DOI:
10.1039/D3RA06831E
(Review Article)
RSC Adv., 2023,
13, 31578-31594
Challenges in natural product-based drug discovery assisted with in silico-based methods
Received
7th October 2023
, Accepted 19th October 2023
First published on 30th October 2023
Abstract
The application of traditional medicine by humans for the treatment of ailments as well as improving the quality of life far outdates recorded history. To date, a significant percentage of humans, especially those living in developing/underprivileged communities still rely on traditional medicine for primary healthcare needs. In silico-based methods have been shown to play a pivotal role in modern pharmaceutical drug discovery processes. The application of these methods in identifying natural product (NP)-based hits has been successful. This is very much observed in many research set-ups that use rationally in silico-based methods in combination with experimental validation techniques. The combination has rendered the use of in silico-based approaches even more popular and successful in the investigation of NPs. However, identifying and proposing novel NP-based hits for experimental validation comes with several challenges such as the availability of compounds by suppliers, the huge task of separating pure compounds from complex mixtures, the quantity of samples available from the natural source to be tested, not to mention the potential ecological impact if the natural source is exhausted. Because most peer-reviewed publications are biased towards “positive results”, these challenges are generally not discussed in publications. In this review, we highlight and discuss these challenges. The idea is to give interested scientists in this field of research an idea of what they can come across or should be expecting as well as prompting them on how to avoid or fix these issues.
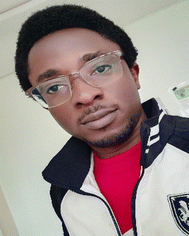 Conrad V. Simoben | Conrad V. Simoben (AMRSC) studied chemistry and obtained his BSc and MSc in 2011 and 2015, respectively, from the University of Buea (Cameroon). He moved to Martin-Luther-University (Germany) in 2016 to pursue a PhD in pharmaceutical and medicinal chemistry in the group of Prof. Wolfgang Sippl under the sponsorship of the German academic exchange service. After completing his PhD in 2021, he was recruited as the head of the computational medicinal chemistry lab of the Centre for Drug Discovery, University of Buea, (UB-CeDD) – Cameroon. He is currently a postdoctoral fellow at the Structural Genomics Consortium, University of Toronto (SGC Toronto), Canada in the group Prof Matthieu Schapira. |
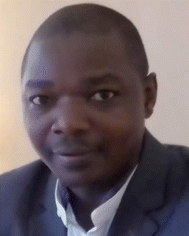 Smith B. Babiaka | Smith B. Babiaka is a Senior Lecturer at the Department of Chemistry, University of Buea (Cameroon), where he received his PhD in Chemistry in 2019. He has served as a consultant at the Agro-Ecohealth Unit, International Institute of Tropical Agriculture (IITA), Cotonou, Benin. Since 2021, he is a Georg Forster Alexander von Humboldt and Georg Forster-Bayer Research Fellow, at the University of Tübingen, Germany where he is searching for novel microbial natural products with interesting antibacterial activity. |
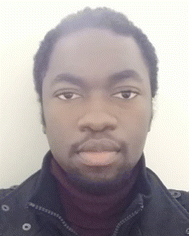 Aurélien F. A. Moumbock | Aurélien F. A. Moumbock is currently a postdoctoral fellow at the University of Freiburg (Germany), where he obtained a PhD in Pharmaceutical Bioinformatics (in 2022) under the guidance of Prof. Stefan Günther. Prior to that, he obtained both BSc and MSc degrees in Chemistry from the University of Buea (Cameroon). His current research involves the development and application of data-intensive computational approaches to accelerate small-molecule drug discovery, with a focus on combating antimicrobial resistance. |
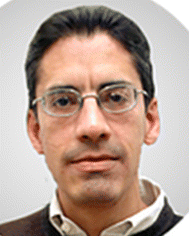 José L. Medina-Franco | José L. Medina-Franco received his PhD degree from the National Autonomous University of Mexico (UNAM). He was a postdoctoral fellow at the University of Arizona and joined the Torrey Pines Institute for Molecular Studies in Florida in 2007. In 2013, he moved to the Mayo Clinic and later joined UNAM as Full Time Research Professor. He currently leads the DIFACQUIM research group. In 2017 he was named Fellow of the Royal Society of Chemistry. His research interests include development and application of chemoinformatics and molecular modeling methods for bioactive compounds with emphasis on drug discovery. |
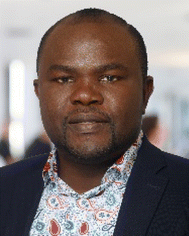 Fidele Ntie-Kang | Fidele Ntie-Kang is an Associate Professor of Pharmaceutical Chemistry at the Department of Chemistry, University of Buea in Cameroon, where he also heads the Center for Drug Discovery. After studying chemistry and physics at the University of Douala in Cameroon, he completed a PhD in computer-aided drug design. He then moved to Germany where he carried out postdoctoral research under Prof. Wolfgang Sippl leading to an habilitation in pharmaceutical chemistry. Since then, his research has been focused on chemoinformatics for natural products (NPs) research, a topic in which he is an editor of a book series. He holds several editorial board appointments and is passionate about drug discovery from NPs identified from African medicinal plants. His major contribution is the development of the African NPs Database. |
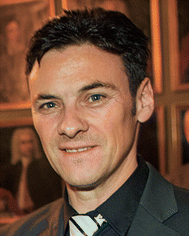 Wolfgang Sippl | Wolfgang Sippl studied Pharmacy at the University in Berlin. He later obtained a PhD in Pharmaceutical Chemistry at the University of Duesseldorf and was a post-doctoral fellow at the University Louis-Pasteur in Strasbourg (France) where he worked with Camille G. Wermuth. He then took a senior researcher position at the University of Duesseldorf before he got a professorship in Medicinal Chemistry in 2003 at the Martin-Luther-University in Halle. His research focuses on the drug design of epigenetic modulators, which not only led to the development of successful virtual screening approaches, but also resulted in the development and biological characterization of novel epigenetic modulators for the treatment of cancer and parasitic diseases. |
Introduction
Communicable and non-communicable diseases continue to be a burden, causing serious affliction to diverse populations globally.1,2 Throughout history, humans have treated diseases and improved the quality of life by applying and using traditional medicine. Knowledge of the preparation or consumption (in several forms) was initially self-taught or passed through generations via word of mouth. It is important to note that formal training in quality control of these natural products (NPs) was not taken into account.3 One possible means of documenting and safeguarding the information and/or standardizing and improving the quality of traditional products being consumed is through scientific exploration/validation of the known traditional methods and the source species.
The richness of the world's flora and fauna is still being explored by c.a. 80% of the population in developing countries as a primary source of healthcare and needs.4 This high percentile dependence of the population can be attributed to socioeconomic reasons, cultural practices, personal beliefs or the difficulty in accessing modern pharmaceutical products, many times associated with the high costs of the latter. This further confirms a shift from synthetically produced medications to the use of NPs.5–8 Although the first pure NPs (e.g., atropine, colchicine, morphine, and strychnine; Fig. 1) were isolated in the early 1800s, it was not until the 1950s that modern medicine and pharmaceutical industry turned toward plants, combinatorial chemistry, and high throughput screening; especially for anticancer drug discovery.9–11 Nowadays, it is evident that global and international marketing of traditional medicinal products has received attention partly due to the popularity, usage, economic value and importance of traditional medicines.12,13
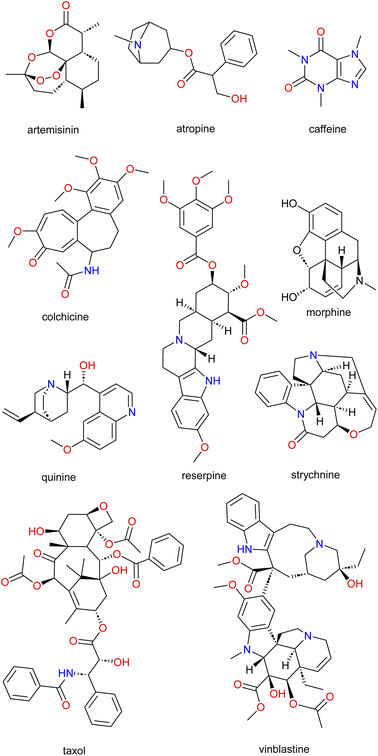 |
| Fig. 1 Examples of well-known NPs. | |
Role of NPs in traditional medicine
The use of traditional medicine in treating diseases throughout history is undebatable and has equally continued to provide a significant contribution to modern medicine. New strategies to search, identify, and develop new drug molecules void of resistance and side effects as well as being cheaper are needed. The revisiting of NPs is one such strategy.14 NPs are isolated from diverse organisms (bacterial, fungi, plants, or animal species). They equally have proven to be a good starting point for the search for pharmacologically active compounds (e.g., caffeine (Coffea spp.), morphine (Papaver somniferum), nicotine (Nicotiana tabacum), antimalarial drugs such as quinine (Cinchona spp.) and artemisinin (Artemisia annua), reserpine (Rauvolfia serpentina) and the anticancer drugs Taxol (Taxus brevifolia) and vinblastine (Catharanthus roseus); Fig. 1) against several ailments.
A broad range of fields such as medicinal chemistry and drug discovery, ecology, biosynthesis and chemical biology, among others, are demonstrating interest in a deeper understanding of NP resources. Likewise, several exciting and new technologies for NP drug discovery for example “smart screening” methods, robotic separation with structural analysis, metabolic engineering, and synthetic biology have seen an absolute increase in recent years.15–18 These exciting and improved strategies coupled with advances in technologies can, therefore, act as a reliable means to acquire more NPs as well as their samples for biological screening.14,19–21 The acquiring of more NPs is in line with what several studies have reported about the advantages of NPs over combinatorially synthesized compounds in the search of biologically relevant and privileged scaffolds.22–33 Principal component analysis (PCA) between NPs, marketed drugs, and synthetic compounds confirmed the aforementioned statement by revealing that combinatorial compounds covered a well-defined and restricted area while drugs and NPs occupied approximately the same chemical space—covering almost all of the combinatorial compounds' diversity space as well as a much larger additional volume.17,20,21,30–39 Designing such NP analogues being inspired by nature is almost impossible, thus, it is necessary to emphasize that the place of NPs remains unique. This uniqueness can therefore be a very helpful step in answering questions such as: (i) which compounds should be prepared? (ii) How should they be prepared (e.g., biological or diversity-oriented synthesis)? and (iii) How should such a biological/diversity-oriented synthesis be planned?17,22–24,40–42
NP databases and in silico-based methods
Low hit rates after screening large synthetic combinatorial databases left drug discoverers the choice to either increase the diversity of combinatorial databases through improved diversity of synthetic reaction(s) and/or make a return to NPs which have worked in the past.43 Historically, NPs have played a significant role in drug discovery, particularly for cancer and infectious diseases. Due to their high structural complexity and diversity, they provide new lead compounds possessing unique scaffolds.14,17,18,20,21,29,34,35,37,44–46 The renewed interest in NPs has invited huge investment in the search (isolation, characterization, and biological evaluation) of NPs from both academia and industrial sectors.14,19 These efforts resulted in an increased number of NPs being isolated, characterised, and reported in the literature.14 NP databases and repositories stand out as one of the major means of safeguarding the collections as well as documenting and sharing the findings.47 There are many examples of these NP databases; from comprehensive47–49 (including compounds from terrestrial, marine and microbial organisms) to focussed ones50,51 (based on a particular disease, or compounds from specific geographical regions or organism types).47,52–54
The application of in silico-based methods55 to explore NP databases to identify potent hit molecules that can be optimized has seen many successful cases.46,56–59 They have played a pivotal role in both pharmaceutical industries and academia during the last three decades and have dramatically reduced the cost factors associated with introducing new drugs to the market.59–63 As an example, virtual screening (VS) procedures (which are useful in narrowing down the number of molecules for experimental testing in biological assays) with/out the combination of other more rigorous in silico-based methods (such as molecular dynamics and binding free energy predictions) are widely used in identifying NP based hit molecules.58–60,62,64 Likewise, accumulated data for NPs have also prompted the growing use of machine learning techniques in several NP drug discovery studies, from the detection of a biosynthetic gene cluster (BGC) to functional annotation of NPs.65,66 Moreover, poor pharmacokinetic profiles, usually discovered at late stages of the drug discovery pipeline (which could be avoided with the help of in silico-based predictions) has prevented many molecules from entering the market.67 Hence, the early computational prediction of absorption, distribution, metabolism, elimination, and toxicity (ADMET) profiles of NP molecules in the drug discovery pipeline has gained increased usage; as well as reducing the cost and the time factor involved for ADMET profiling using standard experimental approaches.67–71 Thus, in silico-based approaches are important for rational drug design-based methods to develop NP analogues with acceptable ADMET properties.17,22–24
Contribution of NPs to modern drug discovery
The global ravages of diseases alongside the challenges of finding drugs with minimal side effects as well as the search for cheaper drug candidates that can treat these diseases, is still a huge challenge to the scientific community. Thus, innovative strategies such as revisiting nature (NPs) which had worked in the past (Fig. 1) can revolutionize and lead to the discovery of novel and potent drugs. Moreover, even with the backdrop of the diminished focus on NPs by the major pharmaceutical companies, scientific studies has shown that NPs still account for about half of the drugs approved by the US Food and Drug Administration (FDA), especially antibiotic and anticancer molecules.44,45,72–79
Review focus
Historically, traditional medicine has been used to treat diseases. The application of these traditional medicinal methods still accounts for the primary source of health care for millions of people. However, the decline in interest by pharmaceutical industries/companies due to challenges such as technical barriers to screening, isolation, characterization and optimization encountered in NPs drug discovery were observed in the 1990s and onwards. Nowadays, several published papers have highlighted the successes of using in silico-based methods in NP drug discovery.43,80–82 Interestingly, the advent of in silico-based approaches which have contributed to boosting the identification of NP hit molecules also comes with some inflated expectations and disappointments.62,82 So far, there is a lack of comprehensive reviews on these challenges, for the scientific community (especially, for early career researchers in this area of research) to access.83
In this review, we highlight the most relevant challenges in the area of in silico-based drug discovery approaches when working on NPs. This will be grounded in a selection of reported challenges encountered during the quest for vital therapeutic agents. We will delve into these challenges through case studies, focusing on specific instances that have been highlighted. The limitations of in silico-based methods have not been included in this review and we would refer the readers to papers that cover that aspect in detail.84,85
Source species and NP databases
Both academia and industry are continually showing a growing interest in NPs as a source for the development of novel and potent molecules or scaffolds for investigation as drug candidates. Investments in this area of NPs have led to the publication of many new molecules, with a good number of the newly published molecules attributed to some positive effects for treating several diseases.48 This rekindled interest in NPs has led to exponential growth in the number of NPs being isolated and characterized. Thus, directly related to the uncontrollable growth in NP databases as a means of sharing information with the scientific community.47 However, this comes with certain challenges for in silico-based drug discovery scientists working on NPs. In the following subsections, the challenges in the development, curation, access, and maintenance of NP databases as well as the problem of choosing from the multitude of NP databases available shall be discussed.
Development of NP databases
This stage is met with challenges that need the help of specialists that are becoming rare and their activities cannot be automated.86,87 Examples of such specialized input include the processes of source species collection and identification, as well as documentation of herbarium information. The problem with source species/organisms is becoming even more challenging with the rate at which natural habitats are rapidly being destroyed amongst other factors.7,88 As of now, an all-inclusive and exhaustive freely accessible database for NPs does not exist. Most of the available NP databases are focus-based, for example on either some particular source organisms,51 geographical locations,48,49 targeted diseases and/or traditional uses.49,50,89 Additionally, no standard protocols have been established for processing and curating available information. Nevertheless, for this to be established, it is going to be another contest on its own, in the quest for whom/which group is going to lead in that aspect. Although this might look difficult, it is feasible and can be achieved; through consolidation and sharing of information via an open platform as proposed by Rutz et al.52 This will enhance and lead to a strong transformative potential for NPs research and beyond. The next challenge in the construction of NP databases after collecting and processing information is the issue of accessibility and maintenance of the databases. Accessibility through web servers that are active and continued over a long period is important. Mitishamba90 is an example of a reported NP database where accessing information is impossible because the provided web links are at present broken or dead. Further accessibility issues are linked to databases that are built for commercial purposes or are not open access.46,47,82 This brings us to the issue of timely maintenance/updating of the provided information. This most often is linked to public databases where funding issues are regularly encountered. Plus, questioning what is considered before an update, is it based on the quantity/quality of new data? A defined time interval? Or what else?
Case study I: the exponential growth of information to be included in NP databases
As aforementioned, NP databases are growing and/or being published with no unified style in their design, construction and/or development like there is for protein sequence and information (UniProt; https://www.uniprot.org),91,92 protein structure (Protein Data Bank, PDB; https://www.rcsb.org)93 or curated classification and nomenclature for organisms (NCBI Taxonomy; https://www.ncbi.nlm.gov/taxonomy).94 With the continuous investment in the area of phytochemicals, a huge amount of data has been generated. However, translating this data into NP databases has seen exponential growth in the content/data information for NP databases, leading to the development of many database systems. The numerous NP databases, however, do not address certain very basic challenges including dereplication46 or cover a significant part of NP resources.12 An exemplified case is that of the NP ATLAS (https://www.npatlas.org); a resourced database maintained at the Simon Fraser University in Canada, focusing on microbial NPs.95,96 Although the database is actively updated, moving from the first version (published in 2019)95 to the recent one (published in 2021)96 saw a great deal of improvement and inclusion in terms of content/information (such as the application programming interface (API), taxonomic descriptions, and chemical ontology amongst others) curated for its end users.
Case study II: choosing NP databases
The area of NP research continues to receive a lot of attention as depicted by the publication of new scientific articles every week to demonstrate the positive effects of NPs on the healing process of various human and animal diseases. This has led to uncontrollable growth in the number of published NP databases.46,47,97 In 2020, Sorokina and Steinbeck47 published a comprehensive review of published NP databases, indicating that less than 50% (of the overwhelming >120) of such resources published and re-used since the year 2000 were open access. It is therefore a real challenge to find a complete, comprehensive, and open-access NP database since available NP databases are mostly constructed to target particular regions,48,98,99 diseases,50 species,51 etc. Therefore, several challenges including data redundancy between the different available databases, poor metadata quality in these databases as well as missing links to other vital databases like the target- and pathogen-centred databases need to be addressed. Addressing them will reduce bias in the exploration of information and increase the connection between chemistry- and biology-centred resources.
Scaffold diversity
In silico-based drug design methods have gained significant applications in the exploration of the uncharted chemical space of small molecules, when searching for new hits in the drug discovery process. However, several questions like which sections of the chemical space should be investigated to identify potential drug candidates? Should chemical space of interest be focused/narrowed to synthetic, NP or pseudo-natural products to identify potential hits? Interestingly, the unique features of NPs (such as enormous scaffold diversity and structural complexity characterised by higher molecular mass, a larger number of sp3 carbon atoms and oxygen atoms, but fewer nitrogen and halogen atoms, higher numbers of H-bond acceptors and donors, lower calculated octanol–water partition coefficients, and greater molecular rigidity compared with synthetic compound libraries)100,101 when compared to synthetic compounds can be explored for innovative solutions in the search of novel therapeutic agents for the treatment of diseases.44,46,47,51,52,59,77,80,102 Thus, the unique features of NPs have offered pharma industries and academic research groups opportunities to focus on cutting-edge computational technologies to facilitate the identification of novel NP-based hits. However, several challenges including expansion of the searchable drug-like chemical space, and revisiting neglected, or non-traditional chemical spaces are encountered in this process. Also, limitations in the synthetic routes required to obtain the complex structures of NPs as well as the laborious process involved in the isolation of a single chemical constituent, usually in low yields are also various types of difficulties encountered in wet labs.103 An example of a study that illustrates the hurdles that could be encountered with the diversity of NPs in in silico-based drug discovery is presented below.
Case study I: an example of a challenge observed with NPs diversity
The spatial arrangement, different configurations, three-dimensional molecular shape and ring complexity of NPs constitute a few of the observed structural complexity. In addition to the structural complexity, the limited amount of biological data makes it challenging to develop in silico-based methods that are focused on NPs. The development and application of such in silico-based methods and algorithms for NPs will necessitate even more complex force fields to deal with their structural complexity. This challenge leaves this research branch of cheminformatics very active.82 Friedrich et al.,104 compared seven free 3D conformer ensemble generators (RDkit DG algorithm, Experimental-Torsion basic knowledge distance Geometry algorithm (ETKDG), Confab, Frog2, Multiconf-DOCK, Balloon DG, and GA algorithms) to eight commercial counterparts (ConfGen, ConfGenX, cxcalc, iCon, MOE Low modeMD, MOE Stochastic, MOE Conformation Import, and OMEGA) using the Platinum Diverse Dataset. The Platinum Diverse Dataset is a high-quality benchmarking dataset of 2859 protein-bound ligand conformations extracted from the PDB.104–106 However, Chen et al.97 in 2018 characterized the chemical space of known and readily available NPs. They reported that of the over 250
000 structures of NPs available from public databases, only ∼2000 NPs were identified with at least one X-ray crystal structure of the compound in complex with a biomacromolecule available from the PDB.97 This is quite a very small data to be used as a representative for the entirety of NPs characterized so far.
Going back to the observations from Friedrich et al.,104–106 the best 3D conformer generators were the commercially available ones. Shortcomings of the free tools include critical errors in bond lengths, bond angles assignment and planarity or out-of-plane errors in the conformers generated. Although the commercially available algorithms were better, some abnormalities regarding geometries were also observed. All these lead to the conclusion that it is a research race to solve the challenge of an accurate algorithm capable of handling the diverse complexity of structures with ideas to include more structures of NPs.
Starting structure (prodrugs)
Prodrugs represent a class of chemotherapeutics which remain inactive in the body until metabolized. The idea of prodrugs is to overcome pharmacokinetic and pharmacodynamic barriers (such as poor solubility, absorption, toxicity, side effects, and poor efficacy among other properties).107 This class of molecules (such as sulfasalazine, latanoprostene, psilocybin, aspirin, codeine, irinotecan, L-dopa, heroin, and several antiviral nucleosides) have enjoyed clinical successes over a long time for treating chronic and acute conditions.108,109 Prodrugs could occur naturally or they could be derived from semisynthetic processes or synthetic-designed intentionally during the rational drug design or unintentionally during the drug development.109
Drug-likeness
The concept of drug-likeness focuses on the similarity of some physicochemical properties such as molecular mass, hydrophobicity, lipophilicity, polarity and hydrogen bond donors/acceptors.110,111 Analysis of these properties shows that approved drugs preferentially fall within a certain range of values and new compounds with physicochemical properties that fall within that range are likely to be considered as “drug-like”. Fascinatingly, in silico-based methods are being used to predict such properties from the molecular structure before the substance is even synthesized and tested. The rule of 5 (Ro5) and the quantitative estimate of drug-likeness (QED) discussed below represent a few of the approaches used to predict drug-likeness.
Rule of five (Ro5)
Since its inception in a seminal work of Lipinski and co-workers in the late 90s,111 Ro5 has gained popularity in the medicinal chemistry community as guidelines to computationally estimate the “drug-likeness” of pharmacologically relevant molecules. This set of guidelines is summarized as follows: molecular weight (MW) < 500 Da, octanol/water partition coefficient (log
P) < 5, H-bond donor (HBD) ≤ 5, and H-bond acceptor (HBA) ≤ 10. Generally, molecules with no more than one Ro5 violation are considered to be “drug-like” or orally available. Despite Lipinski's recommendation that the Ro5 be considered as a guideline, reality has it that it is used routinely to filter chemical libraries, especially during VS as a primary step. Since most NPs have complex structures with more than one Ro5 violation, they have significantly received less attention as potential lead compounds. They are underrepresented in ready-to-use VS libraries such as the ZINC library.112 This does not come as a surprise because the Ro5 did not consider NPs and substrates of biological transporters.
Quantitative estimate of drug-likeness (QED)
Twelve years after the establishment of the Ro5, Bickerton et al.110 proposed an updated measure of drug-likeness based on the concept of desirability called the quantitative estimate of drug-likeness (QED). QED grades a molecule on a range from zero to one representing the favourability of the properties. Molecules with all the properties being unfavourable are graded zero while those with all the properties being favourable are graded one. To demonstrate the utility of this approach, QED was used to describe the desirability functions derived from a set of orally absorbed approved drugs as well as to solve the problem of molecular target druggability on a large set of published bioactive compounds. This approach compared favourably with rule-based metrics such as Lipinski's Ro5 (e.g., at the levels of predictivity, intuitive and simplicity to implement). QED offers an even richer, more nuanced view of drug-likeness as it can identify cases in which a generally unfavourable property may be tolerated when the other parameters are close to ideal.
Case study I: evaluating the drug-likeness of StreptomeDB 2.0
In 2013, Ntie-Kang113 evaluated the drug likeness of about 2400 NPs of StreptomeDB 2.0; currently in its third version.51,114 StreptomeDB represents a database of NPs isolated from Gram-positive bacteria of the genus Streptomyces, constituting the largest source of clinical antibiotics. In addition to Ro5 descriptors, the number of rotatable bonds (NRB) was equally computed following the work of Veber et al.115 From this evaluation, it was observed that 52.5% of the compounds within StreptomeDB 2.0 had at least one Ro5 violation; meanwhile, 22.7% had more than two violations. Taken individually, MW was the descriptor exhibiting the most skewed distribution; approximately 42% of the molecules had MW > 500 Da (Fig. 2). Nevertheless, when pairwise compared in biplots, a trade-off in the interrelationships between these descriptors were observed by Ntie-Kang113 (Fig. 3).
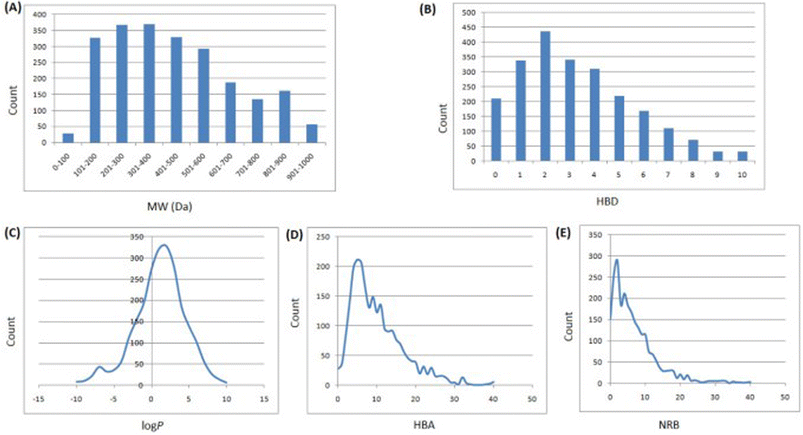 |
| Fig. 2 Ro5 evaluation of StreptomeDB 2.0. (A) MW, (B) HBD, (C) log P, (D) HBA, (E) NRB. The original figure was published under a Creative Commons License. | |
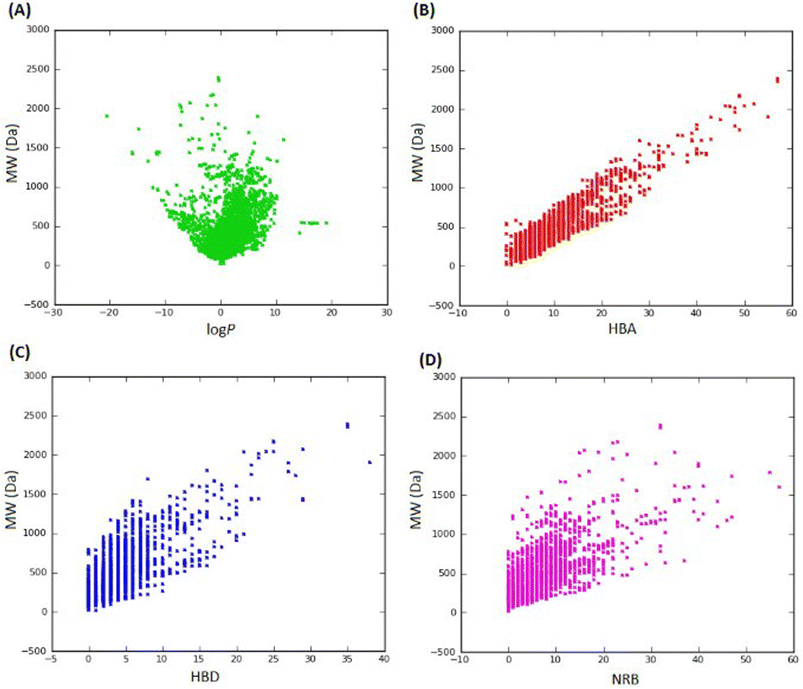 |
| Fig. 3 Pairwise comparison of Ro5 descriptors of StreptomeDB 2.0. (A) MW against log P, (B) MW against HBA, (C) MW against HBD and (D) MW against NRB. The original figure was published under a Creative Commons License. | |
It is worth mentioning that the vast majority of molecules with more than one Ro5 violation (beyond Ro5) in the StreptomeDB 2.0, are polyketides. This class of microbial NPs has previously yielded several important antibiotics such as streptomycin and erythromycin, anticancers such as bleomycin, and dactinomycin, and anthelmintics such as avermectins. But most newly isolated and characterized polyketides of this database remain pharmaceutically untapped.
Case study II: drug-likeness evaluation of marine NPs
More recently, Pilkington performed a drug-likeness evaluation of a dataset of 179 marine NPs originating from three kingdoms (Animalia, Bacteria, and Fungi).116 Ro5 descriptors were computed as well as NRB, topological polar surface area (TPSA) and water solubility (log
S). Based on defined thresholds, the authors grouped these NPs into three categories, namely, leadlike, druglike, and known drug spaces (Table 1).
Table 1 Classification of chemical spaces. Adapted from a paper published under a Creative Commons License116
Descriptor |
Leadlike |
Druglike |
Known drug |
MW |
300 |
500 |
800 |
log P |
3 |
5 |
6.5 |
HBD |
3 |
5 |
7 |
HBA |
3 |
10 |
15 |
TPSA |
60 |
140 |
180 |
NRB |
3 |
10 |
17 |
A PCA was performed to assess the interrelationships of computed descriptors. The two principal components of this analysis were the variability in the data (x-axis) and the dimensionality of variability (y-axis), with values of 71.5% and 17.5%, respectively (Fig. 4).
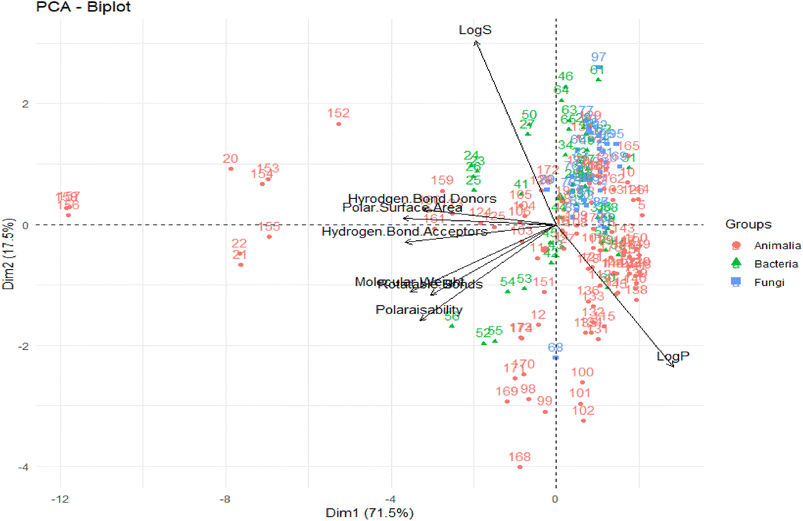 |
| Fig. 4 PCA of computed descriptors. The original figure was published under a Creative Commons License. | |
From this PCA analysis, they observed that except for log
S and log
P, all other descriptors greatly contributed to the variability. Moreover, log
P, log
S, and TPSA contributed the most to the second principal component. Overall, the chemical space distribution of these marine NPs in the “lead-like”, “drug-like” and known drug categories are 0.5%, 39.7%, and 64.8%, respectively. The contrast between the population of the known drug space of marine NPs, and that of the “drug-like” and “lead-like” spaces, rationalizes their underrepresentation in typical VS libraries.
Hits optimization
The next step after the bioassay-guided isolation of bioactive NPs is the optimization of the hit molecule(s) using diverse strategies to improve their pharmacodynamic and pharmacokinetic properties, and in turn increase their biological activities.103,117 While Ro5 provides a quick overview of the oral availability of molecules of interest, a more comprehensive evaluation of their pharmacokinetics and toxicity profiles is generally required. In silico predictions of ADMET properties guide the prioritization of compounds for the more cost- and labour-intensive in vitro and/or in vivo preclinical pharmacokinetics evaluations. This in turn informs lead optimization and drug candidate selection. Some of the most popular software tools used for in silico ADMET predictions include pkCSM,118 ADMETlab,119 QikProp (Schrödinger, LLC, New York, NY),120 SwissADME121 and admetSAR.122 These tools rely upon quantitative structure–property relationship (QSPR) models built from experimentally available data of small-molecules.123 Although ADMET prediction tools have achieved great success for structurally diverse compounds, their reliability is put into question for predictions concerning NPs because these models are mainly trained and benchmarked on experimental data of structurally simple synthetic molecules. Hence, the selection of NPs for preclinical studies has been hampered by their undesirable predicted ADMET properties, despite displaying interesting biological activities in phenotypic screens. We briefly highlight a few of such cases.
Case study I: ADMET evaluation of the Mexican NPs of the BIOFACQUI
In 2020, Medina-Franco and co-workers carried out a computational ADMET evaluation of the Mexican NPs of the BIOFACQUIM.124,125 A comparison was made with the profile of the African NPs of the AfroDB,99 the Brazilian NPs of NuBBEDB,98 the Chinese NPs of the TCM126 and the FDA-approved drugs dataset of the DrugBank.127 The authors used the pkCSM118 and the SwissADME121 webservers. From these predictions, it could be deduced that the absorption profile of BIOFACQUIM was similar to those of NuBBEDB, TCM and AfroDB; meanwhile, its distribution profile was similar to that of the FDA dataset based on the blood–brain barrier (BBB) permeability and the unbound fraction descriptors. Moreover, its metabolism profile was similar to those of AfroDB, TCM, NuBBEDB and the FDA dataset based on CYP1A2, CYP2D6, CYP3A4, and CYP2C19 inhibition, respectively. On the other hand, its excretion profile was more similar to that of TCM than that of the FDA dataset. Its toxicity profile was similar to that of the FDA dataset for the Human Ether-a-go-go-related Gene (hERG) II inhibition and Ames toxicity, and similar to that of TCM only for Ames toxicity. Its toxicity profile for hERG I inhibition is similar to those of AfroDB, NuBBEDB, and TCM. Lastly, its hepatotoxicity profile was similar to those of NuBBEDB and TCM. Altogether, based on a PCA on 16 ADMET descriptors, the chemical space coverage of each dataset was charted (Fig. 5).
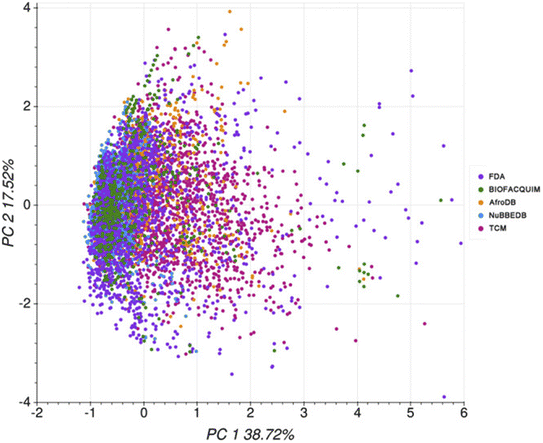 |
| Fig. 5 PCA on ADMET descriptors for selected compound datasets (variance = 56.2%). The original figure was published under a Creative Commons License. | |
Case study II: ADMET profile of a dataset of protein–protein interface inhibitors
In 2017, Lagorce et al.,128 described the computed ADMET profile of a dataset of protein–protein interface inhibitors (iPPIs) collected from the IPPI-DB,129 TIMBAL,130 as well as that of a non-iPPIs dataset of orthosteric and allosteric inhibitors extracted from the ChEMBL database.131 The development of iPPIs is of growing interest because they address targets that are typically considered to be “undruggable”. The ADMET profiles of both constructed datasets were assessed with the pkCSM118 server in a similar fashion as described in the above example. Proteins that molecularly recognize other proteins have a typical binding sites, that is, large surface grooves that cannot be addressed by typical small-molecule ligands but rather by beyond-Ro5 molecules.132 The observations arrived at similar conclusions from their ADMET profiling. Numerous NP (macrocyclic) polyketides have been identified as very potent iPPIs.133 It is worth mentioning that Lagorce et al.128 excluded from the ADMET profiling all NP-(derived) molecules on the basis that this class of molecules are not equally included in training and/or benchmark datasets of ADMET prediction models such as that of pkCSM.118
Sample availability
Physical samples of VS hits with interesting pharmacokinetic properties are needed for screening in wet laboratories against different assays of interest. These physical samples (pure compounds isolated from complex mixtures) form the basis of the standard methodology in NPs drug discovery. However, studies have shown that for a virtual collection of ∼250
000 NPs only about 10% of the collection is readily available for purchase.46 Also, the anti-biopiracy laws, including the Nagoya Protocol have been a major regulation for researchers involved in the bioprospecting of NPs from both the terrestrial and marine ecosystems.134–136 Adoption of the Convention on Biological Diversity (CBD), which came into force on December 29, 1993, aimed to promote sustainable use of biodiversity as well as conservation and benefit sharing of genetic resources. CBD restricts the collection and evaluation of plant, marine, and other samples by researchers. This amongst other factors, makes NP chemists face challenges in the isolation and purification of bioactive NPs from plants and marine organisms.137 In the majority of cases, the bioactive compounds are present in too low concentrations to be efficiently isolated, variation in the active constituents, loss of activity or failure in isolating the target bioactive compounds, degradation of heat labile compounds during the purification process; bioactivity was as a result of synergistic effects between multiple compounds.103 After the identification of some molecules as VS hits, the cost of collection of the samples (plants and access to the deep in the case of marine microorganisms) as well as synthesizing NPs with high structural complexity is expensive, tedious and time-consuming coupled with associated synthesis scale-up issues. A glimpse of a few of the mentioned challenges listed here for some in silico-based driven studies are discussed in the examples below.
Case study I: repurposed use of taxol
With the advent of the COVID-19 pandemic, several studies have been performed in other to suggest different specific treatment regimens as well as propose new antivirals with different therapeutic targets, that can help to reduce the level of morbidity and mortality.138–145 One such computational study to suggest drug candidates to treat a viral infection was reported by Rajput et al.146 In the study, several drug molecules were suggested using drug-target network analysis for repurposing drug molecules as potential antivirals to combat epidemics and pandemics (Fig. 6). One such molecule is paclitaxel (also known as Taxol Fig. 1) an effective agent against the influenza virus. Taxol is an approved molecule for the treatment of Kaposi's sarcoma and lung cancer (by the US FDA) and other studies have equally suggested that it could treat skin disorders, renal and hepatic fibrosis, inflammation, axon regeneration, limb salvage, influenza and coronary artery restenosis at low concentrations. However, the suggestion of Taxol as a potential antiviral agent comes with challenges as it is difficult to obtain meaningful quantities of this bioactive NP for biological screening assay as well as for commercial supply globally.147
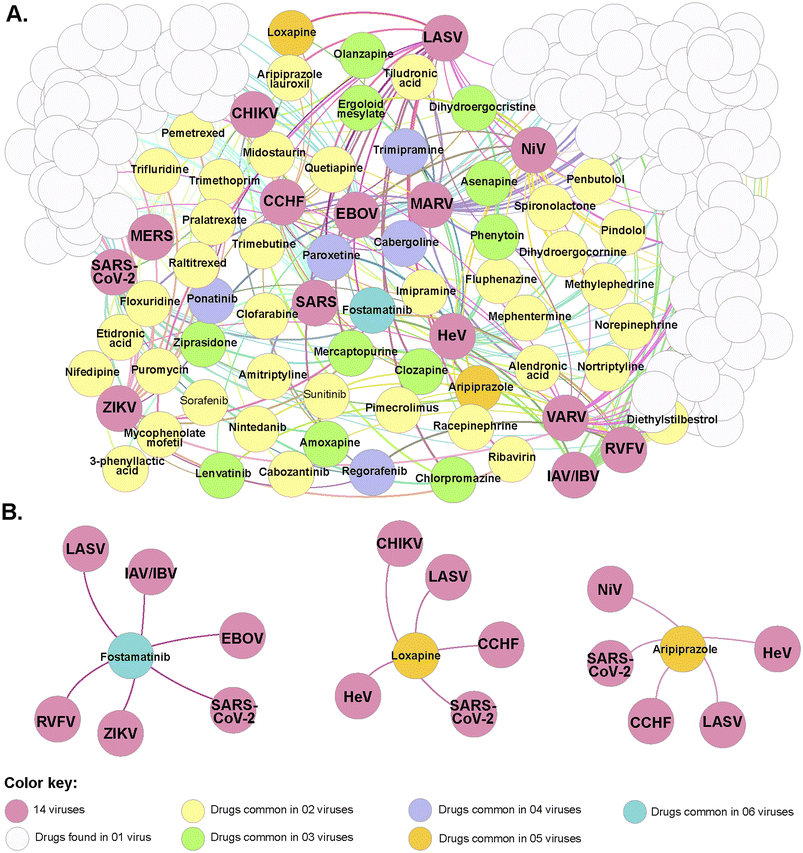 |
| Fig. 6 The network displayed common repurposed drugs between different viruses using the pipeline generated by Rajput et al.146 (A) Correlations between the repurposed drugs identified using the “drug-target-drug” approach and 14 viruses causing epidemics/pandemic. (B) Interaction diagram of identified repurposed drugs found in common for more than five viruses (figure reproduced with permission). | |
Case study II: evaluating the histone deacetylase (HDAC) inhibitory activities of NPs from the African NP database collection
Another example of a work that highlights the challenges in getting and testing NP samples after in silico studies is reported by Simoben et al.148 In this study, several computational methods coupled to biological testing were applied to suggest novel inhibitors of HDAC isoforms.148–150 HDACs represent an interesting class of zinc-dependent enzymes with very important physiological roles that are linked to several diseases such as cancer, cardiac and neurodegenerative diseases, inflammation, metabolic and immune disorders, and viral and parasitic infections.151–155 Interestingly, about two hundred molecules inspired by nature have been investigated as HDAC inhibitors.156–166 Analysis of a database of NPs from African source species showed that they occupy chemical spaces that were not previously reported in published NP databases as well as compounds that are similar to HDAC inhibitors. After the VS of the said database, a set of molecules was proposed as hits (Fig. 7). However, of the seventeen molecules suggested, only three were experimentally tested due to the difficulty in obtaining physical samples or the extravagant cost of some of the hits. Thus, the proposed hits may act as a starting point in a structure-based design and/or in chemical optimization efforts to improve the suggested novel HDAC inhibitors. Also, the study supports the idea that in silico approaches can readily identify novel HDAC modulators.
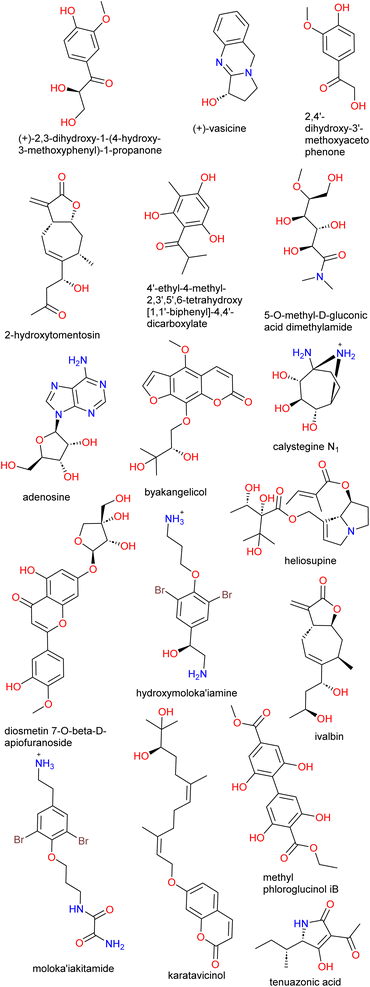 |
| Fig. 7 Virtual hits from ANPDB as HDAC inhibitors. | |
Case study III: in silico identification of bichalcones as sirtuin inhibitors
Another interesting study by Karaman et al. shows that there is a challenge in obtaining physical samples of NPs involved in the in silico-based approach to identify bichalcones as sirtuin inhibitors.167 Sirtuins represent a subset (Class III) of histone deacetylases and are characterized as nicotinamide adenine dinucleotide (NAD+)-dependent.168 Sirtuins have been linked to several diseases, including cancer, HIV, and neurodegeneration.168–171 With the attention NPs have received in the quest to search for sirtuin inhibitors. Karaman et al. performed VS using the pan-African Natural Products Library (p-ANAPL)172 (a collection of physical samples of NPs from African source species) to suggest hit molecules as sirtuin inhibitors. From the virtual study, 22 hits were proposed (Fig. 8). However, only five compounds (about 25% of the suggested hits) had sufficient quantities to further investigate their in vitro activity as sirtuins inhibitors. Of the five molecules biologically evaluated, two of them (the bichalcones rhuschalcone IV and an analogue of rhuschalcone I (Fig. 8); isolated from the medicinal plant Rhus pyroides) showed in vitro activity. The results therefore showed that these molecules could represent a class of compounds that can be optimized to improve the biological activities as sirtuins inhibitors. Additionally, the authors also provided suggestions based on in silico-based studies, on how the biological activities could be improved.
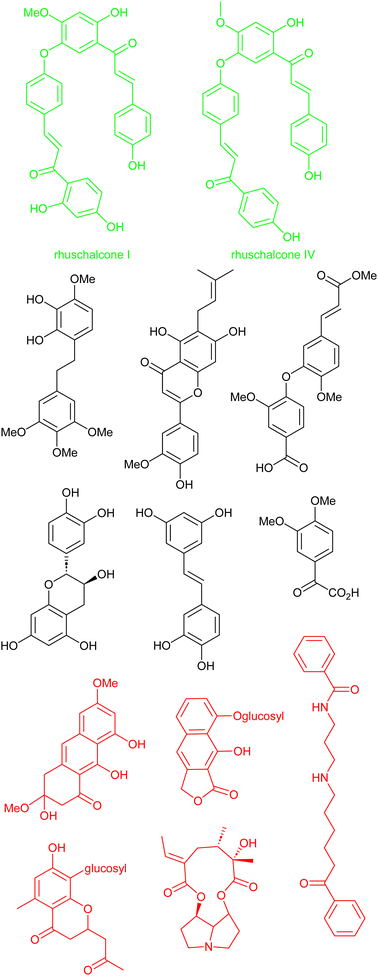 |
| Fig. 8 13 out of the 22 suggested virtual hits: black (>1 mg), red (tested negative) and green (active). | |
Intellectual property
Identification of virtual hits with interesting pharmacokinetic properties needs to be confirmed through different biological screening assays in the wet laboratories using the physical samples. Sadly, accessing sufficient quantities of the identified virtual hits for characterisation and biological assaying may also be challenging. The Nagoya Protocol has disadvantaged NP researchers.134–136 This hurdle even restricted researchers from the collection and evaluation of plant and marine samples after the adoption of the Convention on Biological Diversity (CBD), which came into force on December 29, 1993, to promote sustainable use of biodiversity as well as conservation and benefit sharing of genetic resources.136,173 Further complexities are observed at the levels of (i) filling in for intellectual property (IP)174 rights when it comes to (unmodified) NPs exhibiting very interesting bioactivities and (ii) the benefit sharing due to regulations defined by the countries of origin where the biological material was collected (especially when it concerns marine genetic samples).134–136
Conclusions
NPs have played an important role in improving the living conditions of humans as well as in the treatment of ailments. Despite the many examples of advantages and success stories recorded for NP drug discovery, several challenges encountered have led pharmaceutical companies to reduce programmes in this sector. This review is intended to highlight the challenges that are encountered when applying in silico-based methods in the search for new drug candidates from a NP perspective. Some of the challenges discussed herein include but are not limited to the computational power and skills, to access and explore the numerous and large data collection of NP databases and, the availability of physical samples for compounds identified as hits in reasonable quantity. Having an idea of the discussed challenges would prepare the minds of interested scientists in this area of research as well as give them an orientation.
Author contributions
C. V. S., W. S., J. L. M.-F., and S. G. conceived the idea. All authors were involved in the writing and compilation of the manuscript.
Conflicts of interest
There are no conflicts to declare.
Acknowledgements
S. B. B. acknowledges funding from the Humboldt Foundation for Georg Förster and Georg Forster-Bayer Alexander von Humboldt (Ref.3.4–CMR–1220727–GF-P) postdoctoral research fellowships. F. N. K. acknowledges a Calestous Juma Science Leadership Fellowship from the Bill & Melinda Gates Foundation (award number: INV-036848). F. N. K. also acknowledges joint funding from the Bill & Melinda Gates Foundation and LifeArc (award number: INV-055897) under the African Drug Discovery Accelerator program. FNK acknowledges further funding from the Alexander von Humboldt Foundation for a Research Group Linkage project.
References
- World Health Organization, Noncommunicable diseases, https://www.who.int/news-room/fact-sheets/detail/noncommunicable-diseases, accessed 02-10-2023, 2023.
- World Health Organization, Health products in the pipeline from discovery to market launch for all diseases, https://www.who.int/observatories/global-observatory-on-health-research-and-development/monitoring/health-products-in-the-pipeline-from-discovery-to-market-launch-for-all-diseases, accessed 02-10-2023, 2023.
- P. E. McGovern, A. Mirzoian and G. R. Hall, Proc. Natl. Acad. Sci. U. S. A., 2009, 106, 7361–7366 CrossRef CAS PubMed.
- O. Oyebode, N.-B. Kandala, P. J. Chilton and R. J. Lilford, Health Policy Plan., 2016, 31, 984–991 CrossRef PubMed.
- D. J. Newman, G. M. Cragg and K. M. Snader, Nat. Prod. Rep., 2000, 17, 215–234 RSC.
- G. M. Rishton, Am. J. Cardiol., 2008, 101, 43d–49d CrossRef CAS PubMed.
- G. A. Cordell, Phytochem. Lett., 2014, 10, xxviii–xxvxi CrossRef.
- H. A. R. Suleria, S. Osborne, P. Masci and G. Gobe, Mar. Drugs, 2015, 13, 6336–6351 CrossRef CAS PubMed.
- R. J. Huxtable and S. K. Schwarz, Mol. Interventions, 2001, 1, 189 CAS.
- M. Hesse, Alkaloids: nature's curse or blessing?, John Wiley & Sons, 2002 Search PubMed.
- F. Malerba and L. Orsenigo, Bus. Hist., 2015, 57, 664–687 CrossRef.
- M. J. Rein, M. Renouf, C. Cruz-Hernandez, L. Actis-Goretta, S. K. Thakkar and M. D. Pinto, Br. J. Clin. Pharmacol., 2013, 75, 588–602 CrossRef CAS PubMed.
- B. E. van Wyk, J. Ethnopharmacol., 2008, 119, 342–355 CrossRef PubMed.
- A. L. Harvey, R. Edrada-Ebel and R. J. Quinn, Nat. Rev. Drug Discovery, 2015, 14, 111–129 CrossRef CAS PubMed.
- C. R. Pye, M. J. Bertin, R. S. Lokey, W. H. Gerwick and R. G. Linington, Proc. Natl. Acad. Sci., 2017, 114, 5601–5606 CrossRef CAS PubMed.
- P. R. Jensen, K. L. Chavarria, W. Fenical, B. S. Moore and N. Ziemert, J. Ind. Microbiol. Biotechnol., 2014, 41, 203–209 CrossRef CAS PubMed.
- M. Pascolutti and R. J. Quinn, Drug Discovery Today, 2014, 19, 215–221 CrossRef CAS PubMed.
- G. D. Wright, Can. J. Microbiol., 2014, 60, 147–154 CrossRef CAS PubMed.
- B. O. Bachmann, S. G. Van Lanen and R. H. Baltz, J. Ind. Microbiol. Biotechnol., 2014, 41, 175–184 CrossRef CAS PubMed.
- M. A. Koch, A. Schuffenhauer, M. Scheck, S. Wetzel, M. Casaulta, A. Odermatt, P. Ertl and H. Waldmann, Proc. Natl. Acad. Sci., 2005, 102, 17272–17277 CrossRef CAS PubMed.
- H. Lachance, S. Wetzel, K. Kumar and H. Waldmann, J. Med. Chem., 2012, 55, 5989–6001 CrossRef CAS PubMed.
- M. G. Sankar, L. Mantilli, J. Bull, F. Giordanetto, J. O. Bauer, C. Strohmann, H. Waldmann and K. Kumar, Bioorg. Med. Chem., 2015, 23, 2614–2620 CrossRef CAS PubMed.
- K. Kumar and H. Waldmann, Angew. Chem., Int. Ed., 2009, 48, 3224–3242 CrossRef CAS PubMed.
- N. R. Gade and J. Iqbal, Chemistryselect, 2018, 3, 6262–6266 CrossRef CAS.
- D. Sun and D. Gündisch, Curr. Top. Med. Chem., 2016, 16, 1199 CrossRef CAS PubMed.
- D.-X. Kong, W. Ren, W. Lü and H.-Y. Zhang, J. Chem. Inf. Model., 2009, 49, 2376–2381 CrossRef CAS PubMed.
- M. A. Koch and H. Waldmann, Drug discovery today, 2005, 10, 471–483 CrossRef CAS PubMed.
- P. D. Dobson, Y. Patel and D. B. Kell, Drug discovery today, 2009, 14, 31–40 CrossRef CAS PubMed.
- E. K. Davison and M. A. Brimble, Curr. Opin. Chem. Biol., 2019, 52, 1–8 CrossRef CAS PubMed.
- M. Feher and J. M. Schmidt, J. Chem. Inf. Comput. Sci., 2003, 43, 218–227 CrossRef CAS PubMed.
- K. Grabowski, K.-H. Baringhaus and G. Schneider, Nat. Prod. Rep., 2008, 25, 892–904 RSC.
- N. Singh, R. Guha, M. A. Giulianotti, C. Pinilla, R. A. Houghten and J. L. Medina-Franco, J. Chem. Inf. Model., 2009, 49, 1010–1024 CrossRef CAS PubMed.
- D. Camp, R. A. Davis, M. Campitelli, J. Ebdon and R. J. Quinn, J. Nat. Prod., 2012, 75, 72–81 CrossRef CAS PubMed.
- K. Grabowski and G. Schneider, Curr. Chem. Biol., 2007, 1, 115–127 CAS.
- J. Larsson, J. Gottfries, S. Muresan and A. Backlund, J. Nat. Prod., 2007, 70, 789–794 CrossRef CAS PubMed.
- D. Lowe, Chem. World, 2008, 5, 18 Search PubMed.
- J. Rosén, J. Gottfries, S. Muresan, A. Backlund and T. I. Oprea, J. Med. Chem., 2009, 52, 1953–1962 CrossRef PubMed.
- F. López-Vallejo, M. A. Giulianotti, R. A. Houghten and J. L. Medina-Franco, Drug discovery today, 2012, 17, 718–726 CrossRef.
- J. Gu, Y. Gui, L. Chen, G. Yuan, H.-Z. Lu and X. Xu, PLoS One, 2013, 8, e62839 CrossRef CAS PubMed.
- C. J. Gerry and S. L. Schreiber, Nat. Rev. Drug Discovery, 2018, 17, 333–352 CrossRef CAS.
- H. Van Hattum and H. Waldmann, J. Am. Chem. Soc., 2014, 136, 11853–11859 CrossRef CAS PubMed.
- S. Wetzel, R. S. Bon, K. Kumar and H. Waldmann, Angew. Chem., Int. Ed., 2011, 50, 10800–10826 CrossRef CAS PubMed.
- C. V. Simoben, 2021, https://books.google.ca/books?id=4BxuzgEACAAJ.
- B. G. de la Torre and F. Albericio, Molecules, 2019, 24, 809 CrossRef.
- Y. H. Kang, H. J. Lee, C. J. Lee and J. S. Park, Biomol. Ther., 2019, 27, 503–513 CrossRef CAS PubMed.
- Y. Chen, C. de Bruyn Kops and J. Kirchmair, J. Chem. Inf. Model., 2017, 57, 2099–2111 CrossRef CAS PubMed.
- M. Sorokina and C. Steinbeck, J. Cheminf., 2020, 12, 1–51 Search PubMed.
- F. Ntie-Kang, K. K. Telukunta, K. Doring, C. V. Simoben, A. M. AF, Y. I. Malange, L. E. Njume, J. N. Yong, W. Sippl and S. Gunther, J. Nat. Prod., 2017, 80, 2067–2076 CrossRef CAS PubMed.
- C. V. Simoben, A. Qaseem, A. F. Moumbock, K. K. Telukunta, S. Günther, W. Sippl and F. Ntie-Kang, Mol. Inf., 2020, 39, 2000163 CrossRef CAS PubMed.
- M. Mangal, P. Sagar, H. Singh, G. P. Raghava and S. M. Agarwal, Nucleic Acids Res., 2013, 41, D1124–D1129 CrossRef CAS PubMed.
- A. F. Moumbock, M. Gao, A. Qaseem, J. Li, P. A. Kirchner, B. Ndingkokhar, B. D. Bekono, C. V. Simoben, S. B. Babiaka and Y. I. Malange, Nucleic Acids Res., 2021, 49, D600–D604 CrossRef CAS PubMed.
- A. Rutz, M. Sorokina, J. Galgonek, D. Mietchen, E. Willighagen, J. G. Graham, R. Stephan, R. Page, J. Vondrasek and C. Steinbeck, bioRxiv, 2021, 433265 Search PubMed.
- M. Sorokina, P. Merseburger, K. Rajan, M. A. Yirik and C. Steinbeck, J. Cheminf., 2021, 13, 1–13 Search PubMed.
- A. Rutz, M. Sorokina, J. Galgonek, D. Mietchen, E. Willighagen, A. Gaudry, J. G. Graham, R. Stephan, R. Page and J. Vondrasek, bioRxiv, 2021 Search PubMed , preprint.
- E. López-López, J. r. Bajorath and J. L. Medina-Franco, J. Chem. Inf. Model., 2020, 61, 26–35 CrossRef PubMed.
- N. Tran, T. Van, H. Nguyen and L. Le, Int. J. Med. Sci., 2015, 12, 163–176 CrossRef CAS PubMed.
- S. Kar and K. Roy, Expert Opin. Drug Discovery, 2013, 8, 245–261 CrossRef CAS PubMed.
- R. Pegu, B. P. Sarma, R. Borua, S. Sinha, S. Johari and A. Ghosh, Indian J. Pharmacol., 2014, 46, S37 Search PubMed.
- J. D. Romano and N. P. Tatonetti, Front. Genet., 2019, 10 Search PubMed.
- A. Heifetz, M. Southey, I. Morao, A. Townsend-Nicholson and M. J. Bodkin, Methods Mol. Biol., 2018, 1705, 375–394 CrossRef CAS PubMed.
- H. Willems, S. De Cesco and F. Svensson, J. Med. Chem., 2020, 63, 10158–10169 CrossRef CAS PubMed.
- M. H. Baig, K. Ahmad, S. Roy, J. M. Ashraf, M. Adil, M. H. Siddiqui, S. Khan, M. A. Kamal, I. Provaznik and I. Choi, Curr. Pharm. Des., 2016, 22, 572–581 CrossRef CAS PubMed.
- D. J. Cole, J. T. Horton, L. Nelson and V. Kurdekar, Future Med. Chem., 2019, 11, 2359–2363 CrossRef CAS PubMed.
- F. Fratev, T. Steinbrecher and S. O. Jonsdottir, ACS Omega, 2018, 3, 4357–4371 CrossRef CAS PubMed.
- H. U. Kim, K. Blin, S. Y. Lee and T. Weber, Curr. Opin. Microbiol., 2017, 39, 113–120 CrossRef CAS PubMed.
- D. Prihoda, J. M. Maritz, O. Klempir, D. Dzamba, C. H. Woelk, D. J. Hazuda, D. A. Bitton and G. D. Hannigan, Nat. Prod. Rep., 2021, 38, 1100–1108 RSC.
- F. Darvas, G. Keseru, A. Papp, G. Dorman, L. Urge and P. Krajcsi, Curr. Top. Med. Chem., 2002, 2, 1287–1304 CrossRef CAS PubMed.
- M. P. Gleeson, A. Hersey and S. Hannongbua, Curr. Top. Med. Chem., 2011, 11, 358–381 CrossRef CAS PubMed.
- J. Hodgson, Nat. Biotechnol., 2001, 19, 722–726 CrossRef CAS PubMed.
- M. A. Navia and P. R. Chaturvedi, Drug Discovery Today, 1996, 1, 179–189 CrossRef CAS.
- J. Jeon, S. Kang and H. U. Kim, Nat. Prod. Rep., 2021, 38, 1954–1966 RSC.
- D. J. Newman and G. M. Cragg, J. Nat. Prod., 2016, 79, 629–661 CrossRef CAS PubMed.
- F. Zhu, C. Qin, L. Tao, X. Liu, Z. Shi, X. H. Ma, J. Jia, Y. Tan, C. Cui, J. S. Lin, C. Y. Tan, Y. Y. Jiang and Y. Z. Chen, Proc. Natl. Acad. Sci., 2011, 108, 12943–12948 CrossRef CAS PubMed.
- P. Shinde, P. Banerjee and A. Mandhare, Expert Opin. Ther. Pat., 2019, 29, 283–309 CrossRef CAS PubMed.
- L. Tao, F. Zhu, C. Qin, C. Zhang, S. Y. Chen, P. Zhang, C. L. Zhang, C. Y. Tan, C. M. Gao, Z. Chen, Y. Y. Jiang and Y. Z. Chen, Sci. Rep., 2015, 5, 9325 CrossRef CAS PubMed.
- E. Patridge, P. Gareiss, M. S. Kinch and D. Hoyer, Drug Discovery Today, 2016, 21, 204–207 CrossRef CAS PubMed.
- D. J. Newman and G. M. Cragg, J. Nat. Prod., 2020, 27, 770–803 CrossRef PubMed.
- A. Mullard, Nat. Rev. Drug Discovery, 2018, 17, 81–86 CrossRef CAS PubMed.
- A. Mullard, Nat. Rev. Drug Discovery, 2019, 18, 85–89 CrossRef PubMed.
- J. L. Medina-Franco and F. I. Saldívar-González, Biomolecules, 2020, 10, 1566 CrossRef CAS PubMed.
- J. D. Romano and N. P. Tatonetti, Front. Genet., 2019, 10, 368 CrossRef CAS PubMed.
- Y. Chen and J. Kirchmair, Mol. Inform., 2020, 39, 2000171 CrossRef CAS PubMed.
- A. Mlinarić, M. Horvat and V. Šupak Smolčić, Biochem. Med., 2017, 27, 447–452 Search PubMed.
- K. Santana, L. D. do Nascimento, A. Lima e Lima, V. Damasceno, C. Nahum, R. C. Braga and J. Lameira, Front. Chem., 2021, 9, 155 Search PubMed.
- J. L. Medina-Franco, Front. Drug Discovery, 2021, 1, 728551 CrossRef.
- F. Bucar, A. Wube and M. Schmid, Nat. Prod. Rep., 2013, 30, 525–545 RSC.
- B. David, J.-L. Wolfender and D. A. Dias, Phytochem. Rev., 2015, 14, 299–315 CrossRef CAS.
- G. A. Cordell, Planta Med., 2011, 77, 1129–1138 CrossRef CAS PubMed.
- F. Ntie-Kang, J. N. Nwodo, A. Ibezim, C. V. Simoben, B. Karaman, V. F. Ngwa, W. Sippl, M. U. Adikwu and L. M. Mbaze, J. Chem. Inf. Model., 2014, 54, 2433–2450 CrossRef CAS PubMed.
- S. Derese, A. Ndakala, M. Rogo and J. Oyim, 2015.
- U. Consortium, Nucleic Acids Res., 2019, 47, D506–D515 CrossRef PubMed.
- U. Consortium, Nucleic Acids Res., 2015, 43, D204–D212 CrossRef PubMed.
- S. K. Burley, H. M. Berman, C. Bhikadiya, C. Bi, L. Chen, L. Di Costanzo, C. Christie, K. Dalenberg, J. M. Duarte and S. Dutta, Nucleic Acids Res., 2019, 47, D464–D474 CrossRef CAS PubMed.
- C. L. Schoch, S. Ciufo, M. Domrachev, C. L. Hotton, S. Kannan, R. Khovanskaya, D. Leipe, R. Mcveigh, K. O'Neill and B. Robbertse, Database, 2020, 2020 Search PubMed.
- J. A. Van Santen, G. Jacob, A. L. Singh, V. Aniebok, M. J. Balunas, D. Bunsko, F. C. Neto, L. Castaño-Espriu, C. Chang and T. N. Clark, ACS Cent. Sci., 2019, 5, 1824–1833 CrossRef CAS PubMed.
- J. A. van Santen, E. F. Poynton, D. Iskakova, E. McMann, T. A. Alsup, T. N. Clark, C. H. Fergusson, D. P. Fewer, A. H. Hughes, C. A. McCadden, J. Parra, S. Soldatou, J. D. Rudolf, E. M.-L. Janssen, K. R. Duncan and R. G. Linington, Nucleic Acids Res., 2021, 50, D1317–D1323 CrossRef PubMed.
- Y. Chen, M. Garcia de Lomana, N.-O. Friedrich and J. Kirchmair, J. Chem. Inf. Model., 2018, 58, 1518–1532 CrossRef CAS PubMed.
- A. C. Pilon, M. Valli, A. C. Dametto, M. E. F. Pinto, R. T. Freire, I. Castro-Gamboa, A. D. Andricopulo and V. S. Bolzani, Sci. Rep., 2017, 7, 1–12 CrossRef CAS PubMed.
- F. Ntie-Kang, D. Zofou, S. B. Babiaka, R. Meudom, M. Scharfe, L. L. Lifongo, J. A. Mbah, L. M. Mbaze, W. Sippl and S. M. Efange, PLoS One, 2013, 8, e78085 CrossRef CAS PubMed.
- A. G. Atanasov, S. B. Zotchev, V. M. Dirsch and C. T. Supuran, Nat. Rev. Drug Discovery, 2021, 20, 200–216 CrossRef CAS PubMed.
- E. C. Barnes, R. Kumar and R. A. Davis, Nat. Prod. Rep., 2016, 33, 372–381 RSC.
- P. A. Onguene, C. V. Simoben, G. W. Fotso, K. Andrae-Marobela, S. A. Khalid, B. T. Ngadjui, L. M. Mbaze and F. Ntie-Kang, Comput. Biol. Chem., 2018, 72, 136–149 CrossRef CAS PubMed.
- B. J. Huffman and R. A. Shenvi, J. Am. Chem. Soc., 2019, 141, 3332–3346 CrossRef CAS PubMed.
- N.-O. Friedrich, F. Flachsenberg, A. Meyder, K. Sommer, J. Kirchmair and M. Rarey, J. Chem. Inf. Model., 2019, 59, 731–742 CrossRef CAS PubMed.
- N.-O. Friedrich, A. Meyder, C. de Bruyn Kops, K. Sommer, F. Flachsenberg, M. Rarey and J. Kirchmair, J. Chem. Inf. Model., 2017, 57, 529–539 CrossRef CAS PubMed.
- N.-O. Friedrich, C. de Bruyn Kops, F. Flachsenberg, K. Sommer, M. Rarey and J. Kirchmair, J. Chem. Inf. Model., 2017, 57, 2719–2728 CrossRef CAS PubMed.
- M. Rowland and T. N. Tozer, Clinical pharmacokinetics/pharmacodynamics, Lippincott Williams and Wilkins, Philadelphia, 2005 Search PubMed.
- A. Najjar and R. Karaman, Expert Opin. Drug Delivery, 2019, 16, 1–5 CrossRef PubMed.
- K.-M. Wu, Pharmaceuticals, 2009, 2, 77–81 CrossRef CAS PubMed.
- G. R. Bickerton, G. V. Paolini, J. Besnard, S. Muresan and A. L. Hopkins, Nat. Chem., 2012, 4, 90–98 CrossRef CAS PubMed.
- C. A. Lipinski, F. Lombardo, B. W. Dominy and P. J. Feeney, Adv. Drug Delivery Rev., 1997, 23, 3–25 CrossRef CAS.
- J. J. Irwin, K. G. Tang, J. Young, C. Dandarchuluun, B. R. Wong, M. Khurelbaatar, Y. S. Moroz, J. Mayfield and R. A. Sayle, J. Chem. Inf. Model., 2020, 60, 6065–6073 CrossRef CAS PubMed.
- F. Ntie-Kang, SpringerPlus, 2013, 2, 353 CrossRef PubMed.
- D. Klementz, K. Döring, X. Lucas, K. K. Telukunta, A. Erxleben, D. Deubel, A. Erber, I. Santillana, O. S. Thomas and A. Bechthold, Nucleic Acids Res., 2016, 44, D509–D514 CrossRef CAS PubMed.
- D. F. Veber, S. R. Johnson, H.-Y. Cheng, B. R. Smith, K. W. Ward and K. D. Kopple, J. Med. Chem., 2002, 45, 2615–2623 CrossRef CAS PubMed.
- L. I. Pilkington, Molecules, 2019, 24, 3942 CrossRef CAS PubMed.
- L. Zhang, J. Song, L. Kong, T. Yuan, W. Li, W. Zhang, B. Hou, Y. Lu and G. Du, Pharmacol. Ther., 2020, 107686 CrossRef CAS PubMed.
- D. E. Pires, T. L. Blundell and D. B. Ascher, J. Med. Chem., 2015, 58, 4066–4072 CrossRef CAS PubMed.
- G. Xiong, Z. Wu, J. Yi, L. Fu, Z. Yang, C. Hsieh, M. Yin, X. Zeng, C. Wu and A. Lu, Nucleic Acids Res., 2021, 49, W5–W14 CrossRef CAS PubMed.
- Schrödinger, Release version 2017-2.
- A. Daina, O. Michielin and V. Zoete, Sci. Rep., 2017, 7, 42717 CrossRef PubMed.
- H. Yang, C. Lou, L. Sun, J. Li, Y. Cai, Z. Wang, W. Li, G. Liu and Y. Tang, Bioinformatics, 2019, 35, 1067–1069 CrossRef CAS PubMed.
- I. V. Tetko, P. Bruneau, H.-W. Mewes, D. C. Rohrer and G. I. Poda, Drug discovery today, 2006, 11, 700–707 CrossRef CAS PubMed.
- N. A. Durán-Iturbide, B. r. I. Díaz-Eufracio and J. L. Medina-Franco, ACS Omega, 2020, 5, 16076–16084 CrossRef PubMed.
- B. A. Pilón-Jiménez, F. I. Saldívar-González, B. I. Díaz-Eufracio and J. L. Medina-Franco, Biomolecules, 2019, 9, 31 CrossRef PubMed.
- C. Y.-C. Chen, PLoS One, 2011, 6, e15939 CrossRef CAS PubMed.
- D. S. Wishart, Y. D. Feunang, A. C. Guo, E. J. Lo, A. Marcu, J. R. Grant, T. Sajed, D. Johnson, C. Li and Z. Sayeeda, Nucleic Acids Res., 2018, 46, D1074–D1082 CrossRef CAS PubMed.
- D. Lagorce, D. Douguet, M. A. Miteva and B. O. Villoutreix, Sci. Rep., 2017, 7, 1–15 CrossRef PubMed.
- C. M. Labbé, M. A. Kuenemann, B. Zarzycka, G. Vriend, G. A. Nicolaes, D. Lagorce, M. A. Miteva, B. O. Villoutreix and O. Sperandio, Nucleic Acids Res., 2016, 44, D542–D547 CrossRef PubMed.
- A. P. Higueruelo, H. Jubb and T. L. Blundell, Database, 2013, 2013 Search PubMed.
- A. P. Bento, A. Gaulton, A. Hersey, L. J. Bellis, J. Chambers, M. Davies, F. A. Krüger, Y. Light, L. Mak and S. McGlinchey, Nucleic Acids Res., 2014, 42, D1083–D1090 CrossRef CAS PubMed.
- J. Li, A. F. Moumbock and S. Günther, J. Chem. Inf. Model., 2020, 60, 5225–5233 CrossRef CAS PubMed.
- D. Menche, ChemMedChem, 2021, 16, 2068–2074 CrossRef CAS PubMed.
- M. A. Bagley and A. K. Rai, Virginia Public Law and Legal Theory Research Paper, 2014 Search PubMed.
- F. Friso, F. Mendive, M. Soffiato, V. Bombardelli, A. Hesketh, M. Heinrich, L. Menghini and M. Politi, J. Ethnopharmacol., 2020, 259, 112885 CrossRef PubMed.
- G. Burton and E. A. Evans-Illidge, ACS Chem. Biol., 2014, 9, 588–591 CrossRef CAS PubMed.
- L. K. Caesar and N. B. Cech, Nat. Prod. Rep., 2019, 36, 869–888 RSC.
- N. Chams, S. Chams, R. Badran, A. Shams, A. Araji, M. Raad, S. Mukhopadhyay, E. Stroberg, E. J. Duval and L. M. Barton, Front. Public Health, 2020, 8, 383 CrossRef PubMed.
- Y. Hu, J. Sun, Z. Dai, H. Deng, X. Li, Q. Huang, Y. Wu, L. Sun and Y. Xu, J. Clin. Virol., 2020, 127, 104371 CrossRef CAS PubMed.
- G. Li and E. De Clercq, Nat. Rev. Drug Discovery, 2020, 19, 149–150 CrossRef CAS PubMed.
- H. Li, S.-M. Liu, X.-H. Yu, S.-L. Tang and C.-K. Tang, Int. J. Antimicrob. Agents, 2020, 55, 105951 CrossRef CAS PubMed.
- C. Valle, B. Martin, F. Touret, A. Shannon, B. Canard, J. C. Guillemot, B. Coutard and E. Decroly, Rev. Med. Virol., 2020, 30, 1–10 CrossRef CAS PubMed.
- M. Wang, R. Cao, L. Zhang, X. Yang, J. Liu, M. Xu, Z. Shi, Z. Hu, W. Zhong and G. Xiao, Cell Res., 2020, 30, 269–271 CrossRef CAS PubMed.
- C. G. Benítez-Cardoza and J. L. Vique-Sánchez, Comput. Biol. Med., 2021, 136, 104719 CrossRef PubMed.
- X. Li, J. F.-W. Chan, K. K.-W. Li, E. Y.-K. Tso, C. C.-Y. Yip, S. Sridhar, T. W.-H. Chung, K. H.-Y. Chiu, D. L.-L. Hung and A. K.-L. Wu, Infection, 2021, 49, 257–265 CrossRef CAS PubMed.
- A. Rajput, A. Thakur, A. Rastogi, S. Choudhury and M. Kumar, Comput. Biol. Med., 2021, 136, 104677 CrossRef CAS PubMed.
- J. A. Beutler, Curr. Protoc. Pharmacol., 2009, 46, 9.11.11–19.11.21 Search PubMed.
- C. V. Simoben, Dissertation, Martin-Luther-Universität Halle-Wittenberg, Halle (Saale), 2021, https://books.google.ca/books?id=4BxuzgEACAAJ.
- J. Melesina, L. Praetorius, C. V. Simoben, D. Robaa and W. Sippl, Future Med. Chem., 2018, 10, 1537–1540 CrossRef CAS PubMed.
- J. Melesina, C. V. Simoben, L. Praetorius, E. F. Bülbül, D. Robaa and W. Sippl, ChemMedChem, 2021, 16, 1336–1359 CrossRef CAS PubMed.
- M. Ouaissi and A. Ouaissi, J. Biomed. Biotechnol., 2006, 13474 Search PubMed.
- D. M. Margolis, Curr. Opin. HIV AIDS, 2011, 6, 25–29 CrossRef PubMed.
- T. A. McKinsey, Annu. Rev. Pharmacol., 2012, 52, 303–319 CrossRef CAS PubMed.
- G. S. Hailu, D. Robaa, M. Forgione, W. Sippl, D. Rotili and A. Mai, J. Med. Chem., 2017, 60, 4780–4804 CrossRef CAS PubMed.
- D. N. Divsalar, C. V. Simoben, C. Schonhofer, K. Richard, W. Sippl, F. Ntie-Kang and I. Tietjen, Front. Pharmacol., 2020, 11, 905 CrossRef CAS PubMed.
- T. Eckschlager, J. Plch, M. Stiborova and J. Hrabeta, Int. J. Mol. Sci., 2017, 18, 1414 CrossRef PubMed.
- R. L. Piekarz, R. Frye, M. Turner, J. J. Wright, S. L. Allen, M. H. Kirschbaum, J. Zain, H. M. Prince, J. P. Leonard, L. J. Geskin, C. Reeder, D. Joske, W. D. Figg, E. R. Gardner, S. M. Steinberg, E. S. Jaffe, M. Stetler-Stevenson, S. Lade, A. T. Fojo and S. E. Bates, J. Clin. Oncol., 2009, 27, 5410–5417 CrossRef CAS PubMed.
- P. M. Lombardi, K. E. Cole, D. P. Dowling and D. W. Christianson, Curr. Opin. Struct. Biol., 2011, 21, 735–743 CrossRef CAS PubMed.
- G. S. McKnight, L. Hager and R. D. Palmiter, Cell, 1980, 22, 469–477 CrossRef CAS PubMed.
- H. Itazaki, K. Nagashima, K. Sugita, H. Yoshida, Y. Kawamura, Y. Yasuda, K. Matsumoto, K. Ishii, N. Uotani and H. Nakai, J. Antibiot., 1990, 43, 1524–1532 CrossRef CAS PubMed.
- B. Orlikova, M. Schnekenburger, M. Zloh, F. Golais, M. Diederich and D. Tasdemir, Oncol. Rep., 2012, 28, 797–805 CrossRef CAS PubMed.
- A. Berger, S. Venturelli, M. Kallnischkies, A. Böcker, C. Busch, T. Weiland, S. Noor, C. Leischner, T. S. Weiss and U. M. Lauer, J. Nutr. Biochem., 2013, 24, 977–985 CrossRef CAS PubMed.
- H.-W. Ryu, D.-H. Lee, D.-H. Shin, S. H. Kim and S. H. Kwon, Planta Med., 2015, 81, 222–227 CrossRef CAS PubMed.
- H. Losson, M. Schnekenburger, M. Dicato and M. Diederich, Molecules, 2016, 21, 1608 CrossRef PubMed.
- K. N. Hassell, Diseases, 2019, 7, 57 CrossRef CAS PubMed.
- X. Qiu, L. Zhu, H. Wang, Y. Tan, Z. Yang, L. Yang and L. Wan, Bioorg. Med. Chem., 2021, 52, 116510 CrossRef CAS PubMed.
- B. Karaman, Z. Alhalabi, S. Swyter, S. O. Mihigo, K. Andrae-Marobela, M. Jung, W. Sippl and F. Ntie-Kang, Molecules, 2018, 23, 416 CrossRef PubMed.
- M. C. Haigis and D. A. Sinclair, Annu. Rev. Pathol., 2010, 5, 253 CrossRef CAS PubMed.
- A. M. Davenport, F. M. Huber and A. Hoelz, J. Mol. Biol., 2014, 426, 526–541 CrossRef CAS PubMed.
- J. Hu, H. Jing and H. Lin, Future Med. Chem., 2014, 6, 945–966 CrossRef CAS PubMed.
- T. F. Outeiro, E. Kontopoulos, S. M. Altmann, I. Kufareva, K. E. Strathearn, A. M. Amore, C. B. Volk, M. M. Maxwell, J.-C. Rochet and P. J. McLean, Science, 2007, 317, 516–519 CrossRef CAS PubMed.
- F. Ntie-Kang, P. Amoa Onguéné, G. W. Fotso, K. Andrae-Marobela, M. Bezabih, J. C. Ndom, B. T. Ngadjui, A. O. Ogundaini, B. M. Abegaz and L. M. Meva’a, PLoS One, 2014, 9, e90655 CrossRef PubMed.
- O. Heffernan, Nature, 2020, 580, 20–22 CrossRef PubMed.
- C. Harrison, Nat. Biotechnol., 2014, 32, 403–405 CrossRef CAS PubMed.
Footnote |
† These authors have contributed equally to this work. |
|
This journal is © The Royal Society of Chemistry 2023 |
Click here to see how this site uses Cookies. View our privacy policy here.