DOI:
10.1039/D3CP03752E
(Review Article)
Phys. Chem. Chem. Phys., 2023,
25, 26972-26985
Current stage and future development of Belgrade collisional and radiative databases/datasets of importance for molecular dynamics
Received
5th August 2023
, Accepted 23rd September 2023
First published on 27th September 2023
Abstract
Atomic and molecular (A&M) databases that contain information about species, their identities and radiative/collisional processes are essential and helpful tools that are utilized in many fields of physics, chemistry, and chem/phys-informatics. Errors or inconsistencies in the datasets are a serious issue since they can lead to inaccurate predictions and generate problems with the modeling. This demonstrates that data curation efforts around A&M databases are still indispensable and that in the curation process studious attention is required. Therefore, we herein present research activities around Belgrade “nodes” – datasets of collision/radiative cross-sections and rates needed for spectroscopy analysis in various A&M, optical and plasma physics fields. Methodologies of our research and both present and future aspects of the applications are explained. We explored the possibility to extend our nodes towards building a new database on Judd–Ofelt parameters by using machine learning in order to predict optical properties of luminescence materials. In addition, we hope that public availability of our datasets and their graphical representations will also motivate others to investigate the potential of these data.
1. Introduction
Atomic and molecular physics has made huge contributions to our fundamental understanding of nature. The last few decades have seen remarkable progress in both theoretical/computational and experimental techniques applied to the study of radiative and collisional processes involving atoms and molecules.1,2 Such research has required coordinated studies to provide benchmark data which not only contribute to fundamental science but also have practical implications in various applications and play a crucial role in various fields of science and technology, including astrophysics and space science, plasma physics, nuclear physics, materials science, semiconductor industry, atmospheric sciences, the chemistry of the interstellar medium, medicine, radiation damage and therapy etc.3–8
Amongst the most important atomic and molecular processes are those involving collisions. Collisional processes involve interactions between particles, such as atoms, molecules, ions, electrons, photons, resulting in energy transfer, scattering, or recombination. These processes are essential for understanding many diverse phenomena including gas dynamics, plasma physics, energy transfer and chemical reactions.9 Electron scattering from atoms and molecules is one of the most important collisional process and has been intensively studied over a long period of time. As a target in the collision process, the theoretical representation of molecules acquires a new point of view from the mathematical perspectives of energy quantification methods.10–12 Electron impact collision cross section databases over a wide range of impact energies are required to model and underpin the interactions in various applied fields13 while total cross section data are useful for the study of electron transport properties in gases.14,15 The last few decades have seen tremendous progress in both the computational and experimental techniques applied to study the scattering of electrons by biologically relevant molecules. Much ongoing effort has been undertaken to provide a deeper insight into the mechanisms related to the radiation damage of DNA, which includes many processes (ionization, electronic, rotational, and vibrational excitations, dissociation and dissociative electron attachment). Recently, Ebel and Bald16 presented the first absolute quantification of the energy-dependent strand breakage of a specific sequence of double-stranded DNA by the low-energy electrons (LEE) in the energy range of 5–20 eV (providing accurate quantification of DNA radiation damage of well-defined DNA target sequences in terms of absolute cross sections for LEE-induced DNA strand breaks). An overview of the various applications of collision physics with an emphasis on the importance and power of theoretical and computational techniques has been recently provided by Srivastava and Fursa.17 Recent progress in obtaining experimental benchmark data and the development of highly sophisticated computational methods for use in plasma-using technologies is highlighted in the papers Bartschat and Kushner18 and Schippers et al.19
Particle transport and energy deposition are also one of the essential aspects of processes driving physics and chemistry in the local environment of the collision event. Such processes involve the movement of particles, such as atoms, molecules, or subatomic particles, and the transfer of energy to the surrounding medium. Ongoing research on particle transport and energy deposition aims to improve our knowledge of these processes, leading to advances in areas such as fusion energy (the transport of charged particles is fundamental to understanding phenomena like plasma confinement and fusion reactions), nuclear physics (the deposition of energy by high-energy particles can lead to nuclear reactions or the creation of ionizing radiation), and local chemistry (energy deposition plays a crucial role in processes like chemical reactions, where energy is transferred between reacting species, leading to the breaking or formation of chemical bonds).20–22
Radiative processes involve the emission, absorption, and scattering of electromagnetic radiation by atoms, molecules, and particles.23 They are crucial for investigating the behavior of light and its interactions with matter, as well as the study of various astrophysical phenomena, including stellar spectra, interstellar medium, and cosmology.24 Spectroscopic techniques provide valuable information about the composition and properties of materials and celestial objects. Ongoing advancements in spectroscopy, including high-resolution and multi-wavelength observations, enable scientists to study distant galaxies, planet atmospheres, and diagnose various materials.25–29 Study of radiative processes help us to understand how radiation propagates through different media, such as planetary atmospheres, interstellar clouds, and biological tissues30 Radiative processes also play a crucial role in quantum optics and quantum information science. Controlling and manipulating the emission and absorption of individual photons is crucial in developing quantum cryptography, quantum computing, and quantum sensing.31
As one can see, atomic and molecular (A&M) databases are indispensable tools that support scientific research, technological advancements and innovation in a wide range of disciplines (Fig. 1). They can help solve open questions and challenges on the interaction dynamics of molecules and clusters in the gas phase like “How do molecules form and survive in the interstellar medium?” “What are the conditions and the mechanisms?”, etc. (see e.g. Gatchell & Zettergren32). Here we present research activities in Belgrade that develop databases on collision/radiative cross-sections, describe the development and some technical aspects of the databases, summarize some recent and future developments and currently planned updates and outline our plans for the near future.
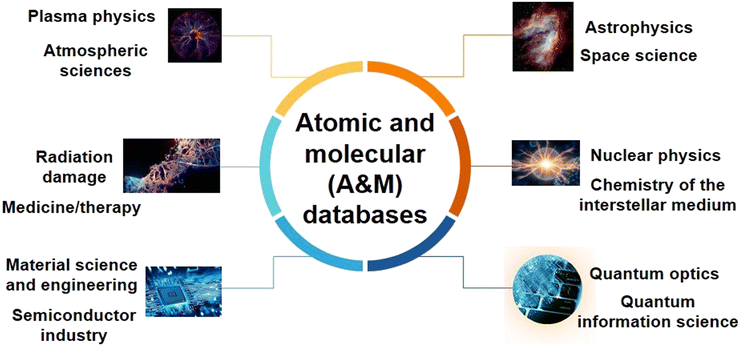 |
| Fig. 1 A&M data and databases play an important role in various fields of science. | |
The BG A&M databases status, challenges and directions are presented in Section 2, while the technical aspects are presented and discussed in Section 3. Section 4 describes building the rare earth data base supported by machine learning (ML) approach. Finally, Section 5 is devoted to some conclusions.
2. Belgrade nodes: status, challenges and directions
A Virtual Atomic and Molecular Data Center (VAMDC) “node” is a separate software entity implemented by a data provider, which consists of a database and web services compliant to VAMDC standards and protocols.34 In practice, this is most often implemented using VAMDC-developed NodeSoftware and adapting it for specifics of the particular dataset and technical environment, on top of which custom web interface and additional (graphical) tools may be built.
Atomic and molecular databases and data are becoming increasingly important for data interpretation, diagnostics, and the development of models and simulations of complex physical processes.35–39 The Belgrade radiative and collisional nodes of VAMDC are hosted by the Serbian Virtual Observatory (SerVO) and currently consists of three databases BEAMDB (https://servo.aob.rs/emol) and MolD (https://servo.aob.rs/mold) and ACol (https://servo.aob.rs/acol). These databases are complementary with other available databases covering similar collisional processes, like KIDA36 and LXCat databases.40 While KIDA is more oriented towards astrochemistry and LXCat towards processes in plasmas, our databases cover data of astrophysical relevance. However, the data and its analysis highlight their interdisciplinary nature and usage, e.g., in physics, astrophysics, chemistry and biology.
2.1 BEAMDB-Belgrade electron-atom(molecule) database
The early stages of Belgrade database development started in 2007 with the creation of the Information system in atomic collision physics.41 In this paper some fundamental aspects of scientific research in the field of atomic physics were discussed where the information system was designed to cover several phases of research from planning the scientific study and literature survey to the creation of databases and dissemination of the results. In conformity with the objectives of two COST (Co-operation in Science and Technology) Actions, P9 Radiation Damage in Biomolecular Systems (RADAM-https://www.cost.eu/actions/P9/) and MP1002 Nano-scale insights in ion beam cancer therapy (Nano-IBCT-https://www.cost.eu/actions/MP1002/), we developed a particular database42 in which data from electron interactions with atomic and molecular targets are maintained with the focus on electron-metal atom cross sections. In the broad scope of the RADAM database43 that covers processes from elementary ones to multi-scale and biological effect processes, BEAMDB represents a node that is restricted to processes governed by electron impact. When RADAM database was invited to join the VAMDC project34 BEAMDB became part of that network.44
Once BEAMDB had been established attention was turned towards the exploitation of data maintained within it. The first successful usage was in the study of electron scattering cross sections, data needs and coverage in BEAMDB Database for the Rosetta Mission46 where the direct evidence for the role of electron induced processing was plainly visible. The emission lines observed in the coma of Comet 67P/Churyumov-Gerasimenko during the Rosetta mission were able to be shown to be due to electron rather than photo induced dissociative excitation. The turbulent processes in solar wind on its routes emerging from the solar corona have been remotely measured47 revealing the existence of two classes of electrons, “warm” and “suprathermal”, while the interaction of the solar wind and the comet have been analysed as a function of heliocentric distance.48 These observations led to the development of a fully 3D kinetic model which simulates the ion and electron dynamics of the solar wind interaction with a weakly outgassing comet 67P covering the complete energy distribution of electrons.49 The model draws on data in the BEAMDB.
The BEAMDB database has also been used in a survey of existing cross section data for elastic electron scattering by methane in order to compare present measurements at the Institute of Physics Belgrade (IPB)50 with those available in literature. The strength of interactions of electrons with a methane molecule is characterised by cross-sections which need to be accurately determined using both experiment and theory. A combined experimental (electron spectrometry measurements) and a theoretical (using two approximations: a simple sum of individual atomic cross-sections and the other with molecular effects taken into the account) study had been performed where differential, integral and momentum transfer cross-sections in the intermediate energy range (50–300 eV) have been obtained.50 Absolute values of elastic cross sections were obtained by using relative flow method51 in comparison with known cross sections of argon as a reference gas.52 In the experiment, effusive beam properties had been predicted and optimized following the work of Lucas.53
Regarding data on electron scattering from metal atom vapours, many of the most important cross sections have been assembled at the IPB using a specifically designed electron spectrometer for handling the metal vapours.55,56 The procedure of obtaining the absolute cross sections for electron scattering by metal atom vapours is different than for other gases. Here the relative flow method can not be performed and we need to rely on other normalisation methods such as converting relative differential cross sections (DCSs) to generalised oscillator strength curves and extrapolation of these to the well-known optical oscillator strength in the zero squared momentum transfer limit.57 Lassettre formula may then be applied in order to put cross sections an absolute scale using the DCSs for the 61 P1 state of mercury58 as reference. An additional improvement has been achieved with the development of forward scattering function method.59 This method has been applied for electronic excitations of resonant lines of zinc,60 lead61 and most recently cadmium.62
Molecular dynamics induced by electron impact has been extensively studied. As an example of the low energy dissociative electron attachment process we refer on the case studies of ozone63,64 where resonant collisions induce formation of atomic and molecular oxygen anions with large cross sections having important consequences for the anion formation processes in the ionosphere. The shape resonances were observed in vibrationally inelastic differential cross sections with long progressions and numerous combination vibrations for all three vibrational modes in energy loss spectra of ozone.64 In BEAMDB we stored data for DCS for vibrational excitation from the ground state v = 0 to the v = 1 of furan molecule calculated on the basis of the unitarized first Born approximation (UFBA) by Čurik.33 Here all 21 modes65 were summed and presented in Fig. 2.
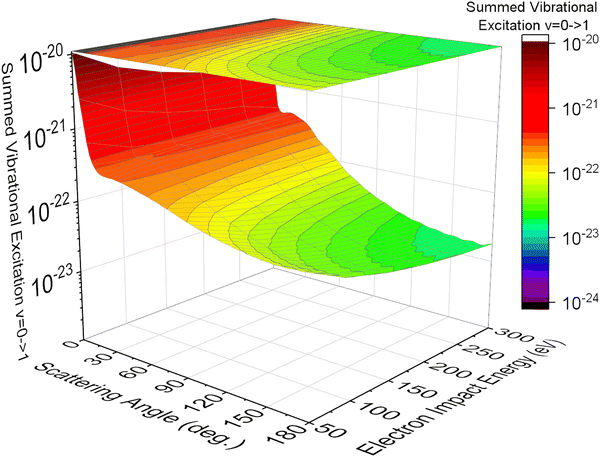 |
| Fig. 2 Sum of 21 modes for rotationally summed 0 → 1 vibrationally inelastic absolute differential cross sections for scattering of electrons by furan molecule, calculated by UFBA method. The dataset is presented in Maljković et al.33 | |
Another example of the importance of electron collisional processes in molecular dynamic studies is presented in the simulations of focused electron beam induced deposition (FEBID). Upon the irradiation of metal containing precursor molecules by high energy electrons the molecule is dissociated resulting in deposition of the metal on the irradiated surface. The process is governed by the convolution of flux densities of primary electron beam, secondary electrons and back scattered electrons and the fragmentation cross section of the precursor molecule.66 Dissociative electron attachment (at electron energies below the first ionization energy), dissociative ionization (at higher electron energies) and neutral dissociation are the processes responsible for the fragmentation of precursor molecules. One of the applications of the FEBID process is presented in nanofabrication of Pt/TiO2/Pt memristor device67 obtained from electron irradiation by trimethyl(methylcyclopentadienyl)platinum(IV) used as a precursor for Pt–C electrode deposition and titanium isopropoxide used as a precursor for the TiO2 layer.
2.2 Photodissociation—The MolD Database
As part of SerVO and VAMDC MolD is made up of a number of parts, including tools for data collecting and user interfaces (e.g. AJAX-enabled queries and visualizations on-site). The MolD database includes information on several molecular species their excited states characterizations and photodissociation cross sections in different rovibrational states. These cross sections can be used to obtain average thermal cross sections (see Fig. 3), rate coefficients for non-local thermal equilibrium models and feed into models of various atmospheres, laboratory plasmas and are used in several technological applications.68–73
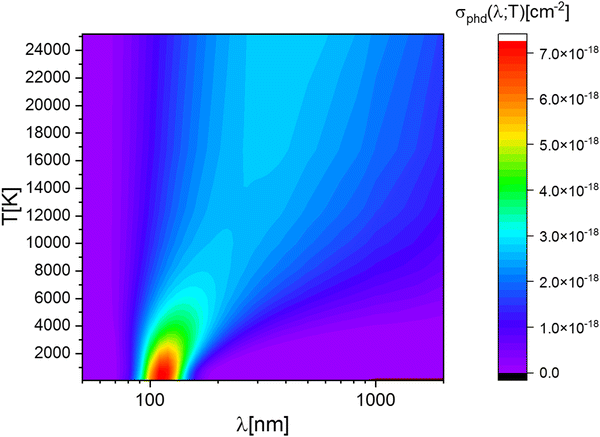 |
| Fig. 3 The surface plot of average cross-section for photodissociation, against λ and T, of H2+ molecular ion. The dataset is obtained in Srećković et al.45 | |
The MolD database was developed in several stages. The first one, which was finished at the end of 2014, was distinguished by the development of a web interface, certain utility programs, and a service for all photodissociation data for hydrogen and helium molecular ions.74 In each subsequent stage, the web service and utility programs (that allow online data visualization of a wide range of data were developed) were upgraded and new species (MgH+, HeH+, LiH+, NaH+, H2+, He2+ …) with photodissociation data are added.
The cross section datasets are obtained using a quantum mechanical method in which the photodissociation process is represented as the result of radiative transitions between the ground and the first excited adiabatic electronic state of the molecular ion (see e.g. Sreckovic et al.75 and references therein). In the dipole approximation, the transitions result from the interaction of the electronic component of the ion-atom system with the electromagnetic field.76
On-site assistance† is available from MolD, which also provides average thermal cross sections based on the temperature for a particular molecule and wavelength. Plotting of average thermal cross-sections along with available wavelengths for a given temperature is made possible by additional on-site utilities in addition to operating as a VAMDC compatible web service, accessible through the VAMDC portal, and other tools created using VAMDC standards.
As part of SerVO and VAMDC, MolD is heavily utilized by astrophysicists to model stellar atmospheres,77–79etc. However, the data can be used for a variety of purposes, including plasma chemistry or experiments like the PLEIADES synchrotron,80–82 as well as plasma fusion, industry, and for several technology applications.83–85
2.3 ACol-database for collisional processes
Moving from the cold gas approximation to the state-to-state approach, the A&M community has demonstrated a growing maturity in developing processes for curating dynamical data pertinent to the kinetic modeling of low temperature plasmas (LTPs), with the goal of furthering the understanding of fundamental processes involving both electron and heavy species collisions.86–94 It has recently been shown that research in the food industry and technology suggest the potential usage of plasmas in the treatment of plants such that they may be treated without pesticides or other toxic agents.95,96 Accordingly A&M datasets of interest for the bio/chemistry and food industry, which include electrons and ions collisions with small molecules and various excited species, are being made available.97
As noted ACol comprises the rate coefficients for the collisional processes of excitation/deexcitation and ionization/recombination in hydrogen, helium, and alkali plasmas. The ACol database is now in development. The dataset may be useful for studying and simulating LTP in the lab as well as weakly ionized layers in various atmospheres, etc. Moreover the existence and development of the ACol database/node might be crucial for diagnostics and modeling of LTPs which are used for the bio/chemistry and food industry.98–100 The ACol database is composed of collisional data for excitation/deexcitation and ionization/recombination processes.
The database is made up of collisional ionization/recombination
| 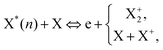 | (1) |
and excitation/deexcitation
| X*(n) + X ⇔ X*(n′ = n + p) + X | (2) |
data obtained at the IPB. Here X, X
+ are atoms and their ions in the ground states, X*(
n) are the Rydberg atoms (RA), and X
2+ their molecular ions. The above mentioned processes are such that system goes through the phase where its parts can be described as a collisional quasi-molecular ion-atom complex.
Fig. 4 presents rate coefficients for associative ionization (upper) and non-associative ionization channels (lower) of eqn (1) as a function of n and T for X = H.
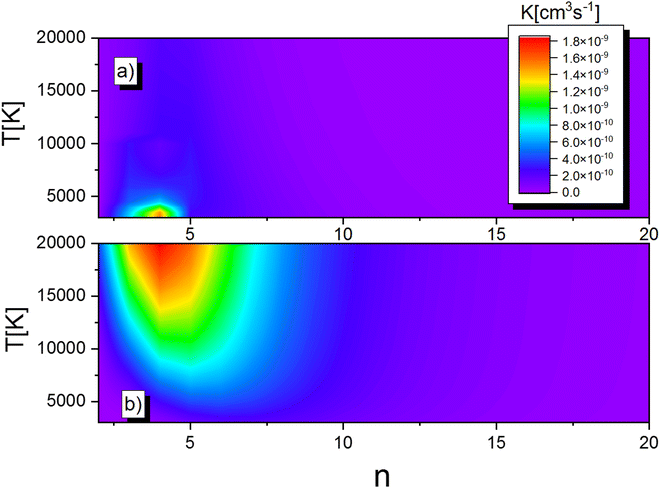 |
| Fig. 4 (a) Rate coefficient for associative ionization channel (upper) of eqn (1) as a function of n and T for the case X = H. (b) same as a) but for non-associative ionization channel (lower) of eqn (1). Dataset is obtained in Srećković et al.54 | |
ACol presents cross-sections and rate coefficients for the ionization/recombination and excitation/deexcitation processes for hydrogen, helium and alkali plasmas, for conditions of interest in the laboratory, in astrochemistry and in LTPs. The results were acquired within the DRM, which means that the collisional reactions were handled by the so-called dipole resonant mechanism (DRM) (see Mihajlov et al.101 and references therein). The processes in this characterisation are driven by the dipole component of the electrostatic interaction between the outer highly excited ion-atom system and the inner (ion-atom) system. This technique works especially well when used with the so-called decay approximation. The details can be found in a set of papers.101–103
The ACol database is hosted by SerVO https://servo.aob.rs/acol/. The queries are hosted locally on the website which also deliver data in XSAMS format defined by VAMDC36 (https://servo.aob.rs/acol/) via AJAX-enabled web page. On-site, we included an additional collisional processes dialogue box for the XSAMS query and added visual representations of temperature depending cross sections for the new datasets. Web service requests are handled by Python-based NodeSoftware which works on top of a relational database and is available for queries via a centralized portal (https://portal.vamdc.eu/vamdc_portal/home.seam).
3 Technical aspects of databases and web services
From their initial stages of development, the Belgrade A&M databases have been closely connected with the Virtual Atomic and Molecular Data Center project (VAMDC-https://vamdc.eu). In the technical sense, we adapted our data models so they can easily convert to the VAMDC tree-structured serialization schema XSAMS.104 For BEAMDB-the database which was implemented first-we specialized a data model suited for collisional reactions, with A&M data presented with two X axes for energy and angle and one Y axis for differential cross sections. Next we implemented a photodissociation database with (radiative) processes and somewhat simplified data model which fit the needs of the MolD dataset. For the third node-ACol-with the collisional data, we took the BEAMDB data model as a base, with major revisions such as insertion of polymorphic behaviour into species and species states, which are inherited by atoms/molecules and atomic states/molecular states, respectively (shown in Fig. 5). This way, flexibility and independence is achieved regardless of type of species which participate in the process. All databases are stored on a MariaDB server (an open-source relational RDBMS system based on MySQL) with regular backups.
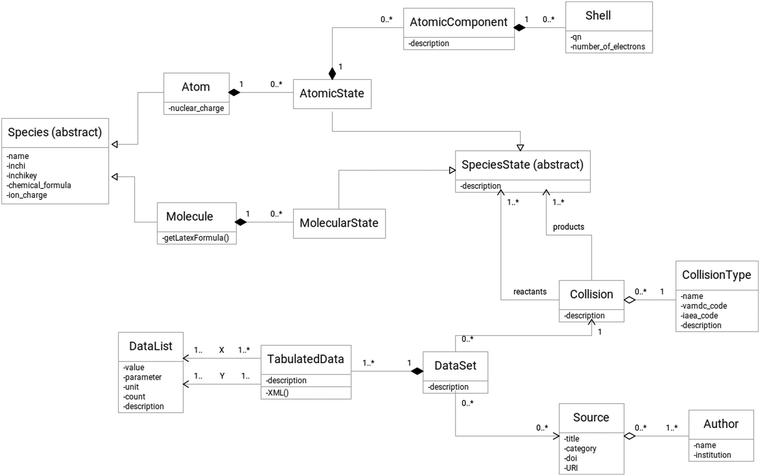 |
| Fig. 5 UML class diagram for the ACol node (logical model). | |
In a recent paper,105 the VAMDC e-infrastructure was evaluated using FAIR methodology106 made by Research Data Alliance. Using guidelines ratified half decade after the NodeSoftware was implemented, VAMDC scored satisfactory except for licence policy (this is more of a legal issue which gets complex because the international nature of the project) and provenance. As stated in the paper, the provenance shortcoming is based on the lack of implementation of an accepted community standard which would ease automation. This flaw might be critical in scenarios with data workflows, but these kind of orchestration tools aren't yet being developed.
4 Future development
4.1 Building the rare earth data base supported on the Judd–Ofelt theory using machine learning approach
The Judd–Ofelt (JO) theory107–111 is a remarkably significant theoretical scientific contribution with vast applications in chemistry, material science, and their various academic progenies. Such applications include solid-state lasers, thermal sensors, optical amplifiers, up-conversion, and various biological contexts.112 One of the main uses of JO theory in these, and indeed many other applications, is to provide a characterization of a materials optical properties. Moreover, use of JO parameters is a convenient tool for predicting materials characteristics,113–117 and we have decided to build our own database with JO parameters (henceforth described as JO Database). JO parameters can be calculated from experimentally obtained emission and excitation spectra of Rare earth doped materials.114–120 A Typical time-resolved spectrum of rare earth doped phosphor (YVO4:Eu) acquired in our measurements is shown in Fig. 6. Europium transitions are denoted, the insert shows CIE plot (color coordinate diagram) calculated from spectral data.
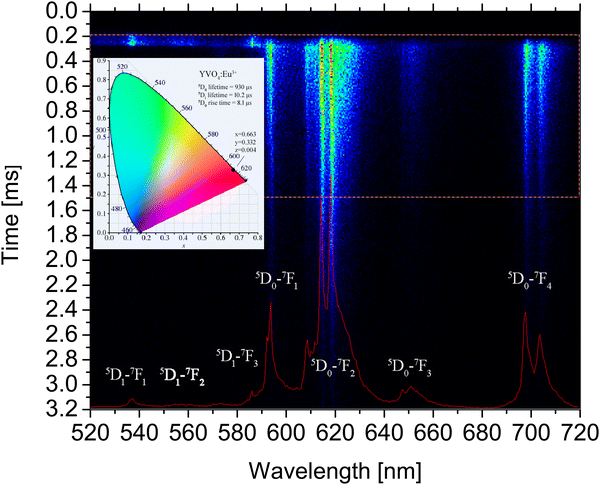 |
| Fig. 6 Typical time-resolved spectrum of rare earth doped phosphor (YVO4:Eu) acquired in our measurements. Europium transitions are denoted. Inset shows CIE plot (color coordinate diagram) calculated from spectral data. | |
There are several software applications for calculation of JO parameters, devoted to some specific cases, for example when europium is used as dopant. We will develop our software to be able to add data to JO Database for those materials which have optical spectra available in literature. Once the JO parameters are known for a specific phosphor important optical properties can be calculated. The rate of spontaneous radiative decay for an induced electric dipole (ED) and the magnetic dipole (MD) optical transitions and the resulting radiative lifetime are easy to calculate.109,111 The total lifetime can be measured experimentally and the non-radiative lifetime and quantum efficiency for emission can be determined.121
4.1.1 Machine learning approach.
Use of ML to predict optical properties of materials is still a novel approach but one that has already shown significant success and great promise. One of the main advantages it offers is that in principle it removes the need to perform difficult and expensive experiments in order to estimate the spectrum of new rare earth doped inorganic phosphors.
Phosphors with a rigid structure and hence with higher Debye temperature tend to have a low probability of nonradiative relaxation and a favorable quantum efficiency. In recently published works122,123 ML techniques have been used for predicting Debye temperatures and screening inorganic phosphor hosts. The ML model to predict Debye temperature first required training using a large, diverse set of data,122,123 obtained from available databases. However, it should be pointed out that material with high quantum yield will not necessarily have color of light useful for artificial lighting of plants or comfortable for human eye, or luminescence lifetimes appropriate for LED display or have characteristics of good thermo-phosphor. Electronic structure descriptor for the discovery of narrow-band red-emitting phosphors are studied in ref. 124 So, our research will not be restricted only to materials with high quantum yield.
ML techniques are also used in another interesting publication where it is described how to predict JO parameters based on the bulk composition in the case of Er3+ doped tellurite glasses.112 The matrix compositions were used to predict the three JO intensity parameters using support vector machine regression, coupled with sparse principal component analysis. This work is very important because it proves that it is possible to predict JO parameters using ML techniques.
In our recent publications125–127 we have used ML to estimate temperature of thermo-phosphors, plasma electron temperature and laser ablation of printed circuit boards all based on line profiles in experimentally obtained spectra. So, it seems natural to us to try to connect JO parameters with the spectral shapes of Rare earth doped materials using ML.
We can apply ML techniques to a wide class of known phosphor materials in order to predict their optical properties, improve on the existing results, extend the methods to be used for an arbitrary combination of host and rare earth dopants, build a comprehensive database of optical properties for such materials, and finally provide easy access to these results to interested users. To achieve this, we will first test and select the best performing ML algorithms and train them to predict JO parameters on a suitable subset of materials of well known optical properties, based on a set of material properties available in online materials databases. Trained algorithms can be used to predict optical properties for a wide set of materials available in materials databases for any new host represented by a list of N descriptors. This would in principle allow for an estimation of JO parameters (and hence optical properties) for a large number of as of yet unsynthetized host-dopant combinations. Such a possibility would be of great value to any researcher searching for a material with favorable optical properties, as it would remove the need to go throug a laborious and potentially costly synthesis/experiment procedure. The results obtained can be used to populate a database of JO parameters (with corresponding optical properties) for all already available materials, as well as for a large number of pregenerated host-dopant combinations.
A block diagram of our proposed building and application of JO Database is shown in Fig. 7. Our method consists of several steps. First, we construct a database containing information available directly in the literature and our previous publications.125,128–137 Where missing the JO parameters will be determined by ML. JO parameters can be used to predict quite practical characteristics of phosphors like luminescence lifetime, CIE color coordinates (color of light as seen by human eye), thermal behavior if used for remote temperature sensing, including useful range of temperature measurements and sensitivity. Other relevant data, like all basic information about structure of the material and the Debye temperature, will be also added to the JO Database. Typical characteristics of phosphors and their importance in applications are summarized in Table 1. Finally, estimates of the JO determined parameters and the optical characteristics of the materials and their possible applications will be added to JO database.
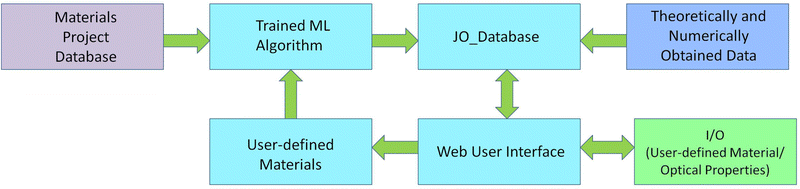 |
| Fig. 7 Building and application of JO database. | |
Table 1 Characteristics of phosphors and their importance in applications
Quantum yield |
Artificial lighting, light sources, displays |
Thermal stability |
All applications |
Intensity ratio between spectral lines |
Remote temperature sensing, color of artificial lighting, light sources, displays |
Luminescence lifetime |
Remote temperature sensing, displays, bioimaging |
Luminescence rise time |
Remote temperature sensing, bioimaging |
4.1.2 Integration of data into a new VAMDC node.
Web access to the database will be developed, featuring a user interface which will allow users to easily find any available host-dopant combination with desired optical properties (whether imported from existing material databases or one of the generated combinations). When queried for materials with certain characteristics, or combinations of characteristics, the JO Database is expected to show which material has the closest characteristics to those desired by the user. In addition, an extended functionality would allow the users to submit their own combination of a host crystal and rare-earth dopant, if it is not already included in the database.
The development of the VAMDC node for the rare earth dataset will begin with writing a data model which fits into the XSAMS tree structure. The excitation and emission spectra will be presented via Radiative process, i.e. radiative transition model, with intensities on Y axis and wavelength and time on two X (or X and Z) axes. In this transition, species (host and dopant) will have their own states which will vary on different parameters, such as excitation wavelength or concentration of dopant element.
4.2 Extension of existing BG VAMDC nodes
Besides the basic principles that govern the maintenance and curation of databases, it is always necessary to follow the current achievements in rapidly growing and innovative IT industry. Also, a paradigm of how the research itself has been conducting is constantly changing in order to provide the answers to the main societal challenges. An open science becomes a golden standard especially when the FAIR data principles (findability, accessibility, interoperability, and reusability) have been introduced.138 We share the vision of the VAMDC Consortia to provide atomic and molecular data to all researchers and stockholders via distributed databases and with a single data portal as an entry point. In that sense database upgrades and new standards include:
• Insertion of newly calculated/measured datasets; It is a constant concern of all data curators to add new datasets within databases. It is not an easy task since data need first to be evaluated (the policy is that all data must be already published and thence peer reviewed), then to add them manually and finally to check for any errors that might arise during the whole process. As this is a very slow process, it needs to be improved by automatic harvesting from other repositories. It is clearly a task that need a distinct project since data are presented with different standards and all of them has to be converted to VAMDC ones.
• Regular updating of Node Software i.e. Python software, Django (https://www.djangoproject.com/), etc. This may necessitate some adjustments to the customization of each node since upgrades are often not compatible in all APIs.
• ML & DB; Our aim is to provide available machine-readable metadata that would deliver information to interpret data in areas which are our main target of interest, such astrophysics, molecular science, radiation damage of biological targets on the molecular level, environmental science, plasma processes or lighting industry. These machine-readable metadata need to be organised according to machine-actionable reporting guidelines and models.139
• Website user interface and on-site new AJAX enabled queries and visualizations/extension; The current version of BEAMDB offers download of data only in xsams format that is not convenient to many users. So, some extension is needed that would transform datasets into usual txt or svc formats. It would also be very convenient to have a graphical representation of 3D data that are covered in the BEAMDB, yuch as differential cross sections for electron elastic scattering or electronic excitations.
5 Conclusions and perspectives
The lack of trustworthy chemical data is one of the difficult issues when modeling plasmas. The BG nodes were created for this reason. This review has summarized the current stage of development of Belgrade nodes of importance for molecular dynamics. The nodes were developed using protocols established by the Virtual Atomic and Molecular Data Centre. The nodes now face the challenge of software upgrades and continuous improvement of data processing due to the rapid expansion of online A&M services required in modern science. We have explored the possibility of extending our nodes towards building a new database of Judd–Ofelt parameters using ML to predict the optical properties of luminescent materials. These databases are expected to have an impact in many fields of physics, chemistry, and chem/phys-informatics sectors.
Author contributions
All of the authors contributed equally to this work. All authors have read and agreed to the published version of the manuscript.
Conflicts of interest
There are no conflicts to declare.
Acknowledgements
The article is based upon work from COST Action CA18212—Molecular Dynamics in the GAS phase (MD-GAS), supported by COST (European Cooperation in Science and Technology). The authors acknowledge the support from the Astronomical Observatory and Institute of Physics Belgrade, which was made possible by grants from the Ministry of Science, Technological Development and Innovations of the Republic of Serbia and the Science Fund of the Republic of Serbia under project ATMOLCOL, Atoms and (bio)molecules-dynamics and collisional processes on short time scale. Part of this work was supported by the VAMDC and the SUP@VAMDC projects funded under the ‘Combination of Collaborative Projects and Coordination and Support Actions’ Funding Scheme of The Seventh Framework Program. S. T. acknowledges support of the Science Fund of the Republic of Serbia, grant no. 7749560, Exploring ultra low global warming potential gases for insulation in high-voltage technology: Experiments and modelling-EGWIn.
References
- K. Ueda, E. Sokell, S. Schippers, F. Aumayr, H. Sadeghpour, J. Burgdörfer, C. Lemell, X.-M. Tong, T. Pfeifer, F. Calegari, A. Palacios, F. Martin, P. Corkum, G. Sansone, E. V. Gryzlova, A. N. Grum-Grzhimailo, M. N. Piancastelli, P. M. Weber, T. Steinle, K. Amini, J. Biegert, N. Berrah, E. Kukk, R. Santra, A. Müller, D. Dowek, R. R. Lucchese, C. W. McCurdy, P. Bolognesi, L. Avaldi, T. Jahnke, M. S. Schöffler, R. Dörner, Y. Mairesse, L. Nahon, O. Smirnova, T. Schlathölter, E. E. B. Campbell, J.-M. Rost, M. Meyer and K. A. Tanaka, J. Phys. B, 2019, 52, 171001 CrossRef CAS.
- M. Satta, M. C. Castrovilli, F. Nicolanti, A. R. Casavola, C. Mancini Terracciano and A. Cartoni, Condens. Matter., 2022, 7, 46 CrossRef CAS.
- M. L. Dubernet, V. Boudon, J. L. Culhane, M. S. Dimitrijevic, A. Z. Fazliev, C. Joblin, F. Kupka, G. Leto, P. Le Sidaner, P. A. Loboda, H. E. Mason, N. J. Mason, C. Mendoza, G. Mulas, T. J. Millar, L. A. Nuñez, V. I. Perevalov, N. Piskunov, Y. Ralchenko, G. Rixon, L. S. Rothman, E. Roueff, T. A. Ryabchikova, A. Ryabtsev, S. Sahal-Bréchot, B. Schmitt, S. Schlemmer, J. Tennyson, V. G. Tyuterev, N. A. Walton, V. Wakelam and C. J. Zeippen, J. Quant. Spectrosc. Radiat. Transf., 2010, 111, 2151–2159 CrossRef CAS.
- K. Hansen, Mass Spectrom. Rev., 2021, 40, 725–740 CrossRef PubMed.
- P. Sneha and C. G. P. Doss, Adv. Protein Chem. Struct. Biol., 2016, 102, 181–224 CAS.
- E. Seperuelo Duarte, A. Domaracka, P. Boduch, H. Rothard, E. Dartois and E. F. da Silveira, Astron. Astrophys., 2010, 512, A71 CrossRef.
-
A. G. Tielens, Molecular astrophysics, Cambridge University Press, 2021 Search PubMed.
-
M. Rengel, arXiv e-prints, 2023, arXiv:2302.05452.
- H. Zettergren, A. Domaracka, T. Schlathölter, P. Bolognesi, S. Daz-Tendero, M. Łabuda, S. Tosic, S. Maclot, P. Johnsson, A. Steber, D. Tikhonov, M. C. Castrovilli, L. Avaldi, S. Bari, A. R. Milosavljević, A. Palacios, S. Faraji, D. G. Piekarski, P. Rousseau, D. Ascenzi, C. Romanzin, E. Erdmann, M. Alcam, J. Kopyra, P. Limão-Vieira, J. Koćišek, J. Fedor, S. Albertini, M. Gatchell, H. Cederquist, H. T. Schmidt, E. Gruber, L. H. Andersen, O. Heber, Y. Toker, K. Hansen, J. A. Noble, C. Jouvet, C. Kjær, S. B. Nielsen, E. Carrascosa, J. Bull, A. Candian and A. Petrignani, Eur. Phys. J. D, 2021, 75, 152 CrossRef CAS.
- H. Yanar, O. Aydoğdu and M. Salt, Molecular Physics, 2016, 114, 3134–3142 CrossRef CAS.
- A. N. Ikot, E. Chukwuocha, M. Onyeaju, C. Onate, B. Ita and M. Udoh, Pramana, 2018, 90, 22 CrossRef.
- F. Iacob, Phys. Lett. A, 2020, 384, 126888 CrossRef CAS.
-
L. G. Christophorou, Electron-molecule Interactions and Their Applications, Academic Press, 2013, vol. 2 Search PubMed.
- P. Limão-Vieira, F. Blanco, J. C. Oller, A. Muñoz, J. M. Pérez, M. Vinodkumar, G. Garca and N. J. Mason, Phys. Rev. A, 2005, 71, 032720 CrossRef.
- S. Dujko, J. Atić, D. Bošnjaković, R. D. White, P. Stokes, K. R. Hamilton, O. Zatsarinny, K. Bartschat, M. S. Rabasović, D. Šević, B. P. Marinković, D. V. Fursa, I. Bray, R. P. McEachran, F. Blanco, G. Garca, D. B. Jones, L. Campbell and M. J. Brunger, Plasma Sources Sci. Technol., 2021, 30, 115019 CrossRef CAS.
- K. Ebel and I. Bald, J. Phys. Chem. Lett., 2022, 13, 4871–4876 CrossRef CAS PubMed.
- R. Srivastava and D. V. Fursa, Atoms, 2023, 11, 31 CrossRef.
- K. Bartschat and M. J. Kushner, Proc. Natl. Acad. Sci. U. S. A., 2016, 113, 7026–7034 CrossRef CAS PubMed.
- S. Schippers, E. Sokell, F. Aumayr, H. Sadeghpour, K. Ueda, I. Bray, K. Bartschat, A. Murray, J. Tennyson, A. Dorn, M. Yamazaki, M. Takahashi, N. Mason, O. Novotný, A. Wolf, L. Sanche, M. Centurion, Y. Yamazaki, G. Laricchia, C. M. Surko, J. Sullivan, G. Gribakin, D. W. Savin, Y. Ralchenko, R. Hoekstra and G. O’Sullivan, J. Phys. B, 2019, 52, 171002 CrossRef CAS.
- H. Weisen, I. Furno and T. C. V. Team, Nucl. Fusion, 2001, 41, 1227–1233 CrossRef CAS.
- S.-T. Jung, S.-H. Pyo, W.-G. Kang, Y.-R. Kim, J.-K. Kim, C. M. Kang, Y.-C. Nho and J.-S. Park, Radiat. Phys. Chem., 2021, 186, 109506 CrossRef CAS.
- W. Liu, D. Liu, Y. Zhang and B. Li, Powder Technol., 2022, 407, 117616 CrossRef CAS.
- P. Bolognesi and L. Avaldi, Phys. Chem. Chem. Phys., 2022, 24, 22356–22370 RSC.
- O. Novotný, P. Wilhelm, D. Paul, Á. Kálosi, S. Saurabh, A. Becker, K. Blaum, S. George, J. Göck, M. Grieser, F. Grussie, R. von Hahn, C. Krantz, H. Kreckel, C. Meyer, P. M. Mishra, D. Muell, F. Nuesslein, D. A. Orlov, M. Rimmler, V. C. Schmidt, A. Shornikov, A. S. Terekhov, S. Vogel, D. Zajfman and A. Wolf, Science, 2019, 365, 676–679 CrossRef PubMed.
- R. J. MacDonald and N. K. Lewis, Astrophys. J., 2022, 929, 20 CrossRef.
- M. G. Wolfire, L. Vallini and M. Chevance, Annu. Rev. Astron. Astrophys., 2022, 60, 247–318 CrossRef.
- M. H. Stockett, J. N. Bull, H. Cederquist, S. Indrajith, M. Ji, J. E. Navarro Navarrete, H. T. Schmidt, H. Zettergren and B. Zhu, Nat. Commun., 2023, 14, 395 CrossRef CAS PubMed.
- S. Samukawa, M. Hori, S. Rauf, K. Tachibana, P. Bruggeman, G. Kroesen, J. C. Whitehead, A. B. Murphy, A. F. Gutsol, S. Starikovskaia, U. Kortshagen, J.-P. Boeuf, T. J. Sommerer, M. J. Kushner, U. Czarnetzki and N. Mason, J. Phys. D, 2012, 45, 253001 CrossRef.
- N. J. Mason, Astron. Geophys., 2009, 50, 3–32 CrossRef.
- O. Hamdy, Z. Abdel-Salam and M. Abdel-Harith, Diagnostics, 2022, 12, 1–14 CrossRef PubMed.
- C. Couteau, S. Barz, T. Durt, T. Gerrits, J. Huwer, R. Prevedel, J. Rarity, A. Shields and G. Weihs, Nat. Rev. Phys., 2023, 1–13 Search PubMed.
- M. Gatchell and H. Zettergren, Commun. Chem., 2022, 5, 28 CrossRef PubMed.
- J. B. Maljković, F. Blanco, R. Čurík, G. García, B. P. Marinković and A. R. Milosavljević, J. Chem. Phys., 2012, 137, 064312 CrossRef PubMed.
- M. L. Dubernet, B. K. Antony, Y. A. Ba, Y. L. Babikov, K. Bartschat, V. Boudon, B. J. Braams, H.-K. Chung, F. Daniel, F. Delahaye, G. D. Zanna, J. de Urquijo, M. S. Dimitrijević, A. Domaracka, M. Doronin, B. J. Drouin, C. P. Endres, A. Z. Fazliev, S. V. Gagarin, I. E. Gordon, P. Gratier, U. Heiter, C. Hill, D. Jevremović, C. Joblin, A. Kasprzak, E. Krishnakumar, G. Leto, P. A. Loboda, T. Louge, S. Maclot, B. P. Marinković, A. Markwick, T. Marquart, H. E. Mason, N. J. Mason, C. Mendoza, A. A. Mihajlov, T. J. Millar, N. Moreau, G. Mulas, Y. Pakhomov, P. Palmeri, S. Pancheshnyi, V. I. Perevalov, N. Piskunov, J. Postler, P. Quinet, E. Quintas-Sánchez, Y. Ralchenko, Y.-J. Rhee, G. Rixon, L. S. Rothman, E. Roueff, T. Ryabchikova, S. Sahal-Bréchot, P. Scheier, S. Schlemmer, B. Schmitt, E. Stempels, S. Tashkun, J. Tennyson, V. G. Tyuterev, V. Vujćić, V. Wakelam, N. A. Walton, O. Zatsarinny, C. J. Zeippen and C. M. Zwölf, J. Phys. B, 2016, 49, 074003 CrossRef.
- A. L. Bail, Phys. Chem. Chem. Phys., 2010, 12, 8521 RSC.
- D. Albert, B. K. Antony, Y. A. Ba, Y. L. Babikov, P. Bollard, V. Boudon, F. Delahaye, G. Del Zanna, M. S. Dimitrijević, B. J. Drouin, M.-L. Dubernet, F. Duensing, M. Emoto, C. P. Endres, A. Z. Fazliev, J.-M. Glorian, I. E. Gordon, P. Gratier, C. Hill, D. Jevremović, C. Joblin, D.-H. Kwon, R. V. Kochanov, E. Krishnakumar, G. Leto, P. A. Loboda, A. A. Lukashevskaya, O. M. Lyulin, B. P. Marinković, A. Markwick, T. Marquart, N. J. Mason, C. Mendoza, T. J. Millar, N. Moreau, S. V. Morozov, T. Möller, H. S. P. Müller, G. Mulas, I. Murakami, Y. Pakhomov, P. Palmeri, J. Penguen, V. I. Perevalov, N. Piskunov, J. Postler, A. I. Privezentsev, P. Quinet, Y. Ralchenko, Y.-J. Rhee, C. Richard, G. Rixon, L. S. Rothman, E. Roueff, T. Ryabchikova, S. Sahal-Bréchot, P. Scheier, P. Schilke, S. Schlemmer, K. W. Smith, B. Schmitt, I. Y. Skobelev, V. A. Srecković, E. Stempels, S. A. Tashkun, J. Tennyson, V. G. Tyuterev, C. Vastel, V. Vujćić, V. Wakelam, N. A. Walton, C. Zeippen and C. M. Zwölf, Atoms, 2020, 8, 76 CrossRef CAS.
- P. Morgante and R. Peverati, Phys. Chem. Chem. Phys., 2019, 21, 19092–19103 RSC.
- F. Aumayr, H. Sadeghpour, J. Burgdörfer, C. Lemell, X.-M. Tong and T. Pfeifer, J. Phys. B, 2019, 52, 62 CrossRef.
-
R. Anirudh, R. Archibald, M. Salman Asif, M. M. Becker, S. Benkadda, P.-T. Bremer, R. H. S. Budé, C. S. Chang, L. Chen, R. M. Churchill, J. Citrin, J. A. Gaffney, A. Gainaru, W. Gekelman, T. Gibbs, S. Hamaguchi, C. Hill, K. Humbird, S. Jalas, S. Kawaguchi, G.-H. Kim, M. Kirchen, S. Klasky, J. L. Kline, K. Krushelnick, B. Kustowski, G. Lapenta, W. Li, T. Ma, N. J. Mason, A. Mesbah, C. Michoski, T. Munson, I. Murakami, H. N. Najm, K. E. J. Olofsson, S. Park, J. L. Peterson, M. Probst, D. Pugmire, B. Sammuli, K. Sawlani, A. Scheinker, D. P. Schissel, R. J. Shalloo, J. Shinagawa, J. Seong, B. K. Spears, J. Tennyson, J. Thiagarajan, C. M. Ticos, J. Trieschmann, J. van Dijk, B. Van Essen, P. Ventzek, H. Wang, J. T. L. Wang, Z. Wang, K. Wende, X. Xu, H. Yamada, T. Yokoyama and X. Zhang, arXiv, e-prints, 2022, arXiv:2205.15832.
- L. C. Pitchford, L. L. Alves, K. Bartschat, S. F. Biagi, M.-C. Bordage, I. Bray, C. E. Brion, M. J. Brunger, L. Campbell, A. Chachereau, B. Chaudhury, L. G. Christophorou, E. Carbone, N. A. Dyatko, C. M. Franck, D. V. Fursa, R. K. Gangwar, V. Guerra, P. Haefliger, G. J. M. Hagelaar, A. Hoesl, Y. Itikawa, I. V. Kochetov, R. P. McEachran, W. L. Morgan, A. P. Napartovich, V. Puech, M. Rabie, L. Sharma, R. Srivastava, A. D. Stauffer, J. Tennyson, J. de Urquijo, J. van Dijk, L. A. Viehland, M. C. Zammit, O. Zatsarinny and S. Pancheshnyi, Plasma Processes Polym., 2017, 14, 1600098 CrossRef.
-
V. Cvjetković, B. Marinković and D. Šević, Advances and Innovations in Systems, Computing Sciences and Software Engineering, Dordrecht, 2007, pp. 485–490 Search PubMed.
- B. P. Marinković, V. Vujćić, G. Sushko, D. Vudragović, D. B. Marinković, S. Đordević, S. Ivanović, M. Nešić, D. Jevremović, A. V. Solovyov and N. J. Mason, Nucl. Instrum. Methods Phys. Res. B, 2015, 354, 90–95 CrossRef.
- S. Denifl, G. Garcia, B. A. Huber, B. P. Marinkovi, N. Mason, J. Postler, H. Rabus, G. Rixon, A. V. Solov'yov, E. Suraud and A. V. Yakubovich, J. Phys. Conf. Ser., 2013, 438, 012016 CrossRef CAS.
- B. P. Marinković, D. Jevremović, V. A. Srećković, V. Vujćić, L. M. Ignjatović, M. S. Dimitrijević and N. J. Mason, Eur. Phys. J. D, 2017, 71, 158 CrossRef.
- V. Srećković, L. Ignjatović, D. Jevremović, V. Vujćić and M. Dimitrijević, Atoms, 2017, 5, 31 CrossRef.
- B. P. Marinković, J. H. Bredehöft, V. Vujćić, D. Jevremović and N. J. Mason, Atoms, 2017, 5, 46 CrossRef.
- C. E. DeForest, W. H. Matthaeus, T. A. Howard and D. R. Rice, Astrophys. J., 2015, 812, 108 CrossRef.
- G. Clark, T. W. Broiles, J. L. Burch, G. A. Collinson, T. Cravens, R. A. Frahm, J. Goldstein, R. Goldstein, K. Mandt, P. Mokashi, M. Samara and C. J. Pollock, Astron. Astrophys., 2015, 583, A24 CrossRef.
- J. Deca, A. Divin, P. Henri, A. Eriksson, S. Markidis, V. Olshevsky and M. Horányi, Phys. Rev. Lett., 2017, 118, 205101 CrossRef.
- J. Vukalović, J. B. Maljković, K. Tökési, B. Predojević and B. P. Marinković, Int. J. Mol. Sci., 2021, 22, 647 CrossRef PubMed.
- S. K. Srivastava, A. Chutjian and S. Trajmar, J. Chem. Phys., 2008, 63, 2659–2665 CrossRef.
- M. L. Ranković, J. B. Maljković, K. Tökési and B. P. Marinković, Eur. Phys. J. D, 2018, 72, 30 CrossRef.
-
C. B. Lucas, Atomic and molecular beams: production and collimation, CRC press, 2013 Search PubMed.
- V. A. Srećković, M. S. Dimitrijević and L. M. Ignjatović, Mon. Not. R. Astron. Soc., 2018, 480, 5078–5083 Search PubMed.
- B. P. Marinković, V. Pejćev, D. M. Filipović and L. Vušković, J. Phys. B, 1991, 24, 1817 CrossRef.
-
B. P. Marinković, V. Pejćev, D. M. Filipović, I. Čadež and L. Vušković, J. Phys. B, 1992, 25, 5179 Search PubMed.
- E. N. Lassettre, A. Skerbele and M. A. Dillon, J. Chem. Phys., 2003, 50, 1829–1839 CrossRef.
- R. Panajotovic, V. Pejcev, M. Konstantinovic, D. Filipovic, V. Bocvarski and B. Marinkovic, J. Phys. B, 1993, 26, 1005 CrossRef CAS.
- N. B. Avdonina, Z. Felfli and A. Z. Msezane, J. Phys. B, 1997, 30, 2591 CrossRef CAS.
- R. Panajotović, D. Šević, V. Pejćev, D. Filipović and B. Marinković, Int. J. Mass Spectrom., 2004, 233, 253–257 CrossRef.
- B. Marinković, V. Pejćev, D. Filipović, D. Šević, S. Milisavljević and B. Predojević, Radiat. Phys. Chem., 2007, 76, 455–460 CrossRef.
- B. P. Marinković, R. P. McEachran, D. V. Fursa, I. Bray, H. Umer, F. Blanco, G. García, M. J. Brunger, L. Campbell and D. B. Jones, J. Phys. Chem. Ref. Data, 2023, 52, 023102 CrossRef.
- G. Senn, J. D. Skalny, A. Stamatovic, N. J. Mason, P. Scheier and T. D. Märk, Phys. Rev. Lett., 1999, 82, 5028–5031 CrossRef CAS.
- M. Allan, K. R. Asmis, D. B. Popović, M. Stepanović, N. J. Mason and J. A. Davies, J. Phys. B, 1996, 29, 4727 CrossRef CAS.
- F. Motte-Tollet, G. Eustatiu and D. Roy, J. Chem. Phys., 1996, 105, 7448–7453 CrossRef CAS.
- A. Prosvetov, A. V. Verkhovtsev, G. Sushko and A. V. Solovyov, Beilstein J. Nanotechnol., 2021, 12, 1151–1172 CrossRef CAS PubMed.
- M. Baranowski, R. Sachser, B. P. Marinković, S. D. Ivanović and M. Huth, Nanomaterials, 2022, 12, 4145 CrossRef CAS PubMed.
- S. W. Crane, J. W. Lee, M. N. Ashfold and D. Rolles, Phys. Chem. Chem. Phys., 2023, 25, 16672–16698 RSC.
- T. Studemund, K. Pollow, M. Förstel and O. Dopfer, Phys. Chem. Chem. Phys., 2023, 25, 17609–17618 RSC.
- D. J. Hillier, Atoms, 2023, 11, 54 CrossRef CAS.
- J. Fontenla, E. Avrett, G. Thuillier and J. Harder, Astrophys. J., 2006, 639, 441 CrossRef CAS.
- A. Giuliani, A. R. Milosavljević, F. Canon and L. Nahon, Mass Spectrom. Rev., 2014, 33, 424–441 CrossRef CAS PubMed.
- A. G. G. M. Tielens, Rev. Mod. Phys., 2013, 85, 1021–1081 CrossRef CAS.
- V. Vujčič, D. Jevremović, A. Mihajlov, L. M. Ignjatović, V. Srećković and M. Dimitrijević, J. Astrophys. Astron., 2015, 36, 693–703 Search PubMed.
- V. A. Srećković, L. M. Ignjatović and M. S. Dimitrijević, Molecules, 2020, 26, 151 CrossRef PubMed.
- A. Mihajlov, L. M. Ignjatović, N. Sakan and M. Dimitrijević, Astron. Astrophys., 2007, 469, 749–754 CrossRef CAS.
- K. Sugimura, C. M. Coppola, K. Omukai, D. Galli and F. Palla, Mon. Not. R. Astron. Soc., 2016, 456, 270–277 CrossRef CAS.
- Z. Majlinger, M. S. Dimitrijević and V. A. Srećković, Data, 2020, 5, 74 CrossRef.
- J. Fontenla, W. Curdt, M. Haberreiter, J. Harder and H. Tian, Astrophys. J., 2009, 707, 482 CrossRef CAS.
- A. Lindblad, J. Söderström, C. Nicolas, E. Robert and C. Miron, Rev. Sci. Instrum., 2013, 84, 113105–113105-11 CrossRef PubMed.
- A. R. Milosavljević, C. Nicolas, J. Lemaire, C. Dehon, R. Thissen, J.-M. Bizau, M. Réfrégiers, L. Nahon and A. Giuliani, Phys. Chem. Chem. Phys., 2011, 13, 15432–15436 RSC.
-
A. R. Milosavljević, A. Giuliani and C. Nicolas, in X-ray and Neutron Techniques for Nanomaterials Characterization, ed. C. S. S. R. Kumar, Springer, 2016, p. 451 Search PubMed.
- V. A. Srećković, L. M. Ignjatović, A. Kolarski, Z. R. Mijić, M. S. Dimitrijević and V. Vujčić, Data, 2022, 7, 129 CrossRef.
- M. L. Ranković, V. Cerovski, F. Canon, L. Nahon, A. Giuliani and A. R. Milosavljević, J. Phys.: Conf. Ser., 2015, 112030 CrossRef.
- R. Beuc, M. Movre and B. Horvatić, Eur. Phys. J. D, 2014, 68, 1–8 CrossRef.
- I. Adamovich, S. D. Baalrud, A. Bogaerts, P. J. Bruggeman, M. Cappelli, V. Colombo, U. Czarnetzki, U. Ebert, J. G. Eden, P. Favia, D. B. Graves, S. Hamaguchi, G. Hieftje, M. Hori, I. D. Kaganovich, U. Kortshagen, M. J. Kushner, N. J. Mason, S. Mazouffre, S. Mededovic Thagard, H. R. Metelmann, A. Mizuno, E. Moreau, A. B. Murphy, B. A. Niemira, G. S. Oehrlein, Z. L. Petrovic, L. C. Pitchford, Y. K. Pu, S. Rauf, O. Sakai, S. Samukawa, S. Starikovskaia, J. Tennyson, K. Terashima, M. M. Turner, M. C. M. van de Sanden and A. Vardelle, J. Phys. D, 2017, 50, 323001 CrossRef.
- S. Niyonzima, N. Pop, F. Iacob, Å. Larson, A. E. Orel, J. Z. Mezei, K. Chakrabarti, V. Laporta, K. Hassouni, D. Benredjem, A. Bultel, J. Tennyson, D. Reiter and I. F. Schneider, Plasma Sources Sci. Technol., 2018, 27, 025015 CrossRef.
- N. Pop, F. Iacob, J. Z. Mezei, O. Motapon, S. Niyonzima, D. O. Kashinski, D. Talbi, A. P. Hickman and I. F. Schneider, TIM17 Physics Conference, AIP Conf. Proc., 2017, 020013 Search PubMed.
- N. Pop, F. Iacob, S. Niyonzima, A. Abdoulanziz, V. Laporta, D. Reiter, I. Schneider and J. Mezei, At. Data Nucl. Data Tables, 2021, 139, 101414 CrossRef CAS.
- F. Iacob, N. Pop, J. Z. Mezei, S. Niyonzima, V. Laporta, K. Chakrabarti and I. F. Schneider, AIP Conf. Proc., 2020, 050010 CrossRef CAS.
- N. Pop, F. Iacob, J. Z. Mezei, S. Niyonzima, O. Motapon and I. F. Schneider, J. Phys.: Conf. Ser., 2020, 172004 CrossRef.
- L. L. Alves, M. M. Becker, J. van Dijk, T. Gans, D. B. Go, K. Stapelmann, J. Tennyson, M. M. Turner and M. J. Kushner, Plasma Sources Sci. Technol., 2023, 32, 023001 CrossRef.
- P. Attri, K. Ishikawa, T. Okumura, K. Koga and M. Shiratani, Processes, 2020, 8, 1–20 CrossRef.
- F. Iacob, T. Meltzer, J. Z. Mezei, I. F. Schneider and J. Tennyson, J. Phys. B: At., Mol. Opt. Phys., 2022, 55, 235202 CrossRef CAS.
- Y. Zambon, N. Contaldo, R. Laurita, E. Várallyay, A. Canel, M. Gherardi, V. Colombo and A. Bertaccini, Sci. Rep., 2020, 10, 19211 CrossRef CAS PubMed.
- A. Shaw, G. Shama and F. Iza, Biointerphases, 2015, 10, 25 CrossRef PubMed.
- I. Adamovich, S. Agarwal, E. Ahedo, L. L. Alves, S. Baalrud, N. Babaeva, A. Bogaerts, A. Bourdon, P. J. Bruggeman, C. Canal, E. H. Choi, S. Coulombe, Z. Donkó, D. B. Graves, S. Hamaguchi, D. Hegemann, M. Hori, H. H. Kim, G. M. W. Kroesen, M. J. Kushner, A. Laricchiuta, X. Li, T. E. Magin, S. Mededovic Thagard, V. Miller, A. B. Murphy, G. S. Oehrlein, N. Puac, R. M. Sankaran, S. Samukawa, M. Shiratani, M. Šimek, N. Tarasenko, K. Terashima, J. Thomas, J. Trieschmann, S. Tsikata, M. M. Turner, I. J. van der Walt, M. C. M. van de Sanden and T. von Woedtke, J. Phys. D, 2022, 55, 373001 CrossRef.
- N. Puač, M. Gherardi and M. Shiratani, Plasma Process Polym., 2018, 2, 1–5 Search PubMed.
- M. Gherardi, N. Puač and M. Shiratani, Plasma Process Polym., 2018, 15, 1877002 CrossRef.
-
J. Trieschmann, L. Vialetto and T. Gergs, arXiv e-prints, 2023, arXiv:2307.00131.
- A. Mihajlov, V. Srećković, L. M. Ignjatović and A. Klyucharev, J. Clust. Sci., 2012, 23, 47–75 CrossRef CAS.
- A. A. Mihajlov, L. M. Ignjatović, V. A. Srećković and M. S. Dimitrijević, Astrophys. J., Suppl. Ser., 2011, 193, 2 CrossRef.
- V. Srećković, M. Dimitrijević, L. Ignjatović, N. Bezuglov and A. Klyucharev, Galaxies, 2018, 6, 72 CrossRef.
-
C. Hill, M. Doronin, T. Marquart, D. Roueff, H. Chung, B. Braams, R. Gagarin and G. Rixon, VAMDC-XSAMS Reference Guide, 2011.
- C. M. Zwölf and N. Moreau, Eur. Phys. J. D, 2023, 77, 70 CrossRef.
- M. D. Wilkinson, M. Dumontier, I. J. Aalbersberg, G. Appleton, M. Axton, A. Baak, N. Blomberg, J.-W. Boiten, L. B. da Silva Santos and P. E. Bourne,
et al.
, Sci. Data, 2016, 3, 1–9 Search PubMed.
- B. R. Judd, Phys. Rev., 1962, 127, 750–761 CrossRef CAS.
- G. S. Ofelt, J. Chem. Phys., 1962, 37, 511–520 CrossRef CAS.
- M. P. Hehlen, M. G. Brik and K. W. Krämer, J. Lumin., 2013, 136, 221–239 CrossRef CAS.
-
L. Smentek, Judd-Ofelt Theory — The Golden (and the Only One) Theoretical Tool of f-Electron Spectroscopy, John Wiley & Sons, 2015 Search PubMed.
-
B. M. Walsh, Advances in Spectroscopy for Lasers and Sensing, Dordrecht, 2006, pp. 403–433 Search PubMed.
- M. Konstantinidis, E. A. Lalla, G. Lopez-Reyes, U. R. Rodrguez-Mendoza, E. A. Lymer, J. Freemantle and M. G. Daly, J. Lumin., 2021, 235, 118020 CrossRef CAS.
- A. Ćirić, Ł. Marciniak and M. D. Dramićanin, Sci. Rep., 2022, 12, 563 CrossRef PubMed.
- A. Martinović, M. D. Dramićanin and A. Ćirić, Adv. Theory Simul., 2022, 5, 2200029 CrossRef.
- K. V. Rao, S. Babu, J. Venkataiah and Y. C. Ratnakaram, J. Mol. Struct., 2015, 1094, 274–280 CrossRef.
- Y. Yang, C. Mi, F. Jiao, X. Su, X. Li, L. Liu, J. Zhang, F. Yu, Y. Liu and Y. Mai, J. Am. Ceram. Soc., 2014, 97, 1769–1775 CrossRef CAS.
- M. H. V. Werts, R. T. F. Jukes and J. W. Verhoeven, Phys. Chem. Chem. Phys., 2002, 4, 1542–1548 RSC.
- M. A. Makram, J. Khaliel, D. A. Rayan, H. Ayoub and Y. H. Elbashar, J. Opt., 2023, 52, 10–15 CrossRef.
- A. Ćirić, S. Stojadinović and M. D. Dramićanin, Opt. Mater., 2018, 85, 261–266 CrossRef.
- Y. A. Yamusa, Y. Musa, T. Muhammad, A. Sa'id, A. Isma'ila, A. U. Abubakar and I. Bulus, Optik, 2022, 267, 169710 CrossRef CAS.
- M. J. Weber, Phys. Rev., 1967, 157, 262–272 CrossRef CAS.
- S. Li and R.-J. Xie, J. Solid State Sci. Technol., 2020, 9, 016013 CrossRef CAS.
- Y. Zhou, A. Mansouri Tehrani, A. Olinyk, A. C. Duke and J. Brgoch, Nat. Commun., 2018, 9, 4377 CrossRef PubMed.
-
Z. Wang, I.-H. Chu, F. Zhou and S. P. Ong, arXiv e-prints, 2016, arXiv:1604.04581.
- D. Sevic, J. Krizan, M. S. Rabasovic and B. P. Marinkovic, Eur. Phys. J. D, 2021, 75, 56 CrossRef CAS.
- M. S. Rabasovic, B. P. Marinkovic and D. Sevic, Adv. Space Res., 2023, 71, 1331–1337 CrossRef.
- M. S. Rabasovic, B. P. Marinkovic and D. Sevic, Publ. Astron. Obs. Belgrade, 2022, 102, 219–222 Search PubMed.
- A. Vlasic, D. Sevic, M. S. Rabasovic, J. Krizan, S. Savic-Sevic, M. D. Rabasovic, M. Mitric, B. P. Marinkovic and M. G. Nikolic, J. Lumin., 2018, 199, 285–292 CrossRef CAS.
- D. Sevic, M. S. Rabasovic, J. Krizan, S. Savic-Sevic, B. P. Marinkovic and M. G. Nikolic, Opt. Quant. Electron., 2022, 54, 523 CrossRef CAS.
- M. G. Nikolic, M. S. Rabasovic, J. Krizan, S. Savic-Sevic, M. D. Rabasovic, B. P. Marinkovic, A. Vlasic and D. Sevic, Opt. Quant. Electron, 2018, 50, 258 CrossRef.
- M. S. Rabasovic, J. Krizan, S. Savic-Sevic, M. Mitric, M. D. Rabasovic, B. P. Marinkovic and D. Sevic, J. Spectroscopy, 2018, 3413864 Search PubMed.
- M. S. Rabasovic, J. Krizan, P. Gregorcic, M. D. Rabasovic, N. Romcevic and D. Sevic, Opt. Quantum Electron., 2016, 48, 163 CrossRef.
- M. S. Rabasovic, D. Sevic, J. Krizan, M. D. Rabasovic, S. Savic-Sevic, M. Mitric, M. Petrovic, M. Gilic and N. Romcevic, Opt. Mater., 2015, 50, 250–255 CrossRef CAS.
- M. S. Rabasovic, D. Sevic, J. Krizan, M. Terzic, J. Mozina, B. P. Marinkovic, S. Savic-Sevic, M. Mitric, M. D. Rabasovic and N. Romcevic, J. Alloys Compd., 2014, 662, 292–295 Search PubMed.
- D. Sevic, M. S. Rabasovic, J. Krizan, S. Savic-Sevic, B. P. Rabasovic, M. D. Marinkovic and M. G. Nikolic, Opt. Quant. Electron., 2022, 52, 232 CrossRef.
- D. Sevic, M. S. Rabasovic, J. Krizan, S. Savic-Sevic, M. G. Nikolic, B. P. Marinkovic and M. D. Rabasovic, J. Phys. D, 2019, 53, 015106 CrossRef.
- D. Sevic, M. S. Rabasovic, J. Krizan, S. Savic-Sevic, M. Mitric, M. Gilic, B. Hadzic and N. Romcevic, Mater. Res. Bull., 2017, 88, 121–126 CrossRef CAS.
-
M. D. Wilkinson, M. Dumontier, I. J. Aalbersberg, G. Appleton, M. Axton, A. Baak, N. Blomberg, J.-W. Boiten, L. B. da Silva Santos, P. E. Bourne, J. Bouwman, A. J. Brookes, T. Clark, M. Crosas, I. Dillo, O. Dumon, S. Edmunds, C. T. Evelo, R. Finkers, A. Gonzalez-Beltran, A. J. Gray, P. Groth, C. Goble, J. S. Grethe, J. Heringa, P. A. t Hoen, R. Hooft, T. Kuhn, R. Kok, J. Kok, S. J. Lusher, M. E. Martone, A. Mons, A. L. Packer, B. Persson, P. Rocca-Serra, M. Roos, R. van Schaik, S.-A. Sansone, E. Schultes, T. Sengstag, T. Slater, G. Strawn, M. A. Swertz, M. Thompson, J. van der Lei, E. van Mulligen, J. Velterop, A. Waagmeester, P. Wittenburg, K. Wolstencroft, J. Zhao and B. Mons, Sci. Data, 2016, 3, 160018 Search PubMed.
- D. Batista, A. Gonzalez-Beltran, S.-A. Sansone and P. Rocca-Serra, Sci. Data, 2022, 9, 592 CrossRef PubMed.
Footnote |
† https://servo.aob.rs/mold/ |
|
This journal is © the Owner Societies 2023 |
Click here to see how this site uses Cookies. View our privacy policy here.