Real-time chemical characterization of primary and aged biomass burning aerosols derived from sub-Saharan African biomass fuels in smoldering fires†
Received
7th August 2024
, Accepted 27th October 2024
First published on 28th October 2024
Abstract
The influence of biomass burning (BB)-derived organic aerosol (OA) emissions on solar radiation via absorption and scattering is related to their physicochemical properties and can change upon atmospheric aging. We systematically examined the compositionally-resolved mass concentration and production of primary and secondary organic aerosol (POA and SOA, respectively) in the NC A&T University smog chamber facility. Mass spectral profiles of OA measured by the Aerosol Chemical Speciation Monitor (ACSM) revealed the influence of dark- and photo-aging, fuel type, and relative humidity. Unit mass resolution (UMR) mapping, the ratio of the fraction of the OA mass spectrum signal at m/z 55 and 57 (f55/f57) vs. the same fraction at m/z 60 (f60) was used to identify source-specific emission profiles. Furthermore, Positive Matrix Factorization (PMF) analysis was conducted using OA mass spectra, identifying four distinct factors: low-volatility oxygenated OA (LV-OOA), primary biomass-burning OA (BBOA), BB secondary OA (BBSOA), and semi-volatile oxygenated OA (SV-OOA). Data supports a robust four-factor solution, providing insights into the chemical transformations under different experimental conditions, including dark- and photo-aged, humidified, and dark oxidation with NO3 radicals. This work presents the first such laboratory study of African-derived BBOA particles, addressing a gap in global atmospheric chemistry research.
Environmental significance
African biomass burning (BB) is a major source of particulate emissions, however, there have not been enough, if any, laboratory studies to characterize their chemical composition. Laboratory studies have been focused predominantly on North American fuels. Quantifying trends in emissions from different regions of the world and their impacts on atmospheric composition will advance fundamental atmospheric chemistry knowledge for predictive capability of the distribution, reactions, and lifetimes of gases and particles. This work represents one of a series of laboratory studies to identify the chemical compositions of BB aerosols derived from sub-Saharan African biomass fuels thereby addressing a gap in global atmospheric chemistry research. This paper adds valuable measurements to the growing body of information on global aerosol properties.
|
1 Introduction
In recent years, biomass burning (BB)-derived aerosols have gained increasing attention due to BB-derived aerosol's crucial role in influencing Earth's atmospheric chemistry and radiative forcing, as well as air quality and human health.1 BB aerosols are emitted from wood and vegetation burning for domestic use, prescribed fires, and wildfires.2 BB is a major global source of primary aerosols, contributing between 59 and 85% of global black carbon3 and primary organic aerosol (POA).4,5 BB aerosols can affect the climate directly by scattering or absorbing radiation, resulting in cooling or warming of the atmosphere, respectively.6,7 OA comprises a complex mixture of organic compounds and can constitute the predominant fraction of BB particulate emissions, ranging from 20–90%.8
Africa is a major contributor to BB emissions, contributing up to 52% of the global carbonaceous BB aerosol burden.2,9,10 Africa's significant gaseous and particulate emissions contribute to climate change, health issues, and air quality concerns on the continent,11 and elsewhere through long-range transport.12,13 This trend may continue because BB and wood fuels remain a major source of energy, accounting for 60–86% of primary energy consumption and up to 98% of residential energy production in sub-Saharan Africa.14 Currently, more than 40% of the population of Africa is without access to electricity, and 70% is without clean cooking facilities.15 Clean cooking refers to using modern, efficient, low-emission technologies and fuels to prepare food.16 This includes cooking methods that minimize the health and environmental impacts associated with traditional biomass fuels such as wood, charcoal, and dung, commonly used in many parts of Africa. It is projected that Africa will comprise over 25% of the world's population by 2050, and will likely remain limited in modern energy access.17 In 2022, more than 990 million people in Africa lacked access to low-emission technologies and fuels for cooking food.15,16 The future trajectory of African emissions will have global significance, as most of these emissions will occur in the tropics, where they are predicted to have a larger influence on atmospheric chemical processes and radiative forcing compared to northern midlatitudes.18,19 Understanding these emissions is not only crucial for their impact on Africa's population but also for the entire world and the global climate.
Fuel type, combustion condition, moisture content, and relative humidity (RH) largely influence the chemical and physical properties of these aerosols and are crucial in understanding their environmental impacts.20,21 Despite their abundance and environmental significance, African-derived BB aerosols remain relatively understudied in comparison to other regions, leaving a significant gap in our knowledge.22,23 The main source of uncertainty in global climate models is the uncertainty in the optical properties of aerosols; reducing the uncertainty in Earth system models and chemical transport models24 requires measuring the optical and chemical properties of aerosols emitted from African sources.24–26 To improve the accuracy of these models, it will be necessary to investigate the dependence of aerosol optical properties and chemical composition on fuel type, atmospheric aging conditions, the impact of RH, and the conditions that lead to the transformation of primary gaseous emissions and POA to secondary organic aerosol (SOA).
Emissions containing a mixture of organic compounds of different volatilities (i.e., semi-volatile, intermediate, and low-volatility) are formed by thermal degradation of combusted fuels. The compound's volatility influences the distribution of that compound between the gas and condensed phases.27–29 Semi-volatile organic compounds (SVOCs) can be found in both the gas and particle phases, and is dependent on atmospheric conditions and temperature; high temperatures and lower RH conditions favor the gas phase.30 Low-volatility organic compounds (LVOCs) have a low evaporation tendency and favor the particle phase.20,21 POA evolves and participates in oxidation processes initiated by photochemistry; this process is referred to as “aging”.31,32 Additionally, SOA is formed when volatile organic compounds (VOCs) and intermediate volatility organic compounds (IVOCs) react with atmospheric oxidants, such as hydroxyl radicals (˙OH), ozone (O3), and nitrate radicals
, to produce less-volatile organic products that either nucleate into new aerosol particles or condense onto existing aerosol particles.20,33–37 SOA can also form when water-soluble gasses participate in multiphase chemistry.38–40
Laboratory and field studies have demonstrated that aging not only increases OA mass and oxygenation,41 but also reduces its volatility. It has been shown that BB contributes less than 70% of the SOA burden and influences the seasonal variation of global SOA.42 A series of laboratory measurements of smoke emission composition profiles from several important North American fuel types burned under a variety of conditions have been conducted at the Fire lab at Missoula, MT (e.g., FLAME)43–46 to provide source profiles for classes of fires believed to severely impact air quality in the western and southeastern U.S.43–46 However, there are very limited studies on fuels for sub-Saharan Africa.47 Data on the evolution of African BB emissions during atmospheric aging is limited and investigating the chemical profile of resultant SOA constituents will help decrease this uncertainty.
Analyzing the complex chemical composition of OA48 is challenging yet indispensable. Previous laboratory and field studies have mainly focused on flaming-dominated, high-temperature fires, fresh emissions, and photochemical aging of BBOA derived from fuels of other regions.4,20,49–51 Recent laboratory chamber studies have contributed to the understanding of BBOA chemical composition.21,52–55
Mass spectrometry has emerged as a common tool for investigating BB-derived aerosols, including real-time monitoring.56 In this study, we used the Aerosol Chemical Speciation Monitor (ACSM, Aerodyne, Inc. Billerica, MA, USA) which gives the unique advantage of capturing the rapid chemical transformation of aerosols as they age. Cellulose, hemi-cellulose, and lignin are present in all biomass fuels53 and produce many particulate organic compounds upon combustion. The evolution of these particulate organics were monitored via characteristic OA mass spectral fragment ions (at mass-to-charge ratios (m/z) 29, 43, 44, 57, 60, and 73) to quantify and identify BBOA emissions.50,52,57,58 Mass spectral profiles, which depict the fragmentation patterns of different chemical species present in aerosols, are crucial for identifying and comparing emissions from specific fuels and regions. These profiles provide a standard reference for laboratory and field based BBOA measurements. In this work, we generate primary BBOA emitted from smoldering-dominated combustion of sub-Saharan African biomass fuels and investigate its chemical evolution in laboratory smog chamber experiments, focusing on the transformation of OA during the transition from night-time to daytime oxidation environments. We demonstrate the influence of elevated RH, aging condition (dark- and photo), and dark oxidation with the addition of
on the fraction of the ACSM OA mass spectral signal explained by characteristic fragment ions; specifically, f43, f44, f55, f57, f60, and f73.
2 Experimental details
2.1. Fuel details
Combusted fuels are characteristic of sub-Saharan Africa and include mokala (Acacia erioloba), savannah grass (unknown), mukusi (Baikiaea plurijuga), eucalyptus (Eucalyptus camaldulensis), wanza (Cordia Africana), mopane branches and leaves (Colophospermum mopane), and cow dung from Ethiopia.26 The fuels combusted in this work are commonly used for domestic cooking and heating in the region.26 Fuels were dried and stored under a fume hood until used to ensure a moisture content of <10% before combustion. Moisture content was verified by a moisture analyzer (PCE-MA 50x,wood Dale, IL, USA). The analyzer measures the sample mass pre- and post-drying; temperature and a time profile is recorded along with moisture content. These measurements were conducted at New Mexico Tech. Before each experiment, all wood fuels were debarked.
2.2. Gas and particle measurement
The fuel was combusted for 10 min, introducing gaseous and primary aerosol emissions into a cyclone (URG-2000-30ENS-1, URG, Chapel-Hill, NC, USA) with a 2.5 μm cut point and then sent into the smog chamber via a ¼-inch stainless steel tube; the furnace was then detached from the chamber.
The smog chamber was equipped with a suite of instrumentation to measure particulate and gaseous emissions during each experiment, including the differential mobility analyzer (DMA; Model 3080; TSI Inc., Shoreview, MN, USA) coupled with a water-based condensation particle counter (WCPC; Model 3787; TSI Inc., Shoreview, MN, USA), with the two functioning in tandem as a scanning mobility particle sizer (SMPS). This measured near real-time aerosol number size distributions, enabling derivation of total aerosol concentrations, geometric mean diameters, and mass concentrations.
Emissions of gas-phase species measured during each experiment, included carbon monoxide (CO) (iQ Series 48, Thermo Scientific, Ramsey, MN, USA), carbon dioxide (CO2) (iQ Series 410, Thermo Scientific, Ramsey, MN, USA), nitrogen oxides (NOx = NO + NO2) (iQ Series 42, Thermo Scientific, Ramsey, MN, USA) and O3 (2B Technologies Model 211 Scrubberless Ozone Monitor, Ramsey, MN, USA). These gas-phase species analyzers, along with the SMPS and quadrupole ACSM (Q-ACSM, Aerodyne, Inc. Billerica, MA, USA), were operated online, allowing real-time measurements. More details about ACSM measurements are provided below.
To ensure a clean chamber environment at the start of each experiment, the smog chamber was continuously flushed for over 24 h with air from a zero-air generator (Aadco Instruments, 747-30, Cleves, OH, USA), until a negligible aerosol mass concentration was reached (i.e., ≤3 μg m−3 as measured by SMPS). This cleaning process was conducted with the UV chamber lights on. In addition, the tube furnace along with the tubing was cleaned to remove any remaining residue in the furnace using LC/MS grade methanol.
2.3. Burning conditions
A total of 19 burns were conducted under smoldering-dominant conditions, verified by the modified combustion efficiency (MCE). MCE, which is calculated using eqn (1) (ref. 59) is a measure of combustion efficiency. MCE values from all experiments conducted in this study lie within the range of 0.73 and 0.90, confirmed by past studies as smoldering-dominated burning conditions.60 |
MCE = ΔCO2/(ΔCO2 + ΔCO)
| (1) |
Burns were repeated for all fuels used in this study, including experiments where combusted fuel mass was less than 0.5 g. Results showed consistent MCE values across replicates, confirming smoldering-dominant conditions. To determine whether the mass of fuel combusted influenced the resulting mass spectra, statistical analyses were performed. Specifically, z-scores and two-sample t-tests (95% confidence interval: α: 0.05). This is reflected in Table S2.† The results indicated no statistically significant differences in the mass spectra across replicates, confirming the consistency and reliability of the experimental data.
2.4. Dark oxidation experiments
Each experiment included measurement of primary and photoaged BBOA, and some included dark oxidation by NO3 radicals. To initiate dark oxidation by NO3 radicals, 500 ppb of NO2 gas (Airgas) was injected into the smog chamber at ∼1.2 L min−1, as measured by the NOx monitor. This was immediately followed by increasing O3 mixing ratios to ∼200 ppb using an O3 generator (Pacific Ozone, model R-LAB210102). All gas-phase species concentrations were verified by their respective gas analyzer (see Section 2.2). 80 min were allotted for the mixing and production of
(with the mixing fan operating at 0.25 of maximum speed for the entire experiment), resulting in ∼250 ppt of NO3 radical. Details of this process are discussed in detail in the literature.61 For dark oxidation experiments, oxidants were added to the chamber before humidification and generation of primary aerosols. Note that not all experiments reported in this study involve dark oxidation, such experiments are noted in the summary of experiments in Table S1.† A summary is presented in Table 1.
Table 1 Summary of experiments conducted
Exp. # |
Fuel type |
RH (%) |
MCE |
Added oxidant |
1 |
Mokala |
<10 |
0.77 |
— |
2 |
Mokala |
≥65 |
0.75 |
— |
3 |
Mukusi |
≥65 |
0.85 |
— |
4 |
Mopane leaves |
≥65 |
0.91 |
— |
5 |
Mopane leaves |
<10 |
0.87 |
— |
6 |
Savannah grass |
≥65 |
0.88 |
— |
7 |
Mukusi |
<10 |
0.90 |
— |
8 |
Mopane leaves |
≥65 |
0.83 |
Yes |
9 |
Dung |
≥65 |
0.88 |
Yes |
10 |
Savannah grass |
<10 |
0.82 |
— |
11 |
Mopane leaves |
≥65 |
0.83 |
— |
12 |
Mukusi |
≥65 |
0.83 |
— |
13 |
Mukusi |
≥65 |
0.74 |
Yes |
13 |
Savannah grass |
≥65 |
0.93 |
Yes |
15 |
Mopane leaves |
≥65 |
0.87 |
Yes |
16 |
Mokala |
≥65 |
0.79 |
Yes |
17 |
Dung |
<10 |
0.86 |
— |
18 |
Mokala |
≥65 |
0.79 |
— |
19 |
Dung |
≥65 |
|
— |
2.5. Chamber humidification
The average RH during chamber experiments was either less than 10% or initially ≥65%, with the latter decreasing throughout the experiment and stabilizing around 50% RH. For experiments conducted at elevated RH (≥65%), the smog chamber was humidified using a technique adopted from Mouton et al.62 Briefly, humidification was achieved by directing a flow of particle-free zero air through a customized bubbler (Quark Glass) containing ∼400 mL of type II water which had <50 ppb of total organic carbon (Thermo Scientific LabTower TII, 2.0 MΩ cm). The humidification setup included a round bottom flask heated by a heating mantle (Chemglass, CG-10000-07) powered by an analog controller (Chemglass, CG-15005-01). Insulated ¼ inch Teflon tubing (Swagelok) was attached to the chamber via a plug valve for ∼45 min until the desired RH (≥65%) is reached. Humidification of the chamber occurred before the introduction of combusted emissions.
2.6. Primary aerosol generation and aging experiments
A variety of sub-Saharan African biomass fuels were combusted in a Carbolite Gero (HST120300-120SN, Hope, Derbyshire, UK) tube furnace operated at 450 °C. For each experiment, ∼0.050–0.500 g of each biomass fuel was weighed on a calibrated analytical balance and placed into a quartz combustion boat (AdValue Technology, FQ-BT-03, Tucson, AZ, USA) before being introduced to the preheated tube furnace. A range of masses was chosen to check the reproducibility of mass spectra for each fuel. Mass spectral values were compared between fuels combusted under similar experimental conditions. Z-scores and t-tests were used to determine their statistical significance, with 95% confidence intervals. Results showed consistent MCE values across replicates, confirming smoldering-dominant conditions. To determine whether the mass of fuel combusted influenced the resulting mass spectra, statistical analyses were performed. Specifically, z-scores and two-sample t-tests (95% confidence interval: α: 0.05). This is reflected in Table S2.† The results indicated no statistically significant differences in the mass spectra across replicates, confirming the consistency and reliability of the combustion process.
Primary emissions were diluted with zero-air at 2.0 L min−1 and injected for 10 min into the ∼9.0 m3 indoor smog chamber facility at North Carolina Agricultural and Technical State University (NC A&T University). The indoor smog chamber features fluorinated ethylene propylene (FEP) Teflon lining and two banks of ultraviolet lights (Sylvania, F30T8/350BL/ECO, 36′′, St. Charles, IL, USA) for a total of 64 lamps (32 on each side). To create a well-mixed atmospheric environment in the chamber, a mixing fan is turned on to 0.5-max speed before the start of combustion and reduced to 0.25-max speed throughout the experiment. Details of the smog chamber facility have been discussed elsewhere.26,61,63–66
Fig. 1 displays the schematics of the experimental setup. The OA produced in each experiment underwent two serial stages of aging: dark- and photo-aging. In each experiment, primary aerosols remained in the chamber under dark conditions for approximately 1 h after the initiation of combustion to allow sufficient mixing, stabilization, and characterization. This constituted the “primary” BBOA sample. Immediately following, 3 h was used to define dark aging. After 30 min of sampling, UV lights were turned on to initiate photo-aging conditions of the BB emissions for 2 h. A summary of experimental burns is presented in Table 1. For additional details, the complete set of experimental conditions is available in Table S1 in the ESI.†
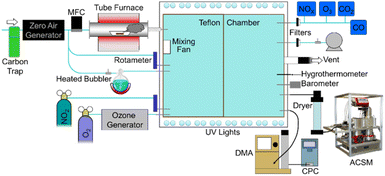 |
| Fig. 1 Experimental laboratory schematic adopted from Pokhrel et al.60 Particle aging was studied in a 9.0 m3 smog chamber at the North Carolina Agricultural and Technical State University (NC A&T University). The setup features a tube furnace that allows temperature control, where ∼0.050–0.50 gram fuel samples were combusted at 450 °C. The chamber has been fully characterized in a prior study.66 | |
The experimental setup is presented in Fig. 1. Details of the characterization and construction of the indoor chamber are reported elsewhere.66 Smith et al.66 also reported particle wall losses at a rate of 9.46 × 10−3 min−1 for aerosol mass concentration, and we measured a wall loss rate of 2.5 × 10−3 min−1 in our experiments, which is the same order of magnitude to the earlier results.
Several experimental conditions were investigated, including experiments at less than 10% RH, at ≥65% RH (elevated humidity), and at elevated RH with the addition of NO3 radicals.
2.7. ACSM measurements
OA chemical and mass concentration data were acquired using an Aerodyne Q-ACSM, which measures real-time non-refractory submicron (NR-PM1) mass concentration and chemical composition of NR-PM1 species that volatilize at 600 °C. The ACSM reports the aerosol mass concentration of organics, chloride, ammonia, sulfate, and nitrate; however, in this work, we report only organic species which occupied over 50% of the chemical composition of all experiments. The instrument design and operation have been described in detail by Ng et al.67 Lens alignment, ionization efficiency (IE) calibration, mass-to-charge (m/z) calibration, tuning, and flow rate calibration were performed using methods defined by Aerodyne Research (Billerica, Massachusetts) and performed at the beginning of the experimental series. IE calibration was achieved using ammonium nitrate and ammonium sulfate, where particles were generated via atomizer and then size selected at 300 nm using an SMPS. The relative ionization efficiencies (RIEs) are 6.11 for ammonium and 0.67 for sulfate. The NO3 response factor (RF) was 9.19 × 10−12. Instrument functionality was checked before and after each experiment with special attention to the internal standard signal at m/z 128, air ion signals, sampling inlet pressure, and vaporizer temperature. ACSM measurements were collected throughout all experiments; a homemade diffusion dryer was placed between the chamber and the inlet of the ACSM. An assumed collection efficiency of 1 was used for all datasets presented in this work. The ACSM operated in the predefined acquisition, single scan mode, with a resolution of 22, reporting data points every ∼15 min, with a scan speed of 200 ms amu−1, as suggested by Aerodyne Inc. Specifically, data points for each stage of the experiment were as follows: primary (4 data points), dark aging (12 data points), and photoaging (8 data points). ACSM data analysis was performed using Igor Pro 8 (Wave metrics, Lake Oswego, OR) using the standard ACSM Local v1.6.1.7, Global Utils, and Frag Table Data from Aerodyne Inc. Herein the ACSM is used to distinguish between various classes of organic species through fractions of characteristic fragment ions (f), which correspond to chemical markers found within POA like BBOA, including non-acidic and acidic organic compounds, saturated hydrocarbons, and oxygenated organic compounds.51,67,68 Characteristic fragment ions that are specific to BBOA were used, where f is the signal intensity at a particular m/z divided by the total OA intensity measured by the ACSM; these included characteristic fragment ions at m/z 29 (f29), 43 (f43), 44 (f44), 57 (f57), 60 (f60), and 73 (f73). Table 2 presents the chemical nature and significance of each f-value fragments in this study.
Table 2 Chemical nature of fragment ions
f |
Potential ions |
Scientific significance |
Behavior upon aging |
60 |
C2H4O2+ |
Specific to BBOA, primary aerosols |
Decrease |
73 |
C3H5O2+ |
43 |
C2H3O+ |
Non-acid organics, oxygenated organics |
Increase |
44 |
CO2+ |
Acidic organics or oxygenated organics |
55 |
C4H7+ |
Saturated hydrocarbons |
Decrease |
C3H3O+ |
Oxygenated organics |
Increase |
57 |
C4H9+ |
Saturated hydrocarbons |
Decrease |
C3H5O+ |
Oxygenated organics |
Increase |
29 |
CHO+ |
Oxygenated organics |
Increase |
C2H5+ |
Organic hydrocarbons |
Decrease |
All of these fragment ions correlate with compounds derived from the pyrolysis of cellulose and lignin present in the vegetation.36,69–71 Characteristic fragment ions at m/z 60 and 73 are especially notable since they have been shown previously to be specific markers for wood burning and are known to decrease in value upon aging.21,52,72,73 f-Values reported here were extracted from mass spectra averaged over a 15 min interval and will be compared across experiments and aging phases, serving as a metric for monitoring chemical changes over time and varying environmental conditions.
2.8. Positive matrix factorization (PMF)
In this work, we performed positive matrix factorization (PMF) analysis of the OA mass spectra data from the ACSM, for 12 selected chamber experiments (Table S3†). A 4-factor solution was ultimately selected to deconvolve OA mass spectra produced via the ACSM, as this approach provided the clearest distinction between sources without excessive factor splitting. Further information on this selection process is provided in the ESI.†. These experiments encompass various aging and experimental conditions: primary-, dark-, and photo-aging under both dry and humid conditions, as well as elevated RH conditions in the dark with the addition of
. ACSM data collected from all experiments were combined into one data matrix to simulate different atmospheric conditions where multiple biomass fuels were present. Chosen data sets correspond to experiments where the mass of the combusted fuel was ≥0.9000 g. For the deconvolution of the spectra, organic variable and error time series matrices were created and exported using PMF Exporting in ACSM local 1.6.1.6 in Igor9. Source apportionment of ACSM OA data involved the application of PMF analysis74 utilizing the PMF evaluation tool.69 Before PMF analysis, fragments with m/z values ≤ 15 Th were removed from organic mass spectra due to negative values, and species with m/z values ≥120 were removed for PMF analysis since their contribution to the overall organics signal was negligible, due to signal to noise ratio.75 Previous studies detailed the selection of factors (P), rotation forcing parameters (FPEAK), and evaluation of PMF solutions.69,74,76 These practices were adopted for our study. PMF results were then used to identify the different processes during chamber experiments. By combining the data sets, representative of different fuels, it was possible to determine which fuels dominated specific factors and m/z values.
3 Results and discussion
For all experiments, peak aerosol mass concentrations ranged from 93–2010 μg m−3 (as measured via SMPS), with OA having the dominant contribution (≥50%) as reported for prior BBOA studies, and with a measured particle density of 1.2 ± 0.1 g cm−3.61 During the characterization period of primary BBOA emissions, aerosol mass concentrations peaked (see Table S1†) and decreased over time during each experiment.
3.1. Characteristic fragment ions for primary and aged BB-derived aerosols
The emission factors (EFs), defined as the OA mass produced per kg of fuel burned and determined by dividing the mass of OA produced (g), which is determined by the ACSM, by the mass of fuel burned (kg) are reported in Table 3. EFs ranged from 1.5 to 5.5 with mukusi having the lowest EF and dung having the highest. The emission factors EFs are calculated using eqn (2). |
 | (2) |
Table 3 Mass OA PM produced per gram of fuel, resulting Emission factors calculated in this work and comparison with work by Andreae et al.4
a
Fuel |
EF (g kg−1) this work |
EF (g kg−1) Andreae et al.4 |
Blanks in Table 3 (—) signify the loss of a data file from an ACSM laptop due to a system crash. Control experiments (RH < 10%) were used for EF calculations. |
Mokala (Acacia erioloba) |
— |
— |
Mopane leaves (Colophospermum mopane) |
1.86 |
4.4 ± 1.9 |
Mukusi (Baikiaea plurijuga) |
1.52 |
4.4 ± 1.9 |
Savannah grass (unknown) |
3.06 |
3.0 ± 1.5 |
Dung |
5.48 |
10.4 ± 9.4 |
As shown in Table 3, Andreae et al.4 report EFOA of 10.4 ± 9.4 g kg−1 for dung burnt with an average MCE of 0.88 ± 0.04. Our value of 5.5 g kg−1 was lower by half, on average, but was well within the range of observed values. Their savanna/grassland values were slightly lower, at 3.0 ± 1.5 g kg−1 at an average MCE of 0.94 ± 0.02, which has very good agreement with our savanna grass value of 3.1 g kg−1. The mopane leaf and mukusi averaged 1.7 g kg−1, which is somewhat lower than Andreae's tropical forest value of 4.4 ± 1.9 g kg−1. The errors in Andreae et al.4 are one standard deviation, so we are within that for everything except mopane leaves and mukusi, where we are within two standard deviations. We are also assuming that 100% of our aerosol makes it to the chamber, which is not the case. These are estimated OA EFs, which can be regarded as lower bounds.
The difference in EFOA values can be attributed to varying burn conditions and moisture content (Table S4†). When compared to dry BB fuel (moisture content 5%), moist BB fuel showed decreased emissions of PM2.5 and oxygenated organic compounds (OOCs), due likely to the presence of water in the system that partially suppressed the production of OOCs.77 While all fuels had a moisture content below 10%, the actual percentage of moisture varied by fuel, as shown in Table S4.† Studies have shown that fuel moisture and elevated humidity can influence the efficiency of a burn, resulting in an increase in the production of organic compounds, therefore affecting the EF of BBOA.52,78,79
Historically, OA levels from primary and aged BB emissions monitored by ACSM and AMS have been described using BB-specific f-values.20,37,52,80 The fractions are used as markers for BBOA, where the fraction is denoted by “f” before the m/z value (e.g., f29), where the fraction is the signal intensity at a particular m/z divided by the total OA signal intensity. Notably, f29, f43, f44, f55, f57, f60, and f73 were enhanced for hardwood (mukusi) and non-hardwood fuels (dung, mopane leaves, savannah grass, mokala), when compared to other fragments in the spectra.20,36,50,71,81–84
Fig. 2 shows the ACSM OA mass spectra from primary emissions of four fuels combusted at <10% RH and serves as a reference point for understanding how the mass spectra shift under varying experimental conditions (e.g., increased RH, aging, and
oxidation). It allows for comparison across experiments and helps with seeing the evolution of OA species. These mass spectra at low RH are consistent with previous wood, grass, and dung burning observations.50,67,72 For example, for dung we observed that f29 contributed to 2.9% of the total signal, closely matching the 2.0% reported by Goetz et al.50 Similarly, f44 was the dominant fragment in all experiments contributing 1.2% of the total signal and reported an average 1.9% of total signal. The lower relative intensities of f-values ≥75 in our work agreed with previous findings. For all experiments, mass spectra include a dominant f44 (CO2+˙) and lower relative intensities for mass fragments at m/z values ≥75. f44 is noted as one of the most reliable markers of oxygenated organic aerosols, along with the less commonly used f18.85 A prominent ion signal at m/z 29 was observed across all experiments, which is associated with C2H5+ and CHO+ and indicates the presence of organic hydrocarbons and oxygenated organics, respectively. f73 is noted to also be attributed to the thermal degradation of lignin and cellulose, where the fragment ion is likely C3H5O2+.54,81,86 However, the unit mass resolution (UMR) of the ACSM does not allow us to distinguish the exact elemental composition of this ion.
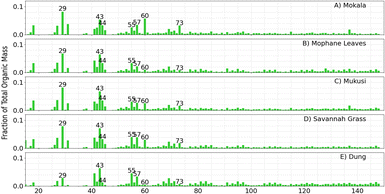 |
| Fig. 2 Representative average OA mass spectra from the ACSM of controlled combustion experiments conducted without the introduction of humidity (RH < 10%) or external oxidants. Note data represents primary aerosol emissions from burning (A) mokala, (B) mopane leaves, (C) mukusi, (D) savannah grass, and (E) dung. ACSM measurements were taken within the first hour after combustion and is representative of data averaged over 15–20 min, which is 1 data point. | |
As shown in Fig. S2,† we also report the ratio of organic ions f55 to f57 against f60 to identify emissions associated with primary laboratory aerosols produced from BB and compare with a recent study, which was the first to report this framework.50 UMR mapping facilitates the identification and separation of emission source types. The similarity of our fraction values with those reported by Goetz et al.50 confirms that our laboratory data, which includes a variety of fuels, is comparable to OA measurements from cookstove-produced OA. Note Goetz et al.50 reports averaged coordinate values (f55/f57, f60), over a series of experiments, for hardwoods, dung, grass, and twigs not sourced from Africa. Despite the geographical and specific fuel type differences, the coordinate values, (f55/f57, f60), align well with BB emission profiles. The ACSM fragment ions at m/z 55 and 57 are likely associated with C4H7+ and C4H9+, respectively, and are relevant to the saturated hydrocarbon series.87 The fragment ion at m/z 60 is typically associated with the elemental formula C2H4O2+, which is produced during the pyrolysis of cellulose and is historically recognized as a levoglucosan marker used for identifying BB fuels. This fragment ion at m/z 60 typically has little to no interference from other OA sources.88
3.2. Effects of RH (humid vs. dry) on primary BBOA composition
The influence of RH on OA species in dark laboratory experiments can be seen in Fig. 3. We observe OA through the f44 vs. f60 triangle plot52,82,89,90 comparing values of experiments conducted under dry and elevated humidity conditions. Values were extracted from mass spectra averaged over a 15 min interval, approximately 30 min after combustion of the fuels. The atmospheric chemistry of BBOA has previously been shown to be sensitive to RH, with enhanced oxidation of BB smoke leading to secondary organic aerosol at high RH.82 The experimental data presented here have a similar trend to those reported by Cubison et al.52 for US fuels. Fig. 3 highlights the complex, fuel-specific responses of primary BBOA composition with increased relative humidity (RH). Three of the five fuels show an increase in f60 (i.e., mukusi by a factor of 1.25, mopane leaves by a factor of 1.25, and savannah grass by a factor of 1.22), while dung and mokala display a decrease by factors of 0.85 and 0.86, respectively. The variability highlights the importance of considering OA behavior as fuel-specific. The effect of humidity cannot be generalized across all fuel types. The plot reveals a general decrease in f44 intensity for most fuel types (i.e., mokala, mukusi, and mopane leaves), indicating a reduction in the contribution of OOA. This could also possibly be because wall losses of carboxylic acid gases were larger at high RH, pulling f44 out of particles. The trend is most pronounced in mukusi, with a 58.4% reduction in the f44 signal. In contrast, savannah grass and dung report an increase in f44 under increased humidity, with savannah grass having the most pronounced increase (42.9%), indicating a unique chemical response to humidity. For f60 values, the signal intensity tends to be higher upon exposure to elevated humidity. This is evident in mukusi, mopane leaves, and savannah grass, with mukusi experiencing the greatest increase at 33.3%. However, dung and mokala show decreases in reporting values of −25.0% and −8.3%, respectively, under elevated humidity. These varied responses to elevated humidity demonstrate the impact of fuel-specific chemical composition on OA behavior. The variability highlights the importance of considering OA behavior as fuel-specific under varying conditions. The effect of humidity cannot be generalized across all fuel types.
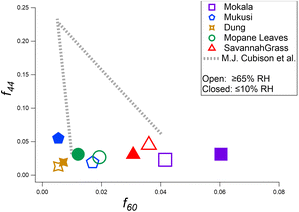 |
| Fig. 3 Primary BBOA composition displayed via f60 versus f44 from ACSM OA mass spectra of primary BBOA mass under dry (<10% RH, closed symbols) or elevated humidity (≥65% RH, open symbols) conditions in the dark for all fuels used in this work. | |
The observed decrease in f44 with increasing humidity could be tied to the retention of less oxidized species, as suggested by Zhang et al.91 Under elevated humidity, the presence of water may alter the chemical pathways, favoring reactions that inhibit further oxidation, thus leading to lower f44 values. This aligns with the findings of Ng et al.,67 where higher humidity conditions favored the formation of less oxidized compounds. Additionally, while f44 decreases were generally observed, wall losses could contribute to this trend by preferentially removing more oxidized, volatile components, although this remains speculative and without direct evidence linking wall losses to f44 reductions in this study.
The variability in f60 across different fuels highlights the fuel-specific nature of OA responses to humidity. While three fuels (mukusi, mopane leaves, and savannah grass) show increased f60 under elevated RH, the remaining two (dung and mokala) display decreases, suggesting that primary BB markers are influenced by the unique chemical composition of each fuel. The observed trends in f60 suggest that some fuels may resist oxidation under higher humidity, further supporting the need for nuanced interpretations of OA behavior under varying environmental conditions.
3.3. Effects of photochemical aging on BBOA composition
Mass spectra, highlighting the fraction of the total OA mass vs. m/z for each fuel, are shown in Fig. S3 and S4† that compares f44 and f60 before and after photo-aging. The results indicate distinct differences between the fuels when comparing f-values between dark- and photo-aging. f43 and f44 values increased upon photoaging for all fuels. The increase at f44 can be attributed to the enhanced oxygenation of either particulate or gas-phase (and subsequent partitioning into the aerosol phase) organics upon exposure to UV light. f44 values have an average increase of 1.5% among all experiments conducted when comparing dark- and photo-aging conditions, which falls between the 0.9–4.2% increase reported in Xu et al., representative of China-sourced fuels.81 Reported f60 values in Fig. 4 are representative of the mass spectra over 15 min within the 2 h of the photo-aging period. The decay of f60 is seen in all experiments and indicates the depletion of levoglucosan-related compounds that contribute to the f60 signal. Additionally, the production of SOA during photochemical aging potentially contributes to the reduction observed for f60 and f73. Trends for f60 and f73, shown here were also observed in the literature, not representative of African sourced fuels.36,52,81 The f73 trend across all fuels is as expected with cellulose pyrolysis generating highly oxygenated species in POA and decreasing with aging. BBOA markers at f60 and f73, specific to wood-burning aerosols,57,72 were also observed in all aging stages of each experiment. f60 and f73 are at their highest values immediately after the combustion of each fuel, and they steadily decrease into stages of aging, which agrees with previous studies.21,50 All fuels show an elevated signal at f18, with f18 having a signal contribution from oxygenated organic compounds, approximately equal to f44, with f18 having a signal contribution from H2O+ and signifying a more oxidized aerosol.57,92 f18 should not include an interference from NH4+ since that would be included in the ammonium factor.
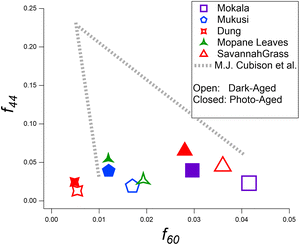 |
| Fig. 4 Scatter plot displaying f60 versus f44 for ACSM OA mass spectra. Values are representative of dark-aged and photoaged OA. | |
3.4. Effects of the addition of 
For experiments involving the formation of
, NO2, and O3 concentrations peaked, then decreased over time (between injection and combustion; see Fig S5†), similar to the findings reported by Kodros et al.82,93 Note in this study, oxidants were injected before the combustion of fuel to avoid ozonolysis of the primary emissions. Thus, these experiments examine changes in aerosol composition resulting from night-time
to daytime (˙OH) chemical processing.
Fig. 5A displays a summary of the difference spectra for dark aging and photoaging. Fig. 5B reports the net influence of subsequent photo-aging that followed dark
exposure of BBOA. Upon photoaging, a decrease in f29 and f57 is seen in mopane leaves and mukusi, possibly due to further oxidation and/or depletion of chemical species that contributed to the f29 and f57 signal of dark aging of that respective experiment. This decrease suggests that the compounds responsible for these signals are being oxidized into more oxygenated products or depleted through transformation into other non-contributing forms implying that the aerosol's chemical composition is evolving.8
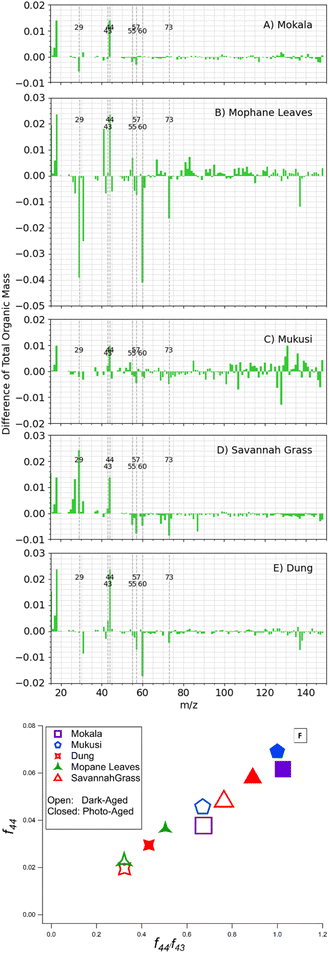 |
| Fig. 5 (A–E) Displays the net influence of subsequent photo-aging that followed dark exposure of BBOA, where values were calculated by subtracting the mass spectra values of dark-aged (with present) from photo-aged mass spectra. That is, negative values have a higher abundance before photo-aging, while positive ones have a higher value afterwards. (F) f44 vs. f44/f43 for all experiments that experienced aging with the addition of . The gray dashed arrows here are added to highlight the increasing degree of oxidation upon photoaging (for 2 h) after the -aged BBOA emissions. | |
The most significant influence of photoaging was observed via f60 and f73 for mopane leaves f60 and f73 show significant changes during photo aging reflected in the change in difference spectra, reflective of their use in tracing the transformation of BBOA to SOA. The two serve as indirect indicators of SOA formation through their decay which signifies the production of more oxidized compounds, also reflected in the increase at f44. This decrease can be attributed to the oxidation of either fresh BBOA particles or gas-phase organics that lead to the formation of SOA during aging. Thus, mopane leaves that were first dark aged with
showed the greatest extent of oxidation upon subsequent photo-aging and decay of f60. Other studies94–96 proposed the use of higher levoglucosan f-values in combination with established levoglucosan-related markers (f60 and f73) to monitor the extent of oxidation upon dark and photoaging with added
.
All fuels show f43 > f44 and an expected increase of f43 upon photoaging. The comparison between f43 with f44 has been proposed to assess the extent of oxidation/aging and to distinguish between LV-OOA and SV-OOA. The increasing f44/f43 ratio is likely indicative of an increasing SV-OOA contribution to the aerosol upon photochemical aging.
Fig. 5B presents the use of f44 and f43, commonly used in the literature to monitor the degree of oxidation.55,69,97,98 The 5 fuels presented each show a clear trend of increasing oxidation upon photo-aging, where higher f44 values are consistent with LV-OOA and higher f43 values are consistent with SV-OOA. The upward shift of the data is dominated by the increases in f44 upon photoaging, highlighting the evolution of OA chemical composition seen in prior studies.20,55,99,100
Prior studies have also proposed using [NO2+/NO+] fragmentation ratios to quantify organic and inorganic nitrates via models of the AMS.93–96,100 However, due to the UMR of the ACSM, resolving organic nitrates from inorganic nitrates was not possible.
3.5. PMF analysis of OA mass spectra
The identifiable factors for the 4-factor solution, include LV-OOA (Factor 1), primary BBOA (Factor 2), biomass-burning secondary organic aerosols (BBSOA) (Factor 3), and SV-OOA (Factor 4). The factor mass contributions are as follows: F1 = 24.7%, F2 = 27.9%, F3 = 26.5%, and F4 = 20.9%. A 4-factor solution was ultimately selected to deconvolve OA mass spectra produced via the ACSM, as this approach provided the clearest distinction between sources without excessive factor splitting. Further information on this selection process is provided in the ESI.†. Fig. S6† shows the results of a 4-factor solution based on exploring the following: %ΔQ/Qexp vs. factor, f peak, mass spectra, and interpretation of prominent peaks at m/z values historically used as BBOA markers.
The mass spectra and time series of factor contributions (Fig. 6) were analyzed to determine the type of OA factors. Fig. 6A and B represent the data set described in Section 2.8, where only one fuel was burned for each experiment in the experimental series used for PMF analysis. The time series presented in Fig. 6A, includes markers that representative of aging conditions on each experimental date, distinguishing between primary, dark-, and photo-aged emissions. The time series was used to identify fuels with the highest mass contributions per factor, as well as the aging conditions that consistently exhibited the largest mass contributions. More specifically, Factor 2 was identified as BBOA POA based on the time series and analysis of the corresponding mass spectra data. This confirmed that in each experiment, where primary emissions dominated the mass fraction in the time series, supporting the elevated f60 in the mass spectra, characteristic of primary BBOA. Using this method, we identified the primary fuel and/or aging perturbation contributor for each factor. Specifically, Factor 1 was dominated by dark-aged mopane leaf emissions (RH
) was the largest contributor to Factor 1, primary mokala emissions (RH ≥ 65%) had the highest Factor 2, humidified primary BBOA mokala emissions (RH ≥ 65%) had the highest Factor 3, and primary mopane leaf emissions (RH ≥ 65%) had the highest contribution to Factor 4.
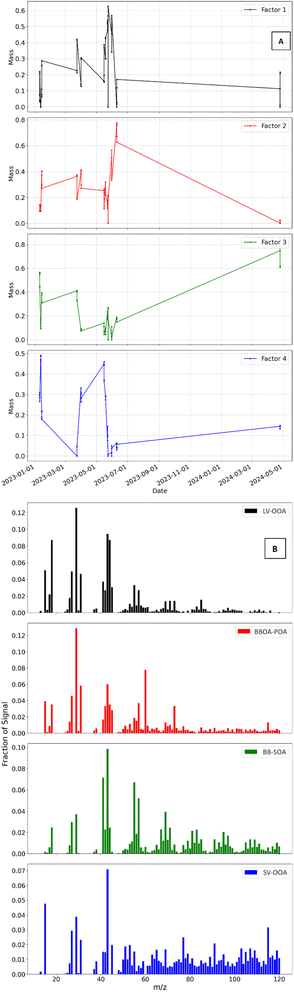 |
| Fig. 6 (A) Time series of mass concentrations of the four factors, the fraction of mass contribution is reported on the y-axis, and ACSM local time is presented on the x-axis. (B) Mass spectra displaying a fraction of signal per factor for the four-factor solution (LV-OOA (Factor 1), BBOA POA (Factor 2), BBSOA (Factor 3), and SV-OOA (Factor 4)). | |
The following findings were found via further analysis of Fig. 6B. F2 represents primary BBOA emissions and decreases in factor contribution with aging, as expected upon dark- and photo-aging. F1 and F4 are representative of SOA being produced because of aging, and we see their contributions increasing within each experiment upon aging. The results are further supported by analysis of BB markers f44 and f60 followed by f43 and f73. Noting an elevated f60 and f73 for F2 and elevated f44 and f43 for F1 and F4.
F1 and F4 are representative of the effects of photochemical aging and are further characterized using the comparison between f43 and f44 to compare the extent of oxidation/aging. This is also seen in the results published by Reyes-Villegas et al.90 The method of distinguishing between LV-OOA and SV-OOA was adopted by Ng et al.97 using ion fragments at m/z 43 and 44. Where f43 > f44 signifies less photochemically aged OA, or SV-OOA, and f43 < f44 signifies more aged LV-OOA.
The mass spectrum of F3 has the highest values of f55 and f57, where the greatest values are observed from mopane leaves and mokala. There was little to no contribution from cow dung for these f values. PMF results for F3, BBSOA show an elevated f44 and diluted f60. Exploration of other markers reveals that the f55/f57 ratio of 1.28 is comparable to the ∼1.2 value for woody-type biomass reported by Goetz et al.50 It is also worth noting that a nitrate radical nor dark aging factor could be deconvolved in the final solution of this study due to lower sensitivity and the narrower mass spectral range of the Q-ACSM.
4 Conclusions
Upon aging African-derived BB emissions, chemical changes (including the formation of SOA) were observed by our ACSM measurements. The monitoring of chemical changes via f values obtained from ACSM mass spectra reveals the importance of fuel type and the presence/absence of light when assessing the impact of BB emissions on aerosol chemistry. The difference in behavior under dark- and photo-aging conditions highlights the role of sunlight in the atmospheric aging of BB-derived aerosols.
We found that all biomass-burning organic aerosol (BBOA) samples underwent oxidation when aged. Among the combusted Africa-sourced fuels, Mokala exhibited the greatest extent of oxidation under all experimental conditions, as indicated by the significant increase in the f44 marker. Additionally, we observed a consistent decrease in the relative intensity of BBOA markers f60 and f73, corresponding to levoglucosan, across all fuels and experimental conditions. This decrease is primarily attributed to photolysis, with the rate of decline varying depending on the specific fuel.
The general trend of increased oxidation and decreased intensity in levoglucosan markers suggests a uniform aging process across BBOA fuels. However, Mokala's distinct behaviour suggests the need for more fuel-specific research to understand the underlying mechanisms driving these differences.
By examining the ratios of f55-to-f57 against f60 we were able to identify distinct clusters, based on fuel-specific mass spectral profiles, corresponding to different fuel types native to sub-Saharan Africa. The use of UMR mapping in this work reinforces its value as a tool in understanding BB emissions, offering insights into the compositional diversity and source apportionment of BB-derived aerosols produced in a laboratory smog chamber setting.
The application of PMF to the OA mass spectra representative of laboratory-combusted African-sourced biomass fuels is reported here with emphasis on primary, dark- and photo-aged, and NO3 radical perturbation under different experimental conditions. In this study, PMF identified four factors (LV-OOA, primary BBOA, BBSOA, and SV-OOA) using evidence from time series, mass spectra, and relevant BB markers. Data does not support a solution with more than four factors for this data set. The initial approach to PMF was to identify factors including those associated with dark aging and aging in the presence of
. Unfortunately, the latter was not achievable with the data sets from the ACSM, attributed to low resolution/sensitivity.
This work represents the first series of laboratory studies of near real-time chemical composition changes of BB aerosols derived from African biomass fuels. Despite the significant contributions of BB aerosols from the continent, there have not been any laboratory studies. Future studies will explore the molecular-level characterization of aged BBOA upon dark- and photo-aging via filter samples that correlate with experiments of this study.
This work will motivate more work in the region to quantity trends in emissions from least sampled regions of the world. Our chemical results provided here could be useful to future field deployments in Africa that use ACSM results.
Data availability
Data generated in these experiments and used to generate figures can be found at https://doi.org/10.6084/m9.figshare.26281102.
Author contributions
M. J.: running chamber experiments, data curation, formal analysis, investigation, methodology, visualization, and writing original draft; V. M.: investigation and methodology, editing and PMF analysis, M. McR. data curation, running chamber experiments M. N. F.: conceptualization, funding acquisition, investigation, and methodology; S. B.: conceptualization, funding acquisition, resources, and supervision. B. J. T.: conceptualization, funding acquisition, methodology, resources, and supervision. J. D. S.: conceptualization, funding acquisition, methodology, resources, and supervision. Review and editing: all co-authors.
Conflicts of interest
There are no conflicts to declare.
Acknowledgements
This work was supported by the US National Science Foundation (NSF) through the Atmospheric and Geospace Sciences (AGS) Division Grant # 2100708. The author (MJ) acknowledges that this work is partially supported by the Department of Education under the Title III HBGI grant. V. M. acknowledges support by the Swiss National Science Foundation (SNSF) under the Postdoc.Mobility Fellowship grant P500PN_210745. Any opinions, findings, conclusions, or recommendations expressed in this material are those of the author(s) and do not necessarily reflect the views of the Department of Education. The author (MJ) also acknowledges the help from Dr Axel Eriksson (Lund University) and Nicolas Buchenau (UNC) with the PMF analysis. The author would also like to acknowledge Phil Croteau (Aerodyne Inc.) for operational training associated with the ACSM. We thank Gizaw Mengistu Tsidu (BIUST)for providing biomass fuel samples from Botswana and Christina Isaxon (Lund University) for providing the cow-dung fuel sample from Ethiopia. We also acknowledge Kip Carrico and Mercy Ikes (New Mexico Tech) for conducting moisture content measurements.
Notes and references
- J. S. Reid, T. F. Eck, S. A. Christopher, R. Koppmann, O. Dubovik, D. P. Eleuterio, B. N. Holben, E. A. Reid and J. Zhang, A review of biomass burning emissions part III: intensive optical properties of biomass burning particles, Atmos. Chem. Phys., 2005, 5, 827–849 CrossRef.
- J. Chen, Z. Li, M. Lv, Y. Wang, W. Wang, Y. Zhang, H. Wang, X. Yan, Y. Sun and M. Cribb, Aerosol hygroscopic growth, contributing factors, and impact on haze events in a severely polluted region in northern China, Atmos. Chem. Phys., 2019, 19, 1327–1342 CrossRef CAS.
- H. Brown, X. Liu, R. Pokhrel, S. Murphy, Z. Lu, R. Saleh, T. Mielonen, H. Kokkola, T. Bergman, G. Myhre, R. B. Skeie, D. Watson-Paris, P. Stier, B. Johnson, N. Bellouin, M. Schulz, V. Vakkari, J. P. Beukes, P. G. Van Zyl, S. Liu and D. Chand, Biomass burning aerosols in most climate models are too absorbing, Nat. Commun., 2021, 12, 277 CrossRef CAS PubMed.
- M. O. Andreae, Emission of trace gases and aerosols from biomass burning – an updated assessment, Atmos. Chem. Phys., 2019, 19, 8523–8546 CrossRef CAS.
- D. G. Streets, T. C. Bond, T. Lee and C. Jang, On the future of carbonaceous aerosol emissions, J. Geophys. Res.: Atmos., 2004, 109, D24212 CrossRef.
- C. A. Randles and V. Ramaswamy, Impacts of Absorbing Biomass Burning Aerosol on the Climate of Southern Africa: A Geophysical Fluid Dynamics Laboratory GCM Sensitivity Study, Atmos. Chem. Phys. Discuss, 2010, 10, 9731–9752 Search PubMed.
- B. Johnson, S. Osborne, J. M. Haywood and M. A. Harrison, Aircraft Measurements of Biomass Burning Aerosol Over West Africa During DABEX, J. Geophys. Res.: Atmos., 2008, 113, D00C06 Search PubMed.
- J. Jimenez, N. Donahue, A. Prevot, Q. Zhang, J. Kroll, P. DeCarlo, J. D. Allan, H. Coe, N. Ng, A. Aiken, K. Docherty, I. Ulbrich, A. Grieshop, A. L. Robinson, J. Duplissy, J. Smith, K. Wilson, V. A. Lanz and D. Worsnop, Evolution of Organic Aerosols in the Atmosphere, Science, 2009, 326, 1525–1529 CrossRef CAS.
- H. Wu, J. W. Taylor, J. M. Langridge, C. Yu, J. D. Allan, K. Szpek, M. I. Cotterell, P. I. Williams, M. Flynn, P. Barker, C. Fox, G. Allen, J. Lee and H. Coe, Rapid transformation of ambient absorbing aerosols from West African biomass burning, Atmos. Chem. Phys., 2021, 21, 9417–9440 CrossRef CAS.
- N. Bellouin, J. Quaas, E. Gryspeerdt, S. Kinne, P. Stier, D. Watson-Parris, O. Boucher, K. S. Carslaw, M. Christensen, A. L. Daniau, J. L. Dufresne, G. Feingold, S. Fiedler, P. Forster, A. Gettelman, J. M. Haywood, U. Lohmann, F. Malavelle, T. Mauritsen, D. T. McCoy, G. Myhre, J. Mülmenstädt, D. Neubauer, A. Possner, M. Rugenstein, Y. Sato, M. Schulz, S. E. Schwartz, O. Sourdeval, T. Storelvmo, V. Toll, D. Winker and B. Stevens, Bounding Global Aerosol Radiative Forcing of Climate Change, Rev. Geophys., 2020, 58(1), 1–45 CrossRef.
- B. R. Simoneit and V. O. Elias, Detecting organic tracers from biomass burning in the atmosphere, Mar. Pollut. Bull., 2001, 42, 805–810 CrossRef PubMed.
- A. Dajuma, K. O. Ogunjobi, H. Vogel, P. Knippertz, S. Silué, E. T. N’Datchoh, V. Yoboue and B. Vogel, Cloud-Venting Induced Downward Mixing of the Central African Biomass Burning Plume During the West Africa Summer Monsoon, Chem. Phys., 2020, 20(9), 5373–5390 Search PubMed.
- G. Mafusire, H. J. Annegarn, V. Vakkari, J. P. Beukes, M. Josipovic, P. G. V. Zyl and L. Laakso, Submicrometer Aerosols and Excess CO as Tracers for Biomass Burning Air Mass Transport Over Southern Africa, J. Geophys. Res.: Atmos., 2016, 121(10), 10262–10282 CrossRef.
- L. Mammino, Biomass Burning in Sub-Saharan Africa: Chemical Issues and Action Outreach, 2020 Search PubMed.
- IEA, Financing Clean Energy in Africa, International Energy Agency, 2023 Search PubMed.
- R. U. Onyeneke, N. M. Chidiebere-Mark, D. A. Ankrah and L. U. Onyeneke, Determinants of Access to Clean Fuels and Technologies for Cooking in Africa: A Panel Autoregressive Distributed Lag Approach, Environ. Prog. Sustainable Energy, 2023, 42, 1–17 Search PubMed.
- I. M. F. C. Department, Picture This: African Century, Finance and Development, 2023, vol. 60, p. 73 Search PubMed.
- S. Bililign, S. S. Brown, D. M. Westervelt, R. Kumar, W. Tang, F. Flocke, W. Vizuete, K. Ture, F. D. Pope, B. Demoz, A. Asa-Awuku, P. F. Levelt, E. Kalisa, G. Raheja, A. Ndyabakira and M. J. Gatari, East African Megacity Air Quality: Rationale and Framework for a Measurement and Modeling Program, Bull. Am. Meteorol. Soc., 2024, 105(8), E1584–E1602 Search PubMed.
- P. J. Crutzen and M. O. Andreae, Biomass Burning in the Tropics: Impact on Atmospheric Chemistry and Biogeochemical Cycles, Science, 1990, 250, 1669–1678 CrossRef PubMed.
- A. P. Grieshop, N. M. Donahue and A. L. Robinson, Laboratory investigation of photochemical oxidation of organic aerosol from wood fires 2: analysis of aerosol mass spectrometer data, Atmos. Chem. Phys., 2009, 9, 2227–2240 CrossRef.
- P. Tiitta, A. Leskinen, L. Hao, P. Yli-Pirilä, M. Kortelainen, J. Grigonyte, J. Tissari, H. Lamberg, A. Hartikainen, K. Kuuspalo, A.-M. Kortelainen, A. Virtanen, K. E. J. Lehtinen, M. Komppula, S. Pieber, A. S. H. Prévôt, T. B. Onasch, D. R. Worsnop, H. Czech, R. Zimmermann, J. Jokiniemi and O. Sippula, Transformation of logwood combustion emissions in a smog chamber: formation of secondary organic aerosol and changes in the primary organic aerosol upon daytime and nighttime aging, Atmos. Chem. Phys., 2016, 16, 13251–13269 CrossRef CAS.
- E. Kalisa, S. Archer, E. Nagato, E. Bizuru, K. Lee, N. Tang, S. Pointing, K. Hayakawa and D. Lacap-Bugler, Chemical and Biological Components of Urban Aerosols in Africa: Current Status and Knowledge Gaps, Int. J. Environ. Res. Public Health, 2019, 16, 941 CrossRef CAS.
- E. Kalisa, E. G. Nagato, E. Bizuru, K. C. Lee, N. Tang, S. B. Pointing, K. Hayakawa, S. D. J. Archer and D. C. Lacap-Bugler, Characterization and Risk Assessment of Atmospheric PM2.5 and PM10 Particulate-Bound PAHs and NPAHs in Rwanda, Central-East Africa, Environ. Sci. Technol., 2018, 52, 12179–12187 CrossRef CAS.
- F. Li, X. Zhang, D. P. Roy and S. Kondragunta, Estimation of biomass-burning emissions by fusing the fire radiative power retrievals from polar-orbiting and geostationary satellites across the conterminous United States, Atmos. Environ., 2019, 211, 274–287 CrossRef CAS.
- S. M. Gaita, J. Boman, M. J. Gatari, J. B. C. Pettersson and S. Janhäll, Source apportionment and seasonal variation of PM2.5 in a Sub-Saharan African city: Nairobi, Kenya, Atmos. Chem. Phys., 2014, 14, 9977–9991 CrossRef.
- V. Moschos, C. Christensen, M. Mouton, M. N. Fiddler, T. Isolabella, F. Mazzei, D. Massabò, B. J. Turpin, S. Bililign and J. D. Surratt, Quantifying the Light-Absorption Properties and Molecular Composition of Brown Carbon Aerosol from Sub-Saharan African Biomass Combustion, Environ. Sci. Technol., 2024, 58(9), 4268–4280 CAS.
- J. H. Kroll, N. M. Donahue, J. L. Jimenez, S. Kessler, M. R. Canagaratna, K. R. Wilson, K. E. Altieri, L. Mazzoleni, A. S. Wozniak, H. Bluhm, E. R. Mysak, J. D. Smith, C. E. Kolb and D. R. Worsnop, Carbon Oxidation State as a Metric for Describing the Chemistry of Atmospheric Organic Aerosol, Nat. Chem., 2011, 3, 133–139 CrossRef CAS PubMed.
- P. J. Ziemann and R. Atkinson, Kinetics, Products, and Mechanisms of Secondary Organic Aerosol Formation, Chem. Soc. Rev., 2012, 41, 6582 RSC.
- F. Bianchi, T. Kurtén, M. Riva, C. Mohr, M. Rissanen, P. Roldin, T. Berndt, J. D. Crounse, P. O. Wennberg, T. F. Mentel, J. Wildt, H. Junninen, T. Jokinen, M. Kulmala, D. R. Worsnop, J. A. Thornton, N. M. Donahue, H. G. Kjaergaard and M. Ehn, Highly Oxygenated Organic Molecules (HOM) From Gas-Phase Autoxidation Involving Peroxy Radicals: A Key Contributor to Atmospheric Aerosol, Chem. Rev., 2019, 119, 3472–3509 CrossRef CAS PubMed.
- W. Wei, C. Mandin, O. Blanchard, F. Mercier, M. Pelletier, B. Le Bot, P. Glorennec and O. Ramalho, Temperature dependence of the particle/gas partition coefficient: An application to predict indoor gas-phase concentrations of semi-volatile organic compounds, Sci. Total Environ., 2016, 563–564, 506–512 CrossRef CAS PubMed.
- A. M. Ortega, D. A. Day, M. J. Cubison, W. H. Brune, D. Bon, J. A. De Gouw and J. L. Jimenez, Secondary organic aerosol formation and primary organic aerosol oxidation from biomass-burning smoke in a flow reactor during FLAME-3, Atmos. Chem. Phys., 2013, 13, 11551–11571 CrossRef.
- A. M. Ortega, P. L. Hayes, Z. Peng, B. B. Palm, W. Hu, D. A. Day, R. Li, M. J. Cubison, W. H. Brune, M. Graus, C. Warneke, J. B. Gilman, W. C. Kuster, J. De Gouw, C. Gutiérrez-Montes and J. L. Jimenez, Real-time measurements of secondary organic aerosol formation and aging from ambient air in an oxidation flow reactor in the Los Angeles area, Atmos. Chem. Phys., 2016, 16, 7411–7433 CrossRef.
- D. Srivastava, T. V. Vu, S. Tong, Z. Shi and R. M. Harrison, Formation of secondary organic aerosols from anthropogenic precursors in laboratory studies, npj Clim. Atmos. Sci., 2022, 5, 22 CrossRef.
- B. Nozière, M. Kalberer, M. Claeys, J. Allan, B. D'Anna, S. Decesari, E. Finessi, M. Glasius, I. Grgić, J. F. Hamilton, T. Hoffmann, Y. Iinuma, M. Jaoui, A. Kahnt, C. J. Kampf, I. Kourtchev, W. Maenhaut, N. Marsden, S. Saarikoski, J. Schnelle-Kreis, J. D. Surratt, S. Szidat, R. Szmigielski and A. Wisthaler, The molecular identification of organic compounds in the atmosphere: state of the art and challenges, Chem. Rev., 2015, 115, 3919–3983 CrossRef.
- M. Hallquist, J. C. Wenger, U. Baltensperger, Y. Rudich, D. Simpson, M. Claeys, J. Dommen, N. M. Donahue, C. George, A. H. Goldstein, J. F. Hamilton, H. Herrmann, T. Hoffmann, Y. Iinuma, M. Jang, M. E. Jenkin, J. L. Jimenez, A. Kiendler-Scharr, W. Maenhaut, G. McFiggans, T. F. Mentel, A. Monod, A. S. H. Prévôt, J. H. Seinfeld, J. D. Surratt, R. Szmigielski and J. Wildt, The formation, properties and impact of secondary organic aerosol: current and emerging issues, Atmos. Chem. Phys., 2009, 9, 5155–5236 CrossRef.
- C. J. Hennigan, M. A. Miracolo, G. J. Engelhart, A. A. May, A. A. Presto, T. Lee, A. P. Sullivan, G. R. McMeeking, H. Coe, C. E. Wold, W. M. Hao, J. B. Gilman, W. C. Kuster, J. De Gouw, B. A. Schichtel, J. L. Collett, S. M. Kreidenweis and A. L. Robinson, Chemical and physical transformations of organic aerosol from the photo-oxidation of open biomass burning emissions in an environmental chamber, Atmos. Chem. Phys., 2011, 11, 7669–7686 CrossRef.
- V. Vakkari, V. M. Kerminen, J. P. Beukes, P. Tiitta, P. G. Zyl, M. Josipovic, A. D. Venter, K. Jaars, D. R. Worsnop, M. Kulmala and L. Laakso, Rapid changes in biomass burning aerosols by atmospheric oxidation, Geophys. Res. Lett., 2014, 41, 2644–2651 CrossRef.
- S. Tomaz, T. Cui, Y. Chen, K. G. Sexton, J. M. Roberts, C. Warneke, R. J. Yokelson, J. D. Surratt and B. J. Turpin, Photochemical Cloud Processing of Primary Wildfire Emissions as a Potential Source of Secondary Organic Aerosol, Environ. Sci. Technol., 2018, 52, 11027–11037 CrossRef PubMed.
- S. Gilardoni, P. Massoli, M. Paglione, L. Giulianelli, C. Carbone, M. Rinaldi, S. Decesari, S. Sandrini, F. Costabile, G. P. Gobbi, M. C. Pietrogrande, M. Visentin, F. Scotto, S. Fuzzi and M. C. Facchini, Direct observation of aqueous secondary organic aerosol from biomass-burning emissions, Proc. Natl. Acad. Sci. U. S. A., 2016, 113, 10013–10018 CrossRef.
- J. D. Blando and B. J. Turpin, Secondary organic aerosol formation in cloud and fog droplets: a literature evaluation of plausibility, Atmos. Environ., 2000, 34, 1623–1632 CrossRef.
- A. Milic, M. D. Mallet, L. T. Cravigan, J. Alroe, Z. D. Ristovski, P. Selleck, S. J. Lawson, J. Ward, M. J. Desservettaz, C. Paton-Walsh, L. R. Williams, M. D. Keywood and B. Miljevic, Biomass burning and biogenic aerosols in northern Australia during the SAFIRED campaign, Atmos. Chem. Phys., 2017, 17, 3945–3961 CrossRef.
- M. Shrivastava, R. C. Easter, X. Liu, A. Zelenyuk, B. Singh, K. Zhang, P. L. Ma, D. Chand, S. Ghan, J. L. Jimenez, Q. Zhang, J. Fast, P. J. Rasch and P. Tiitta, Global transformation and fate of SOA: Implications of low-volatility SOA and gas-phase fragmentation reactions, J. Geophys. Res.: Atmos., 2015, 120, 4169–4195 CrossRef.
- G. R. McMeeking, S. M. Kreidenweis, S. Baker, C. M. Carrico, J. C. Chow, J. L. Collett, W. M. Hao, A. S. Holden, T. W. Kirchstetter, W. C. Malm, H. Moosmüller, A. P. Sullivan and C. E. Wold, Emissions of trace gases and aerosols during the open combustion of biomass in the laboratory, J. Geophys. Res., 2009, 114, D19210 CrossRef.
- G. Engling, C. M. Carrico, S. M. Kreidenweis, J. L. Collett, D. E. Day, W. C. Malm, E. Lincoln, W. Min Hao, Y. Iinuma and H. Herrmann, Determination of levoglucosan in biomass combustion aerosol by high-performance anion-exchange chromatography with pulsed amperometric detection, Atmos. Environ., 2006, 40, 299–311 CrossRef.
- R. K. Chakrabarty, H. Moosmüller, M. A. Garro, W. P. Arnott, J. Walker, R. A. Susott, R. E. Babbitt, C. E. Wold, E. N. Lincoln and W. M. Hao, Emissions from the laboratory combustion of wildland fuels: Particle morphology and size, J. Geophys. Res.: Atmos., 2006, 111, D07204 Search PubMed.
- L. W. A. Chen, H. Moosmüller, W. P. Arnott, J. C. Chow, J. G. Watson, R. A. Susott, R. E. Babbitt, C. E. Wold, E. N. Lincoln and W. M. Hao, Particle emissions from laboratory combustion of wildland fuels: In situ optical and mass measurements, Geophys. Res. Lett., 2006, 33, L04803 Search PubMed.
- T. J. Christian, B. Kleiss, R. J. Yokelson, R. Holzinger, P. J. Crutzen, W. M. Hao, B. H. Saharjo and D. E. Ward, Comprehensive laboratory measurements of biomass-burning emissions: 1. Emissions from Indonesian, African, and other fuels, J. Geophys. Res.: Atmos., 2003, 108(D23), 4719 CrossRef.
- Y. Deng, H. Fujinari, H. Yai, K. Shimada, Y. Miyazaki, E. Tachibana, D. K. Deshmukh, K. Kawamura, T. Nakayama, S. Tatsuta, M. Cai, H. Xu, F. Li, H. Tan, S. Ohata, Y. Kondo, A. Takami, S. Hatakeyama and M. Mochida, Offline analysis of the chemical composition and hygroscopicity of submicrometer aerosol at an Asian outflow receptor site and comparison with online measurements, Atmos. Chem. Phys., 2022, 22, 5515–5533 CrossRef.
- C. D. Cappa, C. Y. Lim, D. H. Hagan, M. Coggon, A. Koss, K. Sekimoto, J. De Gouw, T. B. Onasch, C. Warneke and J. H. Kroll, Biomass-burning-derived particles from a wide variety of fuels – Part 2: Effects of photochemical aging on particle optical and chemical properties, Atmos. Chem. Phys., 2020, 20, 8511–8532 CrossRef.
- J. D. Goetz, M. R. Giordano, C. E. Stockwell, P. V. Bhave, P. S. Puppala, A. K. Panday, T. Jayarathne, E. A. Stone, R. J. Yokelson and P. F. Decarlo, Aerosol Mass Spectral Profiles from NAMaSTE Field-Sampled South Asian Combustion Sources, ACS Earth Space Chem., 2022, 6, 2619–2631 CrossRef PubMed.
- A. P. Sullivan, H. Guo, J. C. Schroder, P. Campuzano-Jost, J. L. Jimenez, T. Campos, V. Shah, L. Jaeglé, B. H. Lee, F. D. Lopez-Hilfiker, J. A. Thornton, S. S. Brown and R. J. Weber, Biomass Burning Markers and Residential Burning in the WINTER Aircraft Campaign, J. Geophys. Res.: Atmos., 2019, 124, 1846–1861 CrossRef.
- M. J. Cubison, A. M. Ortega, P. L. Hayes, D. K. Farmer, D. Day, M. J. Lechner, W. H. Brune, E. Apel, G. S. Diskin, J. A. Fisher, H. E. Fuelberg, A. Hecobian, D. J. Knapp, T. Mikoviny, D. Riemer, G. W. Sachse, W. Sessions, R. J. Weber, A. J. Weinheimer, A. Wisthaler and J. L. Jimenez, Effects of aging on organic aerosol from open biomass burning smoke in aircraft and laboratory studies, Atmos. Chem. Phys., 2011, 11, 12049–12064 CrossRef.
- J. Riaza, R. M. Khatami, Y. A. Levendis, L. Álvarez, M. V. Gil, C. Pevida, F. Rubiera and J. J. Pis, Combustion of single biomass particles in air and in oxy-fuel conditions, Biomass Bioenergy, 2014, 64, 162–174 CrossRef.
- C. Y. Lim, D. H. Hagan, M. M. Coggon, A. R. Koss, K. Sekimoto, J. De Gouw, C. Warneke, C. D. Cappa and J. H. Kroll, Secondary organic aerosol formation from the laboratory oxidation of biomass burning emissions, Atmos. Chem. Phys., 2019, 19, 12797–12809 CrossRef.
- C. F. Fortenberry, M. J. Walker, Y. Zhang, D. Mitroo, W. H. Brune and B. J. Williams, Bulk and molecular-level characterization of laboratory-aged biomass burning organic aerosol from oak leaf and heartwood fuels, Atmos. Chem. Phys., 2018, 18, 2199–2224 CrossRef.
- N. L. Ng, S. C. Herndon, A. Trimborn, M. R. Canagaratna, P. L. Croteau, T. B. Onasch, D. Sueper, D. R. Worsnop, Q. Zhang, Y. L. Sun and J. T. Jayne, An Aerosol Chemical Speciation Monitor (ACSM) for Routine Monitoring of the Composition and Mass Concentrations of Ambient Aerosol, Aerosol Sci. Technol., 2011, 45, 780–794 CrossRef.
- G. Adler, J. M. Flores, A. Abo Riziq, S. Borrmann and Y. Rudich, Chemical, physical, and optical evolution of biomass burning aerosols: a case study, Atmos. Chem. Phys., 2011, 11, 1491–1503 CrossRef.
- A. Yazdani, S. Takahama, J. K. Kodros, M. Paglione, M. Masiol, S. Squizzato, K. Florou, C. Kaltsonoudis, S. D. Jorga, S. N. Pandis and A. Nenes, Chemical evolution of primary and secondary biomass burning aerosols during daytime and nighttime, Atmos. Chem. Phys., 2023, 23, 7461–7477 CrossRef.
- R. Yokelson, D. Griffith and D. Ward, Open-path Fourier transform infrared studies of large-scale laboratory biomass fires, J. Geophys. Res., 1996, 101, 21067 CrossRef.
- R. P. Pokhrel, J. Gordon, M. N. Fiddler and S. Bililign, Impact of combustion conditions on physical and morphological properties of biomass burning aerosol, Aerosol Sci. Technol., 2021, 55, 80–91 CrossRef.
- M. McRee, V. Moschos, M. N. Fiddler, D. Massabò, J. D. Surratt and S. Bililign, Influence of relative humidity and aging on the optical properties of organic aerosols from burning African biomass fuels, Aerosol Sci. Technol., 2024 DOI:10.1080/02786826.2024.2412652.
- M. Mouton, K. A. Malek, M. S. H. James, R. P. Pokhrel, M. N. Fiddler, A. A. Asa-Awuku and S. Bililign, The hygroscopic properties of biomass burning aerosol from Eucalyptus and cow dung under different combustion conditions, Aerosol Sci. Technol., 2023, 57, 665–677 CrossRef.
- D. M. Smith, T. Cui, M. N. Fiddler, R. P. Pokhrel, J. D. Surratt and S. Bililign, Laboratory studies of fresh and aged biomass burning aerosol emitted from east African biomass fuels – Part 2: Chemical properties and characterization, Atmos. Chem. Phys., 2020, 20, 10169–10191 CrossRef.
- D. M. Smith, M. N. Fiddler, R. P. Pokhrel and S. Bililign, Laboratory studies of fresh and aged biomass burning aerosol emitted from east African biomass fuels – Part 1: Optical properties, Atmos. Chem. Phys., 2020, 20, 10149–10168 CrossRef CAS.
- Z. B. Babar, J. H. Park, J. Kang and H. J. Lim, Characterization of a smog chamber for studying formation and physicochemical properties of secondary organic aerosol, Aerosol Air Qual. Res., 2016, 16, 3102–3113 CrossRef.
- D. M. Smith, M. N. Fiddler, K. G. Sexton and S. Bililign, Construction and Characterization of an Indoor Smog Chamber for Measuring the Optical and Physicochemical Properties of Aging Biomass Burning Aerosols, Aerosol Air Qual. Res., 2019, 19, 467–483 CrossRef CAS.
- N. L. Ng, M. R. Canagaratna, J. L. Jimenez, Q. Zhang, I. M. Ulbrich and D. R. Worsnop, Real-Time Methods for Estimating Organic Component Mass Concentrations from Aerosol Mass Spectrometer Data, Environ. Sci. Technol., 2011, 45, 910–916 CrossRef CAS PubMed.
- B. R. T. Simoneit, J. J. Schauer, C. G. Nolte, D. R. Oros, V. O. Elias, M. P. Fraser, W. F. Rogge and G. R. Cass, Levoglucosan, a tracer for cellulose in biomass burning and atmospheric particles, Atmos. Environ., 1999, 33, 173–182 CrossRef CAS.
- I. M. Ulbrich, M. R. Canagaratna, Q. Zhang, D. R. Worsnop and J. L. Jimenez, Interpretation of organic components from Positive Matrix Factorization of aerosol mass spectrometric data, Atmos. Chem. Phys., 2009, 9, 2891–2918 CrossRef CAS.
- A. M. Sage, E. A. Weitkamp, A. L. Robinson and N. M. Donahue, Evolving mass spectra of the oxidized component of organic aerosol: results from aerosol mass spectrometer analyses of aged diesel emissions, Atmos. Chem. Phys., 2008, 8, 1139–1152 CrossRef CAS.
- M. R. Alfarra, A. S. H. Prevot, S. Szidat, J. Sandradewi, S. Weimer, V. A. Lanz, D. Schreiber, M. Mohr and U. Baltensperger, Identification of the Mass Spectral Signature of Organic Aerosols from Wood Burning Emissions, Environ. Sci. Technol., 2007, 41, 5770–5777 CrossRef CAS.
- M. R. Alfarra, A. S. H. Prevot, S. Szidat, J. Sandradewi, S. Weimer, V. A. Lanz, D. Schreiber, M. Mohr and U. Baltensperger, Identification of the Mass Spectral Signature of Organic Aerosols from Wood Burning Emissions, Environ. Sci. Technol., 2007, 41, 5770–5777 CrossRef CAS PubMed.
- T. Lee, A. P. Sullivan, L. Mack, J. L. Jimenez, S. M. Kreidenweis, T. B. Onasch, D. R. Worsnop, W. Malm, C. E. Wold, W. M. Hao and J. L. Collett Jr, Chemical Smoke Marker Emissions During Flaming and Smoldering Phases of Laboratory Open Burning of Wildland Fuels, Aerosol Sci. Technol., 2010, 44, i–v CrossRef CAS.
- P. Paatero and U. Tapper, Positive matrix factorization: A non-negative factor model with optimal utilization of error estimates of data values, Environmetrics, 1994, 5, 111–126 CrossRef.
- Y. Zhang, L. Tang, P. L. Croteau, O. Favez, Y. Sun, M. R. Canagaratna, Z. Wang, F. Couvidat, A. Albinet, H. Zhang, J. Sciare, A. S. H. Prévôt, J. T. Jayne and D. R. Worsnop, Field characterization of the PM2.5 Aerosol Chemical Speciation Monitor: insights into the composition, sources, and processes of fine particles in eastern China, Atmos. Chem. Phys., 2017, 17, 14501–14517 CrossRef CAS.
- Q. Zhang, J. L. Jimenez, M. R. Canagaratna, I. M. Ulbrich, N. L. Ng, D. R. Worsnop and Y. Sun, Understanding atmospheric organic aerosols via factor analysis of aerosol mass spectrometry: a review, Anal. Bioanal. Chem., 2011, 401, 3045–3067 CrossRef.
- M. Jaoui, S. P. Urbanski, R. W. Long and M. S. Landis, Molecular composition and the impact of fuel moisture content on fresh primary organic aerosol emissions during laboratory combustion of ponderosa pine needles, Environ. Chem., 2023, 20, 319–338 CrossRef.
- D. França, K. M. Longo, T. G. S. Neto, J. C. d. Santos, S. R. Freitas, B. F. T. Rudorff, E. V. Cortez, E. Anselmo and J. A. d. Carvalho, Pre-Harvest Sugarcane Burning: Determination of Emission Factors Through Laboratory Measurements, Atmosphere, 2012, 3, 164–180 CrossRef.
- R. J. Yokelson, J. D. Crounse, P. F. DeCarlo, T. Karl, S. Urbanski, E. Atlas, T. Campos, Y. Shinozuka, V. Kapustin, A. D. Clarke, A. Weinheimer, D. J. Knapp, D. D. Montzka, J. Holloway, P. Weibring, F. Flocke, W. Zheng, D. Toohey, P. O. Wennberg, C. Wiedinmyer, L. Mauldin, A. Fried, D. Richter, J. Walega, J. L. Jimenez, K. Adachi, P. R. Buseck, S. R. Hall and R. Shetter, Emissions from biomass burning in the Yucatan, Atmos. Chem. Phys., 2009, 9, 5785–5812 CrossRef.
- M. D. Jolleys, H. Coe, G. McFiggans, G. Capes, J. D. Allan, J. Crosier, P. I. Williams, G. Allen, K. Bower, J. L. Jimenez, L. M. Russell, M. Grütter and D. Baumgardner, Characterizing the Aging of Biomass Burning Organic Aerosol by Use of Mixing Ratios: A Meta-Analysis of Four Regions, Environ. Sci. Technol., 2012, 46, 13093–13102 CrossRef PubMed.
- W. Xu, Y. He, Y. Qiu, C. Chen, C. Xie, L. Lei, Z. Li, J. Sun, J. Li, P. Fu, Z. Wang, D. R. Worsnop and Y. Sun, Mass spectral characterization of primary emissions and implications in source apportionment of organic aerosol, Atmos. Meas. Tech., 2020, 13, 3205–3219 CrossRef.
- J. K. Kodros, D. K. Papanastasiou, M. Paglione, M. Masiol, S. Squizzato, K. Florou, K. Skyllakou, C. Kaltsonoudis, A. Nenes and S. N. Pandis, Rapid dark aging of biomass burning as an overlooked source of oxidized organic aerosol, Proc. Natl. Acad. Sci. U. S. A., 2020, 117, 33028–33033 CrossRef PubMed.
- D. Srivastava, O. Favez, J. E. Petit, Y. Zhang, U. M. Sofowote, P. K. Hopke, N. Bonnaire, E. Perraudin, V. Gros, E. Villenave and A. Albinet, Speciation of organic fractions does matter for aerosol source apportionment. Part 3: Combining off-line and on-line measurements, Sci. Total Environ., 2019, 690, 944–955 CrossRef CAS PubMed.
- C. D. Mcclure, C. Y. Lim, D. H. Hagan, J. H. Kroll and C. D. Cappa, Biomass-burning-derived particles from a wide variety of fuels – Part 1: Properties of primary particles, Atmos. Chem. Phys., 2020, 20, 1531–1547 CrossRef CAS.
- Q. Zhang, M. R. Alfarra, D. R. Worsnop, J. D. Allan, H. Coe, M. R. Canagaratna and J. L. Jimenez, Deconvolution and Quantification of Hydrocarbon-like and Oxygenated Organic Aerosols Based on Aerosol Mass Spectrometry, Environ. Sci. Technol., 2005, 39, 4938–4952 CrossRef CAS PubMed.
- M. D. Jolleys, H. Coe, G. McFiggans, J. W. Taylor, S. J. O'Shea, M. Le Breton, S. J. B. Bauguitte, S. Moller, P. Di Carlo, E. Aruffo, P. I. Palmer, J. D. Lee, C. J. Percival and M. W. Gallagher, Properties and evolution of biomass burning organic aerosol from Canadian boreal forest fires, Atmos. Chem. Phys., 2015, 15, 3077–3095 CrossRef CAS.
- J. Zhang, K. Li, T. Wang, E. Gammelsæter, R. K. Y. Cheung, M. Surdu, S. Bogler, D. Bhattu, D. S. Wang, T. Cui, L. Qi, H. Lamkaddam, I. El Haddad, J. G. Slowik, A. S. H. Prevot and D. M. Bell, Bulk and molecular-level composition of primary organic aerosol from wood, straw, cow dung, and plastic burning, Atmos. Chem. Phys., 2023, 23, 14561–14576 CrossRef CAS.
- B. Vincenti, E. Paris, M. Carnevale, A. Palma, E. Guerriero, D. Borello, V. Paolini and F. Gallucci, Saccharides as Particulate Matter Tracers of Biomass Burning: A Review, Int. J. Environ. Res. Public Health, 2022, 19, 4387 CrossRef CAS.
- A. T. Ahern, E. S. Robinson, D. S. Tkacik, R. Saleh, L. E. Hatch, K. C. Barsanti, C. E. Stockwell, R. J. Yokelson, A. A. Presto, A. L. Robinson, R. C. Sullivan and N. M. Donahue, Production of Secondary Organic Aerosol During Aging of Biomass Burning Smoke From Fresh Fuels and Its Relationship to VOC Precursors, J. Geophys. Res.: Atmos., 2019, 124, 3583–3606 CrossRef CAS.
- E. Reyes-Villegas, D. C. Green, M. Priestman, F. Canonaco, H. Coe, A. S. H. Prévôt and J. D. Allan, Organic aerosol source apportionment in London 2013 with ME-2: exploring the solution space with annual and seasonal analysis, Atmos. Chem. Phys., 2016, 16, 15545–15559 CrossRef.
- R. Zhang, A. F. Khalizov, J. Pagels, D. Zhang, H. Xue and P. H. McMurry, Variability in morphology, hygroscopicity, and optical properties of soot aerosols during atmospheric processing, Proc. Natl. Acad. Sci. U. S. A., 2008, 105, 10291–10296 CrossRef CAS.
- A. Bougiatioti, I. Stavroulas, E. Kostenidou, P. Zarmpas, C. Theodosi, G. Kouvarakis, F. Canonaco, A. S. H. Prévôt, A. Nenes, S. N. Pandis and N. Mihalopoulos, Processing of biomass-burning aerosol in the eastern Mediterranean during summertime, Atmos. Chem. Phys., 2014, 14, 4793–4807 CrossRef.
- J. K. Kodros, C. Kaltsonoudis, M. Paglione, K. Florou, S. Jorga, C. Vasilakopoulou, M. Cirtog, M. Cazaunau, B. Picquet-Varrault, A. Nenes and S. N. Pandis, Secondary aerosol formation during the dark oxidation of residential biomass burning emissions, Environ. Sci.: Atmos., 2022, 2, 1221–1236 CAS.
- J. L. Fry, S. S. Brown, A. M. Middlebrook, P. M. Edwards, P. Campuzano-Jost, D. A. Day, J. L. Jimenez, H. M. Allen, T. B. Ryerson, I. Pollack, M. Graus, C. Warneke, J. A. De Gouw, C. A. Brock, J. Gilman, B. M. Lerner, W. P. Dubé, J. Liao and A. Welti, Secondary organic aerosol (SOA) yields from NO3 radical + isoprene based on nighttime aircraft power plant plume transects, Atmos. Chem. Phys., 2018, 18, 11663–11682 CrossRef CAS.
- N. L. Ng, S. S. Brown, A. T. Archibald, E. Atlas, R. C. Cohen, J. N. Crowley, D. A. Day, N. M. Donahue, J. L. Fry, H. Fuchs, R. J. Griffin, M. I. Guzman, H. Herrmann, A. Hodzic, Y. Iinuma, J. L. Jimenez, A. Kiendler-Scharr, B. H. Lee, D. J. Luecken, J. Mao, R. McLaren, A. Mutzel, H. D. Osthoff, B. Ouyang, B. Picquet-Varrault, U. Platt, H. O. T. Pye, Y. Rudich, R. H. Schwantes, M. Shiraiwa, J. Stutz, J. A. Thornton, A. Tilgner, B. J. Williams and R. A. Zaveri, Nitrate radicals and biogenic volatile organic compounds: oxidation, mechanisms, and organic aerosol, Atmos. Chem. Phys., 2017, 17, 2103–2162 CrossRef CAS.
- L. Xu, S. Suresh, H. Guo, R. J. Weber and N. L. Ng, Aerosol characterization over the southeastern United States using high-resolution aerosol mass spectrometry: spatial and seasonal variation of aerosol composition and sources with a focus on organic
nitrates, Atmos. Chem. Phys., 2015, 15, 7307–7336 CrossRef CAS.
- N. L. Ng, M. R. Canagaratna, Q. Zhang, J. L. Jimenez, J. Tian, I. M. Ulbrich, J. H. Kroll, K. S. Docherty, P. S. Chhabra, R. Bahreini, S. M. Murphy, J. H. Seinfeld, L. Hildebrandt, N. M. Donahue, P. F. Decarlo, V. A. Lanz, A. S. H. Prévôt, E. Dinar, Y. Rudich and D. R. Worsnop, Organic aerosol components observed in Northern Hemispheric datasets from Aerosol Mass Spectrometry, Atmos. Chem. Phys., 2010, 10, 4625–4641 CrossRef.
- J. A. Huffman, K. S. Docherty, A. C. Aiken, M. J. Cubison, I. M. Ulbrich, P. F. Decarlo, D. Sueper, J. T. Jayne, D. R. Worsnop, P. J. Ziemann and J. L. Jimenez, Chemically-resolved aerosol volatility measurements from two megacity field studies, Atmos. Chem. Phys., 2009, 9, 7161–7182 CrossRef.
- J. Duan, R.-J. Huang, Y. Gu, C. Lin, H. Zhong, W. Xu, Q. Liu, Y. You, J. Ovadnevaite, D. Ceburnis, T. Hoffmann and C. O'Dowd, Measurement report: Large contribution of biomass burning and aqueous-phase processes to the wintertime secondary organic aerosol formation in Xi'an, Northwest China, Atmos. Chem. Phys., 2022, 22, 10139–10153 CrossRef.
- M. Paglione, S. Gilardoni, M. Rinaldi, S. Decesari, N. Zanca, S. Sandrini, L. Giulianelli, D. Bacco, S. Ferrari, V. Poluzzi, F. Scotto, A. Trentini, L. Poulain, H. Herrmann, A. Wiedensohler, F. Canonaco, A. S. H. Prévôt, P. Massoli, C. Carbone, M. C. Facchini and S. Fuzzi, The impact of biomass burning and aqueous-phase processing on air quality: a multi-year source apportionment study in the Po Valley, Italy, Atmos. Chem. Phys., 2020, 20, 1233–1254 CrossRef.
|
This journal is © The Royal Society of Chemistry 2024 |
Click here to see how this site uses Cookies. View our privacy policy here.