DOI:
10.1039/D0LC01216E
(Paper)
Lab Chip, 2021,
21, 1333-1351
An in vitro vascularized micro-tumor model of human colorectal cancer recapitulates in vivo responses to standard-of-care therapy†
Received
2nd December 2020
, Accepted 2nd February 2021
First published on 19th February 2021
Abstract
Around 95% of anti-cancer drugs that show promise during preclinical study fail to gain FDA-approval for clinical use. This failure of the preclinical pipeline highlights the need for improved, physiologically-relevant in vitro models that can better serve as reliable drug-screening and disease modeling tools. The vascularized micro-tumor (VMT) is a novel three-dimensional model system (tumor-on-a-chip) that recapitulates the complex human tumor microenvironment, including perfused vasculature, within a transparent microfluidic device, allowing real-time study of drug responses and tumor–stromal interactions. Here we have validated this microphysiological system (MPS) platform for the study of colorectal cancer (CRC), the second leading cause of cancer-related deaths, by showing that gene expression, tumor heterogeneity, and treatment responses in the VMT more closely model CRC tumor clinicopathology than current standard drug screening modalities, including 2-dimensional monolayer culture and 3-dimensional spheroids.
Introduction
Cancer currently accounts for about 25% of all US deaths.1 At the same time, fewer than 5% of drugs entering clinical trials ultimately receive FDA approval,2 with most anti-cancer agents failing in clinical trials despite showing promise during preclinical studies. The conclusion from this is that the current model systems used to develop new drugs are poor predictors of drug response in humans. Traditionally, drug candidates are first tested in 2-dimensional (2D) cell monocultures. However, cell growth in 2D versus 3D environments not only promotes phenotypic changes in cell morphology, response to stimuli, cell functions and gene expression patterns, but also alters response to therapeutic agents.3–7 Indeed, both normal and cancerous cells maintain their specific behaviors in the body owing to their 3D environment, which encompasses heterogeneous and dynamic cell–cell and cell–matrix interactions.8–10 Prior efforts to develop appropriate 3D tumor tissues have been limited to the generation of avascular spheroids in artificial matrix, which fail to recapitulate the structure of a vascularized tumor mass, are not viable for long-term study,11,12 and perhaps most importantly cannot be generated from invasive and often metastatic cell lines that have reduced expression of adhesion molecules. Although animal models have significantly advanced our understanding of complex diseases such as cancer, and are important in the drug development pipeline, these same models raise ethical issues, require substantial time and resources, and species-specific differences can impede clinical translation of results.13,14
To address the need for improved preclinical models, we have designed, fabricated and validated a microfluidic device that supports the formation of a perfused, vascularized micro-tumor (VMT) via co-culture of multiple cell types in an extracellular matrix.15–19 Importantly, growth of the tumor and delivery of therapeutics to the tumor is entirely dependent on “blood” flow through the living vascular network. Physiologic flow rates through the microvasculature are maintained by a gravity-driven pressure differential that does not require external pumps or valves.20 The VMT (aka ‘tumor-on-a-chip’) is composed entirely of human cells, is optically compatible for real-time fluorescent image analysis and is arrayed for high throughput experiments.21 Drugs can be tested within days on limited numbers of cells expanded to form the VMTs, providing high sensitivity and rapid turnaround of results.
CRC represents a major source of morbidity and mortality as the second leading cause of cancer-related deaths in the US and the third most common cancer worldwide, with over a million diagnoses and half a million deaths each year. The majority (75%) of patients present with localized disease (stage I and II) and undergo intentionally curative surgery. However, half of these patients will develop, and ultimately die from advanced disease with a 5 year survival rate for stage III and IV disease at only 10%, highlighting the need for more effective therapies. Achieving this will require more accurately modeling tumor biology in vitro. Here we show that when CRC cell gene expression, heterogeneity, growth and response to standard chemotherapy are examined, cells grown in the VMT more closely resemble those grown as xenografts than do cells grown either as monolayers (2D) or as spheroids (3D). Our studies reveal that the VMT recapitulates key features known to drive CRC disease progression and therapeutic failure, including contributions from the microenvironment, while also capturing unique cellular populations and expression signatures not observable in more simple in vitro models. Specifically, we show how changes in gene expression within the tumor and stroma may underpin drug sensitivities. Our findings support the VMT model as a powerful tool for drug development and disease modeling in oncology.
Results
The VMT serves as a platform for improved modeling and direct visualization of the complex tumor microenvironment
We have previously shown the VMT model to be a robust system for disease modeling and drug screening studies.18,19,21 Multiple tissue units are incorporated within a single microfluidic device that is fitted onto a bottom-less 96-well plate to allow each VMT to be independently treated (Fig. 1A). In response to physiologic flow driven by a hydrostatic pressure gradient across the tissue, endothelial cells (EC), fibroblasts and cancer cells introduced into each tissue unit self-organize within an extracellular matrix to form a complex tumor micro-ecosystem by day 5 of VMT culture (Fig. 1B). Importantly, tumor growth is entirely dependent on delivery of nutrients through the living vascular network. COMSOL Multiphysics simulation on a fully formed, anastomosed and perfused vascular network shows that the surface velocity of medium flowing through the vessels varies across the tissue, with some areas experiencing higher flow than others, mimicking blood flow through a capillary network in vivo (Fig. 1C).
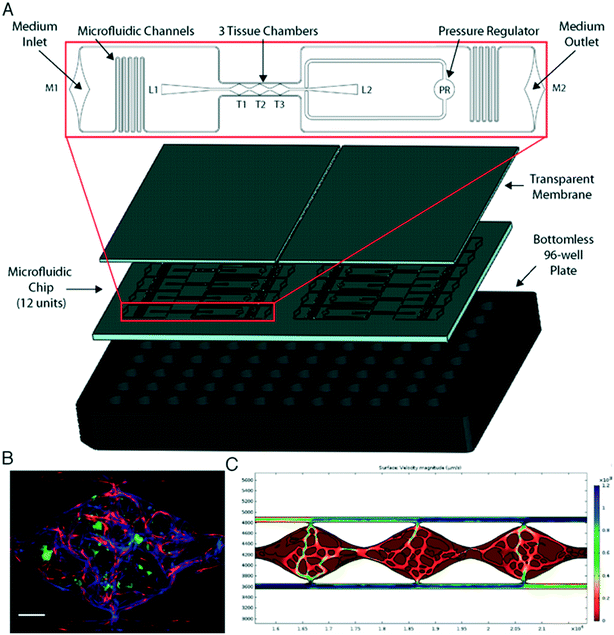 |
| Fig. 1 Vascularized micro-tumors (VMTs) are supported by gravity-driven flow through living human microvessels within a microfluidic platform arrayed for high throughput experiments. (A) The microfluidic chip is bonded to a bottom-less 96-well plate via chemical glue and oxygen plasma. Zoomed view shows a single device unit with 3 tissue chambers (T1-3) fed through microfluidic channels, 2 loading ports (L1-2), medium inlet and outlet (M1-2), and a pressure regulator (PR) to prevent gel bursting during loading. (B) Fluorescent image shows a vascularized micro-tumor with EC (mCherry, red) forming the vascular network, fibroblasts (Az, blue) supporting the tissue, and HCT116 cancer cells (GFP, green). Tissue chamber is ∼2 × 1 × 0.1 mm. Scale bar is 200 μm. (C) COMSOL simulation on a perfused vascular network shows the 2D surface velocity of medium flowing through the vessels. | |
Immunofluorescence staining reveals stark differences in collagen density and organization between the vascularized micro-organ (VMO), a normal tissue construct, and VMT. Collagen III in particular is highly expressed in the VMT, especially near tumor cells, and forms fiber ‘tracks’ throughout the tissue. Expression is more diffuse in the VMO (Fig. S1A–C†). Intriguingly, live imaging studies have shown that cancer cells migrate rapidly on collagen fiber tracks in areas enriched in collagen.22 To capture the full sequence of events that comprise the coordinated self-organization of the VMT as it progresses from single cancer cells embedded in a stromal tissue niche to a fully-formed vascularized tumor mass, we performed a 10 day time-lapse study (Videos S1 and S2†). We observed a remarkable series of events: HT29 colorectal cancer cells divide slowly while the vasculature forms, but once formed and flow is established the microtumors grow rapidly. Remarkably, some of these microtumors undergo a rapid and explosive disaggregation into single cells that migrate radially from the origin, suggesting a coordinated EMT event (Fig. S1D†). Taken together, the defining features of the VMT are changes in ECM composition associated with in vivo tumor progression, and the presence of an abnormal vasculature that closely models known characteristics of tumor-associated vasculature in vivo (see below).
Vessels in the VMT are irregular and leaky, hallmarks of in vivo tumors
Distinct changes in tumor-associated vascular architecture and function are known to occur during tumor progression. Although tumors recruit blood vessels to support their growth, the resulting vasculature is irregular, leaky, and ill-perfused, likely as a result of disrupted microenvironmental cues such as growth factor availability and matrix composition.23–25 Comparisons between vessels in the absence of tumor, in the vascularized micro-organ (VMO), and the VMT show that the vessels in the VMT recapitulate key features of in vivo tumor-associated vasculature, with vessel irregularity and compression becoming more pronounced at later time points, as the tumors grow larger (Fig. 2A and B). Arrowheads show areas of thin, non-perfused vessels (Videos S3 and S4†). These changes are manifest as increases in the number of blind-ended vessels and an overall decrease in vessel length and diameter, and number of junctions (Fig. 2C–F). In line with findings in vivo, tumor vessels in the VMT also show increased leakiness (Fig. 2G and H, Video S5†). Furthermore, as a result of structural heterogeneity in tumor-associated vasculature, the VMT shows differential patterns of perfusion, with highly leaky areas found adjacent to ill- or non-perfused areas (Videos S6–S9†). Overall, the vasculature within the VMT is significantly more leaky than the VMO, indicating that the vessels are affected by the presence of the tumor, as they are in vivo.
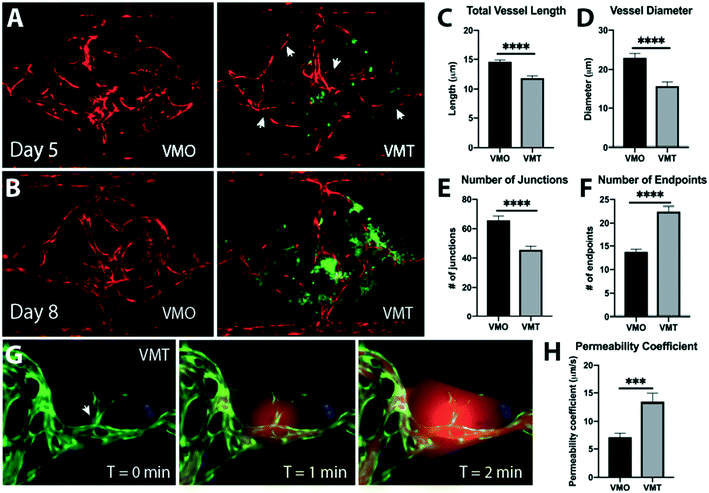 |
| Fig. 2 The VMT models key features of in vivo tumor vasculature. (A) Comparison of VMO and VMT on day 5 and (B) day 8 shows irregular vasculature in the VMT that becomes more prominent as the tumor grows. Arrowheads show skinny, non-perfused vessels as areas of vascular disruption in the VMT. EC (mCherry, red), HCT116 cancer cells (GFP, green). (C) Quantification of total vessel length, (D) vessel diameter, (E) number of junctions and (F) number of endpoints, all are mean and SD, n = 12. Statistical significance was calculated by unpaired t-test. (G) Fluorescent image at T = 0 min shows angiogenic sprouts near an HCT116 tumor cluster (arrowhead). EC (GFP, green), HCT116 cancer cells (azurite, blue). Perfusion with 70 kD rhodamine dextran at T = 1 min and T = 2 min reveals a significant leak from the tumor-associated vessel. (H) Quantification of the permeability coefficient shows that the VMT is twice as leaky as the VMO. Mean and SD, n = 12. Statistical significance was calculated by unpaired t-test. ***p < 0.0001, ***p < 0.00001. | |
Tumor cells grown in the VMT closely resemble xenograft tumors as revealed by transcriptomic profiling
To test our hypothesis that the VMT more closely models in vivo tumors than monolayer cultures, we carried out transcriptomic analysis of 770 cancer-related genes, from 13 cancer-associated pathways (Nanostring, Inc), on HCT116 colorectal cancer (CRC) cells that had been grown either in the VMT, as xenograft tumors, or in 2D monocultures. HCT116 cells expressing mCherry were isolated from all experimental groups (VMT, monolayer and xenograft) by fluorescence activated cell sorting (FACS). Remarkably, we found that the gene expression patterns of tumor cells derived from the VMT closely matched in vivo tumor-derived cells (difference not significant), whereas HCT116 cells grown in monolayer did not (difference significant at p < 0.0001, Fig. 3A). Interestingly, the variation between samples was highest for monolayer cultures (12.8%) and second highest for xenograft tumors (6.6%), with the VMT yielding the least variability between replicates (3.5%), highlighting the robustness and reproducibility of the VMT model. Pathways consistently enriched in the VMT and xenograft-derived CRC cells, but not in 2D monocultures, included PI3K–Akt signaling, MAPK signaling, Ras signaling, FGF signaling, chromosome and micro satellite instability, and epithelial-to-mesenchymal transition (Fig. 3B). Interestingly, genes that were consistently differentially expressed in the VMT compared to xenograft were enriched for beta-1 integrin signaling (COL1A1, COL3A1, FN1) and urokinase-type plasminogen activator receptor-mediated signaling (PDGFRB, HGF, MMP3), indicating that cell–matrix interactions may differ in subtle ways between the VMT and xenograft, possibly due to species cross-compatibility issues in the xenograft.
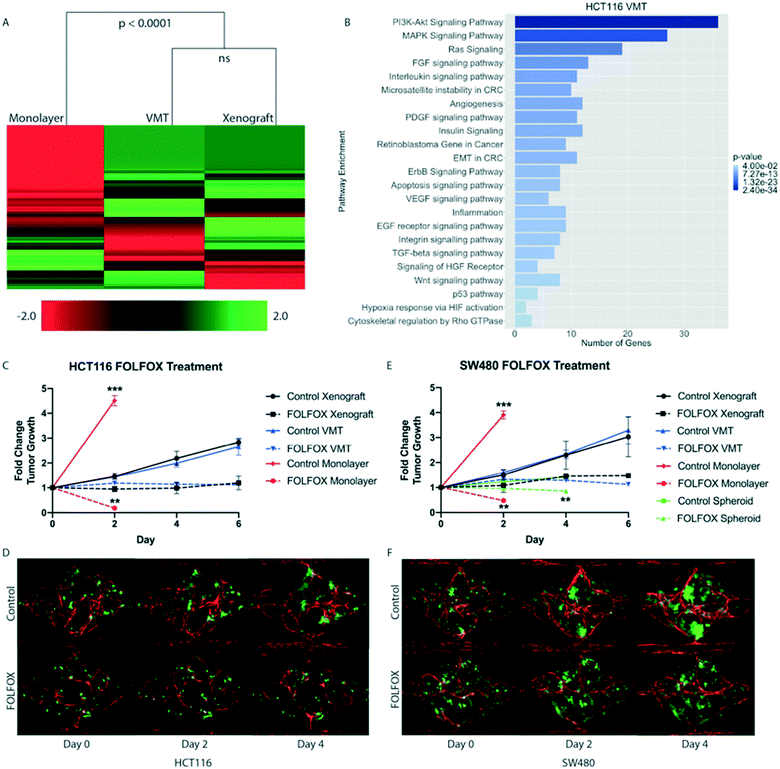 |
| Fig. 3 Comparison of gene expression, tumor growth and response to drug treatment between VMT, 2D and 3D monocultures and xenograft tumors. (A) Nanostring cancer panel of 770 cancer-related genes in HCT116 CRC cell line-derived monolayers, VMT and xenograft tumors. (B) Pathways enriched with differentially expressed genes comparing VMT and xenograft tumor. (C) Growth curves of HCT116 cells grown in monolayer, in mice or in the VMT and treated with standard of care, FOLFOX. Mean and SD shown, n = 16. Statistical significance was calculated by unpaired t-test. See Methods for a description of how tumor size was calculated in each geometry. (D) Representative images of HCT116-VMT (vessels red, tumor cells green). (E) Growth curves of SW480 cells in monolayer, spheroids, VMT and mice treated with FOLFOX. Mean and SD shown, n = 16. Statistical significance was calculated by unpaired t-test. (F) Representative images of SW480-VMT. **p < 0.001, ***p < 0.0001. | |
The VMT recapitulates xenograft tumor growth rates and response to standard-of-care chemotherapy
Based on the significant overlap in gene expression between HCT116 cells grown in the VMT platform or as xenografts, we next compared tumor cells grown in monolayer cultures, as VMTs and as xenograft tumors for growth rates and chemotherapeutic response. To capture the molecular landscape of CRC, we tested two established CRC cell lines originating from tumors of different subtypes: HCT116, which is a primary-derived, MSI-high (microsatellite instable-high), KRAS-mutant, PI3K mutant CRC line, representing about 15% of clinical CRC cases; as well as SW480, a primary-derived, MSS (microsatellite stable), KRAS mutant, APC-mutant line representing the majority (85%) of clinical CRC cases. First-line treatment for advanced metastatic CRC is systemic cytotoxic chemotherapy using FOLFOX (a regimen of 5 fluorouracil (5FU), leucovorin and oxaliplatin).26 Therefore, we treated each system with pharmaceutical-grade FOLFOX at a dose, duration and frequency that approximated the clinical administration, plasma Cmax and clearance for each drug (see Methods). Drugs were added directly to monolayer cultures, delivered through the vasculature in VMTs, and injected I.P. in mice. Tumor cell growth was assayed in each geometry as described in Methods.
Both HCT116 and SW480 grew rapidly in the 2D culture (and outgrew the well in a couple of days), and were also extremely sensitive to drug treatment. In sharp contrast, the growth of both HCT116 and SW480 was much slower in the VMT format, and drugs retarded tumor growth but did not reverse it. Remarkably, these kinetics recapitulated the responses seen when the cells were grown as xenografts (Fig. 3C–F). Specifically, HCT116-derived xenograft and VMT tumors showed no statistically significant difference in growth over the 6 day experimental period (day 6 fold change from baseline: 2.84 ± 1.31 vs. 2.66 ± 0.35, respectively) (Fig. 3C and D). In contrast, monolayer cultures exceeded the maximum growth observed in VMT and xenograft tumors in only 48 hours, to reach a fold change of 4.51 ± 0.21. The 2D cultures were significantly different from both VMT and xenograft at the 2 day time point (p-value < 0.0001) (Fig. 3C). For FOLFOX treatment, we observed that xenograft tumors and VMT exhibited similar drug sensitivity (1.19 ± 0.27 and 1.12 ± 0.01 fold change, respectively) and thus similar effect size (approximately 58%) at 6 days post-treatment (Fig. 3C). Monolayer cultures, however, showed an approximate 96% reduction in tumor cell number at the 48 hour time point, which is significantly different from both xenograft and VMT responses (p-value < 0.001) (Fig. 3C).
We found similar results with SW480-derived xenograft and VMT tumors, with respective fold change 3.03 ± 0.79 and 3.29 ± 0.56 above baseline on day 6, and no significant difference in growth between VMT and xenograft tumor at any time point (Fig. 3E and F). FOLFOX-treated xenograft and VMT-derived tumors also showed similar drug sensitivity (Fig. 3E), while monolayer cultures showed significantly increased cell growth and drug sensitivity at the 48 hour time point compared to xenograft and VMT (Fig. 3E). We also studied SW480 spheroids, and intriguingly, these showed significantly reduced growth compared to xenograft tumors (fold changes at day 4: 1.41 ± 0.06 and 2.29 ± 0.53, respectively) while maintaining roughly the same effect size in response to FOLFOX treatment. However, the absolute response to FOLFOX was significantly different in the spheroid cultures compared to xenograft (0.85 ± 0.16 and 1.45 ± 0.02 fold change in tumor growth, respectively). Hence, for two different subtypes of CRC, we show that while 2D and 3D monocultures are poorly predictive of tumor growth and response to the chemotherapeutic regimen FOLFOX in vivo, the VMT accurately predicts both.
HCT116-derived VMTs retain in vivo tumor heterogeneity not seen in 2D monocultures
Based on stark differences in tumor growth and response to chemotherapy between 2D and 3D monocultures and the VMT, we next sought to explore the distinct gene expression changes that occur at the single-cell level when cells are co-cultured within the dynamic 3D tumor microenvironment of the VMT. Here we used single-cell mRNA sequencing (scRNAseq) to profile the transcriptomes of cells isolated from HCT116-containing VMT and matched HCT116 CRC cells, EC and fibroblasts growing in 2D monocultures (Fig. 4A). Unbiased clustering analysis using the Seurat pipeline27 revealed distinct shifts in gene expression between the cells grown in the VMT and those grown in monolayer (Fig. S2A and B†). In Fig. S2B,† tSNE plotting shows the degree to which subpopulations were unique to either VMT or monolayer culture. Guided by annotated lineage-specific markers in humans,28 along with prior knowledge, two populations were identified as EC (clusters labeled EC A and EC B) by differential expression of PECAM1 (CD31+), two populations were derived from fibroblasts (fibroblast A and B) based on high expression of MMP2 and ACTA2, two populations were enriched for EPCAM and labeled as tumor (A and B), and a third tumor cluster (tumor C) highly expressed KRT5, characteristic of cells undergoing epithelial-to-mesenchymal transition (EMT)29 (Fig. S2C and D†).
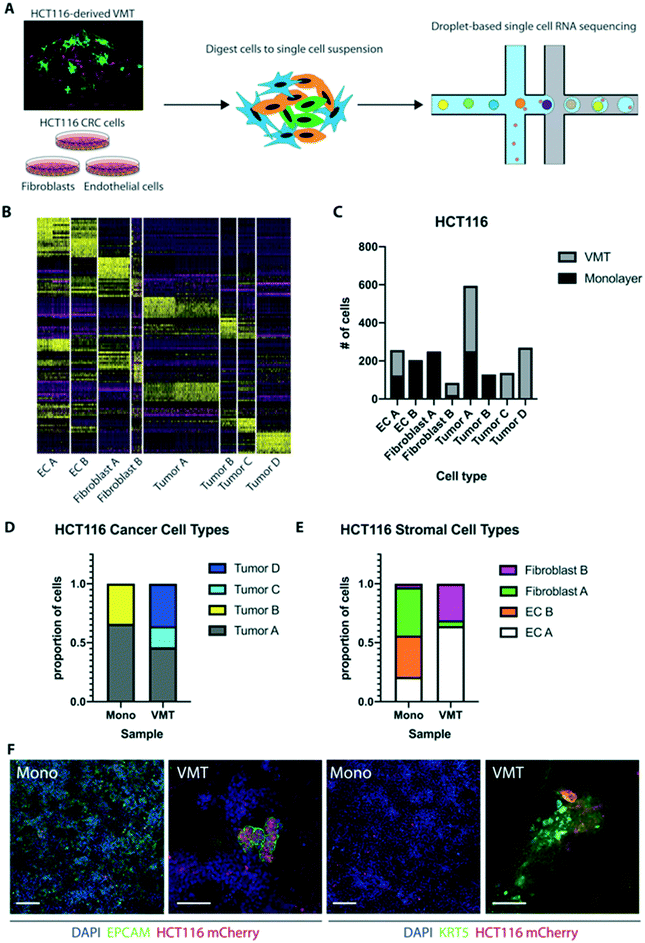 |
| Fig. 4 scRNAseq reveals emerging HCT116 tumor heterogeneity in the VMT platform. (A) Experimental design. HCT116 CRC cells, EC and fibroblasts were grown individually as 2D monocultures and then pooled or co-cultured within the VMT, harvested on day 7 of culture and processed for droplet-based single cell RNA sequencing as separate samples. (B) Heatmap of top 10 differentially-expressed genes shows that clustering resolution retains unique marker gene expression between cell types within the aggregated dataset. The number of clusters emerges from the resolution used in Seurat and was optimized to balance the number of clusters versus the number of unique differentially-expressed genes in each cluster. (C) Distribution of each cluster from the aggregated data between monolayer and VMT culture. (D) Proportion of tumor cell types found in the aggregated dataset that are in VMT and monolayer based on scRNAseq clustering. Tumor C and tumor D populations are found exclusively in the VMT, whereas tumor B is found predominantly in monolayer. (E) Proportion of stromal cell types found in the aggregated dataset that are in VMT and monolayer. VMT is enriched for fibroblast B population and lacks EC B. (F) Immunofluorescent staining of EPCAM and KRT5 in monolayer and the VMT showed heterogeneous and higher expression of both EPCAM and KRT5 in the VMT. Scale bar is 50 μm. | |
The top 10 differentially expressed genes for each cluster were determined within the aggregated dataset (Table S1†). A heat map with the combined top 10 cluster-discriminative genes for HCT116 populations showed that clusters were distinct in their gene signatures (Fig. 4B). The distribution of cells from each sample based on cell type reveals distinct changes in the VMT compared to 2D monocultures (Fig. 4C). Importantly, we found greater tumor heterogeneity in the VMT compared to monolayer, with tumor A, C and D all being present in the VMT, whereas monolayer cultures were comprised predominantly of tumor type A and B (Fig. 4D). The stroma was also distinctly different in the VMT compared to monolayer, with EC B and fibroblast A populations being diagnostic of monolayer culture (Fig. 4E). Overall, cells derived from the VMT showed higher expression of cell-type specific markers than the same cells grown in 2D cultures (Fig. S2E and F†). Immunofluorescence (IF) staining of HCT116 in the VMT or growing in a monolayer confirmed the presence of tumor subpopulations in the VMT (Epcam+, Cytokeratin 5+), with only a subset of the tumors expressing EPCAM in the VMT, and a subset of cells expressing high levels of KRT5. In contrast, HCT116 cells growing in 2D showed ubiquitous but low expression of EPCAM and low levels of KRT5 in a subpopulation of cells (Fig. 4D).
Tumor D, the second cluster of cells unique to the VMT, lacked both EPCAM and KRT5 and showed differential expression of CSPG4 (NG2+, a pericyte marker), however, additional pericyte markers such as PDGFRβ and DES were absent (Fig. S3A†). Furthermore, expression of PAGE5 (a proposed tumor marker) and VGF (a neuroendocrine marker) were restricted to this population, hinting at a tumor origin, although other markers expressed in highly invasive and resistant CRC tumors, such as CHGA and SYP, were absent.30,31
Single nucleotide variation (SNV) profiling of raw reads (see Materials and methods) allowed us to identify the cells as HCT116 in origin, but with enriched copy number variation (CNV) on chromosomes 1, 11 and 19 compared to the other tumor populations. While tumor populations A and B had a similar CNV profile, tumor C clustered with tumor D, and both showed distinguishing patterns of CNV enrichment. This finding suggests a greater degree of tumor heterogeneity within the VMT, compared to monolayer, likely through selection of HCT116 CRC subclones (Fig. S3B†). Taken together, these analyses revealed a higher degree of heterogeneity within VMT-derived tumor subpopulations relative to monolayer cultures.
Lineage hierarchy reconstruction reveals a unique VMT-derived HCT116 tumor population with characteristics of invasive CRC
To further investigate the origins of the NG2+ tumor subpopulation (tumor D) that we observed in the HCT116-derived VMT, we performed pseudotemporal reconstruction of differentiation trajectories using Monocle.32 To interrogate the hierarchical relationships between the tumor subpopulations, HCT116-derived VMT scRNAseq data were subset in Seurat to contain only tumor A–D subpopulations by removing EC and fibroblast subpopulations. Applying Monocle to this subsampled population yielded a connected differentiation trajectory that separated into three main branches representing distinct cellular states – with tumor A and tumor C occupying two cell states and the NG2+ tumor subpopulation D occupying the third state along pseudotime (Fig. 5A). Tumor B is largely absent from the VMT. Repeating this process with fibroblast subpopulations present in the subsampled dataset yielded a surprising finding: that fibroblasts segregate with tumor D to occupy the same state (Fig. S4†). We next determined a list of genes that may be responsible for the transition of HCT116 CRC epithelial cells to a de-differentiated, mesenchymal-like state within the VMT (Fig. 5B). We observed increases in EMT regulatory factors in the NG2+ tumor population, including TWIST1, VIM, TIMP1, and FN1, and decreased expression of differentiation and cellular adhesion markers, such as EPCAM, KRT8, KRT9 and KRT18 (Fig. 5B). KRT5 and KRT23 serve as transitional markers for the tumor C population midway between tumor A and tumor D/fibroblast (mesenchymal) populations, with low expression at the endpoints of pseudotime and high expression at the midpoint. While KRT5 is associated with metastasis,29 a recent study found that KRT23 activates CRC growth and migration, and that high KRT23 expression is prognostic of markedly shorter overall survival in CRC.33 These findings suggest that HCT116 cells are undergoing EMT within the VMT, with each subpopulation occupying a distinct state. Indeed, using time-lapse microscopy we observed collective EMT events within the VMT, with rapid and coordinated movement of whole tumor clusters occurring within the span of an hour (Fig. 5C, Video S10†). Interestingly, this is in contrast to the single-cell migratory phenotype we saw with HT29 cells reported above (Fig. S1D†).
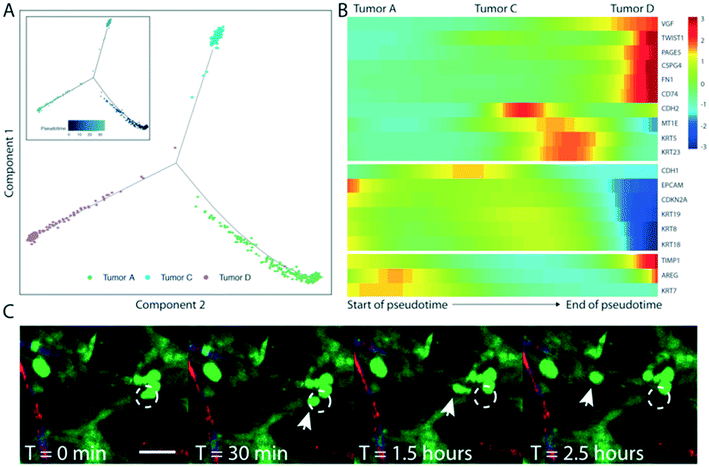 |
| Fig. 5 Lineage hierarchy reconstruction reveals differential tumor states within HCT116-derived VMT. (A) Monocle analyses for HCT116-derived VMT tumor subset showing monocle trajectory plot and pseudotime plot (inset). (B) Pseudotime heatmap for HCT116-derived VMT transition subset showing 3 differentially-expressed clusters across the pseudotime trajectory. (C) Time-lapse confocal imaging showing an EMT event within the HCT116-derived VMT, characterized by collective and coordinated migration of tumor cells. Arrow shows movement of a cluster of tumor cells. Scale bar is 50 μm. | |
SW480-derived VMTs retain in vivo tumor heterogeneity not seen in 2D or 3D monocultures
Given that SW480 CRC cells represent the major subtype of colorectal cancers (∼85%), we sought to validate the robustness of our system by performing additional scRNAseq on cells isolated from SW480-derived VMT, matched monolayer cultures, and tumor spheroids (Fig. 6A). There is a growing use of tumor spheroids to model in vivo tumors as they capture the 3D nature of tumors and better model the cell–cell interactions. Similar to our findings with HCT116 we observed distinct shifts in SW480 gene expression between the VMT and monolayer cultures, and this was also seen for the EC and fibroblast populations (Fig. S5A and B†). We also noted some overlap between VMT, monolayer and spheroid cultures for the SW480 tumor subpopulations. Populations were defined based on the same marker expression used for HCT116 (Fig. S5C†), with a tSNE plot showing the discrimination between clusters (Fig. S5D†). The top 10 differentially expressed genes for each cluster were determined within the aggregated dataset (Table S2†). A heat map with the combined top 10 cluster-discriminative genes for SW480 populations showed that clusters were distinct in their gene signatures (Fig. 6B). Similar to our findings with HCT116, two populations were identified as endothelial cells (EC A and EC B) by differential expression of PECAM1 (CD31+), three populations were derived from fibroblasts (fibroblast A, B and C) based on high expression of MMP2 and ACTA2, three populations were enriched for EPCAM and labeled as tumor (A, B and C), and a third tumor cluster highly expressed EPCAM and KRT5 (tumor D) (Fig. 6B).
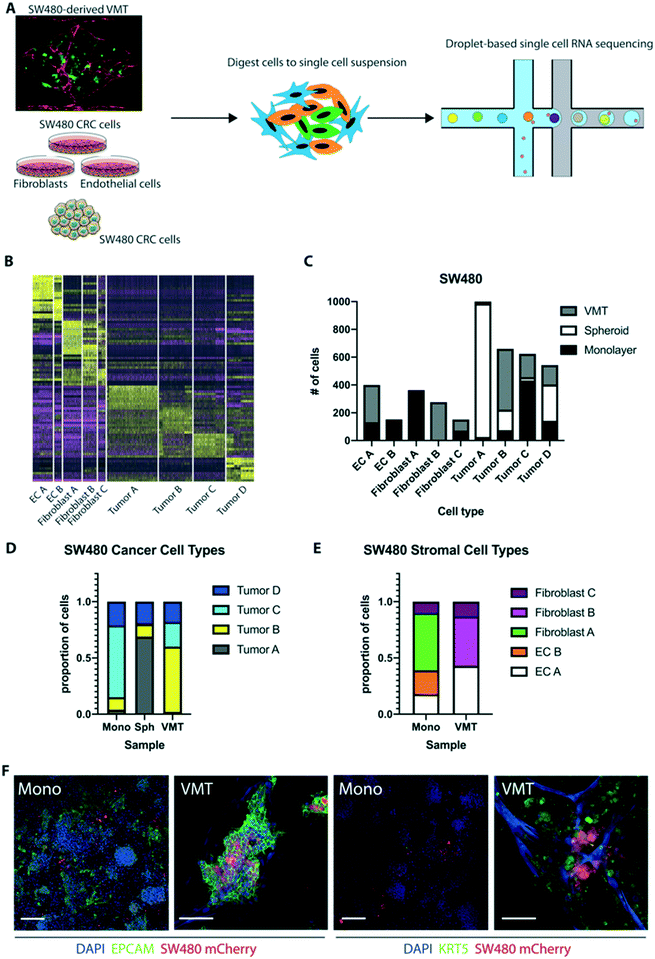 |
| Fig. 6 scRNAseq reveals emerging SW480 tumor heterogeneity in the VMT platform. (A) Experimental design. SW480 CRC cells were grown as 2D or 3D (spheroid) monocultures, EC and fibroblasts were grown as 2D monocultures and then pooled or all 3 cell types were co-cultured within the VMT, harvested on day 7 of culture and processed for droplet-based single cell RNA sequencing as separate samples. (B) Heatmap of top 10 differentially expressed genes with select cluster-discriminative genes noted. (C) Distribution of each cluster from the aggregated data between monolayer, spheroid and VMT. The number of clusters emerges from the resolution used in Seurat and was optimized to balance the number of clusters versus the number of unique differentially-expressed genes in each cluster. (D) Proportion of tumor cell types in VMT, monolayer and spheroid based on clustering. Monolayer consists predominantly of tumor C, spheroid of tumor A, and tumor B is found mostly in the VMT. (E) Proportion of stromal cell types in VMT and monolayer. VMT is enriched for fibroblast B population and lacks fibroblast A and EC B. (F) Immunofluorescent staining of EPCAM and KRT5 in monolayer and the VMT reveals higher expression in the VMT. Scale bar is 50 μm. | |
The distribution of each tumor type (A, B, C or D) between the different geometries was remarkably different, with tumor A dominating the spheroids, tumor B being enriched in VMT, tumor C dominating monolayer cultures, and tumor D being roughly evenly distributed between all geometries (Fig. 6C and D). Similar skewed distributions were observed for the EC and fibroblast populations (Fig. 6E). EPCAM and KRT8 characterized tumor B in the VMT, while tumor A, in the spheroids, had high levels of GAPDH and SOX4. Notably, the VMT had a more balanced distribution of tumor types than spheroids, which were dominated by tumor A. Interestingly, the NG2-enriched population seen in HCT116-derived VMT was absent from SW480. Similar to HCT116, however, we saw upregulation of EC PECAM1 in the VMT compared to monolayer cultures, with EC A in the VMT expressing more maturation markers (CLDN5, COL4A1) whereas EC B had a more angiogenic phenotype (ANGPT2, APLN). KRT5 expression in SW480 was enriched in the VMT compared to both 2D and 3D monocultures. EPCAM was not significantly enriched in a single group for the SW480 dataset (Fig. S5D–F†). IF staining confirmed the presence of tumor populations expressing EPCAM or EPCAM/KRT5 in SW480 cells grown in monolayer and in the VMT (Fig. 6F), with nearly ubiquitous expression of EPCAM in both systems, but higher expression of KRT5 in the VMT, confirming the scRNAseq data. We conclude that SW480 are more heterogeneous in the VMT geometry than they are in spheroids, which were dominated by a single type: tumor A.
Pathways implicated in tumor progression are upregulated in CRC cells grown in the VMT when compared to cells grown as 2D or 3D monocultures
Comprehensive gene set enrichment analyses, based on cell type and state-specific marker genes, revealed pathways that were significantly upregulated in cells grown in the VMT compared to 2D or 3D monocultures (Fig. 7A). Interestingly, the two tumor types show quite different patterns of gene expression, consistent with their different genotypes (SW480 is mutant for APC, KRAS and p53, while HCT116 is mutant for KRAS and PI3K but wild-type for p53 and APC). Both, however show a strong signal for glycolysis, ERBB1 and PDGF signaling, c-myc activation and integrin signaling. Within the VMT, tumor populations with high expression of Wnt signaling (HCT116 tumor A and SW480 tumor B), related target genes and modulators were strongly expressed (Fig. 7B), in agreement with in vivo findings that suggest a critical role for Wnt signaling heterogeneity in CRC cancer stem cell maintenance.34,35
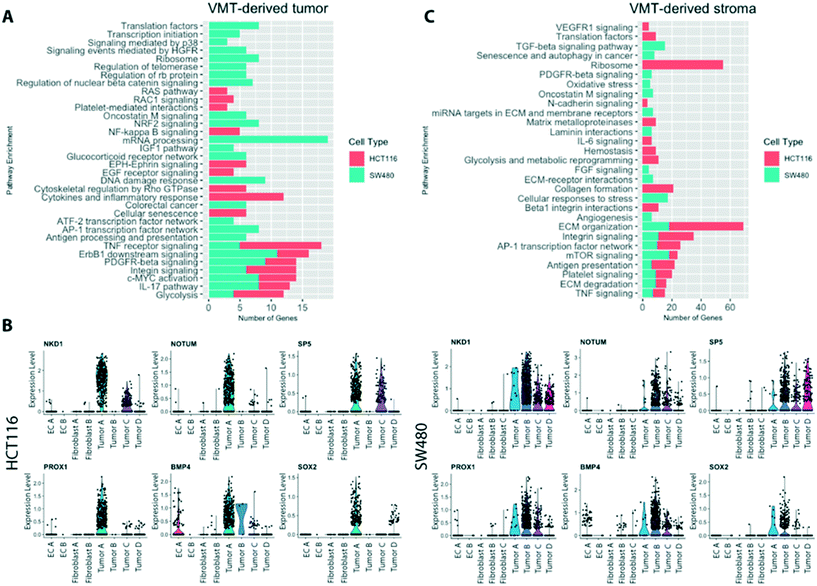 |
| Fig. 7 Pathway enrichment for VMT-derived cells. (A) VMT-derived tumor pathway enrichment for HCT116 and SW480 compared to monolayer cultures. (B) Violin plots for Wnt-related target genes and signaling modulators for HCT116 and SW480. Wnt high cells correlate with a stem-like signature. (C) Pathway enrichment for stromal populations in HCT116 and SW480 VMTs. All pathways shown were below a cut-off of p-value < 0.001 and combined score > 100. | |
When looking at the non-tumor cells in the VMT we noted a strong activation of stromal extracellular remodeling by HCT116 tumor cells (Fig. 7C), consistent with the rapid and robust angiogenic response we see in the presence of HCT116 (unpublished observations). For example, HCT116 VMT-derived fibroblasts displayed upregulated uPA and uPA receptor-mediated signaling (ITGB1, LRP1, ITGB5, ITGA3, FN1; adjusted p-value 0.01) and increased expression of MMPs (MMP14, BSG, TIMP2, TIMP1; adjusted p-value 0.02). Furthermore, we observed an increase in β1 integrin cell surface interactions (ITGB1, CD81, COL4A1, LAMB2, ITGA3, COL6A2, COL6A1, FN1, COL6A3, LAMB1, LAMC1; adjusted p-value 0.0000017). SW480-derived fibroblasts were enriched for PDGFR-beta signaling and FGF signaling. In addition, when looking at the top regulated genes in fibroblast B in the SW480 VMT we find several matrix proteins upregulated, including COL1A1, COL1A2, COL6A2 and IGFBP4, suggesting that these cells are responding to SW480-derived signals. EC within both HCT116- and SW480-VMT expressed high levels of genes associated with angiogenic sprouting and tip-cell formation, including ANGPT2, NID2 APLN, CYTL1 and DLL4, while expression of VWF, a marker of non-sprouting EC, was not differentially expressed between the VMT and monolayer cultures.
Compared to the SW480-containing VMT, SW480 spheroid cultures only showed upregulation of genes involved in HIF1α signaling and glycolysis, suggesting they are hypoxic; we found no other significantly enriched pathways, indicating that these 3D tumor spheroid models are limited in their ability to recapitulate features of in vivo tumors (data not shown). In summary, we find that tumor-associated pathways, including the oncogenic Wnt pathway, are more highly activated in the VMT than in monolayer or spheroid cultures. Taken together, these findings suggest that the VMT, but not spheroid or monolayer culture, recapitulates a pro-tumorigenic microenvironment that promotes CRC pathogenesis.
VMT-derived SW480 CRC cells better model in vivo tumor heterogeneity than 2D and 3D monocultures
To further validate the SW480-VMT as a physiologically relevant drug screening and disease modeling platform for mutant APC-driven CRC, we performed a reference-based integration in Seurat.36 We anchored SW480 CRC cells from our dataset to a reference scRNAseq dataset of xenograft-derived SW480 CRC cells to determine the degree of cell cluster mapping between each of the model systems (VMT, spheroid, and monolayer) and in vivo tumor. Cell anchoring removes technical variation between the separate scRNAseq experiments such that true biological variation can be detected above noise, and apart from batch effects. Of the three in vitro model systems, the VMT showed the greatest similarity to xenograft tumors, with 28% of tested cells accurately mapping to the reference dataset (Table 1). Monolayer and spheroid cultures did very poorly, with only 4% and 7% of cells, respectively, correctly mapping to the reference. Thus, even though these were not directly matched studies we still found a strong concordance between the VMT and in vivo tumors, confirming that the SW480-VMT is a superior model for capturing in vivo tumor complexity than are either monolayer or spheroid cultures.
Table 1 Results from reference based integration of SW480 scRNAseq datasets
Sample |
# True |
% True |
VMT |
188 |
28 |
Spheroid |
50 |
7 |
Monolayer |
24 |
4 |
Targeting the tumor microenvironment suppresses tumor growth in the VMT platform but not in 2D or 3D monocultures
Interactions between tumor cells and their local environment are increasingly being seen as potential anti-tumor targets. Intriguingly, we observed significant enrichment of TGF-β signaling in the fibroblasts derived from SW480-VMTs compared to those same fibroblasts grown in monolayers, while we saw no enhancement of TGF-β signaling in the SW480 cells themselves. Indeed, SW480 cells are reported to be mutant for SMAD4,37 a key canonical signaling component downstream of TGF-β receptors. Specifically, SW480-VMT derived fibroblast B displayed upregulation of TGFB1, TGFBR1, SMAD4, and SMAD2/3 and the TGF-β target SERPINE1, (Fig. 8A). In contrast, HCT116 VMT-derived fibroblasts and tumor populations both showed only modest increases in TGF-β signaling over 2D monocultures (not shown). Importantly, we observed these differences in cancer-associated fibroblasts between SW480- and HCT116 VMTs despite using the same line and passage of fibroblasts (derived from a single vial) in all experiments, suggesting that each tumor line uniquely models the microenvironment and stromal compartment of the VMT. Indeed, these observations are consistent both with our scRNAseq data, and with recent literature defining the role of TGF-β-activated stroma in poor prognosis CRC.38 Although most CRC display mutational inactivation of the TGF-β pathway, these tumors are paradoxically characterized by elevated TGF-β production. Functional studies show that cancer-associated fibroblasts support tumor-initiating cells via enhanced TGF-β signaling, and that poor clinical outcomes are predicted by a gene program induced by TGF-β in tumor-associated stromal cells.38
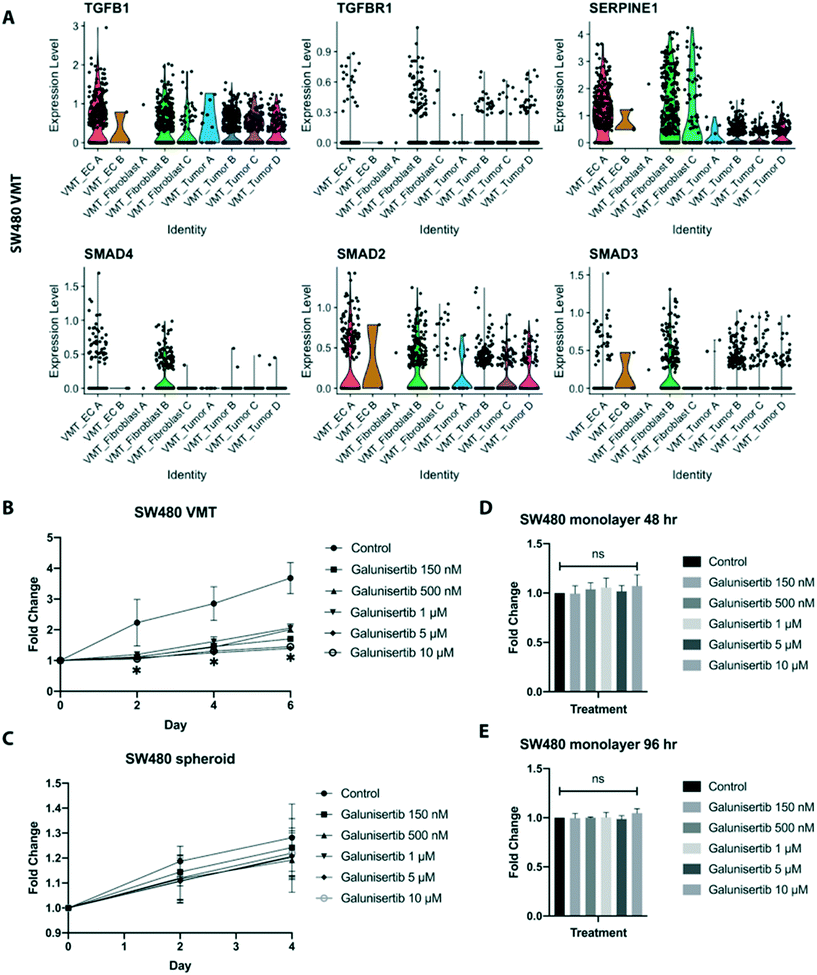 |
| Fig. 8 Targeting TGF-β signaling with galunisertib blocks tumor growth in the VMT but not in monolayer or spheroids. (A) Violin plots showing upregulation of genes involved in TGF-β signaling in the SW480-VMT derived fibroblast B population. (B) The TGF-βR1 inhibitor galunisertib dose-dependently inhibits SW480 growth in the VMT. Tumor size in both the VMT and the spheroid was normalized to 1 on day 0, the first day of drug treatment. Data are mean and SD from n = 16. Statistical significance was calculated by unpaired t-test. (C) Galunisertib has no effect on SW480 spheroid growth. (D) XTT assay for SW480 cells grown in monolayer shows no significant difference between control and galunisertib-treated conditions at 48 hours. (E) As for (D) but at 96 hours (48 hours post-treatment). | |
Based on these findings we next tested whether targeting TGF-β signaling in the reactive stroma could suppress tumor growth in the VMT. Using a physiologically-relevant range of doses, we treated cells in the VMT platform with the TGF-βR1 antagonist galunisertib, which recently completed phase II clinical trials for hepatocellular cancer. We found that while galunisertib had no effect on HCT116 grown in the VMT over the course of 6 days (Fig. S8A†), it potently inhibited SW480 growth in the VMT (Fig. 8B). Importantly, galunisertib had no effects on SW480-derived spheroid growth in response to treatment (Fig. 8C), and the drug also had no effect on SW480, HCT116, or fibroblasts growing as 2D monolayers at any of the concentrations tested at 48 hours or 96 hours (Fig. 8D, E and S8B†). SW480 VMT cell–cell interaction plots revealed that EC A, fibroblast B and fibroblast C populations were the major receivers of TGF-β signals from all populations and were specifically enriched for TGFBR1 (Fig. S8C and D†). Further examination of cancer-associated fibroblast (CAF)-associated genes revealed upregulation of PDGFRA/B, FAP, ACTA2, POSTN, and SPARC in the fibroblast B population (Fig. S8E†). Pathway analysis revealed that fibroblast B has high expression of genes enriched in the following pathways: TGF-β regulation of extracellular matrix, IL-1 regulation of extracellular matrix, and collagen biosynthesis (Fig. S8F†). These findings suggest that SW480 cells, but not HCT116, become dependent on TGF-β signaling in the presence of TGF-β activated stroma, and that CAFs are powerful regulators of tumor growth within the tumor microenvironment that can be therapeutically targeted.
Discussion
Anti-cancer drug development is a costly, time-consuming and inefficient process with a low success rate – only about 5% of drugs in the pipeline will ultimately gain FDA-approval. Recapitulating the complex intercellular crosstalk between the tumor and microenvironment is critical for increasing our understanding of clinical tumor pathology and laying the groundwork for novel therapeutic development. To this end, we demonstrate that the VMT more closely models the tumor growth and chemotherapeutic (FOLFOX) responses observed in preclinical in vivo murine xenograft models than 2D and 3D monocultures, with the added benefit of an entirely human cell system. In addition, we show that the VMT captures tumor cell heterogeneity, vascular disruption and tumor-microenvironment interactions.
In the same way that malignancy progresses via aberrant signaling in the microenvironment, targeting key intercellular signaling pathways between cancer cells and stroma can abrogate malignant progression. High levels of TGF-β are associated with poor outcomes in CRC and a key hallmark of microsatellite-stable CRC (for which SW480 cells are a model) is TGF-β activated stroma. Furthermore, TGF-β can act as a tumor suppressor or tumor promoter depending on the context.39,40 In VMT-derived stromal populations expressing CAF-associated markers, we observed significant upregulation of TGF-β signaling that, when pharmacologically abrogated with galunisertib, resulted in suppressed SW480 tumor growth within the VMT. Notably, the drug had no effect on these cells in 2D or 3D (spheroid) monocultures, underscoring the critical role stromal cells play in disease progression and drug sensitivity. The VMT also captures the in vivo growth characteristics of tumors and response to standard chemotherapy that monolayer and spheroid cultures cannot. Both SW480 and HCT116 cells grow in the VMT at the same rate as they do in vivo, and in each case tumors show cessation of growth but not regression in response to FOLFOX. In sharp contrast, cell growth is rapid in monolayer and cell death is readily apparent after drug treatment. These findings highlight the unique ability of the VMT to recapitulate human-specific and clinically relevant tumor pathologies in vitro that cannot be readily reproduced with standard cell culture or animal models.
It is becoming increasingly clear that understanding cellular heterogeneity in tumors will be key to developing effective therapies. Our scRNAseq studies reveal that the VMT captures this heterogeneity to a much higher degree than do either monolayer or spheroid cultures, and that only tumor cells in the VMT, and not those in monolayer or spheroids, map strongly to tumor cells in vivo. Notably, the NG2+ tumor D population we see in HCT116 VMTs appears to arise through EMT, a phenomenon observed in vivo and within the VMT, but not in 2D or 3D monocultures. Thus, the VMT could be used to facilitate the study and targeting of resistance mechanisms related to this unique population, whereas this would not be possible with 2D or spheroid cultures, and would be difficult and possibly not human-relevant in mice.
Taken together, our findings demonstrate that the VMT not only recapitulates in vivo drug response in terms of tumor growth, but also reconstitutes the cellular diversity of the in vivo tumor. As such, we believe the VMT will prove a useful tool for drug discovery and drug validation, and may find utility in precision medicine settings.
Methods
Cell culture
Human endothelial colony-forming cell-derived endothelial cells (ECFC-EC) are isolated from cord blood with IRB approval according to an established protocol.41 After selection for the CD31+ cell population, ECFC-EC are expanded on gelatin-coated flasks and cultured in EGM2 medium (Lonza). ECFC-EC are used between passages 4–8. Normal human lung fibroblasts (NHLF) are purchased from Lonza and used between passages 6–10. HCT116 colorectal cancer cells were donated from the UC Irvine Chao Family Comprehensive Cancer Center and SW480 colorectal cancer cells were clonally derived and provided by Dr. Marian Waterman. The ECFC-EC and cancer cells were transduced with lentivirus expressing mCherry (LeGO-C2, plasmid # 27339), green fluorescent protein (GFP) (LeGO-V2, plasmid # 27340), or azurite (pLV-azurite, plasmid # 36086) (Addgene, Cambridge, Massachusetts).42,43 Cancer cells and fibroblasts are cultured in DMEM (Corning) containing 10% FBS (Gemini Bio). All cells are cultured at 37 °C, 20% O2, and 5% CO2.
Microfluidic device fabrication and loading
Device fabrication and loading has been described previously.18,21 Briefly, a customized polyurethane master mold is fabricated using 2-part polyurethane liquid plastic (Smooth Cast 310, Smooth-On Inc.). A PDMS layer is then replicated from the master mold, holes are punched for inlets and outlets, and the platform is assembled in two steps. The PDMS layer is first attached to the bottom of a 96-well plate by chemical gluing and oxygen plasma treatment for 2 minutes. A 150 μm thin transparent membrane is then bonded to the bottom of the PDMS device layer by treating with oxygen plasma for an additional 2 minutes. The fully assembled platform is placed in 60 °C oven overnight, covered with a standard 96-well plate polystyrene lid, and sterilized using UV light for 30 minutes prior to loading cells. To load cells, NHLFs and ECFC-ECs are harvested and resuspended in fibrinogen solution at a concentration of 7 × 106 cells per mL and cancer cells (to establish VMT) at a concentration of 2 × 105 cells per mL. Fibrinogen solution is prepared by dissolving 70% clottable bovine fibrinogen (Sigma-Aldrich) in EBM2 basal media (Lonza) to a final concentration of 5 mg mL−1. The final cell-matrix suspension is mixed with thrombin (50 U mL−1, Sigma-Aldrich) for a final concentration of 3 U mL−1, quickly seeded into the microtissue chambers, and allowed to polymerize in a 37 °C incubator for 15 minutes. Laminin (1 mg mL−1, LifeTechnologies) is then introduced into the microfluidic channels through medium inlets and incubated at 37 °C for an additional 15 minutes. After incubation, culture medium (EGM-2, Lonza) is placed into the microfluidic channels and medium wells. Medium is changed every other day and leveled every day to maintain interstitial flow.
Generation of tumor spheroids
SW480 CRC cells were resuspended into 8 mg mL−1 solution of 70% fibrinogen at a concentration of 1 × 105 cells per mL and 50 μL of the cell solution was seeded into a 96-well plate containing 5 U of thrombin. Gels were allowed to set at 37 °C for 15 minutes before addition of 100 μL of EGM2 medium to each well. Medium was changed every other day and drug treatment started on day 5. Spheroid growth was measured by change in fluorescence intensity in the channel representing the tumor cells.
Drug treatment
Medium was changed every other day and hydrostatic pressure restored every day in the high throughput platform. After culturing for 5 days to allow full development of each VMT, culture medium was replaced by medium containing the drugs at the desired concentration, and delivered through the microfluidic channels using the hydrostatic pressure gradient. To mimic pharmacokinetics in the three in vitro systems (2D monoculture, 3D monoculture, and VMT), drugs were diluted into medium at maximal human plasma concentration on the first day of treatment and AUC were modeled according to average plasma clearance curves and drug half-life. Pharmaceutical grade 5-fluorouracil, leucovorin and oxaliplatin (FOLFOX) were purchased from UCI Medical Center pharmacy. Galunisertib (TGF-βR1 inhibitor) was purchased from SelleckChem. Each in vitro model system was exposed to compounds for the desired duration based on parameters defined by clinical administration, and the effect on tumor growth was quantified every 48 hours post-treatment. Cell viability in response to drugs in 2D monolayer cultures was quantified using an XTT assay according to the manufacturer's protocol (Sigma-Aldrich). Briefly, 5000 or 10
000 cells (HCT116, SW480 or NHLF) were seeded in triplicate in a 96-well plate and allowed to grow for 24 hours prior to treatment with drugs. XTT assays were performed after 48 hours of drug exposure or at the 96 hour time point after media changed at 48 hours. Cell viability was normalized to control wells without drug treatment.
Immunofluorescence staining
VMTs were fixed for immunofluorescence staining by perfusing 4% paraformaldehyde (PFA) through the medium inlet for 30 minutes at room temperature. After fixing, VMTs were washed with 1× DPBS overnight at 4 °C. Next, the high throughput plate was inverted and the bottom polymer membrane was carefully removed from the device. Each VMT unit was cut from the platform with a razor blade and placed in a 24-well culture plate, washed with 1× DPBS and then permeabilized for 15 minutes with 0.5% Triton-X100 diluted in DPBS. After permeabilization, VMT were blocked with 10% goat serum for 1 hour at room temperature and then incubated with primary antibody (diluted in 3% goat serum in 1× DPBS) overnight at 4 °C. The following primary antibodies were used in experiments: anti-collagen III (Abcam, ab6310), anti-EpCAM (Abcam, ab71916), and anti-cytokeratin 5 (Abcam, ab53121). After washing with 1× DPBS, VMT were incubated with goat anti-rabbit or goat anti-mouse secondary antibody (1
:
2000 dilution in 5% serum) for 1 hour at room temperature before washing with DPBS and counter-staining with DAPI. Finally, anti-fade solution was added on top of each VMT before mounting with a glass coverslip. Established cell lines were seeded into multi-well cover glass chambers and processed by the same protocol.
Fluorescence imaging and analyses
Fluorescence images were acquired with an Olympus IX70 inverted microscope using SPOT software (SPOT Imaging, Sterling Heights, Michigan). Confocal time-lapse series acquisition and imaging of fluorescent immunostaining was performed on a Leica TCS SP8 confocal microscope using a standard 10× air objective or 20× multi-immersion objective with digital zoom setting. AngioTool software (National Cancer Institute) was used to quantify vessel area, vessel length, number of vascular junctions and endpoints in the VMT. ImageJ software (National Institutes of Health) was utilized to measure vessel diameter and measure the total fluorescence intensity (i.e. mean grey value) for each tumor image to quantify tumor growth. Each chamber was normalized to baseline. Vessels were perfused by adding 25 μg mL−1 FITC- or rhodamine-conjugated 70 kDa dextran to the medium inlet. Once the fluorescent dextran had reached the vascular network, time-lapse image sequences were acquired using a Nikon Ti-E Eclipse epifluorescence microscope with a 4× Plan Apochromat Lambda objective. Perfusion images were analyzed using ImageJ software by measuring change in fluorescence intensity within regions of the extracellular space. Permeability coefficient is calculated by using eqn (1): | 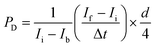 | (1) |
where Ii, If and Ib represent the initial, final and background average intensities, respectively, Δt is the time interval between two captured images and d is the average diameter of the vessel.44
Tumor growth in the VMTs was quantitated by measuring the total fluorescence intensity in the color channel representing the tumor cells. This takes into account both the area of the individual tumors and the depth, as thicker areas appear brighter. It should be noted that although the tumors are 3-dimensional they are quite thin in the z-direction (100–120 μm) and thus area much more closely approximates volume than would be the case for a sphere. Any adjustments made to images are performed on the entire image, with all images in that experimental group adjusted to the same settings.
Finite element simulation
COMSOL Multiphysics®5.2a was used to perform finite element simulations for the interstitial flow inside a developed microvascular network. Thresholded vessel images were smoothed and processed into outlines using ImageJ software, then converted into a .dxf file using Img2cad software. After the vessel outline was closed, and redundant fragments were removed using AutoCAD software, the complete vessel outline was scaled and integrated into the geometry of a microfluidic device. The refined CAD vessel diagram was then built into a 2D free and porous media flow model in COMSOL Multiphysics. Water was chosen to model the flow of culture media through the vascular network. The porosity and permeability of fibrin gel were estimated to be 0.99 and 1.5 × 10−13 m2, respectively, based on our published result.18 Inlet/outlet were designated at the media reservoir boundaries, with pressure specified as 98 and 0.001 Pa, respectively, based on calculated gravity-driven pressure difference in the device, as previously described.18
Cell sorting
The PDMS membrane was carefully removed from the bottom of the platform to expose the VMT tissue chambers. Chambers were washed with HBSS before adding TrypLE express enzyme drop wise to each VMT unit and incubated at 37 °C for 5 minutes to loosen the extra-cellular matrix from the device. Chambers were flushed with a pipette to collect cells into a conical tube and washed once with EGM2 medium to stop the digestion reaction. Next, cells were subjected to centrifugation (340×g for 3 min) and briefly resuspended in an HBSS/collagenase type III solution (1 mg mL−1) to fully digest the matrix. Finally, the single cell suspension was washed with EGM2 and put through a 70 μm filter. Cells were processed for sorting within an hour and kept on ice. Sorting was performed on the BD flow assisted cell sorting (FACS) AriaII with BD FACS Diva software version 8.0.1. Samples were sorted using a 100 μm nozzle at 20 psi with a gating strategy to select for fluorescently labeled (mCherry) cancer cells (Fig. S6†). Note that mCherry labeled CRC cells are pseudocolored green in all figures, while EC (vessels) are colored red.
Animal studies
All animal experiments were approved by the University of California, Irvine (UCI) Institutional Animal Care and Usage Committee (IACUC). For CRC xenograft treatment experiments, male NOD SCID gamma (NSG) mice (Jackson Laboratories) were injected subcutaneously into both flanks with a sterile culture of HCT116 or SW480 (5 × 105 cells) in 100 μL PBS (2 injections per mouse). Tumor volume and body weight were measured every other day using a caliper and scale, respectively. Tumor volume was calculated using eqn (2) with length being the longest measurement of the tumor. | 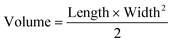 | (2) |
When the tumors reached a volume of 150 mm3, mice in the control group received two intraperitoneal (I.P.) injections of PBS. Mice in the FOLFOX group received one I.P. injection containing a combination of leucovorin (90 mg kg−1) and 5-fluorouracil (50 mg kg−1), followed by an injection of oxaliplatin (6 mg kg−1) two hours later. This treatment occurred weekly for up to 6 weeks or until the tumor reached a volume of 2 cm3. For NanoString experiments, xenograft tumors were established as described. When the tumors reached a volume of 2 cm3, mice were euthanized and tumors were harvested. The tumor tissue was minced into 1 mm pieces and digested in a solution of collagenase IV, hyaluronidase and DNAse I by shaking for 1–2 hours at room temperature. The single cell suspension was then processed and subjected to flow cytometry to select for mCherry labeled cancer cells as outlined above.
NanoString PanCancer human pathways assay
Each biological replicate was established in parallel from a single vial and passage of HCT116 cells. Gene expression analysis was performed using the NanoString PanCancer Human Pathways Assay. Per sample, 10
000 cells were lysed in diluted Buffer RLT (1
:
5 in RNAse-free water) at a concentration of 2000 cells per μL. Samples were submitted to the Genomics High Throughput Core Facility at UCI. Each cell lysate was mixed with a 3′ biotinylated capture probe and a 5′ reporter probe tagged with a fluorescent barcode from the custom gene expression code set. Probes and target transcripts were hybridized at 65 °C for 12–16 h. Hybridized samples were run on the NanoString nCounter preparation station using the recommended manufacturer protocol, in which excess capture and reporter probes were removed and transcript-specific ternary complexes were immobilized on a streptavidin-coated cartridge. The samples were scanned at maximum resolution on an nCounter Digital Analyzer. Data were processed and analyzed using NanoString nCounter nSolver software version 3.0 (NanoString Technologies, Seattle WA). For gene expression analysis, data were normalized using the geometric mean of housekeeping genes selected by the GeNorm algorithm. The raw count from NanoString was subjected to background subtraction and reference gene normalization.
Xenograft tumors for single-cell sequencing and cell anchoring
SW480 CRC cells were transduced with lentivirus carrying pCDH vector from System Biosciences: empty vector (Mock) or vector expressing dnLEF-1, followed by selection with 500 μg mL−1 G418. Transduced cells were collected as a pool for confirmation of expression, and Wnt signaling activity was measured by a SuperTOPFlash luciferase reporter.45 Next, 2.5 × 106 cells in PBS were injected subcutaneously into immunodeficient NSG mice. Tumors were removed after 3 weeks and dissociated for single cell sequencing. Only empty vector (Mock) samples were used for reference integration.
SW480 xenograft dissociation for single cell sequencing
Tumor digestion has been described previously.46 Single tumor cells were resuspended to 5 × 107 cell per mL and stained with CD298-APC (10 μL/106 cells)(BioLegend) and Sytox Green (ThermoFisher) for 20 minutes at 4 °C in the dark, prior to sorting on FACSAria II (BD) for Sytox-cells. Sorted cells were spun down and resuspended to 1 × 106 cells per mL.
Single-cell sequencing
Flow cytometry sorted cells were washed in PBS with 0.04% BSA and re-suspended at a concentration of ∼1000 cell per μL. Cellular suspensions were loaded onto a Chromium Single Cell Instrument (10X Genomics) to generate single-cell gel beads in emulsion (GEMs). GEMs were processed to generate cDNA libraries by using 10X Genomics v2 chemistry according to the Chromium Single Cell 3' Reagents kits v2 user guide: CG00052 Rev B. Quantification of cDNA libraries was performed using Qubit dsDNA HS Assay kit (Life Technologies Q32851), high-sensitivity DNA chips (Agilent 5067-4626) and KAPA qPCR (Kapa Biosystems KK4824). Libraries were sequenced on an Illumina HiSeq4000 to achieve an average of 50
000 reads per cell.
Transcriptome alignment and data processing
After demultiplexing sequencing libraries to individual library FASTQ files, each library was aligned to an indexed GRCh38 reference genome using Cell Ranger (10X Genomics) Count 2.2.0. Aligned libraries are then normalized based on median reads per cell using Cell Ranger Aggr 2.2.0. Raw gene expression matrices were loaded into R (version 3.6.0)47 and converted to a Seurat object using the Seurat R package (version 2.3.4).27 From this, the data were log-transformed and corrected for unwanted sources of variation using the ScaleData function in Seurat. Gene expression matrices were then normalized to total cellular read count and to mitochondrial read count using linear regression as implemented in Seurat's RegressOut function. For quality control filtering, we excluded cells with less than 200 or more than 5000 genes detected, and over 15% UMIs (unique molecular identifiers) derived from mitochondrial genome. In addition, genes that were not detected in at least 3 cells after this trimming were also removed from further analysis. Cell cycle gene regression did not significantly influence PCs or clustering (Fig. S7†) and therefore was not performed in the final analyses.
Single cell genotyping analyses
In order to identify cell origin, Vartrix48 combined with in-house scripts were used to extract single cell variant information from 10X Genomics single cell data for the two HCT116 samples, VMT and monolayer. Variant information for HCT116 cell line was obtained from the Broad Cancer Cell Line Encyclopedia49 containing 39
000 variants. The variant list was first filtered so that common variants in dbSNPs Build ID: 150 (ref. 50) were excluded. For the remaining 18K SNVs, Vartrix was used to extract genotype information at each locus from the BAM files of the two samples analyzed using cellRanger (10X Genomics). Further confirmation of these findings derived from additional barcoding based on Y-chromosome-linked genes since HCT116 CRC cells are derived from a male patient, and the fibroblast line used in the experiments were derived from a female patient (an EC origin for the cells was ruled out by a complete absence of any EC-specific markers). For each cell in the two samples, a genotype score was determined for all 18K SNVs by subtracting the expression of all X-linked genes to quantify the confidence that the cell is derived from HCT116. For copy number variation (CNV) analysis, we utilized a pipeline from the Broad Institute called inferCNV.51 We combined all normal cells (endothelial cells and fibroblasts) as a non-malignant reference population and queried the unknown and malignant populations against this reference set using the following parameters: cutoff = 0.1, cluster by groups = false, HMM = false, and de-noise = true.
Pathway analyses
Marker genes for each subgroup of cells were determined by Seurat following log-transformation. Based on cell type and state specific marker genes, comprehensive gene set enrichment was performed using Enrichr.52 A p-value of 0.05 was used as a cut-off to determine significant enrichment of a pathway or annotated gene grouping.
Cell anchoring
Cell anchoring was performed with Seurat version 3 using reference-based integration.35 For these analyses, ‘Xeno’ (SW480 xenograft tumor scRNAseq dataset) was used as the ‘reference’, and all other SW480 datasets (VMT, spheroid and monolayer) were used as ‘query’. Briefly, canonical correlation analysis was performed followed by L2-normalization of the canonical correlation vectors to project the datasets into a subspace defined by shared correlation structure across datasets. Next, mutual nearest neighbors were identified across reference and query cells and served as ‘anchors’ to guide dataset integration. For each anchor pair, a score was assigned based on the consistency of the anchors across the neighborhood structure of each dataset. Plots were generated using Bioconductor package ‘projectR’.53
Cell–cell signaling networks inference
Cell–cell communication networks were determined via SoptSC54 for TGF-beta signaling pathway. The probability between two cells is quantified by interactions between specific ligand–receptor pairs based on their gene expression levels. The motivation behind the method is that if a ligand is highly expressed in cell i, the cognate receptor is highly expressed in cell j, then there is a chance that communication occurred between these two cells, quantified by the signaling probability. Cluster-level interactions can be revealed by taking the average probability of the cell-level interactions. More details on the formula to compute the probability of the interaction between cells can be found in SoptSC.54 We applied SoptSC to infer cell–cell communication network for VMT. The cluster-level interactions for all ligand–receptor pairs are visualized through dot-heatmap where dot-size represents the number of interactions (log-normalized) and dot-color represents the interaction scores calculated. We also use directed-graph to visualize cluster-to-cluster interactions where nodes represent clusters and the weighted edges represent interactions between clusters. Arrows start from clusters that express ligand and points to clusters that express receptor. Edges are colored by the sender node. We set a threshold (=0.5) to cut off the edge that the interaction score is less than the threshold.
Statistical analyses
Data are represented as mean ± standard error of at least three independent experiments, unless noted otherwise. Comparison between experimental groups of equal variance were analyzed using an unpaired t-test and 95% confidence interval or one-way ANOVA followed by Dunnett's test for multiple comparisons. Statistical calculations were performed using GraphPad Prism 8.0. For Nanostring analyses, gene expression was averaged across each sample (4 replicates each) then the fold change of each sample (i.e. monolayer and VMT), using xenograft tumor as the reference group, was log2-transformed. The mean log fold change between monolayer and VMT, combining all genes vs. each individual gene, was then compared using 2-sample t-test in SAS. Significance cut-off for all analyses was p < 0.05.
Author contributions
S. J. H. performed the majority of the experiments and wrote most of the manuscript. S. M. performed the FOLFOX drug screening in the VMT and G. B. S. performed the FOLFOX and galunisertib drug screening in 2D and 3D monocultures. Q. H. N. performed scRNA-seq data preprocessing and helped with subsequent analyses and figure preparation. A. T. performed perfusion experiments comparing VMO and VMT. J. W. performed cell anchoring and cell genotyping analyses for the scRNA-seq datasets and wrote the corresponding methods. T. H. performed statistical analyses for the Nanostring dataset and wrote the corresponding methods. D. Z. performed COMSOL simulation, prepared the device figure and wrote the corresponding methods. S. W. performed cell–cell interaction analyses and wrote the corresponding methods. M. M. H. helped to establish xenograft tumors in mice. E. C. and S. G. performed drug screening in the VMT. G. T. C. performed the xenograft scRNA-seq experiment. R. T. D. performed the CNV analyses and K. N. and N. P. helped with scRNA-seq analyses. D. A. L. and K. K. provided general guidance. A. P. L., J. L., and M. L. W. provided guidance and scientific feedback. C. C. W. H. directed the research and assisted in writing and editing the manuscript.
Conflicts of interest
All authors except C. C. W. H. and A. P. L. declare no competing interests. C. C. W. H. and A. P. L. have equity interests in Aracari Biosciences, Inc, which is commercializing the microfluidic device used in this paper. The terms of this arrangement have been reviewed and approved by the University of California, Irvine in accordance with its conflict of interest policies.
Acknowledgements
We thank Damie Juat and Brittany Pham for help with cell culture, Hannah Bone for help with device fabrication, and Vanessa Scarfone for help with FACS experiments. S. J. H. is supported by an ARCS Award, Chancellor's Club Fellowship, and Edwards Lifesciences Cardiovascular Technology Fellowship. This study has been supported by U54 CA217378, R01 CA180122, and UH3 TR-000481. C. C. W. H. and M. L. W. receive support from the Chao Family Comprehensive Cancer Center (CFCCC) through an NCI Center Grant [P30A062203]. The UCI Genomics High Throughput Facility and the Biostatistics Core are also supported by the CFCCC through this award. We acknowledge bioRxiv for making a preprint of this manuscript available – doi: 10.1101/2020.03.03.973891v1.
References
- R. L. Siegel, K. D. Miller and A. Jemal, Cancer statistics, 2019, Ca-Cancer J. Clin., 2019, 69, 7–34 CrossRef.
- L. Hutchinson and R. Kirk, High drug attrition rates–where are we going wrong?, Nat. Rev. Clin. Oncol., 2011, 8, 189–190 CrossRef.
- E. S. Nakasone,
et al. Imaging Tumor-Stroma Interactions during Chemotherapy Reveals Contributions of the Microenvironment to Resistance, Cancer Cell, 2012, 21, 488–503 CrossRef CAS.
- L. G. Griffith and M. A. Swartz, Capturing complex 3D tissue physiology in vitro, Nat. Rev. Mol. Cell Biol., 2006, 7, 211–224 CrossRef CAS.
- A. H. Fong,
et al. Three-Dimensional Adult Cardiac Extracellular Matrix Promotes Maturation of Human Induced Pluripotent Stem Cell-Derived Cardiomyocytes, Tissue Eng., Part A, 2016, 22, 1016–1025 CrossRef CAS.
- A. L. Correia and M. J. Bissell, The tumor microenvironment is a dominant force in multidrug resistance, Drug Resist. Updates, 2012, 15, 39–49 CrossRef CAS.
- M. Romero-López,
et al. Recapitulating the human tumor microenvironment: Colon tumor derived extracellular matrix promotes angiogenesis and tumor cell growth, Biomaterials, 2017, 116, 118–129 CrossRef.
- P. Lu, V. M. Weaver and Z. Werb, The extracellular matrix: A dynamic niche in cancer progression, J. Cell Biol., 2012, 196, 395–406 CrossRef CAS.
- M. W. Pickup, J. K. Mouw and V. M. Weaver, The extracellular matrix modulates the hallmarks of cancer, EMBO Rep., 2014, 15, 1243–1253 CrossRef CAS.
- K. Kessenbrock, V. Plaks and Z. Werb, Matrix Metalloproteinases: Regulators of the Tumor Microenvironment, Cell, 2010, 141, 52–67 CrossRef CAS.
- Y. Fang and R. M. Eglen, Three-Dimensional Cell Cultures in Drug Discovery and Development, SLAS Discovery, 2017, 22, 456–472 CAS.
- T. Takebe, B. Zhang and M. Radisic, Synergistic Engineering: Organoids Meet Organs-ona-Chip, Cell Stem Cell, 2017, 21, 297–300 CrossRef CAS.
- S. Aparicio, M. Hidalgo and A. L. Kung, Examining the utility of patient-derived xenograft mouse models, Nat. Rev. Cancer, 2015, 15, 311–316 CrossRef CAS.
- S. Y. C. Choi,
et al. Lessons from patient-derived xenografts for better in vitro modeling of human cancer, Adv. Drug Delivery Rev., 2014, 79, 222–237 CrossRef.
- C. Heylman, A. Sobrino, V. S. Shirure, C. C. Hughes and S. C. George, A strategy for integrating essential three-dimensional microphysiological systems of human organs for realistic anticancer drug screening, Exp. Biol. Med., 2014, 239, 1240–1254 CrossRef.
- Y.-H. Hsu, M. L. Moya, C. C. W. Hughes, S. C. George and A. P. Lee, A microfluidic platform for generating large-scale nearly identical human microphysiological system arrays, Lab Chip, 2013, 13, 2990–2998 RSC.
- M. L. Moya, Y.-H. Hsu, A. P. Lee, C. C. Hughes and S. C. George, In Vitro Perfused Human Capillary Networks, Tissue Eng., Part C, 2013, 19, 730–737 CrossRef CAS.
- A. Sobrino,
et al. 3D microtumors in vitro supported by perfused vascular networks, Sci. Rep., 2016, 6, 31589 CrossRef CAS.
- S. J. Hachey and C. C. W. Hughes, Applications of tumor chip technology, Lab Chip, 2018, 18, 2893–2912 RSC.
- Y. H. Hsu,
et al. Full range physiological mass transport control in 3D tissue cultures, Lab Chip, 2013, 13, 81–89 RSC.
- D. T. T. Phan,
et al. A vascularized and perfused organ-on-a-chip platform for large-scale drug screening applications, Lab Chip, 2017, 17, 511–520 RSC.
- S. N. Kehlet,
et al. Excessive collagen turnover products are released during colorectal cancer progression and elevated in serum from metastatic colorectal cancer patients, Sci. Rep., 2016, 6, 30599 CrossRef CAS.
- R. K. Jain, Molecular regulation of vessel maturation, Nat. Med., 2003, 9, 685–693 CrossRef CAS.
- J. L. Yu,
et al. Heterogeneous Vascular Dependence of Tumor Cell Populations, Am. J. Pathol., 2001, 158, 1325–1334 CrossRef CAS.
- P. Carmeliet and R. K. Jain, Molecular mechanisms and clinical applications of angiogenesis, Nature, 2011, 473, 298–307 CrossRef CAS.
- J. Cassidy,
et al. Randomized Phase III Study of Capecitabine Plus Oxaliplatin Compared With Fluorouracil/Folinic Acid Plus Oxaliplatin As First-Line Therapy for Metastatic Colorectal Cancer, J. Clin. Oncol., 2008, 26, 2006–2012 CrossRef CAS.
- A. Butler, P. Hoffman, P. Smibert, E. Papalexi and R. Satija, Integrating single-cell transcriptomic data across different conditions, technologies, and species, Nat. Biotechnol., 2018, 36, 411–420 CrossRef CAS.
- X. Zhang,
et al. CellMarker: a manually curated resource of cell markers in human and mouse, Nucleic Acids Res., 2019, 47, D721–D728 CrossRef CAS.
- C. Ricciardelli,
et al. Keratin 5 overexpression is associated with serous ovarian cancer recurrence and chemotherapy resistance, Oncotarget, 2017, 8, 17819–17832 CrossRef.
- Y. Liu,
et al. Neuroendocrine differentiation is predictive of poor survival in patients with stage II colorectal cancer, Oncol. Lett., 2017, 13, 2230–2236 CrossRef CAS.
- P. Grabowski,
et al. Neuroendocrine differentiation is a relevant prognostic factor in stage III-IV colorectal cancer, Eur. J. Gastroenterol. Hepatol., 2001, 13, 405–411 CrossRef CAS.
- C. Trapnell,
et al. The dynamics and regulators of cell fate decisions are revealed by pseudotemporal ordering of single cells, Nat. Biotechnol., 2014, 32, 381–386 CrossRef CAS.
- N. Zhang,
et al. Keratin 23 promotes telomerase reverse transcriptase expression and human colorectal cancer growth, Cell Death Dis., 2017, 8, e2961 CrossRef CAS.
- T. Reya and H. Clevers, Wnt signalling in stem cells and cancer, Nature, 2005, 434, 843–850 CrossRef CAS.
- L. Vermeulen,
et al. Wnt activity defines colon cancer stem cells and is regulated by the microenvironment, Nat. Cell Biol., 2010, 12, 468–476 CrossRef CAS.
- T. Stuart,
et al. Comprehensive Integration of Single-Cell Data, Cell, 2019, 177, 1888–1902.e21 CrossRef CAS.
- K. L. Woodford-Richens,
et al. SMAD4 mutations in colorectal cancer probably occur before chromosomal instability, but after divergence of the microsatellite instability pathway, Proc. Natl. Acad. Sci. U. S. A., 2001, 98, 9719–9723 CrossRef CAS.
- M. B. Meads, R. A. Gatenby and W. S. Dalton, Environment-mediated drug resistance: a major contributor to minimal residual disease, Nat. Rev. Cancer, 2009, 9, 665–674 CrossRef CAS.
- A. Calon,
et al. Dependency of Colorectal Cancer on a TGF-β-Driven Program in Stromal Cells for Metastasis Initiation, Cancer Cell, 2012, 22, 571–584 CrossRef CAS.
- J. Massague, TGF-β in Cancer, Cell, 2008, 134, 215–230 CrossRef CAS.
- J. M. Melero-Martin, Z. A. Khan, A. Picard, X. Wu, S. Paruchuri and J. Bischoff, In vivo vasculogenic potential of human blood-derived endothelial progenitor cells, Blood, 2007, 109, 4761–4768 CrossRef CAS.
- K. Weber, U. Bartsch, C. Stocking and B. A. Fehse, Multicolor Panel of Novel Lentiviral Gene Ontology (LeGO) Vectors for Functional Gene Analysis, Mol. Ther., 2008, 16, 698–706 CrossRef CAS.
- M. A. Mena, T. P. Treynor, S. L. Mayo and P. S. Daugherty, Blue fluorescent proteins with enhanced brightness and photostability from a structurally targeted library, Nat. Biotechnol., 2006, 24, 1569–1571 CrossRef CAS.
- J. S. Jeon,
et al. Generation of 3D functional microvascular networks with human mesenchymal stem cells in microfluidic systems, Integr. Biol., 2014, 6, 555–563 CrossRef CAS.
- K. T. Pate,
et al. Wnt signaling directs a metabolic program of glycolysis and angiogenesis in colon cancer, EMBO J., 2014, 33, 1454–1473 CrossRef CAS.
- D. A. Lawson,
et al. Single-cell analysis reveals a stem-cell program in human metastatic breast cancer cells, Nature, 2015, 526, 131 CrossRef CAS.
-
RStudio Team, RStudio: Integrated Development Environment for R, RStudio, Inc., Boston, MA, 2016 Search PubMed.
-
10XGenomics, Vartrix single-cell genotyping tool Search PubMed.
- M. Ghandi,
et al. Next-generation characterization of the cancer cell line encyclopedia, Nature, 2019, 569, 503 CrossRef CAS.
- S. T. Sherry,
et al. DBSNP: The NCBI database of genetic variation, Nucleic Acids Res., 2001, 29, 308–311 CrossRef CAS.
-
T. Tickle, I. Tirosh, C. Georgescu, M. Brown and B. Haas, inferCNV of the Trinity CTAT Project, Klarman Cell Observatory, Broad Institute of MIT and Harvard, Cambridge, MA, USA, 2019 Search PubMed.
-
W. Jawaid, enrichR: Provides an R Interface to ‘Enrichr’, 2019 Search PubMed.
-
G. Sharma and G. Stein-O'Brien, projectR: Functions for the projection of weights from PCA, CoGAPS, NMF, correlation, and clustering, 2016 Search PubMed.
- S. Wang, M. Karikomi, A. L. MacLean and Q. Nie, Cell lineage and communication network inference via optimization for single-cell transcriptomics, Nucleic Acids Res., 2019, 47(11), e66 CrossRef CAS.
Footnote |
† Electronic supplementary information (ESI) available. See DOI: 10.1039/d0lc01216e |
|
This journal is © The Royal Society of Chemistry 2021 |
Click here to see how this site uses Cookies. View our privacy policy here.