Evaluating atmospheric mercury (Hg) uptake by vegetation in a chemistry-transport model†
Received
27th January 2022
, Accepted 21st April 2022
First published on 22nd April 2022
Abstract
Mercury (Hg), a neurotoxic heavy metal, is transferred to marine and terrestrial ecosystems through atmospheric transport. Recent studies have highlighted the role of vegetation uptake as a sink for atmospheric elemental mercury (Hg0) and a source of Hg to soils. However, the global magnitude of the Hg0 vegetation uptake flux is highly uncertain, with estimates ranging 1000–4000 Mg per year. To constrain this sink, we compare simulations in the chemical transport model GEOS-Chem with a compiled database of litterfall, throughfall, and flux tower measurements from 93 forested sites. The prior version of GEOS-Chem predicts median Hg0 dry deposition velocities similar to litterfall measurements from Northern hemisphere temperate and boreal forests (∼0.03 cm s−1), yet it underestimates measurements from a flux tower study (0.04 cm s−1vs. 0.07 cm s−1) and Amazon litterfall (0.05 cm s−1vs. 0.17 cm s−1). After revising the Hg0 reactivity within the dry deposition parametrization to match flux tower and Amazon measurements, GEOS-Chem displays improved agreement with the seasonality of atmospheric Hg0 observations in the Northern midlatitudes. Additionally, the modelled bias in Hg0 concentrations in South America decreases from +0.21 ng m−3 to +0.05 ng m−3. We calculate a global flux of Hg0 dry deposition to land of 2276 Mg per year, approximately double previous model estimates. The Amazon rainforest contributes 29% of the total Hg0 land sink, yet continued deforestation and climate change threatens the rainforest's stability and thus its role as an important Hg sink. In an illustrative worst-case scenario where the Amazon is completely converted to savannah, GEOS-Chem predicts that an additional 283 Mg Hg per year would deposit to the ocean, where it can bioaccumulate in the marine food chain. Biosphere–atmosphere interactions thus play a crucial role in global Hg cycling and should be considered in assessments of future Hg pollution.
Environmental significance
Vegetation uptake is one of largest sinks of atmospheric mercury (Hg) from the atmosphere and a major source of Hg to soils. We better quantify its importance to the global biogeochemical Hg cycle by updating an atmospheric chemistry model with information from newly available measurement datasets. Our revised dry deposition scheme yields improved model agreement with atmospheric Hg seasonality in Northern midlatitudes and Hg concentrations in South America. We calculate a dry deposition flux to land that is approximately double previous model estimates. Using our revised model, we also illustrate the potential importance of land-atmosphere feedbacks: the conversion of the Amazon rainforest to savannah leads to an additional transfer of 283 Mg Hg each year to the ocean due to a reduced land sink.
|
1. Introduction
Mercury (Hg) is a neurotoxic heavy metal that bioaccumulates in marine and terrestrial food webs as methylmercury (MeHg). Approximately 8000 Mg per year of Hg are emitted to the atmosphere each year, of which 30% are emitted directly by anthropogenic sources, 10% are from natural geogenic sources, and 60% are re-emissions of anthropogenic Hg that was previously deposited to land and water.1 Atmospheric Hg is transported to remote regions due to its long lifetime of around 6 months before being ultimately removed by wet and dry deposition.2,3 Recent studies have emphasized the role that vegetation plays in the dry deposition of elemental mercury (Hg0), with estimates of this sink ranging from 1000 to 4046 Mg per year.4–6 Isotopic evidence suggests that atmospheric Hg0 deposition is the source of 57–94% of all Hg in soils.5 Due to this link between atmospheric Hg and the biosphere, atmospheric Hg levels can be altered by seasonality and trends in vegetation productivity.4 Climate change and anthropogenic activities could disturb the Hg vegetation sink through multiple processes, e.g., deforestation,7 CO2 fertilization,8 vegetation species shifts,9 drought,10 and ice storms.11 In particular, there is a risk that the Amazon, the largest rainforest on the planet, could transition into a savannah-like ecosystem due to anthropogenic pressures (i.e. deforestation) and climate change.12–14 An accurate representation of Hg0 vegetation uptake in the global Hg budget is essential for predicting the impact of these perturbations on Hg cycling. However, until now there has been only narrow validation of atmospheric Hg chemistry models with observations of vegetation uptake, mainly focusing on selected sites in North America.9,15–17
Almost all atmospheric Hg chemistry models (including GEOS-Chem, ECHMERIT, GEM-MACH-Hg, and GLEMOS) use a resistance-based dry deposition scheme.15,18 In these schemes, canopy uptake processes are parametrized as a function of leaf area index (LAI) and land cover type.19,20 There is considerable uncertainty associated with the model parameters related to Hg dry deposition.5 The evaluation of model parametrizations is complicated by the systematic biases inherent to different experimental methods quantifying the uptake of Hg by vegetation (Table 1). Litterfall measurements are used to determine the amount of Hg taken up by foliage over a growing season, which isotopic evidence suggests mainly originate from atmospheric Hg0 uptake as opposed to oxidized mercury (Hg2+).21–23 Measurements of throughfall (water falling through the forest canopy) capture another portion of dry deposited Hg, washing off Hg adsorbed to canopy surfaces.24 Isotope evidence suggests that 34% to 82% of Hg in throughfall is derived from adsorbed atmospheric Hg0.25 However, litterfall and throughfall measurements do not account for uptake of Hg by woody tissues, mosses, and lichens in forest ecosystems.6,25 Whole ecosystem net Hg0 exchange has been measured directly by micrometeorological methods, which employ tower measurements and flux-gradient calculations.24,26 Micrometeorological techniques also measure net ecosystem exchange fluxes at higher time resolutions (30 min) than throughfall and litterfall measurements. Despite being the more accurate technique, there is currently only one annual micrometeorological measurement from a temperate deciduous forest site26 to compare with atmospheric model predictions.
Table 1 Review of measurement methods quantifying Hg vegetation uptake, compared in this study with GEOS-Chem simulated Hg0 dry deposition in forested areas
Name |
Description |
Species of Hg |
Potential biases when comparing with modelled Hg0 dry deposition |
Demers et al.;21 Jiskra et al.;22 Zheng et al.23
Wang et al.25
Throughfall is not directly compared to modelled Hg0 dry deposition.
|
Litterfall |
Collecting and analyzing plant litter to measure net Hg uptake in leaves over the growing season |
Hg0a |
Missing uptake from woody tissues, lichens, mosses, and soil; missing Hg0 contribution from throughfall; foliar re-emission not explicitly modelled |
Throughfall |
Measuring flux of Hg washed off leaves during precipitation events with a collector placed under the canopy |
Hg0, Hg2+b |
—c |
Total foliar uptake |
Calculated as litterfall + throughfall − open field wet deposition |
Hg0, Hg2+ |
Missing uptake from woody tissues, lichens, mosses, and soil; includes Hg2+ contribution from throughfall; foliar re-emission not explicitly modelled |
Micrometeorological flux tower methods |
Using Hg0 tower measurements and flux-gradient calculations to calculate whole net-ecosystem exchange |
Hg0 |
Only one annual measurement available in forested areas;26 if soil emissions are high, net-ecosystem exchange underestimates Hg0 vegetation uptake; foliar re-emission not explicitly modelled |
Past studies have calculated a broad range of magnitudes for the global Hg vegetation sink, depending on the monitoring data and scaling approach used. Wang et al.27 estimated that 1180 ± 710 Mg Hg per year deposits as litterfall, using a compilation of litterfall data and statistical modeling. Total throughfall has been estimated to contribute 1338 Mg per year over forests, which includes both wet deposition and dry-deposited Hg washed off leaves.28 By extrapolating flux tower measurements from Harvard Forest, Obrist et al.26 calculated a global Hg0 uptake flux of 3162–3813 Mg per year, approximately a factor of three higher than the litterfall assessment.27 A new global database approach, considering other vegetation components in addition to litterfall, also reported a larger estimate for the global vegetation uptake flux: 2705 ± 504 Mg per year.6 It remains unclear how these larger fluxes can be accommodated within the global Hg budget and whether they are compatible with other observational constraints in the Hg cycle, e.g., atmospheric concentrations, wet deposition fluxes, and isotopic tracers.29 Global atmospheric models like GEOS-Chem are useful tools for evaluating these broader constraints on the global Hg budget. However, recent work suggests that global atmospheric models underestimate vegetation uptake compared to tropical litterfall data5 and net exchange fluxes measured over a midlatitude deciduous forest.26
In this study, we amend the Hg0 dry deposition scheme in GEOS-Chem to integrate information derived from a broad dataset of available vegetation uptake measurements. We focus our comparison between model and measurements in forested sites, since forests are expected to have the largest contribution to vegetation uptake.30 By adjusting the biological reactivity of Hg0 in the dry deposition scheme, we propose model configurations that are compatible with different vegetation uptake measurement methods (litterfall; total foliar uptake; micrometeorological net Hg0 exchange, see Table 1). We evaluate the impacts of these adjustments on the modelled atmospheric Hg seasonality and spatial distribution. Using the model configuration that best matches available observational constraints, we provide improved estimates of the global magnitude of the vegetation sink and other Hg budget terms. We explore the effect of an extreme scenario for Amazon land cover change on the fate of atmospheric Hg and discuss its implications for future Hg cycling. Finally, we discuss future directions for the development of model parametrizations of Hg vegetation uptake.
2. Materials and methods
2.1 Compilation of litterfall, throughfall, and dry deposition measurements
To evaluate the performance of the GEOS-Chem dry deposition scheme in simulating Hg0 dry deposition over forests, we compiled an observational database of vegetation uptake measurements for forested areas. We focused on studies which measured litterfall, throughfall, and/or open field wet deposition. For study sites where all three quantities were measured, we calculated a total foliar uptake flux = litterfall + throughfall − open field wet deposition, which represents the total dry deposited Hg to foliage.24 In addition, we evaluate the model against net ecosystem exchange flux of Hg0 at a temperate deciduous hardwood forest site.26
We used previous reviews of Hg dry deposition as starting points for the database.24,31,32 To identify more recent litterfall studies, we screened 334 results from a Web of Science (Clarivate Analytics) search with the term “mercury litter*“. In total, the database contains 79 publications with measurement-based estimates of Hg uptake fluxes. We extracted additional metadata from the compiled publications: geographic coordinates, altitude, year of study, and land cover type (ESI† Spreadsheet).
The database ranges temporally from 1987 to 2020. Regional trends in atmospheric Hg0 concentrations also affect the magnitude of Hg0 uptake fluxes over this time period; for example, Hg0 has decreased by ∼1–2% per year over North America and Europe from the 1990s to 2010s.33 To compare simulations directly with Hg fluxes, it would be necessary to conduct longer term simulations or reduce the dataset to a selected time period. Instead, we compare modeled Hg0 dry deposition velocities to calculated annual mean Hg0 dry deposition velocities
from the observations, using:
is the measured litterfall or total foliar uptake flux and
is the annual mean atmospheric Hg0 concentration. The atmospheric Hg0 concentrations are taken directly from the measurement study, when reported. Otherwise, we searched for nearby atmospheric measurement sites for the relevant time period in the Atmospheric Mercury Network (AMNet),34 Canadian Air and Precipitation Monitoring Network (CAPMoN),35 European Monitoring and Evaluation Programme (EMEP),36 and Global Mercury Observation System (GMOS).37 Hg0 observations are generally reported in units of ng m−3 at standard temperature and pressure (STP, 273 K and 1 atm), whereas litterfall measurements would have units of μg per m2 per year based on local conditions. Therefore, we apply a temperature and pressure correction using MERRA-2 meteorological data38 to adjust annual mean measured Hg0 concentrations from STP to local conditions. The applied correction yields 3 to 39% decreases in Hg0 concentrations at local conditions compared to STP.
In total, we determined dry deposition velocities at 92 sites based on litterfall measurements, at 33 sites based on total foliar uptake, and at one site based on micrometeorological measurements.26 The micrometeorological measurements were downloaded online39 and analyzed based on annual mean values of dry deposition fluxes and concentrations. The locations of these studies are shown in Fig. 1.
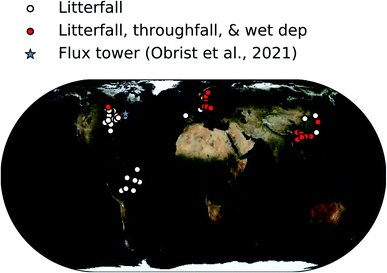 |
| Fig. 1 Map showing locations of forest sites where Hg0 vegetation uptake fluxes were measured in the compiled database. | |
Several issues must be considered when comparing modelled Hg0 dry deposition velocities with the different measurement datasets (Table 1). Litterfall measurements do not account for atmospheric Hg0 that is translocated from foliage into woody tissues, as well as additional uptake from lichens, mosses, and soils.6 Therefore, we consider litterfall measurements to be a lower bound for total Hg0 dry deposition to a forest. Total foliar uptake measurements include an additional contribution from throughfall; however, throughfall fluxes include a fraction of previously dry deposited Hg2+ that is washed off the leaf surface, and thus may not be directly comparable with modelled Hg0 deposition. The contribution of Hg2+ dry deposition to throughfall likely varies spatially, with potentially higher contributions in areas close to anthropogenic Hg2+ emissions. One study in the Southeastern Tibetan Plateau region identified a 34–82% contribution from Hg0 to throughfall using isotopic evidence.25 Micrometeorological measurements of net ecosystem Hg0 exchange may be the most accurate proxy of total Hg0 vegetation uptake.26 Since net Hg0 exchange is measured, derived fluxes can include a negative contribution from soil Hg0 emissions; yet, Obrist et al.26 did not find any periods of net Hg0 emissions from the forest floor during their study. Given the uncertainties of the comparison between modelled Hg0 dry deposition and Hg vegetation uptake measurements, we use other available measurements (e.g., of atmospheric Hg concentrations) as an additional independent constraint on Hg0 dry deposition (Sections 3.2 and 3.3).
The exchange of Hg between the canopy and atmosphere is bi-directional;40 all measurement methods studied here (litterfall, throughfall, and net ecosystem exchange) determine the net uptake flux rather than the gross uptake flux. The GEOS-Chem model does not explicitly include a parametrization for re-emission of Hg0 from foliar surfaces, so the modelled deposition fluxes should also be considered as net fluxes. GEOS-Chem calculates Hg0 emission fluxes from soil separately from the dry deposition scheme, using a parametrization depending on solar radiation and soil Hg concentration.16
2.2 GEOS-Chem description
We employ version 12.8.1 of the chemical transport model GEOS-Chem, whose Hg simulation is described by Horowitz et al.2 We run the mercury simulation globally at 2.0° × 2.5° resolution and 47 vertical layers. The model's atmospheric transport is driven by MERRA-2 assimilated meteorological data.38 The model calculates atmospheric mercury transport in three tracers: elemental mercury, Hg0, divalent mercury, Hg2+, and particulate-bound divalent mercury, HgP. Most of the simulations in this study use the GEOS-Chem v12.8.1 Hg chemical scheme, which considers bromine (Br) to be the primary Hg0 oxidant2 and employs monthly mean Br oxidant concentrations from Schmidt et al.41 Additional simulations (see Section 2.4 and Table 2) were conducted in version 12.8.1 of GEOS-Chem but with the new Hg chemical mechanism from Shah et al.3 In the updated chemical mechanism, new reactions were added for the two-step oxidation of Hg0 to Hg2+ and the photolysis of gas phase Hg+ and Hg2+ compounds, based on new laboratory and computational data.42,43 Whereas Br was the primary Hg0 oxidant in the prior chemical scheme,2 Br and hydroxyl (OH) radicals play comparable roles in the net oxidation of Hg0 in the new chemical scheme.3 Shah et al.3 also use updated Br oxidant fields that include the effect of sea-salt aerosol debromination and correct issues with heterogeneous recycling of Br radicals.44 In both chemical mechanisms, aqueous reduction of Hg2+ to Hg0 is parametrized as a function of the NO2 photolysis rate and organic aerosol concentration, with a tuning parameter employed to optimize agreement with Hg0 observations.2 Partitioning between gaseous and particulate Hg2+ is calculated according to Amos et al.45 as a function of temperature and mass concentration of fine particulate matter (PM2.5). Wet deposition removes Hg2+ and HgP from the atmosphere according to gas45 and particulate46 parametrizations.
Table 2 Description of GEOS-Chem Hg simulations
Simulation name |
Description |
Hg0 biological reactivity (f0) |
Reduction coefficienta (α) |
In GEOS-Chem, the photoreduction rate of aqueous-phase Hg2+–organic complexes is parametrized as α jNO2 [OA] [Hg2+(aq)], where α is a tuned coefficient, jNO2 is the local photolysis rate of NO2, and [OA] is the mass concentration of organic aerosol at STP.
In the new chemical scheme, photoreduction of aqueous Hg2+–organic complexes is parametrized differently, as α jNO2 [Hg2+P (org)], where [Hg2+P (org)] is the concentration of particulate Hg2+–organic complexes. Thus, the α coefficients cannot be directly compared with earlier GEOS-Chem versions.
|
BASE |
Reference GEOS-Chem version 12.8.1 |
10−5 |
0.16 |
OBRIST |
Matching flux tower measurements at Harvard Forest26 |
3 × 10−5 |
0.16 |
OBRIST_R |
Matching Obrist et al.26 measurements; increased Hg2+ reduction |
3 × 10−5 |
0.33 |
AMAZON_L |
Lower bound for Amazon: matching Hg litterfall flux from sites in Fostier et al.31 |
9 × 10−5 (Amazon) |
0.33 |
3 × 10−5 (elsewhere) |
AMAZON_U |
Upper bound for Amazon: matching total foliar uptake of Hg from Fostier et al.31,90 |
0.2 (Amazon) |
0.33 |
3 × 10−5 (elsewhere) |
NEWCHEM |
Reference GEOS-Chem simulation based on new Hg chemical scheme from Shah et al.3 |
10−5 |
0.004b |
NEWCHEM_D |
Increasing dry deposition to parameters from AMAZON_U; new chemistry3 |
0.2 (Amazon) |
0.010b |
3 × 10−5 (elsewhere) |
The Hg0 dry deposition scheme in GEOS-Chem uses a resistance-based approach,19,20 similar to many other chemical transport models.15,18 Dry deposition is parametrized assuming three types of resistances in series: aerodynamic, boundary, and surface resistance. Aerodynamic and boundary resistances depend on grid scale meteorological variables (e.g., temperature and windspeed), whereas surface resistance depends on the land use category, surface parameters (e.g., leaf area index, LAI), chemical compound-specific parameters, and meteorology. The surface resistance is determined by calculating the effect of parallel resistances of vegetation stomata, cuticles, lower canopy surfaces, and the ground surface (including soil and leaf litter). To incorporate compound-specific effects, the Henry's law constant (H*) and biological reactivity (f0) of a compound are used as scaling factors in the resistance calculations (see Section S1† for full set of equations included in the dry deposition model). Compounds that are more soluble (higher H*) and/or more biologically reactive (higher f0) have faster dry deposition velocities,20 since dissolution and reaction are two parallel pathways for deposition to surfaces (Section S1†). A grid cell in GEOS-Chem can contain multiple land use categories; the dry deposition velocity is computed separately for each land use category within a grid cell and then averaged with area-weighting to produce the overall dry deposition velocity in a grid cell.
The implementation of dry deposition in GEOS-Chem uses 11 land use categories, with the categories relevant to this paper being deciduous forests, coniferous forests, and tropical rainforests.47 Since information about grid surface parameters in GEOS-Chem (e.g., LAI) is grouped into 73 land use categories according to Gibbs,48 for the dry deposition scheme each of the 73 land categories is assigned to one of the 11 dry deposition categories. We use a reprocessed version of the Moderate Resolution Imaging Spectroradiometer (MODIS) LAI product49 in the dry deposition scheme. For Hg0 in GEOS-Chem, H* is set to 0.11 M atm−1 (ref. 50) and f0 was originally set to 10−5 to match the observations over North America available at the time.51 Over the ocean, Hg0 dry deposition is calculated as part of the air–sea gas exchange model52 instead of through the resistance-based scheme. Gaseous Hg2+ is biologically unreactive (f0 = 0) but highly soluble (H* = 1014 M atm−1) and the dry deposition of particulate Hg is calculated according to aerosol deposition scheme.53,54
Gridded emissions from anthropogenic sources are based on the 2015 inventory55,56 prepared for the 2018 Global Mercury Assessment.1 All other emissions follow Horowitz et al.2 Monthly mean surface ocean Hg concentrations are taken from Horowitz et al.,2 based on two-way coupling of GEOS-Chem with the MITgcm 3-D mercury ocean model.57 To account for prompt recycling of Hg2+, 20% of Hg2+ wet and dry deposition to terrestrial surfaces are re-emitted to the atmosphere as Hg0 directly after depositing.51
2.3 Offline dry deposition model
We ported the GEOS-Chem dry deposition code to Python so that it could be run with meteorological inputs in offline calculations (https://doi.org/10.5281/zenodo.6498126). This offline model enables quicker simulations of dry deposition velocities than running the full online chemistry-transport model. The inputs to the model are hourly MERRA2 meteorological data for 2015 (including temperature, wind speed, cloud fraction, radiation fluxes), land surface information for the 73 land categories,48 and weekly-averaged satellite-derived LAI maps for 2015.49 The output of the offline model is hourly dry deposition velocity maps for 2015. With an hourly time resolution for meteorological inputs, the offline model shows small enough errors for the current study application compared to online GEOS-Chem velocities (mean grid cell error ∼ 0.1%) (ESI,† Section S2).
In this study, we run offline simulations with the biological reactivity (f0) of Hg0 varying from 10−5 to 1 and compare the resultant deposition velocity maps with the observational database. We focus on uncertainties in f0 since this parameter covers multiple potential biological reaction processes in vegetation uptake and therefore would be difficult to determine experimentally. In GEOS-Chem, f0 of Hg0 has been set to 10−5 based on Selin et al.,51 yet other modelling exercises use a higher Hg0 biological reactivity of 0.1 and 0.2.58,59 We chose to vary the chemical compound-specific parameter f0 instead of general resistance parameters within the dry deposition scheme, since the resistance parameters have been validated in model comparisons with other chemical compounds60 and would require a wider scope of chemical compounds measurements to evaluate (see Section S5† for further discussion).
The compiled observations are made at forest sites, whereas the corresponding model grid cells (2.0° × 2.5°) can cover multiple land types. We account for the specific land use category of the observation site in the offline model calculations following an approach by Silva and Heald.60 To do so, we categorize each observation site within the 11 land type categories used in the dry deposition scheme (e.g., broadleaf, coniferous, and tropical rain forest). We alter the offline model's inputs so that the land type at the observation site accounts for 100% of the model grid cell. The LAI of the grid cell is set to the average LAI of the observed forest category within the grid cell. The surface roughness of the grid cell is adjusted to 1 m when it drops below that threshold, since 1 m is representative of forested areas.61 We present the effects of the land-surface adjustment in the ESI (Section S3†). In the case of Hg0, this adjustment is especially important for coastal grid cells, where the low deposition velocity over the water-covered sub-grid cell reduces the overall grid cell deposition velocity.
2.4 GEOS-Chem simulations
We conducted a four-year spinup of the GEOS-Chem Hg model (2010–2013) to create initial conditions for the subsequent simulations. Based on the comparison between the offline dry deposition model and observations (presented in Section 3.1), we ran two-year online simulations (2014–2015) for the different dry deposition settings (Table 2). Allowing for a one-year equilibration in the troposphere, we analyze year 2015 in the simulations. A reference BASE simulation (f0 = 10−5) is conducted with GEOS-Chem version 12.8.1. Three more simulations with different f0 parameter values were run: an OBRIST simulation with f0 set to 3 × 10−5 globally, as well as simulations where f0 is increased regionally in the Amazon rainforest to 9 × 10−5 (AMAZON_L simulation) and 0.2 (AMAZON_U simulation) and set to 3 × 10−5 elsewhere.
In order to balance the enhanced removal of Hg0 due to dry deposition in the OBRIST simulation, we adjust the reduction rate of Hg2+ to match annual mean observations of atmospheric Hg0 concentrations in the Northern Hemisphere in the OBRIST_R simulation. The larger reduction rate coefficient is also used in the AMAZON_L and AMAZON_U simulations. The Hg2+ reduction rate coefficient has been used as a tuning parameter in past developments of the GEOS-Chem model.2,3 Furthermore, here we have applied an offline three-box atmospheric model to reduce the computational expense of tuning the reduction rate (Section S4†).
To compare the effects of increased Hg0 dry deposition in the updated Hg chemistry mechanism,3 we run a reference simulation for the updated chemistry (NEWCHEM), as well as a simulation accounting for higher Hg0 dry deposition globally (f0 = 3 × 10−5) and in the Amazon (f0 = 0.2) and faster aqueous Hg2+ reduction (NEWCHEM_D) (Table 2). All emission settings remain unchanged from earlier simulations (Section 2.2).
As an illustrative scenario, we investigate the impact of the Amazon transitioning from rainforest to savannah (savannization) on the global vegetation uptake of Hg and the atmospheric Hg budget in GEOS-Chem. We follow the approach used by Alves de Oliveira et al.62 as a worst-case scenario for Amazon savannization due to deforestation and climate change. To set up the simulation, we replace all tropical rain forest land cover in South America with savanna land cover. We substitute Amazon LAI with mean time-varying LAI from current savanna areas in South America. Since the Hg budget of the savannization simulation is compared to AMAZON_U, f0 is set to 3 × 10−5 (no rainforest is present in Amazon, so the regional f0 setting is not applied).
3. Results and discussion
3.1 Comparison of offline model with vegetation uptake measurements
We compare the dry deposition velocities predicted by the offline model simulations to observations of litterfall (separating outside and inside the Amazon), total foliar uptake, and flux tower measurements (Fig. 2). The interquartile range of Hg0 dry deposition velocities predicted by the BASE version of GEOS-Chem (f0 = 10−5), 0.035–0.039 cm s−1, matches well with litterfall measurements outside the Amazon, 0.020–0.041 cm s−1 (Fig. 2). These measurements are located in mainly temperate and boreal forests in North America, Europe, and East Asia. Previous studies have also found good agreement between GEOS-Chem Hg0 dry deposition and a USA subset of litterfall measurements.9,16 When the throughfall dry deposition component is added to litterfall, the median dry deposition velocity of observations increases by ∼36%, from 0.028 cm s−1 to 0.038 cm s−1. The interquartile ranges of BASE (0.034–0.041 cm s−1) also overlap with total foliar uptake observations (0.030–0.055 cm s−1). The modelled dry deposition velocity at Harvard Forest (0.038 cm s−1) underpredicts the flux tower measured value26 (0.072 cm s−1) by a factor of 1.9. Even larger underestimates are seen when comparing the BASE model to deposition velocities derived from Amazon litterfall (−72% from observation median) and Amazon total foliar uptake (−87% from observation median).31
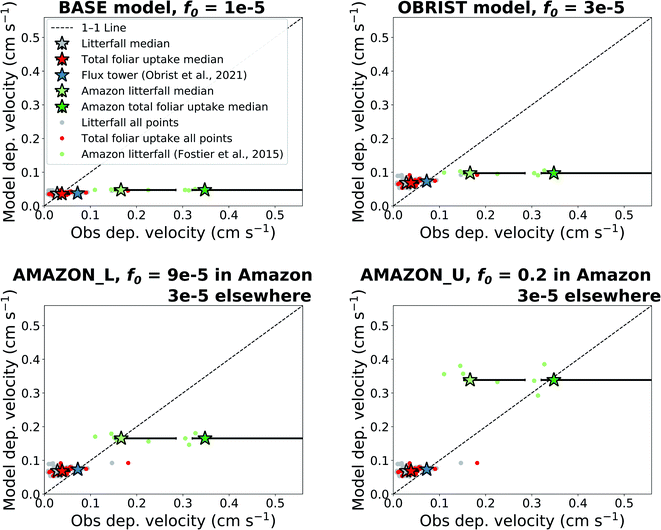 |
| Fig. 2 Comparing observed dry deposition velocities with modelled values extracted from the location coordinates. Four offline model settings are presented, with varying assumptions for the biological reactivity (f0) of Hg0 in the dry deposition scheme. Individual site measurements are indicated with filled circles and overall medians of measurement types are indicated with filled stars. Error bars show the interquartile range of measurements over different measurement locations. Model interquartile ranges are generally smaller than the size of the markers. | |
When the value of f0 is adjusted to 3 × 10−5 (OBRIST simulation), the model matches the Hg0 deposition velocity derived from the flux tower measurements. In this case, the model overestimates median total foliar uptake deposition velocities by 78%. This model-based estimate is only based on one flux tower measurement site, and more flux tower measurements of Hg0 exchange over forests would be necessary to confirm the stronger sink measured by Obrist et al.26 Nevertheless, the larger dry deposition velocities measured by flux tower measurements compared to litterfall could be explained by litterfall measurements not accounting for uptake from woody tissues, lichens and mosses.6
The model continues to underestimate Amazon litterfall measurements when f0 of Hg0 is set globally to 3 × 10−5 (OBRIST) (Fig. 2). A previous study with a different atmospheric Hg model that uses a resistance-based approach to dry deposition (GEM-MACH-Hg) also underestimated deposition fluxes inferred from tropical rainforest litterfall measurements.5 We therefore applied a new regional f0 parameter for the rainforest land type in South America. Setting f0 to 9 × 10−5 within only the Amazon rainforest and retaining f0 = 3 × 10−5 elsewhere (AMAZON_L simulation), leads to a match for the Amazon litterfall deposition velocity (0.17 cm s−1). We take this value as a lower bound estimate for Hg0 dry deposition in the Amazon, since litterfall does not account for all vegetation uptake (Table 1). As an upper bound estimate for the Amazon dry deposition velocity, we use the total foliar uptake estimate, litterfall + throughfall − open field wet deposition (49 + 72 − 18 = 103 μg per m2 per year), reported by Fostier et al.31 Dividing this by the atmospheric Hg0 concentration measured in the GMOS station at Manaus, we calculate a deposition velocity of 0.35 cm s−1. This estimate is considered an upper bound for Amazon Hg0 uptake since lower throughfall fluxes (13.7–23.6 μg per m2 per year) have been reported for other regions of the Brazilian Amazon.63 A recent study from the Peruvian Amazon measured a slightly higher total foliar uptake flux (128 μg per m2 per year); however, the study area is impacted by regional artisanal and small-scale gold mining (ASGM) emissions.64 The upper observed bound of Amazon dry deposition velocity from Fostier et al.31 would correspond to a f0 value of 0.2 (AMAZON_U simulation).
Instead of altering f0, another approach to adjusting the Hg0 dry deposition velocity for the Amazon rainforest would have been to alter the dry deposition canopy resistances for the rainforest land category. We show that an equivalent solution for Hg0 deposition in the Amazon can be found by adjusting resistances (Section S5†). However, since adjusting resistances would affect all dry depositing compounds in GEOS-Chem and perturb their atmospheric budgets, for this study we proceeded with adjustments to the Hg0-specific parameter, f0. Given that both approaches would yield the same Hg0 dry deposition velocities, the choice of approach should not affect the subsequent GEOS-Chem analyses in this study. Nevertheless, this issue should be revisited with a more extensive comparison of multiple chemical compounds, as GEOS-Chem (Section S5†) and another model with a resistance-based approach65 have also been found to underestimate the ozone dry deposition velocity in the Amazon.
3.2 Seasonality of atmospheric Hg0
We compare the atmospheric Hg0 seasonal cycle from online GEOS-Chem simulations (Table 2) with observations in Fig. 3. Under the BASE simulation, GEOS-Chem is within the 1σ spatial variability of Northern Hemisphere (NH) midlatitude observations in all months except April (Fig. 3a). However, the seasonal amplitude of the BASE model (0.17 ng m−3) is only half the observed seasonal amplitude (0.34 ng m−3), showing a weaker summertime minimum in total gaseous mercury (TGM). In the BASE version of GEOS-Chem, seasonality in Hg oxidation drives the summertime minimum in the NH.2,66 In the OBRIST simulation, with increased Hg0 dry deposition, the model's seasonal amplitude (0.28 ng m−3) is closer to observations (0.34 ng m−3) although the mean concentrations are biased low compared to observations. This bias can be offset by adjusting the reduction of Hg2+ to Hg0 in organic aerosol, which has been done to tune previous GEOS-Chem Hg model versions.2,3 The OBRIST_R simulation illustrates that when the Hg2+ reduction rate coefficient is increased by a factor of 2.1, the model based on flux tower measurements can match both the mean (within 1σ) and the seasonal amplitude of observed Hg0 in the NH midlatitudes (Fig. 3a). More information about the tuning procedure for Hg reduction, as well as comparisons with observed Hg0 concentrations globally, can be found in the ESI† (Section S4 and Fig. S9†).
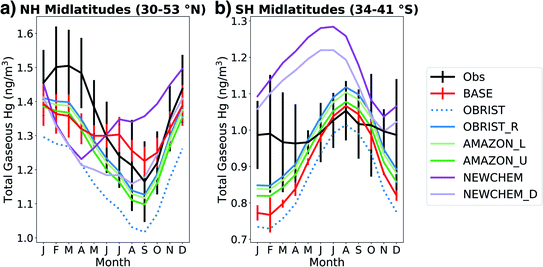 |
| Fig. 3 Seasonal cycle of surface total gaseous mercury (TGM) concentrations in the (a) Northern Hemisphere (NH) midlatitudes (16 observation stations) and (b) Southern Hemisphere (SH) midlatitudes (3 observation stations). Lines indicate monthly means of simulated or observed Hg0 at station locations and error bars indicate standard deviations between measurement sites. To improve plot clarity, model error bars are only shown for the BASE simulation. Measured atmospheric Hg concentrations are sourced from compilations18,91 and are courtesy of Hélène Angot. | |
The improved agreement of the modelled seasonal cycle upon increasing the strength of Hg0 dry deposition supports previous work suggesting that the Hg0 seasonal cycle in the NH is driven mainly by the vegetation sink, not oxidation chemistry.4,5 The simulations that further increase Amazon uptake of Hg0 (AMAZON_L and AMAZON_U) are similar in seasonality to the OBRIST_R simulation in the NH midlatitudes. Thus, the three simulations OBRIST_R, AMAZON_L, and AMAZON_U can all be compatible with NH midlatitude Hg0 observations and suggest that the vegetation uptake in the BASE model is too small.
Shah et al.3 updated the Hg chemical scheme in GEOS-Chem (NEWCHEM), leading to substantial differences in the seasonal cycle of TGM in the NH midlatitudes (Fig. 3a). In the NEWCHEM simulation, the minimum Hg0 concentrations occur in April, whereas BASE chemistry leads to a minimum in September. This difference is caused by the seasonality of Br oxidant concentrations; NEWCHEM uses updated Br fields44 that show maximum concentrations in the NH in late winter–spring, whereas the Br fields used by BASE41 are maximum in summer. When dry deposition is enhanced in the new chemistry version (NEWCHEM_D), the minimum TGM concentration is shifted to August, closer to observations. The seasonal amplitude of NEWCHEM_D (0.25 ng m−3) is similar to the updated simulations using the previous chemical mechanism (OBRIST_R, AMAZON_L, and AMAZON_U), illustrating that NH midlatitude TGM seasonality can be a useful constraint for Hg0 dry deposition.4 However, NEWCHEM_D still shows too low TGM concentrations throughout the boreal spring (March–May), suggesting that further investigation into the uncertainties of atmospheric Hg chemistry is required.
In the Southern Hemisphere (SH) midlatitudes, the observations show a relatively flat seasonal cycle in Hg0 (amplitude of 0.09 ng m−3) (Fig. 3b). Jiskra et al.4 have attributed this lack of seasonality to less land area in the SH, and thus a more minor vegetation sink. In contrast, the BASE version of GEOS-Chem shows a strong seasonal cycle (amplitude of 0.30 ng m−3), with a wintertime maximum and summertime minimum likely driven by oxidation chemistry.2,3 The seasonal amplitude is largely unchanged (0.29 ng m−3) when dry deposition is increased in the OBRIST simulation, because of the limited influence of land in the SH. When the reduction of Hg2+ is increased in OBRIST_R, the seasonality is slightly flattened, with an amplitude of 0.27 ng m−3. Further adjustments to the Amazon dry deposition flux (AMAZON_L and AMAZON_U) lead to reductions in mean SH midlatitude TGM, but limited changes in seasonal amplitude. Simulations with the new chemical mechanism (NEWCHEM and NEWCHEM_D) also show too strong TGM seasonality in the SH (amplitudes of 0.22 and 0.25 ng m−3) and generally overestimate SH midlatitude concentrations (annual mean bias of +0.18 and +0.13 ng m−3). Uncertainties with the GEOS-Chem Hg chemical scheme as well as seasonality of Br oxidant concentrations could be responsible for the bias in TGM seasonality in the SH.3 A further uncertainty is the seasonality of Hg0 emissions from the ocean, which would likely be a dominating source in the SH.2
3.3 Atmospheric Hg0 in South America
Previous validations of GEOS-Chem2,3,66 have paid limited attention to South America, where until now very few measurements were available. Given the importance of the Amazon region as an area of dry deposition (accounting for 29% of the global Hg0 dry deposition to land in AMAZON_U), we evaluate GEOS-Chem results against recent atmospheric Hg measurements from South America37,67,68 (Fig. 4). Atmospheric Hg0 observations from Chacaltaya, a mountain-top observatory in the Bolivian Andes, showed an increasing trend between normal (July 2014–May 2015) and El-Niño Southern Oscillation (ENSO) conditions (June 2015–February 2016).67 We focus our comparison on normal conditions at Chacaltaya, since the Hg0 ocean emissions in GEOS-Chem would not be representative of ENSO conditions.2 In general, Hg concentrations measured in stations surrounded by tropical rainforest (Manaus, Suriname, and Chacaltaya) are all overestimated by the BASE version of GEOS-Chem (BASE mean over stations: 1.27 ng m−3vs. observed mean: 1.03 ng m−3). Only at the midlatitude site Bariloche, which would be less affected by the Amazon vegetation uptake sink, is the BASE model within the measurement 1σ variability. Passive Hg samplers, as part of the Latin American Passive Air sampling Network (LAPAN) network, are a new source of information for Hg cycling in South America.68 We compare the mean of passive-sampler measured annual Hg concentrations from 22 background locations in South America with model simulations (Fig. 4). The BASE simulation also overestimates the mean Hg concentration from the passive sampler network (1.14 ng m−3vs. 0.87 ng m−3), supporting the comparison with other measurement stations.
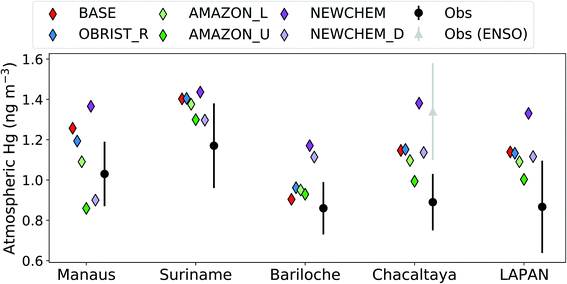 |
| Fig. 4 Comparing GEOS-Chem simulations to available South American observations of atmospheric Hg (LAPAN stands for Latin American Passive Sampling Network). Total gaseous mercury (TGM) concentrations for simulations and observations are compared at each site, except Suriname for which gaseous Hg0 (GEM) concentrations are compared. Error bars for observations indicate the 1σ temporal variability, except for LAPAN which shows the 1σ spatial variability between 22 sites. | |
The OBRIST_R simulation with increased Hg0 biological reactivity globally remains outside the measurement 1σ variability at South American sites, except for the midlatitude site Bariloche. With the adjustment to Amazon Hg0 biological reactivity in AMAZON_L and AMAZON_U, the bias in GEOS-Chem predictions are generally reduced at the South American sites (Fig. 4). Overall, the AMAZON_U simulation seems to agree best with Hg0 measurements, lying within the 1σ variability at all measurement sites except for Manaus, where the AMAZON_L simulation is closest. Our comparison with observations of atmospheric Hg0 concentrations in South America supports the conclusion that the vegetation uptake of Hg in the Amazon is currently underestimated in GEOS-Chem. In addition to dry deposition uncertainties, atmospheric Hg concentrations over South America may also be affected by uncertainties in soil re-emissions, artisanal and small-scale gold mining emissions, air–sea exchange, and volcanic emissions.1,15,67 Nevertheless, correcting GEOS-Chem to be in line with Amazon litterfall and throughfall data, helps reduce the South American Hg0 bias in GEOS-Chem from +0.21 ng m−3 (BASE) to +0.05 ng m−3 (AMAZON_U), averaged over the observations in Fig. 4. The new Hg chemical scheme,3 generally leads to higher Hg concentrations in the SH (Fig. 3b and 4). However, as with the old chemical scheme,2 we find that the enhancement of dry deposition globally and over the Amazon improves the overall South American Hg0 bias from +0.37 ng m−3 (NEWCHEM) to +0.15 ng m−3 (NEWCHEM_D).
3.4 Wet deposition fluxes of Hg
An additional observational constraint on atmospheric Hg cycling is measured wet deposition fluxes. Due to the relative insolubility of Hg0, wet deposition fluxes depend on the atmospheric concentrations of Hg2+. Our adjustments to increase the Hg0 dry deposition to land consequently result in less available Hg0 for oxidation and thus decrease the wet removal sink of Hg2+. The BASE version of GEOS-Chem underestimates observed annual mean wet deposition globally (7.5 μg m−2vs. 8.3 μg m−2, Fig. S10†). The mean simulated wet deposition decreases in AMAZON_U (4.5 μg m−2), increasing the model bias. However, other processes may explain the deviation between GEOS-Chem and wet deposition measurements. For example, GEOS-Chem underestimates Hg2+ in the free troposphere, likely due to uncertainties in atmospheric Hg oxidation, leading to less Hg removal by convective storms.3,69 Indeed, when the new chemistry scheme is applied from Shah et al.3 (NEWCHEM_D), Hg wet deposition increases over Europe compared to AMAZON_U (4.8 μg m−2vs. 3.4 μg m−2, Fig. S12†), but remains too low globally (4.9 μg m−2, Fig. S10†). Other studies have cited the difficulty of resolving Hg2+ hotspots and transport in global models,70,71 the speciation of anthropogenic Hg emissions,33,72 and the lack of Hg in coarse particles in GEOS-Chem73 as possible reasons for the wet deposition underestimation. Further analysis and observational constraints are required to investigate the influence of these uncertainties on Hg wet deposition.
3.5 Importance of Amazon rainforest as a Hg0 sink
Both litterfall flux and atmospheric Hg0 concentration measurements suggest that the Amazon rainforest is currently an important net Hg0 sink, yet this sink is at risk due to continued deforestation and climate change.14,74 Two tipping points have been suggested that could cause the rainforest to transition into a savannah biome: a temperature increase of 4 °C above preindustrial or 20–40% deforestation in the region.75 Once these thresholds are crossed, regional moisture recycling weakens to the extent that there is not enough available water to support tropical rainforest biomes.
To investigate the effect of Amazon savannization on regional and global Hg cycling, we simulated an extreme scenario where the entire Amazon rainforest is converted to savannah land cover, following the approach of Alves de Oliveira et al.62 In the AMAZON_U simulation, median modelled Hg0 dry deposition velocities in the Amazon rainforest are 0.3 cm s−1, while in the savannah scenario this decreases to 0.07 cm s−1 (Fig. 5a). Correspondingly, the Hg0 dry deposition over the Amazon region decreases by 63% in the savannah scenario (Fig. 5b), from 653 Mg per year to 246 Mg per year. Total Hg deposition to the ocean increases by 283 Mg per year, mostly spread evenly across the SH but with an intensified band in the eastern equatorial Pacific. In the absence of the Amazon rainforest uptake sink, the total Hg burden in the atmosphere increases by 194 Mg (+5%) compared to current conditions.
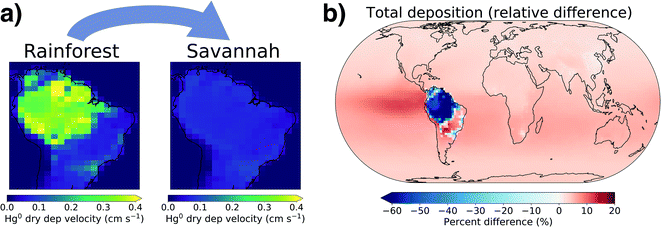 |
| Fig. 5 (a) GEOS-Chem modeled Hg0 dry deposition velocities shown for the Amazon under current land cover (left) and total conversion to savannah (right). (b) Map of the simulated change in total Hg deposition flux due to savannization. | |
In South America, the major direct anthropogenic source of Hg is from ASGM, emitting 340 Mg per year to the atmosphere.1 Much of the ASGM activities occur within the Amazon region.76,77 Due to uptake of Hg by vegetation, terrestrial ecosystems close to ASGM sources are threatened by high Hg exposures.64 The ASGM Hg0 emissions may change in the future as parties to the Minamata Convention on Mercury are obligated to take steps to reduce Hg use in ASGM.78 To check how much of the rainforest Hg0 sink is caused by regional emissions, we ran two scenarios with no South American anthropogenic Hg emissions and either existing rainforest conditions or full savannah conversion. Even when regional emissions are removed, savannization results in an additional 238 Mg per year Hg transferred to the ocean (83% of the effect calculated with current South American emissions). The Amazon rainforest is thus not only a sink of regional Hg but also global background Hg, due to the long atmospheric lifetime of Hg0.
Previous work has identified several processes that mobilize Hg during Amazon deforestation.31 Soil erosion and mobilization increases in deforested areas, with annual Hg mobilization expected to increase by 20–25% between 2014 and 2030 in a Peruvian watershed affected by deforestation.79 Fires used to clear forested areas for agriculture have been estimated to emit 6.7 Mg Hg per year between 2000 and 2008 in the Brazilian Amazon.80 Deforested areas also show higher soil Hg emissions than forested areas, with soils releasing an estimated 2.3 g per Hg per ha in the first year after the fire.7 When scaled by the estimated deforestation rate from the Brazilian Amazon in 2021 (1.3 × 106 ha per year),81 the additional soil source after deforestation would correspond to 3.0 Mg Hg per year. With our current study, we quantified another process leading to increased mobilization of Hg to marine ecosystems: a decreased land sink that leads to additional Hg deposition to the ocean. The estimated increased Hg deposition flux to the ocean (283 Mg per year) represents two-thirds of the total anthropogenic South American emissions (340 Mg per year), emphasizing the importance of Amazon rainforest trends for future Hg contamination. This shift in Hg deposition from land to the ocean increases the overall mobility of Hg in the surface environment since Hg in soil reservoirs has a longer mean residence time than in the surface ocean.82 Increased exposure to Hg health risks may not be limited to marine ecosystems, since deforestation can increase Hg methylation rates in freshwater aquatic ecosystems.83 Efforts to mitigate climate change and avoid deforestation will be crucial to avoid additional mobilization of Hg in the environment, meaning that the goals of the Minamata Convention intersect with other international treaties such as the Paris Climate Accords.78
4. Environmental and modelling implications
Fig. 6 illustrates the global atmospheric Hg budget under the original GEOS-Chem simulation BASE and the updated simulation AMAZON_U (other simulation budgets are shown in Table S2†). The atmospheric Hg0 burden (∼3600 Mg) remains similar in both simulations, given that Hg2+ reduction was increased to compensate for increased Hg0 deposition in AMAZON_U. Overall, dry deposition of Hg0 to land approximately doubles from 1200 Mg per year in BASE to 2276 Mg per year in AMAZON_U. The new global dry deposition flux to land (2276 Mg per year) lies in between the estimate from a previous global litterfall assessment (1180 Mg per year)27 and the estimate extrapolated globally from one flux tower measurement site over a midlatitude forest (3490 Mg per year).26 The new estimate from GEOS-Chem is within the lower end of the range (2705 ± 504 Mg per year) derived by a new assessment that considered uptake to moss, lichens, and woody tissues in addition to litterfall.6 Total Hg deposition to land in AMAZON_U (2960 Mg per year) also matches with the simulated value from GEM-MACH-Hg (2800 Mg per year).5 With increased vegetation uptake of Hg0, AMAZON_U shows improved seasonality of atmospheric Hg0 at NH midlatitude sites and reduced biases in Hg0 over South America, compared to the BASE simulation. In AMAZON_U, the increased flux of Hg0 dry deposition and reduced fluxes of Hg2+ deposition (−1118 Mg per year, −19% compared to BASE) helps resolve part of the model bias identified in isotopic Δ200Hg mass balance studies.29,84 The enhanced role of the land sink in the improved version of GEOS-Chem highlights the importance of considering biosphere-atmosphere feedbacks during climate change in future projections of Hg pollution. Our study also emphasizes the necessity of expanding litterfall monitoring as a lower bound estimate for the magnitude Hg0 uptake and conducting additional flux tower studies in different biomes to monitor net ecosystem exchange. Specifically, there are no litterfall flux measurements of Hg from rainforests in Africa or Southeast Asia, which would be invaluable to investigate whether similar uptake processes occur there as in the Amazon.
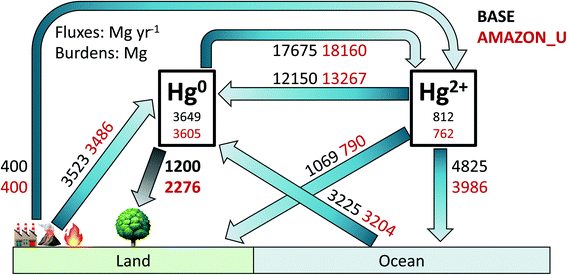 |
| Fig. 6 Atmospheric Hg budget for BASE and AMAZON_U simulations for year 2015. Atmospheric burdens are listed in units Mg and fluxes in Mg per year. The Hg2+ burden and fluxes include both gaseous and particulate Hg2+. | |
Our study has improved the Hg0 dry deposition parametrization by evaluating GEOS-Chem with a wider array of field evidence (79 studies) than the original parametrization.51 Nevertheless, the model is still not able to predict observed deposition velocities from individual studies and observed values generally show more variance than modelled values (Fig. 2 and Section S7†). This result indicates that important controls on vegetation uptake could be missing from the GEOS-Chem dry deposition parametrization. For example, the current parametrization19,20 does not include tree species type or moisture availability, which have been found to influence the variability of foliar Hg uptake across Europe.10 Other studies highlighted the importance of water availability on plant uptake of ozone, especially when considering the impacts of future climate change, and their expanded parametrizations may serve as examples for future GEOS-Chem development.65,85 GEOS-Chem does not explicitly treat the re-emission of Hg0 from foliage, which isotopic evidence suggests is around 30% of the gross uptake flux.86 Other studies have developed bi-directional models of Hg0 exchange in the canopy;40,87 however, the modelled seasonality of Hg0 re-emissions88 disagrees with recent net ecosystem exchange measurements from Harvard Forest.26 Further combined analysis of field measurements and model parametrizations is essential to implement improved atmosphere–biosphere exchange schemes in chemistry transport models.15,89
5. Conclusion
Dry deposition of Hg0 is a major removal pathway of atmospheric Hg and controls the influx of Hg to terrestrial ecosystems. We conducted model simulations in GEOS-Chem that correspond to different observational constraints of Hg0 vegetation uptake. The original BASE simulation agrees with litterfall and total foliar uptake data from outside of the Amazon, yet it cannot capture the seasonality of atmospheric Hg0 in the NH midlatitudes and it overestimates atmospheric Hg0 in South America. We suggest a higher biological reactivity of Hg0 (f0) than the BASE simulation: 0.2 within the Amazon rainforest, in line with available Amazon litterfall and throughfall data,31 and 3 × 10−5 elsewhere, in line with the existing flux tower study.26 This simulation (AMAZON_U) better matches the seasonality of NH midlatitudes Hg0 and agrees best with available atmospheric Hg0 observations in South America. The revised simulation leads to a global Hg0 land sink (2276 Mg per year) that is almost double the original simulation (1200 Mg per year). The Amazon rainforest contributes approximately 29% of the total Hg0 land sink worldwide, but continuing deforestation endangers this sink. In the extreme scenario where the entire rainforest is converted to savannah, an additional 283 Mg per year Hg is transferred to the ocean where it can bioaccumulate in the marine food chain. The improved version of GEOS-Chem can be applied in future studies to understand Hg cycle feedbacks between the terrestrial biosphere and the atmosphere.
Conflicts of interest
There are no conflicts to declare.
Acknowledgements
This research was funded by an Early Postdoc.Mobility grant (P2EZP2_195424) from the Swiss National Science Foundation and a grant (#1924148) from the US National Science Foundation. M. J. acknowledges funding from the Swiss National Science Foundation grant PZ00P2_174101. We thank Daniel Obrist, Katrina MacSween, and Benjamin Geyman for useful discussions during the study preparation. We thank Frits Steenhuisen and Simon Wilson for providing AMAP/UNEP gridded anthropogenic Hg emissions for 2015. We thank Hélène Angot for provision of the Hg measurement data, processing of emission data, and assistance with GEOS-Chem simulations. We thank Frank Wania and Isabel Quant for providing atmospheric Hg0 data from the LAPAN passive sampler network in South America. We acknowledge the researchers involved in measuring and publishing atmospheric mercury measurements as part of the CAPMoN, EMEP, MDN, AMNet, and GMOS networks. We acknowledge researchers involved in collection of the litterfall, throughfall, and wet deposition measurements included in our compilation dataset.
References
-
UNEP, Global Mercury Assessment 2018, UN Environment Programme, Chemicals and Health Branch Geneva, Switzerland, 2019 Search PubMed.
- H. M. Horowitz, D. J. Jacob, Y. Zhang, T. S. Dibble, F. Slemr, H. M. Amos, J. A. Schmidt, E. S. Corbitt, E. A. Marais and E. M. Sunderland, A new mechanism for atmospheric mercury redox chemistry: implications for the global mercury budget, Atmos. Chem. Phys., 2017, 17, 6353–6371 CrossRef CAS.
- V. Shah, D. J. Jacob, C. P. Thackray, X. Wang, E. M. Sunderland, T. S. Dibble, A. Saiz-Lopez, I. Černušák, V. Kellö, P. J. Castro, R. Wu and C. Wang, Improved Mechanistic Model of the Atmospheric Redox Chemistry of Mercury, Environ. Sci. Technol., 2021, 55, 14445–14456 CrossRef CAS PubMed.
- M. Jiskra, J. E. Sonke, D. Obrist, J. Bieser, R. Ebinghaus, C. L. Myhre, K. A. Pfaffhuber, I. Wängberg, K. Kyllönen, D. Worthy, L. G. Martin, C. Labuschagne, T. Mkololo, M. Ramonet, O. Magand and A. Dommergue, A vegetation control on seasonal variations in global atmospheric mercury concentrations, Nat. Geosci., 2018, 11, 244–250 CrossRef CAS.
- J. Zhou, D. Obrist, A. Dastoor, M. Jiskra and A. Ryjkov, Vegetation uptake of mercury and impacts on global cycling, Nat. Rev. Earth Environ., 2021, 2, 269–284 CrossRef.
- J. Zhou and D. Obrist, Global Mercury Assimilation by Vegetation, Environ. Sci. Technol., 2021, 55, 14245–14257 CrossRef CAS PubMed.
- A. Carpi, A. H. Fostier, O. R. Orta, J. C. dos Santos and M. Gittings, Gaseous mercury emissions from soil following forest loss and land use changes: Field experiments in the United States and Brazil, Atmos. Environ., 2014, 96, 423–429 CrossRef CAS.
- O. Hararuk, D. Obrist and Y. Luo, Modelling the sensitivity of soil mercury storage to climate-induced changes in soil carbon pools, Biogeosciences, 2013, 10, 2393–2407 CrossRef CAS.
- H. Zhang, C. D. Holmes and S. Wu, Impacts of changes in climate, land use and land cover on atmospheric mercury, Atmos. Environ., 2016, 141, 230–244 CrossRef CAS.
- L. Wohlgemuth, P. Rautio, B. Ahrends, A. Russ, L. Vesterdal, P. Waldner, V. Timmermann, N. Eickenscheidt, A. Fürst, M. Greve, P. Roskams, A. Thimonier, M. Nicolas, A. Kowalska, M. Ingerslev, P. Merilä, S. Benham, C. Iacoban, G. Hoch, C. Alewell and M. Jiskra, Physiological and climate controls on foliar mercury uptake by European tree species, Biogeosciences, 2022, 19, 1335–1353 CrossRef.
- Y. Yang, L. Meng, R. D. Yanai, M. Montesdeoca, P. H. Templer, H. Asbjornsen, L. E. Rustad and C. T. Driscoll, Climate change may alter mercury fluxes in northern hardwood forests, Biogeochemistry, 2019, 146, 1–16 CrossRef CAS.
- I. Amigo, When will the Amazon hit a tipping point?, Nature, 2020, 578, 505–508 CrossRef CAS PubMed.
-
O. Hoegh-Guldberg, D. Jacob, M. Taylor, M. Bindi, S. Brown, I. Camilloni, A. Diedhiou, R. Djalante, K. Ebi, F. Engelbrecht, J. Guiot, Y. Hijioka, S. Mehrotra, A. Payne, S. I. Seneviratne, A. Thomas, R. Warren and G. Zhou, in Global Warming of 1.5°C. An IPCC Special Report on the Impacts of Global Warming of 1.5°C above Pre-industrial Levels and Related Global Greenhouse Gas Emission Pathways, in the Context of Strengthening the Global Response to the Threat of Climate Change, ed. V. Masson-Delmotte, P. Zhai, H. O. Pörtner, D. Roberts, J. Skea, P. R. Shukla, A. Pirani, W. Moufouma-Okia, C. Péan, R. Pidcock, S. Connors, J. B. R. Matthews, Y. Chen, X. Zhou, M. I. Gomis, E. Lonnoy, T. Maycock, M. Tignor and T. Waterfield, World Meteorological Organization Technical Document, 2018 Search PubMed.
- C. A. Nobre, G. Sampaio, L. S. Borma, J. C. Castilla-Rubio, J. S. Silva and M. Cardoso, Land-use and climate change risks in the Amazon and the need of a novel sustainable development paradigm, Proc. Natl. Acad. Sci. U. S. A., 2016, 113, 10759–10768 CrossRef CAS PubMed.
- T. R. Khan, D. Obrist, Y. Agnan, N. E. Selin and J. A. Perlinger, Atmosphere-terrestrial exchange of gaseous elemental mercury: parameterization improvement through direct comparison with measured ecosystem fluxes, Environ. Sci.: Processes Impacts, 2019, 21, 1699–1712 RSC.
- S. Song, N. E. Selin, A. L. Soerensen, H. Angot, R. Artz, S. Brooks, E.-G. Brunke, G. Conley, A. Dommergue, R. Ebinghaus, T. M. Holsen, D. A. Jaffe, S. Kang, P. Kelley, W. T. Luke, O. Magand, K. Marumoto, K. A. Pfaffhuber, X. Ren, G.-R. Sheu, F. Slemr, T. Warneke, A. Weigelt, P. Weiss-Penzias, D. C. Wip and Q. Zhang, Top-down constraints on atmospheric mercury emissions and implications for global biogeochemical cycling, Atmos. Chem. Phys., 2015, 15, 7103–7125 CrossRef CAS.
- V. L. St. Louis, J. A. Graydon, I. Lehnherr, H. M. Amos, E. M. Sunderland, K. A. St. Pierre, C. A. Emmerton, K. Sandilands, M. Tate, A. Steffen and E. R. Humphreys, Atmospheric Concentrations and Wet/Dry Loadings of Mercury at the Remote Experimental Lakes Area, Northwestern Ontario, Canada, Environ. Sci. Technol., 2019, 53, 8017–8026 CrossRef PubMed.
- O. Travnikov, H. Angot, P. Artaxo, M. Bencardino, J. Bieser, F. D'Amore, A. Dastoor, F. De Simone, M. del C. Diéguez, A. Dommergue, R. Ebinghaus, X. B. Feng, C. N. Gencarelli, I. M. Hedgecock, O. Magand, L. Martin, V. Matthias, N. Mashyanov, N. Pirrone, R. Ramachandran, K. A. Read, A. Ryjkov, N. E. Selin, F. Sena, S. Song, F. Sprovieri, D. Wip, I. Wängberg and X. Yang, Multi-model study of mercury dispersion in the atmosphere: atmospheric processes and model evaluation, Atmos. Chem. Phys., 2017, 17, 5271–5295 CrossRef CAS.
- Y. Wang, D. J. Jacob and J. A. Logan, Global simulation of tropospheric O 3 -NO x -hydrocarbon chemistry: 1. Model formulation, J. Geophys. Res., 1998, 103, 10713–10725 CrossRef CAS.
- M. L. Wesely, Parameterization of surface resistances to gaseous dry deposition in regional-scale numerical models, Atmos. Environ., 1989, 23, 1293–1304 CrossRef CAS.
- J. D. Demers, J. D. Blum and D. R. Zak, Mercury isotopes in a forested ecosystem: Implications for air-surface exchange dynamics and the global mercury cycle, Global Biogeochem. Cycles, 2013, 27, 222–238 CrossRef CAS.
- M. Jiskra, J. G. Wiederhold, U. Skyllberg, R.-M. Kronberg, I. Hajdas and R. Kretzschmar, Mercury Deposition and Re-emission Pathways in Boreal Forest Soils Investigated with Hg Isotope Signatures, Environ. Sci. Technol., 2015, 49, 7188–7196 CrossRef CAS PubMed.
- W. Zheng, D. Obrist, D. Weis and B. A. Bergquist, Mercury isotope compositions across North American forests: Mercury Isotopes Across U.S. Forests, Global Biogeochem. Cycles, 2016, 30, 1475–1492 CrossRef CAS.
- L. P. Wright, L. Zhang and F. J. Marsik, Overview of mercury dry deposition, litterfall, and throughfall studies, Atmos. Chem. Phys., 2016, 16, 13399–13416 CrossRef CAS.
- X. Wang, W. Yuan, C.-J. Lin, J. Luo, F. Wang, X. Feng, X. Fu and C. Liu, Underestimated Sink of Atmospheric Mercury in a Deglaciated Forest Chronosequence, Environ. Sci. Technol., 2020, 54, 8083–8093 CrossRef CAS PubMed.
- D. Obrist, E. M. Roy, J. L. Harrison, C. F. Kwong, J. W. Munger, H. Moosmüller, C. D. Romero, S. Sun, J. Zhou and R. Commane, Previously unaccounted atmospheric mercury deposition in a midlatitude deciduous forest, Proc. Natl. Acad. Sci. U. S. A., 2021, 118, e2105477118 CrossRef CAS PubMed.
- X. Wang, Z. Bao, C.-J. Lin, W. Yuan and X. Feng, Assessment of Global Mercury Deposition through Litterfall, Environ. Sci. Technol., 2016, 50, 8548–8557 CrossRef CAS PubMed.
- X. Fu, W. Zhu, H. Zhang, J. Sommar, B. Yu, X. Yang, X. Wang, C.-J. Lin and X. Feng, Depletion of atmospheric gaseous elemental mercury by plant uptake at Mt.Changbai, Northeast China, Atmos. Chem. Phys., 2016, 16, 12861–12873 CrossRef CAS.
- X. Fu, M. Jiskra, X. Yang, N. Marusczak, M. Enrico, J. Chmeleff, L.-E. Heimbürger-Boavida, F. Gheusi and J. E. Sonke, Mass-Independent Fractionation of Even and Odd Mercury Isotopes during Atmospheric Mercury Redox Reactions, Environ. Sci. Technol., 2021, 55, 10164–10174 CrossRef CAS PubMed.
- D. Obrist, J. L. Kirk, L. Zhang, E. M. Sunderland, M. Jiskra and N. E. Selin, A review of global environmental mercury processes in response to human and natural perturbations: Changes of emissions, climate, and land use, Ambio, 2018, 47, 116–140 CrossRef PubMed.
- A. H. Fostier, J. J. Melendez-Perez and L. Richter, Litter mercury deposition in the Amazonian rainforest, Environ. Pollut., 2015, 206, 605–610 CrossRef CAS PubMed.
- J. Zhou, B. Du, L. Shang, Z. Wang, H. Cui, X. Fan and J. Zhou, Mercury fluxes, budgets, and pools in forest ecosystems of China: A review, Crit. Rev. Environ. Sci. Technol., 2020, 50, 1411–1450 CrossRef CAS.
- Y. Zhang, D. J. Jacob, H. M. Horowitz, L. Chen, H. M. Amos, D. P. Krabbenhoft, F. Slemr, V. L. St. Louis and E. M. Sunderland, Observed decrease in atmospheric mercury explained by global decline in anthropogenic emissions, Proc. Natl. Acad. Sci. U. S. A., 2016, 113, 526–531 CrossRef CAS PubMed.
- D. A. Gay, D. Schmeltz, E. Prestbo, M. Olson, T. Sharac and R. Tordon, The Atmospheric Mercury Network: measurement and initial examination of an ongoing atmospheric mercury record across North America, Atmos. Chem. Phys., 2013, 13, 11339–11349 CrossRef CAS.
- A. Cole, A. Steffen, C. Eckley, J. Narayan, M. Pilote, R. Tordon, J. Graydon, V. St. Louis, X. Xu and B. Branfireun, A Survey of Mercury in Air and Precipitation across Canada: Patterns and Trends, Atmosphere, 2014, 5, 635–668 CrossRef.
- K. Tørseth, W. Aas, K. Breivik, A. M. Fjæraa, M. Fiebig, A. G. Hjellbrekke, C. Lund Myhre, S. Solberg and K. E. Yttri, Introduction to the European Monitoring and Evaluation Programme (EMEP) and observed atmospheric composition change during 1972–2009, Atmos. Chem. Phys., 2012, 12, 5447–5481 CrossRef.
- F. Sprovieri, N. Pirrone, M. Bencardino, F. D'Amore, F. Carbone, S. Cinnirella, V. Mannarino, M. Landis, R. Ebinghaus, A. Weigelt, E.-G. Brunke, C. Labuschagne, L. Martin, J. Munthe, I. Wängberg, P. Artaxo, F. Morais, H. de M. J. Barbosa, J. Brito, W. Cairns, C. Barbante, M. del C. Diéguez, P. E. Garcia, A. Dommergue, H. Angot, O. Magand, H. Skov, M. Horvat, J. Kotnik, K. A. Read, L. M. Neves, B. M. Gawlik, F. Sena, N. Mashyanov, V. Obolkin, D. Wip, X. B. Feng, H. Zhang, X. Fu, R. Ramachandran, D. Cossa, J. Knoery, N. Marusczak, M. Nerentorp and C. Norstrom, Atmospheric mercury concentrations observed at ground-based monitoring sites globally distributed in the framework of the GMOS network, Atmos. Chem. Phys., 2016, 16, 11915–11935 CrossRef CAS PubMed.
- R. Gelaro, W. McCarty, M. J. Suárez, R. Todling, A. Molod, L. Takacs, C. A. Randles, A. Darmenov, M. G. Bosilovich, R. Reichle, K. Wargan, L. Coy, R. Cullather, C. Draper, S. Akella, V. Buchard, A. Conaty, A. M. da Silva, W. Gu, G.-K. Kim, R. Koster, R. Lucchesi, D. Merkova, J. E. Nielsen, G. Partyka, S. Pawson, W. Putman, M. Rienecker, S. D. Schubert, M. Sienkiewicz and B. Zhao, The Modern-Era Retrospective Analysis for Research and Applications, Version 2 (MERRA-2), J. Clim., 2017, 30, 5419–5454 CrossRef PubMed.
-
D. Obrist, W. Munger and R. Commane, Atmospheric Gaseous Elemental Mercury Fluxes at Harvard Forest EMS Tower 2019-2020 Ver 1, 2021, DOI: DOI:10.6073/pasta/0d9d994b069bc1d2a11eb9a7dc783bff.
- L. P. Wright and L. Zhang, An approach estimating bidirectional air-surface exchange for gaseous elemental mercury at AMNet sites, J. Adv. Model. Earth Syst., 2015, 7, 35–49 CrossRef.
- J. A. Schmidt, D. J. Jacob, H. M. Horowitz, L. Hu, T. Sherwen, M. J. Evans, Q. Liang, R. M. Suleiman, D. E. Oram, M. Le Breton, C. J. Percival, S. Wang, B. Dix and R. Volkamer, Modeling the observed tropospheric BrO background: Importance of multiphase chemistry and implications for ozone, OH, and mercury, J. Geophys. Res.: Atmos., 2016, 121, 11819–11835 CAS.
- T. S. Dibble, H. L. Tetu, Y. Jiao, C. P. Thackray and D. J. Jacob, Modeling the OH-Initiated Oxidation of Mercury in the Global Atmosphere without Violating Physical Laws, J. Phys. Chem. A, 2020, 124, 444–453 CrossRef CAS PubMed.
- A. Saiz-Lopez, O. Travnikov, J. E. Sonke, C. P. Thackray, D. J. Jacob, J. Carmona-García, A. Francés-Monerris, D. Roca-Sanjuán, A. U. Acuña, J. Z. Dávalos, C. A. Cuevas, M. Jiskra, F. Wang, J. Bieser, J. M. C. Plane and J. S. Francisco, Photochemistry of oxidized Hg(I) and Hg(II) species suggests missing mercury oxidation in the troposphere, Proc. Natl. Acad. Sci. U. S. A., 2020, 117, 30949–30956 CrossRef CAS PubMed.
- X. Wang, D. J. Jacob, W. Downs, S. Zhai, L. Zhu, V. Shah, C. D. Holmes, T. Sherwen, B. Alexander, M. J. Evans, S. D. Eastham, J. A. Neuman, P. R. Veres, T. K. Koenig, R. Volkamer, L. G. Huey, T. J. Bannan, C. J. Percival, B. H. Lee and J. A. Thornton, Global tropospheric halogen (Cl, Br, I) chemistry and its impact on oxidants, Atmos. Chem. Phys., 2021, 21, 13973–13996 CrossRef CAS.
- H. M. Amos, D. J. Jacob, C. D. Holmes, J. A. Fisher, Q. Wang, R. M. Yantosca, E. S. Corbitt, E. Galarneau, A. P. Rutter, M. S. Gustin, A. Steffen, J. J. Schauer, J. A. Graydon, V. L. St. Louis, R. W. Talbot, E. S. Edgerton, Y. Zhang and E. M. Sunderland, Gas-particle partitioning of atmospheric Hg(II) and its effect on global mercury deposition, Atmos. Chem. Phys., 2012, 12, 591–603 CrossRef CAS.
- H. Liu, D. J. Jacob, I. Bey and R. M. Yantosca, Constraints from 210 Pb and 7 Be on wet deposition and transport in a global three-dimensional chemical tracer model driven by assimilated meteorological fields, J. Geophys. Res., 2001, 106, 12109–12128 CrossRef CAS.
- D. J. Jacob and S. C. Wofsy, Budgets of reactive nitrogen, hydrocarbons, and ozone over the Amazon forest during the wet season, J. Geophys. Res., 1990, 95, 16737 CrossRef CAS.
-
H. K. Gibbs, Olson's Major World Ecosystem Complexes Ranked by Carbon in Live Vegetation: an Updated Database Using the GLC2000 Land Cover Product (NDP-017b), 2006, https://www.osti.gov/biblio/1389498 Search PubMed.
- H. Yuan, Y. Dai, Z. Xiao, D. Ji and W. Shangguan, Reprocessing the MODIS Leaf Area Index products for land surface and climate modelling, Remote Sens. Environ., 2011, 115, 1171–1187 CrossRef.
- C.-J. Lin and S. O. Pehkonen, The chemistry of atmospheric mercury: a review, Atmos. Environ., 1999, 33, 2067–2079 CrossRef CAS.
- N. E. Selin, D. J. Jacob, R. M. Yantosca, S. Strode, L. Jaeglé and E. M. Sunderland, Global 3-D land-ocean-atmosphere model for mercury: Present-day versus preindustrial cycles and anthropogenic enrichment factors for deposition, Global Biogeochem. Cycles, 2008, 22, GB2011 Search PubMed.
- S. A. Strode, L. Jaeglé, N. E. Selin, D. J. Jacob, R. J. Park, R. M. Yantosca, R. P. Mason and F. Slemr, Air-sea exchange in the global mercury cycle, Global Biogeochem. Cycles, 2007, 21, GB1017 CrossRef.
- J. A. Fisher, D. J. Jacob, Q. Wang, R. Bahreini, C. C. Carouge, M. J. Cubison, J. E. Dibb, T. Diehl, J. L. Jimenez, E. M. Leibensperger, Z. Lu, M. B. J. Meinders, H. O. T. Pye, P. K. Quinn, S. Sharma, D. G. Streets, A. van Donkelaar and R. M. Yantosca, Sources, distribution, and acidity of sulfate–ammonium aerosol in the Arctic in winter–spring, Atmos. Environ., 2011, 45, 7301–7318 CrossRef CAS.
- L. Zhang, S. Gong, J. Padro and L. Barrie, A size-segregated particle dry deposition scheme for an atmospheric aerosol module, Atmos. Environ., 2001, 35, 549–560 CrossRef CAS.
-
F. Steenhuisen and S. J. Wilson, Geospatially Distributed (Gridded) Global Mercury Emissions to Air from Anthropogenic Sources in 2015, 2022, DOI: DOI:10.34894/SZ2KOI.
- F. Steenhuisen and S. J. Wilson, Development and application of an updated geospatial distribution model for gridding 2015 global mercury emissions, Atmos. Environ., 2019, 211, 138–150 CrossRef CAS.
- Y. Zhang, D. J. Jacob, S. Dutkiewicz, H. M. Amos, M. S. Long and E. M. Sunderland, Biogeochemical drivers of the fate of riverine mercury discharged to the global and Arctic oceans, Global Biogeochem. Cycles, 2015, 29, 854–864 CrossRef CAS.
- L. Zhang, L. P. Wright and P. Blanchard, A review of current knowledge concerning dry deposition of atmospheric mercury, Atmos. Environ., 2009, 43, 5853–5864 CrossRef CAS.
- L. Zhang, P. Blanchard, D. A. Gay, E. M. Prestbo, M. R. Risch, D. Johnson, J. Narayan, R. Zsolway, T. M. Holsen, E. K. Miller, M. S. Castro, J. A. Graydon, V. L. St. Louis and J. Dalziel, Estimation of speciated and total mercury dry deposition at monitoring locations in eastern and central North America, Atmos. Chem. Phys., 2012, 12, 4327–4340 CrossRef CAS.
- S. J. Silva and C. L. Heald, Investigating Dry Deposition of Ozone to Vegetation, J. Geophys. Res.: Atmos., 2018, 123, 559–573 CrossRef CAS.
- G. J. McRae, W. R. Goodin and J. H. Seinfeld, Development of a second-generation mathematical model for Urban air pollution—I. Model formulation, Atmos. Environ., 1982, 16, 679–696 CrossRef CAS.
- B. F. Alves de Oliveira, M. J. Bottino, P. Nobre and C. A. Nobre, Deforestation and climate change are projected to increase heat stress risk in the Brazilian Amazon, Commun. Earth Environ., 2021, 2, 207 CrossRef.
-
J. R. P. Peleja, Balanço de massas de mercúrio (Hg) total em duas microbacias da Amazônia Central, Instituto Nacional de Pesquisas da Amazônia-INPA, https://repositorio.inpa.gov.br/handle/1/11464, 2007 Search PubMed.
- J. R. Gerson, N. Szponar, A. A. Zambrano, B. Bergquist, E. Broadbent, C. T. Driscoll, G. Erkenswick, D. C. Evers, L. E. Fernandez, H. Hsu-Kim, G. Inga, K. N. Lansdale, M. J. Marchese, A. Martinez, C. Moore, W. K. Pan, R. P. Purizaca, V. Sánchez, M. Silman, E. A. Ury, C. Vega, M. Watsa and E. S. Bernhardt, Amazon forests capture high levels of atmospheric mercury pollution from artisanal gold mining, Nat. Commun., 2022, 13, 559 CrossRef CAS PubMed.
- M. Lin, S. Malyshev, E. Shevliakova, F. Paulot, L. W. Horowitz, S. Fares, T. N. Mikkelsen and L. Zhang, Sensitivity of Ozone Dry Deposition to Ecosystem-Atmosphere Interactions: A Critical Appraisal of Observations and Simulations, Global Biogeochem. Cycles, 2019, 33, 1264–1288 CrossRef CAS.
- C. D. Holmes, D. J. Jacob, E. S. Corbitt, J. Mao, X. Yang, R. Talbot and F. Slemr, Global atmospheric model for mercury including oxidation by bromine atoms, Atmos. Chem. Phys., 2010, 10, 12037–12057 CrossRef CAS.
- A. M. Koenig, O. Magand, P. Laj, M. Andrade, I. Moreno, F. Velarde, G. Salvatierra, R. Gutierrez, L. Blacutt, D. Aliaga, T. Reichler, K. Sellegri, O. Laurent, M. Ramonet and A. Dommergue, Seasonal patterns of atmospheric mercury in tropical South America as inferred by a continuous total gaseous mercury record at Chacaltaya station (5240 m) in Bolivia, Atmos. Chem. Phys., 2021, 21, 3447–3472 CrossRef CAS.
-
M. I. Quant, MSc thesis, University of Toronto, 2021, http://hdl.handle.net/1807/109628.
- C. D. Holmes, N. P. Krishnamurthy, J. M. Caffrey, W. M. Landing, E. S. Edgerton, K. R. Knapp and U. S. Nair, Thunderstorms Increase Mercury Wet Deposition, Environ. Sci. Technol., 2016, 50, 9343–9350 CrossRef CAS PubMed.
- X. Xu, X. Feng, H. Lin, P. Zhang, S. Huang, Z. Song, Y. Peng, T.-M. Fu and Y. Zhang, Modelling the High Mercury Wet deposition in the Southeastern US by WRF-GC, Geosci. Model Dev. Discuss., 2022, 1–21 Search PubMed.
- Y. Zhang, L. Jaeglé, A. van Donkelaar, R. V. Martin, C. D. Holmes, H. M. Amos, Q. Wang, R. Talbot, R. Artz, S. Brooks, W. Luke, T. M. Holsen, D. Felton, E. K. Miller, K. D. Perry, D. Schmeltz, A. Steffen, R. Tordon, P. Weiss-Penzias and R. Zsolway, Nested-grid simulation of mercury over North America, Atmos. Chem. Phys., 2012, 12, 6095–6111 CrossRef CAS.
- K. Liu, Q. Wu, L. Wang, S. Wang, T. Liu, D. Ding, Y. Tang, G. Li, H. Tian, L. Duan, X. Wang, X. Fu, X. Feng and J. Hao, Measure-Specific Effectiveness of Air Pollution Control on China's Atmospheric Mercury Concentration and Deposition during 2013–2017, Environ. Sci. Technol., 2019, 53, 8938–8946 CrossRef CAS PubMed.
- I. Cheng, L. Zhang and H. Mao, Relative contributions of gaseous oxidized mercury and fine and coarse particle-bound mercury to mercury wet deposition at nine monitoring sites in North America, J. Geophys. Res.: Atmos., 2015, 120, 8549–8562 CrossRef CAS.
- L. V. Gatti, L. S. Basso, J. B. Miller, M. Gloor, L. Gatti Domingues, H. L. G. Cassol, G. Tejada, L. E. O. C. Aragão, C. Nobre, W. Peters, L. Marani, E. Arai, A. H. Sanches, S. M. Corrêa, L. Anderson, C. Von Randow, C. S. C. Correia, S. P. Crispim and R. A. L. Neves, Amazonia as a carbon source linked to deforestation and climate change, Nature, 2021, 595, 388–393 CrossRef CAS PubMed.
- T. E. Lovejoy and C. Nobre, Amazon Tipping Point, Sci. Adv., 2018, 4, eaat2340 CrossRef PubMed.
- G. P. Asner, W. Llactayo, R. Tupayachi and E. R. Luna, Elevated rates of gold mining in the Amazon revealed through high-resolution monitoring, Proc. Natl. Acad. Sci. U. S. A., 2013, 110, 18454–18459 CrossRef CAS PubMed.
- F. Lobo, M. Costa, E. Novo and K. Telmer, Distribution of Artisanal and Small-Scale Gold Mining in the Tapajós River Basin (Brazilian Amazon) over the Past 40 Years and Relationship with Water Siltation, Remote Sens., 2016, 8, 579 CrossRef.
- H. Selin, S. E. Keane, S. Wang, N. E. Selin, K. Davis and D. Bally, Linking science and policy to support the implementation of the Minamata Convention on Mercury, Ambio, 2018, 47, 198–215 CrossRef PubMed.
- S. E. Diringer, A. J. Berky, M. Marani, E. J. Ortiz, O. Karatum, D. L. Plata, W. K. Pan and H. Hsu-Kim, Deforestation Due to Artisanal and Small-Scale Gold Mining Exacerbates Soil and Mercury Mobilization in Madre de Dios, Peru, Environ. Sci. Technol., 2020, 54, 286–296 CrossRef CAS PubMed.
- P. A. M. Michelazzo, A. H. Fostier, G. Magarelli, J. C. Santos and J. A. de Carvalho, Mercury emissions from forest burning in southern Amazon, Geophys. Res. Lett., 2010, 37, L09809 CrossRef.
- L. F. F. G. Assis, K. R. Ferreira, L. Vinhas, L. Maurano, C. Almeida, A. Carvalho, J. Rodrigues, A. Maciel and C. Camargo, TerraBrasilis: A Spatial Data Analytics Infrastructure for Large-Scale Thematic Mapping, ISPRS Int. J. Geo-Inf., 2019, 8, 513 CrossRef.
- H. M. Amos, D. J. Jacob, D. G. Streets and E. M. Sunderland, Legacy impacts of all-time anthropogenic emissions on the global mercury cycle, Global Biogeochem. Cycles, 2013, 27, 410–421 CrossRef CAS.
- J. R. Gerson, S. N. Topp, C. M. Vega, J. R. Gardner, X. Yang, L. E. Fernandez, E. S. Bernhardt and T. M. Pavelsky, Artificial lake expansion amplifies mercury pollution from gold mining, Sci. Adv., 2020, 6, eabd4953 CrossRef CAS PubMed.
- M. Jiskra, L.-E. Heimbürger-Boavida, M.-M. Desgranges, M. V. Petrova, A. Dufour, B. Ferreira-Araujo, J. Masbou, J. Chmeleff, M. Thyssen, D. Point and J. E. Sonke, Mercury stable isotopes constrain atmospheric sources to the ocean, Nature, 2021, 597, 678–682 CrossRef CAS PubMed.
- O. E. Clifton, D. L. Lombardozzi, A. M. Fiore, F. Paulot and L. W. Horowitz, Stomatal conductance influences interannual variability and long-term changes in regional cumulative plant uptake of ozone, Environ. Res. Lett., 2020, 15, 114059 CrossRef CAS.
- W. Yuan, J. Sommar, C.-J. Lin, X. Wang, K. Li, Y. Liu, H. Zhang, Z. Lu, C. Wu and X. Feng, Stable Isotope Evidence Shows Re-emission of Elemental Mercury Vapor Occurring after Reductive Loss from Foliage, Environ. Sci. Technol., 2019, 53, 651–660 CrossRef CAS PubMed.
- J. O. Bash, Description and initial simulation of a dynamic bidirectional air-surface exchange model for mercury in Community Multiscale Air Quality (CMAQ) model, J. Geophys. Res., 2010, 115, D06305 CrossRef.
- L. Zhang, Z. Wu, I. Cheng, L. P. Wright, M. L. Olson, D. A. Gay, M. R. Risch, S. Brooks, M. S. Castro, G. D. Conley, E. S. Edgerton, T. M. Holsen, W. Luke, R. Tordon and P. Weiss-Penzias, The Estimated Six-Year Mercury Dry Deposition Across North America, Environ. Sci. Technol., 2016, 50, 12864–12873 CrossRef CAS PubMed.
- E. W. Emerson, A. L. Hodshire, H. M. DeBolt, K. R. Bilsback, J. R. Pierce, G. R. McMeeking and D. K. Farmer, Revisiting particle dry deposition and its role in radiative effect estimates, Proc. Natl. Acad. Sci. U. S. A., 2020, 117, 26076–26082 CrossRef CAS PubMed.
- A. H. Fostier, M. C. Forti, J. R. Guimarães, A. J. Melfi, R. Boulet, C. M. Espirito Santo and F. J. Krug, Mercury fluxes in a natural forested Amazonian catchment (Serra do Navio, Amapá State, Brazil), Sci. Total Environ., 2000, 260, 201–211 CrossRef CAS PubMed.
-
AMAP/UN Environment, Technical Background Report for the Global Mercury Assessment 2018, Arctic Monitoring and Assessment Programme, Oslo, Norway/UN Environment Programme, Chemicals and Health Branch, Geneva, Switzerland, 2019 Search PubMed.
|
This journal is © The Royal Society of Chemistry 2022 |
Click here to see how this site uses Cookies. View our privacy policy here.