, K. Gustavsson, B. Mehlig and G. Volpe, Nat. Mach. Intell., 2020, 2, 94–103 CrossRef.
- Q. Wang, K. F. Chan, K. Schweizer, X. Du, D. Jin, S. C. H. Yu, B. J. Nelson and L. Zhang, Sci. Adv., 2021, 7, eabe5914 CrossRef CAS PubMed.
- S. Shahrokhi, L. Lin, C. Ertel, M. Wan and A. T. Becker, IEEE Trans. Robot., 2017, 34, 207–219 Search PubMed.
-
D. Ceraso and G. Spezzano, Controlling swarms of medical nanorobots using CPPSO on a GPU, 2016 International Conference on High Performance Computing & Simulation (HPCS), Innsbruck, Austria, 2016, pp. 58–65, DOI:10.1109/HPCSim.2016.7568316.
- S. Muiños-Landin, A. Fischer, V. Holubec and F. Cichos, Sci. Robot., 2021, 6, eabd9285 CrossRef PubMed.
- Y. Liu, Z. Zou, O. S. Pak and A. C. Tsang, Sci. Rep., 2023, 13, 9397 CrossRef CAS PubMed.
- M. Filippi, O. Yasa, R. D. Kamm, R. Raman and R. K. Katzschmann, Proc. Natl. Acad. Sci. U. S. A., 2022, 119, e2200741119 CrossRef CAS PubMed.
- T. Patiño, X. Arqué, R. Mestre, L. Palacios and S. Sánchez, Acc. Chem. Res., 2018, 51, 2662–2671 CrossRef PubMed.
-
Y. Wang, X. Jin and C. Castro, bioRxiv, 2023, preprint, DOI:10.1101/2023.05.11.540408.
- A. Mirzaiebadizi, H. Ravan, S. Dabiri, P. Mohammadi, A. Shahba, M. Ziasistani and M. Khatami, Bioprocess Biosyst. Eng., 2022, 45, 1781–1797 CrossRef CAS PubMed.
- H. Chen, Y. Li, Y. Wang, P. Ning, Y. Shen, X. Wei, Q. Feng, Y. Liu, Z. Li and C. Xu, ACS Nano, 2022, 16, 6118–6133 CrossRef CAS PubMed.
- Y. Wang, J. Shen, S. Handschuh-Wang, M. Qiu, S. Du and B. Wang, ACS Nano, 2022, 17, 27–50 CrossRef PubMed.
- S. M. Mirvakili and R. Langer, Nat. Electron., 2021, 4, 464–477 CrossRef.
- Z. Yu, L. Li, F. Mou, S. Yu, D. Zhang, M. Yang, Q. Zhao, H. Ma, W. Luo and T. Li, InfoMat, 2023, e12464 CrossRef CAS.
- J. Yoo, S. Tang and W. Gao, Nat. Biomed. Eng., 2023, 1–3 Search PubMed.
- A. V. Singh, V. Chandrasekar, P. Janapareddy, D. E. Mathews, P. Laux, A. Luch, Y. Yang, B. Garcia-Canibano, S. Balakrishnan and J. Abinahed, ACS Chem. Neurosci., 2021, 12, 1835–1853 CrossRef CAS PubMed.
- S. P. Lacour, G. Courtine and J. Guck, Nat. Rev. Mater., 2016, 1, 1–14 Search PubMed.
- S. Alsenan, I. Al-Turaiki and A. Hafez, Comput. Biol. Chem., 2020, 89, 107377 CrossRef CAS PubMed.
- A. V. Singh, R.-S. Maharjan, A. Kanase, K. Siewert, D. Rosenkranz, R. Singh, P. Laux and A. Luch, ACS Appl. Mater. Interfaces, 2020, 13, 1943–1955 CrossRef PubMed.
- A. Hasanzadeh, M. R. Hamblin, J. Kiani, H. Noori, J. M. Hardie, M. Karimi and H. Shafiee, Nano Today, 2022, 47, 101665 CrossRef CAS PubMed.
- A. Schwarzer, S. R. Talbot, A. Selich, M. Morgan, J. W. Schott, O. Dittrich-Breiholz, A. L. Bastone, B. Weigel, T. C. Ha and V. Dziadek, Mol. Ther., 2021, 29, 3383–3397 CrossRef CAS PubMed.
- S. Berg, D. Kutra, T. Kroeger, C. N. Straehle, B. X. Kausler, C. Haubold, M. Schiegg, J. Ales, T. Beier and M. Rudy, Nat. Methods, 2019, 16, 1226–1232 CrossRef CAS PubMed.
- W. J. Thrift and R. Ragan, Anal. Chem., 2019, 91, 13337–13342 CrossRef CAS PubMed.
- W. J. Thrift, S. Ronaghi, M. Samad, H. Wei, D. G. Nguyen, A. S. Cabuslay, C. E. Groome, P. J. Santiago, P. Baldi and A. I. Hochbaum, ACS Nano, 2020, 14, 15336–15348 CrossRef CAS PubMed.
- A. Mukhopadhyay, J. Sumner, L. H. Ling, R. H. C. Quek, A. T. H. Tan, G. G. Teng, S. K. Seetharaman, S. P. K. Gollamudi, D. Ho and M. Motani, Int. J. Environ. Health, 2022, 19, 8979 Search PubMed.
- V. Agrahari, V. Agrahari, M.-L. Chou, C. H. Chew, J. Noll and T. Burnouf, Biomaterials, 2020, 260, 120163 CrossRef CAS PubMed.
- W. Chen, H. Zhou, B. Zhang, Q. Cao, B. Wang and X. Ma, Adv. Funct. Mater., 2022, 32, 2110625 CrossRef CAS.
-
M. Grammatikopoulou, L. Zhang and G. Z. Yang, Depth Estimation of Optically Transparent Microrobots Using Convolutional and Recurrent Neural Networks, 2018 IEEE/RSJ International Conference on Intelligent Robots and Systems (IROS), Madrid, Spain, 2018, pp. 4895–4900, DOI:10.1109/IROS.2018.8593776.
- M. Schrage, M. Medany and D. Ahmed, Adv. Mater. Technol., 2023, 2201702 CrossRef CAS.
- Q. Wang, J. Zhang, J. Yu, J. Lang, Z. Lyu, Y. Chen and L. Zhang, ACS Nano, 2023, 17, 13081–13109 CrossRef CAS PubMed.
- M. Busek, A. Aizenshtadt, T. Koch, A. Frank, L. Delon, M. A. Martinez, A. Golovin, C. Dumas, J. Stokowiec and S. Gruenzner, Lab Chip, 2023, 23, 591–608 RSC.
- Y. Leng, X. Li, F. Zheng, H. Liu, C. Wang, X. Wang, Y. Liao, J. Liu, K. Meng and J. Yu, Adv. Mater., 2023, 2211059 CrossRef CAS PubMed.
- A. V. Singh, Z. Hosseinidoust, B.-W. Park, O. Yasa and M. Sitti, ACS Nano, 2017, 11, 9759–9769 CrossRef CAS PubMed.
- S. Jeon, S. Kim, S. Ha, S. Lee, E. Kim, S. Y. Kim, S. H. Park, J. H. Jeon, S. W. Kim and C. Moon, Sci. Robot., 2019, 4, eaav4317 CrossRef PubMed.
- L. Sun, Z. Chen, F. Bian and Y. Zhao, Adv. Funct. Mater., 2020, 30, 1907820 CrossRef CAS.
- R. Raman, C. Cvetkovic, S. G. Uzel, R. J. Platt, P. Sengupta, R. D. Kamm and R. Bashir, Proc. Natl. Acad. Sci. U. S. A., 2016, 113, 3497–3502 CrossRef CAS PubMed.
- S. Deng, C. Li, J. Cao, Z. Cui, J. Du, Z. Fu, H. Yang and P. Chen, Theranostics, 2023, 13, 4526 CrossRef CAS PubMed.
- A. Mencattini, F. Mattei, G. Schiavoni, A. Gerardino, L. Businaro, C. Di Natale and E. Martinelli, Front. Pharmacol., 2019, 10, 100 CrossRef CAS PubMed.
- M. C. Comes, J. Filippi, A. Mencattini, P. Casti, G. Cerrato, A. Sauvat, E. Vacchelli, A. De Ninno, D. Di Giuseppe and M. D'Orazio, Neural. Comput. Appl., 2021, 33, 3671–3689 CrossRef.
- M. Pérez-Aliacar, M. H. Doweidar, M. Doblaré and J. Ayensa-Jiménez, Comput. Biol. Med., 2021, 135, 104547 CrossRef PubMed.
- L. H. Chong, T. Ching, H. J. Farm, G. Grenci, K.-H. Chiam and Y.-C. Toh, Lab Chip, 2022, 22, 1890–1904 RSC.
- E. Gultepe, J. S. Randhawa, S. Kadam, S. Yamanaka, F. M. Selaru, E. J. Shin, A. N. Kalloo and D. H. Gracias, Adv. Mater., 2013, 25, 514–519 CrossRef CAS PubMed.
- P. Actis, M. M. Maalouf, H. J. Kim, A. Lohith, B. Vilozny, R. A. Seger and N. Pourmand, ACS Nano, 2014, 8, 546–553 CrossRef CAS PubMed.
- A. Shakoor, W. Gao, L. Zhao, Z. Jiang and D. Sun, Microsyst. Nanoeng., 2022, 8, 47 CrossRef CAS PubMed.
- J. Li, B. Esteban-Fernández de Ávila, W. Gao, L. Zhang and J. Wang, Sci. Robot., 2017, 2, eaam6431 CrossRef PubMed.
- S. Shivalkar, A. Roy, S. Chaudhary, S. K. Samanta, P. Chowdhary and A. K. Sahoo, Biomed. Mater., 2023, 18, 062003 CrossRef PubMed.
- G. Go, A. Yoo, K. T. Nguyen, M. Nan, B. A. Darmawan, S. Zheng, B. Kang, C.-S. Kim, D. Bang and S. Lee, Sci. Adv., 2022, 8, eabq8545 CrossRef CAS PubMed.
- S. Cao, J. Shao, H. Wu, S. Song, M. T. De Martino, I. A. Pijpers, H. Friedrich, L. K. Abdelmohsen, D. S. Williams and J. C. van Hest, Nat. Commun., 2021, 12, 2077 CrossRef CAS PubMed.
- Z. Lin, C. Gao, D. Wang and Q. He, Angew. Chem., Int. Ed., 2021, 60, 8750–8754 CrossRef CAS PubMed.
- D. Zhang, F. P.-W. Lo, J.-Q. Zheng, W. Bai, G.-Z. Yang and B. Lo, ACS Photonics, 2020, 7, 3003–3014 CrossRef CAS.
- M. Xie, A. Shakoor, Y. Shen, J. K. Mills and D. Sun, IEEE Trans. Biomed. Eng., 2018, 1 Search PubMed.
- Y. Yang, M. A. Bevan and B. Li, Adv. Intell. Syst., 2020, 2, 1900106 CrossRef.
- M. Javaid, A. Haleem, R. P. Singh and R. Suman, Biomed. Technol., 2023, 1, 39–47 CrossRef.
- M. Ussia, M. Urso, S. Kment, T. Fialova, K. Klima, K. Dolezelikova and M. Pumera, Small, 2022, 18, 2200708 CrossRef CAS PubMed.
- C. C. Mayorga-Martinez, J. Zelenka, K. Klima, P. Mayorga-Burrezo, L. Hoang, T. Ruml and M. Pumera, ACS Nano, 2022, 16, 8694–8703 CrossRef CAS PubMed.
- C. C. Mayorga-Martinez, J. Zelenka, K. Klima, M. Kubanova, T. Ruml and M. Pumera, Adv. Mater., 2023, 2300191 CrossRef CAS PubMed.
- Y. Dong, L. Wang, K. Yuan, F. Ji, J. Gao, Z. Zhang, X. Du, Y. Tian, Q. Wang and L. Zhang, ACS Nano, 2021, 15, 5056–5067 CrossRef CAS PubMed.
- G. Chatzipirpiridis, O. Ergeneman, J. Pokki, F. Ullrich, S. Fusco, J. A. Ortega, K. M. Sivaraman, B. J. Nelson and S. Pané, Adv. Healthcare Mater., 2015, 4, 209–214 CrossRef CAS PubMed.
- S. Adel, A. Zaher, N. El Harouni, A. Venugopal, P. Premjani and N. Vaid, BioMed Res. Int., 2021, 2021, 1–16 CrossRef PubMed.
- B. D. Bhat, S. Bhandary, R. Naik and D. Shetty, Journal of Dental Research and Review, 2017, 4, 67–68 CrossRef.
- C. Mo, G. Li and X. Bian, Front. Phys., 2023, 11, 1279883 CrossRef.
|
This journal is © The Royal Society of Chemistry 2024 |