Computational design of amyloid self-assembling peptides bearing aromatic residues and the cell adhesive motif Arg-Gly-Asp†
Received
7th March 2017
, Accepted 22nd June 2017
First published on 22nd June 2017
Abstract
The properties of self-assembling peptides can be tuned through changes at their sequence level, and thus amyloid-forming peptides are increasingly gaining interest as potential biomaterials for a series of applications. Recently, we rationally designed a self-assembling peptide with sequence RGDSGAITIGC, which comprises the amyloidogenic core GAITIG, extracted from the adenovirus fiber shaft, as well as the functional motif RGD and a cysteine residue encompassing cell attachment properties and an exposed free thiol group, respectively. Here, we aimed to further stabilize the aforementioned amyloid fibril by modifying the last residue position and observed that aromatic residues, including tyrosine, contribute to the stabilization of the amyloid fibril through the formation of π–π interactions in the parallel β-sheets formed by the peptides. Inspired by the proximity of tyrosine–tyrosine residues in the intermolecular aromatic network of the newly designed fibrils, we computationally investigated the capacity of a designed amyloid-forming peptide to concurrently possess dityrosine crosslinking formation potential in addition to cell adhesive properties. We experimentally confirmed the ability of the designer peptides to self-assemble into amyloid-type fibrils. The designed amyloid peptide biomaterials may constitute promising agents in future tissue engineering applications.
Design, System, Application
In this article, we introduced a computational strategy to energetically stabilize and potentially functionalize an amyloid peptide fibril. The flexible structures used as designable scaffolds in the model comprised of two independent sets of computationally derived, parallel and antiparallel highly ordered and well-aligned β-sheet states formed by peptide RGDSGAITIGC. A computational design model was employed at the beginning of the strategy to minimize the energy of the designable scaffolds upon a single amino acid mutation at position 11, so as to preserve the peptide's amyloidogenic and cell-adhesive properties. This enabled us to investigate the mutational effect on realistically modeled β-sheet arrangements of peptides. The introduction of aromatic (Tyr, Trp, Phe) or imidazole ring (His) groups at residue 11 were among the most energetically favorable mutations in parallel β-sheet bonded peptides. A posteriori, an additional constraint was introduced to the computational design model to investigate the crosslinking capacity of tyrosine residues belonging to adjacent β-sheet pairs of peptide RGDSGAITIGY. Furthermore, we used replica exchange molecular dynamics simulations and free energy calculations depicting that the designed peptides self-assemble into parallel β-sheets, and suggesting that the highly ordered and well-aligned amyloid fibrils formed by RGDSGAITIGY can combine potential cell adhesive and crosslinking properties. Experiments confirmed that designer peptides self-assemble into amyloid-type fibrils, and ongoing studies aim to exploit the predicted bifunctional properties of RGDSGAITIGY in biomaterial and tissue engineering applications.
|
Introduction
Self-assembly can be defined as the spontaneous organization of individual components into an ordered structure without human intervention.1 One of the key challenges in molecular self-assembly is the design of molecular building blocks that can undergo spontaneous organization into stable, well-defined macroscopic structures, and can simultaneously encompass functional properties into materials with a genuine future in real-life applications.2 The properties of self-assembling peptides and proteins can be tuned through changes at the peptides' sequence level, and thus they are increasingly gaining interest for their potential use as amyloid biomaterial scaffolds in many applications, including biomedicine and tissue-engineering,3,4 technology,5–12 as well as energy and environment.13–16 Computational methods are also increasingly becoming powerful tools in the investigation, structural elucidation and design of self-assembling protein peptides,17–34 and can provide impetus18 for the discovery of novel functional amyloid biomaterials.4,7–12
Naturally occurring sequences from amyloidogenic proteins or natural fibrous proteins may constitute elementary scaffolds for functional biomaterials.5,19 Senile plaques comprised of amyloid-β peptide (Aβ) and pancreatic islet amyloid comprised of islet amyloid polypeptide (IAPP) are pathological features of Alzheimer's disease and type 2 diabetes, respectively.35–38 Amyloidogenic fragments KLVFFAE39 extracted from Aβ as well as NFGAIL40 and NFLVHSS41 extracted from IAPP maintain their amyloidogenic properties and self-assemble outside the context of their respective full-length proteins. A series of studies, including the ones aforementioned, suggested that aromatic interactions are important in amyloid formation.20,42–44 For example, interactions between the aromatic residues of fragments KLVFFAE,39 NFGAIL,40 and NFLVHSS41 play a central role in molecular recognition and self-assembly,42 and remarkably, while the 5-residue fragment FGAIL derived from NFGAIL also forms ordered fibrils, the 4-residue fragment GAIL cannot.40 Apart from aromaticity, the presence of hydrophobic residues has also been suggested – in specific cases – to constitute a driving force toward amyloidogenicity. Within this context, peptide sequences extracted from β-sheet rich regions of fibrous proteins (e.g., the adenovirus fiber shaft45), can also self-assemble into amyloid fibrils outside the context of the entire protein.46 NSGAITIG is an example amyloid-forming sequence extracted from the adenovirus fiber shaft which has an exposed NS amino-terminal fragment that is not part of the amyloid β-sheet core.18 This result provided impetus for the design of functional biomaterials through introduced mutations and modifications to the N-terminal domain of the self-assembling peptide.4,7–11 Recently, we also utilized this finding to design a novel self-assembling peptide with sequence RGDSGAITIGC which can potentially be used as a novel sandwich self-assembly construct with multi-functional cell targeting and functionalization properties, toward geometrically and spatially directing the fate of a cell population.47
RGD is the principal integrin-binding domain present within extracellular matrix (ECM) proteins such as fibronectin, vitronectin, fibrinogen, osteopontin, and bone sialoprotein; due to the capacity of the RGD sequence to bind to multiple integrin species, synthetic RGD peptides offer several advantages for biomaterial applications.48 RGD bearing self-assembling peptide amphiphiles not only have cellular adhesion properties but also serve as ideal scaffolds to induce bone tissue growth and biomineralization, to induce osteogenic differentiation of rat mesenchymal stem cells (rMSCs), and can be used for the functionalization of bone implants.3,49–51 In some natural biomaterials, dityrosine residues are found, which form through covalent crosslinking of tyrosines. For example, tyrosine–tyrosine crosslink networks found in resilin provide outstanding mechanical properties such as elasticity,52–62 which were exploited for the formation of peptide hollow nanocapsules and peptide lamella films,52 as well as to engineer small tyrosine-containing peptide hydrogels with enhanced mechanical properties.63
In this study, we developed a computational design strategy to introduce mutations to a set of modeled self-assembling β-sheet fibril structures with the aim to further stabilize and potentially functionalize the amyloid fibrils. Using our recently computationally derived structures of RGDSGAITIGC β-sheet self-assembling scaffolds,47 we designed novel amyloid fibrils that bear cell adhesive motifs and are highly stabilized by aromatic interactions. Inspired by the proximity of tyrosine–tyrosine residues in the intermolecular aromatic network of our newly designed fibrils, we computationally investigated the capacity of the designed peptide RGDSGAITIGY to concurrently possess dityrosine crosslinking and cell adhesive properties. Subsequently, we experimentally investigated the self-assembling properties of the designer peptides. TEM, SEM, AFM and fiber diffraction experiments validated the ability of the peptides to form amyloid-type fibrils. These designer peptides may find promising biomaterial and tissue engineering applications in the future. Table 1 provides an overview of all the peptide sequences investigated in the present study.
Table 1 Overview of the peptide sequences investigated. All peptides have an amidated C-terminal end
Peptide |
Sequence |
1 |
RGDSGAITIGY |
2 |
RGDSGAITIGW |
3 |
RGDSGAITIGH |
4 |
RGDSGAITIGF |
Methods
Computational methods
Computational design – modification at position 11.
We introduced a computational design model to stabilize the self-assembling β-sheet peptides by minimizing the energy of a short modeled amyloid fibril upon single or multiple amino acid substitutions. The energy term used in the objective function under minimization accounts for protein–protein interactions (first term in eqn S1 in ESI† Methods), analogously to ref. 29 and 64–67, as well as protein–water interactions (second term of eqn S1 in ESI† Methods) which could also play a key role in stabilizing the structure of self-assembling peptide. As for the first term, the minimization of the total energy of a protein or a protein–protein complex has been implemented as a strategy in several protein design studies29,64–68 using as principle the decomposition of energy into protein–protein residue pairwise terms (e.g., ref. 64 and 69–71).
For the purpose of the specific study, we followed a minimalistic approach which aimed to preserve the initial amyloidogenic and cell adhesive regions of the sequence to the highest possible extent, and simultaneously further stabilize and potentially functionalize the fibril by mutating the last residue position 11 of amyloid forming peptide RGDSGAITIGC.47 Mutations at residue position 11 were introduced to two sets of 4-stranded highly ordered and well-aligned β-sheet fibrils of RGDSGAITIGC,47 referred to as designable scaffolds, which comprised of computationally derived elementary structural units of fibrils.47 Of the two sets, one set comprised of highly ordered and well-aligned 4-stranded parallel β-sheet fibrils and the other set comprised of highly ordered and well-aligned 4-stranded antiparallel β-sheet fibrils. Both antiparallel and parallel designable scaffolds were used to investigate in which configuration, parallel or antiparallel, a specific mutation at residue position 11 could potentially be most favorable to be introduced. The use of highly accurate modeled fibrils as designable scaffolds as well as the consideration of both antiparallel and parallel configurations proved significantly advantageous, as through this approach, we were able to directly investigate the energetic effect of a mutation on realistically modeled β-sheet configurations of the peptides (see ESI† Methods).
In the computational design model, we used a coarse-grained surface residue pairwise force field, SIPPER Surface72 to account for the protein–protein residue pairwise energy among all possible types of amino acids. The specific force field is based on residue composition of protein surfaces and was trained to consider residue pairs with separated partners having relative accessible surface area (ASA) ≥5% (with respect to that of the given residue type when it is exposed). We considered the specific force field more appropriate to estimate energetic effects of a mutated residue at the fibril's surface than other coarse-grained residue pairwise force fields trained to account for residue pairwise interactions in globular proteins in general (e.g., ref. 73–75). The computational design model (eqn S1†) was solved in FORTRAN using exhaustive enumeration. According to the results, apart from a cysteine placement at position 11 (investigated by us in ref. 47), aromatic residues (tyrosine, tryptophan and phenylalanine), methionine, histidine and arginine can additionally be considered energetically favorable substitutions at position 11 according to the energy term under minimization in eqn S1.† Motivated by our findings in conjunction with previously published studies suggesting the role of aromatic residues with π-stacking properties in peptide self-assembly,20,42–44 we focused our investigation on residue substitutions containing an aromatic (tyrosine, tryptophan and phenylalanine) or an imidazole ring (histidine) (see Table 1 and ESI† Methods). According to the coarse-grained computational design, the specific residue substitutions were among the most energetically favorable mutations in parallel β-sheet bonded peptides. Thus, a tyrosine mutation at position 11 may lead to potential functionalization of the self-assembled peptides via tyrosine crosslinking between adjacent β-sheet peptides bonded in parallel upon fibril formation.
Constraint introduced to investigate the potential crosslinking capacity between tyrosine residues upon fibril formation.
The aforementioned results suggest that the mutation of a tyrosine at position 11 stabilizes the self-assembling β-sheet peptides arranged in a parallel configuration. It is possible that owing to the proximity of aromatic/imidazole-ring containing residues in adjacent β-sheet bonded peptide strands, π–π interactions formed between residues at position 11 belonging to adjacent peptide strands might play a key role in the fibrils' stabilization. Inspired by the fact that tyrosine–tyrosine crosslink networks found in resilin have outstanding mechanical properties such as elasticity,52–62 we introduced an additional constraint (eqn S3†) during the solution of the computational design problem. This constraint examines the possibility of tyrosine residues in adjacent β-sheet forming peptides to be crosslinkable upon fibril formation, in the designed peptide RGDSGAITIGY. Through the constraint defined in eqn S3† we investigated whether the Cα:Cα distance, D(s)jliakb, between residues at position 11 of adjacent peptides in parallel β-sheet designable scaffolds can be less than 10.65 Å, the maximum Cα:Cα distance for covalently bonded dityrosine molecules deposited in the ZINC database.76 Specifically, for the dityrosine compounds of the ZINC database76 the Cα:Cα distance between the two covalently bonded tyrosines ranges from 7 Å to 10.65 Å (see ESI† Methods).
Infinite dilution simulations.
We first simulated an isolated peptide of each of the four designed peptides NH3+-RGDSGAITIG(Y/W/H/F)-CONH2 in aqueous solution. The aqueous solvent effects were modeled implicitly using the FACTS19 (ref. 77) solvation model in conjunction with the CHARMM19 all-atom force field.78 The value of surface tension coefficient in the solvation model is taken to be 0.015 kcal mol−1 Å−2, which is in line with the CHARMM19 force-field for reversible folding simulations of the structured peptides. We performed replica exchange molecular dynamics (REMD) simulations,79–84 employing a total of eight replicas with temperatures 283, 300, 318, 336, 356, 377, 403, and 432 K, and using Langevin dynamics with a friction coefficient of 5.0 ps−1 on heavy atoms and 0 ps−1 on hydrogen atoms. The total simulation time for all temperatures per designed peptide system was 1.6 μs. We analyzed 20
000 snapshots of the 300 K trajectory, extracted at 100 ps intervals. To extract the most representative conformations, we performed a root mean square deviation (RMSD)-based clustering analysis on the extracted snapshots. The clustering analysis was conducted via WORDOM85 employing all backbone atoms and a clustering radius of 2 Å. The six most representative conformations were extracted from the six most populated clusters for each of the four designed peptides and were used as initial structures for simulations in finite dilution described below (ESI,† Fig. S2).
Self-assembly simulations.
We investigated the self-assembly properties of the four computationally designed peptides, listed in Table 1, using REMD simulations analogous to the computational protocol presented in ref. 17. The protocol was used successfully in previous studies to elucidate the self-assembly properties of short peptides, as well as for the design of amyloid-forming peptides.17–19,21,47 Using the replica-exchange method,79–84 we performed MD simulations of the designed peptide systems. We placed six copies of each peptide sequence, with a positively charged N-terminal and an amidated C-terminal end, with different initial conformations in a cubic periodic 148 Å box, resulting in an approximate 3 mg ml−1 peptide concentration. The simulations were performed using CHARMM,86 analogously to ref. 17–21 and 47. Initially the six copies of each peptide sequence were placed at the center. After the initial placement, peptides 1, 3, and 5 were translated by +25 Å in the x, y, and z directions, respectively, and peptides 2, 4, and 6 were translated by −25 Å in the x, y, and z directions, respectively. The initially modeled structures showing six copies of the designed peptides prior to the execution of the REMD simulations is presented in ESI,† Fig. S2. We modeled water implicitly using the FACTS 19 (ref. 77) solvation model, as in ref. 17–19, 21, 47, and the surface tension coefficient was set to 0.015 kcal mol−1 Å−2). For the purpose of Langevin dynamics, a 5.0 ps−1 friction coefficient was introduced on all non-hydrogen atoms of each peptide. We employed a total of sixteen temperatures (290, 295, 300, 310, 305, 315, 321, 327, 333, 339, 345, 352, 359, 366, 373 and 380 K) with each replica exchange run's duration being equal to 10 ps. The total simulation time for all temperatures per system was equal to 16 μs. We collected the final conformations of each replica exchange run at 300 K for each peptide sequence. These conformations were combined into one trajectory per peptide sequence, corresponding to a length of 1000 ns and 100
000 snapshots per self-assembling peptide provided in Table 1. Implicit solvent representation was used to improve and accelerate the sampling of the β-sheet structures owing to the absence of the solvent friction when explicit water molecules are present. The FACTS implicit solvent model for the study of structures of amyloidogenic peptides in an infinite dilution regime can reproduce results obtained by explicit solvent simulations. The choice of the FACTS implicit solvent model provides a fast analytical continuum treatment of solvation, and a precision comparable to the most accurate GB implementations.77
Replica exchange simulations were used as a sampling method to enhance peptides sampling at the 300 K trajectory, and avoid the trapping of peptides in local energetic minima. Compared to serial MD simulations, replica exchange MD simulations have some competitive advantages which include the following (i) The exchange of replicas allows low-temperature replicas to escape local minima and to borrow the sampling efficiency of high-temperature replicas. (ii) The Metropolis criterion ensures that energetically inaccessible conformations (at a given temperature) are not allowed, and that all replicas gain access to conformations with the proper thermodynamic weight. (iii) The high-temperature runs permit the frequent dissolution and reformation of aggregates and the sampling of a wide variety of intermolecular structures.17 However, attention should be taken for several criteria to be satisfied during the execution of REMD simulations for enhanced sampling.17
Categorization of β-sheet conformations and key β-sheet interactions.
We used DSSP87 to determine the secondary structure of the designed peptides within the simulations, and identified the formation of intermolecular β-sheet structures. We categorized the β-sheets into antiparallel, parallel and mixed conformations comprising 2 to 5 peptide strands and the categorization was performed following the computation protocol by Tamamis et al., in ref. 17. The (%) moving averages of antiparallel, parallel and mixed β-sheet conformations, composed of 2-, 3-, 4-, and 5-stranded peptides depict that the designed peptides in the simulations have the tendency to self-assemble into β-sheet rich conformations. For all four computationally designed peptides, an overall tendency for the formation of parallel, rather than antiparallel β-sheets is observed. 4- and 5-stranded antiparallel and parallel β-sheets have higher complexity than 2- and 3-stranded β-sheets; hence, the structural patterns observed in the former can potentially correspond to patterns that may exist in naturally occurring fibrils formed by the peptides. Since parallel configuration is primarily the dominant configuration for the designed peptides, we extracted the 4- and 5-stranded parallel β-sheets from the corresponding MD simulation snapshots for each designed peptide sequence. We calculated the percent probability of an intermolecular pair of residues, belonging to two adjacent β-sheet peptides in the 4- and 5-stranded parallel β-sheet states of the four designed peptides, to be involved in a β-sheet (or β-bridge) conformation. The propensities of β-intermolecular interactions between adjacent residue pairs are presented in two-dimensional maps (see Results). Through this analysis we identified the predominant patterns of pairwise β-sheet interactions between intermolecular residues, and the key amyloidogenic regions of each peptide. In addition, this analysis can be used to indicate the residues that are unlikely to be involved in the formation of intermolecular β-sheets in each peptide sequence, and thus, can be mutated for functionalization purposes.
Identification of highly ordered and well-aligned β-sheet conformations using P1 and P2 parameters.
Using the polar order-parameter, P1, and the nematic order-parameter, P2, defined in eqn (1), we examined the extent of peptide alignment and order for the extracted 4- and 5-stranded parallel β-sheet conformations. These parameters are commonly used in the structural characterization of liquid crystals,88–94 and have been employed successfully in simulation studies of peptide aggregation.17–19,21,47,95 |  | (1) |
In eqn (1), N is the number of molecules in the simulation;
is a unit vector along a suitably defined molecular direction;
is a unit vector along a preferred direction of alignment that emerges from the properties of the system. For all four peptides, we selected
to be defined by the segment spanning from the Cα atom of residue 5 to the Cα atom of 10, as these residues primarily comprise the key β-sheet regions of each designed peptide (see Results).
By definition, P1 can distinguish between parallel, antiparallel and mixed orientations, while P2 can distinguish between ordered (P2 ≈ 1 for perfect alignment) and disordered conformations (
, for a system of N peptides96). Using WORDOM,85 we computed P1 and P2 parameters for the 4- and 5-stranded parallel β-sheets of the designed peptides (ESI,† Fig. S3). The results revealed that among the 4- and 5-stranded parallel β-sheets across all systems, β-sheets that are both highly populated and highly ordered occur only in 4-stranded parallel β-sheet conformations. The lower population and lower degree of order observed in 5-stranded parallel β-sheets can partly be attributed to the limited number of peptides used in the self-assembly simulations. Thus, we focused our further analysis on 4-stranded parallel β-sheets and examined the structural properties of highly ordered and well-aligned conformations formed by the designed peptides. We constructed free energy landscapes for the specific 4-stranded parallel β-sheets, using the two dimensional probability P(P1, P2) and eqn (2):
| G(P1, P2) = −kBT ln[P(P1, P2)] | (2) |
In each case we identified the free energy basin that encompasses the majority of conformations and the location of the global minimum. We extracted the states with the highest polar order and highest nematic order, corresponding to P1, P2 regions [P1 = 0.8
:
1.0, P2 = 0.75
:
1.0] for parallel β-sheet conformations of the designed peptides. The selected highly ordered and well-aligned 4-stranded parallel conformations were utilized for subsequent analysis focusing on the peptides' structural properties. Representative conformations of the selected highly ordered and well-aligned parallel β-sheet states are shown in the Results.
Amyloid zipper formation, salt bridge formation and exposure of functional motifs.
We investigated the propensity of intramolecular and intermolecular contacts formed between the residue side chains of peptides within the 4-stranded highly ordered and well-aligned parallel β-sheet states. We defined a distance threshold of 6.5 Å between geometric centers of two side chains as a criterion for a contact. Furthermore, we calculated the average propensity for Arg1 and Asp3 to be involved in the (i) intermolecular salt bridge, (ii) intramolecular salt bridge, and (iii) intra or intermolecular salt bridge for the selected 4-stranded highly ordered and well-aligned β-sheet states in each of the four designed peptides. A distance cutoff of 3.7 Å between the corresponding nitrogen:oxygen atoms of the oppositely charged groups was used as a criterion to denote a salt bridge formation between Arg1 and Asp3.
In addition, we investigated the degree of solvent accessibility of the RGD sequence motifs and residues at position 11 (Y/W/H/F) within the 4-stranded highly ordered and well-aligned parallel β-sheet states of the designed peptides. We calculated the solvent accessible surface areas for the backbone and side chain moieties belonging to the RGD sequence motif and the residue at position 11 (Y/W/H/F) of the two central peptides in each 4-stranded highly ordered and well-aligned parallel β-sheet state. A 1.4 Å radius sphere (approximating the radius of a water molecule) was introduced to “probe” the surface of the molecule for the solvent accessible and total accessible surface area calculations.97 The total accessible surface area of a specific moiety in a peptide strand was approximated as the maximum solvent accessible surface area (SASA) and was calculated by assuming that the specific moiety in that strand is not part of the peptide system. While this approximation is expected to overestimate the total solvent accessible surface of a specific moiety, we used this definition as we considered it an appropriate way to perform a fair comparison of the investigated moieties across the four designed peptides, as well as in the context of the entire fibronectin (see Results).47 Subsequently, we summed the total accessible surface area of a specific moiety in the two central peptides, and calculated the ratio of solvent accessible surface area/total accessible surface area for these specific moieties. The solvent accessible surface area and total accessible surface area values for the two outer peptides are not reported as the degree of solvent accessibility of the two outer peptides is artificially high due to the absence of interacting peptides on the ends.47 Nevertheless, the two outer peptides were appropriately considered for the purpose of calculating the solvent accessibility areas of specific regions belonging to the central peptides.47
RGD sequence motif is known for its capacity to promote cell adhesive properties in extracellular matrix (ECM) proteins such as fibronectin, vitronectin, and fibrinogen.98–101 The RGD sequences in the ECM proteins are solvent exposed, thus aiding the ECM proteins in fast recognition and binding to the cell adhesive receptors.102 To uncover the potential cell adhesive properties of the RGD motif in our designed peptides, we compared their solvent accessibility properties to the NMR-resolved functional cell adhesive RGD motif of the fibronectin (PDB code: 1TTF102).
Potential of crosslinking in the computationally designed self-assembling peptide RGDSGAITIGY.
We calculated the distance (D) and angle (A) between the tyrosine's aromatic rings of the adjacent interacting peptides in the highly ordered and well-aligned 4-stranded parallel β-sheet states of the computationally designed self-assembling peptide RGDSGAITIGY. To investigate the peptides' crosslinking potential upon fibril formation, we extracted the distance and angle between the tyrosine's aromatic rings in the covalently bonded dityrosine compounds from the ZINC database,76 and compared the values of these parameters with the ones calculated for the designed peptide RGDSGAITIGY.
We projected the 4-stranded highly ordered and well-aligned parallel β-sheet states of the computationally designed peptide RGDSGAITIGY on the free energy landscape using the aforementioned distances (D) and angles (A) as reaction coordinates. We divided the (D, A) subspace into grids of size (0.5 Å, 2.5°) and then we calculated the two-dimensional probability P(D, A). Subsequently, the free energy landscape was constructed through eqn (3).
| G(D, A) = −kBT ln[P(D, A)] | (3) |
We identified two free energy minima regions and compared the distances (D) and angles (A) between the tyrosine's aromatic rings of the adjacent interacting peptides in highly ordered and well-aligned 4-stranded parallel β-sheets of the peptide RGDSGAITIGY with the distances (D) and angles (A) extracted from the covalently bonded dityrosine compounds in ZINC database.76
Experimental materials and methods
Self-assembly, amyloid fibril formation
Materials.
The peptides were purchased from Genecust (Luxemburg) and possessed a degree of purity higher than 95%. These synthetic lyophilized peptide powders were dissolved in sterile water pH 7 or in 10 mM phosphate buffer pH 7.4 at concentrations of 3 mg ml−1.
Transmission electron microscopy.
Sample preparation included the deposition of an 8 microlitre droplet of the sample onto a formvar covered 300 mesh copper grid (used for TEM). After 2 minutes, the excess fluid was soaked by a filter paper, and an 8 microlitre droplet of a solution of 2% uranyl acetate was deposited onto the mesh. After that, the excess fluid was removed in the same way, and the grid placed in the grid holder. Experiments were performed on a JEOL JEM-2100 Microscope, at the Electron Microscopy Lab “Vassilis Galanopoulos”, at the Department of Biology, University of Crete. For observation, a voltage of 100–120 kV and Ultra High Vacuum were used.
Atomic force microscopy.
Samples of 8 microliter droplets of peptide solutions were left to dry onto glass discs (12 mm). A Digital Instruments Multimode Scanning Probe Microscope with a Nanoscope IIIA controller was used in Tapping Mode.
Field emission scanning electron microscopy (FESEM).
For field emission SEM (FESEM), samples were fixed in 2% glutaraldehyde, 2% paraformaldehyde (PFA) in 0.1 M sodium cacodylate buffer pH 7.4 (45 min at 4 °C). They were subsequently washed in the aforementioned buffer twice, post-fixed in 1% aqueous OsO4 for 30 min at 4 °C and rinsed as above. Afterwards, they were dehydrated through a graded ethanol series, 30–50–70–90–100% at 4 °C followed by dry alcohol at room temperature. Dehydrated samples were critical point dried (Baltec CPD 030) and mounted on stubs prior to sputter coating with 10–15 nm thick gold (Baltec SCD 050). Observations were carried out using a FESEM microscope – JEOL 7000 at 10 kV operating voltage.
X-ray fiber diffraction.
A peptide droplet of 3 mg ml−1 to 10 mg ml−1 concentration and of 4.5–8.5 microliters volume was left to dry while anchored between two aligned glass rods 1–2 mm apart. X-ray fiber diffraction experiments were carried out at the European Synchrotron Radiation Facility (ESRF in Grenoble) on MASSIF-1/ID30a103 with a wavelength of 0.966 Å and a beam size of 15 μm for RGDSGAITIGY, RGDSGAITIGW, RGDSGAITIGH (ESI,† Fig. S4A, B and D), a beam size of 30 μm was used for RDGSGAITIGF. Exposure times were of the order of 1 seconds for RGDSGAITIGY, 3 seconds for RGDSGAITIGW and RGDSGAITIGH, and 0.5 seconds for RGDSGAITIGF. The sample-detector distance was set to 371.9 mm for Fig. S4C,† giving a resolution of 3 Å at the edge of the detector. For Fig. S4A, B and D,† the resolution is 3.5 Å at the edge with a sample-detector distance of 435.14 mm.
Results and discussion
Computational design – modification at position 11
We started from our computationally derived highly ordered and well-aligned antiparallel as well as parallel β-sheets formed by peptide RGDSGAITIGC.47 We initially aimed to further stabilize the fibrils by not interfering with either the residues in the sequence motif RGD that encompass cell adhesive properties or with the residues of the amyloidogenic core (S)GAITIG of the peptide RGDSGAITIGC. Thus, we selected position 11 as a mutable position, and identified optimum mutations which could potentially lead to amyloid fibrils with improved properties (e.g., stability, rigidity, functionality). Apart from the placement of cysteine, additional energetically favored mutations were identified which primarily involved the presence of aromatic or imidazole ring side chain groups at residue position 11. As mentioned in the Methods, motivated by our findings in conjunction with previously published studies suggesting the role of aromatic residues with π-stacking properties in peptide self-assembly,20,42–44 we focused our investigation on residue substitutions containing an aromatic (tyrosine, tryptophan and phenylalanine) or an imidazole ring (histidine). The introduction of aromatic (Tyr, Trp, Phe) or imidazole ring (His) groups at residue 11 were among the most energetically favorable mutations in a parallel arrangement of peptides, while the introduction of cysteine seems to favor an antiparallel arrangement, in line with our previous findings.47 It is possible that the interactions between the non-covalently bonded cysteine residues in the parallel β-sheet arrangement of RGDSGAITIGC may not suffice to sufficiently counterbalance energetically the unfavorable presence of two-positively charged groups at the first N-terminal position (charged N-terminal group and charged arginine residue).47
Constraint introduced to investigate the potential crosslinking capacity between tyrosine residues upon fibril formation
Upon the solution of the model, we introduced an additional constraint (eqn S3†) during the solution of the computational design problem to investigate the feasibility of crosslinking between adjacent tyrosine residues upon fibril formation, in the parallel β-sheet designable scaffolds of peptide RGDSGAITIGY. According to the ZINC database,76 the Cα:Cα distance between covalently bonded tyrosines in the dityrosine compounds is less than 10.65 Å, and thus, the specific distance was considered in the constraint. In 42 out of 50 parallel β-sheet designable scaffolds we used in the computational design, the Cα:Cα distance between residues at position 11 of adjacent β-sheet forming peptides is less than 10.65 Å. Thus, according to the specific criterion investigated by the constraint, tyrosine residues in the RGDSGAITIGY fibrils can potentially be crosslinkable upon fibril formation.
Computational categorization of β-sheet conformations and key β-sheet interactions
Following the computational design results, we investigated the self-assembly properties of the four designed peptides in Table 1 using REMD simulations. Within the simulations, all peptides were frequently arranged into β-sheet conformations (Fig. 1), which include 2-, 3-, 4-, and 5-stranded β-sheet states. 2-stranded β-sheets include parallel/antiparallel β-sheets (Fig. 1A–D); 3-, 4-, and 5-stranded include parallel/antiparallel and mixed β-sheets (Fig. 1E to P). Mixed conformations are composed by at least one pair of parallel and one pair of antiparallel β-sheets and, due to their high entropic favorability, have large populations. Non-symmetric β-sheets (containing strands in different orientations, such as mixed arrangements rather than entirely antiparallel or parallel) are entropically favored because of the larger number of possible configurations that the peptides can be arranged into.17 These conformations are not investigated in this study as they are not expected to be formed naturally due to their asymmetry. Configurations in which one peptide is forming β-sheet interactions with more than two peptides at a specific instance were considered “complex” and were not investigated in detail either as they are not expected to be present in the naturally occurring amyloid fibrils. The analysis of the REMD simulations indicates that the parallel configuration is overall more favorable than the antiparallel one for all the computationally designed peptides (Fig. 1). While all peptides have a predominantly higher tendency to form parallel β-sheets, it is worth noting that in specific cases, e.g., mainly involving 2-stranded or 5-stranded β-sheets, the population of antiparallel β-sheets can be larger. Yet, this can be attributed to the fact that 2-stranded β-sheets are of low complexity as only two peptides are involved in the β-sheet, while the population of 5-stranded β-sheets is low and the statistics is poorer. The overall higher tendency of parallel β-sheet formation suggests that the favorable intermolecular π-stacking interactions which can be formed by histidine, tyrosine, tryptophan or phenylalanine residues in the parallel-fashion (see below), can counterbalance the unfavorable presence of two-positively charged groups at the first N-terminal position owing to a positively charged N-terminal and the positively charged group of arginine.
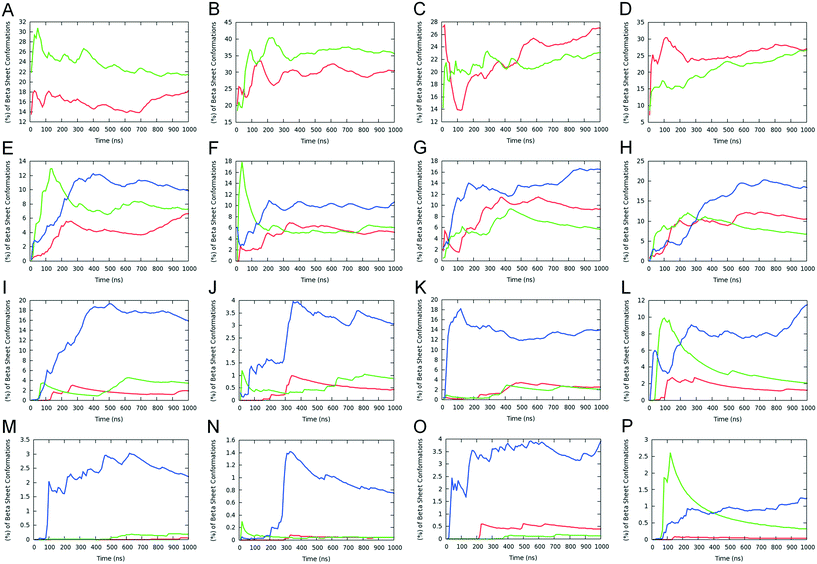 |
| Fig. 1 Moving averages of the fraction (%) of conformations with intermolecular β-sheets (Y-axis) in the REMD simulations at 300 K with respect to time (X-axis). Figures A, E, I, and M correspond to 2-, 3-, 4-, and 5-stranded β-sheets of peptide RGDSGAITIGY, respectively. Figures B, F, J, and N correspond to 2-, 3-, 4-, and 5-stranded β-sheets of peptide RGDSGAITIGW, respectively. Figures C, G, K, and O correspond to 2-, 3-, 4-, and 5-stranded β-sheets of peptide RGDSGAITIGH, respectively. Figures D, H, L, and P correspond to 2-, 3-, 4-, and 5-stranded β-sheets of peptide RGDSGAITIGF, respectively. Red color corresponds to antiparallel β-sheets, green color corresponds to parallel β-sheets, and blue color corresponds to mixed β-sheets. | |
From the four computationally investigated peptides listed in Table 1, we focused our analysis on 4-, 5-stranded parallel β-sheet conformations as they may represent to a higher extent the naturally occurring fibrils compared to lower complexity states, e.g., 2- and 3-stranded β-sheets. The propensities of two residues which belong to adjacent β-sheet interacting peptides to form intermolecular “β-bridge” interactions were calculated from the simulations at 300 K, and the results are presented in Fig. 2. In all four designed peptides, the residues involved in the β-sheet interactions are primarily the residues in the GAITIG motif (see Fig. 2A to H). Intermolecular parallel β-sheets formed by the peptides RGDSGAITIGY and RGDSGAITIGH are primarily in-register 5–10
:
5–10 patterns. Both peptides in some cases also form off-register (e.g., 5–9
:
6–10) patterns. (Fig. 2A and E for the peptide RGDSGAITIGY and Fig. 2C and G for the peptide RGDSGAITIGH). Intermolecular parallel β-sheets formed by the peptides RGDSGAITIGW and RGDSGAITIGF are primarily in-register 6–10
:
6–10 patterns. (Fig. 2B and F for the peptide RGDSGAITIGW and Fig. 2D and H for the peptide RGDSGAITIGF). In all four of the designed peptides, both the RGD motif and the residue at position 11 (Tyr11/Trp11/His11/Phe11) are not involved in the β-sheet interactions, indicating that the RGD and Y/W/F/H motifs can be exposed at the surface of the fibril and possess functional properties, including cell adhesion and crosslinking as is the case for RGD and tyrosine, respectively.
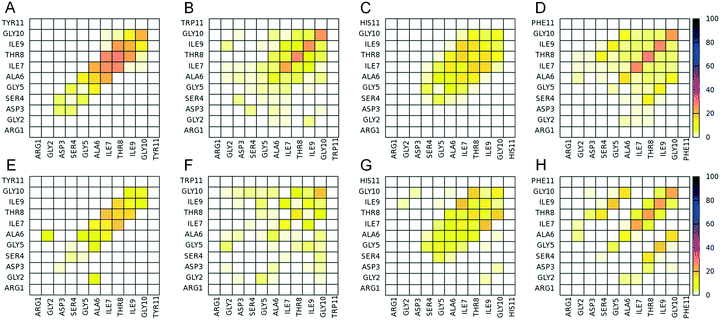 |
| Fig. 2 Density (%) maps of residue pairs forming intermolecular β-bridges. The pairs of residues belong to adjacent peptides participating in an isolated β-bridge or extended β-sheet conformation in the REMD simulations at 300 K. Figures A and E correspond to 4- and 5-stranded parallel configurations of peptide RGDSGAITIGY, respectively. Figures B and F correspond to 4- and 5-stranded parallel configurations of peptide RGDSGAITIGW, respectively. Figures C and G correspond to 4- and 5-stranded parallel configurations of peptide RGDSGAITIGH, respectively. Figures D and H correspond to 4- and 5-stranded parallel configurations of peptide RGDSGAITIGF, respectively. | |
Experimental amyloid fibril formation
The lyophilized powders were dissolved in ultrapure water and followed by TEM and AFM over time in order to determine whether the designed peptides could self-assemble into amyloid fibrils. Moreover, samples were observed with FESEM following critical point drying. Fig. 3 shows TEM micrographs of the four designed peptides after negative staining. For all peptides, straight, non-branched fibrils with diameters ranging from 10 to 50 nm and reaching several micrometers in length are observed. Similar patterns are observed with AFM (Fig. 4). A porous interconnected network of fibrils is observed by FESEM for all four peptides following critical point drying. (Fig. 5). The peptide samples were also visually inspected for an increase in viscosity or gel formation. Once a change in viscosity or a gel was observed, aligned fibrils were prepared between two glass rods and examined by X-ray fiber diffraction as described in Methods. The amyloid fibrils formed from the peptides show characteristic cross-β X-ray diffraction patterns with a 4.6–4.7 Å reflection corresponding to the hydrogen bonding distance between β-strands and a ∼8–10 Å reflection corresponding to the intersheet packing distance (ESI,† Fig. S4). For the RGDSGAITIGH peptide, the ∼8–10 Å reflection could not be recorded. This is the diffraction pattern (ESI,† Fig. S4) characteristic of the amyloid-type nature of these peptides. Overall, the TEM, SEM and AFM results and the X-ray fiber diffraction data confirm that the four designed peptides self-assemble into β-amyloid fibrils, validating that the in silico introduced mutations at position 11, indeed can be accommodated in the amyloid-like β-sheet self-assembling peptide RGDSGAITIGC.
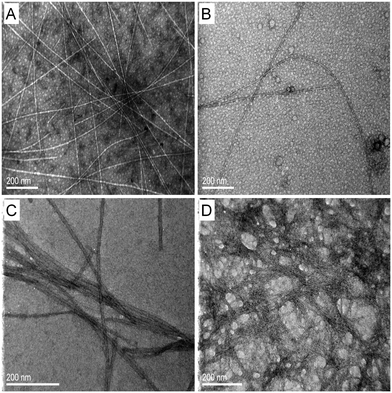 |
| Fig. 3 TEM images of peptide solutions of 3 mg ml−1 concentration. (A) RGDSGAITIGY after 24 hours in water. (B) RGDSGAITIGW after 24 hours in water. (C) RGDSGAITIGH after 24 hours in phosphate buffer (10 mM-pH 7.4). (D) RGDSGAITIGF after 24 hours in water. | |
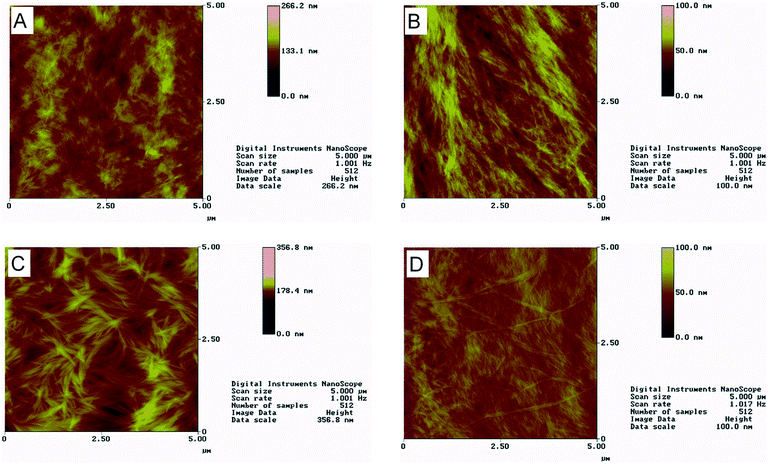 |
| Fig. 4 AFM images of peptide solutions of 3 mg ml−1 concentration. (A) RGDSGAITIGY after 24 hours in water. (B) RGDSGAITIGW after 24 hours in water. (C) RGDSGAITIGH after 24 hours in phosphate buffer (10 mM-pH 7.4). (D) RGDSGAITIGF after 24 hours in water. | |
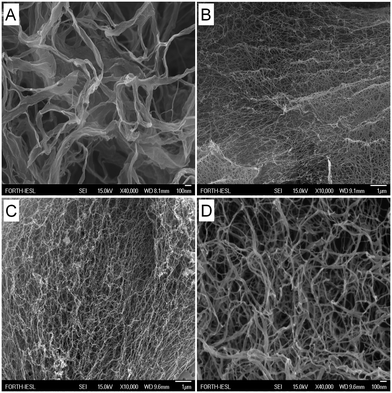 |
| Fig. 5 FESEM images of peptide solutions of 3 mg ml−1 concentration. (A) RGDSGAITIGY after 24 hours in water. (B) RGDSGAITIGW after 24 hours in water. (C) RGDSGAITIGH after 24 hours in phosphate buffer. (10 mM-pH 7.4) (D) RGDSGAITIGF after 24 hours in water. | |
Computational identification of highly ordered and well-aligned β-sheet conformations using P1 and P2 parameters
We computationally evaluated the degree of alignment and order by plotting polar order parameter P1 against nematic order parameter P2 for the 4- and 5-stranded parallel β-sheets formed by the four designed peptides listed in Table 1 (ESI,† Fig. S3). For all the peptides that were investigated computationally, highly ordered and well-aligned states predominantly occur in 4-stranded rather than 5-stranded parallel β-sheet conformations (ESI,† Fig. S3A–H). This can possibly be attributed to larger population of 4-stranded compared to 5-stranded parallel β-sheet conformations. We constructed two dimensional free energy landscapes of 4-stranded parallel β-sheets formed by the designed peptides using the calculated P1 and P2 parameters as reaction coordinates (Fig. 6A–D), to investigate the degree of order and identify β-sheet conformations containing highly ordered and well-aligned strands that most likely correspond to naturally occurring amyloid fibrils. The global free energy minima in the plots are located in basins, marked with black dashed lines. From these basins we extracted the highly ordered and well-aligned β-sheet states for all subsequent computational analysis, as these conformations likely correspond to elementary β-sheet structural units of the naturally occurring fibrils. Representative conformations of the selected highly ordered and well-aligned β-sheet states are shown in the bottom panel of Fig. 6 displaying the exposed residues and the amyloid zipper region of each peptide.
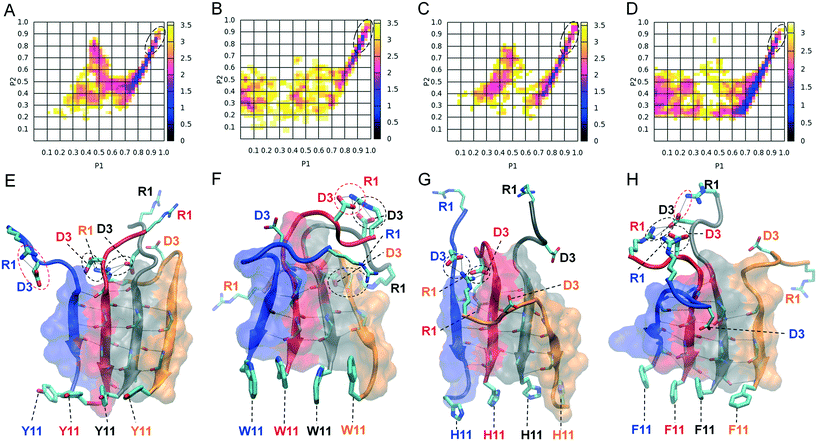 |
| Fig. 6 Upper panel: Free energy landscapes constructed from the 2-D probabilities of order parameters P1 and P2, calculated using the 4-stranded parallel β-sheets observed in the REMD simulations at 300 K. The surface in panels A, B, C and D correspond to 4-stranded parallel β-sheets formed by RGDSGAITIGY, RGDSGAITIGW, RGDSGAITIGH and RGDSGAITIGF, respectively. The global free energy minima in the plots are located in basins which are marked using black dashed lines, and within these we observe the presence of highly ordered and well-aligned β-sheet conformations. Bottom panel: Molecular graphic images of representative highly ordered and well-aligned conformations of peptides RDSGAITIGY (E), RGDSGAITIGW (F), RGDSGAITIGH (G) and RGDSGAITIGF (H) in parallel arrangement. The peptides' backbone is shown in new cartoon representation and the backbone atoms forming β-bridges are shown in thin licorice representation and are colored by name, and the β-bridge associated hydrogen bonds are shown using black dashed lines. Individual peptides are colored in blue, red, gray and orange from left to right for each 4-stranded β-sheet. Residue moieties 5–10 in each of the four designed peptides form amyloid-zipper like patterns and are shown in transparent surface representation. Side chain atoms of arginines and aspartic acids forming intra- or intermolecular salt bridges and residues at position 11 are shown in thick licorice representation, are colored by name and the interactions between them are marked using black dashed lines. Intramolecular salt bridges are marked using red dashed circles and intermolecular salt bridges are marked using black dashed circles. Side chain atoms of arginines and aspartic acids not participating in intra- or intermolecular salt bridges are shown in thin licorice representation and are colored by name. Each of the arginine, aspartic acid or aromatic residues at position 11 are labeled in color in correspondence to the color of the peptide they belong to. | |
Computational analysis of amyloid zipper formation, salt bridge formation and exposure of functional motifs
We performed a statistical analysis of the intra- and intermolecular side chain contacts within the highly ordered and well-aligned states of 4-stranded parallel β-sheets formed by the four computationally designed peptides (Fig. 7). The intermolecular contacts involve side chain interactions between adjacent β-sheet interacting peptides only, while intramolecular contacts involve side chain interactions between residues within the same peptide. In general (i) the side chains of aromatic residues at position 11 interact significantly with each other (Fig. 7A–D), indicating the presence of aromatic π–π interactions (aromatic residues are participating in such π-stacking in the bottom panels of Fig. 6), and (ii) the side chains within the residue moiety GAITIG stabilize the β-sheet formation and tend to cluster across the β-sheet region forming an “amyloid zipper” (shown in surface representation in Fig. 6E–H). According to the contact maps, Arg1 and Asp3 of the RGD motif have the tendency to form both intra- and intermolecular contacts (Fig. 7), as a result of intra- and intermolecular salt bridges formed by the two residues. The corresponding average propensities of salt bridge formation are similar for the four designed peptides. The average propensity for Arg1 and Asp3 to be involved in an (i) intermolecular salt bridge, (ii) intramolecular salt bridge, and (iii) intra or intermolecular salt bridge is 47%, 19%, 57% in the RGDSGAITIGY 4-stranded highly ordered and well-aligned parallel β-sheet states; 32%, 33%, 54% in the RGDSGAITIGW 4-stranded highly ordered and well-aligned parallel β-sheet states; 40%, 17%, 52% in the RGDSGAITIGH 4-stranded highly ordered and well-aligned parallel β-sheet states; 29%, 35%, 60% in the RGDSGAITIGF 4-stranded highly ordered and well-aligned parallel β-sheet states. Additionally, the probability of an arginine residue to form either an intra- or intermolecular salt bridge never exceeds 60%, indicating that arginine residues are sufficiently exposed. Fig. 6E–H show representative intramolecular salt bridges (shown in red dashed circles) and intermolecular salt bridges (shown in black dashed circles) formed by the four designed peptides. A detailed investigation of highly ordered and well-aligned β-sheet states formed by the four designed peptides within the REMD simulations, revealed the importance of intermolecular π–π interactions formed by the mutated residues at position 11 in the stabilization of the amyloid fibrils. This result complies with findings of previous studies outlining the key role of π-stacking in peptide and protein self-assembly.20,42–44
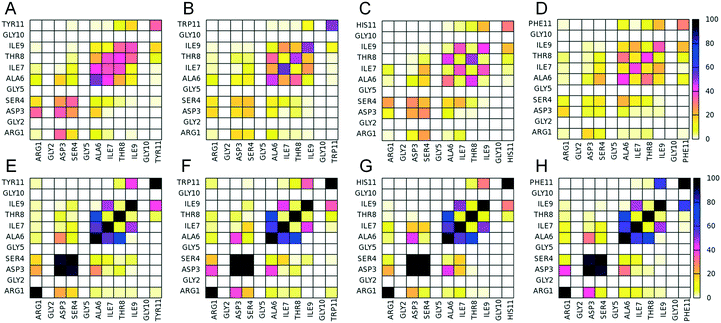 |
| Fig. 7 Percentage (%) probability density maps of side chain contacts of the highly ordered and well-aligned 4-stranded parallel β-sheets observed at 300 K. Maps A, B, C and D correspond to intermolecular side chain residue–residue contacts between adjacent interacting peptides. Maps E, F, G and H correspond to intramolecular side chain residue–residue contacts. A contact is present when the two geometric centers of the two side chain are within 6.5 Å. Figures A and E correspond to 4-stranded parallel configurations of peptide RGDSGAITIGY, respectively. Figures B and F correspond to 4-stranded parallel configurations of peptide RGDSGAITIGW, respectively. Figures C and G correspond to 4-stranded parallel configurations of peptide RGDSGAITIGH, respectively. Figures D and H correspond to 4-stranded parallel configurations of peptide RGDSGAITIGF, respectively. | |
We examined the degree of exposure of the entire functional motif RGD as well as the side chain of the designed residue at position 11 belonging to the two central peptides in the highly ordered and well-aligned states of 4-stranded parallel β-sheets formed by the four computationally designed peptides. The extent to which the RGD motif is solvent accessible in the four designed peptides is similar, and is equal to 58 ± 8%, 63 ± 7%, 66 ± 9%, 66 ± 7%, respectively for peptides RGDSGAITIG(Y/W/H/F)-CONH2. The degree of solvent accessibility of the RGD motif in the ensemble of NMR structures of the fibronectin protein102 is equal to 70 ± 5%, and in the selected 4-stranded highly ordered and well-aligned antiparallel β-sheet states of the self-assembling peptide RGDSGAITIGC,47 which was experimentally verified for its cell adhesive properties, is equal to 66 ± 8%. Thus, a comparison between the degree of solvent accessibility of the RGD motif in our designed peptides and fibronectin as well as the cell adhesive fibrils formed by RGDSGAITIGC suggests that the RGD motif in the designed peptides of the present study is adequately solvent exposed to potentially possess cell adhesion properties. Despite the fact that the ratio is slightly smaller in the RGDSGAITIGY (58 ± 8%) peptide compared to fibronectin or the amyloid fibrils formed by RGDSGAITIGC,47 the solvent accessibility of the RGD motif in 39% of the 4-stranded highly ordered and well-aligned parallel β-sheet formed by RGDSGAITIGY is within the range of solvent accessibility of the corresponding motif in the ensemble of NMR structures of fibronectin. Also, the solvent accessibility of the RGD motif in 90% of the 4-stranded highly ordered and well-aligned parallel β-sheets formed by RGDSGAITIGY is within the range of solvent accessibility of the corresponding motif in the 4-stranded highly ordered and well-aligned antiparallel β-sheet conformations formed by RGDSGAITIGC.47 Thus, the aforementioned results suggest that in the highly ordered and well-aligned parallel β-sheet states of the four designed peptides, the RGD motif is not part of the β-sheet core, it is exposed and it can possess functional cell adhesive properties within the fibrils formed.
The side chain moieties of residues Tyr11/Trp11/His11/Phe11 in the designed peptides are also adequately solvent exposed, and the ratios of solvent over total accessible surface areas ranges from 0.37 ± 0.09 (RGDSGAITIGH) to 0.46 ± 0.08 (RGDSGAITIGY). The significant degree of solvent accessibility of the tyrosine residues in the self-assembled structures is in agreement with the fact that the tyrosine residues are not part for the amyloid-like zipper formed. This further suggests that the tyrosine residues of adjacent β-sheet bonded peptides can be crosslinkable in the amyloids formed by the peptide with sequence RGDSGAITIGY, which is analyzed in detail below.
Potential of crosslinking in the computationally designed self-assembling peptide RGDSGAITIGY
The aromatic residues at position 11 in each of the four designed peptides are not part of the amyloidogenic core and are accessible to the solvent, indicating that tyrosine residues within the designed peptide with sequence RGDSGAITIGY can presumably be crosslinked upon fibril formation. As tyrosine–tyrosine crosslink networks have outstanding mechanical properties52–63 and a possible crosslinking between tyrosine residues within the RGDSGAITIGY fibrils can be of utmost importance for the designed fibril's stability, we focused our investigation on the designed peptide RGDSGAITIGY and examined its potential crosslinking properties. We calculated the distance (D) and angle (A) between the aromatic ring centers of tyrosines that belong to adjacent peptides of the 4-stranded highly ordered and well-aligned β-sheet states of RGDSGAITIGY. Using distance (D) and angle (A) as reaction coordinates (Fig. 8) we constructed a free energy landscape to investigate the correlation between the distance and angle between tyrosine aromatic ring centers in the π–π interactions (e.g., when D is below approximately 7.5 Å). In the landscape, two free energy minima basins are observed in which the distance between the tyrosine aromatic ring centers is primarily within the range of 4–6 Å, and the angle between the tyrosine aromatic ring centers is primarily within the range of 60–80° and 10–30° in the first and second free energy minima, respectively. Notably, the values of the distance and angle between tyrosine aromatic ring centers in the first minimum are close to corresponding distance and angle of the covalently bonded dityrosine compounds found in the ZINC database76 (marked using a black “X” in Fig. 8); the smaller value of the distance between the aromatic ring centers in the covalently bonded tyrosine compounds can be attributed to the fact that the two rings are covalently bonded and thus can be in closer proximity. Nevertheless, the relatively close proximity between the tyrosine aromatic ring centers in the modeled amyloid fibrils, in combination with the appropriate angle between the aromatic ring centers provide an additional indication that tyrosine residues can potentially be crosslinked upon fibril formation.
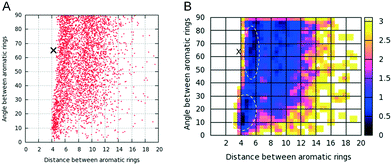 |
| Fig. 8 Angle between aromatic rings (Y-axis) as a function of distance between aromatic rings (X-axis). The angle and distance between the aromatic rings in the covalently bonded dityrosine compounds taken from the ZINC database is marked using a black “X”. Panel A: The angle and the distance between the aromatic rings of tyrosine residues in adjacent interacting peptides of 4-stranded highly ordered and well-aligned parallel β-sheet states observed in the REMD simulations at 300 K of the peptide RGDSGAITIGY are shown in red dots. Panel B: Free energy landscape constructed from the 2-D probabilities, using as reaction coordinates, the distance and angle between the aromatic rings of tyrosine residues in adjacent interacting peptides of 4-stranded highly-ordered and well-aligned parallel β-sheet states observed in the REMD simulations at 300 K of the peptide RGDSGAITIGY. | |
Conclusions
In the present work, we introduced a novel computational design strategy to energetically stabilize and potentially functionalize an amyloid peptide fibril. In summary, the following five features were innovatively combined and led to the successful design of a novel amyloid biomaterial with potential cell adhesive and crosslinking properties: (i) the minimized energy function in the computational design model, considered both protein–protein and protein-water interactions; (ii) the designable scaffolds (flexible templates) corresponded to an ensemble of computationally modeled highly ordered and well-aligned β-sheet states and not random peptide aggregates; (iii) the designable scaffolds comprised of both antiparallel and parallel modeled β-sheets independently, and thus, both possible configurations parallel versus antiparallel were energetically examined upon an amino acid substitution; (iv) the additional constraints introduced to verify the functionality of the designed peptide sequences for potential crosslinking applications; (v) the use of REMD simulations and free energy calculations to extract highly-accurate structures as modeled fibrils investigating the computationally designed peptides, and showing that they (a) self-assemble into highly ordered and well-aligned parallel β-sheet states, and (b) could possess potential bifunctional cell adhesion and crosslinking properties. Notably, according to points (ii) and (iii), during the design mutations are introduced on realistically modeled β-sheet arrangements of peptides, parallel or antiparallel, which can constitute elementary structural units of the fibrils. Our study suggests that analogous computational design strategies can be introduced for the design of novel functional biomaterials with diverse applications. Considering the predominant parallel arrangement of the four designed peptides studied here, as well as the predominant antiparallel arrangement of the RGDSGAITIGC peptide studied in ref. 47, it is possible that the computational design strategy introduced here may provide insights on the arrangement of the peptides as well; yet, this needs further investigation in future studies.
According to the computational design and analysis, a cysteine to tyrosine substitution from RGDSGAITIGC to RGDSGAITIGY “switches” the preferred β-sheet configuration from antiparallel47 to parallel, and the distance between adjacent tyrosine residues in the parallel β-sheet designable scaffolds is appropriate for crosslinking upon fibril formation. In addition, according to the highly ordered and well-aligned parallel β-sheet states formed by RGDSGAITIGY peptides within the simulations, the geometric properties of tyrosine residues belonging to adjacent β-sheet forming peptides are expected to be appropriate to accommodate crosslinking properties upon fibril formation. Preliminary experiments suggest the crosslinking ability of the specific peptide fibril scaffold, which in combination with the findings of the current study, indicates that the amyloid fibrils formed by the RGDSGAITIGY peptide may pave the way for future promising biomaterial and tissue engineering applications.
Acknowledgements
All MD simulations were performed on the Ada supercomputing cluster at the Texas A&M High Performance Research Computing Facility. The work was supported by startup funding from the Artie McFerrin Department of Chemical Engineering of Texas A&M awarded to P. T. A. A. O. acknowledges the TAMU Office of Graduate and Professional Studies for financial support through the Texas A&M University Graduate Diversity Fellowship. E. O. and A. M. acknowledge support from “PHOTOPEPMAT” Design, production and Laser PHOTO structuring of self-assembling PEPtides and proteins destined for bioMATerials applications Greek Secretariat for Research and Technology, ARISTEIA II Excellence grant No 3941 which is part of the Action “Education and Lifelong Learning” and co-financed by the European Social Fund and National Funds. P. T. thanks Juan Fernandez-Recio for helpful discussions with regards to the SIPPER force field. We are grateful to Ms. Aleka Manoussaki for technical assistance with FESEM experiments, and to Ms. Katerina Tsagkaraki for AFM measurements.
References
- G. M. Whitesides and M. Boncheva, Proc. Natl. Acad. Sci. U. S. A., 2002, 99, 4769–4774 CrossRef CAS PubMed.
- D. N. Woolfson and Z. N. Mahmoud, Chem. Soc. Rev., 2010, 39, 3464–3479 RSC.
- Y. Loo, M. Goktas, A. B. Tekinay, M. O. Guler, C. A. Hauser and A. Mitraki, Adv. Healthcare Mater., 2015, 4, 2557–2586 CrossRef CAS PubMed.
- K. Terzaki, E. Kalloudi, E. Mossou, E. P. Mitchell, V. T. Forsyth, E. Rosseeva, P. Simon, M. Vamvakaki, M. Chatzinikolaidou, A. Mitraki and M. Farsari, Biofabrication, 2013, 5, 045002 CrossRef PubMed.
- M. Reches and E. Gazit, Science, 2003, 300, 625–627 CrossRef CAS PubMed.
- L. Adler-Abramovich and E. Gazit, J. Pept. Sci., 2008, 14, 217–223 CrossRef CAS PubMed.
- E. Kasotakis, E. Mossou, L. Adler-Abramovich, E. P. Mitchell, V. T. Forsyth, E. Gazit and A. Mitraki, Biopolymers, 2009, 92, 164–172 CrossRef CAS PubMed.
- E. Kasotakis and A. Mitraki, Methods Mol. Biol., 2013, 996, 195–202 CAS.
- E. Kasotakis and A. Mitraki, Biopolymers, 2012, 98, 501–509 CrossRef CAS PubMed.
-
A. Mitraki and E. Kasotakis, in Self-Assembled Peptide Nanostructures, ed. J. Castillo, L. Sasso and W. E. Svendsen, Pan Stanford Publishing, 2012, ch. 2, pp. 39–66 Search PubMed.
- B. Viguier, K. Zór, E. Kasotakis, A. Mitraki, C. H. Clausen, W. E. Svendsen and J. Castillo-León, ACS Appl. Mater. Interfaces, 2011, 3, 1594–1600 CAS.
- E. Kasotakis, A. Kostopoulou, M. Spuch-Calvar, M. Androulidaki, N. Pelekanos, A. G. Kanaras, A. Lappas and A. Mitraki, Appl. Phys. A, 2014, 116, 977–985 CrossRef CAS.
- D. Li, H. Furukawa, H. Deng, C. Liu, O. M. Yaghi and D. S. Eisenberg, Proc. Natl. Acad. Sci. U. S. A., 2014, 111, 191–196 CrossRef CAS PubMed.
- D. Li, E. M. Jones, M. R. Sawaya, H. Furukawa, F. Luo, M. Ivanova, S. A. Sievers, W. Wang, O. M. Yaghi, C. Liu and D. S. Eisenberg, J. Am. Chem. Soc., 2014, 136, 18044–18051 CrossRef CAS PubMed.
- S. Bolisetty and R. Mezzenga, Nat. Nanotechnol., 2016, 11, 365–371 CrossRef CAS PubMed.
- G. Charalambidis, E. Kasotakis, T. Lazarides, A. Mitraki and A. G. Coutsolelos, Chemistry, 2011, 17, 7213–7219 CrossRef CAS PubMed.
- P. Tamamis, E. Kasotakis, G. Archontis and A. Mitraki, Methods Mol. Biol., 2014, 1216, 53–70 CAS.
- P. Tamamis, E. Kasotakis, A. Mitraki and G. Archontis, J. Phys. Chem. B, 2009, 113, 15639–15647 CrossRef CAS PubMed.
- P. Tamamis, K. Terzaki, M. Kassinopoulos, L. Mastrogiannis, E. Mossou, V. T. Forsyth, E. P. Mitchell, A. Mitraki and G. Archontis, J. Phys. Chem. B, 2014, 118, 1765–1774 CrossRef CAS PubMed.
- P. Tamamis, L. Adler-Abramovich, M. Reches, K. Marshall, P. Sikorski, L. Serpell, E. Gazit and G. Archontis, Biophys. J., 2009, 96, 5020–5029 CrossRef CAS PubMed.
- P. Tamamis and G. Archontis, J. Non-Cryst. Solids, 2011, 357, 717–722 CrossRef CAS.
- L. Serrano, Adv. Protein Chem., 2000, 53, 49–85 CrossRef CAS PubMed.
- E. Lacroix, T. Kortemme, M. Lopez de la Paz and L. Serrano, Curr. Opin. Struct. Biol., 1999, 9, 487–493 CrossRef CAS PubMed.
- E. Paci, J. Gsponer, X. Salvatella and M. Vendruscolo, J. Mol. Biol., 2004, 340, 555–569 CrossRef CAS PubMed.
- Y. Miller, B. Ma and R. Nussinov, J. Phys. Chem. B, 2015, 119, 482–490 CrossRef CAS PubMed.
- W. Song, G. Wei, N. Mousseau and P. Derreumaux, J. Phys. Chem. B, 2008, 112, 4410–4418 CrossRef CAS PubMed.
- R. Pellarin, E. Guarnera and A. Caflisch, J. Mol. Biol., 2007, 374, 917–924 CrossRef CAS PubMed.
- B. A. Thurston, J. D. Tovar and A. L. Fergusone, Mol. Simul., 2016, 42, 955–975 CrossRef CAS.
- J. Smadbeck, K. H. Chan, G. A. Khoury, B. Xue, R. C. Robinson, C. A. Hauser and C. A. Floudas, PLoS Comput. Biol., 2014, 10, e1003718 Search PubMed.
- N. P. King, W. Sheffler, M. R. Sawaya, B. S. Vollmar, J. P. Sumida, I. André, T. Gonen, T. O. Yeates and D. Baker, Science, 2012, 336, 1171–1174 CrossRef CAS PubMed.
- S. Kaltofen, C. Li, P. S. Huang, L. C. Serpell, A. Barth and I. André, J. Mol. Biol., 2015, 427, 550–562 CrossRef CAS PubMed.
- C. H. Norn and I. André, Curr. Opin. Struct. Biol., 2016, 39, 39–45 CrossRef CAS PubMed.
- H. V. Zhang, F. Polzer, M. J. Haider, Y. Tian, J. A. Villegas, K. L. Kiick, D. J. Pochan and J. G. Saven, Sci. Adv., 2016, 2, e1600307 Search PubMed.
- M. C. Childers and V. Daggett, Mol. Syst. Des. Eng., 2017, 2, 9–33 CAS.
- Y. Huang and L. Mucke, Cell, 2012, 148, 1204–1222 CrossRef CAS PubMed.
- H. A. Lashuel, C. A. Overk, A. Oueslati and E. Masliah, Nat. Rev. Neurosci., 2013, 14, 38–48 CrossRef CAS PubMed.
- P. Westermark, A. Andersson and G. T. Westermark, Physiol. Rev., 2011, 91, 795–826 CrossRef CAS PubMed.
- A. A. Orr, M. M. Wördehoff, W. Hoyer and P. Tamamis, J. Phys. Chem. B, 2016, 120, 12781–12794 CrossRef CAS PubMed.
- K. Tao, J. Wang, P. Zhou, C. Wang, H. Xu, X. Zhao and J. R. Lu, Langmuir, 2011, 27, 2723–2730 CrossRef CAS PubMed.
- K. Tenidis, M. Waldner, J. Bernhagen, W. Fischle, M. Bergmann, M. Weber, M. L. Merkle, W. Voelter, H. Brunner and A. Kapurniotu, J. Mol. Biol., 2000, 295, 1055–1071 CrossRef CAS PubMed.
- S. Gilead and E. Gazit, Exp. Diabetes Res., 2008, 2008, 256954 CrossRef PubMed.
- E. Gazit, FASEB J., 2002, 16, 77–83 CrossRef CAS PubMed.
- E. Gazit, FEBS J., 2005, 272, 5971–5978 CrossRef CAS PubMed.
- E. Gazit, Prion, 2007, 1, 32–35 CrossRef PubMed.
- M. J. van Raaij, A. Mitraki, G. Lavigne and S. Cusack, Nature, 1999, 401, 935–938 CrossRef CAS PubMed.
- K. Papanikolopoulou, G. Schoehn, V. Forge, V. T. Forsyth, C. Riekel, J. F. Hernandez, R. W. Ruigrok and A. Mitraki, J. Biol. Chem., 2005, 280, 2481–2490 CrossRef CAS PubMed.
- G. Deidda, S. V. R. Jonnalagadda, J. W. Spies, A. Ranella, E. Mossou, V. T. Forsyth, E. P. Mitchell, A. Mitraki and P. Tamamis, ACS Biomater. Sci. Eng. DOI:10.1021/acsbiomaterials.6b00570 , Article ASAP.
- S. L. Bellis, Biomaterials, 2011, 32, 4205–4210 CrossRef CAS PubMed.
- T. D. Sargeant, C. Aparicio, J. E. Goldberger, H. Cui and S. I. Stupp, Acta Biomater., 2012, 8, 2456–2465 CrossRef CAS PubMed.
- H. Hosseinkhani, M. Hosseinkhani, F. Tian, H. Kobayashi and Y. Tabata, Biomaterials, 2006, 27, 4079–4086 CrossRef CAS PubMed.
- T. D. Sargeant, M. S. Rao, C. Y. Koh and S. I. Stupp, Biomaterials, 2008, 29, 1085–1098 CrossRef CAS PubMed.
- K. I. Min, G. Yun, Y. Jang, K. R. Kim, Y. H. Ko, H. S. Jang, Y. S. Lee, K. Kim and D. P. Kim, Angew. Chem., Int. Ed., 2016, 55, 6925–6928 CrossRef CAS PubMed.
- G. Qin, A. Rivkin, S. Lapidot, X. Hu, I. Preis, S. B. Arinus, O. Dgany, O. Shoseyov and D. L. Kaplan, Biomaterials, 2011, 32, 9231–9243 CrossRef CAS PubMed.
- B. P. Partlow, C. W. Hanna, J. Rnjak-Kovacina, J. E. Moreau, M. B. Applegate, K. A. Burke, B. Marelli, A. N. Mitropoulos, F. G. Omenetto and D. L. Kaplan, Adv. Funct. Mater., 2014, 24, 4615–4624 CrossRef CAS PubMed.
- Y. F. Huang, S. C. Lu, Y. C. Huang and J. S. Jan, Small, 2014, 10, 1939–1944 CrossRef CAS PubMed.
- D. Ding, P. A. Guerette, J. Fu, L. Zhang, S. A. Irvine and A. Miserez, Adv. Mater., 2015, 27, 3953–3961 CrossRef CAS PubMed.
- E. Y. Jeon, B. H. Hwang, Y. J. Yang, B. J. Kim, B. H. Choi, G. Y. Jung and H. J. Cha, Biomaterials, 2015, 67, 11–19 CrossRef CAS PubMed.
- F. LaBella, F. Keeley, S. Vivian and D. Thornhill, Biochem. Biophys. Res. Commun., 1967, 26, 748–753 CrossRef CAS PubMed.
- S. O. Anderson, Acta Physiol. Scand., Suppl., 1966, 263, 1–81 CAS.
- C. M. Elvin, A. G. Carr, M. G. Huson, J. M. Maxwell, R. D. Pearson, T. Vuocolo, N. E. Liyou, D. C. Wong, D. J. Merritt and N. E. Dixon, Nature, 2005, 437, 999–1002 CrossRef CAS PubMed.
- S. Garcia-Castineiras, J. Dillon and A. Spector, Science, 1978, 199, 897–899 CAS.
- P. Briza, G. Winkler, H. Kalchhauser and M. Breitenbach, J. Biol. Chem., 1986, 261, 4288–4294 CAS.
- Y. Ding, Y. Li, M. Qin, Y. Cao and W. Wang, Langmuir, 2013, 29, 13299–13306 CrossRef CAS PubMed.
- J. Smadbeck, M. B. Peterson, G. A. Khoury, M. S. Taylor and C. A. Floudas, J. Visualized Exp., 2013, e50476 Search PubMed.
- P. Tamamis, A. López de Victoria, R. D. Gorham Jr, M. L. Bellows-Peterson, P. Pierou, C. A. Floudas, D. Morikis and G. Archontis, Chem. Biol. Drug Des., 2012, 79, 703–718 CAS.
- H. K. Fung, M. S. Taylor and C. A. Floudas, Optim. Methods Softw., 2007, 22, 51–71 CrossRef.
- H. K. Fung, C. A. Floudas, M. S. Taylor, L. Zhang and D. Morikis, Biophys. J., 2008, 94, 584–599 CrossRef CAS PubMed.
- B. I. Dahiyat and S. L. Mayo, Science, 1997, 278, 82–87 CrossRef CAS PubMed.
- D. Tobi and R. Elber, Proteins, 2000, 41, 40–46 CrossRef CAS.
- V. N. Maiorov and G. M. Crippen, J. Mol. Biol., 1992, 227, 876–888 CrossRef CAS PubMed.
- M. Vendruscolo, R. Najmanovich and E. Domany, Proteins, 2000, 38, 134–148 CrossRef CAS.
- C. Pons, D. Talavera, X. de la Cruz, M. Orozco and J. Fernandez-Recio, J. Chem. Inf. Model., 2011, 51, 370–377 CrossRef CAS PubMed.
- R. Rajgaria, S. R. McAllister and C. A. Floudas, Proteins, 2006, 65, 726–741 CrossRef CAS PubMed.
- R. Rajgaria, S. R. McAllister and C. A. Floudas, Proteins, 2008, 70, 950–970 CrossRef CAS PubMed.
- M. Berrera, H. Molinari and F. Fogolari, BMC Bioinf., 2003, 4, 8 CrossRef PubMed.
- J. J. Irwin and B. K. Shoichet, J. Chem. Inf. Model., 2005, 45, 177–182 CrossRef CAS PubMed.
- U. Haberthür and A. Caflisch, J. Comput. Chem., 2008, 29, 701–715 CrossRef PubMed.
- B. R. Brooks, R. E. Bruccoleri, B. D. Olafson, D. J. States, S. Swaminathan and M. Karplus, J. Comput. Chem., 1983, 4, 187–217 CrossRef CAS.
- R. Swendsen and J. Wang, Phys. Rev. Lett., 1987, 57, 2607–2609 CrossRef PubMed.
- K. Hukushima and K. Nemoto, J. Phys. Soc. Jpn., 1996, 65, 1604–1608 CrossRef CAS.
- U. Hansmann, Chem. Phys. Lett., 1997, 281, 140–150 CrossRef CAS.
- Y. Sugita and Y. Okamoto, Chem. Phys. Lett., 1999, 314, 141–151 CrossRef CAS.
- K. Y. Sanbonmatsu and A. E. Garcia, Proteins, 2002, 46, 225–234 CrossRef CAS PubMed.
- H. Nymeyer, S. Gnanakaran and A. E. Garcia, Methods Enzymol., 2004, 30, 119–149 Search PubMed.
- M. Seeber, M. Cecchini, F. Rao, G. Settanni and A. Caflisch, Bioinformatics, 2007, 23, 2625–2627 CrossRef CAS PubMed.
- B. R. Brooks, C. L. Brooks III, A. D. MacKerell Jr., L. Nilsson, R. J. Petrella, B. Roux, Y. Won, G. Archontis, C. Bartels, S. Boresch, A. Caflisch, L. Caves, Q. Cui, A. R. Dinner, M. Feig, S. Fischer, J. Gao, M. Hodoscek, W. Im, K. Kuczera, T. Lazaridis, J. Ma, V. Ovchinnikov, E. Paci, R. W. Pastor, C. B. Post, J. Z. Pu, M. Schaefer, B. Tidor, R. M. Venable, H. L. Woodcock, X. Wu, W. Yang, D. M. York and M. Karplus, J. Comput. Chem., 2009, 30, 1545–1614 CrossRef CAS PubMed.
- W. Kabsch and C. Sander, Biopolymers, 1983, 22, 2577–2637 CrossRef CAS PubMed.
-
S. Chandrasekhar, Liquid Crystals, Cambridge University Press, Cambridge, England, 1992 Search PubMed.
-
P. G. de Gennes and J. Prost, The Physics of Liquid Crystals, Oxford University Press, Oxford, UK, 1993 Search PubMed.
- C. Zannoni, J. Mater. Chem., 2001, 11, 2637–2646 RSC.
- R. Berardi, L. Muccioli and C. Zannoni, ChemPhysChem, 2004, 5, 104–111 CrossRef CAS PubMed.
-
M. E. Rose, Elementary Theory of Angular Momentum, Wiley, New York, 1957 Search PubMed.
-
C. Zannoni, in The Molecular Physics of Liquid Crystals, Academic, London, 1979, ch. 3, pp. 51–83 Search PubMed.
-
M. P. Allen and D. Tildesley, J. Computer Simulation of Liquids, Oxford Science, Oxford, UK, 1987 Search PubMed.
- M. Cecchini, F. Rao, M. Seeber and A. Caflisch, J. Chem. Phys., 2004, 121, 10748–10756 CrossRef CAS PubMed.
- T. P. Doerr, D. Heman, H. Mathur and P. L. Taylor, Europhys. Lett., 2002, 59, 398–402 CrossRef CAS.
- L. Wesson and D. Eisenberg, Protein Sci., 1992, 1, 227–235 CrossRef CAS PubMed.
- E. Ruoslahti, Annu. Rev. Cell Dev. Biol., 1996, 12, 697–715 CrossRef CAS PubMed.
- M. D. Pierschbacher and E. Ruoslahti, Proc. Natl. Acad. Sci. U. S. A., 1984, 81, 5985–5988 CrossRef CAS.
- E. F. Plow, M. D. Pierschbacher, E. Ruoslahti, G. A. Marguerie and M. H. Ginsberg, Proc. Natl. Acad. Sci. U. S. A., 1985, 82, 8057–8061 CrossRef CAS.
- S. Suzuki, A. Oldberg, E. G. Hayman, M. D. Pierschbacher and E. Ruoslahti, EMBO J., 1985, 4, 2519–2524 CAS.
- A. L. Main, T. S. Harvey, M. Baron, J. Boyd and I. D. Campbell, Cell, 1992, 71, 671–678 CrossRef CAS PubMed.
- M. W. Bowler, D. Nurizzo, R. Barrett, A. Beteva, M. Bodin, H. Caserotto, S. Delagenière, F. Dobias, D. Flot, T. Giraud, N. Guichard, M. Guijarro, M. Lentini, G. A. Leonard, S. McSweeney, M. Oskarsson, W. Schmidt, A. Snigirev, D. von Stetten, J. Surr, O. Svensson, P. Theveneau and C. Mueller-Dieckmann, J. Synchrotron Radiat., 2015, 22, 1540–1547 Search PubMed.
Footnotes |
† Electronic supplementary information (ESI) available. See DOI: 10.1039/c7me00016b |
‡ S. V. R. J. and E. O. are equally contributing first authors. |
|
This journal is © The Royal Society of Chemistry 2017 |