DOI:
10.1039/D0RA08304F
(Paper)
RSC Adv., 2020,
10, 40244-40263
Antiviral agents against COVID-19: structure-based design of specific peptidomimetic inhibitors of SARS-CoV-2 main protease
Received
28th September 2020
, Accepted 30th October 2020
First published on 4th November 2020
Abstract
Despite the intense development of vaccines and antiviral therapeutics, no specific treatment of coronavirus disease 2019 (COVID-19), caused by the new severe acute respiratory syndrome coronavirus 2 (SARS-CoV-2), is currently available. Recently, X-ray crystallographic structures of a validated pharmacological target of SARS-CoV-2, the main protease (Mpro also called 3CLpro) in complex with peptide-like irreversible inhibitors have been published. We have carried out computer-aided structure-based design and optimization of peptidomimetic irreversible α-ketoamide Mpro inhibitors and their analogues using MM, MD and QM/MM methodology, with the goal to propose lead compounds with improved binding affinity to SARS-CoV-2 Mpro, enhanced specificity for pathogenic coronaviruses, decreased peptidic character, and favourable drug-like properties. The best inhibitor candidates designed in this work show largely improved interaction energies towards the Mpro and enhanced specificity due to 6 additional hydrogen bonds to the active site residues. The presented results on new SARS-CoV-2 Mpro inhibitors are expected to stimulate further research towards the development of specific anti-COVID-19 drugs.
Introduction
The outbreak of the new severe acute respiratory syndrome coronavirus 2 (SARS-CoV-2) belonging to the b-lineage of the betacoronaviridae family, which causes severe viral pneumonia in humans known as coronavirus disease 2019 (COVID-19), commenced in Wuhan, China in December 2019 and spread widely in 2020.1,2 Although intense research and development of vaccines and antiviral therapeutics is ongoing worldwide, at present only one intravenous broad-spectrum antiviral medication has been approved for treatment of COVID-19.3 SARS-CoV-2 is a positive-sense single-stranded enveloped RNA virus containing an RNA sequence of approx. 30 thousand bases. This unusually large viral genome codes for 4 structural proteins: spike, envelope and membrane proteins creating the viral envelope, and nucleocapsid protein holding the RNA genome, and 16 non-structural proteins (nsp1–nsp16) forming the replication–transcription complex of SARS-CoV-2.4,5 These proteins are expressed in the form of two polypeptides: pp1ab and pp1a, which are then processed by virally encoded chymotrypsin-like protease (called main protease Mpro or also 3CLpro), and papain-like protease (PLpro). The 33.8 kDa cysteine protease Mpro (EC 3.4.22.69) encoded in the nsp5 is a key viral enzyme essential for the viral life cycle of coronaviruses. The Mpro digests the larger polyprotein pp1ab (∼790 kDa) at 11 or more conserved sites with recognition sequences P3[Val, hydrophob., cationic] − P2[Leu, hydrophob., aromat.] − P1[Gln, His] + P1′[Ser, Ala, Gly] starting with an autocatalytic cleavage, to produce the functional nsps.6–8 In absence of closely related homologues in humans or proteases sharing similar cleavage site specificity, the Mpro forms an attractive pharmacological target for antiviral drug discovery.5,9 Recently, X-ray crystallographic structures of the Mpro of SARS-CoV-2 in complex with peptide-like irreversible inhibitors – Michael acceptor N310,11 and α-ketoamide 13b,12 were resolved (PDB entries 6LU7 and 6Y2F),12–14 Fig. 1. The N3 and 13b are potent covalent inhibitors of the SARS-CoV (2003) Mpro that act through a two-step irreversible inactivation mechanism. The inhibitor first associates with the Mpro to form enzyme-inhibitor complex (E⋯I) with an equilibrium binding constant. Then, a stable covalent bond is formed between the inhibitors and Mpro via nucleophilic attack of the catalytic cysteine upon the vinyl group of N3 or α-ketoamide group of 13b (Fig. 1), characterized by high rate constant of Mpro inactivation resulting in a thiohemiketal formation.10,14 The N3 shows notable structural similarity to rupintrivir (AG-7088), a peptidomimetic antiviral drug which inhibits 3CLpro proteases of rhinoviruses and is investigated also for treatment of infections caused by picornaviruses, norovirus, and coronaviruses, such as SARS.4,15–17
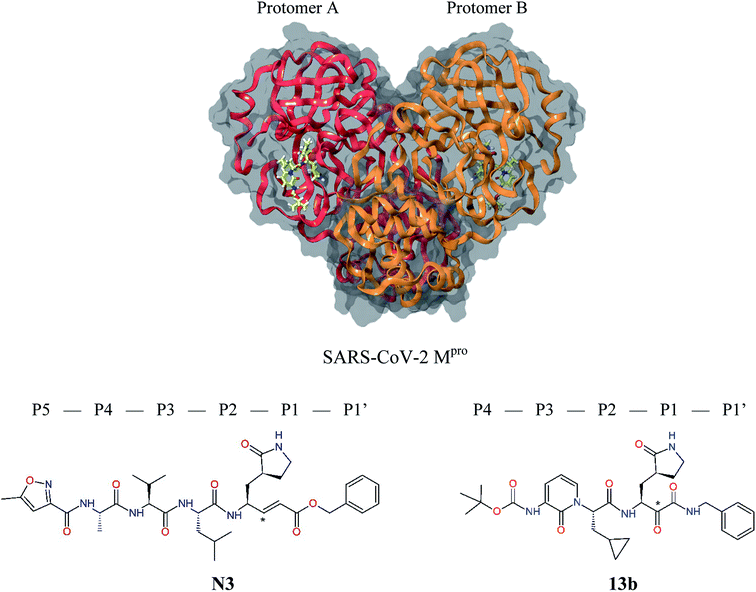 |
| Fig. 1 Above: ribbon representation of 3D-structure of SARS-CoV-2 Mpro homodimer (PDB ID 6Y2F)12 with inhibitor 13b (stick model, H – white, C – yellow, N – blue, O – red) bound at the active sites of the protomers A and B. Partially transparent molecular surface is shown in grey colour. Below: chemical structures of peptidomimetic covalent inhibitors N3 and 13b12,14 and corresponding standard notation of protease substrate residues. Stars (*) indicate the sites of nucleophilic attack of anionic sulphur of cysteine of the catalytic dyad His41 – Cys145 of the cysteine protease on the trans-α,β-unsaturated benzyl ester of the Michael acceptor N314 or the α-ketoamide group of 13b,12 leading to thiohemiketal linkage formation with the Cys145.9 | |
The 3D structure of SARS-CoV-2 Mpro forms, like the main proteases of other coronaviruses, a C2-symmetric dimer of two protomers A and B, each composed of three domains. Domains I and II (residues 8–184) contain six-stranded antiparallel β-barrels that adopt the chymotrypsin fold, while domain III (residues 201–306) forms α-helical structure connected to domain II by a long linking loop (residues 185–200).5,9,10,12–14 Active site of the Mpro containing Cys–His catalytic dyad, in which the Cys145 behaves as the nucleophile, while the His41 acts as the general acid/base. The substrate binding pocket is in a shallow cleft between domains I and II bordered by residues 164–168 of a long β-strand on one side and the linking loop residues 189–191 on the other side. The N-terminal part of each monomer (N-finger, residues 1–7) takes part in the Mpro dimerization and formation of the active site of the other monomer by interacting with Glu166, a residue important for the substrate recognition and inhibitor binding.9,10,12,18 The subsite S1 of the Mpro confers almost absolute specificity for the Gln residues of substrate. The binding site of Mpro is highly conserved among all coronavirus species suggesting that inhibitors targeting this site should display broad-spectrum anti-coronavirus activity.12,14 Therefore, the binding site of Mpro is likely to remain constant and less susceptible to drug-resistance conferring mutations also in the progeny strains of the SARS-CoV-2 virus of the 2019/20 season.
Since the emergence of SARS in 2003 and identification of coronavirus as the causative agent of the disease, a considerable number of peptidomimetic and small-molecule inhibitors of the Mpro were developed. These antiviral compounds comprise also covalent inhibitors using reactive warhead groups, which include Michael acceptors, aldehydes, epoxy ketones, electrophilic ketones such as halomethyl ketones, trifluoromethyl ketones and α-ketoamides.9,13,18–23 Unfortunately, due to reactivity, potential for toxicity and undesired side-effects, rapid in vivo metabolism and reduced oral bioavailability, the irreversible inhibitors are less likely to make efficient therapeutic agents.9,14 In fact, till present, there is no effective antiviral therapy for the treatment of SARS, MERS, and COVID-19 in humans.12,24,25 The price for lacking chemotherapeutics for coronaviruses in terms of lost human lives is too high and can be even higher in the future.
Due to the extent and death toll of the present SARS-CoV-2 pandemic and limited therapeutic options, it is rather urgent to develop improved reversible or irreversible Mpro inhibitors as potential antiviral agents for the treatment of COVID-19. The structure of SARS-CoV-2 Mpro in complex with N3,14 13b12 and series of α-ketoamide inhibitors 11a–11u23 provided a solid ground for design and discovery of new coronavirus inhibitors. We have performed computer-assisted structure-based design and optimization of peptidomimetic α-ketoamide Mpro inhibitors validated by a QSAR model starting from compounds published in ref. 10, 12 and 23 and their reversible analogues with the aim to propose antiviral lead compounds with improved specificity and binding affinity to SARS-CoV-2 Mpro, decreased peptidic character and favourable drug-like properties. The inhibitor design employed molecular mechanics (MM), conformational searching, validation by a QSAR model, molecular dynamics (MD), and was also supported by rigorous quantum chemistry method (QM/MM).
Methods
Receptor preparation
The 3D-structures of the SARS-CoV-2 Mpro in complex with covalent inhibitors N3 and 13b were obtained from recently published crystallographic data stored in the Protein Data Bank26 (PDB ID: 6LU7 and 6Y2F).12,14 In these complexes, the electrophilic warheads – unsaturated benzyl ester of the Michael-acceptor N3 and α-ketoamide of 13b (Fig. 1) were covalently linked to the catalytic residue Cys145.10–12 These covalent bonds were removed. Protonation and tautomeric states at physiological pH of amino acids of the Mpro and of inhibitors were assigned according to predicted pKa values computed by Epik.27,28 All crystallographic water molecules and other crystallization additives were removed. The complexes were refined by molecular mechanics (MM) energy minimization to a minimum on the potential energy landscape by employing Polak–Ribière conjugate gradient method with convergence criterion set to energy gradient of 0.01 kJ mol−1 Å−1. During the geometry optimization an extended cut-off distance of 20 Å was used for electrostatic interactions. The OPLS3e force field, which is suitable for simulations of small molecules and proteins,29 together with implicit GB/SA solvent model (water),30 were employed throughout the minimization in MacroModel (Schrödinger, LLC.).31 Complexes of SARS-CoV-2 Mpro with other known or new inhibitors were prepared by in situ modification of the ligands N3 and 13b in the relaxed consensus structure of the Mpro-N3 and Mpro-13b complexes (6Y2F12 and 6LU7,14 rmsd = 1.79 Å for 26 active site residues, 420 atoms), by changing the side chains or backbone atoms of P3 to P1′ residues into novel molecular fragments. Initial conformations of the built-in function groups were selected according to location of energy minima on the conformational maps for rotations over rotatable bonds of the side chains, which were explored by dihedral angle coordinate scans of MacroModel,31 as illustrated in ref. 35–38. Total MM energy of the reference unliganded SARS-CoV-2 Mpro was obtained by relaxation of the proteases after removing the inhibitor by energy-minimization as the lower energy state of the two Mpro structures. Total energies of free inhibitors in solution was determined by conformational searching using mixed torsional/low-mode sampling method, which combines random changes in torsion angles and/or molecular position with exploring low-vibrational-frequency eigenvectors of the system that are related to ‘soft’ degrees of freedom, such as the variable torsion angles,39 followed by energy minimization of generated conformers.31
QSAR model
To prepare a QSAR model of inhibition of the coronavirus Mpro by peptidomimetics we have considered a series of eleven α-ketoamides 11a–11u23 with consistent experimental inhibition potencies IC50exp determined for the SARS-CoV Mpro (2003).23 The Mpro of SARS-CoV-2 (2019/20) and SARS-CoV (2003) share very high degree of sequence identity. Crystal structure of the (2003) SARS-CoV Mpro-11a complex (PDB ID 5N19 (ref. 40)) was used to model the complexes of the training set inhibitors.23 The P2 residue side chain conformations of the peptidomimetic inhibitors were modelled by in situ modification of the ligand of the Mpro-11a complex combined with a 360-degree conformational search over the torsion angles of Cα–Cβ and Cβ–Cγ bonds with an increment of 10 degrees using the coordinate scan of MacroModel.31 The correlation between computed relative interaction energies ΔΔEint,MM of the series 11a–11u23 and experimental inhibition potencies IC50exp was obtained by linear regression and outliers removal. Due to limited number of the α-ketoamide inhibitors with IC50exp data splitting of the series into training set and test set was not appropriate.
Molecular dynamics simulations
Conformational stability of the modelled ligands and interactions at the active site of the Mpro-inhibitor complexes were tested by molecular dynamics (MD) simulations. We have carried out 200 ns long simulations of the solvated Mpro-inhibitor complexes in the NPT statistical ensemble (300 K, 1 bar) by using Desmond.32 A periodic box with 10 Å buffer containing the Mpro-inhibitor complex was filled with approx. 11
000 TIP3P water molecules and neutralized by adding four Na+ ions to reach the electro-neutrality. During the simulation, OPLS3e force field, 1.5 fs integration step, and coulombic interaction cut-off of 14 Å, were used. The Nose–Hoover chains thermostat and Martyna–Tobias–Klein barostat methods were employed during the simulations.33,34 After initial heating and relaxation, the productive simulation trajectory was recorder and analysed for ligand-receptor interactions every 400 ps.
Interaction energy calculations
The MM interaction energies of inhibitors to Mpro were calculated by the supermolecular approach (for details see the footnote of Table 1).35–38 In addition to MM calculations, we have computed the inhibitor binding energies by hybrid quantum mechanical/molecular mechanical (QM/MM) approach using the density functional theory by employing QSite41,42 and Jaguar.43–45 In the Mpro-inhibitor complexes, the quantum region of the QM/MM calculation included the inhibitor and selected polar active site residues forming hydrogen bonds (HBs) or polar interactions with the ligands. The quantum region included the ligand (82 to 99 atoms) plus active site residues: Gln19sc, Thr26·, His41sc, Asn142–Gly143–Ser144–Cys145, His163sc, ·Glu166·, His172sc, Gln189–Thr190· (155 atoms of the protease, sc – side chain only, (·) – an additional bond along the backbone was included into the quantum region, total charge q = −1e). The individual residues or groups were terminated by H-caps and electrostatic interactions of atoms in the MM region neighbouring the H-caps with the quantum motif were represented by a Gaussian grid.44 Full geometry optimizations of the enzyme – inhibitor systems were done using DFT-M06-2X/6-311++G(d,p)//MM-OPLS-2005 level of theory using electronic embedding and analytic gradients with default convergence criteria and extended electrostatic interaction cut-off (20 Å).44 The embedding consists of coulombic interaction (OPLS-2005 point charges of the MM region within cut-off distance explicitly contribute to one-electron part of the QM Hamiltonian) and van der Waals interaction between the QM and MM regions (OPLS-2005 force field parameters are used for atoms of both regions).44 The effect of solvent was included by means of implicit solvation model as a single point calculation for the optimized geometry DFT-M06-2X/6-311++G(d,p)//MM-OPLS-2005-PBF (water). The meta-GGA global exchange-correlation density functional M06-2X with Hartree–Fock exchange is widely employed in computational chemistry for calculating energy-related quantities, and was recommended for describing thermochemistry, kinetics and non-covalent interactions by its developers.46,47 The flexible split-valence triple-zeta basis set with additional polarization and diffuse functions on all atoms 6-311++G(d,p)48 was previously found to lead to satisfactory predicted molecular geometries.49 In the QM/MM calculations the OPLS-2005 force field was employed,41,42,50 since until recently it was the only force field available in QSite with parameterization suitable for describing the interface between quantum and classical regions.44 The Poisson–Boltzmann continuum solvation model (PBF)51 that uses finite-element method on a high-resolution grid was employed to mimic the effect of the physiological aqueous environment on the molecular structure and ligand-receptor binding.45 The disadvantage of the QM/MM calculations using larger basis sets and extensive quantum regions, such as inhibitor bound to enzyme active site, is in rather high computational costs.†
Table 1 Comparison of computed relative MM interaction energies of known inhibitors to the SARS-CoV-2 Mpro deriving from crystal structures 6Y2F and 6LU7 (ref. 12 and 14)
Inhibitor |
Formula: P5–P4–P3–P2–P1–P1′ |
ΔΔEint,MMa [kcal mol−1] |
Mwb [g mol−1] |
IC50expc SARS-CoV (2003) Mpro [μM] |
IC50expd SARS-CoV-2 (2019/20) Mpro [μM] |
Relative interaction energy taken with respect to the reference inhibitor 13b was calculated by molecular mechanics (MM-OPLS3e) in solution: ΔΔEint,MM = ΔEint,MM(Ix) − ΔEint,MM(13b) = [Etot,MM{Mpro–Ix}aq − Etot,MM{Mpro}aq − Etot,MM{Ix}aq] − ΔEint,MM(13b), where Etot,MM is total energy of solvated enzyme-inhibitor complex {Mpro–Ix}aq, solvated enzyme {Mpro}aq, or solvated inhibitor {Ix}aq.35–38 The relative interaction energy ΔΔEint,MM describes changes in bonding and non-bonding components of potential energy of the enzyme and inhibitor upon the enzyme-inhibitor complex formation. Molar mass. Half-maximal inhibitory concentration determined in enzyme-inhibition assay for the Mpro of SARS-CoV from the 2003 outbreak.14,23 Half-maximal inhibitory concentration determined in enzyme-inhibition assay for the Mpro of SARS-CoV-2 from the 2019/20 outbreak.12 Interaction energy of irreversible Michael acceptor or α-ketoamide inhibitors was computed after breaking the covalent bond of the P1 residue to the catalytic Cys145. Taken from ref. 12. Taken from ref. 14. Taken from ref. 23. The 13b was used as the reference inhibitor in all calculations of the relative interaction energy ΔΔEint,MM. |
13ae,f |
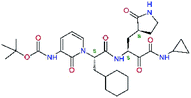 |
4.4 |
583.7 |
— |
2.39 |
13bf |
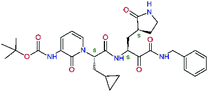 |
0.0i |
591.7 |
0.90 |
0.67 |
N3g |
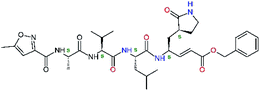 |
−4.1 |
680.8 |
— |
— |
11nh |
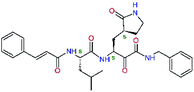 |
7.6 |
532.6 |
0.33 |
— |
11rf,h |
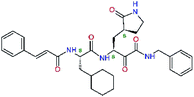 |
5.6 |
572.7 |
0.71 |
0.18 |
Results and discussion
Inhibitor design
Recently, the Hilgenfeld laboratory described a series of peptidomimetic α-ketoamides as broad-spectrum covalent inhibitors of coronavirus and enterovirus replication23 and proposed some modifications directed against the main protease of the SARS-CoV-2 (2019/20).12 Based on their work, we decided to carry on the inhibitor optimization, explore a wider section of the chemical space of covalent and non-covalent tight-binding peptidomimetic inhibitors, and propose new potent lead compounds specific for the SARS-CoV-2 Mpro by means of computer-aided drug design. The optimization procedure departed from the crystal structures of SARS-CoV-2 Mpro in complex with the Michael-acceptor N3 (PDB ID 6LU7),14 and α-ketoamide 13b (PDB ID 6Y2F),12 which were recently made available in the Protein Data Bank.26 Both inhibitors N3 and 13b are bound at the active site of the Mpro in predominantly extended conformations,11,12,14 it was therefore possible to model the binding of peptidomimetic inhibitors, which share some of P1 to P3 residues with the N3 or 13b, by in situ modification of the Mpro-inhibitor crystal structures.12,14 To determine a quantity related to the binding affinity of inhibitors to the Mpro, we have computed relative interaction energies (ΔΔEint,MM) of known and designed inhibitors using molecular mechanics (MM) and hybrid QM/MM approach (ΔΔEint,QM/MM, see the Methods section). The MM interaction energy guided us in the search for more potent Mpro inhibitors generated by structure-based design. Table 1 gives computed ΔΔEint,MM of known Mpro inhibitors together with their experimental half-maximal inhibitory concentrations (IC50exp) determined in enzyme inhibition assays on the SARS-CoV Mpro (2003) and the SARS-CoV-2 Mpro (2019/20).12,14,23 All inhibitors shown in Table 1 share the γ-lactam derivative of glutamine as the P1 residue, which was found to enhance the inhibitory potency compared to the flexible glutamine side chain, most probably due to reduction of entropy loss upon binding to the Mpro.23,52,53 The largest inhibitor N3 containing unsaturated benzyl ester electrophilic linkage, which occupies binding site specificity pockets S5 to S1′ and satisfies the known cleavage site preference of SARS-CoV Mpro for P1 to P3, displayed the strongest computed interaction energy. Unfortunately, the IC50exp value for the N3 inhibition of SARS-CoV-2 Mpro is not known.14 On the other hand, the IC50exp values of the α-ketoamide inhibitors 11r23 and 13b12 against the Mpro of SARS-CoV (2003) and SARS-CoV-2 (2019/20), are available and fall into submicromolar concentration range (Table 1).12 As it can be expected from the 96% sequence identity and similar 3D-structures of both viral proteases the IC50exp of these two viral strains are rather similar.
QSAR model of Mpro inhibition
Experimental activities of the SARS-CoV-2 Mpro inhibition are available only for a couple of compounds (Table 1,12,14) and do not permit direct validation of our computational design approach by comparison of computed ΔΔEint,MM with the observed potencies IC50exp. However, a training set of eleven peptidomimetic α-ketoamide inhibitors 11a–11u were recently assayed for the Mpro of SARS-CoV (2003) inhibition.23 The 306 residues of Mpro of SARS-CoV (2003) display a 96% overall sequence identity to the Mpro of SARS-CoV-2 (2019/20). Moreover, for the 32 residues located within the distance of 5 Å form the bound inhibitor 13b (6Y2F),12 forming the core of the Mpro active site, the sequence identity increased to 97% (31 out of 32 residues) with a single conservative residue replacement Ser46Ala. Thus, we have validated the design approach and computed Mpro-inhibitor relative interaction energies ΔΔEint,MM by preparing a QSAR model of the SARS-CoV (2003) Mpro inhibition (PDB entry 5N19,40), Table 2 and Fig. 2. The structural variability of the training set is restricted to the P2 residue only, consequently, the range of observed activities of this set of inhibitors is relatively narrow (3 orders of magnitude). Nevertheless, in the absence of other consistent sets of activity data of peptidomimetic inhibitors of SASR-CoV-2 Mpro in the literature, this series can be used for the validation of the computational approach.
Table 2 Computed relative interaction energies and observed half-maximal inhibition concentrations of a training set of peptidomimetic α-ketoamide inhibitors of Mpro of SARS-CoV (2003)23,a
Inhibitor |
Formula: P3–P2–P1–P1′ |
ΔΔEint,MMa [kcal mol−1] |
IC50expc SARS-CoV Mpro [μM] |
−log10 IC50exp |
a,cSee the footnote of Table 1. Reference inhibitor 11a. Outliers are marked by purple colour. |
11a |
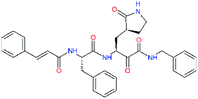 |
0.0 |
1.95 ± 0.24 |
−0.290 |
11f |
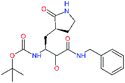 |
8.9 |
>50 |
−1.699 |
11m |
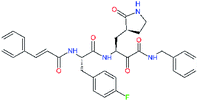 |
−1.9 |
>50 |
−1.699 |
11n |
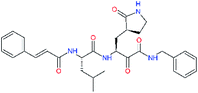 |
−1.7 |
0.33 ± 0.04 |
0.481 |
11o |
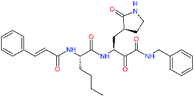 |
−0.3 |
8.50 ± 3.71 |
−0.929 |
11p |
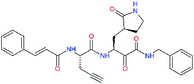 |
−1.1 |
10.68 ± 7.34 |
−1.028 |
11q |
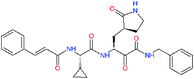 |
4.8 |
6.27 ± 2.87 |
−0.797 |
11r |
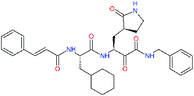 |
−0.2 |
0.71 ± 0.36 |
0.149 |
11s |
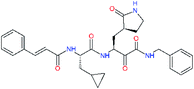 |
6.3 |
0.24 ± 0.08 |
0.619 |
11t |
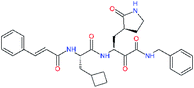 |
0.2 |
1.44 ± 0.40 |
−0.158 |
11u |
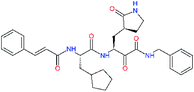 |
−1.7 |
1.27 ± 0.34 |
−0.104 |
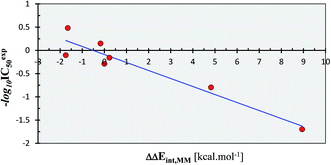 |
| Fig. 2 QSAR model of SARS-CoV (2003) Mpro inhibition by a training set of peptidomimetic α-ketoamide inhibitors23 (Table 2). Plot of correlation equation: −log10 IC50exp = −0.1723 × ΔΔEint,MM − 0.0890 obtained by linear regression. Number of compounds: n = 11, number of removed outliers: no = 4, squared regression coefficient: R2 = 0.91, leave-one-out cross-validated squared regression coefficient: Rxv2 = 0.87, statistical significance of the regression (Fisher F-test): F = 48.62, standard error: se = 0.23, level of statistical significance: α > 95%. | |
As we can see from the statistical parameters of the correlation (Fig. 2), the computed MM relative interaction energy in solution ΔΔEint,MM can fairly well reproduce the observed differences in the inhibitory activities of the peptidomimetic α-ketoamides towards the Mpro. This correlation explained about 91% of the IC50exp data variation and underlined the role of the enthalpic contribution to the binding affinity of the peptidomimetic inhibitors (entropic effects of the ligand binding were not considered in this correlation). Therefore, we believe that the structural model and computational procedure can be applied to predict binding of similar peptidomimetics also to the Mpro of SARS-CoV-2 (2019/20).
Structure-based inhibitor optimization
We have modelled the potent α-ketoamide inhibitor 11n23 at the binding site of the SARS-CoV-2 Mpro,12,14 and optimized the P1 residue that fills the specificity pocket S1 of the protease. The S1 subsite is formed by the side chains of residues Phe140, Asn142, Glu166, His163, and His172 of protomer A and in part also by the side chain of Ser1 of the other protomer B. In addition, main chain atoms of Phe140 and Leu141 also contribute to the S1 subsite formation. This polar pocket offers the potential for more than 2 hydrogen bonds (HB) formed by the glutamine side chain. Therefore, we have explored the replacement of Glu side chain by γ-lactam, unsaturated γ-lactams, and hydantoin moieties, Table 3. When comparing the ΔΔEint,MM values (representing here the approximate binding affinity of the ligands to Mpro) of the inhibitor candidates C1 to C4, the hydantoin heterocyclic moiety improved the predicted binding by more than 5 kcal mol−1. The reason for this enhancement is that in the relaxed structure of the Mpro-C4 complex the P1 residue penetrates deeper into the S1 pocket compared to N3 or 13b and forms four HBs with the main-chain of Phe140 and side chains of Asn142, His163, and His172. The main-chain carbonyl group of the P1 residue makes 3 HBs with the backbone NH groups of Gly143, Ser144, and Cys145, which create the canonical “oxyanion hole” of the Mpro cysteine protease.12,14 Therefore, we retained the glutamine hydantoin residue as the best building block in the P1 position of all following inhibitor candidates.
Table 3 Optimization of P1 residue of new candidates for the SARS-CoV-2 Mpro α-ketoamide inhibitorsa
Compound |
Formula: P3–P2–P1–P1′ |
ΔΔEint,MMa [kcal mol−1] |
Mwb [g mol−1] |
a,bSee the footnote of Table 1. |
C1 |
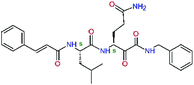 |
6.6 |
506.6 |
11n |
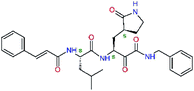 |
7.6 |
532.6 |
C2 |
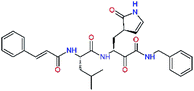 |
3.5 |
530.6 |
C3 |
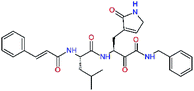 |
5.2 |
530.6 |
C4 |
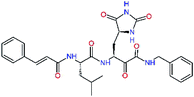 |
1.3 |
547.6 |
The substrate selectivity of the S2 pocket of the active site of coronaviruses Mpro for leucine was determined previously.6,7 Recently, the substrate recognition and cleavage site preference of SARS-CoV-2 Mpro were probed by a library of fluorogenic substrates, with glutamine in the P1 position, containing natural and a large panel of unnatural amino acids.8 Rut et al.8 concluded that the most preferred amino acid at the P2 position of SARS-CoV-2 Mpro is leucine. In addition, the S2 pocket can accommodate also other larger hydrophobic residues, such as 2-Abz, Phe(4-NO2), 3-Abz, β-Ala, Dht, hLeu, Met, and Ile (see table S1 of ref. 8 for the chemical structures of these unnatural a.u.). However, the substrate library of Rut et al.8 did not contain any unusual amino acids with bulkier and branched aliphatic side chains, as such chemicals are commercially not available. We have tested the preference of the S2 subsite of the SARS-CoV-2 Mpro for further branched or cyclic aliphatic side chains larger than that of leucine. The reason for selecting bulkier P2 residues was based on the 3D structure of inhibitor-Mpro complexes, which indicated that larger and branched side chains could be accommodated in the deeper S2 pocket and could extend also into the neighbouring larger hydrophobic S1′ subsite thus anchoring the inhibitor in the substrate binding pocket. The computed the ΔΔEint,MM values of inhibitor candidates C4–C10 (Table 4) suggest that besides leucine and isoleucine the P2 residue can almost equally well be composed of larger branched side chains, e.g. such as 4-isoheptane (C9), that is housed both by the S2 and S1′ subsites, Table 4 and Fig. 3. We have selected the C9 as the inhibitor with optimal P2 residue and retained it throughout optimization of the remaining residues of new inhibitors. We may assume that the larger and branched structure of the side chain of P2 residue could enhance the specificity for the SARS-CoV-2 Mpro over main proteases of other coronaviruses and enteroviruses which universally prefer Leu at the P2 position of their substrates.
Table 4 Optimization of P2 residue of new candidates for the SARS-CoV-2 Mpro α-ketoamide inhibitorsa
Compound |
Formula: P3–P2–P1–P1′ |
ΔΔEint,MMa [kcal mol−1] |
Mwb [g mol−1] |
a,bSee the footnote of Table 1. |
C4 |
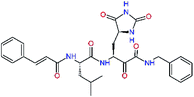 |
1.3 |
547.6 |
C5 |
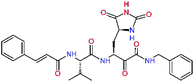 |
1.8 |
533.6 |
C6 |
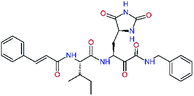 |
0.5 |
547.6 |
C7 |
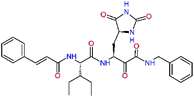 |
3.5 |
561.6 |
C8 |
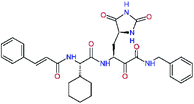 |
3.7 |
573.6 |
C9 |
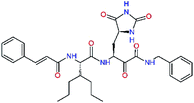 |
0.7 |
589.7 |
C10 |
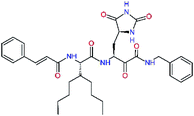 |
1.4 |
617.7 |
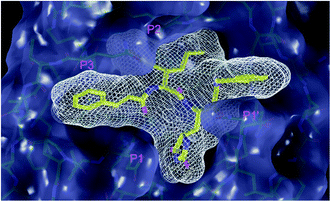 |
| Fig. 3 Partially transparent molecular surface of the SARS-CoV-2 Mpro binding site with bound inhibitor candidate C9 in stick representation (yellow – carbon, blue – nitrogen, red – oxygen, hydrogen atoms are not shown) and enclosed by a ligand surface (white mesh). The branched and bulky side chain of the P2 residue of C9 is harboured by the S2 pocket lined with residues His41, Met49, Tyr54, Met165 and Asp187 and also by S1′ pocket formed by residues Leu27, His41, Val42, and Cys145. The N-benzylformamide group in P1′ position of C9 partially sticks out of the S1′ into the solvent. | |
The S3 subsite of the Mpro is solvent-exposed, which suggests that this site can tolerate a wider range of functional groups.12,14 To diminish unfavourable entropic effects associated with the ligand binding we decided to reduce the linker length and flexibility of the flanking P3 residue. In addition, we have extended the solvent exposed surface area of the P3 moiety by introducing rigid condensed aromatic systems that contribute to the inhibitor binding by an elevated hydrophobic effect. Moreover, we have increased the contribution of the P3 to the overall binding affinity by adding specific HB interactions to the binding site residues that occupy the S3 and S4 subsites. The S4 subsite is composed of Met165, Leu167, Pro168, Gln182, and Gln189, which can enter polar as well as nonpolar interactions with the ligand. Thus, we have introduced a hydroxyl group making a proton-donor HB to the backbone carbonyl of Thr190 (C11, Table 5). Next, we have considered introducing a larger heterocyclic moiety into the P3 position to decrease flexibility and peptidic character of the P3 residue and rise the stability of the inhibitor structure. The 1,4-dihydroquinoxaline at the P3 position brought an additional proton-donor HB to backbone carbonyl of Glu166 (C12). Other condensed aromatic moieties included quinoxalin-1(4H)-ol (C14), quinolin-4(1H)-one (C15), 4H-1,4-benzoxazine (C16), and 4H-1,4-benzothiazine (C17). Various heterocycles were considered for the P3 residues with the goal to gain an additional HB of the heteroatoms or function groups to the side chain of Gln189. In addition, the substituted fused ring of the P3 residue of C12 to C17 contribute to the ligand binding by a lone pair – π interaction with the nitrogen of Pro168.54,55 Out of the inhibitor candidates with modified P3 residue the C17 appeared as the most perspective one displaying binding affinity enhancement by more than 4 kcal mol−1 compared to C9 (Table 4), and increased specificity of binding by 2 additional HBs to the residues of the Mpro active site. Interestingly, the hydroxylamine function group of quinoxaline in C14 showed to be less favourable than the thioether group of benzothiazine in C17 probably due to its elevated hydrophobic character.
Table 5 Optimization of P3 residue of new candidates for the SARS-CoV-2 Mpro α-ketoamide inhibitorsa
Compound |
Formula: P3–P2–P1–P1′ |
ΔΔEint,MMa [kcal mol−1] |
Mwb [g mol−1] |
a,bSee the footnote of Table 1. |
C9 |
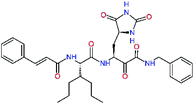 |
0.7 |
589.7 |
C11 |
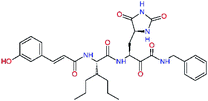 |
1.1 |
605.7 |
C12 |
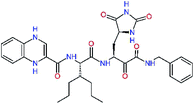 |
0.0 |
617.7 |
C13 |
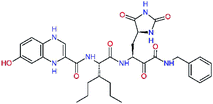 |
−0.9 |
633.7 |
C14 |
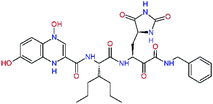 |
−2.8 |
649.7 |
C15 |
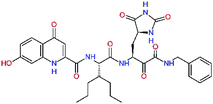 |
−0.5 |
646.7 |
C16 |
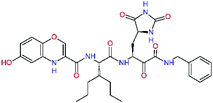 |
−0.7 |
634.7 |
C17 |
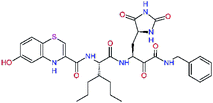 |
−3.6 |
650.7 |
The S1′ site of SARS-CoV-2 Mpro (2019/20) is formed chiefly by hydrophobic residues Leu27, His41, Val42, and Cys145 and the P1′ benzyl ester portion of the inhibitor N3 is protruding out of the S1′ into the solvent.14 Alike the P3 residue optimization strategy, the improvement of the P1′ building block involved shortening of the linkage, introducing condensed aromatic system that could partially occupy the hydrophobic S1′ subsite, and adding new function groups able to interact with Gln19 located at the edge of the S1′ pocket (C17–C23, Table 5). Naphthalene diol of C20, which forms a proton-donor HBs to the side chain of Gln19 appeared as the most suitable replacement of the P1′ phenylmethanamine of the C17. This modification enhanced the binding affinity of C20 to the Mpro by over 11 kcal mol−1 compared to C17 (Table 6). Other benzenediol, naphthalenediol, phenol, or hydroxynaphthalene groups in C17–C23 proved to be less effective.
Table 6 Optimization of P1′ residue of new candidates for the SARS-CoV-2 Mpro α-ketoamide inhibitorsa
Compound |
Formula: P3–P2–P1–P1′ |
ΔΔEint,MMa [kcal mol−1] |
Mwb [g mol−1] |
a,bSee the footnote of Table 1. |
C17 |
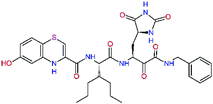 |
−3.6 |
650.7 |
C18 |
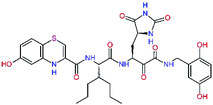 |
−1.3 |
682.7 |
C19 |
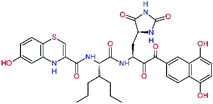 |
−12.2 |
703.8 |
C20 |
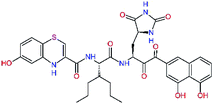 |
−14.7 |
703.8 |
C21 |
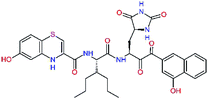 |
−11.4 |
687.8 |
C22 |
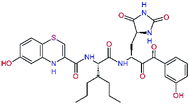 |
−9.1 |
637.7 |
C23 |
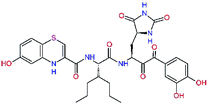 |
−8.5 |
653.7 |
In the Table 7 we present computed binding affinities of inhibitor candidates C20–C28, which contain structural modifications leading to increased proteolytic stability of the peptidomimetic inhibitors. We have adopted replacements of the α-ketoamide linkage and peptide bonds by isosteres non-cleavable by proteases to reduce reactivity, potential toxicity, side effects and instability of the covalent inhibitors (C24–C26). In addition, we have tested introduction of a rigid cyclic molecular core that could stabilize the peptidomimetics in their bound conformation (C27, C28). The non-covalent inhibitor candidate C25 with diacetyl group replaced by the ester linkage appears as a plausible alternative peptidomimetic inhibitor candidate of the SARS-CoV-2 Mpro.
Table 7 Replacement of peptide bonds and cyclization of the SARS-CoV-2 Mpro α-ketoamide inhibitor candidatesa
Compound |
Formula: P3–P2–P1–P1′ |
ΔΔEint,MMa [kcal mol−1] |
Mwb [g mol−1] |
a,bSee the footnote of Table 1. |
C20 |
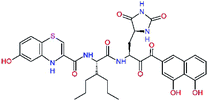 |
−14.7 |
703.8 |
C24 |
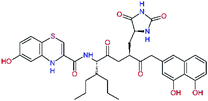 |
−10.9 |
688.8 |
C25 |
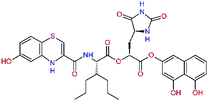 |
−14.5 |
692.7 |
C26 |
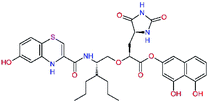 |
−6.6 |
678.7 |
C27 |
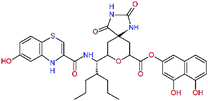 |
−5.9 |
690.8 |
C28 |
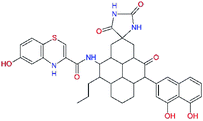 |
−6.3 |
722.8 |
In the last optimization step, we have evaluated whether additional combinations of the flanking P3 and P1′ residues and peptide and ester linkage replacements could lead to smaller, stable and more potent inhibitors. Table 8 shows that the best inhibitor structures with the lowest computed relative interaction energy to the SARS-CoV-2 Mpro are the butanedione linked candidates C31 and C33 with phenol or o-cresol at the P1′ position, modest molecular mass, and 9 HBs to residues: Thr26, Gly143, Ser144, His163, Glu166, His172, Gln189, and Thr190, Fig. 4. The 2 substituents on the phenyl ring of P1′ residue in C33 and C34 form HBs and van der Waals contacts with the main-chain and side chain of Thr26. These interactions in combination with the effect of solvent contribute favourably to the ligand binding. It is interesting to notice that replacement of ester bridge to the P1′ residue with the butanedione linkage enhanced the affinity of the peptidomimetic inhibitors towards the Mpro. Also, analogue C34 containing the ester linkage of the P1′ residue represents a promising lead compound, which displays predicted interaction energy to the SARS-CoV-2 Mpro better than the submicromolar inhibitors 13b and 11r (Table 8).12
Table 8 Cooperativity between P3, P2, P1 and P1′ residues and downsizing of optimized SARS-CoV-2 Mpro α-ketoamide and ester inhibitor candidatesa
Compound |
Formula: P3–P2–P1–P1′ |
ΔΔEint,MMa [kcal mol−1] |
Mwb [g mol−1] |
a,bSee the footnote of Table 1. |
C25 |
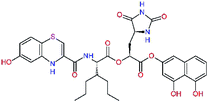 |
−14.5 |
692.7 |
C29 |
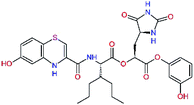 |
−14.4 |
626.7 |
C30 |
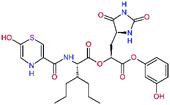 |
−11.2 |
560.6 |
C31 |
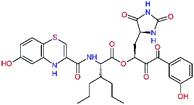 |
−16.3 |
638.7 |
C32 |
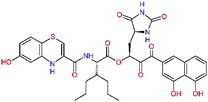 |
−15.8 |
704.7 |
C33 |
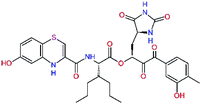 |
−16.7 |
652.7 |
C34 |
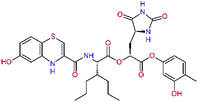 |
−16.8 |
640.7 |
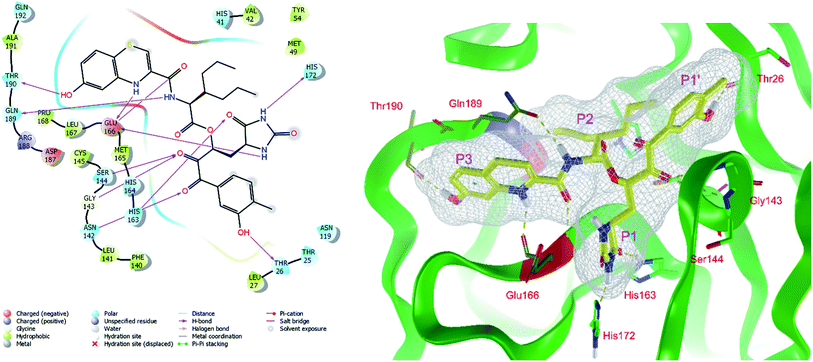 |
| Fig. 4 Left: 2D-interactions scheme of inhibitor candidate C33 at the SARS-CoV-2 Mpro binding site optimized by MM. Right: 3D structure of inhibitor C33 bound to Mpro in tube representation (yellow – carbon, blue – nitrogen, red – oxygen, beige – sulphur, hydrogens are not displayed). Hydrogen bonds are shown as yellow dashed lines. The protein ribbon is coloured by residue charge (blue – cationic, green – neutral, red – anionic). | |
MD simulations
To evaluate the stability of selected modelled Mpro-inhibitor complexes and conformational flexibility of the bound inhibitors we have carried out 200 ns MD simulations (see the Methods section) using Desmond software.32 Fig. 5 illustrates the simulated system and evolutions of active site interactions within the complexes and ligand properties during the simulation.
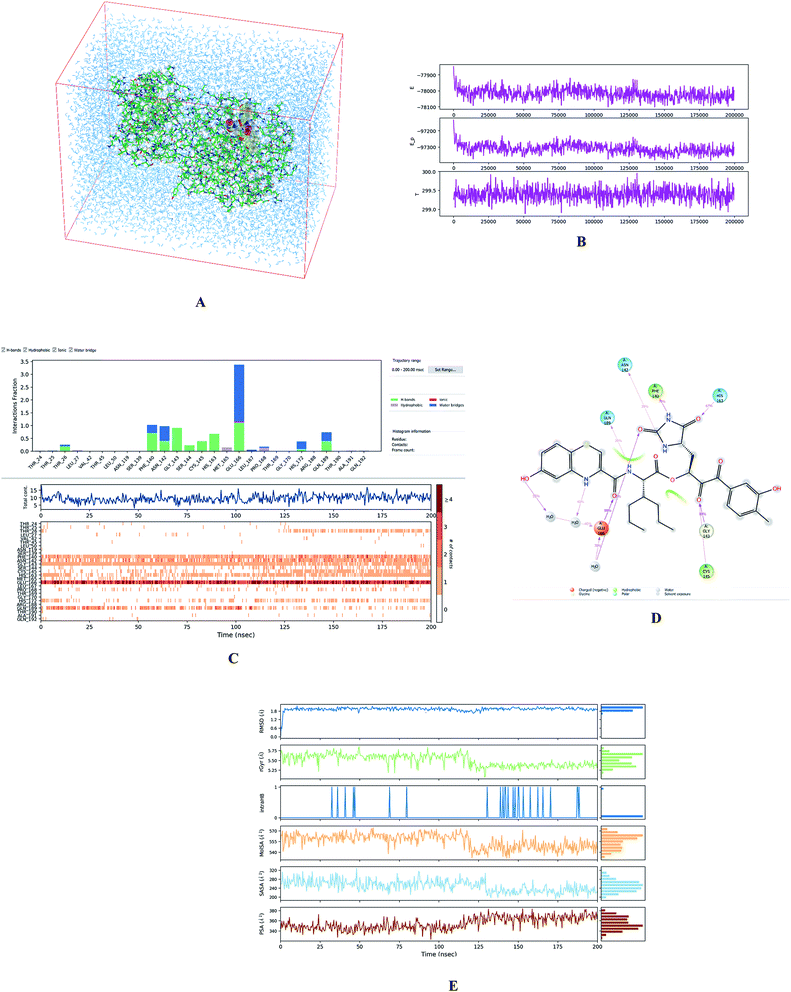 |
| Fig. 5 (A) Periodic box with solvated Mpro-C33 complex. (B) Top: plot of total energy of the system during the 200 ns simulation in Desmond32 (〈E〉 = −78 018 ± 32 kcal mol−1), middle: potential energy (〈Ep〉 = −97 311 ± 28 kcal mol−1), bottom: temperature (〈T〉 = 299.4 ± 0.2 K). (C) Analysis of enzyme-inhibitor interactions. Top: contribution of individual active site residues to inhibitor binding (HB – green, ionic interactions – magenta, hydrophobic – purple, water bridges – blue); middle: number of favourable contacts between the Mpro and C33, bottom: time-evolution of the interactions between inhibitor and individual active site residues. (D) 2D representation of the most populated attractive interactions between C33 and individual active site residues of SARS-CoV-2 Mpro occurring at least in 1/3 of the 500 analysed frames. (E) Evolution of properties of the bound inhibitor during the simulations. Top to bottom: root mean square deviation from the initial structure (RMSD), radius of gyration (rGyr), number of intramolecular hydrogen bonds (intraHB), molecular surface area (MolSA), solvent-accessible surface area (SASA), and polar surface area (PSA). | |
The assessment showed that complexes of the most stable proposed inhibitor candidates C31–C34 preserve the binding mode as well as the ligand conformation during the MD simulation. The binding analysis also indicated that the main contribution to the inhibitor binding originates from HB and polar interactions of the P3 residue with the Glu166, which is essential for the structure of Mpro dimer and its catalytic function.9,10,12,18 The other significant contribution to the inhibitor binding comes from the novel glutamine hydantoin residue P1 that maintains the HBs with Phe140, His163, and His172 residues. Also, other substitutions guided by the Mpro structure, such as introduction of polar hydroxyl groups to the aromatic rings of flanking P3 and P1′ residues, which bind through HBs to the Thr26, Thr190, and Gln192, and water molecules, were preserved during most of the MD simulation time and stabilized the Mpro-C33 complex (Fig. 5C and D). The results of MD simulations confirmed the validity of the binding mode of peptidomimetic inhibitors predicted from modelling of the Mpro-inhibitor complexes and MM calculations.
QM/MM calculations
In the crystal structure of SARS-CoV-2 Mpro-13b12 the residues P1 and P1′ of the inhibitor make polar contacts with amino acid side chains forming the S1 and S1′ subsites. Moreover, the 13b and N3 form at least 4 HBs with the main chain of the active site residues, which helps to lock the inhibitor inside the substrate binding pocket.12 To evaluate the binding affinities of designed inhibitors towards the Mpro with a higher accuracy, including also the effects of polarization, charge transfer, lone pair – aromatic interactions, and solvent polarization, we have used a more rigorous QM/MM approach at the DFT-M06-2X/6-311++G(d,p)//MM-OPLS-2005-PBF (water) level of theory (see the Methods section) for a limited number of known inhibitors and new inhibitor candidates. Table 9 gives a more accurate estimate of the enzyme – inhibitor interaction energies ΔΔEint,QM/MM for 3 reference inhibitors 13b, 11n and 11r,12,23 and 3 designed inhibitor candidates C31, C33 and C34. Both the α-ketoamide covalent inhibitors C31 and C33 as well as the ester analogue C34 show considerably better predicted interaction energies to Mpro then the submicromolar α-ketoamides 13b, 11n, and 11r, Table 9. The QM/MM calculations confirmed the trend in ΔΔEint,MM obtained by simpler MM calculations shown above (Tables 3–8). The quantum mechanical description of the enzyme-inhibitor interactions within the active site clearly favoured the more polar new molecules C31, C33 and C34 over the known inhibitors. The specificity of these lead compounds towards the SARS-CoV-2 Mpro is highly increased. Compared to the submicromolar inhibitor 13b, the leads C31, C33, and C34 form 6 additional HBs to the binding site residues of the Mpro. The novel hydantoin moiety, which occupies the S1 subsite, contributes alone by 3 HBs to His163, Glu166, and His172 side chains towards the inhibitor binding affinity and specificity (Fig. 6). The compound 13b inhibits the recombinant SARS-CoV-2 Mpro with the IC50exp of 0.67 μM (ref. 12) and 11r with IC50exp = 0.18 μM, Table 9.12,23 The predicted significantly enhanced binding affinities of C31, C33, and C34 compared to the known inhibitors 13b, 11n, and 11r suggest that these analogues could represent new promising lead compounds worthwhile of further development.
Table 9 Comparison of relative enzyme-inhibitor interaction energies of known and designed α-ketoamide and ester Mpro inhibitors computed by the QM/MM method
Inhibitor |
Formula: P3–P2–P1–P1′ |
ΔΔEint,QM/MMa [kcal mol−1] |
Mwb [g mol−1] |
QC*c [è] |
IC50expd SARS-CoV-2 (2019/20) Mpro [μM] |
Relative enzyme-inhibitor interaction energies taken with respect to the reference inhibitor 13b were calculated by the hybrid QM/MM method DFT-M06-2X/6-311++G(d,p)//MM-OPLS-2005-PBF (water) in solution: ΔΔEint,QM/MM = ΔEint,QM/MM(Ix) − ΔEint,QM/MM(13b) = [Etot,QM/MM{Mpro–Ix}aq − Etot,QM/MM{Mpro}aq − Etot,QM/MM{Ix}aq] − ΔEint,QM/MM(13b), where Etot,QM/MM is total QM/MM energy of solvated enzyme-inhibitor complex {Mpro–Ix}aq, solvated enzyme {Mpro}aq, or solvated inhibitor {Ix}aq. Molar mass. Net Mulliken atomic charge,56 obtained by molecular orbital analysis, on the carbon of P1 residue (indicated by *) undergoing the nucleophilic attack of the sulphur Sγ of catalytic Cys145 residue during peptide bond cleavage by the Mpro, was calculated by the DFT method in vacuum for bound inhibitor in the Mpro–Ix complex. Experimental half-maximal inhibitory concentrations (IC50exp) of SARS-CoV-2 (2019/20) Mpro inhibition were taken from ref. 12. The experimental inhibitory activities are available only for inhibitors 13b and 11r. The interaction energy of the irreversible inhibitors (13b, 11n and 11r) was computed after breaking the covalent bond of their P1 residue to the catalytic Cys145. Taken from ref. 12. Reference value. Taken from ref. 23. |
13be,f |
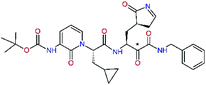 |
0.0g |
591.7 |
0.133 |
0.67 |
11nh |
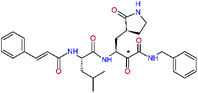 |
1.6 |
532.6 |
0.183 |
— |
11rh |
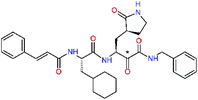 |
4.8 |
572.7 |
0.183 |
0.18 |
C31 |
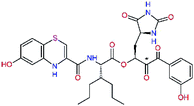 |
−18.6 |
638.7 |
0.143 |
— |
C33 |
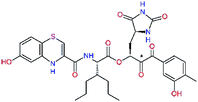 |
−17.4 |
652.7 |
0.150 |
— |
C34 |
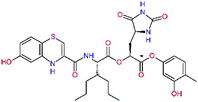 |
−15.2 |
640.7 |
0.315 |
— |
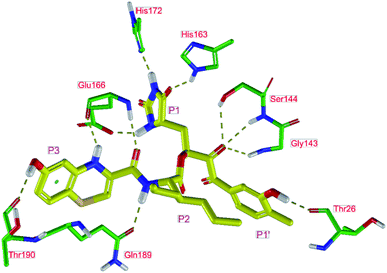 |
| Fig. 6 Detailed view of HB interactions of inhibitor candidate C33 bound to the active site of SARS-CoV-2 Mpro obtained by QM/MM geometry optimization of the enzyme inhibitor complex (in tube representation, yellow – carbon, blue – nitrogen, red – oxygen, beige – sulphur, nonpolar hydrogens are not displayed). Eleven HBs of C33 to eight Mpro active site residues are shown as beige dashed lines. | |
The net atomic charge (QC*) of the electrophilic carbon atom of the carbonyl group (indicated by * in Table 9),56 targeted during the nucleophilic attack by the Sγ of the catalytic Cys145, demonstrates the susceptibility of the ligands to start formation of the covalent thiohemiketal linkage to the Cys145.9 As we can see from Table 9, the net charge QC* of inhibitor candidates C31 and C33 is comparable to that in the α-ketoamide inhibitors 13b, 11n, and 11r, which indicates similar reactivity towards nucleophiles and equivalent potential for undesired side-effects. On the other hand, the ester analogue C34 with QC* of 0.315e may represent a stronger electrophile than the reference α-ketoamide inhibitors.
ADME properties
Many lead compounds fail at an advanced stage of pharmaceutical development due to adverse pharmacokinetic profiles.57 It is therefore essential to incorporate ADME properties prediction already into the lead prioritization. Therefore, we have calculated a set of 24 ADME-related descriptors of known Mpro inhibitors as well as the 3 best designed inhibitor candidates by the QikProp software.58 Table 10 lists 9 selected descriptors, which were computed by the methods of Jorgensen.59–61 The overall drug-likeness parameter (#stars), which describes the compliance of ADME properties with the requirements for drug-like molecules, characterizes in a simple manner the pharmacokinetic profile of inhibitor candidates and can serve as a secondary compound selection criterion. The new inhibitor candidates listed in Table 10, C31, C33, and C34 display acceptable ADME properties. Therefore, these molecules can be recommended for further pharmaceutical development.
Table 10 Selected ADME-related properties of inhibitors of Mpro of SARS-CoV-2 predicted with help of QikProp58
Inhibitor |
vRoFa |
log Po/wb |
log Swatc |
log KHSAd |
Pcacoe |
#metabf |
HOAg |
log HERGh |
#starsi |
Number of violations of Lipinski's rule of five62 and drug-like character of compounds. The rule requires that: Mw < 500 Da, log Po/w < 5, #HBdon ≤ 5, #HBacc ≤ 10. Optimum range of values: vRoF ≤ 4. Logarithm of predicted octanol/water partition coefficient. Optimum range of values: −2.0 to +6.5. Logarithm of predicted aqueous solubility (S in [mol dm−3]) gives the concentration of the solute in a saturated solution that is in equilibrium with the crystalline solid. Optimum range of values: −6.5 to +0.5. Logarithm of predicted binding constant to human serum albumin. Optimum range of values: −1.5 to +1.5. Predicted apparent gut/blood barrier permeability by passive transport in [nm s−1] using the Caco-2 cells model. Optimum range of values: Pcaco < 25 nm s−1 is poor, Pcaco > 500 nm s−1 is great. Number of likely metabolic reactions. Predicted human oral absorption expressed in %. Optimum range of values: HOA > 80% is high, HOA < 25% is poor. Logarithm of predicted IC50 value for blockage of the HERG K+ channels. Optimum range of values: concern if log HERG <−5. Number of property or descriptor values that fall outside the 95% range of similar values of known drugs for 24 descriptors calculated in QikProp58 (the remaining 15 calculated descriptors are not shown) documents drug-like character and pharmacokinetic profile of a compound. Optimum range of values: 0–5. Taken from ref. 12. Taken from ref. 23. |
13bj |
2 |
2.5 |
−3.8 |
−0.4 |
78 |
6 |
50 |
−4.2 |
0 |
11nk |
1 |
3.2 |
−3.3 |
−0.1 |
56 |
4 |
64 |
−3.0 |
0 |
11rk |
1 |
3.9 |
−3.9 |
0.2 |
50 |
4 |
67 |
−3.1 |
0 |
C31 |
3 |
2.8 |
−3.5 |
−0.1 |
68 |
9 |
37 |
−4.8 |
5 |
C33 |
3 |
3.0 |
−3.7 |
0.1 |
68 |
9 |
38 |
−4.5 |
4 |
C34 |
3 |
3.2 |
−4.0 |
0.2 |
104 |
9 |
43 |
−4.5 |
1 |
Conclusions
The SARS-CoV-2 Mpro was recognized as a validated pharmacological target for the design of antiviral drugs, which are urgently needed to combat the ongoing COVID-19 pandemic. Computer-aided structure-based design of reversible and irreversible inhibitors of the Mpro took advantage of the crystal structures of complexes of viral protease co-crystallized with peptidomimetic inhibitors N3 and 13b.12,14 Hydantoin, benzothiazine and cresol moieties were identified as promising design elements that can occupy S1, S3–S4, and S1′ subsites of the Mpro active site. Our effort resulted in the identification of new analogues C31, C33 and C34 with predicted enhanced binding affinities to Mpro, elevated specificity, and favourable ADME-related properties. Predictions based on MM calculations and QSAR model of Mpro inhibition were assessed by MD simulations and qualitatively confirmed by the more comprehensive QM/MM approach.
Therefore, we encourage medicinal chemistry laboratories working in the field of drug design and development against the COVID-19 to verify our computational predictions by synthesis and enzyme inhibition assays of the proposed SARS-CoV-2 Mpro inhibitor candidates.
Author contributions
The manuscript was written through contributions of all authors. All authors have given approval to the final version of the manuscript.
Funding sources
This research was funded by the grants APVV-17-0239 and PP-COVID-20-0010 of the Slovak Research and Development Agency as well as the grant VEGA 1/0228/17 of the Granting Agency of Slovak Ministry of Education and Slovak Academy of Sciences.
Abbreviations
3CLpro or Mpro | Main protease of SARS-CoV-2 |
3D | Three-dimensional |
#stars | ADME-related overall drug–likeness parameter |
ADME | Adsorption, distribution, metabolism, and excretion |
COVID-19 | Coronavirus disease 2019 |
DFT | Density functional theory |
GB/SA | Generalized Born solvent accessible surface area |
MM | Molecular mechanics |
M06-2X | Minnesota class 06 hybrid exchange-correlation density functional with double amount of nonlocal exchange (2X) |
MERS | Middle East respiratory syndrome |
meta-GGA | Meta generalized gradient approximation functional |
OPLS3e | Optimized potential for liquid simulations version 3 extended |
OPLS-2005 | All atom force field developed by Schrödinger for organic molecules |
PBF | Poisson Boltzmann finite element method |
PDB | Protein Data Bank |
QM/MM | Mixed quantum mechanics/molecular mechanics approach |
SARS | Severe acute respiratory syndrome |
Conflicts of interest
The authors declare no conflict of interest.
Acknowledgements
Partial financial support by the Slovak Research and Development Agency grant APVV-17-0239 and PP-COVID-20-0010 as well as Granting Agency of Slovak Ministry of Education and Slovak Academy of Sciences grant VEGA 1/0228/17 is gratefully acknowledged. This work was supported by services, staff expertise and high-performance computing facilities CLARA@UNIBA.SK of the Centre for Information Technology of Comenius University in Bratislava.
References
- F. Wu, S. Zhao, B. Yu, Y. M. Chen, W. Wang, Z. G. Song, Y. Hu, Z. W. Tao, J. H. Tian, Y. Y. Pei, M. L. Yuan, Y. L. Zhang, F. H. Dai, Y. Liu, Q. M. Wang, J. J. Zheng, L. Xu, E. C. Holmes and Y. Z. Zhang, A new coronavirus associated with human respiratory disease in China, Nature, 2020, 579(7798), 265–269 CrossRef CAS.
- P. Zhou, X. L. Yang, X. G. Wang, B. Hu, L. Zhang, W. Zhang, H. R. Si, Y. Zhu, B. Li, C. L. Huang, H. D. Chen, J. Chen, Y. Luo, H. Guo, R. D. Jiang, M. Q. Liu, Y. Chen, X. R. Shen, X. Wang, X. S. Zheng, K. Zhao, Q. J. Chen, F. Deng, L. L. Liu, B. Yan, F. X. Zhan, Y. Y. Wang, G. F. Xiao and Z. L. Shi, A pneumonia outbreak associated with a new coronavirus of probable bat origin, Nature, 2020, 579(7798), 270–273 CrossRef CAS.
- C. Scavone, S. Brusco, M. Bertini, L. Sportiello, C. Rafaniello, A. Zoccoli, L. Berrino, G. Racagni, F. Rossi and A. Capuano, Current pharmacological treatments for COVID-19: what's next?, Br. J. Pharmacol., 2020, 177(21), 4813–4824 CrossRef CAS.
- Y. Chen, Q. Liu and D. Guo, Emerging coronaviruses: genome structure, replication, and pathogenesis, J. Med. Virol., 2020, 92(4), 418–423 CrossRef CAS.
- K. Anand, J. Ziebuhr, P. Wadhwani, J. R. Mesters and R. Hilgenfeld, Coronavirus main proteinase (3CLpro) structure: basis for design of anti-SARS drugs, Science, 2003, 300(5626), 1763–1767 CrossRef CAS.
- D. H. Goetz, Y. Choe, E. Hansell, Y. T. Chen, M. McDowell, C. B. Jonsson, W. R. Roush, J. McKerrow and C. S. Craik, Substrate specificity profiling and identification of a new class of inhibitor for the major protease of the SARS coronavirus, Biochemistry, 2007, 46(30), 8744–8752 CrossRef CAS.
- C. P. Chuck, L. T. Chong, C. Chen, H. F. Chow, D. C. C. Wan and K. B. Wong, Profiling of substrate specificity of SARS-CoV 3CLpro, PLoS One, 2010, 5(10), e13197 CrossRef.
- W. Rut, K. Groborz, L. Zhang, X. Sun, M. Zmudzinski, R. Hilgenfeld and M. Drag, Substrate specificity profiling of SARS-CoV-2 Mpro protease provides basis for anti-COVID-19 drug design, bioRxiv, 2020 DOI:10.1101/2020.03.07.981928.
- T. Pillaiyar, M. Manickam, V. Namasivayam, Y. Hayashi and S. H. Jung, An overview of severe acute respiratory syndrome-coronavirus (SARS-CoV) 3CL protease inhibitors: peptidomimetics and small molecule chemotherapy, J. Med. Chem., 2016, 59(14), 6595–6628 CrossRef CAS.
- H. Yang, W. Xie, X. Xue, K. Yang, J. Ma, W. Liang, Q. Zhao, Z. Zhou, D. Pei, J. Ziebuhr, R. Hilgenfeld, K. Y. Yuen, L. Wong, G. Gao, S. Chen, Z. Chen, D. Ma, M. Bartlam and Z. Rao, Design of wide-spectrum inhibitors targeting coronavirus main proteases, PLoS Biol., 2005, 3(10), e324 CrossRef.
- X. Xue, H. Yu, H. Yang, F. Xue, Z. Wu, W. Shen, J. Li, Z. Zhou, Y. Ding, Q. Zhao, X. C. Zhang, M. Liao, M. Bartlam and Z. Raoet, Structures of two coronavirus main proteases: implications for substrate binding and antiviral drug design, J. Virol., 2008, 82(5), 2515–2527 CrossRef CAS.
- L. Zhang, D. Lin, X. Sun, U. Curth, C. Drosten, L. Sauerhering, S. Becker, K. Rox and R. Hilgenfeld, Crystal structure of SARS-CoV-2 main protease provides a basis for design of improved α-ketoamide inhibitors, Science, 2020, 368(6489), 409–412 CrossRef CAS , (PDB ID 6Y2F).
- H. Yang, M. Yang, Y. Ding, Y. Liu, Z. Lou, Z. Zhou, L. Sun, L. Mo, S. Ye, H. Pang, G. F. Gao, K. Anand, M. Bartlam, R. Hilgenfeld and Z. Rao, The crystal structures of severe acute respiratory syndrome virus main protease and its complex with an inhibitor, Proc. Natl. Acad. Sci. U. S. A., 2003, 100(23), 13190–13195 CrossRef CAS.
- Z. Jin, X. Du, Y. Xu, Y. Deng, M. Liu, Y. Zhao, B. Zhang, X. Li, L. Zhang, C. Peng, Y. Duan, J. Yu, L. Wang, K. Yang, F. Liu, R. Jiang, X. Yang, T. You, X. Liu, X. Yang, F. Bai, H. Liu, X. Liu, L. W. Guddat, W. Xu, G. Xiao, C. Qin, Z. Shi, H. Jiang, Z. Rao and H. Yang, Structure of Mpro from COVID-19 virus and discovery of its inhibitors, Nature, 2020, 582(7811), 289–293 CrossRef CAS , (PDB ID 6LU7).
- P. S. Dragovich, T. J. Prins, R. Zhou, S. E. Webber, J. T. Marakovits, S. A. Fuhrman, A. K. Patick, D. A. Matthews, C. A. Lee, C. E. Ford, B. J. Burke, P. A. Rejto, T. F. Hendrickson, T. Tuntland, E. L. Brown, J. W. Meador III, R. A. Ferre, J. E. Harr, M. B. Kosa and S. T. Worland, Structure-based design, synthesis, and biological evaluation of irreversible human rhinovirus 3C protease inhibitors. 4. Incorporation of P1 lactam moieties as L-glutamine replacements, J. Med. Chem., 1999, 42(7), 1213–1224 CrossRef CAS.
- D. L. Barnard, Current status of anti-picornavirus therapies, Curr. Pharm. Des., 2006, 12(11), 1379–1390 CrossRef CAS.
- J. Rocha-Pereira, M. S. Nascimento, Q. Ma, R. Hilgenfeld, J. Neyts and D. Jochmans, The enterovirus protease inhibitor rupintrivir exerts cross-genotypic anti-norovirus activity and clears cells from the norovirus replicon, Antimicrob. Agents Chemother., 2014, 58(8), 4675–4681 CrossRef CAS.
- A. K. Ghosh, M. Brindisi, D. Shahabi, M. E. Chapman and A. D. Mesecar, Drug development and medicinal chemistry efforts toward SARS-coronavirus and COVID-19 therapeutics, ChemMedChem, 2020, 15(11), 907–932 CrossRef CAS.
- A. K. Ghosh, K. Xi, K. Ratia, B. D. Santarsiero, W. Fu, B. H. Harcourt, P. A. Rota, S. C. Baker, M. E. Johnson and A. D. Mesecar, Design and synthesis of peptidomimetic severe acute respiratory syndrome chymotrypsin-like protease inhibitors, J. Med. Chem., 2005, 48(22), 6767–6771 CrossRef CAS.
- A. K. Ghosh, K. Xi, V. Grum-Tokars, X. Xu, K. Ratia, W. Fu, K. V. Houser, S. C. Baker, M. E. Johnson and A. D. Mesecar, Structure-based design, synthesis, and biological evaluation of peptidomimetic SARS-CoV 3CLpro inhibitors, Bioorg. Med. Chem. Lett., 2007, 17(21), 5876–5880 CrossRef CAS.
- S. Yang, S. J. Chen, M. F. Hsu, J. D. Wu, C. T. Tseng, Y. F. Liu, H. C. Chen, C. W. Kuo, C. S. Wu, L. W. Chang, W. C. Chen, S. Y. Liao, T. Y. Chang, H. H. Hung, H. L. Shr, C. Y. Liu, Y. A. Huang, L. Y. Chang, J. C. Hsu, C. J. Peters, A. H. Wang and M. C. Hsu, Synthesis, crystal structure, structure-activity relationships, and antiviral activity of a potent SARS coronavirus 3CL protease inhibitor, J. Med. Chem., 2006, 49(16), 4971–4980 CrossRef CAS.
- T. Regnier, D. Sarma, K. Hidaka, U. Bacha, E. Freire, Y. Hayashi and Y. Kiso, New developments for the design, synthesis and biological evaluation of potent SARS-CoV 3CL(pro) inhibitors, Bioorg. Med. Chem. Lett., 2009, 19(10), 2722–2727 CrossRef CAS.
- L. Zhang, D. Lin, Y. Kusov, Y. Nian, Q. Ma, J. Wang, A. von Brunn, P. Leyssen, K. Lanko, J. Neyts, A. de Wilde, E. J. Snijder, H. Liu and R. Hilgenfeld, α-Ketoamides as broad-spectrum inhibitors of coronavirus and enterovirus replication: structure-based design, synthesis, and activity assessment, J. Med. Chem., 2020, 63(9), 4562–4578 CrossRef CAS.
- J. Dyall, C. M. Coleman, B. J. Hart, T. Venkataraman, M. R. Holbrook, J. Kindrachuk, R. F. Johnson, G. G. Olinger Jr, P. B. Jahrling, M. Laidlaw, L. M. Johansen, C. M. Lear-Rooney, P. J. Glass, L. E. Hensley and M. B. Frieman, Repurposing of clinically developed drugs for treatment of Middle East respiratory syndrome coronavirus infection, Antimicrob. Agents Chemother., 2014, 58(8), 4885–4893 CrossRef.
- T. Pillaiyar, M. Manickam and S. H. Jung, Middle East respiratory syndrome coronavirus (MERS-CoV): an updated overview and pharmacotherapeutics, Med. Chem., 2015, 5(8), 361–372 Search PubMed.
- H. M. Berman, J. Westbrook, Z. Feng, G. Gilliland, T. N. Bhat, H. Weissig, I. N. Shindyalov and P. E. Bourne, The Protein Data Bank, Nucleic Acids Res., 2000, 28(1), 235–242 CrossRef CAS.
- J. C. Shelley, A. Cholleti, L. Frye, J. R. Greenwood, M. R. Timlin and M. Uchimaya, Epik: a software program for pKa prediction and protonation state generation for drug-like molecules, J. Comput.-Aided Mol. Des., 2007, 21(12), 681–691 CrossRef CAS.
- Epik, Small-Molecule Discovery Suite, version 5.1, Schrödinger, LLC., New York, NY, USA, release 2020-1, 2020 Search PubMed.
- E. Harder, W. Damm, J. Maple, C. Wu, M. Reboul, J. Y. Xiang, L. Wang, D. Lupyan, M. K. Dahlgren, J. L. Knight, J. W. Kaus, D. Cerutti, G. Krilov, W. L. Jorgensen, R. Abel and R. A. Friesner, OPLS3: a force field providing broad coverage of drug-like small molecules and proteins, J. Chem. Theory Comput., 2016, 12(1), 281–296 CrossRef CAS.
- W. C. Still, A. Tempczyk, R. C. Hawlely and T. A. Hendrickson, General treatment of solvation for molecular mechanics, J. Am. Chem. Soc., 1990, 112(16), 6127–6129 CrossRef CAS.
- MacroModel, Small-Molecule Discovery Suite, version 12.7, Schrödinger, LLC., New York, NY, USA, release 2020-1, 2020 Search PubMed.
- Desmond Molecular Dynamics System, version 3.6, D. E. Shaw Research, New York, NY, 2020, Maestro-Desmond Interoperability Tools, Schrödinger, New York, NY, 2020 Search PubMed.
- G. J. Martyna, M. L. Klein and M. Tuckerman, Nose–Hoover chains: the canonical ensemble via continuous dynamics, J. Chem. Phys., 1992, 97(4), 2635–2643 CrossRef.
- G. J. Martyna, D. J. Tobias and M. L. Klein, Constant pressure molecular dynamics algorithms, J. Chem. Phys., 1994, 101(5), 4177–4189 CrossRef CAS.
- V. Frecer, F. Berti, F. Benedetti and S. Miertus, Design of peptidomimetic inhibitors of aspartic protease of HIV-1 including –PheΨPro– core and favourable ADME properties, J. Mol. Graphics Modell., 2008, 27(3), 376–387 CrossRef CAS.
- V. Frecer, E. Megnassan and S. Miertus, Design and in silico screening of combinatorial library of antimalarial analogues of triclosan inhibiting Plasmodium falciparum enoyl-acyl carrier protein reductase, Eur. J. Med. Chem., 2009, 44(7), 3009–3019 CrossRef CAS.
- V. Frecer and S. Miertus, Design, structure-based focusing and in silico screening of combinatorial library of peptidomimetic inhibitors of dengue virus NS2B-NS3 protease, J. Comput.-Aided Mol. Des., 2010, 24(3), 195–212 CrossRef CAS.
- V. Frecer, P. Seneci and S. Miertus, Computer-assisted combinatorial design of bicyclic thymidine analogues as inhibitors of Mycobacterium tuberculosis thymidine monophosphate kinase, J. Comput.-Aided Mol. Des., 2011, 25(1), 31–49 CrossRef CAS.
- I. Kolossváry and W. C. Guida, Low mode search. An efficient, automated computational method for conformational analysis: application to cyclic and acyclic alkanes and cyclic peptides, J. Am. Chem. Soc., 1996, 118(21), 5011–5019 CrossRef.
- D. Lin, L. Zhang, Y. Kusov, Y. Nian, Q. Ma, J. Wang, P. Leyssen, K. Lanko, J. Neyts, A. de Wilde, E. J. Snijder, H. Liu and R. Hilgenfeld, Alpha-ketoamides as broad-spectrum inhibitors of coronavirus and enterovirus replication, to be published, PDB ID 5N19 Search PubMed.
- R. B. Murphy, D. M. Philipp and R. A. Friesner, A mixed quantum mechanics/molecular mechanics (QM/MM) method for large-scale modelling of chemistry in protein environments, J. Comput. Chem., 2000, 21(16), 1442–1457 CrossRef CAS.
- D. M. Philipp and R. A. Friesner, Mixed ab initio QM/MM modelling using frozen orbitals and tests with alanine dipeptide and tetrapeptide, J. Comput. Chem., 1999, 20(14), 1468–1494 CrossRef CAS.
- A. D. Bochevarov, E. Harder, T. F. Hughes, J. R. Greenwood, D. A. Braden, D. M. Philipp, D. Rinaldo, M. D. Halls, J. Zhang and R. A. Friesner, Jaguar: a high-performance quantum chemistry software program with strengths in life and materials sciences, Int. J. Quantum Chem., 2013, 113(18), 2110–2142 CrossRef CAS.
- QSite, Small-Molecule Discovery Suite, Schrödinger, LLC., New York, NY, USA, release 2020-1, 2020 Search PubMed.
- Jaguar, Small-Molecule Discovery Suite, version 10.3 and Impact, version 82011, Schrödinger, LLC., New York, NY, USA, release 2020-1, 2020 Search PubMed.
- Y. Zhao and D. G. Truhlar, Density functionals with broad applicability in chemistry, Acc. Chem. Res., 2008, 41(2), 157–167 CrossRef CAS.
- Y. Zhao and D. G. Truhlar, The M06 suite of density functionals for main group thermochemistry, thermochemical kinetics, noncovalent interactions, excited states, and 20 transition elements: two new functionals and systematic testing of four M06-class functionals and 12 other function, Theor. Chem. Acc., 2008, 120(1–3), 215–241 Search PubMed.
- K. Raghavachari, J. S. Binkley, R. Seeger and J. A. Pople, Self-consistent molecular orbital methods. 20. Basis set for correlated wave-functions, J. Chem. Phys., 1980, 72(1), 650–654 CrossRef.
- K. B. Wiberg, Basis set effects on calculated geometries: 6-311++G** vs. aug-cc-pVDZ, J. Comput. Chem., 2004, 25(11), 1342–1346 CrossRef CAS.
- G. A. Kaminski, R. A. Friesner, J. Tirado-Rives and W. L. Jorgensen, Evaluation and reparametrization of the OPLS-AA force field for proteins via comparison with accurate quantum chemical calculations on peptides, J. Phys. Chem. B, 2001, 105(28), 6474–6487 CrossRef CAS.
- B. Marten, K. Kim, C. Cortis, R. A. Friesner, R. B. Murphy, M. N. Ringnalda, D. Sitkoff and B. Honig, New model for calculation of solvation free energies:
correction of self-consistent reaction field continuum dielectric theory for short-range hydrogen-bonding effects, J. Phys. Chem., 1996, 100(28), 11775–11788 CrossRef CAS. - J. Tan, S. George, Y. Kusov, M. Perbandt, S. Anemuller, J. R. Mesters, H. Norder, B. Coutard, C. Lacroix, P. Leyssen, J. Neyts and R. Hilgenfeld, 3C protease of enterovirus 68: structure-based design of Michael acceptor inhibitors and their broad-spectrum antiviral effects against picornaviruses, J. Virol., 2013, 87(8), 4339–4351 CrossRef CAS.
- P. S. Dragovich, R. Zhou, D. J. Skalitzky, S. A. Fuhrman, A. K. Patick, C. E. Ford, J. W. Meador III and S. T. Worland, Solid-phase synthesis of irreversible human rhinovirus 3C protease inhibitors. Part 1: Optimization of tripeptides incorporating N-terminal amides, Bioorg. Med. Chem., 1999, 7(4), 589–598 CrossRef CAS.
- M. Egli and S. Sarkhel, Lone pair – aromatic interactions:
to stabilize or not to stabilize, Acc. Chem. Res., 2007, 40(3), 197–205 CrossRef CAS. - A. M. Sapse, B. S. Schwiezer, A. P. Dicker, J. R. Bertino and V. Frecer, Ab initio studies of aromatic-aromatic and aromatic-polar interactions in the binding of substrate and inhibitor to dihydrofolate-reductase, Int. J. Pept. Protein Res., 1992, 39(1), 18–23 CrossRef CAS.
- R. S. Mulliken, Electronic population analysis on LCAO–MO molecular wave functions. I., J. Chem. Phys., 1955, 23(10), 1833–1840 CrossRef CAS.
- F. Darvas, G. Keseru, A. Papp, G. Dorman, L. Urge and P. Krajcsi, In silico and ex silico ADME approaches for drug discovery, Curr. Top. Med. Chem., 2002, 2(12), 1287–1304 CrossRef CAS.
- QikProp, Small-Molecule Discovery Suite, version 6.3, Schrödinger, LLC., New York, NY, USA, release 2020-1, 2020 Search PubMed.
- E. M. Duffy and W. L. Jorgensen, Prediction of properties from simulations: free energies of solvation in hexadecane, octanol, and water, J. Am. Chem. Soc., 2000, 122(12), 2878–2888 CrossRef CAS.
- W. L. Jorgensen and E. M. Duffy, Prediction of drug solubility from Monte Carlo simulations, Bioorg. Med. Chem. Lett., 2000, 10(11), 1155–1158 CrossRef CAS.
- W. L. Jorgensen and E. M. Duffy, Prediction of drug solubility from structure, Adv. Drug Delivery Rev., 2002, 54(3), 355–366 CrossRef CAS.
- C. A. Lipinski, F. Lombardo, B. W. Dominy and P. J. Feeney, Experimental and computational approaches to estimate solubility and permeability in drug discovery and development settings, Adv. Drug Delivery Rev., 2001, 46(1–3), 3–26 CrossRef CAS.
Footnote |
† The basis set of the QM region of Mpro-13b complex consisted of 3662 basis functions (966 electrons). The computer run time for the full QM/MM geometry optimization of this complex (approx. 150 iterations) on 32 threads of Intel Xeon Silver (16 core, AVX-512 FMA), lasted approximately 12 days. |
|
This journal is © The Royal Society of Chemistry 2020 |