Interface design of SARS-CoV-2 symmetrical nsp7 dimer and machine learning-guided nsp7 sequence prediction reveals physicochemical properties and hotspots for nsp7 stability, adaptation, and therapeutic design†
Received
7th March 2024
, Accepted 15th April 2024
First published on 16th April 2024
Abstract
The COVID-19 pandemic, driven by severe acute respiratory syndrome coronavirus 2 (SARS-CoV-2), necessitates a profound understanding of the virus and its lifecycle. As an RNA virus with high mutation rates, SARS-CoV-2 exhibits genetic variability leading to the emergence of variants with potential implications. Among its key proteins, the RNA-dependent RNA polymerase (RdRp) is pivotal for viral replication. Notably, RdRp forms dimers via non-structural protein (nsp) subunits, particularly nsp7, crucial for efficient viral RNA copying. Similar to the main protease (Mpro) of SARS-CoV-2, there is a possibility that the nsp7 might also undergo mutational selection events to generate more stable and adaptable versions of nsp7 dimer during virus evolution. However, efforts to obtain such cohesive and comprehensive information are lacking. To address this, we performed this study focused on deciphering the molecular intricacies of nsp7 dimerization using a multifaceted approach. Leveraging computational protein design (CPD), machine learning (ML), AlphaFold v2.0-based structural analysis, and several related computational approaches, we aimed to identify critical residues and mutations influencing nsp7 dimer stability and adaptation. Our methodology involved identifying potential hotspot residues within the dimeric nsp7 interface using an interface-based CPD approach. Through Rosetta-based symmetrical protein design, we designed and modulated nsp7 dimerization, considering selected interface residues. Analysis of physicochemical features revealed acceptable structural changes and several structural and residue-specific insights emphasizing the intricate nature of such protein–protein complexes. Our ML models, particularly the random forest regressor (RFR), accurately predicted binding affinities and ML-guided sequence predictions corroborated CPD findings, elucidating potential nsp7 mutations and their impact on binding affinity. Validation against clinical sequencing data demonstrated the predictive accuracy of our approach. Moreover, AlphaFold v2.0 structural analyses validated optimal dimeric configurations of affinity-enhancing designs, affirming methodological precision. Affinity-enhancing designs exhibited favourable energetics and higher binding affinity as compared to their counterparts. The obtained physicochemical properties, molecular interactions, and sequence predictions advance our understanding of SARS-CoV-2 evolution and inform potential avenues for therapeutic intervention against COVID-19.
Introduction
The ongoing COVID-19 pandemic, caused by SARS-CoV-2, has posed an exemplary worldwide dilemma, demanding a comprehensive understanding of the virus, its components, and its life cycle for effective prevention and control.1,2 SARS-CoV-2, belonging to the Coronaviridae family, is an enveloped, positive-sense, single-stranded RNA virus with the largest genome among known RNA viruses.3 The genome of SARS-CoV-2 is approximately 30 kilobases in length and encodes numerous proteins involved in viral replication, transcription, host interaction, and immune evasion.4 Key structural proteins include spike (S), envelope (E), membrane (M), and nucleocapsid (N) proteins.5 Several non-structural proteins also play key roles in regulating the immune system and viral replication.6 In addition to the structural components, non-structural proteins, and accessory proteins, SARS-CoV-2 has a positive-sense, single-stranded RNA genome, making it unique among coronaviruses.7
SARS-CoV-2, like all RNA viruses, exhibits genetic variability due to its high mutation rate.8 This genetic diversity has given rise to various SARS-CoV-2 lineages and variants, some of which have garnered substantial attention due to their potential impact on transmissibility, severity, vaccine effectiveness, and diagnostic accuracy.9 Variants such as alpha, beta, gamma, delta, omicron and recently identified sub-variants like JN.1 have specific mutations in the spike protein and other regions of the genome, which have raised concerns about their ability to evade immunity, influence viral replication, and alter the course of the pandemic.10 These variants mostly emerged from mutations in the intracellular proteins of SARS-CoV-2, and to fully grasp the impact of these variants, it is crucial to investigate the molecular mechanisms that underpin viral replication, immune evasion, and pathogenesis.11 This examination is particularly relevant for intracellular proteins, as they play a pivotal role in the lifecycle of the virus and its interactions with host cells.12,13
Among the intracellular proteins, RdRp is a key player in the viral life cycle, which serves as the primary enzyme responsible for replicating the viral genome.14 It catalyzes the formation of negative-sense RNA strands from positive-sense RNA templates, a critical step in viral replication.15 The high fidelity and activity of RdRp are critical for ensuring viral genome integrity and efficient replication.16 The molecular intricacies of RdRp and the dimerization of non-structural protein (nsp) subunits hold the key to deciphering viral replication mechanisms and exploring therapeutic interventions.17 One of the intriguing aspects of RdRp is its ability to form dimers (via its nsp7 subunits), which is essential for its functionality in SARS-CoV-2.18 RdRp dimerization is a complex and tightly regulated process that involves interactions with nsp subunits, particularly nsp7 and nsp8.19 The dimeric RdRp, in its antiparallel arrangement, plays a crucial role in governing the processivity of the enzyme and efficiency in copying the viral RNA.20 While much research has focused on RdRp as an individual entity and targeting it, the molecular mechanisms and structural basis of RdRp dimerization, specifically the nsp subunits, are an emerging area of interest, holding the potential to unveil new antiviral strategies and therapeutic targets.14
In our recent studies, we harnessed the power of high-throughput protein design methodologies to pinpoint critical residues and mutations within intracellular proteins including main protease (Mpro), RdRp, and spike protein receptor binding domain (RBD) of SARS-CoV-2, which could make the virus more tolerant and adaptable to antiviral drugs such as remdesivir, molnupiravir, favipiravir, nirmatrelvir and bebtelovimab.21–26 While conducting this research, we found that mutational and residue-specific changes can induce relative stabilities and adaptability of the RdRp, for instance, the nsp7 by forming symmetrical dimers.20 This realization prompted us to perform an extensive protein design and ML-based endeavour, which was further complemented by a comprehensive multi-parametric analysis.27 To obtain these insights for the dimeric RdRp formed via the nsp7 subunits, we employed a unique protein design approach, followed by evaluation of various physicochemical features, comparative analysis against clinical sequencing data, ML-guided binding affinity, and nsp7 sequence prediction, and rigorous structural validation through the utilization of AlphaFold v2.0.28 This concerted effort led us to discern the specific hotspot residues within the dimeric RdRp of the virus that display a heightened proclivity towards stability, adaptation, and improved fitness.20
Materials and methods
Identification of interface residues in nsp7
The electron microscopic structure of the dimeric form of SARS-CoV-2 RdRp in complex with nsp7
:
nsp8
:
nsp12
:
primer dsRNA (PDB ID: 7OYG) was utilized to obtain detailed information on nsp7–nsp7 dimerization and its interface residues. The RdRp dimer structure in its antiparallel arrangement, possesses one copy of nsp8 each and dimerizes via the nsp7 subunits.20 This analysis identified 30 nsp7 residues that are part of the dimeric interface and helped in its dimerization. Following this, the structure was used for the interface-based symmetrical protein design.
Interface-based symmetrical dimer design of nsp7
The structure was first energy-minimized and refined using Rosetta's relax protocol. Multiple structures were generated during this step to identify the lowest-energy structure. A symmetry definition file was created using the lowest-energy dimeric structure of nsp7. The relaxed structure was then used as an input for designing the nsp7 interface residues involved in the dimerization of nsp7.29–31 Incorporating backbone flexibility, the Rosetta macromolecular modeling suite was applied to redesign the interface residues of the nsp7 dimer. In this step, the 30 interfacial residues of nsp7 dimer were designed with selected single nucleotide polymorphisms (SNPs).32 A modified Rosetta script with backbone flexibility of nsp7 was considered while designing in addition to the Monte-Carlo simulated annealing and the Rosetta all-atom force field model. The dimeric interface of nsp7 residues was studied for potential hotspots that could change to allow for a stronger and more stable nsp7 dimer and their adaptation. From the design experiment, a total of 100
000 dimeric designs of nsp7 were generated. The Rosetta total score, root mean square deviation (RMSD), Rosetta interface ΔΔG (illustrating the binding affinities between the two designed symmetrical nsp7 monomers), and the sequence identities (%) of the designs from the native nsp7 were examined to comprehend the physicochemical features, the impact of mutations on nsp7 dimerization potential, and the overall stability of RdRp.32,33 The superpose (CCP4: Supported Program), MayaChemTools, and ViroBLAST packages were used to calculate RMSD, FASTA sequence of nsp7 designs, and sequence identities of all 100
000 designs against the native nsp7, respectively.
Validation of the interface-based design and ML-based results
To assess the accuracy of our design strategy, the favourable mutations (resulting in enhanced binding affinity) obtained from Rosetta were compared with the already reported SARS-CoV-2 RdRp–nsp7 mutations reported in the CoV-GLUE database.34,35 The frequency of mutations (at different min. proportion values of 0, 0.0001, 0.001, 0.01, 0.1, 0.25 0.5, 0.75, 0.95, and 1.0) were compared with nsp7 designs to assess the precision of our design method. Heat maps were generated using the heat mapper server,36 which shows the mutations at the interface and their frequencies obtained from CoV-GLUE.
To validate our ML-based predictions, an nsp7 mutant, S25L, a known stabilizer of the supercomplex, was also considered. To assess the impact of the mutation on protein stability, the MUpro server was utilized, which evaluates protein stability through three metrics: ΔΔG (using a support vector machine), confidence score (using a support vector machine), and confidence score (using a neural network), where scores can range from −1 to 1.37 In this case, a score less than zero denotes a decrease in protein stability caused by the mutation, whereas a score more than zero denotes an increase in protein stability. Scores close to 1 represent higher confidence in prediction.
Mutational landscape profiles of affinity-enhancing and affinity-reducing designs
The mutational landscape profiles of the designed amino acid residues in the top-scored affinity-enhancing and affinity-reducing designs were extracted and plotted using WebLogo.38 This enabled us to obtain and visually represent the types and frequencies of the nsp7-designed amino acid residues. Further, this information helped us to analyze the designed nsp7 protein mutants, their effect on stability, and the dimerization of nsp7 and RdRp.
ML-based approaches to predict the binding affinities between the monomers in the nsp7 designs
To extrapolate trends from the CPD-generated dataset, different ML algorithms were employed. Further, to overcome the sole reliance on structural information, we leveraged ML methodologies to predict nsp7 dimer stabilizing mutations by computing the binding affinities between the nsp7 monomers. Initially, the CPD-generated dataset was utilized, and features derived from CPD—such as Rosetta total score, ΔΔG (binding affinity), sequence identity, mutation positions, and mutated amino acids—were selected. Post-data preprocessing, which involved one hot encoding for converting categorical amino acid columns into numeric ones, along with data visualization and feature engineering, the dataset was split into training (80%, 80
000 data points) and test sets (20%, 20
000 data points). The ΔΔG and Rosetta total scores were designated as separate target attributes for subsequent model construction. Following this, RFR, XGBoost Regressor, and decision tree regressor were utilized to evaluate their performances. The RFR (being the top-performing algorithm in terms of accuracy as obtained in our work and assessed through the R2 score and mean absolute error) was subsequently used for model construction and further analysis. Optimal parameters for the RFR were obtained using “GridSearchCV.” To gauge the correlation between actual (CPD-derived) and predicted (ML-derived) binding affinity, the “cross_val_score” function was used with a CV value of 10, representing the mean of ten random cross-validation observations. The R2 score was then utilized to calculate the correlation between actual and predicted binding affinity, where a score of 1 signifies perfect predictions and 0 indicates imperfect predictions.
Here,
where
yi are the actual target values,
yj are the predicted target values, and
yk are the mean values of actual target values.
ML-based approach for predicting nsp7 sequences that enhance or reduce the binding affinity between nsp7 monomers
After utilizing the CPD results to predict affinity, we adopted a reverse approach to predict nsp7 sequences that may enhance or reduce the binding affinity between the nsp7 monomers based on affinity values generated using the CPD approach. To obtain the nsp7 sequences, ‘multiclass-multioutput classification’ was utilized, which is a classification task that assigns each sample a set of non-binary properties. Thus, in this case, both the number of properties (positions) and the number of classes (sampled amino acids) per property exceed 2. In the multiclass-multioutput classification model, the RFR was utilized. Given the 30 specific mutation positions for which CPD data were generated, our model encompassed 30 targets. Each target involved various classifications of amino acids that potentially serve as mutations of native residues. The input features for this prediction task were the Rosetta total score or ΔΔG (binding affinity). Based on these features, mutations and eventually the nsp7 sequences at 30 different positions were predicted, thereby generating the diverse sequences.
Intermolecular interactions between the top-scored affinity-enhancing and affinity-reducing nsp7 designs
Arpeggio and PRODIGY were utilized to calculate the intermolecular interactions between the top-scored affinity-enhancing and affinity-reducing nsp7 designs.39,40 Various types of interactions and energies were obtained from this analysis, indicating how they modulate the strength and binding of the nsp7 dimers.
Structure prediction and modeling of the affinity-enhancing and affinity-reducing nsp7 designs using AlphaFold v2.0
To assess the performance of the ML-based predictions, the sequences predicted to enhance and reduce the binding affinity by the ML model were used as input for the structure prediction program AlphaFold v2.0.28 Specifically, the designed symmetric nsp7 of RdRp intended for affinity enhancement and reduction as predicted from the ML-based model was subjected to structure prediction (in their dimeric state) using the run_docker.py python script. Each affinity-enhancing and affinity-reducing sequence yielded five models, and the top-ranked structure from each of them was subjected to further analysis based on confidence and the predicted local distance difference test (pLDDT) scores.
Normal mode analysis of the dimeric structures of wild-type nsp7, affinity-enhancing, and affinity-reducing designs
Normal mode analysis (NMA) was performed on the dimeric configurations of wild-type nsp7, as well as affinity-enhancing and affinity-reducing designs, employing the iMODS server.41 This analysis aimed to discern internal coordinates, collective protein motions, and possible conformational alterations, thereby aiding in the evaluation of protein stability. Subsequently, a comprehensive investigation into protein dynamics was undertaken, encompassing the computation of main-chain deformability fluctuation maps, eigenvalues, and correlation matrices. Here, the elastic network model (ENM) was also employed to elucidate the stability of the protein.
Results
Analysis of dimeric nsp7 interface residues for design
By examining the electron microscopic structure of the dimeric form of SARS-CoV-2 RdRp complexed with nsp7
:
nsp8
:
nsp12 with dsRNA primers, specific amino acid residues involved in the dimeric interface of nsp7 were identified (Fig. 1A and B). A total of 30 residues within the dimeric nsp7 interface were identified for further analysis (Fig. 1C). These residues were subjected to interface-based symmetrical protein design by sampling with specific SNPs (Table 1).
 |
| Fig. 1 Structure of the dimeric form of the SARS-CoV-2 RNA-dependent RNA polymerase (RdRp) complex. (A) The cryo-EM structure of the dimeric form of SARS-CoV-2 RdRp in complex with nsp12 : nsp7 : nsp8 : dsRNA and zinc ion is shown, where each subunit is shown as a cartoon in different colours. (B) and (C) The dimeric structure of the nsp7 subunit is shown as a cartoon and the interactions formed by the interface residues are shown and labelled as sticks (with one-letter amino acid codes). The yellow colour dotted line represents the polar contacts between and within the chains. | |
Table 1 The nsp7 interface residues that are designed with corresponding SNPs of the native nsp7 sequence. A limited number of amino acids for each position were sampled in the design experiments because they are more likely to evolve naturally than the others
S. no. |
Designed native nsp7 residues |
Sampled SNPs in designs |
S. no. |
Designed native nsp7 residues |
Sampled SNPs in designs |
1 |
Ser1 |
ACLFPTWY |
16 |
Ser25 |
ACLFPTWY |
2 |
Lys2 |
RNQEIMT |
17 |
Ser26 |
ACLFPTWY |
3 |
Ser4 |
ACLFPTWY |
18 |
Lys27 |
RNQEIMT |
4 |
Asp5 |
ANEGHYV |
19 |
Trp29 |
RCGLS |
5 |
Lys7 |
RNQEIMT |
20 |
Val33 |
ADEGILMF |
6 |
Cys8 |
RGFSWY |
21 |
His36 |
RNDQLPY |
7 |
Thr9 |
ARNIKMPS |
22 |
Asn37 |
DHIKSTY |
8 |
Val11 |
ADEGILMF |
23 |
Leu40 |
RQHIFPSWV |
9 |
Val12 |
ADEGILMF |
24 |
Phe49 |
CILSYV |
10 |
Leu13 |
RQHIFPSWV |
25 |
Glu50 |
ADQGKV |
11 |
Leu14 |
RQHIFPSWV |
26 |
Met52 |
RILKTV |
12 |
Ser15 |
ACLFPTWY |
27 |
Val53 |
ADEGILMF |
13 |
Gln18 |
REHLKP |
28 |
Leu56 |
RQHIFPSWV |
14 |
Glu23 |
ADQGKV |
29 |
Leu59 |
RQHIFPSWV |
15 |
Ser24 |
ACLFPTWY |
30 |
Leu60 |
RQHIFPSWV |
Interface-based design of symmetrical nsp7 dimer and analysis of various physicochemical features
A Rosetta-based symmetrical protein design was carried out to generate dimeric nsp7 designs of the RdRp. The purpose of this design strategy was to identify the hotspot residues of nsp7 important for modulating the dimerization and the physicochemical properties and to comprehend the stability and adaptation of nsp7 dimer in the RdRp. For design, 30 residues of dimeric nsp7 were selected, each of which was mutated and sampled with other amino acids that naturally occur more frequently during the evolution of proteins (Table 1).
First, we analyzed the Rosetta total score vs. the RMSDs of the designs as compared to the native nsp7 dimer. It was found that, in the distribution of the Rosetta total score, RMSD ranged from 0.66 to 3.33 Å. This demonstrated that over half of the designs retained RMSDs in the range of 1 Å to 2 Å (Fig. 2A). It was observed that, in such intricate small protein–protein complexes, acceptable structural changes can occur during the design of the 30 residues of the nsp7 and with the introduction of mutations (Fig. 2A). Here, the weighted sum of various energy terms, such as van der Waals interactions, electrostatics, and other statistical variables, is represented by the Rosetta total score. Furthermore, we conducted a control run where only repacking was performed on the 30 residues of nsp7. As a result, we were able to distinguish between affinity-enhancing and affinity-reducing designs. Due to the occurrence of a few unfavourable mutations, some affinity-reducing designs had RMSDs > 3 Å (Fig. 2A). Second, we compared the Rosetta total score with the sequence identities of all the designs. It was found that all the designs exhibited sequence identities in the range of 50.94–66.66% compared to the native nsp7 (Fig. 2B). Even though merely 25 to 30 residues were designed that form the dimeric interface, only a small number of hotspot residues were susceptible to sequence alterations (Fig. 2B).
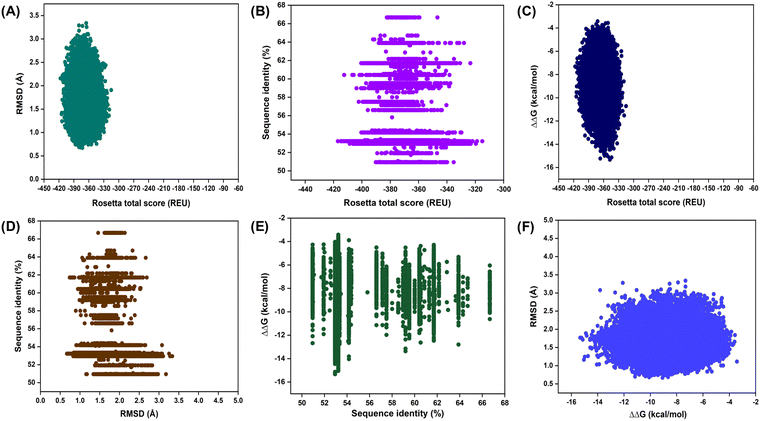 |
| Fig. 2 Physicochemical properties derived from the interface-based symmetrical dimer design of nsp7. (A) Rosetta total score vs. RMSD of all the obtained designs of the dimeric nsp7 is displayed. (B) Rosetta total score vs. percentage sequence identity of all the designs of the dimeric nsp7 subunit is shown. (C) Rosetta total score vs. ΔΔG of all the nsp7 designs is depicted. (D) RMSD vs. percentage sequence identity of nsp7 designs is displayed. (E) Percentage sequence identity vs. ΔΔG of all nsp7 designs is shown. (F) ΔΔG vs. RMSD of all the nsp7 designs is displayed. Here, the designed nsp7 dimers revealed that over half of the designs maintained structural changes within the 1–2 Å RMSD range. Sequence identities ranged from 50.94% to 66.66%, and affinity-enhancing designs exhibited lower Rosetta total scores and higher binding affinities. The RMSDs of these designs correlated with both sequence identity and ΔΔG, suggesting that hotspot residues are crucial. Affinity-enhancing designs predominantly displayed RMSDs between 1 and 2 Å, indicating stabilized interactions due to favourable mutations. | |
Third, the designs were analyzed for ΔΔG vs. the Rosetta total score. It was observed that the affinity-enhancing designs showed higher binding affinity and lower Rosetta total scores as compared to the affinity-reducing designs (Fig. 2C). This implies that favourable mutations positively influence the energetics, binding interface, and Rosetta total scores in the affinity-enhancing designs. Next, the RMSDs of the designs were plotted against the percentage sequence identity scores. It was found that, in the designs with the varying RMSDs from 0.66 to 3.33 Å, the percentage sequence identity also varied from 50.94% to 66.66% (Fig. 2D). Next, the ΔΔG values of the generated designs were compared with their sequence identities, which indicated that designs with higher binding affinity have a relatively higher percentage sequence identity, indicating that only a few of the 30 interfacial residues designed for nsp7 are sufficient and probably the hotspot residues susceptible to mutation (Fig. 2E). Compared to the native nsp7, the CPD designs retained ΔΔG in the range of −3.42 to −15.32 kcal mol−1 and sequence identity of 50.94% to 66.66% (Fig. 2E). Finally, we evaluated the RMSD vs. ΔΔG for all the designs. We observed that the majority of the affinity-enhancing designs displayed RMSDs between 1.0 and 2.0 Å, suggesting that the associated mutations stabilized the interactions (Fig. 2F).
Validation of the interface-based symmetrical nsp7 design approach
To examine and confirm the accuracy of our symmetric dimer design approach, a computational control analysis was carried out, where the designed mutations that enhance the binding affinity, stability, and hence the dimerization ability of the nsp7 were compared to the clinically reported nsp7 mutations of SARS-CoV-2 in the CoV-GLUE database. A comparison is conducted between the prevalence of mutations obtained from the patients and that of the nsp7 designs to reflect upon the design accuracy.
Based on the comparison, it was found that 62 out of the 235 mutations were positively selected and likely to result in stable dimers, yielding a 26.38% correlation and matching with the sequencing data (nsp7 sequences were retrieved from CoV-GLUE on 02 April 2024) (Fig. 3). Several high-frequency mutations, including K2T, V11L, and V11E, were already found to be favourable in our design computations (Fig. 3). Moreover, using the MinProp cut-off of 0.0001, one mutation out of 7 was predicted to be adaptive and positively selected to form stable nsp7 dimers in our design computations, thereby resulting in ∼14.28% correlation and matching with the clinically available data (Fig. S1, ESI†). As more sequencing data become available, other mutations from different populations will likely appear in addition to those sampled from our designs, thereby increasing the correlation. Therefore, this control investigation supported our design strategy and we highlighted the mutations that might serve an essential part in the adaptation and generation of high-affinity and stable nsp7 dimers.
 |
| Fig. 3 Heat map displaying nsp7 mutations and their frequencies at the dimeric nsp7 interface, derived from the CoV-GLUE database. The SARS-CoV-2 nsp7 mutations derived from the CoV-GLUE database are shown. In this illustration, the frequencies of the mutations among COVID-19 patients ranging from low to high numbers are represented using green-to-white-to-pink colours. The mutations that strengthened the dimeric nsp7 and that are derived from the CPD approach are represented with a box adjacent to the mutants. 62 mutations out of 235 were predicted to be adaptive and positively selected to form stable nsp7 dimers in our design computations, thereby resulting in ∼26.38% correlation and matching with the clinically available data. | |
Subsequently, we conducted a validation of our ML-based approach and its outcomes through a case study involving the S25L mutant of nsp7, known for its purported role in strengthening the RdRp–nsp7–nsp8 supercomplex. Previous studies have highlighted the significance of Ser25 and Ser26 residues in the nsp7 subunit for stabilizing the supercomplex, specifically the S25L mutation, which enhances surface complementarity by 10%, thus contributing to supercomplex stability.42 Our ML-based predictions also identified an nsp7 sequence harbouring the S25L mutation, indicating potential enhanced stability for the nsp7 dimer. To independently verify this prediction, we employed the MUpro server,37 assessing protein stability through three metrics: ΔΔG (support vector machine), confidence score (support vector machine), and confidence score (neural network). The results indicated ΔΔG and confidence score (from SVM and neural network) to be 0.46, 0.88, and 0.83, respectively, thereby consistently supporting our ML-based prediction, and confirming that the S25L mutation augments protein stability (Table 2).
Table 2 The effect of the S25L mutation on the stability of nsp7 protein as obtained from MUpro. The sequences are shown below and the native to mutated residues (S25 and L25) are underlined and shown in bold, respectively
S. no. |
nsp7 sequence |
Single point mutation |
ΔΔG (using SVM) |
Confidence score (using SVM) |
Confidence score (using neural network) |
Protein stability |
1 |
SKMSDVKCTSVVLLSVLQQLRVES SKLWAQCVQLHNDILLAKDTTEAFEKMVSLLSVLSM |
No mutation |
— |
— |
— |
— |
2 |
STMAEVRRASIESITVLLQLRVKT PELGAQCLQLNIDIMLAKDTTEACAKTLSLVSVMVSM |
28 mutations + S25L mutation |
0.46 |
0.88 |
0.83 |
Increase protein stability |
Analysis of the mutational landscape profile and sequence variation in nsp7 dimeric designs
To gain further insights into the key differences in the types and frequencies of residues between affinity-enhancing and affinity-reducing designs, we compared the designs with each other. We analyzed the mutational landscape profiles of the designed amino acids and plotted their types and frequencies for the affinity-enhancing and affinity-reducing designs (hundred from each). The top-scored affinity-enhancing and affinity-reducing designs were analyzed based on the ΔΔG values and compared for their differences in resulting mutated amino acids and hence their sequence variations (Fig. 4). This analysis showed that certain nsp7 residues such as 4(Asp5), 8(Val11), 12(Ser15), 14(Glu23), 16(Ser25), 17(Ser26), 19(Trp29), 20(Val33), 21(His36), 23(Leu40), 29(Leu59), and 30(Leu60) acquired mutations with a variety of different residues (Fig. 4). Additionally, a few residues were sampled with nearly similar residues, including those at positions 11(Leu14) and 25(Glu50) (Fig. 4). Moreover, all other residues were sampled nearly identical in both affinity-enhancing and affinity-reducing designs (Fig. 4). Through these analyses, the nsp7 hotspot residues that have the propensity to produce stable dimeric nsp7 were identified.
 |
| Fig. 4 Sequence logos illustrating the type and occurrence of sampled nsp7 interface residues. The sequence logo of the 100 top-scored affinity-enhancing designs and affinity-reducing nsp7 designs are shown, where native nsp7 residues are mentioned in the bottom section. In each figure, the nsp7 residues are shown on the X-axis and the Y-axis denotes the frequency of occurrence of each amino acid. The height of each symbol represents the relative frequency of a specific amino acid at the given position. The residues with diverse sequence variations between the affinity-enhancing and affinity-reducing designs are shown using red arrows and those exhibiting nearly similar residues are shown using purple arrows. | |
ML-based approaches for predicting binding affinities between the monomers of the nsp7 designs
One of the primary objectives of this study was to employ a robust ML-based approach to predict the binding affinity between the nsp7 monomers in the dimeric nsp7 designs of SARS-CoV-2. This approach aimed to enhance our understanding of favourable mutations at the nsp7 dimeric interface, contributing to the stabilization of nsp7. The predictions were evaluated against CPD results, assessing their accuracy and efficiency compared to standalone CPD methods. Among the three algorithms tested, the RFR was selected due to its superior accuracy (measured by the R2 score and mean absolute error). The analysis involved features like the Rosetta total score, ΔΔG, sequence identity, mutation positions, and altered amino acids. The RFR prediction model exhibited a high correlation compared to other models. In the distribution plot for the Rosetta total score and ΔΔG, it was observed that the majority of designs clustered between Rosetta total scores of −360 and −370 REU and −7 and −9 kcal mol−1 ΔΔG, respectively (Fig. 5A and B). The obtained R2 score of 0.80 and mean absolute error (MAE) of 3.4 between actual (CPD-derived) and predicted Rosetta total scores, along with an R2 score of 0.70 and a MAE of 0.52 between actual and predicted ΔΔG with 10-fold cross-validation, highlighted the accuracy of our model (Fig. 5C and D). These findings validate the precision and reliability of our predictive model.
 |
| Fig. 5 Assessment of the Rosetta total score and binding affinity correlation between CPD and ML for the nsp7 sequences. (A) and (B) Plots showing the distribution of the Rosetta total score and ΔΔG with their frequency for all the CPD designs, respectively. Most designs retained the Rosetta total score centered around −370 REU and ΔΔG around −8 kcal mol−1, respectively, in these distributions. (C) and (D) ML-predicted Rosetta total score vs. CPD-derived actual Rosetta total score and ML-predicted ΔΔG vs. CPD-derived ΔΔG are shown as scatter plots, respectively. A correlation coefficient of 0.80 and 0.70 (marked by a red colour line) was obtained when taking the Rosetta total score and ΔΔG as targets, respectively. | |
ML-based sequence prediction yielding affinity-enhancing and affinity-reducing nsp7 designs
Next, to predict the nsp7 sequences harbouring mutations and to classify them as affinity-enhancing and affinity-reducing groups, an ML-based approach was utilized that relies on ΔΔG (binding affinities) generated through CPD. The input for this process consisted of the Rosetta total score or ΔΔG, which eventually predicted mutations at 30 distinct positions and subsequently generated the diverse nsp7 sequences (Fig. 6 and Tables S1, S2, ESI†). Our multiclass-multioutput classification model utilized an RFR. The integration of ML and CPD affirmed that affinity-enhancing designs exhibit a higher ΔΔG compared to affinity-reducing designs. The predicted sequences were then juxtaposed with the native nsp7, and the resulting comparisons including ΔΔG, % sequence similarity, and RMSD of affinity-enhancing and affinity-reducing nsp7 designs were derived as shown in Tables 3 and 4.
 |
| Fig. 6 Scheme for ML-based prediction of binding affinity and newer nsp7 sequence variations. A schematic figure showing the ML-based approach and its steps that take various CPD-based features such as the Rosetta total score or ΔΔG (binding affinity) as input for the model building, model assessment, and predicting the nsp7 sequences having mutations and their corresponding ΔΔG. | |
Table 3 ML-predicted nsp7 affinity-enhancing design sequences, their predicted ΔΔG, % sequence similarity, and backbone RMSDs as compared to the native nsp7 AlphaFold v2.0 predicted structure
S. no. |
ML predicted affinity-enhancing design sequences |
ΔΔG (kcal mol−1) |
Sequence similarity (%) |
RMSD (Å) |
1 |
STMAEVQRISFLMFLVLKQLRVDATPELGAQCLQLRIDIQLAKDTTEACQKTLSLVSVSISM |
−14 |
77.4 |
3.0 |
2 |
STMAEVRRASIESITVLLQLRVKTTPELGAQCLQLNIDIMLAKDTTEACAKTLSLVSVMVSM |
−13 |
80.6 |
2.6 |
3 |
SQMAEVIWASFLVSLVLKQLRVDATPELGAQCDQLQKDISLAKDTTEACQKLLSLVSVSVSM |
−13.5 |
77.4 |
4.4 |
4 |
STMAEVRRASEESSTVLRQLRVDTTPELGAQCAQLQKDIQLAKDTTEACQKTLSLISVSFSM |
−12.5 |
72.6 |
2.2 |
Table 4 ML-predicted nsp7 affinity-reducing design sequences, their predicted ΔΔG, % sequence similarity, and backbone RMSDs as compared to the native nsp7 AlphaFold v2.0 predicted structure
S. no. |
ML predicted affinity-reducing design sequences |
ΔΔG (kcal mol−1) |
Sequence similarity (%) |
RMSD (Å) |
1 |
STMTEVIRASIISFLVLLQLRVQATPELLAQCEQLNKDIILAKDTTEASQK |
−4 |
75.8 |
13.7 |
LISLVSVQSSM |
2 |
STMTAVIRASILVFLVLLQLRVDTTPELGAQCLQLNIDIILAKDTTEASQK |
−5 |
77.4 |
13.27 |
LASLVSVQMSM |
3 |
SQMAEVQWASFIIILVLLQLRVKATPELGAQCLQLNKDIILAKDTTEACQ |
−4.5 |
79.0 |
12.6 |
KTLSLSSVSFSM |
4 |
STMTEVIRISILSFLVLKQLRVDTTPELGAQCEQLNKDIVLAKDTTEASQ |
−5.5 |
75.8 |
11.9 |
KTLSLSSVSMSM |
Interactions between the top-scored affinity-enhancing and affinity-reducing nsp7 designs
The intermolecular interactions and energetics between the top-scored affinity-enhancing and affinity-reducing nsp7 designs (as derived from CPD) were evaluated using Arpeggio and PRODIGY. In the design experiments, the affinity-enhancing design exhibited a greater number of interactions (primarily due to van der Waals interactions, hydrogen bonds, hydrophobic interactions, and proximal and carbonyl interactions) as compared to the affinity-reducing design (Table 5). Next, various types of intermolecular contacts, the number of charged and polar, apolar contacts, binding affinity, and disassociation constants were calculated between the top-scored affinity-enhancing and affinity-reducing nsp7 designs using PRODIGY. It was observed that the affinity-enhancing design retained a higher number of intermolecular interactions, a greater number of contacts such as charged–charged contacts, charged–polar contacts, charged–apolar contacts, polar–polar contacts, and therefore significantly higher binding affinity as compared to affinity-reducing design (Table 6).
Table 5 Intermolecular interactions obtained between the nsp7 monomers for the affinity-enhancing and affinity-reducing designs (CPD-derived) using Arpeggio
S. no. |
Types of interactions |
Affinity-enhancing design |
Affinity-reducing design |
1 |
van der Waals interactions |
17 |
5 |
2 |
vdW clash interactions |
14 |
4 |
3 |
Proximal interactions |
557 |
350 |
4 |
Polar contacts |
21 |
8 |
5 |
Weak polar contacts |
19 |
9 |
6 |
Hydrogen bonds |
11 |
2 |
7 |
Weak hydrogen bonds |
12 |
16 |
8 |
Hydrophobic contacts |
56 |
51 |
|
Total number of contacts |
588 |
359 |
Table 6 Intermolecular interactions obtained between the nsp7 monomers for the affinity-enhancing and affinity-reducing designs (CPD-derived) using PRODIGY
S. no. |
Types of contacts |
Affinity-enhancing design |
Affinity-reducing design |
1 |
No. of intermolecular contacts |
53 |
38 |
2 |
No. of charged–charged contacts |
6 |
0 |
3 |
No. of charged–polar contacts |
10 |
2 |
4 |
No. of charged–apolar contacts |
14 |
2 |
5 |
No. of polar–polar contacts |
5 |
3 |
6 |
No. of apolar–polar contacts |
8 |
9 |
7 |
No. of apolar–apolar contacts |
10 |
22 |
8 |
Percentage of apolar NIS residues |
40.00 |
51.92 |
9 |
Percentage of charged NIS residues |
30.00 |
17.31 |
10 |
Predicted binding affinity (kcal mol−1) |
−7.1 |
−5.5 |
11 |
Predicted dissociation constant (M) at 25.0 °C |
5.9 × 10−6 |
9.1 × 10−5 |
Further, intermolecular contacts and binding affinity were obtained for eight different nsp7 sequences (four as affinity-enhancing and four as affinity-reducing) predicted by the ML-based approach. It was found that the affinity-enhancing designs retained a higher number of intermolecular contacts and various types of interactions, thereby resulting in greater binding affinity as compared to affinity-reducing designs (Tables 7 and 8). For instance, while affinity-enhancing designs had binding affinities ranging from −9.7 to −12 kcal mol−1, the affinity-reducing designs could only show binding affinity ranging from −6.5 to −7.7 kcal mol−1, thereby demonstrating a key difference in binding affinity as a result of certain mutations in the hotspots of nsp7.
Table 7 Intermolecular contacts and predicted binding affinities obtained using PRODIGY between nsp7 monomers for the ML-predicted affinity-enhancing nsp7 designs
S. no. |
Types of contacts |
Affinity-enhancing design1 |
Affinity-enhancing design2 |
Affinity-enhancing design3 |
Affinity-enhancing design4 |
1 |
No. of intermolecular contacts |
90 |
69 |
97 |
69 |
2 |
No. of charged–charged contacts |
0 |
0 |
0 |
0 |
3 |
No. of charged–polar contacts |
0 |
8 |
8 |
8 |
4 |
No. of charged–apolar contacts |
8 |
8 |
16 |
10 |
5 |
No. of polar–polar contacts |
0 |
7 |
8 |
0 |
6 |
No. of apolar–polar contacts |
22 |
26 |
35 |
18 |
7 |
No. of apolar–apolar contacts |
60 |
20 |
30 |
33 |
8 |
Percentage of apolar NIS residues |
38.00 |
31.25 |
46.00 |
43.14 |
9 |
Percentage of charged NIS residues |
24.00 |
31.25 |
24.00 |
23.53 |
10 |
Predicted binding affinity (kcal mol−1) |
−11.3 |
−11.1 |
−12.0 |
−9.7 |
11 |
Predicted dissociation constant (M) at 25.0 °C |
5.0 × 10−9 |
7.0 × 10−9 |
1.6 × 10−9 |
7.5 × 10−8 |
Table 8 Intermolecular contacts and predicted binding affinities obtained using PRODIGY between nsp7 monomers for the ML-predicted affinity-reducing nsp7 designs
S. no. |
Types of contacts |
Affinity-reducing design1 |
Affinity-reducing design2 |
Affinity-reducing design3 |
Affinity-reducing design4 |
1 |
No. of intermolecular contacts |
39 |
52 |
46 |
47 |
2 |
No. of charged–charged contacts |
1 |
0 |
0 |
2 |
3 |
No. of charged–polar contacts |
4 |
2 |
2 |
0 |
4 |
No. of charged–apolar contacts |
4 |
8 |
9 |
6 |
5 |
No. of polar–polar contacts |
2 |
7 |
0 |
3 |
6 |
No. of apolar–polar contacts |
9 |
15 |
9 |
12 |
7 |
No. of apolar–apolar contacts |
19 |
20 |
26 |
24 |
8 |
Percentage of apolar NIS residues |
49.07 |
44.44 |
52.73 |
45.63 |
9 |
Percentage of charged NIS residues |
17.59 |
20.37 |
16.36 |
27.18 |
10 |
Predicted binding affinity (kcal mol−1) |
−6.5 |
−7.7 |
−6.8 |
−6.6 |
11 |
Predicted dissociation constant (M) at 25.0 °C |
1.7 × 10−5 |
2.4 × 10−6 |
1.1 × 10−5 |
1.5 × 10−5 |
Structural analysis of the ML-predicted affinity-enhancing and affinity-reducing nsp7 designs
The structural evaluations of the ML-predicted affinity-enhancing and affinity-reducing nsp7 designs were performed using AlphaFold v2.0. After the model building of the sequences, the backbone RMSDs were calculated by superposing the obtained dimeric structures with the wild-type nsp7 dimeric structure. This analysis showed that the affinity-enhancing designs exhibited an optimal dimeric and symmetric nsp7 configuration with RMSDs measuring 2.2 Å, 2.6 Å, 3.0 Å, and 4.4 Å, as illustrated in Fig. 7A–D, respectively. Conversely, the affinity-reducing designs displayed higher RMSDs, ranging from 11.9 Å to 13.7 Å, as depicted in Fig. 7G–J. Notably, in an affinity-enhancing design structure, one of the nsp7 monomeric subunits demonstrated an appropriate folded structure, but failed to maintain the overall dimeric arrangement, resulting in an RMSD of 4.4 Å (Fig. 7D). Although this affinity-enhancing design displayed an RMSD of 4.4 Å, this value pales in comparison to the affinity-reducing designs, which exhibited RMSDs ranging from 11 to 13 Å. This discrepancy underscores the relatively higher RMSD observed for the enhancing design, emphasizing its significance in our analysis. Further, most models exhibited per residue pLDDT scores exceeding 90, indicating a high level of accuracy in the affinity-enhancing models as compared to affinity-reducing models (Fig. 7E, F, K and L). These analyses substantiate the reliability and precision of our dimeric nsp7 design approach.
 |
| Fig. 7 Structure prediction and modeling of the affinity-enhancing and affinity-reducing nsp7 designs using AlphaFold v2.0. In (A)–(D) and (G)–(J), AlphaFold v2.0 derived structures of the affinity-enhancing and affinity-reducing nsp7 designs along with their backbone RMSDs when superposed with native nsp7 dimer are presented, respectively. In (E), (F), (K) and (L), the predicted LDDT (pLDDT) of the top-ranked models for the affinity-enhancing and affinity-reducing designs are displayed, respectively, to assess the quality of the models. | |
Normal mode analysis of the dimeric structures of wild-type nsp7, affinity-enhancing, and affinity-reducing designs
The NMA study, utilizing internal coordinates, unveiled protein mobility, flexibility, collective motion, and potential conformational changes, aiding in assessing protein stability of the dimeric wild-type nsp7, affinity-enhancing, and affinity-reducing designs. Deformability highlights protein flexibility, with peaks indicating flexible regions like hinges. The plot comparing atom index vs. deformability values across normal modes for dimeric wild-type nsp7, affinity-enhancing, and affinity-reducing designs revealed heightened flexibility in the affinity-enhancing design as compared to the wild-type and affinity-reducing nsp7 designs (Fig. 8A). Next, the evaluation of the correlation covariance matrix illustrated variations for the dimeric nsp7 wild-type, as well as for the affinity-enhancing and affinity-reducing designs (Fig. 8B–D). The colours blue, white, and red were used to designate the anticorrelated, uncorrelated, and correlated states of atomic motion, respectively. The black boxes in each plot depicted the localized variation in the correlation between wild-type, affinity-enhancing, and affinity-reducing designs (Fig. 8B–D). Finally, the eigenvalue analysis demonstrated significantly higher eigenvalues in the affinity-enhancing design, suggesting enhanced localized motions such as side-chain vibrations, as compared to the dimeric wild-type nsp7 and affinity-reducing designs (Fig. S2, ESI†).
 |
| Fig. 8 Results obtained from the NMA-based analysis of the dimeric structures of wild-type nsp7, affinity-enhancing, and affinity-reducing designs. Panel (A) presents the deformability plot, depicting the atom index vs. deformability scores across normal modes for the dimeric structures of nsp7 wild-type, as well as for the affinity-enhanced and affinity-reducing designs conducted using iMODS. Panels (B)–(D) display the covariance matrix for the dimeric nsp7 wild-type, affinity-enhancing, and affinity-reducing designs, with red, white, and blue colours indicating correlated, uncorrelated, and anti-correlated motions, respectively. The black boxes in each plot in panels (B)–(D) illustrate the localized differences in correlation among the wild-type, affinity-enhancing, and affinity-reducing designs. | |
Discussion
The ongoing COVID-19 pandemic, caused by SARS-CoV-2, underscores the need for comprehensive insights into the components and life cycle of the virus.43 SARS-CoV-2, a positive-sense, single-stranded RNA virus, exhibits genetic variability, resulting in the development of variants with potential implications for transmissibility, severity, and vaccine efficacy.44 Understanding the molecular mechanisms underlying viral replication, immune evasion, and pathogenesis is crucial, particularly regarding the roles of the intracellular proteins in the lifecycle of the virus.45 The RdRp, a central player in viral replication, catalyzes the synthesis of negative-sense RNA strands crucial for the survival of the virus.46 While the standalone RdRp exhibits minimal activity due to nsp12, the incorporation of its cofactors, nsp7 and nsp8, significantly amplifies its polymerase activity.47,48 Although different viral nsp subunits play a role in the replication and transcription processes, the nsp12–nsp7–nsp8 complex serves as the minimal configuration essential for nucleotide polymerization.19 The dimerization of RdRp via the nsp subunits, notably nsp7, is essential for its functionality, governing viral RNA copying efficiency. The antiparallel arrangement of the RdRp dimer reveals the interaction of the two polymerases through nsp7 subunits, facilitated by the α1 and α3 helices (residues 2–20 and 44–62). Dissociation of nsp8b exposes the dimerization region of nsp7, enabling the formation of an nsp7–nsp7 dimer interface. Previous studies have indicated that mutations in RdRp subunits can impact the stability of both monomers and dimers.20 Therefore, investigating RdRp dimerization mechanisms could offer insights into potential antiviral strategies and therapeutic targets.
In some of our previous studies, we implemented high-throughput protein design methodologies to figure out critical residues and mutations within the intracellular proteins of SARS-CoV-2, including RdRp,33 main protease (Mpro),32 and spike protein RBD,26 impacting the adaptability of the virus to antiviral drugs and antibody therapeutics. This study delved into the design and analysis of nsp7 mutations that may have an impact on the stability, adaptation, and fitness of nsp7 dimers. This comprehensive approach involved an integration of high-throughput symmetrical protein design, ML, structural analysis, and validation of the computational predictions using an array of strategies (Fig. 3). The electron microscopic structure of the RdRp complex utilized by the CPD approach guided the identification of potential hotspot residues within the dimeric nsp7 interface (Fig. 1). Symmetrical protein design using Rosetta aimed to modulate the dimerization of nsp7, considering 30 selected residues (Table 1). The results demonstrated acceptable structural changes, emphasizing the intricate nature of small protein–protein complexes. Several features, including the Rosetta total score, RMSD, and sequence identity, were analyzed to assess the designs, their physicochemical properties, and mutational landscape profiles (Fig. 2 and 4). Affinity-enhancing designs exhibited favourable energetics, lower Rosetta total scores, and higher binding affinity compared to affinity-reducing counterparts. Subsequently, ML-predicted results correlated well with the CPD results and provided additional insights. The RFR model outperformed other algorithms, showcasing its accuracy in predicting binding affinities (Fig. 5). ML-based sequence prediction further elucidated the potential nsp7 sequences harbouring mutations (indicating affinity-enhancing and affinity-reducing classifications), providing insights into their binding affinity, Rosetta total score, and capability to derive new sequence combinations (Fig. 6 and Tables 7, 8). The AlphaFold v2.0 structural analysis provided insights into the optimal dimeric configurations of affinity-enhancing designs, further validating the precision of our approach presented here (Fig. 7). Employing NMA-based analysis, our study further provided insights into the protein dynamics and stability of the dimeric structures of wild-type nsp7, affinity-enhancing, and affinity-reducing designs. Deformability plots highlighted the flexible regions, with affinity-enhancing designs showing increased flexibility (Fig. 8A). Correlation covariance matrices revealed distinct atomic motion states (Fig. 8B–D). Eigenvalue analysis indicated localized motions, emphasizing differences between the dimeric wild-type nsp7, and the affinity-enhancing and affinity-reducing designs (Fig. S2, ESI†). Finally, our comprehensive study not only provided valuable insights into the mechanisms governing nsp7 affinity-enhancing and affinity-reducing designs but also contributed to the understanding of the dynamic nature of viral mutations and their impact on viral fitness. The revealed physicochemical properties, molecular interactions, and sequence predictions of the dimeric nsp7 subunit may offer potential avenues for therapeutic intervention in combating COVID-19.
Limitations of the study
The study has certain limitations. Firstly, the complexity of the Rosetta symmetrical dimeric interface design script constrained our ability to carry out a symmetric design of all subunits of the RdRp. Specifically, only the nsp7 subunit was designed, necessitating the development of an advanced symmetrical dimeric interface protocol to encompass all RdRp subunits for a more comprehensive assessment of mutation effects. Secondly, while CPD- and ML-based approaches successfully predicted favourable mutations on the dimeric nsp7 interface, experimental validation is indispensable to ascertain the actual binding affinity between the designed nsp7 monomers. Thirdly, other robust computational approaches could be utilized to obtain single-point mutations of nsp7 that may drive the sequences towards a stronger or weaker binding between the nsp7 monomers. However, to address these limitations and validate the precision of our approach, we cross-referenced our predictions with clinically known mutations in the SARS-CoV-2 nsp7 subunit of RdRp. These mutations, known in clinical contexts, provide additional evidence supporting the adaptability and stability of nsp7 dimers.
Conclusion
In conclusion, our study addresses the critical aspects of SARS-CoV-2 intracellular proteins, exploring the intricate dynamics of its RdRp, particularly the nsp7 subunit. Concerning the COVID-19 pandemic, understanding the molecular intricacies of viral components becomes paramount. Our research employs a comprehensive approach, shedding light on the stability, adaptation, and fitness of nsp7 dimers. The study reveals that nsp7, a crucial player in viral replication, forms symmetrical dimers through a complex interface, influencing binding affinity and stability. By employing a Rosetta-based design strategy and ML-based predictions, we identified specific hotspot residues within the dimeric nsp7 interface. Affinity-enhancing designs exhibited favourable energetics and higher binding affinity, validated through structural analyses using AlphaFold v2.0. Importantly, our predictions align with clinically known mutations, providing real-world validation. While our focus on the nsp7 subunit offers substantial insights, acknowledging the necessity for a comprehensive analysis involving all RdRp subunits becomes crucial. In the broader context, our findings contribute valuable information for potential therapeutic interventions against COVID-19, emphasizing the dynamic nature of viral mutations and their impact on viral fitness.
Author contributions
Amar Jeet Yadav, Shivank Kumar, Shweata Maurya, Khushboo Bhagat, and Aditya K. Padhi contributed to the conceptualization, formal analysis, investigation, methodology, and writing of the original draft. Aditya K. Padhi contributed to conceptualization. Aditya K. Padhi contributed to funding acquisition and project administration. All authors contributed to the writing and preparation of the final version of the manuscript.
Conflicts of interest
There are no conflicts to declare.
Acknowledgements
The authors sincerely acknowledge the infrastructure facilities of IIT (BHU) Varanasi and DST-funded I-DAPT Hub Foundation, IIT (BHU) [DST/NMICPS/TIH11/IIT(BHU)2020/02]. Further, the computing resources of PARAM Shivay Facility under the National Supercomputing Mission, Government of India at the IIT (BHU), Varanasi, are gratefully acknowledged. A. J. Y., S. M., and K. B. acknowledge the Ministry of Human Resource Development (MHRD), the Government of India, and IIT (BHU) for their research fellowships. A. K. P. is grateful for the financial support received from the Science & Engineering Research Board (SERB), Government of India's Project No. SRG/2023/000167 and Indian Institute of Technology (Banaras Hindu University) Varanasi's Seed Grant ref. No. IIT (BHU)/Budget/19-(14)/2022-23/17507.
References
- P. V. Markov, M. Ghafari, M. Beer, K. Lythgoe, P. Simmonds, N. I. Stilianakis and A. Katzourakis, The evolution of SARS-CoV-2, Nat. Rev. Microbiol., 2023, 21, 361–379 CrossRef CAS PubMed.
- A. Sharma, S. Tiwari, M. K. Deb and J. L. Marty, Severe acute respiratory syndrome coronavirus-2 (SARS-CoV-2): a global pandemic and treatment strategies, Int. J. Antimicrob. Agents, 2020, 56, 106054 CrossRef CAS PubMed.
- A. A. T. Naqvi, K. Fatima, T. Mohammad, U. Fatima, I. K. Singh, A. Singh, S. M. Atif, G. Hariprasad, G. M. Hasan and Md. I. Hassan, Insights into SARS-CoV-2 genome, structure, evolution, pathogenesis and therapies: structural genomics approach, Biochim. Biophys. Acta, Mol. Basis Dis., 2020, 1866, 165878 CrossRef CAS PubMed.
- H. Yang and Z. Rao, Structural biology of SARS-CoV-2 and implications for therapeutic development, Nat. Rev. Microbiol., 2021, 19, 685–700 CrossRef CAS PubMed.
- R. Yadav, J. K. Chaudhary, N. Jain, P. K. Chaudhary, S. Khanra, P. Dhamija, A. Sharma, A. Kumar and S. Handu, Role of Structural and Non-Structural Proteins and Therapeutic Targets of SARS-CoV-2 for COVID-19, Cells, 2021, 10, 821 CrossRef CAS PubMed.
- C. B. Jackson, M. Farzan, B. Chen and H. Choe, Mechanisms of SARS-CoV-2 entry into cells, Nat. Rev. Mol. Cell Biol., 2022, 23, 3–20 CrossRef CAS PubMed.
- P. V’kovski, A. Kratzel, S. Steiner, H. Stalder and V. Thiel, Coronavirus biology and replication: implications for SARS-CoV-2, Nat. Rev. Microbiol., 2021, 19, 155–170 CrossRef PubMed.
- Z. Almubaid and H. Al-Mubaid, Analysis and comparison of genetic variants and mutations of the novel coronavirus SARS-CoV-2, Gene Rep, 2021, 23, 101064 CrossRef CAS PubMed.
- A. A. Rabaan, S. H. Al-Ahmed, H. Albayat, S. Alwarthan, M. Alhajri, M. A. Najim, B. M. AlShehail, W. Al-Adsani, A. Alghadeer, W. A. Abduljabbar, N. Alotaibi, J. Alsalman, A. H. Gorab, R. S. Almaghrabi, A. A. Zaidan, S. Aldossary, M. Alissa, L. M. Alburaiky, F. M. Alsalim, N. Thakur, G. Verma and M. Dhawan, Variants of SARS-CoV-2: Influences on the Vaccines’ Effectiveness and Possible Strategies to Overcome Their Consequences, Medicina (B Aires), 2023, 59, 507 CrossRef PubMed.
- A. M. Carabelli, T. P. Peacock, L. G. Thorne, W. T. Harvey, J. Hughes, T. I. de Silva, S. J. Peacock, W. S. Barclay, T. I. de Silva, G. J. Towers and D. L. Robertson, SARS-CoV-2 variant biology: immune escape, transmission and fitness, Nat. Rev. Microbiol., 2023, 21, 162–177 CAS.
- J. K. Das, B. Thakuri, K. MohanKumar, S. Roy, A. Sljoka, G.-Q. Sun and A. Chakraborty, Mutation-Induced Long-Range Allosteric Interactions in the Spike Protein Determine the Infectivity of SARS-CoV-2 Emerging Variants, ACS Omega, 2021, 6, 31305–31320 CrossRef CAS PubMed.
- Q. Zhang, R. Xiang, S. Huo, Y. Zhou, S. Jiang, Q. Wang and F. Yu, Molecular mechanism of interaction between SARS-CoV-2 and host cells and interventional therapy, Signal Transduction Targeted Ther., 2021, 6, 233 CrossRef CAS PubMed.
- W. Wu, Y. Cheng, H. Zhou, C. Sun and S. Zhang, The SARS-CoV-2 nucleocapsid protein: its role in the viral life cycle, structure and functions, and use as a potential target in the development of vaccines and diagnostics, Virol. J., 2023, 20, 6 CrossRef CAS PubMed.
- Y. Jiang, W. Yin and H. E. Xu, RNA-dependent RNA polymerase: structure, mechanism, and drug discovery for COVID-19, Biochem. Biophys. Res. Commun., 2021, 538, 47–53 CrossRef CAS PubMed.
- A. J. W. te Velthuis, J. M. Grimes and E. Fodor, Structural insights into RNA polymerases of negative-sense RNA viruses, Nat. Rev. Microbiol., 2021, 19, 303–318 CrossRef CAS PubMed.
- X. Yin, H. Popa, A. Stapon, E. Bouda and M. Garcia-Diaz, Fidelity of Ribonucleotide Incorporation by the SARS-CoV-2 Replication Complex, J. Mol. Biol., 2023, 435, 167973 CrossRef CAS PubMed.
- E. J. Snijder, E. Decroly and J. Ziebuhr, The Nonstructural Proteins Directing Coronavirus RNA Synthesis and Processing, Adv. Virus Res., 2016, 96, 59–126 CAS.
- E. Konkolova, M. Klima, R. Nencka and E. Boura, Structural analysis of the putative SARS-CoV-2 primase complex, J. Struct. Biol., 2020, 211, 107548 CrossRef CAS PubMed.
- R. N. Kirchdoerfer and A. B. Ward, Structure of the SARS-CoV nsp12 polymerase bound to nsp7 and nsp8 co-factors, Nat. Commun., 2019, 10, 2342 CrossRef PubMed.
- F. A. Jochheim, D. Tegunov, H. S. Hillen, J. Schmitzová, G. Kokic, C. Dienemann and P. Cramer, The structure of a dimeric form of SARS-CoV-2 polymerase, Commun. Biol., 2021, 4, 999 CrossRef CAS PubMed.
- A. K. Padhi and T. Tripathi, A comprehensive protein design protocol to identify resistance mutations and signatures of adaptation in pathogens, Brief Funct. Genomics, 2023, 22, 195–203 CrossRef CAS PubMed.
- P. Kalita, T. Tripathi and A. K. Padhi, Computational Protein Design for COVID-19 Research and Emerging Therapeutics, ACS Cent. Sci., 2023, 9, 602–613 CrossRef CAS PubMed.
- A. K. Padhi, R. Shukla, P. Saudagar and T. Tripathi, High-throughput rational design of the remdesivir binding site in the RdRp of SARS-CoV-2: implications for potential resistance, iScience, 2021, 24, 101992 CrossRef CAS PubMed.
- F. Kabinger, C. Stiller, J. Schmitzová, C. Dienemann, G. Kokic, H. S. Hillen, C. Höbartner and P. Cramer, Mechanism of molnupiravir-induced SARS-CoV-2 mutagenesis, Nat. Struct. Mol. Biol., 2021, 28, 740–746 CrossRef CAS PubMed.
- A. K. Padhi and T. Tripathi, Hotspot residues and resistance mutations in the nirmatrelvir-binding site of SARS-CoV-2 main protease: design, identification, and correlation with globally circulating viral genomes, Biochem. Biophys. Res. Commun., 2022, 629, 54–60 CrossRef CAS PubMed.
- S. Maurya, S. Kumar and A. K. Padhi, Interface-Guided Computational Protein Design Reveals Bebtelovimab-Resistance Mutations in SARS-CoV-2 RBD: Correlation with Global Viral Genomes and Bebtelovimab-Escape Mutations, ChemistrySelect, 2023, 8(46), e20230290 CrossRef.
- K. K. Yang, Z. Wu and F. H. Arnold, Machine-learning-guided directed evolution for protein engineering, Nat. Methods, 2019, 16, 687–694 CrossRef CAS PubMed.
- J. Jumper, R. Evans, A. Pritzel, T. Green, M. Figurnov, O. Ronneberger, K. Tunyasuvunakool, R. Bates, A. Žídek, A. Potapenko, A. Bridgland, C. Meyer, S. A. A. Kohl, A. J. Ballard, A. Cowie, B. Romera-Paredes, S. Nikolov, R. Jain, J. Adler, T. Back, S. Petersen, D. Reiman, E. Clancy, M. Zielinski, M. Steinegger, M. Pacholska, T. Berghammer, S. Bodenstein, D. Silver, O. Vinyals, A. W. Senior, K. Kavukcuoglu, P. Kohli and D. Hassabis, Highly accurate protein structure prediction with AlphaFold, Nature, 2021, 596, 583–589 CrossRef CAS PubMed.
- R. F. Alford, A. Leaver-Fay, J. R. Jeliazkov, M. J. O’Meara, F. P. DiMaio, H. Park, M. V. Shapovalov, P. D. Renfrew, V. K. Mulligan, K. Kappel, J. W. Labonte, M. S. Pacella, R. Bonneau, P. Bradley, R. L. Dunbrack, R. Das, D. Baker, B. Kuhlman, T. Kortemme and J. J. Gray, The Rosetta All-Atom Energy Function for Macromolecular Modeling and Design, J. Chem. Theory Comput., 2017, 13, 3031–3048 CrossRef CAS PubMed.
- S. J. Fleishman, A. Leaver-Fay, J. E. Corn, E.-M. Strauch, S. D. Khare, N. Koga, J. Ashworth, P. Murphy, F. Richter, G. Lemmon, J. Meiler and D. Baker, RosettaScripts: A Scripting Language Interface to the Rosetta Macromolecular Modeling Suite, PLoS One, 2011, 6, e20161 CrossRef CAS PubMed.
- P. B. Stranges, M. Machius, M. J. Miley, A. Tripathy and B. Kuhlman, Computational design of a symmetric homodimer using β-strand assembly, Proc. Natl. Acad. Sci. U. S. A., 2011, 108, 20562–20567 CrossRef CAS PubMed.
- A. K. Padhi and T. Tripathi, High-throughput design of symmetrical dimeric SARS-CoV-2 main protease: structural and physical insights into hotspots for adaptation and therapeutics, Phys. Chem. Chem. Phys., 2022, 24, 9141–9145 RSC.
- A. K. Padhi, J. Dandapat, P. Saudagar, V. N. Uversky and T. Tripathi, Interface-based design of the favipiravir-binding site in SARS-CoV-2 RNA-dependent RNA polymerase reveals mutations conferring resistance to chain termination, FEBS Lett., 2021, 595, 2366–2382 CrossRef CAS PubMed.
- S. Elbe and G. Buckland-Merrett, Data, disease and diplomacy: GISAID's innovative contribution to global health, Global Challenges, 2017, 1, 33–46 CrossRef PubMed.
- G. R. C. M. R. D. Singer J, CoV-GLUE: a web application for tracking SARS-CoV-2 genomic variation, Preprints (Basel).
- S. Babicki, D. Arndt, A. Marcu, Y. Liang, J. R. Grant, A. Maciejewski and D. S. Wishart, Heatmapper: web-enabled heat mapping for all, Nucleic Acids Res., 2016, 44, W147–W153 CrossRef CAS PubMed.
- J. Cheng, A. Randall and P. Baldi, Prediction of protein stability changes for single-site mutations using support vector machines, Proteins: Struct., Funct., Bioinf., 2006, 62, 1125–1132 CrossRef CAS PubMed.
- G. E. Crooks, G. Hon, J.-M. Chandonia and S. E. Brenner, WebLogo: A Sequence Logo Generator: Fig. 1, Genome Res., 2004, 14, 1188–1190 CrossRef CAS PubMed.
- H. C. Jubb, A. P. Higueruelo, B. Ochoa-Montaño, W. R. Pitt, D. B. Ascher and T. L. Blundell, Arpeggio: A Web Server for Calculating and Visualising Interatomic Interactions in Protein Structures, J. Mol. Biol., 2017, 429, 365–371 CrossRef CAS PubMed.
- L. C. Xue, J. P. Rodrigues, P. L. Kastritis, A. M. Bonvin and A. Vangone, PRODIGY: a web server for predicting the binding affinity of protein–protein complexes, Bioinformatics, 2016, 32, 3676–3678 CrossRef CAS PubMed.
- J. R. López-Blanco, J. I. Aliaga, E. S. Quintana-Ortí and P. Chacón, iMODS: internal coordinates normal mode analysis server, Nucleic Acids Res., 2014, 42, W271–W276 CrossRef PubMed.
- S. M. S. Reshamwala, V. Likhite, M. S. Degani, S. S. Deb and S. B. Noronha, Mutations in SARS-CoV-2 nsp7 and nsp8 proteins and their predicted impact on replication/transcription complex structure, J. Med. Virol., 2021, 93, 4616–4619 CrossRef CAS PubMed.
- I. P. Trougakos, K. Stamatelopoulos, E. Terpos, O. E. Tsitsilonis, E. Aivalioti, D. Paraskevis, E. Kastritis, G. N. Pavlakis and M. A. Dimopoulos, Insights to SARS-CoV-2 life cycle, pathophysiology, and rationalized treatments that target COVID-19 clinical complications, J. Biomed. Sci., 2021, 28, 9 CrossRef CAS PubMed.
- A. Dubey, S. Choudhary, P. Kumar and S. Tomar, Emerging SARS-CoV-2 Variants: Genetic Variability and Clinical Implications, Curr. Microbiol., 2021, 79, 20 CrossRef PubMed.
- T. Nelemans and M. Kikkert, Viral Innate Immune Evasion and the Pathogenesis of Emerging RNA Virus Infections, Viruses, 2019, 11, 961 CrossRef CAS PubMed.
- S. Venkataraman, B. V. L. S. Prasad and R. Selvarajan, RNA Dependent RNA Polymerases: Insights from Structure, Function and Evolution, Viruses, 2018, 10, 76 CrossRef PubMed.
- Q. Peng, R. Peng, B. Yuan, J. Zhao, M. Wang, X. Wang, Q. Wang, Y. Sun, Z. Fan, J. Qi, G. F. Gao and Y. Shi, Structural and Biochemical Characterization of the nsp12–nsp7–nsp8 Core Polymerase Complex from SARS-CoV-2, Cell Rep., 2020, 31, 107774 CrossRef CAS PubMed.
- L. Subissi, C. C. Posthuma, A. Collet, J. C. Zevenhoven-Dobbe, A. E. Gorbalenya, E. Decroly, E. J. Snijder, B. Canard and I. Imbert, One severe acute respiratory syndrome coronavirus protein complex integrates processive RNA polymerase and exonuclease activities, Proc. Natl. Acad. Sci. U. S. A., 2014, 111(37), E3900–9 CrossRef CAS PubMed.
|
This journal is © the Owner Societies 2024 |