DOI:
10.1039/D3NH00510K
(Communication)
Nanoscale Horiz., 2024,
9, 799-816
Sources of biases in the in vitro testing of nanomaterials: the role of the biomolecular corona†
Received
19th November 2023
, Accepted 12th March 2024
First published on 13th March 2024
Abstract
The biological fate of nanomaterials (NMs) is driven by specific interactions through which biomolecules, naturally adhering onto their surface, engage with cell membrane receptors and intracellular organelles. The molecular composition of this layer, called the biomolecular corona (BMC), depends on both the physical–chemical features of the NMs and the biological media in which the NMs are dispersed and cells grow. In this work, we demonstrate that the widespread use of 10% fetal bovine serum in an in vitro assay cannot recapitulate the complexity of in vivo systemic administration, with NMs being transported by the blood. For this purpose, we undertook a comparative journey involving proteomics, lipidomics, high throughput multiparametric in vitro screening, and single molecular feature analysis to investigate the molecular details behind this in vivo/in vitro bias. Our work indirectly highlights the need to introduce novel, more physiological-like media closer in composition to human plasma to produce realistic in vitro screening data for NMs. We also aim to set the basis to reduce this in vitro–in vivo mismatch, which currently limits the formulation of NMs for clinical settings.
New concepts
We explored the molecular and biological biases arising from the well-known in vitro/in vivo mismatch in nanomedicine due to the biomolecular corona, the well-accepted biological identity of nanomaterials (NMs) formed in different biological scenarios. The cell culture media where NMs are dispersed (supplemented with 10% fetal bovine serum, FBS) significantly diverge from the in vivo biological fluids encountered by NMs in a systemic administration scenario. This means that the biomolecular corona will have a remarkably different profile when formed in vivo. This problem has never been tackled because of compliance with conventional cell culture protocols and the challenge of growing healthy cells in plasma-supplemented media. It is now necessary for the bio-nanocommunity to firmly acknowledge this issue. Our work highlights biases in bio-nanointeractions in in vitro studies arising from this mismatch and defines a new starting point to re-tune the in vitro readouts to obtain a more realistic prediction of the putative in vivo behavior of NMs. Our advanced data analysis also demonstrates how omics data can be used to describe and address this bias in a material-specific way, thus opening up the desirable application of machine learning to large data sets of NM safety data.
|
Introduction
The ever-growing role of nanotechnology in developing novel therapeutic and diagnostic technologies often involves the systemic administration of nanomaterials (NMs). Once NMs enter the bloodstream, they interact with a dynamic biological environment populated by a variety of biomolecules (proteins, lipids, sugars, metabolites, exogenous compounds, etc.) that adsorb onto the material's surface. This process, known as “coronation”,1 is driven by the physical–chemical characteristics of the NMs and the ratio between the exposed NM surface and the local concentration of biomolecules. The formed biomolecular corona (BMC) defines the biological identity of the NMs and drives their engagement with the biological machinery2–7 in terms of anatomical distribution,8 cytotoxicity,9 and cell uptake.10–13 Given our ability to manufacture a plethora of different nano-formulations for diverse medical applications, robust and shared protocols for in vitro screening are essential but, unfortunately, relatively overlooked. Based on current literature, toxicity assessment of NMs has been performed on many different cell lines by dispersing the NMs in the medium used for cell growth, which is commonly supplemented with 10% of fetal bovine serum (FBS). This practice was introduced in the 1950s, and since then, FBS has been used as a universal supplement in culture media for human and animal cells in research and pharmaceutical settings.14 This clearly entails an immediate unsuitable matching of cells and media for human settings. Only a few attempts have been made so far to break tradition, considering that particular applications and cellular experiments might require a more specific biological environment, not consistently recapitulated well by the use of FBS.15–21 In some cases, different media have been considered to mimic possible entry routes, such as lung inhalation,22–24 ocular injection,25 and intramuscular injection (as in the case of vaccines).26 These alternative media have had less impact in the nanotoxicology space than they deserved.27 In the case of the most common in vitro screening of NMs intended for systemic intravenous administration, it is evident that 10% FBS poorly resembles the composition of human blood, and the question should arise whether this experimental approach can be representative of the bloodstream scenario, especially concerning the formation of BMC and consequent nano-bio interactions. Not to mention the numerous cases in which serum-free conditions are used, which can influence interactions (i.e., adhesion) between manufactured materials and cell membranes.28,29 In addition, serum-free conditions induce a starvation state in the cells, which can also contribute to promoting internalization. In previous works, the idea of testing NMs in vitro using higher serum concentrations (50% FBS or human serum) to reduce the mismatch between in vitro and in vivo results was already discussed.4,30,31
In this work, we selected, as representative examples, two NMs with very different physical–chemical properties, both extensively studied in nanomedicine. The first ones, gold nanoparticles (GNPs), are among the most frequently employed nanomaterials in nanomedicine and are largely investigated in terms of BMC analysis.32 The second, graphene oxide (GO), is a 2D material widely investigated in the last decade for bio-applications.33–36 Nowadays, we have extensive control over both materials' synthesis and properties, as well as knowledge of their bio-nano interactions.37,38
This study explores the molecular and biological biases arising from the mismatch between using 10% FBS (employed for in vitro biological readouts) and whole human plasma (HP, closer to the in vivo administration scenario) in the BMC profile of GNPs and GO. Of note, in the profiling of BMC composition, the aim of the investigation is often limited to proteins,8,39–41 hence overlooking other biomolecules42–45 (and originating the widely used “protein corona” term). In the present work, we also included the lipid contribution,46 since the interplay between proteins and lipids in mediating reciprocal interactions with the NM surface remains unclear.18,20,40,47–50 Using dedicated statistical tools, we identified specific protein–lipid associations and investigated the correlation between human and bovine proteins in the BMC of each NM. With this approach, we derived NM-specific molecular signatures recapitulating the plasma-to-medium transition. After this extensive molecular profiling, we then acquired a set of biological readouts using high-content imaging to highlight corona-derived effects on cellular interactions. Given the impossibility of culturing cells in HP, we show how the remaining features from the original HP-BMC are sufficient to affect different common biological readouts significantly. Finally, we discriminated the components of an HP-derived BMC that are more resistant to the exchange with other biomolecules (“static” components) from those biomolecules that are more prone to being substituted (“dynamic” components). Using these two lists of proteins, we performed protein–protein functional association analysis, revealing interesting network associations.
While out of scope for the present work, a comprehensive link between NM-BMC-biological readouts is an extremely complex task and should be based on massive amounts of data accounting for different NMs (with variations of size, shape, and surface chemistry), different medium conditions (pH, temperature, and oxygen content), and a plethora of cell models (i.e., including immune system51 and primary cells).
However, the presented quantitative assessment highlights in detail a series of biases in established experimental practices; gaining awareness of these possible issues is essential for the development of explicit and reliable association rules between molecular signatures and biological readouts in vitro/vivo, which will allow significant progress in the nanomedicine and nanotoxicology arena.
Results and discussion
Multiomics profiling of the biomolecular corona
We investigated the molecular composition of the BMC formed under four experimental conditions (for more details, see the Material and Methods section) for both GO and GNPs (the materials’ characterization is shown in Fig. S1 and S2, ESI†). For the first condition, we incubated GO/GNPs directly in the complete cell culture medium (cDMEM) to mimic the pure in vitro setting. This condition will be called “M”. The second condition, mimicking the in vivo setting, consists of GO/GNPs incubated in human plasma and will be called “HP”. For the third and fourth conditions, the GO/GNPs were allowed to form their BMC in HP and then, after washing loosely bound components, were transferred to cDMEM and sampled after 1 h (“HP-M1”) and 24 h (“HP-M24”), respectively, to study the protein and lipid exchange dynamics. Fig. 1 summarizes these experimental conditions.
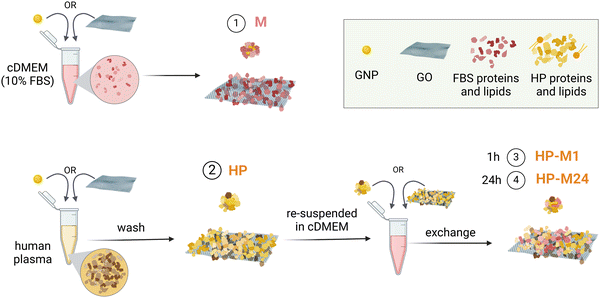 |
| Fig. 1 Schematics of the experimental conditions. (1) BMC formed in cDMEM (FBS proteins and lipids): M. (2) BMC formed in human plasma (proteins and lipids): HP. (3) BMC formed in HP, washed by centrifugation, and then transferred to cDMEM for either 1 h: HP-M1 or (4) 24 h: HP-M24. | |
To remove the non-tightly bound biomolecules, we washed the formed BMC with phosphate-buffered saline (PBS) solution at the end of each incubation. We then performed a combined, detailed, and quantitative proteomic and lipidomic profiling of the four samples. We first investigated the total amount of biomolecules expressed as the total observed mass spectrometry coupled to liquid chromatography (LC-MS) area of proteins and lipids. It has been noted that estimating the GO total surface area with a geometrical calculation is not possible due to inhomogeneity in the size, shape, and number of sheets in the flakes. Therefore, our aim is not to perform a direct quantitative BMC comparison between the two NMs but to analyze how each NM could be affected by different biological environments.
Interestingly, as shown in Fig. 2A and B, both NMs directly exposed to medium (M) incorporated a much lower lipid content than that naturally present in the BMC in vivo. This indicates that the lipid “coating” involved in the interactions with cells is very different if NMs are previously exposed or not to HP. Indeed, the cell culture medium, with its 10% FBS, is largely insufficient to mimic a realistic BMC lipid content of a given NM when transported in the bloodstream. However, if the materials are pre-exposed to HP, a significant amount of lipids persist on the BMC even after exposure to 10% FBS (Fig. 2A and B).
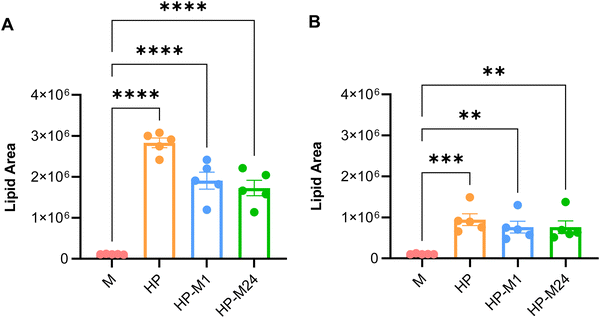 |
| Fig. 2 Lipidomics analysis. (A) and (B) Total lipid content observed in the BMC of GNPs (A) and GO (B) for each BMC experimental group. Statistical analysis: one-way ANOVA/Tukey's test, ***p < 0.0001, ***p < 0.001, **p < 0.01. | |
We then selectively discriminated proteins from either Homo Sapiens or Bos Taurus species by using peptides unique for a specific protein in a given species (proteotypic peptides) for quantification. A set of 817 and 710 proteins were quantified in the BMC of GO and GNPs, respectively, while 156 and 225 unique lipid features were tracked for GO and GNPs, respectively (the full set of data can be found in File S1 for proteins and File S2 for lipid features, ESI†). The most abundant proteins (top 20, based on the observed LC-MS protein area) found in the BMC of both materials under all the tested conditions are listed in Tables S1 and S2 (ESI†). The protein profiles obtained for GNPs and GO in HP are comparable with those of previous studies.52–56 Notwithstanding the differences in the NP size and experimental conditions, some recurring proteins in the GNP BMC can be detected, such as albumin, glycoproteins (vitronectin), blood coagulation proteins (fibrinogen and kininogen-1), anti-inflammatory proteins (inter-alpha-trypsin inhibitor heavy chain H4), and apolipoproteins (A-I and E). For GO, recurring highly abundant proteins in the BMC are albumin, apolipoproteins (A-I and A-II), immunoglobulin heavy constant, and fibrinogen.54 We then moved to analyzing the exchange dynamics of this complex molecular system.
Human features in the BMC are quickly replaced by bovine proteins in the cell culture medium
The protein and lipid exchange mechanisms occurring on the surface of each NM when the HP BMC was transferred into the cell culture medium (HP-M1, HP-M24) were subsequently analyzed, and the results are summarized in Fig. 3A and B.
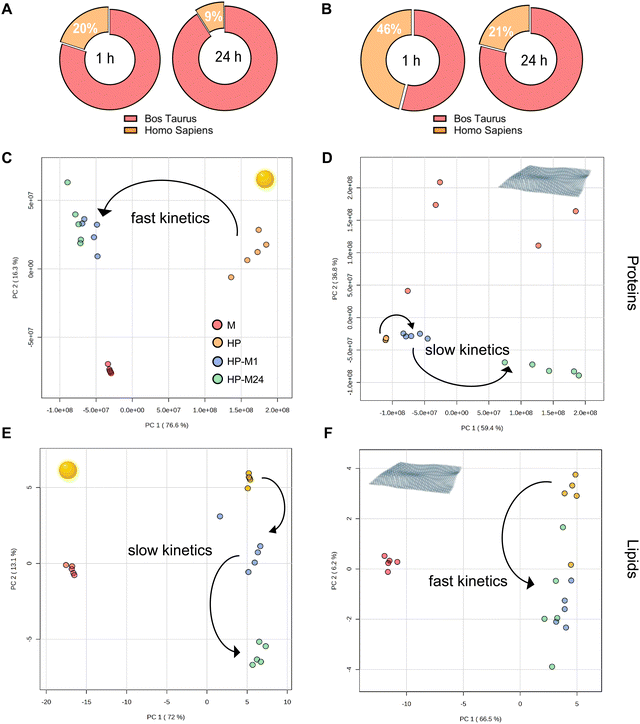 |
| Fig. 3 Human/bovine exchange dynamics. (A) and (B) Relative bovine/human protein content at 1 and 24 h time points for both GNPs (A) and GO (B). (C)–(F) Principal component analysis (PCA) illustrating the protein (C) and (D) and lipid (E) and (F) dynamics of GNPs (C) and (E) and GO (D) and (F) in the HP → M transition. The GNP BMC shows a swift change for HP protein clusters to M (the clusters for 1 and 24 h time points are indistinguishable). The kinetics for GO is slower, and the transition through the 1 h time point is clearly visible in the PCA. For the lipid content dynamics, the kinetics is slower for GNPs than for GO (the cluster of 1 h time point is separated for GNPs but not for GO). | |
As far as proteins are concerned, we observed that, for both NMs, human proteins are quickly replaced by bovine. After 24 h of exchange, only 9% and 21% of original HP proteins (for GNPs and GO, respectively) persist in the BMC profile (Fig. 3A and B). However, the exchange dynamics occur with different kinetics, as highlighted by the principal component analysis (PCA) shown in Fig. 3C–F.
For GO, the BMC proteome remains relatively stable for the first hour after being transferred to the 10% FBS-supplemented medium. At 24 h, in contrast, the BMC proteome is totally different from the original one, with human proteins largely replaced by bovine ones. Of note, the protein content of the medium alone fails to homogenously coat the surface of this material, as demonstrated by the scattered distribution of the “medium” (M) condition in the PCA plot.
This dynamic process is much faster for GNPs: 1 h after the transfer to the cell culture medium, the proteome was already significantly replaced by the new one, and a further 23 h of incubation only produced little additional changes in the BMC proteome, now largely bovine. Intriguingly, for lipids, the trend goes in the opposite direction: the original human content was replaced with slower dynamics for GNPs (the group HP-M1 is still clearly separated in the PCA plot) than for GO (only the HP group is clustering in the PCA).
Specific protein–lipid associations are maintained in the dynamic exchange
To further investigate the dynamic changes occurring in the BMC of these two NMs, we then explored existing associations between proteins and lipids in the corona by conducting protein–lipid data association analysis, as reported in Fig. S3 (ESI†). We first screened the observed lipid features (File S2, ESI†), filtering out a subset of confidently annotated individual lipids (File S3, annotated lipids, ESI†). By performing multiomics data association analysis, we then identified some significant correlations between proteins and lipids in the BMC of both NMs. A set of 7 well-defined lipids resulted in significant correlations with the presence of 125 human and bovine proteins in the GNP BMC. For GO, our analysis revealed the existence of significant between-omics correlations involving 14 well-defined lipids and 59 proteins. As reported in the detailed data set (File S4, ESI†), our analysis identified four main lipid/protein clusters for GNPs and two for GO. In the Excel file, lipids are indicated in blue, while bovine and human proteins are indicated in pink and orange, respectively. Quite interestingly, some common patterns can be observed in the composition of the BMC for these two different NMs. Five lipids (phosphatidylcholines) PC (16
:
0/18
:
2), PC (18
:
0/18
:
2), PC (18
:
0/20
:
4), and phosphatidylethanolamines (PE (38
:
3) and PE (38
:
4)), and eight human proteins (apolipoprotein A2 and A5, fibrinogen gamma, insulin growth factor binding proteins 2 and 3, α2-antiplasmin, serpin A10, and bovine CD9 antigen) are involved in significant correlation networks in the BMC of both NMs. This might indicate that these biomolecules are already preferentially interacting with each other in HP, meaning that when these proteins are adsorbed on NMs, they carry these specific lipids with them. From the biological standpoint, these biomolecules (fibrinogen in primis) are associated with the coagulation cascade. α2-Antiplasmin (A2AP) (also known as serpin F2) plays a crucial role in blood coagulation by inhibiting serine protease.57 On the same line, it is interesting to observe that, among all the many annotated lipid species, only a handful (and almost exclusively phospholipids) are strongly associated with networks of proteins in the corona. Of these, not surprisingly, phosphatidylethanolamines (PEs) found in platelet membranes and related to the activation of clotting factors,58 are among the nodes of the observed protein–lipid associations, with the most frequent PE species 38
:
3, 38
:
4 and 36
:
2. Lipids are also transported by lipoprotein complexes as high-density lipoproteins (HDLs; also containing apolipoprotein A2) and very low-density lipoproteins (VLDLs; also containing apolipoprotein A5) and play a crucial role in the body energy metabolism.59 In this respect, the investigation of BMC might be used as a tool to identify specific carrier proteins for lipids and other biomolecules.60,61
Residual HP features in the BMC are sufficient to promote distinct intracellular trafficking pathways
After completing the detailed molecular profiling of the BMC, we analyzed the effect of different BMC conditions on a few representative cellular readouts that can be of interest in the context of nano-drug delivery, indeed not exhaustive for more specific applications. To this aim, we employed high content analysis (HCA), often used in nanomedicine as a screening platform, to measure simultaneously several indirect readouts for exposure to different concentrations of NPs in different cell lines.62,63 Cell culture using HP-supplemented media is not reported in the literature, and from our tests, it seemed to impact the cells' healthy state. Therefore, we decided to culture the cells in a classic FBS-supplemented medium and expose them to NMs pre-coated with the HP-derived BMC. Despite the BMC exchange with FBS components described before, a non-negligible part of HP-derived biomolecules (especially lipids) will still persist onto the NMs, with potential implications.
First, we analyzed the cell viability in three models, namely CFPAC-1 (ductal pancreatic adenocarcinoma), T84 (lung carcinoma), and SH-SY5Y (neuroblastoma). Interestingly, we observed a significant decrease (up to 50%) in the proliferation of T84 and SH-SY5Y cells exposed to GO dispersed in media for 1 and 24 h (M1, M24), while GO with pre-formed HP corona (HP-M1 and HP-M24) did not exhibit any significant effect (Fig. S4, ESI†). Considering previous observations on GO, where its toxicity was reduced by the presence of protein corona,56,64,65 suggesting that 10% FBS supplement is insufficient to fully and persistently cover the GO surface (as already observed in the PCA analysis in Fig. 3D), we believe that the HP-precoating allowed for a better biomolecule coverage of the flakes, which prevented the toxicity observed in some of our cell models.
We performed further in vitro investigation on NM intracellular pathways using CFPAC-1 cells as a model, for which we observed good viability for all tested conditions (Fig. S4, ESI†).
Although GNPs and GO are internalized through different mechanisms,36,66,67 they are supposed to follow similar intracellular trafficking pathways, commonly the endolysosomal ones. We performed immunostaining for early and late endosomes using EEA1 and RAB7 antibodies, respectively,68,69 and we employed both immunostaining with lysosome-associated membrane protein-1 (LAMP-1)70 and the pH-dependent fluorescent probe lysotracker for the lysosomes analysis.
As shown in Fig. 4, after 1 h incubation, residual HP features on GNPs induced a different early endosomes’ size and distribution, observed by a significant increase in the intensity and the area of EEA1-positive spots with a parallel decrease in the total number of spots per cell (Fig. 4A–C). A similar trend was observed for cells treated with GO (Fig. 4A1–C1).
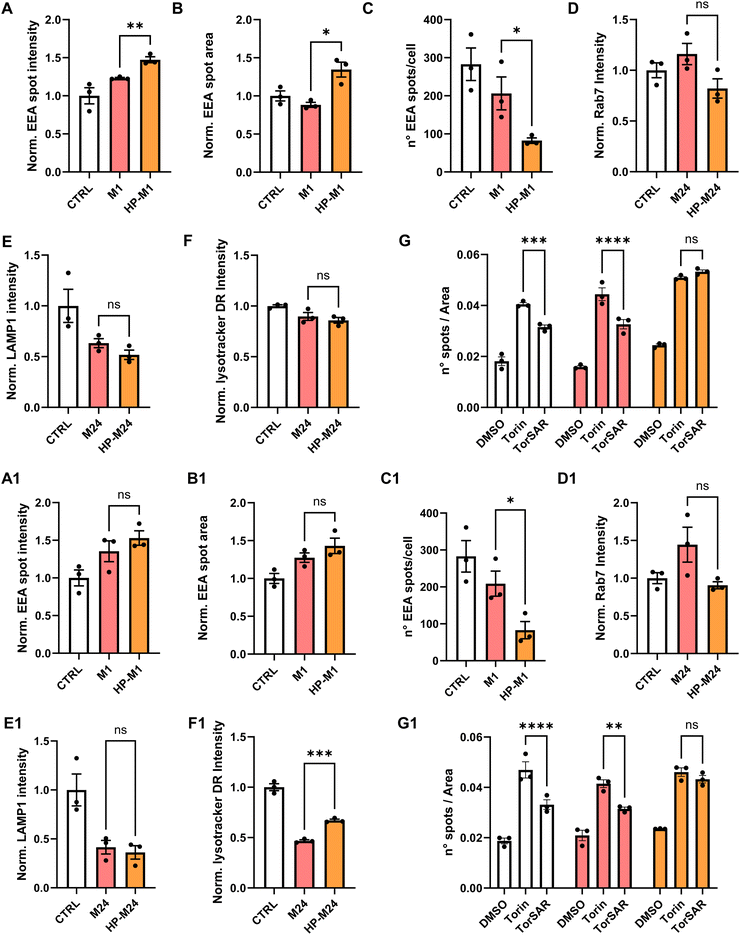 |
| Fig. 4 Analysis of intracellular trafficking for CFPAC-1 cells exposed to 25 μg mL−1 of GNPs or 10 μg mL−1 of GNPs under different BMC conditions. (A-GNPs, A1-GO) Quantification of fluorescence intensity for the EEA1 marker, (B-GNPs, B1-GO) normalized average area of EEA1-positive spots, and (C-GNPs, C1-GO) average number of EEA1-positive spots per cell after 1 h of GNP incubation. Quantification of fluorescence intensity for RAB7 (D-GNPs, D1-GO), LAMP-1 (E-GNPs, E1-GO), and lysotracker DR (F-GNP, F1-GO) after 24 h of GNP incubation. White bars represent the control conditions of untreated cells. Pink bars represent cells treated with GNPs directly dispersed in the cell culture medium (M). Orange bars represent cells treated with GNPs with a pre-formed HP corona dispersed in the cell culture medium for 24 h (HP-M24). Data are normalized over untreated cells (CTRL) and expressed as means ± SEM of N = 3 biological replicates. Statistical analysis: *p < 0.05, **p < 0.01, unpaired Student's t-test. (G-GNPs, G1-GO) Analysis of autophagic pathways. White bars represent the control conditions of untreated cells. Pink bars represent cells treated with GNPs directly dispersed in the cell culture medium (M). Orange bars represent cells treated with GNPs with a pre-formed HP corona dispersed in the cell culture medium for 24 h (HP-M24). For all conditions, cells were treated with DMSO, Torin-1 (positive control for autophagy induction) alone or in combination with SAR-405 (TorSAR – positive control for autophagy inhibition). All data are expressed as numbers of MDC spots per area and expressed as means ± SEM of n = 3 biological replicates. Statistical analysis: *p < 0.05, ***p < 0.001, ****p < 0.0001, one-way ANOVA/Tukey's tests. | |
Of note, after 1 h, the original preserved HP-derived features on NMs are about twice the ones after 24 h (Fig. 2). This might be related to the fact that we did not observe statistically significant changes in the late endosome marker (Fig. 4D and D1), even though a tendency to a decreased fluorescence intensity in HP samples can be noted, which could be related to the aforementioned smaller number of early endosomes exhibited by both NMs.
Lysosomal markers were also analyzed after 24 h. While LAMP1 analysis did not show significant outcomes (Fig. 4E and E1), the lysotraker intensity was altered by the presence of GO. Again, this might be related to the higher % of HP-derived proteins retained by GO over time (Fig. 2). In our case, as for other examples in the literature,71,72 we observed a tendency of both NMs to alkalinize the lysosomal compartment compared to controls (Fig. 4F and F1). However, in the case of GO, for the HP-derived sample, the lysosomal pH resulted significantly more acidic than for the M one (Fig. 4F1), while this was not observed for GNPs (Fig. 4F).
Overall, these observations, independently from the differences among the two NMs, indicate that the presence of even a small percentage of residual HP-derived features can significantly impact NM intracellular trafficking, suggesting that, under full HP conditions, striking differences might be expected.
To straighten this point, differences were also observed in the context of autophagy, a “self-digestion” mechanism that maintains physiological cell homeostasis, strictly correlated to pathways shown before. NP accumulation has been reported to affect the autophagic cascade.73,74 For the analysis of potential dysregulation of the autophagic cascade by HCA, cells were exposed to monodansylcadaverine (MDC), which accumulates in autophagosomes and becomes fluorescent upon fusion of autophagosomes with lysosomes. We treated the cells with Torin-1 (Tor), an effective inducer of autophagy, alone or in the presence of SAR-405 (TorSAR), a well-known autophagy inhibitor,75 able to repristinate the basal autophagy level (more details are given in the Materials and Methods section). The results are reported in Fig. 4G. Under basal conditions, GNPs did not induce autophagy per se. However, upon GNP incubation, when treating the cells with TorSAR, the recovery of MDC fluorescence intensity was only observed for GNPs directly exposed to the medium (M). This suggests that, again, remaining HP-derived features on GNPs might affect intracellular dynamics. The same behavior was observed for GO (Fig. 4G1).
There are several examples in the literature on how GNPs and GO can affect the autophagic cascade71,76–79 depending on the size, shape, surface chemistry, and NP concentration involved, and all this will obviously also depend on the biological model studied. Our results suggest that besides the aforementioned physical–chemical features and experimental protocols, the BMC composition is an additional parameter that has a significant impact, which could, however, be overlooked when using non-representative biological media for cell culture.
Establishing a direct, mechanistic link between intracellular trafficking dysregulation and BMC features (especially under HP conditions, difficult to test in cell models) is out of the scope of our investigation, and it would require a massive amount of biological and molecular data. However, given that the BMC appeared to be responsible for the observed variations in the biological readouts, besides the dynamic of exchange already analyzed for the whole proteome and lipidome, it is interesting to analyze how the BMC composition is transformed under different conditions employed. We then introduced a workflow aiming to identify specific components that might play a major role in this context.
Complement proteins, coagulation cascades, and apolipoproteins get lost from the BMC in the HP → M transition
We then re-analyzed all BMC data, taking into account every single observed molecular feature (proteins or lipids) and the variation of its abundance across the different corona conditions (see scheme in Fig. 5A). When moving along the y-axis of the graphs reported in Fig. 5B and C, we can observe the difference between the relative abundance of an individual protein or a lipid in HP- and M-derived NM-BMC. Therefore, as expected, passing from HP to M, the abundance of all plasma proteins (in orange) and all lipids (in blue) decreases while the BMC content of serum proteins (in pink) increases.
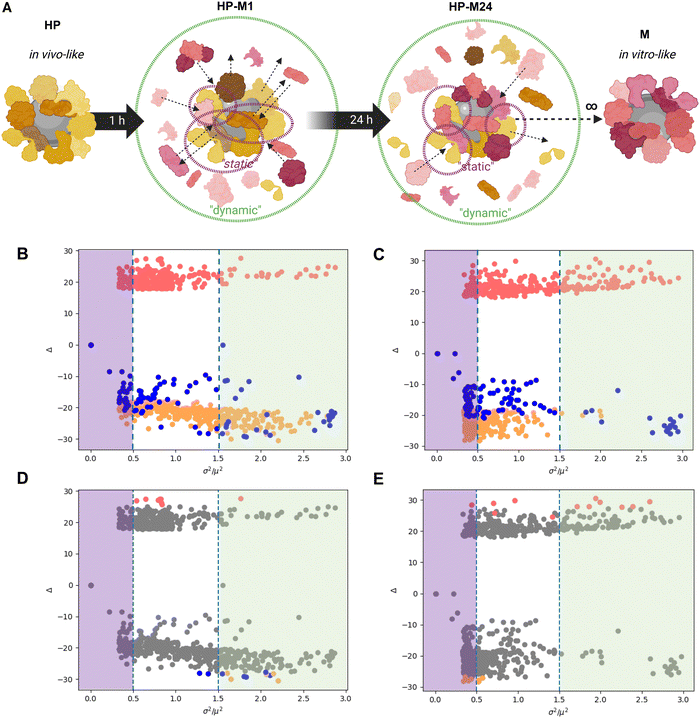 |
| Fig. 5 Identification of static (long-lasting) and dynamic (lost/acquired) elements of corona for GNPs and GO. (A) Schematics of the protein exchange in the HP → M transition. Some HP-derived proteins remain in the BMC (“static”), while others are exchanged overtime with FBS proteins (“dynamic”), as illustrated by the black arrows. (B) and (E) The graphs report the results of the analysis of the relative abundance of single molecular features (dots) under the four BMC conditions for GNPs (B) and (D) and GO (C) and (E), respectively. The y-axis (log-transformed) reports the difference between the relative abundance of individual biomolecules (proteins and lipids) under HP and M conditions. The x-axis represents the S/N observed for the same features (as the ratio between squared variance and squared average). Graphs on top (B) and (C) show lipids as blue dots and human (plasma) and bovine (serum) proteins as orange and pink dots, respectively. Graphs below (D) and (E) highlight the most abundant features (in absolute terms) overlapped with the overall feature population. For clarity, the thresholds for “static” and “dynamic” features are indicated in panels D and E as purple and green areas, respectively. | |
However, our analysis aimed at differentiating the most “static” (the long-lasting) and the most “dynamic” (the ones that get lost or are introduced during the exchange) components of the BMC; in other words, we investigated which part of the in vivo-like biological identity is preserved once transposed to an in vitro setting and, on the opposite end, which are the biomolecules most affected by the exchange processes. On the x-axis, we plotted the signal-to-noise ratio (S/N) observed for each feature, calculated as its observed variance over its squared average value. In other words, the higher the S/N, the higher the relative variation of that feature in the omics data sets.
We hypothesize that the difference observed in the presented biological readouts on intracellular trafficking should be mainly related to biomolecules belonging to the “static” part, given that only these features are likely to linger on the BMC. Instead, the “dynamic” features constitute the part of the BMC that is quickly altered by the exchange of the original human components with the bovine ones. While impacting less in our in vitro study, these components might be, in principle, some of those ignored key players behind the poor in vitro/in vivo translation.
To discriminate between high and low variant features, we then set an arbitrary threshold on the S/N ratio. Looking for a compromise between incorporating too few and too many features in our refined analysis, we considered biomolecules with S/N x < 0.5 as “static” (i.e., biomolecules more resistant to displacement, therefore showing a stronger affinity for the given NMs). Biomolecules with higher S/N values (x > 1.5) were considered, in contrast to “dynamic” features (i.e., more prone to be quickly replaced in the BMC).
In Fig. 5D and E, we reported the same graphs as Fig. 5B and C, where we highlighted the most abundant (in absolute terms) human (orange), bovine (pink) proteins, and lipids (blue). Interestingly, a set of human proteins (orange dots) in the BMC of GO (Fig. 5E) show overall high abundance and relatively mild variations (x values around 0.5). Conversely, some lipids (blue dots) and HP proteins (orange dots) in the BMC of GNPs are abundant in absolute terms, although they show significant variations (x values around 1.5 to 2). With this representation, we differentiated between the relative magnitude in the observed variation and the absolute abundance of the given feature, i.e., proteins/lipids that might be overall minor components of the BMC but still show significant relative variations in the transition from HP to M and vice versa. In fact, an analysis limited to the “top N” most abundant observed features might fail to recapitulate the role played by minor components having significant relative changes in their abundance. This helps contextualize our statistical analysis: large variations of a feature, in relative terms, might have a relevant biological impact even if its absolute abundance is relatively low in the BMC. The detailed lists of molecular features (proteins and lipids) showing the lowest (static) and the highest (dynamic) changes when the original HP BMC was transferred to the cell culture medium are available in Files S5 and S6 (ESI†) (static and dynamic features), respectively.
We first focused on the dynamic list of features (File S6, ESI†) and performed an extensive association analysis using the STRING database.80 This tool allows us to highlight the experimental and predicted interactions between proteins as both direct (physical interactions) and indirect (functional) associations. For this purpose, we first translated the bovine proteins of the BMC into their human equivalents (see the Materials and Methods section for details), and we then investigated the presence of specific protein–protein interactions within the BMC. This analysis revealed interesting network associations (list reported in File S7 (ESI†), dynamic associations), as visually reported in Fig. 6.
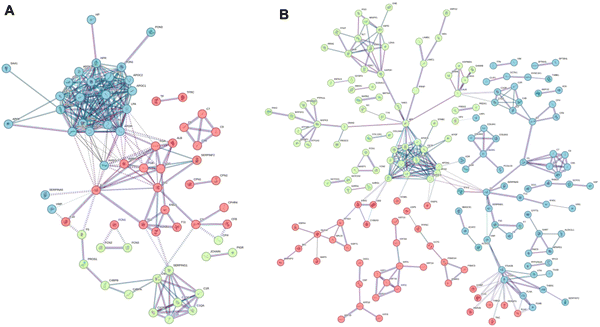 |
| Fig. 6 STRING functional network association for the “dynamic” list of features observed in the BMC of (A) GNPs and (B) GO. Colors refer to the results of the k-means clustering for the top 3 clusters. | |
For both GO and GNPs, it is interesting to report that the most represented dynamic association networks observed are related to complement and coagulation cascades, with many apolipoproteins represented in the dynamic list (APOE, APOA1, APOA4, APOB, etc.). Correspondingly, well-known apolipoproteins payloads, like sterols and triacylglycerols, are present in the same dynamic list of features (see File S6, ESI†). Complement and coagulation cascade proteins and lipoproteins found in the BMC were also involved in significant between-omics correlation networks common to both GNPs and GO (File S4, ESI†). This indicates that the inability of the 10% FBS medium to efficiently populate the BMC with apolipoproteins and the corresponding carried lipids (cholesterols and triacylglycerols) is at least one of the reasons behind the in vitro/in vivo mismatch. On the other hand, the important biological role of cholesterol in BMC has recently been described.81
Interestingly, these apolar lipids are almost absent from the static list of molecular features (File S5, ESI†), which is more enriched in lipids carrying strongly positively charged head groups (like phosphatidylcholines and sphingomyelins). This suggests that these charged molecules are more significantly interacting, perhaps by electrostatic interactions, with the NM itself and not exclusively with the BMC protein content. This might explain why, despite dynamic changes in the protein content, their relative abundance remains high enough to classify them as static components of the BMC.
Finally, from the opposite standpoint, we extrapolated the human core of proteins from the static list, therefore surviving in the BMC of both NMs after 24 h of incubation in the 10% FBS-supplemented medium. As reported in Fig. S5 (ESI†), Venn overlap analysis revealed 30 proteins in common in the human cores of both NMs, while 41 and 283 were specific for GNPs and GO, respectively. With the aim of identifying HP-derived proteins that might play a material-specific role in our in vitro testing, we then excluded the common proteins from the analysis and, using again STRING, we identified enriched protein–protein networks in the human cores that are specific to each NM (Fig. 7). In File S8 (ESI†) (static human core), we report the identified enriched gene ontology terms (processes, functions, and components), specifically enriched in the human core of each NM.
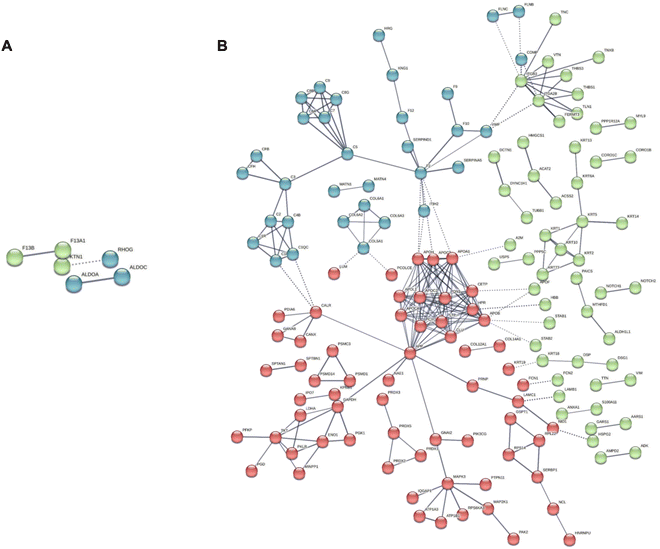 |
| Fig. 7 STRING functional network association analysis for the core of human proteins in the BMC of (A) GNPs and (B) GO. Colors refer to the results of the k-means clustering for the top 3 clusters. | |
It is interesting to note that, for GNPs, the gene ontology components identified are only 6 and mainly involve phagocytic vesicles and secretory vesicles, where recurring associated proteins are Ras-related proteins 10 and 14 (Rab10, 14) and lysosome-associated membrane glycoprotein 2 (LAMP2). These proteins are likely to be associated with the observed differences in the intracellular trafficking of our in vitro model. Rab10 and Rab 14 indeed are responsible for regulating the formation, transport, and fusion of secretory vesicles involved in exocytosis.82,83 Rab14, in particular, was found to regulate a separate recycling pathway and to be also associated with cell motility.84 Another identified protein was coronin-1, also involved in phagocytosis processes and chemotaxis.85 Indeed, besides the phagocytic vesicles, the other extracted gene ontology components are related to the cortical cytoskeleton.
For GO, the landscape is more complex, with 93 specific enriched gene ontology components. Still, we could identify components related to early endosomes, lysosomes, and secretory granules involving several apolipoproteins (i.e., APOH, APOB, APOA1, APOC2, and APOC3), Rab1A, known to play a role both in secretion and regulation of autophagy86 and ficolin-1, found in secretory granules in the complement pathway activation.87,88 Complement proteins were also found in the list (C2, C3, C5, and C1q) together with several components related to extracellular vesicles, with the recurring presence of serpin proteins (A1, A3, B1, and B12). Serpin proteins were also found to be involved in significant correlation networks in the BMC of both NMs in the multiomics analysis.
While lacking sufficient data to provide BMC predictions, this workflow still highlights interesting protein players in the BMC of the two different NMs that can be linked to observe biological readouts in our model conditions.
Conclusions
In this work, we moved beyond the simple, though detailed, description of the BMC composition to raise experimental and data-based concerns about the possibility of obtaining meaningful insights into the biological properties of NMs from current in vitro settings. To do so, using two well-known reference NMs (spherical GNPs of 15 nm and GO flakes), we established an analytical-in vitro, statistics, and bioinformatics workflow to characterize the BMC. By analyzing the BMC of the two NMs under different biological settings (in vitro- and in vivo-like), using 10% FBS and HP as biofluids, we showed that it is possible to track dynamic changes in the BMC composition, monitoring the replacement of HP proteins and lipids with those present in 10% FBS. We demonstrated that the kinetics of this re-equilibration is material-dependent: by tracking hundreds of biomolecules in a dynamically changing environment, our analysis highlighted individual proteins and lipids that are either significantly enriched or depleted with respect to the composition of naïve plasma, indicating (1) specific affinities of these molecules for the two different surfaces and (2) specific protein–protein and protein–lipids associations. Moreover, the biological readouts we collected with high-content image analysis clearly show that cell viability, trafficking pathways, and the autophagic cascade are all influenced by the presence or absence of a pre-formed (yet dynamically changing, with all the limitations associated with this model) HP BMC corona. We also specifically pinpointed which components of the human BMC are more likely (and quickly) replaced by bovine biomolecules, and we differentiated them from their counterparts showing the opposite trend, i.e., less likely (and less quickly) replaced in in vitro settings.
The complexity of the bio-nanointeractions, considering the multitude of variables into play (different NMs, physical–chemical features, medium compositions, and biological models), is such that the community has still not reached a consensus on the next steps to be undertaken as a simple, definitive solution to this issue which is still not conceivable at present. Indeed, from our data, it is clear how not even a precoating with plasma proteins and lipids is sufficient to provide an accurate proxy of the in vivo scenario; blood cells, platelets, and other blood components are still completely missing in this picture. However, our case study gives a detailed description of how inadequate, while experimentally convenient, is testing NMs in a classic 10% FBS-supplemented medium.
For reliable in vitro screening, the presence of at least human plasma-derived proteins on the corona should, in principle, always be taken into account since it might generate a significantly different experimental outcome. It has also been noted that the differences we observed in the analyzed cellular readouts are expected to be markedly larger in vivo, where the HP corona is fully preserved, compared to our “proxy” platform, in which we know bovine serum components heavily modify the BMC.
The evidence we reported here, together with the molecular data, clearly indicates that the results of in vitro tests of NMs might fail to represent the real in vivo situation, and new shared protocols are now becoming necessary to elevate the accuracy and the predictive power of in vitro models for nanomedicine.
A complete screening of the variety of NM features in different biological contexts might be the only viable option at present, possible only with a joint laboratory effort and the support of machine learning tools.
The presented work is clearly not intended to provide such screening, but to raise a red flag instead.
The aim of this work is, indeed, to highlight biases arising from the use of current in vitro standard conditions for toxicology readouts for NMs and to produce an integrated experimental framework to generate and support new knowledge in the bio-nano interaction field.
Our experimental effort, together with a recently published analysis of possible roadmaps to exploit BMC for human benefits,32 helps in tiding up the observations emerged in the last few years around this issue and illustrates the central role of the development of novel shared standard protocols for BMC and bio-nanointeraction analysis.
Our approach might perhaps soon be used to systematically address this point if coupled with advanced predictive tools, like artificial intelligence and machine learning, to trigger predictions of the in vivo behavior of NMs based on well-structured, large-scale multiomics data sets. As for many other topics in biomedicine, this last point (availability of large and structured datasets) is perhaps still the biggest limitation to an effective implementation of these approaches. In the future, these efforts might be supported at several levels by computer science and chemical/physical modeling, employing both statistical/machine learning tools and atomistic-level simulations.89 In a larger view, biomolecular corona analysis for nanotoxicology and nanomedicine might follow the same trend of big data analysis in health care, moving towards personalized solutions in precision medicine.90–93
Materials and methods
GNP and GO preparation and characterization
For the synthesis of GNPs, 300 μL of HAuCl4 (Merck, 7.5 × 10−6 mol, 2.5 × 10−2 M) was added to 30 mL of ultrapure water and heated to boiling under reflux, followed by a quick injection of 0.9 mL of Na3Ct (Merck, 3 × 10−5 mol, 3.4 × 10−2 M) into the mixture under vigorous stirring and leaving under reflux for 10 min. Here, the formation of a ruby red color can be observed. At this point, the flask was placed on ice and allowed to cool to room temperature. The resulting GNP suspension was filtered through a 0.2 μm Millipore® filter. For GNP characterization, absorption spectra were acquired with a Thermo Fisher NanoDrop® (350–900 nm range) instrument using small-volume PMMA disposable cuvettes (Sarstedt, Nümbrecht, Germany). The samples were diluted prior to analysis to obtain an absorbance of ≤1. Dynamic light scattering (DLS) analysis was performed using a Zetasizer Nano Range (Malvern, Worcestershire, UK) instrument, and the reported values are an average of three independent measurements (each consisting of an accumulation of 11 runs).
For transmission electron microscopy (TEM) analysis, the samples were prepared by drop casting 1 μL of a BGNP suspension on a formvar-coated copper grid cleaned with oxygen plasma (200 mesh, Ted Pella, Redding, CA, USA) and were allowed to dry in air for 2 h. A JEOL JEM 1400 microscope (JEOL, Tokyo, Japan) operating at 120 kV was employed for imaging. The images were analyzed using ImageJ for the statistical size distribution. GO was supplied from Antolin group (Burgos, Spain) and was washed several times with water until a pH of around 5 was obtained. GO was characterized using a high-resolution transmission electron microscope (HRTEM, JEOL 2100 at an accelerating voltage of 100 kV). Raman studies were performed using an InVia Renishaw microspectrometer equipped with a 532 nm point-based laser, with a power density below 1 mW mm2 to avoid laser heating effects, measuring at least 30/40 random locations on each sample. Thermogravimetric analysis (TGA) was performed using a Q50 thermogravimetric analyzer of TA Instruments, at 10C min−1 under a nitrogen flow, from 100° to 800 °C. Both materials were kept at 4 °C to minimize bacterial contamination.
BMC formation protocols
The corona was formed under four conditions, as illustrated in Fig. 1:
1. Cell culture medium (DMEM) supplemented with 10% FBS (cDMEM);
2. 100% HP;
3. 100% HP washed and re-suspended in cDMEM for 1 h;
4. 100% HP washed and re-suspended in cDMEM for 24 h.
For HP/cDMEM corona formation (1,2), 50 μL of GNPs (dispersed in water at 0.6 mg mL−1 stock concentration) and 25 μL of GO (dispersed in water at 1 mg mL−1 stock concentration) were incubated in 1 mL of commercial human plasma (Tebu Bio, Milano, Italy) at 37 °C for 1 h. At the end of the incubation, the samples were centrifuged for 30 min at 20
000 × g at 4 °C and the supernatant was discarded. The pellets were then washed three times with 500 μL of fresh PBS and recovered in PBS for further processing and analysis.
Alternatively, samples exposed to HP were centrifuged for 30 min at 20
000 × g at 4 °C and re-suspended in 1 mL of cDMEM. The samples were then incubated for 1 h (3) or 24 h (4) prior to further washing steps by centrifugation and then recovered in PBS for further processing and analysis.
Sample preparation and data acquisition
The lipid content of the biomolecular corona was then extracted and analyzed, as already published by our group.46 In short, coronated NMs were extracted with 1 mL of isopropanol and centrifuged, and the supernatant was removed and dried under a nitrogen stream. The resulting pellet was kept for proteomic analysis. The day of analysis, the samples were redissolved in 50 μL of 9
:
1 methanol
:
chloroform for high-resolution LC-MS analysis and analyzed using a Synapt G2 QToF mass spectrometer, coupled with an Acquity UPLC chromatographic system (both purchased from Waters Inc., Milford, USA). The instrumental parameters were identical to those already published by our group.46
The protein content of BMC was prepared and digested (except for the TMT labeling) as already published by our group:94 in short, the protein pellets were dissolved and reduced by adding 100 μL of 8 M urea, 1% SDS and 10 mM dithiothreitol dissolved in 100 mM ammonium bicarbonate 50 mM and incubating at 56 °C for 1 h. After centrifugation at 20
000 × g for 20 min, the supernatant was collected, and cysteine was alkylated with iodoacetamide 55 mM for 20 min in the dark and digested with trypsin at 37 °C. The resulting peptides were then analyzed using a Thermo Exploris 480 high-resolution LC-MS system coupled with a nano HPLC system. Peptides were separated using an Aurora (75 μm × 20 cm) nano-LC column with a linear gradient of acetonitrile in water. Both eluents were added with 0.1% formic acid. Proteomics data were acquired in data-independent mode as already described.95
Analysis on the average BMC sample
Data analysis, feature identification, and statistics.
All proteomics data were searched against the reference proteome of both Homo Sapiens and Bos Taurus, using the corresponding FASTA files available from the UNIPROT database and retrieving only the reviewed sequences. A database search was performed using Spectronaut software (purchased from Biognosys). The maximum allowed false discovery rate (FDR) was set to 1% and at least two peptides were used for positive protein identification and quantification. To discriminate between human and bovine genomes, only proteotypic peptides were used for quantification. The final data matrices obtained are reported in File S1 (ESI†). All lipidomic features were extracted from the RAW data as accurate mass and retention time (RT) data points, integrated and realigned over all the runs using Markerlynx software (Waters) as already described46 to produce the data matrices reported in File S2 (ESI†). All the data were then analyzed using the Metaboanalyst96 free software package. All the missing values in the data set were replaced with 1/5 of the minimum value observed for each feature.97 For PCVA analysis, proteomics data were automatically normalized by Spectronaut but not transformed for multivariate data analysis. Only a mean scaling was applied. Lipidomics data were normalized by the sum of the total observed features, log
10 transformed, and Pareto scaled.98
The OmicsAnalyst99 tool was used for multiomics correlation analysis between proteins and lipids. For this purpose, data were mean-centered but not further normalized. Pearson r was used as the distance measure96 using the following parameters: degree cutoff: 0; betweenness filter: 10; correlation threshold (inter-omics): 0.90, both directions; max edges: 150. For univariate data analysis, unpaired Student's t-test was performed using GraphPad Prism (GLS Biotech).
Feature annotation for lipidomics.
As described before,46 all the relevant features, so far indicated by a RT_m/z data pair, were then manually annotated, inspecting each corresponding m/z value at the given RT from the RAW data. The observed accurate mass was then searched in the Lipid Maps database,100 including the following as possible adduct species: [M + H]+, [M − H2O + H]+, [M + NH4]+, [M + Na]+ for ESI+ and [M − H]−, [M + HCOO]− for ESI−. A maximum tolerance of 0.01 m/z was allowed. The list of potential matches was then scrutinized, taking into account the consistency of the lipid structure with the experimental RT101 and with the corresponding MS/MS spectrum. It should be reported that none of the proposed annotations was further confirmed with the use of a corresponding authentic reference standard. The identification level of each lipid is thus to be considered higher than 1, based on current guidelines102 and IDs are to be considered putative. Unless otherwise indicated (sphingomyelins, for example), no inference on the exact composition of the fatty acyl chains is made in the text and the corresponding condensed structure is reported, for example, PE (36
:
2) instead of PE (18
:
1/18
:
1).
Analysis of the single biological entities (static and dynamic features)
To single out which are the most significantly variant molecular entities across the experiments, we transformed the original data set into a 2D plot. First, we averaged out abundance values for each experimental condition across repetitions. Next, for each molecular entity (either lipid or protein), we computed the average concentration and its variance along the experiments. We then computed the inverse of the signal-to-noise ratio (S/N), namely the ratio between the variance and the square of the sample mean. This quantity correctly captures whether or not the variation observed across the experiments is significant as it compares the variation to the average “signal”. This first observable is the x-axis in Fig. 5. Together with this first quantity, we computed the difference of the logarithm of the final and initial values; “initial” here means the plasma conditions, whereas “final” means the medium condition. This allows us to identify the sign of the variation, and thanks to the logarithm, the domain results normalized. We used this quantity as the y-axis in Fig. 5. Taken together, x and y in the same plot allow us to identify significantly dynamic and static molecular entities easily, including their variation direction. We term a molecular entity dynamic when its S/N ratio is above 1.5, whereas we define it static when this ratio is below 0.5. We coded the analysis in Python 3.11 and took advantage of largely stable and widely used libraries such as numpy, pandas, and matplotlib.
STRING analysis
The static and dynamic lists of proteins were first uniformed to the Homo Sapiens species. For this purpose, we used REACTOME103 to project the bovine proteins into their human counterparts. We then used the STRING80 database to identify specific protein functional association networks. The following settings were used: confidence threshold was set to high (0.7), and co-expression, expression, and databases were used as only sources of data. Nodes not connected with any other protein were excluded from the network. A k-means clustering algorithm was then applied to the data set, limiting the maximum number of clusters to three (default settings). The detailed lists of significant clusters (maximum false discovery value set to 0.05) for both materials, under both conditions, are available in Files S7 and S8 (ESI†).
Cell culture and treatment with GNPs and GO
CFPAC-1 cells were grown in DMEM-F12 (Euroclone), while T84 and SH-SY5Y cells were grown in the DMEM (Euroclone). Cell culture media were supplemented with 10% FBS (Gibco), 2 mM L-glutamine, 100 U mL−1 penicillin, and 100 μg mL−1 streptomycin (Euroclone). CFPAC-1 (or T84, SH-SY5Y) cells were plated (20
000 cells per well) on good-quality clear-bottom 96-well black microplates (Corning) suitable for high-content imaging. For CFPAC-1 cells, plates were coated with poly-D-lysine (Merck KGaA, Darmstadt, Germany) to increase cellular adherence to the bottom of the wells. The following day, the medium was changed, and cells were then treated with GO (10 μg mL−1) or GNPs (25 μg mL−1) without or with a pre-formed HP corona (see conditions in the “BMC formation protocols” section), either for 24 h, or, alternatively, for 1 h but starting from 23 h after the medium change. Cells were then processed for the different assays.
Proliferation study
Microplates containing CFPAC-1 (or T84, SH-SY5Y) cells were treated with GO or GNPs for either 1 h or 24 h, and cell nuclei were then counterstained with Hoechst 33342 and propidium iodide to visualize total cells and apoptotic cells, respectively. Cells were then formalin-fixed, and imaged by using an Opera Phenix® (PerkinElmer) high-content screening system. Excitation and emission wavelengths for visualization of the Hoechst 33342 signal were 405 and between 435 and 480 nm, respectively. The propidium iodide signal was laser-excited at 490–500 nm, and the emission wavelengths were collected between 570 and 630 nm.
Analysis of GO and GNP internalization
Microplates containing CFPAC-1 cells treated with GO or GNPs for either 1 h or 24 h were formalin-fixed (5 min). Cells were then permeabilized with Triton X-100 0.1% in Dulbecco-PBS (D-PBS) for 3 min and then blocked with a blocking buffer (5% BSA in PBS) for 2 h. Cells were incubated with primary antibodies overnight at 4 °C. Early endosomes were visualized using a mouse monoclonal anti-EEA1 (#SAB1404579, Merck) antibody in cells treated with GO or GNPs for 1 h together with rabbit polyclonal anti-LAMP1 to visualize lysosomes, while late endosomes were visualized using a mouse monoclonal anti-Rab7 (#R8779, Merck) antibody together with rabbit polyclonal anti-LAMP1 in cells treated with GO or GNPs for 24 h. The following day, cells were then incubated with donkey anti-mouse secondary antibody conjugated to AlexaFluor 647 and goat anti-rabbit secondary antibody conjugated to AlexaFluor 488 plus at RT for 1 h (Thermo Fisher Scientific). Nuclei were counterstained with Hoechst 33342. Negative control samples with only secondary antibodies staining were performed to determine background fluorescence levels. High-content imaging was performed using an Opera Phenix (PerkinElmer) high-content screening system. Wells were imaged in confocal mode, using a 40× water-immersion objective. The AlexaFluor 647 signal was laser-excited at 640 nm, and the emission wavelengths were collected between 650 and 760 nm, while the AlexaFluor 488 signal was laser-excited at 488 nm, and the emission wavelengths were collected between 500 and 550 nm. Excitation and emission wavelengths for visualization of the Hoechst 33342 signal were 405 and between 435 and 480 nm, respectively. Image analysis of signal intensity was performed using the Harmony software (version 4.9) of the Opera Phenix high-content system.
Analysis of autophagic pathways in cells exposed to GO and GNPs
CFPAC-1 cells treated with GO or GNPs for 24 h were also exposed, in the last 6 h of incubation, to SAR-405 2 μM (autophagy inhibitor) and Torin-1 20 nM (autophagy activator). Cells were then loaded with 50 μM MDC (Sigma-Aldrich)104 in PBS at 37 °C for 10 min. After incubation, cells were washed three times with PBS and immediately analyzed. High-content imaging and data analysis were performed using an Opera Phenix (PerkinElmer) high-content screening system. Wells were imaged in confocal mode, using a 40× water-immersion objective. The MDC signal was laser-excited at 405 nm, and the emission wavelengths were collected between 435 and 550 nm. Data analysis of the MDC spot number was performed using the Harmony software (ver. 4.9) of the Opera Phenix high-content system.
Analysis of lysosomal compartment in cells exposed to GO and GNPs
CFPAC-1 cells, following treatment with GO or GNPs for either 1 h or 24 h, were live stained with Lysotracker Deep Red. Briefly, cells were incubated in the culture medium supplemented with Lysotracker Deep Red for 30 min at 37 °C in a CO2 incubator. After incubation, cells were placed in D-PBS supplemented with 10% FBS (Gibco) and 5.5 mM glucose (Merck) and immediately analyzed. High-content imaging and data analysis were performed using an Opera Phenix (PerkinElmer) high-content screening system. Wells were imaged in confocal mode, using a 40× water-immersion objective. The lysotracker deep red signal was laser-excited at 640 nm, and the emission wavelengths were collected between 650 and 760 nm. Data analysis of the lysotracker signal was performed using the Harmony software (ver. 4.9) of the Opera Phenix high-content system.
Author contributions
V. C., V. T., L. B., and C. B. performed all wet lab activities. A. A. performed proteomic, lipidomic and STRING analyses. N. P., P. P. P., and F. B. critically revised the work and manuscript. SD performed the analysis on single biological entities and wrote the corresponding sections. A. A. and V. C. conceived the original idea, wrote the paper, and supervised the project.
Data availability
All omics RAW data have been uploaded to the PRIDE database with provisional identifier 1-20231103-110756-94970109.
Conflicts of interest
The authors have no conflicts of interest of any kind to report.
Acknowledgements
This project has received funding from the European Union's Horizon 2020 Research and Innovation Programme under Grant Agreement No 881603 Graphene Flagship, Core3. This work was also supported by the Italian Ministry of Health through “5 × 1000” and Ricerca Corrente at IRCCS Ospedale Policlinico San Martino, Genova, Italy (to FB and VC) and IRCCS Istituto Giannina Gaslini, Genova, Italy (to NP). The authors acknowledge Ester Vazquez for providing the GO material.
References
- M. P. Monopoli, C. Åberg, A. Salvati and K. A. Dawson, Biomolecular coronas provide the biological identity of nanosized materials, Nat. Nanotechnol., 2012, 7(12), 779–786 CrossRef CAS PubMed
.
- P. C. Ke, S. Lin, W. J. Parak, T. P. Davis and F. Caruso, A Decade of the Protein Corona, ACS Nano, 2017, 11(12), 11773–11776 CrossRef CAS PubMed
.
- E. Casals and V. F. Puntes, Inorganic nanoparticle biomolecular corona: formation, evolution and biological impact, Nanomedicine, 2012, 7(12), 1917–1930 CrossRef CAS PubMed
.
- S. Lara, F. Alnasser, E. Polo, D. Garry, M. C. Lo Giudice, D. R. Hristov, L. Rocks, A. Salvati, Y. Yan and K. A. Dawson, Identification of Receptor Binding to the Biomolecular Corona of Nanoparticles, ACS Nano, 2017, 11(2), 1884–1893 CrossRef CAS PubMed
.
- D. Docter, D. Westmeier, M. Markiewicz, S. Stolte, S. Knauer and R. Stauber, The nanoparticle biomolecule corona: lessons learned–challenge accepted?, Chem. Soc. Rev., 2015, 44(17), 6094–6121 RSC
.
- D. Guarnieri, M. A. Malvindi, V. Belli, P. P. Pompa and P. Netti, Effect of silica nanoparticles with variable size and surface functionalization on human endothelial cell viability and angiogenic activity, J. Nanopart. Res., 2014, 16(2), 2229 CrossRef
.
- G. Tarricone, V. Castagnola, V. Mastronardi, L. Cursi, D. Debellis, D. Z. Ciobanu, A. Armirotti, F. Benfenati, L. Boselli and P. P. Pompa, Catalytic Bioswitch of Platinum Nanozymes: Mechanistic Insights of Reactive Oxygen Species Scavenging in the Neurovascular Unit, Nano Lett., 2023, 23(10), 4660–4668 CrossRef CAS PubMed
.
- S. Tenzer, D. Docter, J. Kuharev, A. Musyanovych, V. Fetz, R. Hecht, F. Schlenk, D. Fischer, K. Kiouptsi, C. Reinhardt, K. Landfester, H. Schild, M. Maskos, S. K. Knauer and R. H. Stauber, Rapid formation of plasma protein corona critically affects nanoparticle pathophysiology, Nat. Nanotechnol., 2013, 8(10), 772–781 CrossRef CAS PubMed
.
- W. Hu, C. Peng, M. Lv, X. Li, Y. Zhang, N. Chen, C. Fan and Q. Huang, Protein corona-mediated mitigation of cytotoxicity of graphene oxide, ACS Nano, 2011, 5(5), 3693–3700 CrossRef CAS PubMed
.
- M. P. Monopoli, C. Aberg, A. Salvati and K. A. Dawson, Biomolecular coronas provide the biological identity of nanosized materials, Nat. Nanotechnol., 2012, 7(12), 779–786 CrossRef CAS PubMed
.
- G. Caracciolo, O. C. Farokhzad and M. Mahmoudi, Biological Identity of Nanoparticles In Vivo: Clinical Implications of the Protein Corona, Trends Biotechnol., 2017, 35(3), 257–264 CrossRef CAS PubMed
.
- G. Maiorano, S. Sabella, B. Sorce, V. Brunetti, M. A. Malvindi, R. Cingolani and P. P. Pompa, Effects of cell culture media on the dynamic formation of protein− nanoparticle complexes and influence on the cellular response, ACS Nano, 2010, 4(12), 7481–7491 CrossRef CAS PubMed
.
- A. Lesniak, F. Fenaroli, M. P. Monopoli, C. Åberg, K. A. Dawson and A. Salvati, Effects of the presence or absence of a protein corona on silica nanoparticle uptake and impact on cells, ACS Nano, 2012, 6(7), 5845–5857 CrossRef CAS PubMed
.
- T. T. Puck, S. J. Cieciura and A. Robinson, Genetics of somatic mammalian cells: III. Long-term cultivation of euploid cells from human and animal subjects, J. Exp. Med., 1958, 108(6), 945 CrossRef CAS PubMed
.
- J. Van der Valk, D. Brunner, K. De Smet, Å. F. Svenningsen, P. Honegger, L. E. Knudsen, T. Lindl, J. Noraberg, A. Price and M. Scarino, Optimization of chemically defined cell culture media–replacing fetal bovine serum in mammalian in vitro methods, Toxicol. In Vitro, 2010, 24(4), 1053–1063 CrossRef CAS PubMed
.
- S. Lara, F. Alnasser, E. Polo, D. Garry, M. C. Lo Giudice, D. R. Hristov, L. Rocks, A. Salvati, Y. Yan and K. A. Dawson, Identification of Receptor Binding to the Biomolecular Corona of Nanoparticles, ACS Nano, 2017, 1884–1893 CrossRef CAS PubMed
.
- F. Alnasser, V. Castagnola, L. Boselli, M. Esquivel-Gaon, E. Efeoglu, J. McIntyre, H. J. Byrne and K. A. Dawson, Graphene Nanoflakes Uptake Mediated by Scavenger Receptors, Nano Lett., 2019, 19(2), 1260–1268 CrossRef CAS PubMed
.
- N. Bertrand, P. Grenier, M. Mahmoudi, E. M. Lima, E. A. Appel, F. Dormont, J. M. Lim, R. Karnik, R. Langer and O. C. Farokhzad, Mechanistic understanding of in vivo protein corona formation on polymeric nanoparticles and impact on pharmacokinetics, Nat. Commun., 2017, 8(1), 777 CrossRef PubMed
.
- G. Caracciolo, O. C. Farokhzad and M. Mahmoudi, Biological identity of nanoparticles in vivo: clinical implications of the protein corona, Trends Biotechnol., 2017, 35(3), 257–264 CrossRef CAS PubMed
.
- S. S. Raesch, S. Tenzer, W. Storck, A. Rurainski, D. Selzer, C. A. Ruge, J. Perez-Gil, U. F. Schaefer and C. M. Lehr, Proteomic and Lipidomic Analysis of Nanoparticle Corona upon Contact with Lung Surfactant Reveals Differences in Protein, but Not Lipid Composition, ACS Nano, 2015, 9(12), 11872–11885 CrossRef CAS PubMed
.
- C. A. Shaw, G. M. Mortimer, Z. J. Deng, E. S. Carter, S. P. Connell, M. R. Miller, R. Duffin, D. E. Newby, P. W. Hadoke and R. F. Minchin, Protein corona formation in bronchoalveolar fluid enhances diesel exhaust nanoparticle uptake and pro-inflammatory responses in macrophages, Nanotoxicology, 2016, 10(7), 981–991 CrossRef CAS PubMed
.
- K. M. Poulsen, M. C. Albright, N. J. Niemuth, R. M. Tighe and C. K. Payne, Interaction of TiO2 nanoparticles with lung fluid proteins and the resulting macrophage inflammatory response, Environ. Sci.: Nano, 2023, 10(9), 2427–2436 RSC
.
- M. Falahati, A. Hasan, H. A. Zeinabad, V. Serpooshan, J. von der Thüsen and T. L. ten Hagen, Engineering of pulmonary surfactant corona on inhaled nanoparticles to operate in the lung system, Nano Today, 2023, 52, 101998 CrossRef CAS
.
- Q. Hu, X. Bai, G. Hu and Y. Y. Zuo, Unveiling the molecular structure of pulmonary surfactant corona on nanoparticles, ACS Nano, 2017, 11(7), 6832–6842 CrossRef CAS PubMed
.
- D. H. Jo, J. H. Kim, J. G. Son, K. S. Dan, S. H. Song, T. G. Lee and J. H. Kim, Nanoparticle-protein complexes mimicking corona formation in ocular environment, Biomaterials, 2016, 109, 23–31 CrossRef CAS PubMed
.
- A. Amici, D. Pozzi, C. Marchini and G. Caracciolo, The Transformative Potential of Lipid Nanoparticle–Protein Corona for Next-Generation Vaccines and Therapeutics, Mol. Pharmaceutics, 2023, 20(11), 5247–5253 CrossRef CAS PubMed
.
- P. Foroozandeh and A. A. Aziz, Merging worlds of nanomaterials and biological environment: factors governing protein corona formation on nanoparticles and its biological consequences, Nanoscale Res. Lett., 2015, 10, 1–12 CrossRef CAS PubMed
.
- A. Lesniak, A. Salvati, M. J. Santos-Martinez, M. W. Radomski, K. A. Dawson and C. Åberg, Nanoparticle adhesion to the cell membrane and its effect on nanoparticle uptake efficiency, J. Am. Chem. Soc., 2013, 135(4), 1438–1444 CrossRef CAS PubMed
.
- Y. Dror, R. Sorkin, G. Brand, O. Boubriak, J. Urban and J. Klein, The effect of the serum corona on interactions between a single nano-object and a living cell, Sci. Rep., 2017, 7(1), 45758 CrossRef CAS PubMed
.
- F. Alnasser, V. Castagnola, L. Boselli, M. Esquivel-Gaon, E. Efeoglu, J. McIntyre, H. J. Byrne and K. A. Dawson, Graphene Nanoflake Uptake Mediated by Scavenger Receptors, Nano Lett., 2019, 19(2), 1260–1268 CrossRef CAS PubMed
.
- S. Lara, A. Perez-Potti, L. M. Herda, L. Adumeau, K. A. Dawson and Y. Yan, Differential Recognition of Nanoparticle Protein Corona and Modified Low Density Lipoprotein by Macrophage Receptor with Collagenous Structure, ACS Nano, 2018, 4930–4937 CrossRef CAS PubMed
.
- L. Boselli, V. Castagnola, A. Armirotti, F. Benfenati and P. P. Pompa, Biomolecular Corona of Gold Nanoparticles: The Urgent Need for Strong Roots to Grow Strong Branches, Small, 2023, 2306474 CrossRef PubMed
.
- F. Ahmed and D. F. Rodrigues, Investigation of acute effects of graphene oxide on wastewater microbial community: a case study, J. Hazard. Mater., 2013, 256, 33–39 CrossRef PubMed
.
- D. Bitounis, H. Ali-Boucetta, B. H. Hong, D. H. Min and K. Kostarelos, Prospects and challenges of graphene in biomedical applications, Adv. Mater., 2013, 25(16), 2258–2268 CrossRef CAS PubMed
.
- M. Bramini, G. Alberini, E. Colombo, M. Chiacchiaretta, M. L. DiFrancesco, J. F. Maya-Vetencourt, L. Maragliano, F. Benfenati and F. Cesca, Interfacing graphene-based materials with neural cells, Front. Syst. Neurosci., 2018, 12, 12 CrossRef PubMed
.
- V. Castagnola, L. Deleye, A. Podestà, E. Jaho, F. Loiacono, D. Debellis, M. Trevisani, D. Z. Ciobanu, A. Armirotti and F. Pisani, Interactions of Graphene Oxide and Few-Layer Graphene with the Blood–Brain Barrier, Nano Lett., 2023, 7, 2981–2990 CrossRef PubMed
.
- R. Arvizo, R. Bhattacharya and P. Mukherjee, Gold nanoparticles: opportunities and challenges in nanomedicine, Expert Opin. Drug Delivery, 2010, 7(6), 753–763 CrossRef CAS PubMed
.
- E. Boisselier and D. Astruc, Gold nanoparticles in nanomedicine: preparations, imaging, diagnostics, therapies and toxicity, Chem. Soc. Rev., 2009, 38(6), 1759–1782 RSC
.
- M. Lundqvist, J. Stigler, G. Elia, I. Lynch, T. Cedervall and K. A. Dawson, Nanoparticle size and surface properties determine the protein corona with possible implications for biological impacts, Proc. Natl. Acad. Sci. U. S. A., 2008, 105(38), 14265–14270 CrossRef CAS PubMed
.
- V. Castagnola, W. Zhao, L. Boselli, M. C. Lo Giudice, F. Meder, E. Polo, K. R. Paton, C. Backes, J. N. Coleman and K. A. Dawson, Biological recognition of graphene nanoflakes, Nat. Commun., 2018, 9(1), 1577 CrossRef CAS PubMed
.
- Y. Zhang, J. L. Y. Wu, J. Lazarovits and W. C. W. Chan, An Analysis of the Binding Function and Structural Organization of the Protein Corona, J. Am. Chem. Soc., 2020, 142(19), 8827–8836 CrossRef PubMed
.
- T. Lima, K. Bernfur, M. Vilanova and T. Cedervall, Understanding the Lipid and Protein Corona Formation on Different Sized Polymeric Nanoparticles, Sci. Rep., 2020, 10(1), 1129 CrossRef CAS PubMed
.
- S. Wan, P. M. Kelly, E. Mahon, H. Stockmann, P. M. Rudd, F. Caruso, K. A. Dawson, Y. Yan and M. P. Monopoli, The “sweet” side of the protein corona: effects of glycosylation on nanoparticle-cell interactions, ACS Nano, 2015, 9(2), 2157–2166 CrossRef CAS PubMed
.
- M. Gasser, B. Rothen-Rutishauser, H. F. Krug, P. Gehr, M. Nelle, B. Yan and P. Wick, The adsorption of biomolecules to multi-walled carbon nanotubes is influenced by both pulmonary surfactant lipids and surface chemistry, J. Nanobiotechnol., 2010, 8, 31 CrossRef CAS PubMed
.
- A. A. Kapralov, W. H. Feng, A. A. Amoscato, N. Yanamala, K. Balasubramanian, D. E. Winnica, E. R. Kisin, G. P. Kotchey, P. Gou, L. J. Sparvero, P. Ray, R. K. Mallampalli, J. Klein-Seetharaman, B. Fadeel, A. Star, A. A. Shvedova and V. E. Kagan, Adsorption of surfactant lipids by single-walled carbon nanotubes in mouse lung upon pharyngeal aspiration, ACS Nano, 2012, 6(5), 4147–4156 CrossRef CAS PubMed
.
- C. Braccia, V. Castagnola, E. Vázquez, V. J. González, F. Loiacono, F. Benfenati and A. Armirotti, The lipid composition of few layers graphene and graphene oxide biomolecular corona, Carbon, 2021, 185, 591–598 CrossRef CAS
.
- R. Coreas, X. Cao, G. M. Deloid, P. Demokritou and W. Zhong, Lipid and protein corona of food-grade TiO2 nanoparticles in simulated gastrointestinal digestion, NanoImpact, 2020, 20, 100272 CrossRef PubMed
.
- G. La Barbera, A. L. Capriotti, G. Caracciolo, C. Cavaliere, A. Cerrato, C. M. Montone, S. Piovesana, D. Pozzi, E. Quagliarini and A. Lagana, A comprehensive analysis of liposomal biomolecular corona upon human plasma incubation: the evolution towards the lipid corona, Talanta, 2020, 209, 120487 CrossRef CAS PubMed
.
- X. Zhang, A. K. Pandiakumar, R. J. Hamers and C. J. Murphy, Quantification of Lipid Corona Formation on Colloidal Nanoparticles from Lipid Vesicles, Anal. Chem., 2018, 90(24), 14387–14394 CrossRef CAS PubMed
.
- L. Papafilippou, A. Nicolaou, A. C. Kendall, D. Camacho-Muñoz and M. Hadjidemetriou, The lipidomic profile of the nanoparticle-biomolecule corona reflects the diversity of plasma lipids, Nanoscale, 2023, 15(26), 11038–11051 RSC
.
- Y. Yan, K. T. Gause, M. M. Kamphuis, C.-S. Ang, N. M. O’Brien-Simpson, J. C. Lenzo, E. C. Reynolds, E. C. Nice and F. Caruso, Differential roles of the protein corona in the cellular uptake of nanoporous polymer particles by monocyte and macrophage cell lines, ACS Nano, 2013, 7(12), 10960–10970 CrossRef CAS PubMed
.
- M. A. Dobrovolskaia, A. K. Patri, J. Zheng, J. D. Clogston, N. Ayub, P. Aggarwal, B. W. Neun, J. B. Hall and S. E. McNeil, Interaction of colloidal gold nanoparticles with human blood: effects on particle size and analysis of plasma protein binding profiles, Nanomedicine, 2009, 5(2), 106–117 CrossRef CAS PubMed
.
- W. Lai, Q. Wang, L. Li, Z. Hu, J. Chen and Q. Fang, Interaction of gold and silver nanoparticles with human plasma: analysis of protein corona reveals specific binding patterns, Colloids Surf., B, 2017, 152, 317–325 CrossRef CAS PubMed
.
- R. Di Santo, L. Digiacomo, E. Quagliarini, A. L. Capriotti, A. Laganà, R. Zenezini Chiozzi, D. Caputo, C. Cascone, R. Coppola and D. Pozzi, Personalized graphene oxide-protein corona in the human plasma of pancreatic cancer patients, Front. Bioeng. Biotechnol., 2020, 8, 491 CrossRef PubMed
.
- K. P. Loh and C. T. Lim, Molecular interactions of graphene oxide with human blood plasma proteins, Nanoscale, 2016, 8(17), 9425–9441 RSC
.
- M. J. Hajipour, J. Raheb, O. Akhavan, S. Arjmand, O. Mashinchian, M. Rahman, M. Abdolahad, V. Serpooshan, S. Laurent and M. Mahmoudi, Personalized disease-specific protein corona influences the therapeutic impact of graphene oxide, Nanoscale, 2015, 7(19), 8978–8994 RSC
.
- S. Singh, S. Saleem and G. L. Reed, Alpha2-antiplasmin: the devil you don't know in cerebrovascular and cardiovascular disease, Front. Cardiovascular Med., 2020, 7, 608899 CrossRef CAS PubMed
.
-
R. F. Zwaal, E. M. Bevers and J. Rosing, Phospholipids and the clotting process, Phospholipid-Binding Antibodies, CRC Press, 2020, pp. 31–56 Search PubMed
.
- J. N. van der Veen, J. P. Kennelly, S. Wan, J. E. Vance, D. E. Vance and R. L. Jacobs, The critical role of phosphatidylcholine and phosphatidylethanolamine metabolism in health and disease, Biochim. Biophys. Acta, Biomembr., 2017, 1859(9), 1558–1572 CrossRef CAS PubMed
.
- V. Corradi, B. I. Sejdiu, H. Mesa-Galloso, H. Abdizadeh, S. Y. Noskov, S. J. Marrink and D. P. Tieleman, Emerging diversity in lipid–protein interactions, Chem. Rev., 2019, 119(9), 5775–5848 CrossRef CAS PubMed
.
- A.-E. Saliba, I. Vonkova and A.-C. Gavin, The systematic analysis of protein–lipid interactions comes of age, Nat. Rev. Mol. Cell Biol., 2015, 16(12), 753–761 CrossRef CAS PubMed
.
- S. Anguissola, D. Garry, A. Salvati, P. J. O'Brien and K. A. Dawson, High content analysis provides mechanistic insights on the pathways of toxicity induced by amine-modified polystyrene nanoparticles, PLoS One, 2014, 9(9), e108025 CrossRef PubMed
.
- E. Jan, S. J. Byrne, M. Cuddihy, A. M. Davies, Y. Volkov, Y. K. Gun’ko and N. A. Kotov, High-content screening as a universal tool for fingerprinting of cytotoxicity of nanoparticles, ACS Nano, 2008, 2(5), 928–938 CrossRef CAS PubMed
.
- G. Duan, S.-G. Kang, X. Tian, J. A. Garate, L. Zhao, C. Ge and R. Zhou, Protein corona mitigates the cytotoxicity of graphene oxide by reducing its physical interaction with cell membrane, Nanoscale, 2015, 7(37), 15214–15224 RSC
.
- W. Hu, C. Peng, M. Lv, X. Li, Y. Zhang, N. Chen, C. Fan and Q. Huang, Protein corona-mediated mitigation of cytotoxicity of graphene oxide, ACS Nano, 2011, 5(5), 3693–3700 CrossRef CAS PubMed
.
- C. T. Ng, F. M. A. Tang, J. J. E. Li, C. Ong, L. L. Y. Yung and B. H. Bay, Clathrin-mediated endocytosis of gold nanoparticles in vitro, Anatomical Record, 2015, 298(2), 418–427 CrossRef CAS PubMed
.
- L. Ding, C. Yao, X. Yin, C. Li, Y. Huang, M. Wu, B. Wang, X. Guo, Y. Wang and M. Wu, Size, shape, and protein corona determine cellular uptake and removal mechanisms of gold nanoparticles, Small, 2018, 14(42), 1801451 CrossRef PubMed
.
- F.-T. Mu, J. M. Callaghan, O. Steele-Mortimer, H. Stenmark, R. G. Parton, P. L. Campbell, J. McCluskey, J.-P. Yeo, E. P. Tock and B.-H. Toh, EEA1, an Early Endosome-Associated Protein.: EEA1 IS A CONSERVED α-HELICAL PERIPHERAL MEMBRANE PROTEIN FLANKED BY CYSTEINE “FINGERS” AND CONTAINS A CALMODULIN-BINDING IQ MOTIF, J. Biol. Chem., 1995, 270(22), 13503–13511 CrossRef CAS PubMed
.
- P. A. Vanlandingham and B. P. Ceresa, Rab7 regulates late endocytic trafficking downstream of multivesicular body biogenesis and cargo sequestration, J. Biol. Chem., 2009, 284(18), 12110–12124 CrossRef CAS PubMed
.
- E.-L. Eskelinen, Roles of LAMP-1 and LAMP-2 in lysosome biogenesis and autophagy, Mol. Aspects Med., 2006, 27(5–6), 495–502 CrossRef CAS PubMed
.
- X. Ma, Y. Wu, S. Jin, Y. Tian, X. Zhang, Y. Zhao, L. Yu and X.-J. Liang, Gold nanoparticles induce autophagosome accumulation through size-dependent nanoparticle uptake and lysosome impairment, ACS Nano, 2011, 5(11), 8629–8639 CrossRef CAS PubMed
.
- C. Zhang, X. Feng, L. He, Y. Zhang and L. Shao, The interrupted effect of autophagic flux and lysosomal function induced by graphene oxide in p62-dependent apoptosis of F98 cells, J. Nanobiotechnol., 2020, 18(1), 1–17 CrossRef PubMed
.
- X. Zhang, H. Zhang, X. Liang, J. Zhang, W. Tao, X. Zhu, D. Chang, X. Zeng, G. Liu and L. Mei, Iron oxide nanoparticles induce autophagosome accumulation through multiple mechanisms: lysosome impairment, mitochondrial damage, and ER stress, Mol. Pharmaceutics, 2016, 13(7), 2578–2587 CrossRef CAS PubMed
.
- I. Schütz, T. Lopez-Hernandez, Q. Gao, D. Puchkov, S. Jabs, D. Nordmeyer, M. Schmudde, E. Rühl, C. M. Graf and V. Haucke, Lysosomal dysfunction caused by cellular accumulation of silica nanoparticles, J. Biol. Chem., 2016, 291(27), 14170–14184 CrossRef PubMed
.
- B. Ronan, O. Flamand, L. Vescovi, C. Dureuil, L. Durand, F. Fassy, M.-F. Bachelot, A. Lamberton, M. Mathieu and T. Bertrand, A highly potent and selective Vps34 inhibitor alters vesicle trafficking and autophagy, Nat. Chem. Biol., 2014, 10(12), 1013–1019 CrossRef CAS PubMed
.
- G. Y. Chen, C. L. Chen, H. Y. Tuan, P. X. Yuan, K. C. Li, H. J. Yang and Y. C. Hu, Graphene oxide triggers toll-like receptors/autophagy responses in vitro and inhibits tumor growth in vivo, Adv. Healthcare Mater., 2014, 3(9), 1486–1495 CrossRef CAS PubMed
.
- X. Feng, L. Chen, W. Guo, Y. Zhang, X. Lai, L. Shao and Y. Li, Graphene oxide induces p62/SQSTM-dependent apoptosis through the impairment of autophagic flux and lysosomal dysfunction in PC12 cells, Acta Biomater., 2018, 81, 278–292 CrossRef CAS PubMed
.
- A. Taheriazam, G. G. Y. Abad, S. Hajimazdarany, M. H. Imani, S. Ziaolhagh, M. A. Zandieh, S. D. Bayanzadeh, S. Mirzaei, M. R. Hamblin and M. Entezari, Graphene oxide nanoarchitectures in cancer biology: nano-modulators of autophagy and apoptosis, J. Controlled Release, 2023, 354, 503–522 CrossRef CAS PubMed
.
- M. Wei, Z. Fu, C. Wang, W. Zheng, S. Li and W. Le, Graphene oxide nanocolloids induce autophagy-lysosome dysfunction in mouse embryonic stem cells, J. Biomed. Nanotechnol., 2019, 15(2), 340–351 CrossRef CAS PubMed
.
- D. Szklarczyk, R. Kirsch, M. Koutrouli, K. Nastou, F. Mehryary, R. Hachilif, A. L. Gable, T. Fang, N. T. Doncheva and S. Pyysalo, The STRING database in 2023: protein–protein association networks and functional enrichment analyses for any sequenced genome of interest, Nucleic Acids Res., 2023, 51(D1), D638–D646 CrossRef CAS PubMed
.
- N. Mahmoudi and M. Mahmoudi, Effects of cholesterol on biomolecular corona, Nat. Nanotechnol., 2023, 1–3 Search PubMed
.
- H. Stenmark, Rab GTPases as coordinators of vesicle traffic, Nat. Rev. Mol. Cell Biol., 2009, 10(8), 513–525 CrossRef CAS PubMed
.
- T. Bhuin and J. K. Roy, Rab proteins: the key regulators of intracellular vesicle transport, Exp. Cell Res., 2014, 328(1), 1–19 CrossRef CAS PubMed
.
- R. Prekeris, The art of “cut and run”: the role of Rab14 GTPase in regulating N-cadherin shedding and cell motility, Dev. Cell, 2012, 22(5), 909–910 CrossRef CAS PubMed
.
- M. Yan, D. Ciano-Oliveira, S. Grinstein and W. S. Trimble, Coronin function is required for chemotaxis and phagocytosis in human neutrophils, J. Immunol., 2007, 178(9), 5769–5778 CrossRef CAS PubMed
.
- V. Gyurkovska, R. Murtazina, S. F. Zhao, S. Shikano, Y. Okamoto and N. Segev, Dual function of Rab1A in secretion and autophagy: hypervariable domain dependence, Life Sci. Alliance, 2023, 6(5), e202201810 CrossRef CAS PubMed
.
- Y. Liu, Y. Endo, D. Iwaki, M. Nakata, M. Matsushita, I. Wada, K. Inoue, M. Munakata and T. Fujita, Human M-ficolin is a secretory protein that activates the lectin complement pathway, J. Immunol., 2005, 175(5), 3150–3156 CrossRef CAS PubMed
.
- C. Honoré, S. Rørvig, L. Munthe-Fog, T. Hummelshøj, H. O. Madsen, N. Borregaard and P. Garred, The innate pattern recognition molecule Ficolin-1 is secreted by monocytes/macrophages and is circulating in human plasma, Mol. Immunol., 2008, 45(10), 2782–2789 CrossRef PubMed
.
- S. Decherchi and A. Cavalli, Thermodynamics and kinetics of drug-target binding by molecular simulation, Chem. Rev., 2020, 120(23), 12788–12833 CrossRef CAS PubMed
.
- A. V. Singh, M. Varma, P. Laux, S. Choudhary, A. K. Datusalia, N. Gupta, A. Luch, A. Gandhi, P. Kulkarni and B. Nath, Artificial intelligence and machine learning disciplines with the potential to improve the nanotoxicology and nanomedicine fields: a comprehensive review, Arch. Toxicol., 2023, 97(4), 963–979 CrossRef CAS PubMed
.
- R. J. Hickman, P. Bannigan, Z. Bao, A. Aspuru-Guzik and C. Allen, Self-driving laboratories: a paradigm shift in nanomedicine development, Matter, 2023, 6(4), 1071–1081 CrossRef CAS PubMed
.
-
P. Tan, X. Chen, H. Zhang, Q. Wei and K. Luo, Artificial intelligence aids in development of nanomedicines for cancer management, Seminars in Cancer Biology, Elsevier, 2023 Search PubMed
.
-
A. Skepu, B. Phakathi, M. Makgoka, Z. Mbita, B. P. Damane, D. Demetriou and Z. Dlamini, AI and Nanomedicine in Realizing the Goal of Precision Medicine: Tailoring the Best Treatment for Personalized Cancer Treatment, Artificial Intelligence and Precision Oncology: Bridging Cancer Research and Clinical Decision Support, Springer, 2023, pp. 181–194 Search PubMed
.
- N. Liessi, L. Maragliano, V. Castagnola, M. Bramini, F. Benfenati and A. Armirotti, Isobaric labeling proteomics allows a high-throughput investigation of protein corona orientation, Anal. Chem., 2020, 93(2), 784–791 CrossRef PubMed
.
- V. Castagnola, L. Deleye, A. Podestà, E. Jaho, F. Loiacono, D. Debellis, M. Trevisani, D. Z. Ciobanu, A. Armirotti and F. Pisani, Interactions of Graphene Oxide and Few-Layer Graphene with the Blood–Brain Barrier, Nano Lett., 2023, 23(7), 2981–2990 CrossRef CAS PubMed
.
- J. Chong and J. Xia, Using MetaboAnalyst 4.0 for Metabolomics Data Analysis, Interpretation, and Integration with Other Omics Data, Methods Mol. Biol., 2020, 2104, 337–360 CrossRef CAS PubMed
.
- P. S. Gromski, Y. Xu, H. L. Kotze, E. Correa, D. I. Ellis, E. G. Armitage, M. L. Turner and R. Goodacre, Influence of missing values substitutes on multivariate analysis of metabolomics data, Metabolites, 2014, 4(2), 433–452 CrossRef PubMed
.
- R. A. van den Berg, H. C. Hoefsloot, J. A. Westerhuis, A. K. Smilde and M. J. van der Werf, Centering, scaling, and transformations: improving the biological information content of metabolomics data, BMC Genomics, 2006, 7, 142 CrossRef PubMed
.
- G. Zhou, J. Ewald and J. Xia, OmicsAnalyst: a comprehensive web-based platform for visual analytics of multi-omics data, Nucleic Acids Res., 2021, 49(W1), W476–W482 CrossRef CAS PubMed
.
- V. B. O’Donnell, E. A. Dennis, M. J. O. Wakelam and S. Subramaniam, LIPID MAPS: serving the next generation of lipid researchers with tools, resources, data, and training, Sci. Signal., 2019, 12(563), eaaw2964 CrossRef PubMed
.
- T. Cajka and O. Fiehn, Toward Merging Untargeted and Targeted Methods in Mass Spectrometry-Based Metabolomics and Lipidomics, Anal. Chem., 2016, 88(1), 524–545 CrossRef CAS PubMed
.
- R. A. Spicer, R. Salek and C. Steinbeck, Compliance with minimum information guidelines in public metabolomics repositories, Sci. Data, 2017, 4, 170137 CrossRef PubMed
.
- M. Gillespie, B. Jassal, R. Stephan, M. Milacic, K. Rothfels, A. Senff-Ribeiro, J. Griss, C. Sevilla, L. Matthews and C. Gong, The reactome pathway knowledgebase 2022, Nucleic Acids Res., 2022, 50(D1), D687–D692 CrossRef CAS PubMed
.
- A. Biederbick, H. Kern and H. Elsässer, Monodansylcadaverine (MDC) is a specific in vivo marker for autophagic vacuoles, Eur. J. Cell Biol., 1995, 66(1), 3–14 CAS
.
|
This journal is © The Royal Society of Chemistry 2024 |
Click here to see how this site uses Cookies. View our privacy policy here.