Performance of Vehicle Add-on Mobile Monitoring System PM2.5 measurements during wildland fire episodes†
Received
6th December 2023
, Accepted 20th February 2024
First published on 26th February 2024
Abstract
Fine particulate matter (PM2.5) resulting from wildland fire is a significant public health risk in the United States (U.S.). The existing stationary monitoring network and the tools used to alert the public of smoke conditions, such as the Air Quality Index or NowCast, are not optimized to capture actual exposure concentrations in impacted communities given that wildland fire smoke plumes have characteristically steep exposure concentration gradients that can vary over fine spatiotemporal scales. In response, we developed and evaluated a lightweight, universally attachable mobile PM2.5 monitoring system to provide supplemental, real-time air quality information during wildfire incidents and prescribed burning activities. We retroactively assessed the performance of the mobile monitor compared to nearby (100–1500 m) stationary low-cost sensors and regulatory monitors using 1 minute averaged data collected during two large wildfires in the western U.S. and during one small, prescribed burn in the Midwest. The mobile measurements were highly correlated (R2 > 0.85) with the stationary network during the large wildfires. Further, 1 minute averaged mobile measurements differed from three collocated stationary instruments by <25% on average for fourteen out of fifteen total passages. For the small, prescribed burn, rapidly changing conditions near the fire border complicated the comparison of mobile and stationary measurements but the spatial maximum concentrations measured by both instruments were consistent. In general, this work highlights the value of using portable sensor technologies to address the monitoring challenges presented by dynamic wildland fire conditions and demonstrates the value in combining mobile monitoring with stationary data where possible.
Environmental significance
Wildland fire smoke presents a major growing threat to public health. The existing stationary monitoring network used to alert the public of smoke conditions is not optimized to capture concentration differences that occur over fine spatiotemporal scales, limiting the ability of impacted communities to take quick, protective action to reduce their exposure. This study demonstrates how a loan program of portable fine particulate matter monitoring systems can support wildfire incident responders by providing a flexible means to collect real-time air quality information in affected areas. We found that mobile measurements from this system were comparable to low cost and research grade stationary measurements under real wildfire conditions, suggesting the monitor can collect reliable data to assist in emergency response activities.
|
1 Introduction
Smoke from wildland fire, which includes wildfires and prescribed fires, can expose nearby communities to harmful air pollutants, including fine particulate matter or PM2.5.1 Many studies have shown that exposure to PM2.5 from wildland fire is linked to acute and chronic health effects.2–5 In the United States (U. S.), wildfires are a major contributor to ambient PM2.5 concentrations in recent years, accounting for an estimated 25–50% of ambient PM2.5 depending on the region.6 Given that large wildfires (>400 ha) have increased in frequency over the past two decades,7 the number of people at risk for smoke related health impacts is expected to grow.
To keep the public abreast of air quality conditions in their area, the U. S. uses the Air Quality Index (AQI). The AQI indicates how clean or polluted the air is, if associated health effects might be a concern (particularly for sensitive populations), and recommends health protective actions.8 The U.S. Environmental Protection Agency (EPA) establishes an AQI for five criteria pollutants (fine and coarse particulate matter, ozone, carbon monoxide, sulfur dioxide, and nitrogen dioxide). During wildland fire events, PM2.5 typically drives the AQI in areas impacted by smoke. The PM2.5 AQI is calculated using the 24 hour mean concentration; however, when conditions can change rapidly such as during wildfire smoke episodes, the U.S. EPA relies on the NowCast AQI to communicate risk at a higher time resolution.9 Higher time resolution information helps the public take action to reduce or mitigate their exposure while smoke episodes occur. Further, reports have found causal or likely to be causal associations between short-term PM2.5 exposure and respiratory and cardiovascular effects and reduced cognitive function.10–12 Associations between short-term exposures and non-accidental mortality were strongest at lags from 0 to 1 day,10 suggesting sub-daily exposure periods can result in health impacts. Given the evidence, there is a clear need for localized high temporal resolution PM2.5 concentration data to support public decision making in smoke impacted areas.
Characterizing the extent and impact of wildland fire smoke plumes remains a challenge, partially due to uncertainty in the spatial quantification of the smoke pollutant concentrations, complicating air quality and public health assessments.2,13 Wildland fire plumes are spatially heterogeneous with characteristically steep exposure concentration gradients, influenced by factors like topography, weather, and fire conditions.1 Interpolated PM2.5 concentrations from the existing regulatory monitoring network may not be representative of actual exposures in impacted communities. For example, using a network of low-cost sensors (mean density: 6.8 per km2) and a Gaussian process model, Kelly et al. (2021)14 found spatial differences in PM2.5 concentration within a small region (<500 km2) during a wildfire event in Salt Lake City that were not apparent on U.S. EPA AirNow visualizations (heatmaps based on the interpolation of data from only government monitoring stations). Notably, the newer U.S. EPA system designed for smoke events (fire.airnow.gov (http://fire.airnow.gov)) includes point data from the PurpleAir low-cost sensor network to add granular information but does not include spatial interpolation. Interpolating and modelling predicted concentrations over complex terrain is complicated, even with the increased information offered by the stationary low-cost sensor network. Citing uncertainty in their model, Kelly et al. (2021)14 found that it was difficult to determine if the smoke plume was flowing down the canyon or over the mountain into the valley. Other recent work combining modelling and the low-cost sensor network in the Salt Lake Valley also pointed to a need for more information on local smoke drainage behavior, given the topographic relief in the region.15
To provide needed supplementary information in smoke impacted areas with limited access to air quality data from the existing stationary network, the U.S. EPA launched the Wildfire Smoke Air Monitoring Response Technology (WSMART) Pilot in 2021 as part of a federal government response to address wildfire smoke impacts that are of public health concern.16 WSMART expands the reach of supplemental wildfire smoke monitoring by supporting data sparse areas through its equipment loan program. The loan program relies on an inventory of lower-cost, portable instruments designed for use by onsite emergency response personnel who are often non-experts in air monitoring. WSMART can thus support supplemental monitoring for multiple fire events without requiring highly trained specialists to operate the equipment. Loans are available to state, local, and tribal air quality or public health organizations and to the Interagency Wildland Fire Air Quality Response Program (IWFAQRP) for use by Air Resource Advisors (ARAs). ARAs are dispatched to major wildfire incidents in the U.S. to assist with air quality and smoke assessment for the public and incident personnel.17,18 Presently, ARAs typically have access to a national cache of stationary PM2.5 monitoring kits including E-samplers and E-BAMs (Met One Instruments, Inc.) that can be deployed in locations impacted by smoke.19 The WSMART program expands the smoke monitoring cache by loaning two types of supplemental air monitors, a multipollutant sensor system and a mobile monitoring system (the focus of this manuscript) to ARAs on request. ARAs have used the mobile monitoring system for a variety of applications including roadway visibility assessment, general situational awareness, spatial variability characterization, to identify locations suitable for additional stationary monitoring, and for comparison with nearby monitors.
While mobile monitoring has been conducted extensively to examine urban and industrial air pollution, its use for wildland fire emissions characterization and air quality assessment is nascent. Most mobile studies on wildland fire are focused on quantifying emissions20,21 and characterizing fire conditions.22 Further, much of the literature for mobile monitoring, and thus widely accepted measurement and data analysis methods, has focused on characterizing persistent temporal and spatial urban air quality trends.23–28 Wildland fire is episodic and complex, and conditions change rapidly, which is incompatible with the mobile sampling methodologies developed for urban environments. Consequently, alternative methods are needed to assess the performance of a mobile monitor used for real-time smoke impact and air quality characterization.
The primary objective of this work is to evaluate the WSMART mobile monitor, known as the Vehicle Add-on Mobile Monitoring System or VAMMS, for use in characterizing semi-quantitative regional and local smoke impacts from wildland fires to ultimately provide information on air quality conditions and inform public health decision making. To address this objective, we use novel, proof of concept methodologies and analyses to interpret VAMMS data collected by ARAs during two major wildfires in western U.S. national forests, and data we collected during localized prescribed burning of a protected area of tallgrass prairie. Additionally, we explore the interpretation of high time resolution (1 min) data from the VAMMS in comparison to measurements from other lower time resolution data sources. In conclusion, we discuss potential use applications and limitations of our datasets and the WSMART loan equipment.
2 Methodology
2.1 Vehicle add-on mobile monitoring system
The VAMMS was designed to measure smoke using any vehicle, facilitating rapid and flexible deployment by first responders and researchers at wildland fire events. The compact monitoring system, weighing 15 lbs and measuring 17′′ by 14′′ by 8′′, is entirely contained in a crush resistant case (Pelican, 1450) to enable overnight shipping to the incident while protecting the system components (Fig. S1†). The system is equipped with a research grade particulate matter monitor (pDR-1500, Thermo Scientific) that uses a nephelometer to measure mass concentrations at a 1 second resolution. The instrument has a cyclone on the inlet with a size cut of 2.5 μm to measure PM2.5 concentration and an internal 37 mm filter for gravimetric analysis.
Additionally, the VAMMS includes a global positioning system (GPS, Ultimate GPS, Adafruit) to log location and a microprocessor (RT1062 Teensy 4.0, Adafruit) to integrate data into a single data file per day saved on a local microSD card. The GPS time is used to adjust the microprocessor time to account for drift in the real-time clock. The data is automatically formatted for upload to EPA's Real-time Geospatial Data Viewer (RETIGO, https://www.epa.gov/hesc/real-time-geospatial-data-viewer-retigo) where the data can be visualized on a map or as a time series.
The VAMMS samples through ¼′′ conductive tubing attached to a ¼′′ stainless steel probe with a 7.5° cone and 0.084′′ inlet facing forward into the air stream. The probe is housed in a mounting block that can be attached to the passenger window of any vehicle and secured to the window with an adjustable thumbscrew (Fig. S2†). The VAMMS is battery powered (4.5 AH 12 V Lithium Ion, BLF-12045W, Bienno) or it can be powered via the vehicle auxiliary charging port. The battery power system allows ∼15 h of continuous operation in typical ambient conditions (temperature ∼20 °C). The VAMMS includes an AC adaptor power cable to recharge the battery using wall power when not in use. To date, twenty-four VAMMS units have been produced.
The VAMMS probe provides isokinetic sampling at approximately 35 mph at the 3.5 LPM sample flow required for the PM2.5 cyclone nominal cut point. The target driving speed of 35 mph aligns with common off-highway driving speeds by emergency responders at fires; however, it is not feasible to always maintain the isokinetic sampling velocity due to the real-world driving conditions. We estimated the bias that anisokinetic sampling had on VAMMS PM2.5 measurements for each sampling effort (Table S2†). Images of the VAMMS, the sampling deployment configuration, and details of the isokinetic velocity and mass bias calculations are given in Section 1 of the ESI.†
2.2 Quality assurance and control
The personal DataRAM™ Aerosol Monitor pDR-1500 (Thermo Scientific) in each VAMMS had the same instrument settings. The zero concentration and flow rate were confirmed before and after a deployment. The zero level was required to be within ±3 μg m−3 of 0 and was determined by attaching a HEPA filter to the inlet. If this criterion was not met, the instrument was re-zeroed according to the instrument manual. The flow rate was measured with a TSI Air Flow Calibrator (Model 4199) and was required to be within ±0.3 of 3.5 LPM. The pDR-1500 instrument manual recommends using the relative humidity (RH) correction feature for ambient applications. We do not have a record of when this feature was enabled or disabled for VAMMS deployments prior to November 2022. However, the VAMMS were typically deployed in dry, fire conditions, so we do not expect the use (or not) of the RH correction to interfere with the interpretation of the data presented here.
A pre-weighed glass fiber filter was installed in the pDR-1500 before each new deployment. After the VAMMS was returned, the filter was removed, stored, and post-weighed. In addition, over the 2022–2023 fire season we collected six handling and dynamic blank filters to estimate the amount of mass deposited or lost from the filter due the installation/removal process and from turning the instrument on during the zero and flow rate checks required as part of the quality assurance steps.
VAMMS data lacking geospatial information (e.g., indoor measurements and data collected during start-up before a GPS lock was attained) were excluded. Local sources (i.e., dust from unpaved roads, tailpipe emissions from other vehicles) were detected and removed using a running coefficient of variation (COV) method.29,30 Details of the COV method are given in Section 2 of the ESI.†
2.3 Field sampling strategy
For wildfire events, the VAMMS data were collected by an ARA. These sampling events were sometimes opportunistic, meaning the route was not selected solely for the intention of monitoring, rather monitoring data were collected while the ARA performed their incident responsibilities. These responsibilities may include driving to locations with reports of heavy smoke, setting up temporary stationary monitoring sites, or attending community events to publicly communicate information about smoke conditions. For the prescribed fire event, we collected VAMMS data along intentional driving routes selected to characterize spatial variation upwind and downwind of the burn, including higher-concentration smoke plumes near the fire. For all events, driving speeds were not restricted (other than local speed limits) and varied with the route. The median and mean (±standard deviation) driving speeds for each data set are given in Table S1.†
2.4 Data sets for performance assessment
A summary of the data sets used to evaluate the VAMMS is given in Table 1.
Table 1 Overview of events included in this analysis. For type, Lab = laboratory experiment, Rx = prescribed burning, WF = wildfire. ‘Data removed’ refers to the percentage of the data set identified as a local source (e.g., dust from roadway) and excluded (Section 2.2). The mean and maximum PM2.5 concentration (μg m−3) calculated from the 1 min averaged VAMMS data from each campaign are given. n/a = not applicable
Event |
Type |
Location |
Sampling period |
Hours of data |
Data removed |
PM2.5 mean (μg m−3) |
PM2.5 max (μg m−3) |
Large chamber |
Lab |
Durham, NC |
10/21/22 |
19.7 |
n/a |
10.8 |
150 |
Konza Prairie |
Rx |
Manhattan, KS |
09/14/21–09/15/21 |
3.7 |
1% |
34.9 |
1466 |
Monument fire |
WF |
Trinity County, CA |
08/10/21–09/19/21 |
111 |
0.95% |
226 |
2382 |
Cedar Creek fire |
WF |
Oakridge, OR |
09/24/22–10/12/22 |
27.9 |
1% |
120 |
2759 |
2.4.1 Large chamber experiments.
To evaluate the precision of the VAMMS under controlled conditions, we placed four VAMMS in the U.S. EPA Research Triangle Park large chamber facility and exposed them to an injection of simulated wildfire smoke from the combustion of 0.4 g of pine straw in a tube furnace.31 We also collected a gravimetric filter sample in one of the VAMMS to compare with published correction factors for the pDR-1500.
2.4.2 Cedar Creek wildfire.
The Cedar Creek fire was started on August 1, 2022, by a lightning storm in the Willamette National Forest near Oakridge, OR. The 18 day VAMMS monitoring period started about two months later, during which fire growth (from approximately 114
000 to 122
700 acres in size) and the continued burning and smoldering of interior fuels (typical of any large fire) contributed to heavy smoke. During this time, the border of the fire was within 15 km of the Oakridge, OR regulatory air quality monitoring station (AQMS). The Lane Regional Air Protection Agency (LRAPA) maintains three low-cost PurpleAir sensors, an Ambilabs nephelometer, and a federal equivalent method (FEM) regulatory monitor at the Oakridge AQMS. The ARAs assigned to the incident drove past the Oakridge AQMS on multiple sampling runs, allowing for a high-time resolution evaluation of the VAMMS compared to these instruments.
2.4.3 Monument wildfire.
The Monument fire was started on July 30, 2021, by a lightning strike in the Shasta-Trinity National Forest in Trinity County, CA. During the period the ARAs assigned to the fire periodically operated a VAMMS in the region, the fire grew from approximately 67
000 to over 200
000 acres. The density of the stationary monitoring network in the region (i.e., dozens of PurpleAir sensors and two regulatory monitoring stations in Weaverville and Redding, CA), and the extensive area covered by the VAMMS over the measurement period, allows for a macro-scale inter-comparison of VAMMS, PurpleAir, and regulatory measurements.
2.4.4 Konza Prairie prescribed burns.
The Konza Prairie Biological Station is a protected area of native prairie grass in the Flint Hills of Kansas. To maintain the grasslands, land managers conduct regular controlled burns. The land is organized into 3-acre plots, separated by fire breaks. In September 2021, five adjacent plots were burned over a two-day period. We deployed four PurpleAir sensors upwind and downwind of the plots and used the VAMMS to characterize downwind smoke impacts during the burns. VAMMS data were collected on a 4 × 4 all-terrain vehicle along lightly trafficked dirt fire breaks in between plots. In contrast to the large wildfires, the smoke impacted area was small and the driving paths were designed to capture the variation in smoke concentrations. We were able to get closer to the fire perimeter given the controlled nature of the burn.
2.5 Additional instrumentation
A summary of the instruments included in this analysis used to evaluate the VAMMS is given in Table 2.
Table 2 Overview of instruments used to evaluate the VAMMS. FEM = federal equivalent method, LRAPA = lane regional air protection agency
Instrument |
Measurement principle |
Type |
Corrections |
Sampling resolution |
Events |
PurpleAir PA-II |
Nephelometer |
Low-cost |
Holder et al. (2020) |
80 s, 2 min, 10 min |
All |
Ambilabs 2-Win |
Nephelometer |
Research-grade |
Derived by LRAPA |
1 min |
Cedar Creek |
Met One BAM-1022 |
Optical |
FEM |
None |
1 h |
Both wildfires |
Met One BAM-1020 |
Optical |
FEM |
None |
1 h |
Monument fire |
2.5.1 PurpleAir PA-II sensors.
For the Konza Prairie prescribed burning, we temporarily deployed four PurpleAir PA-II sensors. Data was retrieved manually via the SD card (80 s resolution). For the wildfire events, we retrieved open-access historical data (2 min or 10 min resolution) from the PurpleAir server using their Application Programming Interface (API). PurpleAir sensors of interest were identified using a bounding box query (i.e., if they were within a specified distance of the VAMMS route). We used a different distance threshold for each evaluation: within 100 m for the Konza Prairie prescribed burns, 400 m for the Cedar Creek fire, and 1500 m for the Monument fire. The threshold values were selected to increase with the size of the fire and impacted area and the spatial extent of the VAMMS monitoring area.
2.5.2 Research-grade instruments.
For the Cedar Creek fire, upon request, LRAPA provided 1 min resolution data from an Ambilabs 2-Win Two Wavelength Integrating nephelometer that runs in parallel to the on-site regulatory monitor at the Oakridge, OR AQMS.
2.5.3 Reference-grade stationary monitors.
Regulatory PM2.5 concentration data from FEM instruments were obtained via AirNow-Tech, a password-protected website for U.S. air quality data (https://www.airnowtech.org/). The Oakridge, OR (AQMS #410392013) and Redding, CA (AQMS #060890004) air quality monitoring stations had BAM-1022 Beta Attenuation Mass Monitors (Met One Instruments). The Weaverville, CA (AQMS #061050002) site had a BAM-1020 Beta Attenuation Mass Monitor (Met One Instruments), the previous generation instrument. The BAM-1020 actively samples for 42 minutes of each reported hourly measurement (to allow for filter replacement), while the BAM-1022 samples for 59 minutes.32 The BAM-1022 has been found to be a reliable instrument even at concentrations reflective of wildfire conditions, with a measurement accuracy of 88.6% compared to the filter-based federal reference method during controlled chamber burns.32
2.6 Corrections
Data shown in the text were corrected using the following approaches for each instrument. Details for each, including the form of the equations and evaluation results, are given in Section 3 of the ESI.†
2.6.1 VAMMS.
To facilitate rapid data interpretation, the pDR-1500 PM2.5 measurement was adjusted by the microprocessor in real time using a linear adjustment factor of 0.53 developed for California wildfire smoke.33 The corrected pDR-1500 PM2.5 data were compared to the blank-corrected integrated filter mass concentrations derived from the laboratory evaluation and deployments and were found to provide comparable results (Fig. S6 in Section 3 of the ESI†).
2.6.2 PurpleAir.
We only used data designated as ‘outdoor’ to identify sensors believed to be deployed outside in ambient conditions. We required that the difference of the A and B [cf = 1] channels be <70% or <5 μg m−3 at the highest time resolution available, a data quality assurance step described in detail elsewhere.34 If the measurement met this requirement, then the mean of both channels was taken to obtain one value for each timestamp. We then compared the performance of two wildfire-smoke specific correction equations,35,36 both of which use the [cf = 1] data, as the authors stated it is more strongly correlated to reference monitors over the full range of concentrations considered.34,36 We decided to use the Holder et al. (2020)35 smoke correction for all PurpleAir data in the Results section as we found it to be the best available existing correction for our data sets. However, we observed that smoke-corrected data underestimated the FEM at ambient concentrations exceeding 600 μg m−3 (Fig. S7†).
2.6.3 Ambilabs nephelometer.
LRAPA corrected the real-time nephelometer data using data from the on-site FEM. The light scattering coefficient from the nephelometer was linearly fit to the FEM concentration using 24 h averaged data from 2017 to 2022 to obtain corrected PM2.5 concentration data in units of μg m−3. The agency develops and uses two corrections, one for wildfire smoke (used from June to September or October, depending on when fire season ends) and another for ambient, non-fire conditions (the rest of the year). The data used in this analysis were corrected by LRAPA using the wildfire correction (personal communication, 2/8/2023).
2.7 Data processing
2.7.1 Temporal alignment and averaging.
Data from collocated instruments were aligned using the highest time resolution available. For the large chamber experiment, the time series concentration trends from each instrument were visually compared to confirm alignment. For the mobile and stationary comparisons during the fires, we used only the timestamp from each instrument to align the datasets. The timestamp from the real-time clock in the VAMMS was updated each time the VAMMS was turned on and the GPS obtained a lock. Since we only used data that had a GPS lock, we do not expect clock drift to be an issue for the VAMMS data. Similarly, the timestamps for the PurpleAir sensors on the open-access, online database were synced to the system time from the server. Given this, we do not expect there to be a time lag between timestamps from the VAMMS and online PurpleAir instruments. For data obtained locally from the SD card of the PurpleAir for the Konza Prairie experiments, the sensors were not connected to the internet during data collection. Instead, we checked the timestamps post-deployment during the large chamber experiments to quantify the difference between a VAMMS with a GPS lock and each PurpleAir. We used these time differences (230 to 360 s lags, depending on the PurpleAir) to adjust the Konza Prairie timestamps in post-processing to ensure the integrity of the timestamps for the PurpleAir SD card data.
Given differences in the sampling rates of the instruments, we resampled (or averaged) the datasets as needed. We did this by creating a reference timestamp at the target resolution (i.e., 1 min, 10 min, 1 h) and averaged all data within the specified interval for each instrument. The timestamp represents the beginning of the averaging period. We applied a 75% completeness requirement when resampling the PurpleAir and nephelometer data sets (i.e., at least 45 minutes of data were required to obtain a 60 min average, and so on). We used a less stringent 25% completeness requirement when resampling the 1 s VAMMS data to 1 min, given the instantaneous nature of these high-resolution sampling rates. Time-alignment and resampling were performed in Igor Pro (v8).
2.7.2 “Approximate AQI”.
Throughout the manuscript, data are colored using the approximate color scheme and upper and lower PM2.5 concentration bounds of the AQI categories for PM2.5 (defined in Table S6 in Section 4 of the ESI†). We opted for this as we believe the familiarity and intuitiveness of the AQI scale improves the readability of the figures and simplifies the discussion around semi-quantitative agreement between different instruments. Note that we use the same concentration ranges, colors, and categories indiscriminately for every time resolution (i.e., 1 s, 1 min, 2 min, 1 h, etc.). To distinguish our categorization from the true AQI based on a 24 hours averaging period, we refer to this as the “approximate AQI” throughout the manuscript. The formal recommendations and health effects associated with each AQI category are not directly applicable to the higher time-resolution values presented in this analysis.
2.7.3 Assessment metrics.
To assess the VAMMS, we used the performance testing metrics suggested for fine particulate matter air sensors for use in ambient, outdoor, fixed site, non-regulatory supplemental and informational monitoring applications:37 the COV, normalized root mean square error (nRMSE), the coefficient of determination (R2), and the intercept (b) and slope (m) linear regression coefficients. Duvall et al. (2021)37 also suggests target values for each metric: COV and nRMSE < 30%, R2 > 0.70, m = 1.0 ± 0.35, and b = 0 ± 5 μg m−3. We reference these target values to provide a general indication of performance, but do not use them as definitive threshold values meant to endorse or disqualify a sensor for use.
In Section 3.4, we calculate the percent difference between high time resolution (1 min, 2 min, 5 min, and 10 min) concentration measurements within an hour and the mean 1 h concentration for that given hour as:
where

is the 1 h averaged concentration value and

is the 1 min, 2 min, 5 min, or 10 min averaged concentration value.
3 Results and discussion
3.1 Performance during controlled chamber experiments
The gravimetric filter concentration (cumulative from two consecutive smoke injection and decay experiments) was 8.19 μg m−3, and the mean pDR-1500 concentration was 14.6 μg m−3, suggesting a linear adjustment factor of 0.56. This is comparable to the Delp and Singer (2020)33 wildfire smoke correction value for the pDR-1500 (0.53). Given this, we opted to use the Delp and Singer (2020)33 value to correct all VAMMS pDR-1500 data as their correction factor was developed using real wildfire smoke over a wider concentration range and for a longer period. The Delp and Singer (2020)33 corrected VAMMS units were accurate (mean RMSE < 3 μg m−3) compared to the gravimetric mass and showed high precision (COV = 8.4%) across the four instruments. The corrected VAMMS data also showed little bias (mean slope = 0.93 and intercept = 0.87 μg m−3) relative to the gravimetric filter-corrected data.
3.2 Performance during large wildfire conditions
To provide a more representative view of instrument performance outside of the controlled conditions of the laboratory experiments, we retroactively assessed the performance of the VAMMS during two wildfires using additional instruments available near the field sites.
3.2.1 Cedar Creek fire.
To evaluate the VAMMS at high time resolution, we identified a subset of measurements when the VAMMS was within 400 m of the Oakridge AQMS at the Willamette Activity Center. In each case (n = 15 passages over 8 days), the VAMMS typically passed within range of the site for a few minutes at a time. For each passage, we compared the 1 min averaged VAMMS value to the nearest 1 min nephelometer value (N = 23) and to the nearest 10 min averaged PurpleAir value (N = 15). For context, we also compared all three higher time resolution measurements to the 1 h averaged regulatory value from the FEM. Fig. 1 shows the time series for all four instruments. The complete time series for the VAMMS and regulatory data are shown, but data points for the PurpleAir and nephelometer are only shown when the VAMMS was within 400 m of them. We opt to show the full timeseries from the regulatory FEM monitor, even when the VAMMS is not in the immediate vicinity, as that measurement formally represents the local and regional conditions for the area. During the four VAMMS sampling runs shown in Fig. 1, the closest FEM monitor was at the Oakridge site, except between 10
:
00 and 11
:
30 PM UTC on 10/10/22 (Fig. 1d) when the VAMMS travelled roundtrip northwest toward Eugene and Springfield, which have their own regulatory stations (data from those monitors are not shown in Fig. 1).
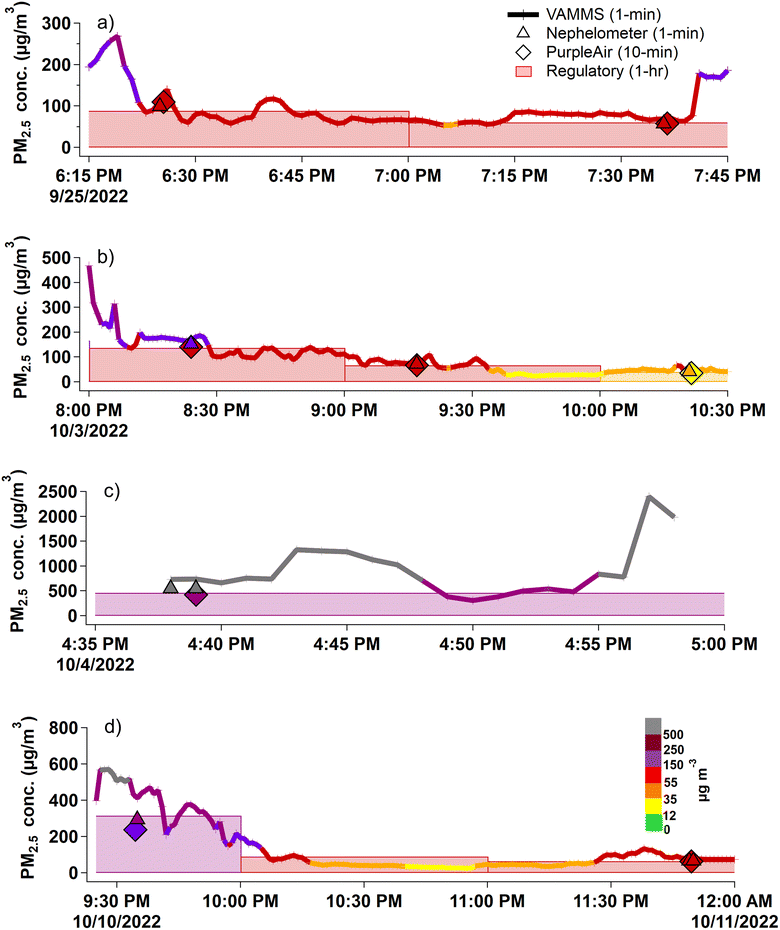 |
| Fig. 1 Timeseries of VAMMS (1 min), nephelometer (1 min), PurpleAir (10 min), and BAM 1022 (60 min) PM2.5 measurements at the Oakridge, OR air quality monitoring station during the Cedar Creek fire for four sampling days (a–d), colored by the corresponding “approximate AQI” category. Data from the nephelometer and PurpleAir are shown only when the VAMMS was within 400 m of them. The timestamp is given in universal coordinated time. | |
Eight of the fifteen VAMMS passages are shown in Fig. 1 and the remaining seven are shown in Fig. S9.† Including all instances where the VAMMS passed by the Oakridge AQMS, in 9 out of 15 passages (∼60%), all four instruments agreed in “approximate AQI” category (displayed as the color in Fig. 1) and the relative difference between the VAMMS PM2.5 concentration and the corresponding concentration from the other instruments was <20% on average. Five of the six remaining passages where the instruments did not agree in “approximate AQI” category were “edge cases” (e.g., Fig. 1b and d), where concentrations were near an “approximate AQI” concentration breakpoint (i.e., at 12, 35, 55 μg m−3etc.) and the percent difference between instruments (24% on average) was comparable to the passages when all the instruments were in agreement. In the remaining instance (Fig. 1c), the VAMMS and nephelometer 1 min measurements differed by almost 200 μg m−3 (VAMMS = 730 μg m−3, nephelometer = 540 μg m−3, mean n = 2) while the smoke-corrected PurpleAir (10 min) and BAM-1022 (1 h) reported lower mean concentrations around 470 μg m−3. There are a few possible explanations for this discrepancy. Firstly, the two 1 min VAMMS data points for this passage were collected within the first three minutes of the VAMMS being turned on. It could be that the pDR-1500 was still powering up and equilibrating when these data points were recorded. Though we expect any ‘start-up’ effect to be minimal, the extreme PM2.5 levels (>500 μg m−3) experienced immediately upon start-up in this instance may explain the poor comparison between the VAMMS and the other measurements. It is also possible that there was residual particulate matter in the sampling line. Additionally, the 1 min stationary nephelometer data at the AQMS show that the two 1 min VAMMS measurements were collected during a 10 minute window when concentrations peaked over the hour. At the start of the hour, concentrations were near 340 μg m−3 and steadily rising. They peaked around 550 μg m−3 about 40 minutes into the hour, coinciding with the two minutes of VAMMS sampling. Levels then began reducing, back to around 460 μg m−3 by the end of the hour. Taken together, the conditions present in this example may explain why the 1 h BAM 1022 value (460 μg m−3) was more than 250 μg m−3 lower than the VAMMS and 100 μg m−3 lower than the nephelometer. Despite the discrepancy, all instruments suggested ambient concentrations in the region were ‘Hazardous’ or higher during this period, which would result in similar public health guidance (i.e., to remain indoors).
3.2.2 Monument fire.
In this section, we evaluated the VAMMS compared to the existing stationary monitoring network (regulatory and open-access PurpleAir) over a large (>520
000 km2) area. Fig. 2 shows a map of the Shasta Trinity County, CA region including the locations of two regulatory sites (Weaverville and Redding) and twenty-seven PurpleAir sites. Fig. 2a contains markers for the 2 min VAMMS data collected over 22 days during the Monument wildfire incident. The PurpleAir sensors were required to be within 1500 m of the VAMMS to be included, but most were closer (median distance = 400 m). GPS coordinates, ID number, name, number of data points, and distance from the VAMMS for each of the PurpleAir sensors are given in Table S5 in Section 3 of the ESI.† As a specific example, Fig. 2b shows data from all instruments on just one day of sampling (08/11/2021), colored by “approximate AQI” category.
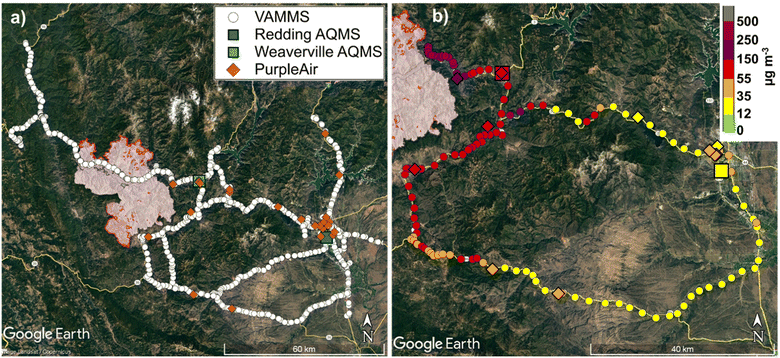 |
| Fig. 2 Map of VAMMS measurement locations (a) for the entire deployment period of the Monument fire and (b) on 08/11/2021 only. VAMMS data are shown as small circles, the twenty-seven PurpleAir within 1500 m of the VAMMS route are shown as diamond markers, and the two nearby regulatory air quality monitoring stations (AQMS) are shown as large squares. The border of the Monument fire on 08/11/2021 is shown in red (infrared map, source: InciWeb). In panel (b), the data are colored by PM2.5 concentration. VAMMS and PurpleAir data are 2 min averaged, while the regulatory AQMS data are 1 h averaged. PurpleAir and regulatory AQMS data are shown at the time of the VAMMS passing by. Image source: Google Earth Pro Version 7.3.4.8248. Shasta-Trinity County, USA. Accessed: April 19, 2023. © Google Earth 2023. | |
Fig. 3 shows a scatter plot of the PM2.5 concentration from the mobile VAMMS and the stationary high-time resolution sensors for both the Cedar Creek and Monument fire comparisons. Fig. 3a compares the VAMMS to the nephelometer at the Oakridge AQMS for the Cedar Creek fire and Fig. 3b compares the VAMMS to the open-access PurpleAir network for the Monument fire. For the Cedar Creek fire, the VAMMS met most target values for performance compared to the nephelometer. The VAMMS data overestimated compared to the nephelometer measurements (slope = 1.2) and though this was generally more pronounced at higher concentrations, it was not universal.
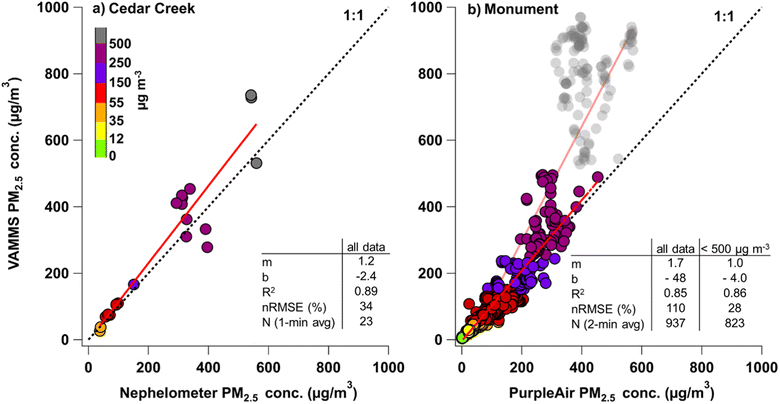 |
| Fig. 3 Scatter plot of the mobile VAMMS compared to (a) the stationary nephelometer at the Oakridge, OR air quality monitoring station and (b) stationary, open-access PurpleAir network (N = 27 sensors) in the Shasta Trinity County, CA area, corrected using the Holder et al. (2020)35 equation. The VAMMS was within 400 m of the stationary monitor for (a) and within 1500 m for (b). Linear regression coefficients are given as y = mx + b, where m is slope and b is intercept. R2 = coefficient of determination, nRMSE = normalized root mean square error, N = number of data points. A one-to-one line is shown as a black dotted line and the linear fit line is shown as a red, solid line. A second linear fit line for data where the PurpleAir is <500 μg m−3 is shown as a red dashed line in (b). Gray data beyond the “approximate AQI” on (b) are transparent to indicate that the corrected PurpleAir (the reference measurement) data are potentially inaccurate in this high concentration regime. | |
For the Monument fire, statistics-based performance was worse than the Cedar Creek comparison (higher slope, lower intercept, lower R2 and high nRMSE). However, the data agreed well until the upper end of the concentration range (gray points on Fig. 3b). Excluding these data brings all performance metric values within the target ranges and the linear fit approaches the one-to-one line. These high concentration data (>500 μg m−3) were collected over three days at the beginning of the measurement period, near the Weaverville AQMS. The hourly PM2.5 data from the Weaverville BAM-1020 FEM suggest that real ambient concentrations were between 600 and 900 μg m−3 during this period. This is also the concentration range we observed the smoke-corrected PurpleAir data to underestimate FEM concentrations at the Weaverville and Oakridge AQMS during the Monument and Cedar Creek fires, respectively (Fig. S7 and S8a†). Taken together, this implies that the smoke-corrected PurpleAir data in Fig. 3b are underestimating the true ambient concentration. The low-biased PurpleAir data makes the VAMMS data appear to overestimate PM2.5 concentrations, partially explaining the non-linear slope. The impact of anisokinetic sampling on the measured PM2.5 concentration for both these events was estimated to be negligible (Table S2†), so we do not expect that to explain this observation. In all, findings from the Cedar Creek and Monument fire comparisons suggest that the VAMMS was accurate at high-time resolutions even while mobile, at least at concentrations <500 μg m−3. At higher concentrations there is greater uncertainty in the VAMMS accuracy, since the primary FEM reference instruments (e.g., Met One BAM-1020 or BAM-1022), used to evaluate the nephelometer and PurpleAir, are also not formally evaluated to operate in this range.38
3.3 Performance under near-field, prescribed burning conditions
In this section, we assess how the VAMMS performed near a small, prescribed fire by comparing it to stationary PurpleAir sensors in an area without regulatory monitors. The active burning phase of each 3-acre plot lasted only about an hour, during which the plume could be seen, though the plots continued smoldering for several hours after the burn. A map, locations of the temporary stationary PurpleAir monitors, the VAMMS sampling route, and the location and size of the burn plots are shown in Fig. 4. Additional images of the deployment terrain, the plume shape, and details on the burn schedule and conditions are given in Section 6 of the ESI.†
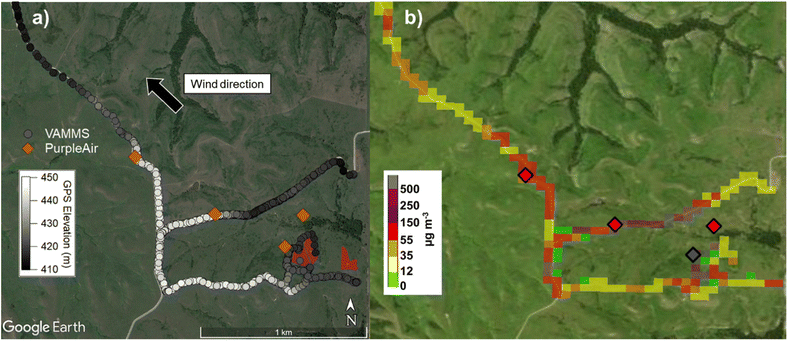 |
| Fig. 4 Map of (a) GPS elevation (10 s average) and (b) maximum PM2.5 concentration from the VAMMS (25 m spatially smoothed maximum) and PurpleAir during the consecutive prescribed burning of three 3-acre plots. The primary wind direction during the burns and the burned area of the three plots (red polygons) is shown on panel (a). These data were collected from 16:05 to 19:45 UTC on 09/15/2021 at the Konza Prairie Biological Station near Manhattan, KS. Image source: (a) Google Earth Pro Version 7.3.4.8248. Konza Prairie Biological Research Station, USA. Accessed: April 19, 2023. © Google Earth 2023. (b) Esri. “NAIP Imagery Hybrid” [basemap]. (Accessed: July 17, 2023). | |
Unlike for the two large wildfires where conditions were relatively stable over several minutes or even hours, we observed rapidly changing plume dynamics within 500 m of the border of these small 3-acre fires. PurpleAir PM2.5 concentrations could change by an order of magnitude in an 80 s period. For context, Fig. S12† shows the time series from each of the four stationary PurpleAir sensors for one of the three burns. The VAMMS passed within 100 m of the PurpleAir for only 6 or 7 seconds at a time and kept moving, such that under these quickly changing conditions, the 80 s mobile mean was not typically comparable to the 80 s stationary mean.
Fig. S13† shows the time series of the instruments when the VAMMS was within 100 m of the nearest stationary PurpleAir (N = 18 passages). For ∼50% of all passages, the two instruments were within about 10 μg m−3 of each other or better and had the same “approximate AQI”. Of those passages, 30% had measurements from both instruments that were nearly identical. For the remaining 50% of passages, the instruments were one or more “approximate AQI” categories different. Ultimately, this meant that the VAMMS and PurpleAir measurements showed poor agreement about half of the time. There are a few explanations for this observation. Firstly, in these conditions, it was possible for the VAMMS to be within 100 m of a PurpleAir sensor but be behind (or upwind) of the plume or be up to 50 m closer to the fire border. This explained some of the passages with the poorest agreements of the two sensors (indicated with text labels on Fig. S13†). Secondly, steep elevation changes (Fig. 4a) and shifting wind conditions contributed to poor agreement with the mid-downwind PurpleAir positioned at the edge of the ridgeline. The road that the VAMMS was travelling on passed by the mid-downwind location and then dropped steeply into the valley below. Depending on the wind conditions, the plume fumigated the valley or was lofted above it, meaning the smoke conditions in the valley floor and at the top of the ridge could vary significantly despite the proximity of the two locations. Finally, we estimated that super-isokinetic sampling conditions resulting from the slow median sampling velocity of this non-road vehicle (∼5 mph) may have biased the VAMMS PM2.5 concentrations low by about 16% (Table S2†).
Though real-time, coincident measurements from the stationary and mobile instruments were not comparable under these conditions, Fig. 4b highlights another use of mobile monitoring data. Given that the VAMMS passed by the PurpleAir sensors multiple times in a short period, instead of comparing each passage, we looked at the 25 m spatially smoothed maximum value for the VAMMS data compared to the maximum value measured by each PurpleAir over the full duration of burning. This presentation provides a more complete picture of the downwind concentrations resulting from the burning activity and circumvents any issues that arise from time misalignments. The near downwind and far downwind locations had the same “approximate AQI”, but for the mid-downwind location on the ridge, the VAMMS measured one “approximate AQI” level higher than the PurpleAir sensor, possibly due to the changing elevation of the VAMMS measurements averaged over that area. The upwind location (not located directly on the VAMMS route like the other PurpleAir sensors) was impacted during one of three burns and was consistent with, though not identical to, the nearest VAMMS measurements.
Given that common plume prediction tools used in prescribed burn decision making, like Vsmoke and BlueSky, predict the maximum value for a given burn over a spatial area, this type of spatially maximized data from the VAMMS could be used to compare to and even evaluate predictions from these tools. The VAMMS data also more clearly captured the impact of lower elevation on increased downwind PM2.5 concentration. Still, temporary stationary monitoring was useful to capture temporal variations in the plume but was limited by longer averaging times. For small, prescribed fires where meteorological conditions are well characterized, the monitoring duration is short, and near-field plume dynamics are highly variable, stationary monitors may better represent concentrations for personnel on the ground, while the mobile monitor can provide data with higher temporal and spatial extent that would be useful for validating smoke plume models.
3.4 Interpretation of high-time resolution measurements
One difficulty in comparing mobile and stationary monitoring data is reconciling the high-time resolution data typical of mobile monitors (1 s or 1 min) with the low-time resolution data typical of the ambient PM2.5 monitoring network (1 h), given the variability inherent in an instantaneous measurement. In this section, we quantify the expected variation that a single high-time resolution measurement has compared to a 1 h averaged measurement, using data from the Oakridge AQMS during the Cedar Creek fire. Since there were only a few instances when the mobile VAMMS passed by the stationary site, we opted to use data from the stationary nephelometer (1 min and 1 h averaged) for this analysis. The goal of this analysis is to give users a quantitative understanding for how representative an instantaneous VAMMS measurement may be and more broadly, how to interpret a high-time resolution measurement compared to a low-time resolution measurement. As a caveat, the findings from this section are not expected to be universally applicable. For example, the results could reasonably be extended to interpret measurements from other large wildfires with persistent, regional impacts but cannot be expected to hold for small, volatile fires where local and regional conditions are likely to change much more rapidly.
For each of the hours that included a VAMMS passage (N = 14 hours), we separated the hours by ‘variable’ or ‘stable’ conditions using the COV of the 1 min nephelometer measurements for each given hour. If the COV was less than 15% for the hour, we considered the conditions to be ‘stable’. For this subset of the data, ‘variable’ and ‘stable’ conditions were equally likely (7 out of 14 hours each). Fig. 5a and c show the PM2.5 concentration, averaged in four different intervals (1 min, 2 min, 5 min, and 10 min), for the ‘stable’ and ‘variable’ hours, respectively. Fig. 5b and d shows the percent difference of the interval measurements compared to the 1 h mean concentration for each hour (equation given in Section 2.7).
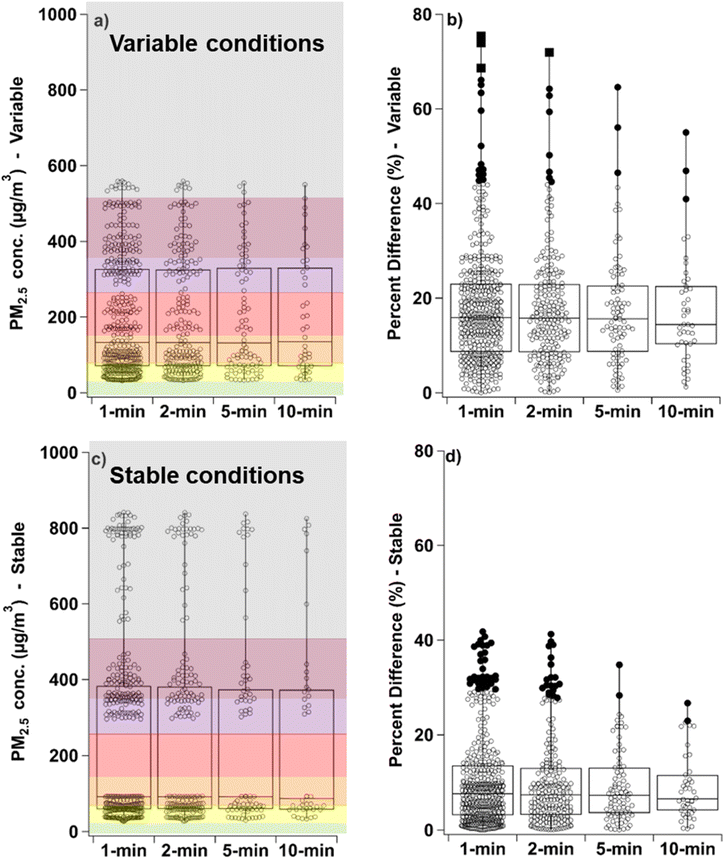 |
| Fig. 5 Box plots of the PM2.5 concentration from the nephelometer at the Oakridge, OR air quality monitoring station during (a) variable and (c) stable conditions. The percent difference of the nephelometer concentration measurement compared to the 1 h mean is shown for each set of conditions and each interval (b and d). For the boxes, the median is the line, the top and bottom of the box are the 75th and 25th quartiles, the whiskers are the minimum and maximum value. Outliers are shown as dark circles and far outliers as dark squares. The boxes are shaded using “approximate AQI” breakpoints and colors. These data were collected between Sep 25 and Oct 12, 2022, during the Cedar Creek wildfire. | |
This analysis suggests that under ‘variable’ conditions, the percent difference for a 1 min measurement could be as high as 75% but was most frequently around 15%. For ‘stable’ conditions, the median percent difference for the 1 min measurements was around 8% and the maximum was around 40%. Unless concentrations were near the breakpoint of an AQI level, or the air quality were good to moderate, a difference of this magnitude would be unlikely to impede accurate characterization of the current air quality conditions. Additional sampling (2, 5, or 10 min) had little impact on reducing the median difference for either ‘variable’ or ‘stable’ conditions, though after 10 min the far outliers (3 × interquartile range) were lower by about 40%.
For the 15 passages of the VAMMS (Fig. 3), the median percent difference for the 1 min VAMMS measurement was 20% compared to the corresponding 1 h mean from the nephelometer, under both ‘variable’ and ‘stable’ conditions. This is higher than the 15% and 8% median difference for ‘variable’ and ‘stable’ conditions, respectively, predicted by the intra-instrument comparison shown in Fig. 5. However, the observed difference included all VAMMS passages, including those identified in Section 3.2 that may have been impacted by starting the VAMMS up under high concentrations. In any case, this suggests it would be more realistic to expect that a 1 min measurement from the VAMMS will most often be between 15-20% different from the 1 h mean concentration in the area (within 400 m in this case). We surmise that 20% difference (or better) is acceptable if the goal is to obtain a semi-quantitative indicator of air quality conditions. Though if the user's goal is to inform a decision related to public health, it would be prudent to sample for up to 10 min in ‘stable’ conditions, and potentially even longer (up to 30 min) in ‘variable’ conditions.
The VAMMS sampling resolution is 1 s, so it is pertinent to consider how a 1 s measurement compares to a 1 min averaged measurement for cases where users are interpreting raw data before averaging. For the ‘stable’ and ‘variable’ periods, we compared the 1 s measurements from the VAMMS to the 1 min mean from the VAMMS for each minute that the VAMMS passed by the Oakridge, OR AQMS. The percent difference between the 1 s measurements and the corresponding 1 min mean for each given minute is shown in Fig. S16 in Section 7 of the ESI.† For both ‘stable’ and ‘variable’ periods, the median percent difference was less than 5%.
3.5 Comparison with urban mobile monitoring approaches
We determined some approaches taken in other mobile monitoring studies23,39 (e.g., accounting for the sensor lag period29,40 or self-pollution) to likely be inapplicable to our use cases. For example, the sensor lag period is the time that it takes for the sampled air to reach the instrument and be measured and recorded. For stationary or low velocity monitoring, a few second lag period would be insignificant for interpreting VAMMS data. However, at highway speeds, the spatial error introduced by an unaccounted-for lag could be more significant under specific circumstances resulting from rapidly changing concentrations, such as sampling along a steep elevation gradient. Otherwise, sensor lag is primarily an issue for studies measuring multiple parameters requiring the alignment of timestamps from multiple instruments, accounting for differences in sampling resolution and averaging interval, as well as real differences between the instrument's internal real-time clocks. The VAMMS is typically used independently, so in most cases we expect a few-second lag period to be insignificant for users interpreting these data sets.
In urban mobile studies, ‘self-pollution’ refers to the sampling equipment capturing emissions from the monitoring vehicle itself. For our application, we expect that any particle pollution from the vehicle itself is negligible compared to the PM2.5 emitted by wildland fire in the target region.
As for spatial fidelity (i.e., the ability of a single measurement to meaningfully represent concentrations in a specific area),24,25,41 given that our goal is to assess real-time concentrations and provide actionable air quality information, we surmise that conducting repeated runs, intended to reduce spatial uncertainty, is of limited value for this application. Conversely, repeated runs may be useful to identify persistent trends in a region, such as the impact on smoke concentrations due to an atmospheric inversion lifting each morning of the monitoring period.
3.6 Limitations and future work
There are some limitations to this dataset and analysis. For example, during post-deployment quality assurance checks, we noted that the probe was partially clogged on the VAMMS returned from the Cedar Creek fire, causing the pDR-1500 to slightly underestimate ambient concentrations when sampling through the probe versus open sampling (i.e., no sampling probe or line attached to the inlet). Presently, we are unable to quantify the impact that a heavy loaded filter or clogged probe or sampling line has on the pDR-1500 measurements. Though given the good agreement between the pDR-1500 and corrected nephelometer at the Oakridge AQMS throughout the sampling period, we suspect that this effect was small and did not compromise the integrity of the VAMMS measurements for this data set or analysis. Future research will focus on identifying the appropriate maintenance schedules for heavy smoke conditions to avoid degradation in performance of the pDR-1500. However, we did observe in the Cedar Creek evaluation that data collected within 2–3 minutes of starting up the VAMMS under extreme smoke conditions may have contributed to disagreement between the instruments, indicating the pDR-1500 may require an equilibration period under those conditions. Though it is possible that a local source (e.g., another vehicle) could explain this observation, since the VAMMS was started up in a parking lot in this instance. In any case, if this observation is repeatable, we plan to add a post-deployment quality check step of removing data collected within a given time period of start-up. In addition, we plan to explore the linearity and accuracy of the pDR-1500 response in extreme wildland fire smoke conditions (600 to >2500 μg m−3). With the available data sets (i.e., large chamber, Monument wildfire), we were unable to determine if and to what degree the VAMMS was overestimating concentrations in this range. The pDR-1500 is reported to have a concentration range up to 400 mg m−3 for SAE/ISO Fine Test Dust (Thermo Fisher, 2019) but future research will explore if and how the maximum concentration range is impacted when sampling other types of aerosols.
Variable sampling velocity and the resultant inability to maintain isokinetic conditions are notable limitations of sampling with the VAMMS. This could result in the VAMMS pDR-1500 underestimating the true PM2.5 mass concentration. For the on-road wildfire datasets (Cedar Creek and Monument fires), this effect was negligible or modest. Given the good agreement we observed between the VAMMS and stationary monitors (Fig. 3), and since the median vehicle velocity for these test cases was close to the isokinetic sampling velocity (Table S1†), we expect this effect to have minimal impact for users interpreting typical on-road VAMMS data sampling wildfire aerosol. On the other hand, the bias estimated from the Konza Prairie dataset suggested that VAMMS data collected at very low speeds (5 mph) may lead to underestimates of the mass concentration. Niche research uses for the VAMMS, such as sampling via non-road all-terrain vehicles or boats, will require users to consider and quantify the impact of anisokinetic sampling before further analysis. On a similar note, by design we were unable to anticipate or characterize the vehicle profile which is constantly changing for each VAMMS deployment. Different vehicle heights and shapes can impact flow streamlines42 and previous work has shown that mobile sensor performance can also be impacted by sampler height, and whether or not it is deployed inside an enclosure.23 Since we are unable to impose restrictions on the users sampling velocity or vehicle type to reduce these effects, future research will aim to better characterize and quantify the potential impacts of these factors on the collected data.
Lastly, we determined that high-time resolution measurements had a median difference of around 20% compared to 1 h measurements during the Cedar Creek wildfire. Future research should look at how this finding translates to other instruments (which may have variable sampling rates and rely on different optical measurement methods), and potentially other fires and smoke conditions. Though we expect the findings for smoke conditions to be similar if the same determination for ‘variable’ and ‘stable’ conditions are maintained, it is possible that differences in source fuels from fires in different regions could impact the interpretation of high-time resolution data compared to the nearest FEM or FRM monitor.
4 Conclusion
We found that mobile measurements from the VAMMS were comparable to stationary measurements under real wildfire conditions, suggesting that the VAMMS can collect actionable data in impacted regions for assisting in emergency response activities. However, future work should aim to increase functionality (such as incorporating a real-time indicator display) and improve user access to data processing and visualization tools, such as allowing users to apply data quality control steps and select specific time periods and/or locations for further analysis.
In general, this work highlights the value of using portable sensor technologies to address some of the monitoring challenges presented specifically by dynamic wildland fire conditions. For example, mobile monitoring can assist in identifying the most impacted areas or sites that would benefit from additional temporary stationary monitoring. Smoke plume dynamics depend on many factors but expansive plumes, such as those from large wildfires, are likely to be stable over longer periods of time. Mobile monitoring is prime for these conditions, as the user can have more assurance that the location to location changes they observe during their route are representative of real spatial differences and not just temporal changes in the plume. Future research efforts should explore how portable sensors can be used to characterize and improve our understanding and ability to model smoke flow plumes in regions with steep and complex or mountainous terrain.
We also found supportive evidence for combining mobile monitoring with stationary data where possible. For small plumes, such as the Kansas Prairie experiments, the plume was observed to vary over short time intervals and small spatial scales, highlighting the value of temporary stationary monitoring. Proper site selection and the time required for deployment and retrieval are non-trivial factors in temporary stationary monitoring, however if the fire and impacted area are small, only a few monitors may be needed to create a network sufficiently dense to map and create a timelapse of the plume, which could also help to inform fine-scale plume modelling efforts.
Author contributions
AH, WM, and GH were responsible for funding acquisition and project administration. AH, GH, and ASB were responsible for conceptualization and investigation. WM was responsible for resources and software for the design of the VAMMS. All authors contributed to the methodology of the study. ASB was responsible for data curation, formal analysis, validation, and visualization under the supervision of AH and APG. ASB wrote the original draft. AH, APG, and GH provided review and editing.
Conflicts of interest
There are no conflicts of interest to declare.
Acknowledgements
The views expressed in this article are those of the authors and do not necessarily represent the views or policies of the US Environmental Protection Agency. This research was supported, in part, by an appointment to the Research Participation Program for the US Environmental Protection Agency, Office of Research and Development, administered by the Oak Ridge Associated Universities through an interagency agreement between the US Department of Energy and Environmental Protection Agency. This research was also supported, in part, by NSF INTERN Supplement Award #1923568: CNH-L: Energy Transitions and Environmental Change in East and Southern Africa's Coupled Human, Terrestrial, and Atmospheric Systems. We would like to thank the Air Resource Advisors that participated in the WSMART program and collected the data used in this analysis including Ali Kamal, Josh Hall, Tod Johnston, Ari Sarzotti, Beth Anderson, and Jeremy Ash. In addition, Pete Lahm (US Forest Service), who leads the Interagency Wildland Fire Air Quality Response Program (IWFAQRP), is appreciated for his role facilitating WSMART equipment loans to ARAs. We would also like to thank Kansas State University and the Konza Prairie Biological Station staff for their assistance and support facilitating data collection on their property. Finally, we thank Lance Giles of the Lane Regional Air Protection Agency in Springfield, Oregon for providing information on the Oakridge, OR monitoring site and the 1 min Ambilabs 2Win nephelometer data set.
References
- D. A. Jaffe, S. M. O'Neill, N. K. Larkin, A. L. Holder, D. L. Peterson, J. E. Halofsky and A. G. Rappold, Wildfire and prescribed burning impacts on air quality in the United States, J. Air Waste Manage. Assoc., 2020, 70, 583–615 CrossRef CAS PubMed.
- W. E. Cascio, Wildland fire smoke and human health, Sci. Total Environ., 2018, 624, 586–595 CrossRef CAS PubMed.
- H. Youssouf, C. Liousse, L. Roblou, E.-M. Assamoi, R. O. Salonen, C. Maesano, S. Banerjee and I. Annesi-Maesano, Non-Accidental Health Impacts of Wildfire Smoke, Int. J. Environ. Res. Public Health, 2014, 11, 11772–11804 CrossRef PubMed.
- J. C. Liu, G. Pereira, S. A. Uhl, M. A. Bravo and M. L. Bell, A systematic review of the physical health impacts from non-occupational exposure to wildfire smoke, Environ. Res., 2015, 136, 120–132 CrossRef CAS PubMed.
- C. E. Reid, M. Brauer, F. H. Johnston, M. Jerrett, J. R. Balmes and C. T. Elliott, Critical Review of Health Impacts of Wildfire Smoke Exposure, Environ. Health Perspect., 2016, 124, 1334–1343 CrossRef PubMed.
- M. Burke, A. Driscoll, S. Heft-Neal, J. Xue, J. Burney and M. Wara, The changing risk and burden of wildfire in the United States, Proc. Natl. Acad. Sci. U. S. A., 2021, 118, e2011048118 CrossRef CAS PubMed.
- A. L. Westerling, Increasing western US forest wildfire activity: sensitivity to changes in the timing of spring, Philos. Trans. R. Soc. London, Ser. B, 2016, 371, 20150178 CrossRef PubMed.
-
O.U.S. EPA, Air Data Basic Information, https://www.epa.gov/outdoor-air-quality-data/air-data-basic-information, accessed 12 May 2023 Search PubMed.
-
U.S. EPA, Using Air Quality Index | AirNow.gov, https://www.airnow.gov/aqi/aqi-basics/using-air-quality-index, accessed 12 May 2023 Search PubMed.
-
U.S. EPA, Integrated Science Assessment (ISA) for Particulate Matter (Final Report), U.S. Environmental Protection Agency, Office of Research and Development, National Center for Environmental Assessment, Washington, DC, 2019 Search PubMed.
- Y. Xi, D. B. Richardson, A. V. Kshirsagar, T. J. Wade, J. E. Flythe, E. A. Whitsel, G. C. Peterson, L. H. Wyatt and A. G. Rappold, Effects of short-term ambient PM2.5 exposure on cardiovascular disease incidence and mortality among U.S. hemodialysis patients: a retrospective cohort study, Environ. Health, 2022, 21, 33 CrossRef CAS PubMed.
- S. E. Cleland, L. H. Wyatt, L. Wei, N. Paul, M. L. Serre, J. J. West, S. B. Henderson and A. G. Rappold, Short-Term Exposure to Wildfire Smoke and PM2.5 and Cognitive Performance in a Brain-Training Game: A Longitudinal Study of U.S. Adults, Environ. Health Perspect., 2022, 130(6) DOI:10.1289/EHP10498.
- M. M. Johnson and F. Garcia-Menendez, Uncertainty in Health Impact Assessments of Smoke From a Wildfire Event, GeoHealth, 2022, 6, e2021GH000526 CrossRef PubMed.
- K. E. Kelly, W. W. Xing, T. Sayahi, L. Mitchell, T. Becnel, P.-E. Gaillardon, M. Meyer and R. T. Whitaker, Community-Based Measurements Reveal Unseen Differences during Air Pollution Episodes, Environ. Sci. Technol., 2021, 55, 120–128 CrossRef CAS PubMed.
- D. V. Mallia, A. K. Kochanski, K. E. Kelly, R. Whitaker, W. Xing, L. E. Mitchell, A. Jacques, A. Farguell, J. Mandel, P.-E. Gaillardon, T. Becnel and S. K. Krueger, Evaluating Wildfire Smoke Transport Within a Coupled Fire-Atmosphere Model Using a High-Density Observation Network for an Episodic Smoke Event Along Utah's Wasatch Front, J. Geophys. Res.: Atmos., 2020, 125, e2020JD032712 CrossRef CAS.
-
O.US EPA, Wildfire Smoke Air Monitoring Response Technology (WSMART) Pilot, https://www.epa.gov/air-sensor-toolbox/wildfire-smoke-air-monitoring-response-technology-wsmart-pilot, accessed 9 January 2023 Search PubMed.
-
J. L. Peterson, M. C. Pitrolo, D. W. Schweizer, R. L. Striplin, L. H. Geiser, S. M. Holm, J. D. Hunter, J. M. Croft, L. M. Chappell, P. W. Lahm, G. E. Amezquita, T. J. Brown, R. G. Cisneros, S. J. Connolly, J. E. Halofsky, E. L. Loudermilk, K. M. Navarro, A. L. Nick, C. T. Procter, H. C. Provencio, T. Pusina, S. L. Stone, L. W. Tarnay and C. D. West, in Wildland Fire Smoke in the United States: A Scientific Assessment, ed. D. L. Peterson, S. M. McCaffrey and T. Patel-Weynand, Springer International Publishing, Cham, 2022, pp. 239–277 Search PubMed.
-
O US EPA, EPA Expands Air Monitoring Capabilities to Support Wildfire-Impacted States, Tribes and Their Frontline Firefighters, https://www.epa.gov/sciencematters/epa-expands-air-monitoring-capabilities-support-wildfire-impacted-states-tribes-and, accessed 9 January 2023 Search PubMed.
-
IWFAQRP – Smoke Monitoring, https://www.wildlandfiresmoke.net/home/smoke-monitoring, accessed 9 March 2023 Search PubMed.
- L. Pirjola, A. Virkkula, T. Petäjä, J. Levula, J. Kukkonen and M. Kulmala, Mobile ground-based measurements of aerosol and trace gases during a prescribed burning experiment in boreal forest in Finland, Boreal Environ. Res., 2015, 20, 105–119 Search PubMed.
- F. Y. Majluf, J. E. Krechmer, C. Daube, W. B. Knighton, C. Dyroff, A. T. Lambe, E. C. Fortner, T. I. Yacovitch, J. R. Roscioli, S. C. Herndon, D. R. Worsnop and M. R. Canagaratna, Mobile Near-Field Measurements of Biomass Burning Volatile Organic Compounds: Emission Ratios and Factor Analysis, Environ. Sci. Technol. Lett., 2022, 9, 383–390 CrossRef CAS.
-
N. Tam and C. Adams, Characterization of Air Quality during the 2016 Horse River Wildfire Using Permanent and Portable Monitoring, Government of Alberta, Ministry of Environment and Parks, 2019 Search PubMed.
- W. Mui, B. Der Boghossian, A. Collier-Oxandale, S. Boddeker, J. Low, V. Papapostolou and A. Polidori, Development of a Performance Evaluation Protocol for Air Sensors Deployed on a Google Street View Car, Environ. Sci. Technol., 2021, 55, 1477–1486 CrossRef CAS PubMed.
- D. R. Ranasinghe, W. Choi, A. M. Winer and S. E. Paulson, Developing High Spatial Resolution Concentration Maps Using Mobile Air Quality Measurements, Aerosol Air Qual. Res., 2016, 16, 1841–1853 CrossRef CAS.
- J. Van den Bossche, J. Peters, J. Verwaeren, D. Botteldooren, J. Theunis and B. De Baets, Mobile monitoring for mapping spatial variation in urban air quality: Development and validation of a methodology based on an extensive dataset, Atmos. Environ., 2015, 105, 148–161 CrossRef CAS.
- M. Van Poppel, J. Peters and N. Bleux, Methodology for setup and data processing of mobile air quality measurements to assess the spatial variability of concentrations in urban environments, Environ. Pollut., 2013, 183, 224–233 CrossRef CAS PubMed.
- Z. Li, J. C. H. Fung and A. K. H. Lau, High spatiotemporal characterization of on-road PM2.5 concentrations in high-density urban areas using mobile monitoring, Build. Environ., 2018, 143, 196–205 CrossRef.
- J. S. Apte, K. P. Messier, S. Gani, M. Brauer, T. W. Kirchstetter, M. M. Lunden, J. D. Marshall, C. J. Portier, R. C. H. Vermeulen and S. P. Hamburg, High-Resolution Air Pollution Mapping with Google Street View Cars: Exploiting Big Data, Environ. Sci. Technol., 2017, 51, 6999–7008 CrossRef CAS PubMed.
- H. L. Brantley, G. S. W. Hagler, E. S. Kimbrough, R. W. Williams, S. Mukerjee and L. M. Neas, Mobile air monitoring data-processing strategies and effects on spatial air pollution trends, Atmos. Meas. Tech., 2014, 7, 2169–2183 CrossRef.
- G. S. W. Hagler, M.-Y. Lin, A. Khlystov, R. W. Baldauf, V. Isakov, J. Faircloth and L. E. Jackson, Field investigation of roadside vegetative and structural barrier impact on near-road
ultrafine particle concentrations under a variety of wind conditions, Sci. Total Environ., 2012, 419, 7–15 CrossRef CAS PubMed.
- A. L. Holder, H. S. Halliday and L. Virtaranta, Impact of do-it-yourself air cleaner design on the reduction of simulated wildfire smoke in a controlled chamber environment, Indoor Air, 2022, 32, e13163 CrossRef PubMed.
- R. W. Long, S. P. Urbanski, E. Lincoln, M. Colón, S. Kaushik, J. D. Krug, R. W. Vanderpool and M. S. Landis, Summary of PM2.5 Measurement Artifacts Associated with the Teledyne T640 PM Mass Analyzer Under Controlled Chamber Experimental Conditions Using Polydisperse Ammonium Sulfate Aerosols and Biomass Smoke, J. Air Waste Manage. Assoc., 2023, 295–312 CrossRef CAS PubMed.
- W. W. Delp and B. C. Singer, Wildfire Smoke Adjustment Factors for Low-Cost and Professional PM2.5 Monitors with Optical Sensors, Sensors, 2020, 20, 3683 CrossRef PubMed.
- K. K. Barkjohn, B. Gantt and A. L. Clements, Development and application of a United States-wide correction for PM2.5 data collected with the PurpleAir sensor, Atmos. Meas. Tech., 2021, 14, 4617–4637 CrossRef PubMed.
- A. L. Holder, A. K. Mebust, L. A. Maghran, M. R. McGown, K. E. Stewart, D. M. Vallano, R. A. Elleman and K. R. Baker, Field Evaluation of Low-Cost Particulate Matter Sensors for Measuring Wildfire Smoke, Sensors, 2020, 20, 4796 CrossRef PubMed.
- K. K. Barkjohn, A. L. Holder, S. G. Frederick and A. L. Clements, Correction and Accuracy of PurpleAir PM2.5 Measurements for Extreme Wildfire Smoke, Sensors, 2022, 22, 9669 CrossRef PubMed.
-
R. Duvall, A. Clements, G. S. W. Hagler, A. Kamal, L. Vasu Kilaru, L. Goodman, S. G. Frederick, K. Johnson Barkjohn, I. VonWald, D. Greene and T. Dye, Performance Testing Protocols, Metrics, and Target Values for Fine Particulate Matter Air Sensors: Use in Ambient, Outdoor, Fixed Site, Non-regulatory Supplemental and Informational Monitoring Applications, U.S. EPA Office of Research and Development, Washington, DC, 2021 Search PubMed.
- G. Hagler, T. Hanley, B. Hassett-Sipple, R. Vanderpool, M. Smith, J. Wilbur, T. Wilbur, T. Oliver, D. Shand, V. Vidacek, C. Johnson, R. Allen and C. D'Angelo, Evaluation of two collocated federal equivalent method PM2.5 instruments over a wide range of concentrations in Sarajevo, Bosnia and Herzegovina, Atmos. Pollut. Res., 2022, 13, 101374 CrossRef CAS PubMed.
- T. Xia, J. Catalan, C. Hu and S. Batterman, Development of a mobile platform for monitoring gaseous, particulate, and greenhouse gas (GHG) pollutants, Environ. Monit. Assess., 2020, 193, 7 CrossRef PubMed.
- M. D. Adams and D. Corr, A Mobile Air Pollution Monitoring Data Set, Data, 2019, 4, 2 CrossRef.
- A. R. Whitehill, M. Lunden, S. Kaushik and P. Solomon, Uncertainty in collocated mobile measurements of air quality, Atmos. Environ. X, 2020, 7, 100080 CAS.
- G. Sivaraj, K. Parammasivam, M. Prasath, P. Vadivelu and D. Lakshmanan, Flow analysis of rear end body shape of the vehicle for better aerodynamic performance, Mater. Today: Proc., 2021, 47, 2175–2181 Search PubMed.
|
This journal is © The Royal Society of Chemistry 2024 |
Click here to see how this site uses Cookies. View our privacy policy here.