DOI:
10.1039/D3EN00810J
(Paper)
Environ. Sci.: Nano, 2024, Advance Article
In chemico methodology for engineered nanomaterial categorization according to number, nature and oxidative potential of reactive surface sites†
Received
10th November 2023
, Accepted 5th July 2024
First published on 9th July 2024
Abstract
Methanol probe chemisorption quantifies the number of reactive sites at the surface of engineered nanomaterials, enabling normalization per reactive site in reactivity and toxicity tests, rather than per mass or physical surface area. Subsequent temperature-programmed surface reaction (TPSR) of chemisorbed methanol identifies the reactive nature of surface sites (acidic, basic, redox or combination thereof) and their reactivity. Complementary to the methanol assay, a dithiothreitol (DTT) probe oxidation reaction is used to evaluate the oxidation capacity. These acellular approaches to quantify the number, nature, and reactivity of surface sites constitute a new approach methodology (NAM) for site-specific classification of nanomaterials. As a proof of concept, CuO, CeO2, ZnO, Fe3O4, CuFe2O4, Co3O4 and two TiO2 nanomaterials were probed. A harmonized reactive descriptor for ENMs was obtained: the DTT oxidation rate per reactive surface site, or oxidative turnover frequency (OxTOF). CuO and CuFe2O4 ENMs exhibit the largest reactive site surface density and possess the highest oxidizing ability in the series, as estimated by the DTT probe reaction, followed by CeO2 NM-211 and then titania nanomaterials (DT-51 and NM-101) and Fe3O4. DTT depletion for ZnO NM-110 was associated with dissolved zinc ions rather than the ZnO particles; however, the basic characteristics of the ZnO NM-110 particles were evidenced by methanol TPSR. These acellular assays allow ranking the eight nanomaterials into three categories with statistically different oxidative potentials: CuO, CuFe2O4 and Co3O4 are the most reactive; ceria exhibits a moderate reactivity; and iron oxide and the titanias possess a low oxidative potential.
Environmental significance
The hazard of nanomaterials is associated with their surface reactivity. We report an in chemico approach to probe the number, nature and reactivity of surface sites. This approach demonstrates how many sites we have at the surface of nanomaterials that are relevant for a dose metric based on actual sites rather than on the surface or mass. This would allow for better insight into dose–response investigations. This NAM provides insights into whether sites are oxidative, acidic, basic, or a combination thereof, and additionally aids in ranking NMs by reactivity, which is crucial for understanding their mechanisms of toxicity. In a broader view, it can characterize nanomaterials and how their reactivity evolves as they change, making multicomponent nanomaterials and as they age during operation and in the environment.
|
1 Introduction
The surface of engineered nanomaterials (ENMs)
Metal oxides possess a lattice in which the unit cell repeats ad infinitum. However, materials are finite and interact with the surrounding environment through their surface, which represents the end of the lattice periodic structure. This surface is characterized by descriptors such as specific surface area (BET area), pore size, or zeta potential (ζ).1 Surface chemistry defines materials' reactivity (type and strength); in metal oxides, it is often associated with surface oxygen species, such as bridging oxygen, oxide, superoxide, peroxide, or hydroxyl sites (Fig. 1), the properties of which are determined by underlying cations, defects, and the bulk structure. Charge unbalances, such as surface vacancies and defects, are stabilized to maintain the material neutrality, typically by surface interactions, e.g., with environmental water, generating surface hydroxyl groups. The compensation mechanisms for defects may vary depending on the nature of the material. For instance, in ionic materials, defect compensation might involve the the formation of farb centers; incovalent ENMs, it could involve covalent; and in transition metal oxides, it may involve transitions between the valance and the conduction band.2 Surface relevance is maximized in non-soluble nanomaterials (NMs, with one dimension in the 1–100 nm range),3 in which a high surface-to-volume ratio confers them with distinctive properties. For example, in the field of ecotoxicology, 40 mg L−1 of nano-sized CuO particles completely inhibit the growth of S. cerevisiae, while 4000 mg L−1 of CuO bulk material is needed to achieve this;4 the number of exposed sites is probably not dramatically different between these two very different amounts of CuO materials.
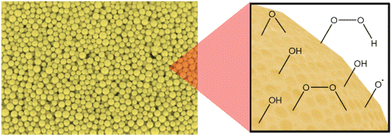 |
| Fig. 1 Reactive sites on the ENM surface. | |
Rise of engineered nanomaterials and concerns about their toxicity
Engineered nanomaterial applications have grown significantly, influencing societal challenges and the economy, especially in Asia-Pacific, America, and Europe.5–7 Transition metal oxide ENMs, including TiO2, CuO, and ZnO, have versatile uses, such as pigments and catalysts.8,9 This has prompted numerous characterization, exposure and hazard studies10–12 to understand and prevent possible adverse effects or pathologies, for example, those derived from reactive oxygen species (ROS) release,13,14 and to adopt a knowledge-based safe-by-design (SbD) approach,15,16 which is essential to ensure safe ENM applications as well as faster, more economical and more effective production routes.17,18 Integrated information related to toxicity (in vitro and in vivo testing) and physicochemical properties underpins hazard prediction16,19,20–23 with machine learning serving as the primary tool.24 Grouping ENMs based on similarities can optimize resource management, aligning with OCDE guidelines for risk assessment and promoting non-animal testing methodologies.25,26 New approach methodologies (NAMs) may provide the basis for this objective.27–29 The overarching aim is to contribute to the understanding of adverse outcome pathways (AOPs) and, particularly, what reactive properties are associated with the triggering of adverse effects by nanomaterials.30
New approach methodology based on surface site reactivity
Nanomaterial surface reactivity plays a vital role in oxidative-stress-induced adverse effects.31–34 Because reactivity is an extrinsic property considered a key parameter to describe the interaction of ENMs with their surroundings,16,19 the development of abiotic in chemico assays to evaluate surface reactivity and link it with key events in reactive-based nanotoxicity would help to fundamentally understand the modes of action35–37 and better group ENMs while minimizing in vivo testing.38 Surface reactivity characterization complements other physicochemical information relevant to the nanotoxicity field19 to investigate toxic ion release, lung fibrosis, inflammasome activation, and interference with embryonic hatching or membrane lysis, among others. In the context of reactive-based toxicity assessment of engineered nanomaterials, it is widely acknowledged that materials with identical chemical compositions can lead to significantly varied biological oxidative damage.34 Thus, characterizing the amount, nature and reactivity of surface sites is essential for identifying an additional parameter impacting nanomaterial's effects. The interaction with biological systems depends on the surface properties of the nanomaterials, and the presence of any kind of active site may have effects on their interaction with molecules. We hypothesize that mapping all reactive sites (redox, acidic and basic) may provide a better reactive description of nanomaterials than just oxidative sites and enable a more reliable grouping of nanomaterials based on their surface reactivity. Formally equivalent problems have formally equivalent solutions: as key events in reactive-based toxicity and catalytic reactions occur at the surface; more specifically, at the reactive sites, we propose the use of catalytic methods based on the adsorption and reaction of probe molecules to quantify the surface reactive sites of ENMs and characterize their reactive nature, thus delivering descriptors relevant for ENM classification.39,40
Reactive characterization may also provide new dose metrics. In in vitro tests with different cell lines, quantitative dose-dependent cellular/biological effects are typically normalized by the mass or physical BET area. These dose metrics may sometimes not be useful to compare exposure because the mass or exposed physical area does not necessarily correlate with the number of reactive sites, which trigger chemical processes, e.g., ROS formation (the generation of ROS by particles is one of the possible molecular initiating events that lead to adverse outcomes, as confirmed, e.g., for PM, CuO, or photo-activated TiO2). We do not tackle photocatalytic phenomena that are unlikely to happen inside the body. Research on heterogeneous catalysis has traditionally faced the same challenge when comparing the activities of catalytic materials and has reached a consensus that the most relevant metric is the turnover frequency (TOF). TOF is the number of times that the overall catalytic reaction occurs (i.e., molecules that react) per reactive site and unit time.39,41–44 Our research posits that probe molecules allow the quantification of reactive surface sites, their nature and reactivity; TOF calculation can thus be made based on relevant probe reactions (e.g., DTT), offering new metrics for reactivity and toxicological studies.
The most typical probe molecules used to quantify reactive sites in heterogeneous catalysis are carbon monoxide for metal NPs45 and methanol for metal oxides, both in the gas/vapor phase. The latter is considered a “smart” probe molecule that can quantify the number of surface sites by chemisorption and report on their reactive profile by temperature-programmed surface reaction (TPSR),46,47 a powerful technique to identify and quantify acidic, basic, redox, and bifunctional sites48,49 on materials that are not thermally sensitive. In addition, several probe molecules in the liquid phase may specifically assess the oxidative potential (OP),50–55 which is particularly relevant to human health due to its involvement in cellular damage caused by oxidative stress.10,56–60 Among them, dithiothreitol (DTT) depletion is suggested here as an acellular, liquid-phase, low-temperature probe reaction to assess the nanomaterial OP because this molecule has been previously used to quantify the oxidative capacity of particulate matter.61–66 We therefore introduce a NAM using gas-phase methanol chemisorption and subsequent TPSR as well as liquid-phase DTT consumption in PBS-water solutions with nanomaterials. By normalizing the DTT oxidation rate via methanol chemisorption, we derive the oxidative turnover frequency (OxTOF) to measure surface site reactivity. We suggest dose normalization of the amount and reactivity of the reactive sites. As a proof of concept, seven metal oxide nanomaterials (CeO2, ZnO, CuO, Fe3O4, Co3O4, and two TiO2 variants), one bimetallic nanooxide (CuFe2O4) and an oxide with larger particles (Co3O4) are analyzed to investigate the usefulness of this NAM to 1), categorize the reactivity of eight benchmark engineered nanomaterials; 2) assess the differences in reactivity between ENMs with the same composition (TiO2 NM-101 vs. TiO2 DT-51); 3), assess the effect of bimetallic compositions on the surface reactivity of metal nano-oxides (monometallic vs. bimetallic); 4), calculate reactive rankings according to three dose metrics based on mass, surface area and surface sites; and 5), assess the size-dependent reactivity of a material by comparing Co3O4 nanoparticles to its larger counterpart.
2 Experimental
2.1 Nanomaterials
All nanomaterials were used as supplied. Two anatase TiO2 powders were compared: DT51 (CristalACTiV™) and NM-101 (labeled JRCNM01001a by the supplier, the Joint Research Centre, JRC). In addition, two more JRC samples: CeO2 NM-211 (JRCNM02101a) and ZnO NM-110 (JRCNM62101a), as well as four commercial samples from Sigma-Aldrich (CuO (ref. number: 544868, CuO-SA), CuFe2O4 (ref. number: 641723, CuFe2O4-SA), Fe3O4 (ref. number: 637106, Fe3O4-SA), and Co3O4 (ref. number: 637025, Co3O4-SA)) were evaluated. Table S1† summarizes the data and information on these proof-of-concept samples. The size dependence of reactivity was evaluated using Co3O4 microparticles (ref. number: 221643, Sigma-Aldrich <10 μm).
2.2 Specific surface area
The specific surface area was calculated by applying the BET method with data obtained in Micromeritics ASAP 2020 adsorption isotherm equipment. All ENMs were pretreated by degassing under vacuum for 16 h at 120 °C before nitrogen adsorption at liquid nitrogen temperature.
2.3 Methanol chemisorption and subsequent temperature-programmed surface reaction (TPSR)
Methanol chemisorption/TPSR procedure (see a detailed description in the ESI,† Fig. S1A and S2) is made on a clean dehydrated sample. 100–250 mg of nanomaterial (aggregated samples with aggregates ranging from 25 to 100 μm) were diluted with 500 mg of inert SiC (black 180, Navarro SiC S.A.) under isothermal conditions and placed in a fixed-bed reactor (0.4 cm internal diameter). The sample is first pretreated by heating from room temperature to 450 °C at 10 °C min−1 in a 150 mL min−1 synthetic air flow and kept at this temperature for 35 min to ensure the removal of moisture and burn away impurities from its surface. After pretreatment, the sample is cooled to 100 °C (or 50 °C for highly reactive ENMs) in synthetic air. After such treatment, the surface remains hydroxylated, but not hydrated; next, the flow feed is switched to argon (100 mL min−1) purge. The chemisorption temperature was optimized to prevent the formation of multilayers in the case of highly reactive materials, looking for a balance between methanol condensation at lower temperatures and methanol reaction at higher temperatures, either of which would lead to an overestimation of the surface sites.67,68 After purging, at 100 °C (or 50 °C for highly reactive ENMs), 100 mL min−1 of 2000 ppm methanol in argon with 5% helium is fed until saturation, as determined by online mass spectrometry residual gas analysis (cf. ESI†). The 5% helium in the argon stream is used as an internal reference for online mass spectrometry. The methanol vapor chemisorbs titrating surface hydroxyl groups; this process converts the CH3OH molecule into a chemisorbed CH3O-moiety. The missing hydrogen atom reacts with the surface hydroxyl, thus releasing an H2O molecule per CH3OH molecule that chemisorbs. We monitor the effluent gases by applying a quadrupole residual gas analyzer Pfeiffer OmniStar mass spectrometer. The m/z values followed were CH3OH (methanol) = 31, HCHO (formaldehyde) = 30, CH3OCH3 (dimethyl ether, DME) = 45, CH3OOCH (methylformate) = 60, (CH3O)2CH2 (dimethoxy methane) = 75, H2O (water) = 18, and CO2 (carbon dioxide) = 44. Blank tests were performed with 500 mg of inert SiC (Fig. S3†). Details on the procedure, the calculation of the reactive surface sites and the surface reactions in methanol-TPSR (eqn (S1)–(S5)) are available in the ESI† material. This methodology is limited to thermally stable samples, such as metal oxides.
2.4 DTT consumption assay
DDT catalytic oxidation was performed using a batch reactor for 1 h. First, a 200 μg mL−1 suspension of ENM in 1 mM phosphate buffer is obtained by sonication, following NanoGenoTox SOP (16 min at 400 W and 10% amplitude).69 3 mL of the ENM suspension is incubated for 1 h at 37 °C and 500 rpm with 3 mL of 100 μM DTT, obtaining a 6 mL reaction mixture with 100 μg mL−1 of ENM and 50 μM DTT. Then, the nanoparticles are removed by filtration, and the filtrate, with the unreacted DTT and the reaction products, is mixed with an equal volume of 1 mM Ellman's reagent (5,5′-dithiobis-(2-nitrobenzoic acid), DTNB) to quantify the non-oxidized DTT (Fig. S1B†). Ellman's reagent reacts with the thiol groups (–SH) of the free DTT molecules, forming 5-mercapto-2-nitrobenzoic acid, a colorful complex that is measured at 412 nm by UV-vis spectrophotometry (Shimadzu, UV-2100). In parallel, as a negative control, DTT in phosphate buffer without ENM is incubated under the same conditions and mixed with the Ellman's reagent to evaluate the DTT consumed by direct reaction without catalyst. Hydrogen peroxide 30% (w/w) in H2O is used as a positive control because it provides DTT conversion similar to that of 1,4-naphthoquinone,62 which is safer and does not require filtration. All reactions were performed in triplicate. Linearity in the measurements at 412 nm of the DTT-DTNB nm complex was calibrated (Fig. S4†). The DTT oxidative potential is expressed as DTT conversion (eqn (1)), as a normalized index of oxidant generation using hydrogen peroxide as a positive control (eqn (2)), or as the DTT reaction rate, normalized vs. mass (eqn (3)), vs. the ENM surface area (eqn (4)), or vs. the number of reactive sites (eqn (5)), i.e., OxTOF. |
 | (1) |
|
 | (2) |
|
 | (3) |
|
 | (4) |
|
 | (5) |
2.5 Statistical analysis
DTT OPmas, OParea and OxTOF are expressed as average ± sd (standard deviation). The statistical analysis was performed with SPSS 20 (IBM, Armonk, USA) using logarithmic values to obtain a better normal distribution. One-way ANOVA (analysis of variance) was performed to determine statistically significant differences. Subsequently, a Tukey test was performed to assess pairwise differences with a significance level of p < 0.05 and classify ENMs according to the oxidative potential.
3 Results
3.1 Surface area and reactive sites
In the series, TiO2 NM-101 exhibits the largest BET area, 225 m2 g−1; the rest of the ENMs have significantly lower BET values: TiO2-DT51, 84 m2 g−1; CeO2 NM-211, 76 m2 g−1; CuO-SA, 12 m2 g−1; ZnO NM-110, 9 m2 g−1; CuFe2O4-SA, 33 m2 g−1; Fe3O4-SA, 11 m2 g−1; and Co3O4-SA, 26 m2 g−1 (Fig. 2A). These data are consistent with the values reported in the literature and the supplier's technical sheets.70–74 Fig. 2B illustrates the specific number of sites (mmol g−1). Although the order of materials remains similar to BET area, the relative values change significantly between Fig. 2A and B. Thus, the reactive site surface density (site per nm2) may follow a different trend. Fig. 2C shows this surface descriptor calculated from the data shown in Fig. 2A and B. Interestingly, the ENMs with smaller surface areas have higher reactive site surface densities: 16.6 and 21.8 sites per nm2 for ZnO NM-110 and CuO-SA, respectively (values obtained at 50 °C). These data show that due to differences in site types and/or distribution, the numbers of surface area and reactive sites do not linearly correlate for these samples, as might be erroneously assumed. It is remarkable that TiO2-DT51, with a specific surface area 2.7 times lower than that of TiO2 NM-101, doubles its reactive site surface density (14 vs. 7 sites per nm2). Therefore, BET (physical) may not be the most relevant descriptor of ENM surface chemistry. Fig. 2D summarizes the trends shown in Fig. 2A–C after data normalization to the most described nanomaterial in the literature: TiO2 NM-101.
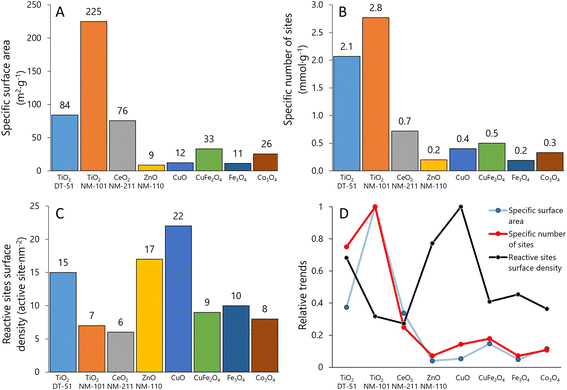 |
| Fig. 2 Surface analysis: A) specific surface area obtained by N2 adsorption isotherm, B) specific number of reactive sites obtained by methanol chemisorption, C) reactive site surface density obtained by combination of A and B, and D) comparison of the three surface descriptors (values normalized to the maximum). | |
3.2 Reactive profile
The methanol TPSR profiles in Fig. 3 provide information on the reactive sites and their reactivity. The typical TPSR products are DME, HCHO and CO2.
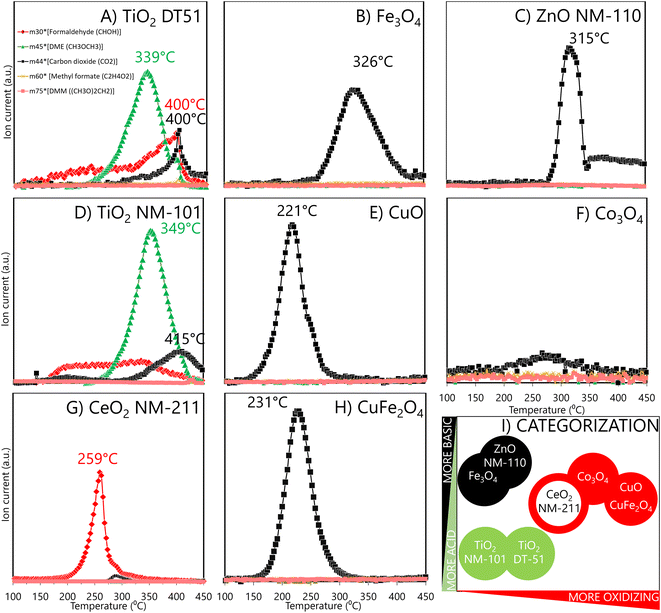 |
| Fig. 3 Temperature-programmed surface reaction products of pre-adsorbed methanol analysed by mass spectroscopy for two different anatase TiO2: DT51 (A) and NM-101 (D), for Fe3O4-SA (B), for ZnO NM-110 (C), for CuO-SA (E), for Co3O4-SA (F) for CeO2 NM-211 (G), and for CuFe2O4-SA (H). Formaldehyde signal (red) is obtained for redox sites, a dimethyl ether signal (green) for acid sites, and carbon dioxide (black) for basic or high reactive redox sites. ENM classification by MeOH-TPSR results is shown in I) with the same colour code. For a given colour, filled circles are more reactive than empty circles. | |
TiO2 ENMs (DT51 and NM-101) mainly form dimethyl ether (Fig. 3A and D), the characteristic product of acidic reactivity. The maximum production of dimethyl ether occurs at 349 °C for NM-101 and at 339 °C for DT51, indicating a weaker acidity of the sites of the latter, which are also fewer, as indicated by the smaller area under the curve. Redox (HCHO) and basic (CO2) reaction products also form on both titania samples. The redox site is active in a broad temperature range, which indicates a broad distribution of oxidation reactivities, and that the oxidation capacity is moderate because rather high temperatures are required to express it.
Oxidative sites produce HCHO. Thus, CeO2 exhibits redox sites where methanol is oxidized to formaldehyde, with a maximum near 259 °C (Fig. 3G); ceria oxidative sites exhibit a narrower peak, indicating that most oxidizing sites have similar reactivity. This is unlike the broad distribution of oxidative site types on the titanias in the 150 to 400 °C range. Moreover, ceria has a higher oxidation capacity because its maximum is at a lower temperature than the average of HCHO formation on the titania samples.
ZnO NM-110, CuFe2O4-SA, Fe3O4-SA, Co3O4-SA and CuO-SA (Fig. 3C–H) produce mainly CO2, but the temperatures at which CO2 reaches a maximum differ significantly; for some, it is near 220–250 °C, and for others, it is above 300 °C. The significantly higher maximum temperature for CO2 production indicates basic materials, where the methoxy species adsorb strongly and can only desorb at very high temperatures before being combusted (ZnO NM-110 and Fe3O4-SA). Instead, the easier formation of CO2 (some 100 °C lower temperatures) indicates a high oxidation capacity, leading to total oxidation of CO2 rather than to partial oxidation formaldehyde (CuO-SA, CuFe2O4-SA and Co3O4-SA).49 Evaluated as a reference, micrometric Co3O4 (Fig. S5†) showed a similar reactive profile to Co3O4 nanoparticles. Thus, the nature of the surface sites in nano and micro CuO remains essentially alike, with the critical difference that a minimum part of the reactive sites is exposed in the larger CuO particles; hence, the risk of exposure to larger CuO particles is minimized. In summary, methanol TPSR reactive profiles may classify materials based on a linear combination of their acidic/basic reactive profile vs. their oxidation profile (Fig. 3I). In this categorization, the X-axis qualitatively indicates how oxidizing the material is, while the Y-axis moves from an acidic to a basic character. Thus, TiO2 DT51 and NM-101, ZnO NM-110, and Fe2O3-SA have moderate oxidation capacities, while Fe2O3-SA and ZnO NM-110 are more basic, and both TiO2 variants are more acidic. However, CeO2 NM-211 and Co3O4-SA exhibit increased oxidation capacity, and the highest is for CuO-SA and CuFe2O4-SA.
3.3 Oxidative potential
Fig. 4 illustrates DDT catalytic oxidation results for 1 h reaction, normalized vs. different descriptors; the corresponding classification of the ENMs based on Tukey's test using the logarithm of OPmass, OParea and OxTOF is provided on the right side of the plots. ZnO NM-110 is not included in the analysis because it dissolves in the reaction media and Zn cations are complexed by DTT;66 therefore, no free and uncomplexed DTT is available for interaction with the ZnO NM-110 surface, and thus the results are close to the negative control.66 The relative oxidative potential of the other ENMs significantly depends on the descriptor. The positive control normalization has little impact on the relative conversion trend (Fig. S6†), which is similar to that of the specific reaction rate shown in Fig. 4A: CuO-SA ≈ CuFe2O4-SA ⋙ Co3O4-SA ≈ TiO2 NM-DT-51 ≈ TiO2 NM-101 ≈ CeO2 NM-211 > Fe3O4-SA. According to Tukey's test, only CuO-SA and CuFe2O4-SA are classified as significantly highly reactive ENMs. The differences between the reactivities of these ENMs are clearly amplified when the oxidation rate is normalized to the ENM surface area (Fig. 4B), which underlines that the CuO-SA surface, being small (Fig. 2A), is significantly more reactive than other ENM surfaces in the series. Actually, Tukey's test reveals three reactivity groups of ENMs according to OParea descriptor: CuO-SA > Co3O4-SA ≈ CuFe2O4-SA > TiO2 DT-51 ≈ CeO2 NM-211 ≈ TiO2 NM-101 ≈ Fe3O4-SA. Mass or BET normalizations cannot tell how reactive each site is, so this trend assumes that all physical areas are equally populated by equally reactive sites, which is not the case. Normalization per reactive site (Fig. 4C) delivers the OxTOF, which shows that the CuO-SA and CuFe2O4-SA sites are the most oxidizing sites, followed by Co3O4-SA, and ca. fourfold more reactive than the ceria sites. The remaining group of materials exhibits significantly lower oxidation activity according to Tukey's test: CeO2 NM-211 > TiO2 DT-51 ≈ TiO2 NM-101 ≈ Fe3O4-SA. A larger number of sites can make up for an individual site's lower reactivity; therefore, both pieces of reactivity information, global (per material dose) and individual (per site), are important to understand and classify ENMs. Among these descriptors, only OxTOF can identify with statistical significance that ceria has more reactive redox sites than titania ENMs. From a chemical perspective, turnover frequency values may allow for a better quantitative comparison of oxidative potential and provide more accurate insight on reactivity at a molecular scale.
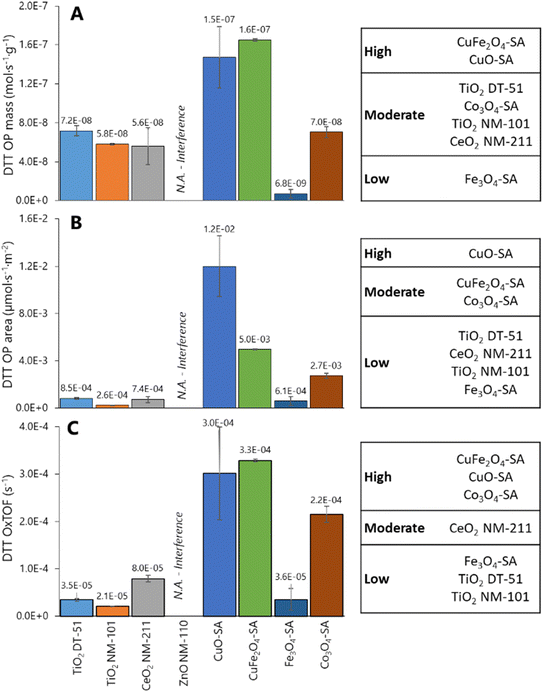 |
| Fig. 4 Oxidative potential evaluated by DTT assay and expressed as reaction rate per mass (A), reaction rate per surface (B), and oxidative turnover frequency (reaction rate per reactive site) (C). Left: Averaged OP values (n = 3) with error bars indicating the standard deviation. Right: Statistical analysis for classification of the ENMs by OP based on Tukey's test comparison. | |
Size dependence of reactivity was evidenced via comparison of Co3O4 nanoparticles vs. microparticles, which exhibited a DTT depletion of 6.7 ± 4.2% of DTT depletion, equivalent to a NIOG of 0.08 ± 0.04, that is, around 7 times lower oxidative capacity than its nanoparticle counterpart. This is essentially due to the significantly smaller fraction of reactive sites exposed.
3.4 Dose metrics applied to bibliographic toxicological data
Bibliographic toxicity information for TiO2 NM-101, CeO2 NM-211, ZnO NM-110, CuO-SA, Fe3O4, CuFe2O4 and Co3O4 was extracted from eNanoMapper75,76 and the literature to investigate possible correlations of the surface reactivity with in vitro toxicity descriptors (Table S2†).
CuO-SA. CuO-SA is highly toxic to pulmonary cells, causing cell death and impairing cell functions after 24 hour exposure.77 The mechanism involves ion release, autophagy activation, and increased lipid peroxidation.78–80 Animal models support lung inflammatory effects but do not show teratogenic potential.81,82 In terms of dose metrics, this ENM, the most oxidant in the series, exhibits significant effects on A549 cell viability at 5, 10 and 17.75 μg mL−1 gravimetric doses, equivalent to 2, 4 and 7.1 μmol L−1 site doses in different studies.78
CuFe2O4-SA. CuFe2O4-SA cytotoxic effects on human lung (A549) and liver (HepG2) cells were analyzed, illustrating dose-dependent toxicity in a concentration range of 10–100 μg ml−1 (i.e., 5–50 μmol site per L). Key observations include mitochondrial membrane potential (MMP) depletion, upregulation of the caspase-3 gene, and increased caspase-3 enzyme activity, suggesting apoptotic cell death as a consequence of exposure to these ENMs. Furthermore, an imbalance in cellular redox status was evident through the induction of ROS and depletion of glutathione (GSH), indicating oxidative stress as a potential underlying mechanism of cytotoxicity.83
ZnO NM-110. ZnO NM-110, extensively studied in vitro, exhibits adverse effects in multiple cell lines, with immune system alterations observed in Raw 264.7 and MH-S macrophages (EC50: 10–25 μg mL−1, i.e., 3–7.5 μmol L−1 sites doses); pulmonary cell lines display cytotoxic and genotoxic effects (LC50: 76 μg mL−1, that is, 22.8 μmol sites per L),84,85 and respiratory and male reproductive cell lines are affected (EC50 < 20 μg mL−1, significantly less than 6 μmol sites per L).86 Hepatic damage in C3A was evidenced through the WST-1 test.87 Proteomic analysis in NRK-52E reveals pronounced effects, particularly in actin carbonylation; this ENM is classified as highly cytotoxic and a protein carbonylation agent.88 Caco-2 cell lines exhibit cytotoxicity due to dissolved Zn2+, and HUVEC cell lines show reduced mitochondrial viability attributed to intracellular Zn ions and ROS. In contrast, TiO2 NM-101 showed no cytotoxicity or inflammatory markers.89,90
TiO2 NM-101. TiO2 NM-101 exhibited no significant cytotoxicity in the A549, HepG2, HK-2, and C3A cell lines. However, the C3A cells showed IL-8 release, indicating inflammation.87,91 BEAS-2B cell viability was unaffected by TiO2 at concentrations in the range of 1–100 μg mL−1, i.e., 2.8–280 μmol sites per L, but DNA damage and IL-6 release were observed at 10 (28 μmol sites per L) and 100 μg mL−1, respectively. RAW 264.7 macrophages exposed to TiO2 NM-101 released IL-6 and TNF-α at higher concentrations (100 μg mL−1).92
CeO2 NM-211. CeO2 NM-211 the toxic mechanism of CeO2 NM-211 remains unclear, but protein aggregation and fibrillation are proposed hypotheses.93,94 CeO2 NM-211 induced moderate pro-inflammatory cytokine release in rat precision-cut lung slices (PCLuS) at 100 μg mL−1, representing a site concentration of 70 μmol L−1. This is in line with other in vivo studies, where inflammatory markers increased in the bronchoalveolar lavage fluid (BALF) after 14 days of exposure.95,96 In vitro, A549 cells exposed to similar cerium oxide nanoparticles at concentrations up to 100 μg mL−1 showed no cytotoxicity.97 In contrast, NR8383 alveolar macrophages exhibited cytotoxicity at 90 μg mL−1 (63 μmol site per L) along with signs of inflammation, including TNF-α release, after CeO2 NM-211 exposure.98 These results are analyzed in subsection 4.2 with respect to our in chemico method, as gravimetric, surface, and reactive site-based concentrations offer insights into the diverse doses of ENMs in toxicology.
Fe3O4-SA. Fe3O4-SA showed no adverse effects on A549 cell viability for 24–72 h at concentrations of up to 100 μg mL−1 (equivalent to 20 μmol site per L), even after being internalized within the cells following 12 h exposure. Furthermore, an increase in lysosomal activity was not detected after 6 h. However, a concentration-dependent decrease in mitochondrial membrane potential at 100 μg mL−1 was statistically significant. There was no induction of pro-inflammatory cytokine secretion, including IL-1β, IL-6, IL-8, and TNF-α. This evidence suggests that iron oxide exhibits low cytotoxicity.99
Co3O4-SA. Co3O4-SA cyto-genotoxic and inflammatory responses of Co3O4-SA in human alveolar (A549) and bronchial (BEAS-2B) cell lines were evaluated at concentrations ranging from 1–40 μg ml−1, which is equivalent to 0.3–12 μmol site per L. Notably, A549 cells exhibited no cytotoxicity, while BEAS-2B cells showed reduced viability at 40 μg ml−1 and early membrane damage at 1, 5, and 40 μg ml−1. Significant direct and oxidative DNA damage was observed in A549 cells at 20 and 40 μg ml−1, with no impact on cytokine release. Conversely, the BEAS-2B cells exhibited significant direct DNA damage at 40 μg ml−1 and notable oxidative DNA damage at lower concentrations, coupled with increased TNF-α and IL-8 release at specific concentrations and exposure times. These results underline the differential cellular responses to cobalt oxide nanoparticles, highlighting the enhanced sensitivity of BEAS-2B cells to cytotoxic, genotoxic, and pro-inflammatory effects.100 The genotoxic effects of cobalt oxide in Chinese hamster lung fibroblast (V79) cells, primarily mediated by reactive oxygen species, were used to compare with their bulk counterparts: Co3O4-SA nanoparticles exhibit pronounced genotoxic effects compared to bulk Co3O4 macroparticles due to significant cytotoxicity and DNA damage attributed to enhanced ROS generation. The mitigation of genotoxic effects with N-acetylcysteine, a ROS scavenger, further confirms the central role of ROS in nanoparticle-induced toxicity. Nano-sized particles facilitate closer cellular interactions, leading to significant cytotoxicity and DNA damage from ROS, unlike the minimal interaction and impact observed with bulk materials.101
4 Discussion
4.1 ENM surface sites and reactivity
The exponential increase in surface-to-volume ratio as the particle size decreases to a few nanometers is crucial. Additionally, quantum confinement and discrete energy levels alter electronic states and surface reactivity. We focus on the phenomenological consequences of this, rather than on its origins. A comprehensive categorization of ENM requires an understanding of their reactivity characteristics: the number of reactive surface sites, their reactive nature and relative reactivity. Nanomaterials can oxidize molecules directly or generate ROS through interactions with biological systems, which may alter their properties. These interactions depend on the surface properties of the nanomaterials, including the reactive sites beyond the physical surface area. Although oxidative potential is often highlighted, acidic and basic sites also significantly impact molecular interactions. Our method maps all reactive sites to provide a comprehensive description of nanomaterials and to enable more reliable grouping based on their chemical surfaces. According to our data, reactivity-triggered nanotoxicity depends not only on the number and reactivity of redox sites but also on the presence of basic and acidic surface sites. Their interplay determines how ENMs interact with the environment and physiology. Parameters, such as site-specific numbers and TOF (e.g., DTT OxTOF), are essential in elucidating the reactive potential of ENMs and linking them to potential adverse effects. Additionally, TPSR profiles provide insights into the relative presence and reactivity of these sites, influencing toxicity profiles. The OxTOF tendency in Fig. 4C shows CuO-SA ≈ CuFe2O4-SA > Co3O4-SA ≫ Ce2O3 NM-211, in line with the oxidation capacity assessed by methanol-TPSR. Following this correlation, the titanias, Fe3O4-SA and ZnO NM-110, exhibit very little methanol oxidation. Therefore, the DTT probe reaction is oxidative dehydrogenation, forming a disulfide group that appears to run mechanistically parallel to the oxidative dehydrogenation of methanol to formaldehyde in TPSR experiments. The use of chemisorption avoids interference from ion release, buffer reactivity (as observed with some probe reactions in phosphate medium),102 or agglomeration that occurs in liquid-phase assays.
Reactive site surface density quantified by methanol chemisorption in our series is consistent with values reported in the literature for oxide ENMs, ranging from 0.4 to 22 sites per nm2, but typically up to ca. 7 sites per nm2, corresponding to a monolayer.39 The high number of reactive sites on CuO-SA (22) and ZnO NM-110 (17) surfaces must be related to a highly reactive interaction with chemisorbed methoxy groups. Multilayer formation is likely on ZnO NM-110 basic sites, as suggested for La2O3, MgO or Cr2O3,39 whereas CuO-SA is a highly oxidizing material that transforms surface methoxy groups into formate groups.103–105 This is consistent with the extensive CO2 desorption profile during MeOH-TPSR. Hence, the chemisorption temperature was set to 50 °C in these materials. CO2 formation at low temperatures on highly reactive CuO-SA is characteristic of formate decomposition, while the formation of CO2 at high temperatures on alkaline ZnO NM-110 is associated with the decomposition of carbonates.104 The determination of reactive sites provides complementary insight to ROS determination probes, whose sensitivity depends on different features. For instance, the basic character of ZnO NM-110 and its high reactive site surface density correlates with the ferric reduction ability of serum (FRAS) assay, an indirect measurement of ROS by total antioxidant depletion, and protein carbonylation assay.106 In another study, electron spin resonance (ESR) spectroscopy with 3-carboxy-2,2,5,5-tetramethylpyrrolidine 1-oxyl (CPH) and 5,5-dimethyl-1-pyrroline N-oxide (DMPO) probe molecules determined the oxidative potential of ENMs by determining the ROS production.107 Little ROS were produced by CeO2 NM-211 and ZnO NM-110 when compared with CuO,106 which agreed with our reactive ranking based on OxTOF data, and the higher number of reactive surface sites of the latter. The CPH spin probe (more sensitive to singlet oxygen, superoxide radicals, and peroxynitrites) revealed higher ROS production by ceria than by zinc oxide, whereas the DMPO spin trap (more sensitive to hydroxyls and superoxide radicals) showed the opposite trend. Raman spectroscopy, which is highly sensitive to peroxide-related species, can be used to further analyze this. In situ108,109 and operando110 Raman spectra show that superoxide and peroxide species are generated at the surface of different ceria materials by interaction with molecular oxygen, but there are no reports of superoxide species formed at the surface of ZnO and TiO2. Thus, reactive superoxide species only account for DMPO and CPH by CeO2 NM-211 but not by ZnO NM-110 or titania; therefore, ZnO NM-110 must generate more hydroxyls than CeO2 NM-211 to account for the DMPO probe results.
Titania, the least-reactive material in our series, highlights the complexity of categorizing nanomaterials. Even with the same composition (TiO2) and crystalline phase (anatase), titania samples differ significantly in BET surface area, reactive site density, and strength. TiO2 NM-101, as measured by terephthalic acid assay, generates ROS upon photoirradiation but not in the dark.111 Conversely, ROS generation detected via the DMPO trap was significantly higher than the control not only upon irradiation but also in the dark, although to a lesser extent.111 Several studies on titania reactivity and photoreactivity highlight the impact of species in biological systems (e.g., carboxylic acids, amines) that strongly adsorb onto titania surfaces, affecting reactivity.102,112 This underscores the importance of characterizing all surface reactive sites: acidic, basic, and redox. The band gap of metal nano-oxides, crucial for correlating with oxidative stress and pulmonary inflammation from photocatalytic ENMs, strongly depends on particle size, nuclearity, and the nature of nearby elements, serving as an indicator of increasing quantum effects.113–115
The strong influence of the titania structural variety on its surface reactivity is described from the perspective of nanoinformatics,116,117 which uses computational approaches to understand the surface structure and reactivity of ENMs, using this data in a FAIR (findability, accessibility, interoperability and reusability) implementation for the nanosafety community.118,119
4.2 Oxidative surface sites and in vitro cell viability
As in vitro assays monitor different effects (cell viability, protein release, inflammation, etc.) in specific cell lines (A549, dTHP-1, etc.) and do not provide information about biodistribution, biopersistency or biotransformation23 of nanomaterials, they are limited in predicting the overall toxicological profile, comparisons are not straightforward, and correlations with physicochemical properties of ENMs can only be done as a first approximation.77,120 The in vitro toxic effects of ENMs, which over-oxidize methanol to CO2 in TPSR and show redox surface reactivity (Co3O4-SA, CuFe2O4-SA and CuO-SA), significantly affected different cell lines,83,101,120 underscoring the implication of reactive surface sites in nanotoxicity field. When CuO-SA and ZnO NM-110 are compared, the higher reactive site surface density and the lower temperature of maximum methanol conversion to CO2 of the former indicate a higher reactivity of CuO-SA, which correlates with the higher toxicity reported by cell viability assays with the A549 line: EC50 for 24 h exposure is 17.75 for CuO-SA and 76 μg mL−1 for ZnO NM-110.84,120 Site-based dose metrics underlines the higher in vitro toxicity of CuO-SA sites. DTT OxTOF could not be evaluated for ZnO NM-110; moreover, the physical–chemical properties, oxidation number, ionic potential, surface reducibility and redox reactivity reported in the literature are consistent with its high in vitro toxicity.121 Nevertheless, ZnO NM-110 is a complex ENM because its surface reactivity has biocidal properties,122 but its mode of action is essentially by dissolution.121
CuO-SA has the highest reactive site surface density in the series although not the most sites per gram and shows the highest OxTOF (Fig. 2D and 4E) along with CuFe2O4-SA. This correlates with their inflammatory effects, commonly associated with ROS generation and oxidative stress, making these ENMs the most toxic in the series.123 CuO-SA's oxidative damage was evaluated in HepG2 cells, with endocytosis transporting nanoparticles to endo/lysosomes, leading to lysosome disruption and copper ion overload.124 This mechanism may involve surface reactivity, which is initially overlooked due to lack of information on reactive sites. CuO-SA induced oxidative changes in A549 cells, increasing protein carbonylation, oxidizing protein thiols, and decreasing cell viability, with no effects from dissolved copper ions.125 These effects were more pronounced in CuO-SA with higher crystalline defects and ROS production likely due to higher reactive site surface density.125 Other studies reported CuO-SA's distinct cytotoxicity in A549 and HeLa S3 cells from direct interactions with cellular components, facilitated by greater surface area and reactive site density compared to microparticles. This parameter could facilitate the surface interactions of CuO-SA with its surroundings. Therefore, elevated intracellular levels can disrupt copper homeostasis, leading to pro-oxidative reactions126 produced at the surface of CuO-SA nanoparticles.127
Co3O4-SA nanoparticles exhibited higher reactivity for DTT depletion than the bulk material, which was reflected in the genotoxic effects of V79 cells and was primarily mediated by reactive oxygen species. This indicates that nano-sized Co3O4-SA induces significant cytotoxicity and DNA damage, unlike larger particles.101 The toxicity of TiO2 NM-101 is not fully understood due to inconsistent evidence across different tests.
In the case of CeO2, the literature has typically linked its toxicity with reactive oxygen species generation and the Ce3+/Ce4+ ratio,128 related to the exposed phase of ceria129,130 and its defects, which are also key for catalytic activity.108 These properties can be easily determined by several techniques.131–135 CeO2 NM-211, with redox surface sites and moderate oxidative capacity, causes cell death by apoptosis and DNA damage in pulmonary cell lines.136,137 The OxTOF of CeO2 NM-211 is between those of the titania ENMs and CuO-SA, despite its low BET area. This is consistent with the intense formaldehyde production in MeOH-TPSR, maximum at 259 °C, and with the characterization reported in the literature: CeO2 NM-211 surface contains 22% Ce(III) (XPS), indicating redox sites, which induce ROS generation, as detected by ESR.131–135 CeO2 is highly oxidizing, while defect-rich CeO2−x has antioxidant properties. This versatility is used to engineer ceria nanoparticles by tuning their properties138,139 and, thus, their performance (e.g. in catalysis), from combustion to selective oxidation,131,140,141 and in biomedical applications, from biocidal to antioxidant.138,139,142 The dynamic states of ceria nanomaterials in aqueous media143 or biological media132,144,145 resulting in defective ceria have been extensively investigated.146
ZnO NM-110, which induces protein carbonylation,147 has a high reactive site surface density, facilitating the formation of a protein corona. This aligns with reports on BSA–ZnO interactions, which demonstrate that protein adsorption on the ZnO NM-110 surface is higher compared to other ENMs, such as TiO2 NM-110.84 Despite TiO2 NM-110 having a larger surface area, its reactive site surface density is lower than that of ZnO NM-110.
Titania and iron oxide exhibit the lowest reactivity. Fe3O4-SA showed no adverse effects even after being internalized within the cells following 12 h exposure. Similarly, both titania samples convert methanol into carbon dioxide, but above 400 °C and to a limited extent, because they are essentially acidic. This low redox reactivity is consistent with their low DTT OxTOF. In line with our hypothesis, the high BET area of TiO2 NM-101 does not directly correlate with adverse effects. Although it has a high surface area (a physical feature), its chemical reactive profile counterpart does not run in parallel. The number of surface reactive sites is low, and their reactivity is moderate. TiO2 NM-101 is a relatively safe ENM, with no cytotoxicity for cell viability in immune, hepatic, reproductive and pulmonary cell lines, such as A549, HepG2, HK-2 or C3A. There are no toxicological data for TiO2-DT51, but the lower number and reactivity of its sites predict that DT51 would be safer than NM-101. Fe3O4, classified as low redox reactive via DTT OxTOF, is described as a safe ENM in terms of in vitro evaluation in the literature.99
An in chemico classification of ENMs can thus be proposed based on methanol chemisorption, reactivity of surface sites and DTT oxidative turnover frequency that may correlate with in vitro toxicity site-based dose metrics: CuO-SA ≈ CuFe2O4-SA > Co3O4-SA ≈ ZnO NM-110 ≫ CeO2 NM-211 ≥ Fe3O4-SA ≈ TiO2 NM-110.
4.3 Reactive surface site-based dose metrics
Recently, some studies emphasized the critical importance of adopting dose metrics that reflect the relevance of surface and particle number when assessing the nanotoxicity of ENMs, as traditional mass-based dose metrics are insufficient for evaluating the unique toxicological responses of nanoscale particles.148,149 These studies collectively underscore the need for more accurate dose metrics to assess the potential risks associated with ENMs. Due to the assumption that not all physical areas are equally populated by equally reactive sites, the reactive site concentration is proposed as a tool to better quantify the ENM exposition. For example, TiO2 NM-101 has a 4 times higher specific number of reactive sites than CeO2 NM-211 (2.8 vs. 0.7 mmol g−1) but also a 3 times higher surface area, so the reactive sites surface density is only slightly higher for the titania (7 vs. 6 sites per nm2), and therefore the comparison is similar to that of DTT OxTOF and OParea: TiO2 NM-101 with 3–4 times less oxidative potential than CeO2 NM-211. A different impression is provided by OPmass. Hence, because mass or physical surface area does not provide site-relevant dose metrics, the number of reactive sites connects with reactivity-triggered effects. This may serve as a new possible dose metric for assessing exposure to nanomaterials. The differences are greater when TiO2 NM-101 is compared to CuO-SA, with a very low specific surface area, and thus high reactive site surface density. These are much more reactive than those of titania and ceria, as observed by DTT OxTOF, but not as much as OParea indicates. Moreover, dose metrics based on reactive sites underscore that CuO-SA, with a dose of sites of 4 μmol L−1, can produce a significant adverse effect in A549, while 280 μmol L−1 of titania sites did not significantly decrease cell viability.
5 Conclusions and outlook
Reactive-based nanotoxicity is primarily governed by the surface chemistry of engineered nanomaterials, making catalysis science principles highly relevant to describing the reactive nature of ENMs. Our study's findings are as follows. 1) The specific surface area does not reliably correlate with nanomaterial reactivity, necessitating consideration of surface site quantity, nature, and reactivity for categorization and site-specific dosing. 2) To address this reactive categorization and the specific-dosing approach, a new approach methodology that quantifies and describes reactive surface sites by chemisorption and reaction tests using probe molecules. 3) Methanol offers triple benefits, namely, it quantifies surface sites through chemisorption, characterizes surface reactivity (acidic, basic, or redox) via temperature-programmed surface reaction, and overcomes limitations of liquid-phase reactions, such as possible ion release, pH-dependent agglomeration, effects of the dispersion protocol, or stability issues, providing insights into the primary reactivity of thermally stable nanomaterials, such as metal oxides. 4) Combining site quantification with physiologically relevant oxidation reactions, such as DTT, allows for calculating site-specific oxidative reactivity (OxTOF), aiding nanomaterial classification. CuO-SA, CuFe2O4-SA, and Co3O4-SA are the most oxidizing ENMs according to a higher in vitro toxicity, while less reactive ENMs do not produce adverse effects in in vitro models.
CuO-SA, CuFe2O4-SA, Co3O4-SA, Fe3O4-SA, ZnO NM-110, CeO2 NM-211 and two TiO2 ENMs (DT51 and NM-101) are ranked into three categories with statistically different reactivity based on DTT. This fundamental site-specific reactivity information is a relevant descriptor for grouping ENMs and, ultimately, for understanding nanotoxicity. Moreover, the behavior of a given material not only depends on its specific nanoform (e.g., crystallinity, size, band gap, solubility, hydrophobicity, surface charge, aspect ratio or shape) but also on its chemical reactive features, such as the number of surface reactive sites, their nature, their reactivity and their relative populations. In other cases, the adverse effect is not related to the reactivity but to other features, such as in multiwalled carbon nanotubes.150
This new methodology offers a complementary in chemico approach to unravel nanomaterial modes of action. To validate its effectiveness, further testing with additional reference and real-life ENMs as well as relevant and comparable toxicity information are essential. On a broader vista, the correlation with cellular assays will help establish molecular insight on the reactive basis of nanotoxicity. There are, however, significant structure, reactivity and toxicity data gaps to connect adverse effects with chemical reactivity. This approach aims to elucidate the specific pathways impacted by ENMs, highlighting their role in achieving a comprehensive understanding of nanomaterial toxicity and advocating safe-by-design principles. Filling these data gaps is part of the mission of nanoinformatics and nanosafety projects supported by platforms such as eNanoMapper. Mapping all reactive properties enables a more relevant grouping of nanomaterials because the acidic, basic and redox properties not only reflect their reactivity for adverse effects but also for interaction with species and molecules in biological systems.
Data availability
All data supporting the findings of this study are presented in the main article and the ESI.†
Author contributions
M. A. Bañares: conceptualization, original idea, supervision, writing – original draft preparation; V. Alcolea-Rodriguez: data curation, writing – original draft preparation, investigation, statistical analysis, experimental methodology; R. Portela: analysis, planning, supervision, writing – original draft preparation. V. Calvino-Casilda: reviewing and editing. All authors equally contributed to the writing.
Conflicts of interest
All authors have given approval to the final version of the manuscript. The authors declare no competing financial interest.
Acknowledgements
The authors acknowledge the financial support by the European Commission H2020 project NanoInformaTIX (GA 814426). JRC reference materials were supplied by Dr. Juan Riego Sintes at JRC-Ispra.
References
- A. Serrano-Lotina, R. Portela, P. Baeza, V. Alcolea-Rodriguez, M. Villarroel and P. Ávila, Zeta potential as a tool for functional materials development, Catal. Today, 2023, 423 DOI:10.1016/j.cattod.2022.08.004.
- G. Pacchioni, Oxygen Vacancy: The Invisible Agent on Oxide Surfaces, ChemPhysChem, 2003, 4, 1041–1047 CrossRef CAS PubMed.
- European Commission, Joint Research Centre, Rauscher, V. H. Kestens, K. Rasmussen, T. Linsinger and E. Stefaniak, Guidance on the implementation of the Commission Recommendation 2022/C 229/01 on the definition of nanomaterial, Publications Office of the European Union, 2023, DOI:10.2760/237496.
- K. Kasemets, A. Ivask, H. C. Dubourguier and A. Kahru, Toxicity of nanoparticles of ZnO, CuO and TiO2 to yeast Saccharomyces cerevisiae, Toxicol. In Vitro, 2009, 23, 1116–1122 CrossRef CAS PubMed.
- A. Nel, T. Xia, L. Mädler and N. Li, Toxic Potential of Materials at the Nanolevel, Science, 2006, 311, 622–627 CrossRef CAS PubMed.
- C. Buzea, I. I. Pacheco and K. Robbie, Nanomaterials and nanoparticles: Sources and toxicity, Biointerphases, 2007, 2, MR17–MR71 CrossRef PubMed.
- S. Talebian, T. Rodrigues, J. Das Neves, B. Sarmento, R. Langer and J. Conde, Facts and Figures on Materials Science and Nanotechnology Progress and Investment, ACS Nano, 2021, 15, 15940–15952 CrossRef CAS PubMed.
- I. Khan, K. Saeed and I. Khan, Nanoparticles: Properties, applications and toxicities, Arabian J. Chem., 2019, 12(7) DOI:10.1016/j.arabjc.2017.05.011.
- C. Kinnear, T. L. Moore, L. Rodriguez-Lorenzo, B. Rothen-Rutishauser and A. Petri-Fink, Form Follows Function: Nanoparticle Shape and Its Implications for Nanomedicine, Chem. Rev., 2017, 117, 11476–11521 CrossRef CAS PubMed.
- A. Haase and F. Klaessig, EU US Roadmap Nanoinformatics 2030, EU Nanosafety Cluster, 2018, DOI:10.5281/zenodo.1486012.
- N. Jeliazkova, M. D. Apostolova, C. Andreoli, F. Barone, A. Barrick and C. Battistelli, et al. Towards FAIR nanosafety data, Nat. Nanotechnol., 2021, 16, 644–654 CrossRef CAS PubMed.
- A. V. Singh, P. Laux, A. Luch, C. Sudrik, S. Wiehr and A. M. Wild, et al. Review of emerging concepts in nanotoxicology: opportunities and challenges for safer nanomaterial design, Toxicol. Mech. Methods, 2019, 29, 378–387 CrossRef CAS PubMed.
- A. Manke, L. Wang and Y. Rojanasakul, Mechanisms of Nanoparticle-Induced Oxidative Stress and Toxicity, BioMed Res. Int., 2013, 2013, 1–15 CrossRef PubMed.
- H. Choi, B. Choi, J. Han, H. E. Shin, W. Park and D. Kim, Reactive Oxygen Species Responsive Cleavable Hierarchical Metallic Supra-Nanostructure, Small, 2022, 18, 2202694 CrossRef CAS PubMed.
- C. Schwarz-Plaschg, A. Kallhoff and I. Eisenberger, Making Nanomaterials Safer by Design?, Nanoethics, 2017, 11, 277–281 CrossRef.
- N. Jeliazkova, E. Bleeker, R. Cross, A. Haase, G. Janer and W. Peijnenburg, et al. How can we justify grouping of nanoforms for hazard assessment? Concepts and tools to quantify similarity, NanoImpact, 2022, 25, 100366 CrossRef CAS PubMed.
- A. Kraegeloh, B. Suarez-Merino, T. Sluijters and C. Micheletti, Implementation of safe-by-design for nanomaterial development and safe innovation: Why we need a comprehensive approach, Nanomaterials, 2018, 8, 239 CrossRef PubMed.
- M. A. Bañares, A. Haase, L. Tran, V. Lobaskin, G. Oberdörster and R. Rallo, et al. CompNanoTox2015: novel perspectives from a European conference on computational nanotoxicology on predictive nanotoxicology, Nanotoxicology, 2017, 11, 839–845 CrossRef PubMed.
- V. Stone, S. Gottardo, E. A. J. Bleeker, H. Braakhuis, S. Dekkers and T. Fernandes, et al. A framework for grouping and read-across of nanomaterials-supporting innovation and risk assessment, Nano Today, 2020, 35, 1–15 CrossRef.
- R. Verdon, V. Stone, F. Murphy, E. Christopher, H. Johnston and S. Doak, et al. The application of existing genotoxicity methodologies for grouping of nanomaterials: towards an integrated approach to testing and assessment, Part. Fibre Toxicol., 2022, 19, 1–9 CrossRef PubMed.
- F. A. Murphy, H. J. Johnston, S. Dekkers, E. A. J. Bleeker, A. G. Oomen and T. F. Fernandes, et al. How to formulate hypotheses and IATAs to support grouping and read-across of nanoforms, ALTEX, 2023, 40, 125–140 Search PubMed.
- Safety Issues and Regulatory Challenges of Nanomaterials A, presented at A Symposium organized by four EU FP7 Projects and the JRC 3-4 May 2012, ed. J. R. Sintes, M. Blázquez, S. Moya and S. Vazquez, San Sebastián, Spain, 2012 Search PubMed.
- M. I. Setyawati, Z. Zhao and K. W. Ng, Transformation of Nanomaterials and Its Implications in Gut Nanotoxicology, Small, 2020, 16, 1–17 CrossRef PubMed.
- N. Shirokii, Y. Din, I. Petrov, Y. Seregin, S. Sirotenko and J. Razlivina, et al. Quantitative Prediction of Inorganic Nanomaterial Cellular Toxicity via Machine Learning, Small, 2023, 19, 1–7 CrossRef PubMed.
- H. Godwin, C. Nameth, D. Avery, L. L. Bergeson, D. Bernard and E. Beryt, et al. Nanomaterial Categorization for Assessing Risk Potential To Facilitate Regulatory Decision-Making, ACS Nano, 2015, 9, 3409–3417 CrossRef CAS PubMed.
- I. Lynch, C. Weiss and E. Valsami-Jones, A strategy for grouping of nanomaterials based on key physico-chemical descriptors as a basis for safer-by-design NMs, Nano Today, 2014, 9, 266–270 CrossRef CAS.
- I. Fischer, C. Milton and H. Wallace, Toxicity testing is evolving!, Toxicol. Res., 2020, 9, 67–80 CrossRef PubMed.
- S. Schmeisser, A. Miccoli, M. von Bergen, E. Berggren, A. Braeuning and W. Busch, et al. New approach methodologies in human regulatory toxicology – Not if, but how and when!, Environ. Int., 2023, 178, 108082 CrossRef CAS PubMed.
- S. H. Doak, M. J. D. Clift, A. Costa, C. Delmaar, I. Gosens and S. Halappanavar, et al. The Road to Achieving the European Commission's Chemicals Strategy for Nanomaterial Sustainability—A PATROLS Perspective on New Approach Methodologies, Small, 2022, 18, 2200231 CrossRef CAS PubMed.
- A. Bahl, C. Ibrahim, K. Plate, A. Haase, J. Dengjel and P. Nymark, et al. PROTEOMAS: a workflow enabling harmonized proteomic meta-analysis and proteomic signature mapping, Aust. J. Chem., 2023, 15, 1–17 Search PubMed.
- T. Padmore, C. Stark, L. A. Turkevich and J. A. Champion, Quantitative analysis of the role of fiber length on phagocytosis and inflammatory response by alveolar macrophages, Biochim. Biophys. Acta, Gen. Subj., 2017, 1861, 58–67 CrossRef CAS PubMed.
- M. S. P. Boyles, L. Young, D. M. Brown, L. MacCalman, H. Cowie and A. Moisala, et al. Multi-walled carbon nanotube induced frustrated phagocytosis, cytotoxicity and pro-inflammatory conditions in macrophages are length dependent and greater than that of asbestos, Toxicol. In Vitro, 2015, 29, 1513–1528 CrossRef CAS PubMed.
- A. Nel, T. Xia, H. Meng, X. Wang, S. Lin and Z. Ji, et al. Nanomaterial toxicity testing in the 21st century: Use of a predictive toxicological approach and high-throughput screening, Acc. Chem. Res., 2013, 46, 607–621 CrossRef CAS PubMed.
- S. Hsieh, D. Bello, D. F. Schmidt, A. K. Pal, A. Stella and J. A. Isaacs, et al. Mapping the Biological Oxidative Damage of Engineered Nanomaterials, Small, 2013, 9, 1853–1865 CrossRef CAS PubMed.
- S. Halappanavar, S. Van Den Brule, P. Nymark, L. Gaté, C. Seidel and S. Valentino, et al. Adverse outcome pathways as a tool for the design of testing strategies to support the safety assessment of emerging advanced materials at the nanoscale, Part. Fibre Toxicol., 2020, 17, 1–24 CrossRef PubMed.
- K. Gerloff, B. Landesmann, A. Worth, S. Munn, T. Palosaari and M. Whelan, The Adverse Outcome Pathway approach in nanotoxicology, Comput. Toxicol., 2017, 1, 3–11 CrossRef.
- S. Labib, A. Williams, C. L. Yauk, J. K. Nikota, H. Wallin and U. Vogel, et al. Nano-risk Science: Application of toxicogenomics in an adverse outcome pathway framework for risk assessment of multi-walled carbon nanotubes, Part. Fibre Toxicol., 2016, 13, 1–17 Search PubMed.
- M. T. D. Cronin, F. Bajot, S. J. Enoch, J. C. Madden, D. W. Roberts and J. Schwöbel, The in chemico-in silico interface: Challenges for integrating experimental and computational chemistry to identify toxicity, ATLA, Altern. Lab. Anim., 2009, 37, 513–521 CrossRef CAS PubMed.
- I. E. Wachs, Number of surface sites and turnover frequencies for oxide catalysts, J. Catal., 2022, 405, 462–472 CrossRef CAS.
- S. Lwin, Y. Li, A. I. Frenkel and I. E. Wachs, Nature of WOx Sites on SiO2 and Their Molecular Structure-Reactivity/Selectivity Relationships for Propylene Metathesis, ACS Catal., 2016, 6, 3061–3071 CrossRef CAS.
- J. M. Thomas and W. J. Thomas, Principles and Practice of Heterogeneous Catalysis, Wiley, VCH, 1997, p. 27 Search PubMed.
- L. E. Briand, A. M. Hirt and I. E. Wachs, Quantitative determination of the number of surface active sites and the turnover frequencies for methanol oxidation over metal oxide catalysts: Application to bulk metal molybdates and pure metal oxide catalysts, J. Catal., 2001, 202, 268–278 CrossRef CAS.
- D. Kulkarni and I. E. Wachs, Isopropanol oxidation by pure metal oxide catalysts: Number of active surface sites and turnover frequencies, Appl. Catal., A, 2002, 237, 121–137 CrossRef CAS.
- L. E. Briand, J. M. Jehng, L. Cornaglia, A. M. Hirt and I. E. Wachs, Quantitative determination of the number of surface active sites and the turnover frequency for methanol oxidation over bulk metal vanadates, Catal. Today, 2003, 78, 257–268 CrossRef CAS.
- B. C. Gates, Catalytic Chemistry, 1991 Search PubMed.
- J. M. Tatibouët, Methanol oxidation as a catalytic surface probe, Appl. Catal., A, 1997, 148, 213–252 CrossRef.
- J.-M. Jehng, I. E. Wachs and M. Ford, Temperature-Programmed (TP) Techniques, in Springer Handbook of Advanced Catalyst Characterization, Springer, 2023, ch. 45, pp. 1005–1029 Search PubMed.
- Y. Wu, F. Gao, H. Wang, L. Kovarik, B. Sudduth and Y. Wang, Probing Acid–Base Properties of Anatase TiO 2 Nanoparticles with Dominant {001} and {101} Facets Using Methanol Chemisorption and Surface Reactions, J. Phys. Chem. C, 2021, 125, 3988–4000 CrossRef CAS.
- J. M. Jehng, I. E. Wachs, G. S. Patience and Y. M. Dai, Experimental methods in chemical engineering: Temperature programmed surface reaction spectroscopy—TPSR, Can. J. Chem. Eng., 2021, 99, 423–434 CrossRef CAS.
- K. R. Daellenbach, G. Uzu, J. Jiang, L. E. Cassagnes, Z. Leni and A. Vlachou, et al. Sources of particulate-matter air pollution and its oxidative potential in Europe, Nature, 2020, 587, 414–419 CrossRef CAS PubMed.
- N. A. H. Janssen, A. Yang, M. Strak, M. Steenhof, B. Hellack and M. E. Gerlofs-Nijland, et al. Oxidative potential of particulate matter collected at sites with different source characteristics, Sci. Total Environ., 2014, 472, 572–581 CrossRef CAS PubMed.
- D. Gao, S. Ripley, S. Weichenthal and K. J. Godri Pollitt, Ambient particulate matter oxidative potential: Chemical determinants, associated health effects, and strategies for risk management, Free Radical Biol. Med., 2020, 151, 7–25 CrossRef CAS PubMed.
- D. Breznan, N. Nazemof, F. Kunc, M. Hill, D. Vladisavljevic and J. Gomes, et al. Acellular oxidative potential assay for screening of amorphous silica nanoparticles, Analyst, 2020, 145, 4867–4879 RSC.
- L. Rao, L. Zhang, X. Wang, T. Xie, S. Zhou and S. Lu, et al. Oxidative potential induced by ambient particulate matters with acellular assays: A review, Processes, 2020, 8, 1–21 CrossRef.
- A. L. Kramer, S. Dorn, A. Perez, C. Roper, I. A. Titaley and K. Cayton, et al. Assessing the oxidative potential of PAHs in ambient PM2.5 using the DTT consumption assay, Environ. Pollut., 2021, 285, 117411 CrossRef CAS PubMed.
- J. Jeevanandam, A. Barhoum, Y. S. Chan, A. Dufresne and M. K. Danquah, Review on nanoparticles and nanostructured materials: History, sources, toxicity and regulations, Beilstein J. Nanotechnol., 2018, 9, 1050–1074 CrossRef CAS PubMed.
- H. Yang, C. Liu, D. Yang, H. Zhang and Z. Xi, Comparative study of cytotoxicity, oxidative stress and genotoxicity induced by four typical nanomaterials: The role of particle size, shape and composition, J. Appl. Toxicol., 2009, 29, 69–78 CrossRef CAS PubMed.
- M. I. Setyawati, C. Y. Tay and D. T. Leong, Effect of zinc oxide nanomaterials-induced oxidative stress on the p53 pathway, Biomaterials, 2013, 34, 10133–10142 CrossRef CAS PubMed.
- J. Čapek and T. Roušar, Detection of oxidative stress induced by nanomaterials in cells—the roles of reactive oxygen species and glutathione, Molecules, 2021, 26, 4710 CrossRef PubMed.
- R. P. Mendoza and J. M. Brown, Engineered nanomaterials and oxidative stress: Current understanding and future challenges, Curr. Opin. Toxicol., 2019, 13, 74–80 CrossRef PubMed.
- B. Crobeddu, L. Aragao-Santiago, L. C. Bui, S. Boland and A. B. Squiban, Oxidative potential of particulate matter 2.5 as predictive indicator of cellular stress, Environ. Pollut., 2017, 230, 125–133 CrossRef CAS PubMed.
- H. Jiang, C. M. Sabbir Ahmed, A. Canchola, J. Y. Chen and Y. H. Lin, Use of dithiothreitol assay to evaluate the oxidative potential of atmospheric aerosols, Atmosphere, 2019, 10, 1–21 Search PubMed.
- J. Øvrevik, Oxidative Potential Versus Biological Effects: A Review on the Relevance of Cell-Free/Abiotic Assays as Predictors of Toxicity from Airborne Particulate Matter, Int. J. Mol. Sci., 2019, 20, 4772 CrossRef PubMed.
- B. Crobeddu, I. Baudrimont, J. Deweirdt, J. Sciare, A. Badel and A. C. Camproux, et al. Lung Antioxidant Depletion: A Predictive Indicator of Cellular Stress Induced by Ambient Fine Particles, Environ. Sci. Technol., 2020, 54, 2360–2369 CrossRef CAS PubMed.
- J. J. Sauvain, S. Deslarzes and M. Riediker, Nanoparticle reactivity toward dithiothreitol, Nanotoxicology, 2008, 2, 121–129 CrossRef CAS.
- J. J. Sauvain, M. J. Rossi and M. Riediker, Comparison of three acellular tests for assessing the oxidation potential of nanomaterials, Aerosol Sci. Technol., 2013, 47, 218–227 CrossRef CAS.
- L. E. Briand, A. M. Hirt and I. E. Wachs, Quantitative determination of the number of surface active sites and the turnover frequencies for methanol oxidation over metal oxide catalysts: Application to bulk metal molybdates and pure metal oxide catalysts, J. Catal., 2001, 202, 268–278 CrossRef CAS.
- I. E. Wachs, Number of surface sites and turnover frequencies for oxide catalysts, J. Catal., 2022, 405, 462–472 CrossRef CAS.
- K. A. Jensen, NanoGenoTox. WP4, Deliverable 3: Final protocol for producing suitable MN exposure media, The generic NANOGENOTOX dispersion protocol, Standard Operation Procedure (SOP) and background documentation, 2011.
- JRC Nanomaterials Repository, List of Representative Nanomaterials, 2016.
- K. Rasmussen, J. Mast, P. De Temmerman, E. Verleysen, N. Waegeneers and F. Van Steen, et al. Titanium Dioxide, NM-100, NM-101, NM-102, NM-103, NM-104, NM-105: Characterisation and Physico-Chemical Properties, 2014, DOI:10.2788/79554.
- C. Singh, Europäische Kommission Gemeinsame Forschungsstelle Institute for Health and Consumer Protection, Cerium Dioxide NM-211, NM-212, NM-213,
characterisation and test item preparation JRC repository: NM-series of representative manufactured nanomaterials, 2014, DOI:10.2788/80203.
- C. Singh, S. Friedrichs, M. Levin, R. Birkedal, K. A. Jensen and G. Pojana, et al. Zinc Oxide NM-110, NM-111, NM-112, NM-113 Characterisation and Test Item Preparation, 2011, DOI:10.2787/55008.
- J. Martín-Gómez, J. Hidalgo-Carrillo, V. Montes, R. C. Estévez-Toledano, J. C. Escamilla and A. Marinas, et al. EPR and CV studies cast further light on the origin of the enhanced hydrogen production through glycerol photoreforming on CuO:TiO2 physical mixtures, J. Environ. Chem. Eng., 2021, 9, 105336 CrossRef.
- N. Kochev, N. Jeliazkova, V. Paskaleva, G. Tancheva, L. Iliev and P. Ritchie, et al. Your spreadsheets can be fair: A tool and fairification workflow for the enanomapper database, Nanomaterials, 2020, 10, 1–23 CrossRef PubMed.
- J. Hastings, N. Jeliazkova, G. Owen, G. Tsiliki, C. R. Munteanu and C. Steinbeck, et al. eNanoMapper: Harnessing ontologies to enable data integration for nanomaterial risk assessment, J. Biomed. Semant., 2015, 6, 1–15 CrossRef PubMed.
- E. Moschini, G. Colombo, G. Chirico, G. Capitani, I. Dalle-Donne and P. Mantecca, Biological mechanism of cell oxidative stress and death during short-term exposure to nano CuO, Sci. Rep., 2023, 13, 2326 CrossRef CAS PubMed.
- E. Moschini, M. Gualtieri, M. Colombo, U. Fascio, M. Camatini and P. Mantecca, The modality of cell-particle interactions drives the toxicity of nanosized CuO and TiO2 in human alveolar epithelial cells, Toxicol. Lett., 2013, 222, 102–116 CrossRef CAS PubMed.
- A. Semisch, J. Ohle, B. Witt and A. Hartwig, Cytotoxicity and genotoxicity of nano- and microparticulate copper oxide: role of solubility and intracellular bioavailability, Part. Fibre Toxicol., 2014, 11, 10 CrossRef PubMed.
- J.-T. Kwon, Y. Kim, S. Choi, B. Yoon, H.-S. Kim and I. Shim, et al. Pulmonary Toxicity and Proteomic Analysis in Bronchoalveolar Lavage Fluids and Lungs of Rats Exposed to Copper Oxide Nanoparticles, Int. J. Mol. Sci., 2022, 23, 13265 CrossRef CAS PubMed.
- I. Perelshtein, A. Lipovsky, N. Perkas, A. Gedanken, E. Moschini and P. Mantecca, The influence of the crystalline nature of nano-metal oxides on their antibacterial and toxicity properties, Nano Res., 2015, 8, 695–707 CrossRef CAS.
- I. Mouche, L. Malésic and O. Gillardeaux, FETAX assay for evaluation of developmental toxicity, Methods Mol. Biol., 2017, 1641, 311–324 CrossRef CAS PubMed.
- J. Ahmad, H. A. Alhadlaq, A. Alshamsan, M. A. Siddiqui, Q. Saquib and S. T. Khan, et al. Differential cytotoxicity of copper ferrite nanoparticles in different human cells, J. Appl. Toxicol., 2016, 36, 1284–1293 CrossRef CAS PubMed.
- A. Precupas, D. Gheorghe, A. Botea-Petcu, A. R. Leonties, R. Sandu and V. T. Popa, et al. Thermodynamic Parameters at Bio-Nano Interface and Nanomaterial Toxicity: A Case Study on BSA Interaction with ZnO, SiO2, and TiO2, Chem. Res. Toxicol., 2020, 33, 2054–2071 Search PubMed.
- N. El Yamani, A. R. Collins, E. Rundén-Pran, L. M. Fjellsbø, S. Shaposhnikov and S. Zienolddiny, et al. In vitro genotoxicity testing of four reference metal nanomaterials, titanium dioxide, zinc oxide, cerium oxide and silver: Towards reliable hazard assessment, Mutagenesis, 2017, 32, 117–126 CrossRef CAS PubMed.
- L. Farcal, F. T. Andón, L. Di Cristo, B. M. Rotoli, O. Bussolati and E. Bergamaschi, et al. Comprehensive in vitro toxicity testing of a panel of representative oxide nanomaterials: First steps towards an intelligent testing strategy, PLoS One, 2015, 10, 1–34 CrossRef PubMed.
- A. Kermanizadeh, G. Pojana, B. K. Gaiser, R. Birkedal, D. Bilaničová and H. Wallin, et al. In vitro assessment of engineered nanomaterials using a hepatocyte cell line: Cytotoxicity, pro-inflammatory cytokines and functional markers, Nanotoxicology, 2013, 7, 301–313 CrossRef CAS PubMed.
- M. D. Driessen, S. Mues, A. Vennemann, B. Hellack, A. Bannuscher and V. Vimalakanthan, et al. Proteomic analysis of protein carbonylation: A useful tool to unravel nanoparticle toxicity mechanisms, Part. Fibre Toxicol., 2015, 12, 1–18 CrossRef PubMed.
- Y. Cao, M. Roursgaard, A. Kermanizadeh, S. Loft and P. Møller, Synergistic effects of zinc oxide nanoparticles and fatty acids on toxicity to caco-2 cells, Int. J. Toxicol., 2015, 34, 67–76 CrossRef CAS PubMed.
- Y. Gu, S. Cheng, G. Chen, Y. Shen, X. Li and Q. Jiang, et al. The effects of endoplasmic reticulum stress inducer thapsigargin on the toxicity of ZnO or TiO2 nanoparticles to human endothelial cells, Toxicol. Mech. Methods, 2017, 27, 191–200 CrossRef CAS PubMed.
- W. Thongkam, K. Gerloff, D. van Berlo, C. Albrecht and R. P. F. Schins, Oxidant generation, DNA damage and cytotoxicity by a panel of engineered nanomaterials in three different human epithelial cell lines, Mutagenesis, 2017, 32, 105–115 CrossRef CAS PubMed.
- A. Zijno, D. Cavallo, G. Di Felice, J. Ponti, B. Barletta and C. Butteroni, et al. Use of a common European approach for nanomaterials' testing to support regulation: a case study on titanium and silicon dioxide representative nanomaterials, J. Appl. Toxicol., 2020, 40, 1511–1525 CrossRef CAS PubMed.
- A. E. Nel, L. Mädler, D. Velegol, T. Xia, E. M. V. Hoek and P. Somasundaran, et al. Understanding biophysicochemical interactions at the nano-bio interface, Nat. Mater., 2009, 8, 543–557 CrossRef CAS PubMed.
- T. Xia, M. Kovochich, M. Liong, L. Mädler, B. Gilbert and H. Shi, et al. Comparison of the mechanism of toxicity of zinc oxide and cerium oxide nanoparticles based on dissolution and oxidative stress properties, ACS Nano, 2008, 2, 2121–2134 CrossRef CAS PubMed.
- U. G. Sauer, S. Vogel, A. Aumann, A. Hess, S. N. Kolle and L. Ma-Hock, et al. Applicability of rat precision-cut lung slices in evaluating nanomaterial cytotoxicity, apoptosis, oxidative stress, and inflammation, Toxicol. Appl. Pharmacol., 2014, 276, 1–20 CrossRef CAS PubMed.
- A. Srinivas, P. J. Rao, G. Selvam, P. B. Murthy and P. N. Reddy, Acute inhalation toxicity of cerium oxide nanoparticles in rats, Toxicol. Lett., 2011, 205, 105–115 CrossRef CAS PubMed.
- P. Demokritou, S. Gass, G. Pyrgiotakis, J. M. Cohen, W. Goldsmith and W. McKinney, et al. An in vivo and in vitro toxicological characterisation of realistic nanoscale CeO2 inhalation exposures, Nanotoxicology, 2013, 7, 1338–1350 CrossRef CAS PubMed.
- M. Wiemann, A. Vennemann, U. G. Sauer, K. Wiench, L. Ma-Hock and R. Landsiedel, An in vitro alveolar macrophage assay for predicting the short-term inhalation toxicity of nanomaterials, J. Nanobiotechnol., 2016, 14, 16 CrossRef PubMed.
- A. Solorio-Rodríguez, V. Escamilla-Rivera, M. Uribe-Ramírez, S. González-Pozos, J. Hernández-Soto and L. Rafael-Vázquez, et al. In vitro cytotoxicity study of superparamagnetic iron oxide and silica nanoparticles on pneumocyte organelles, Toxicol. In Vitro, 2021, 72, 105071 CrossRef PubMed.
- D. Cavallo, A. Ciervo, A. M. Fresegna, R. Maiello, P. Tassone and G. Buresti, et al. Investigation on cobalt-oxide nanoparticles cyto-genotoxicity and inflammatory response in two types of respiratory cells, J. Appl. Toxicol., 2015, 35, 1102–1113 CrossRef CAS PubMed.
- O. Lugun, J. Singh, R. Singh Thakur and A. K. Pandey, Cobalt oxide (Co3O4) nanoparticles induced genotoxicity in Chinese hamster lung fibroblast (V79) cells through modulation of reactive oxygen species, Mutagenesis, 2022, 37, 44–59 CrossRef CAS PubMed.
- A. Marucco, E. Carella and I. Fenoglio, A comparative study on the efficacy of different probes to predict the photo-activity of nano-titanium dioxide toward biomolecules, RSC Adv., 2015, 5, 89559–89568 RSC.
- A. Davydov, Molecular Spectroscopy of Oxide Catalyst Surfaces, Wiley, 2003, DOI:10.1002/0470867981.
- S. E. Collins, L. E. Briand, L. A. Gambaro, M. A. Baltanás and A. L. Bonivardi, Adsorption and Decomposition of Methanol on Gallium Oxide Polymorphs, J. Phys. Chem. C, 2008, 112, 14988–15000 CrossRef CAS.
- P. Bazin, S. Thomas, O. Marie and M. Daturi, New insights into the methanol oxidation mechanism over Au/CeO2 catalyst through complementary kinetic and FTIR operando SSITKA approaches, Catal. Today, 2012, 182, 3–11 CrossRef CAS.
- A. Bahl, B. Hellack, M. Wiemann, A. Giusti, K. Werle and A. Haase, et al. Nanomaterial categorization by surface reactivity: A case study comparing 35 materials with four different test methods, NanoImpact, 2020, 19, 100234 CrossRef.
- B. Hellack, C. Nickel and R. P. F. Schins, Oxidative potential of silver nanoparticles measured by electron paramagnetic resonance spectroscopy, J. Nanopart. Res., 2017, 19, 404 CrossRef.
- Z. Wu, M. Li, J. Howe, H. M. Meyer and S. H. Overbury, Probing defect sites on CeO2 nanocrystals with well-defined surface planes by raman spectroscopy and O2 adsorption, Langmuir, 2010, 26, 16595–16606 CrossRef CAS PubMed.
- V. V. Pushkarev, V. I. Kovalchuk and J. L. D'Itri, Probing defect sites on the CeO2 surface with dioxygen, J. Phys. Chem. B, 2004, 108, 5341–5348 CrossRef CAS.
- Q. Wang, Y. Li, A. Serrano-Lotina, W. Han, R. Portela and R. Wang, et al. Operando Investigation of Toluene Oxidation over 1D Pt@CeO 2 Derived from Pt Cluster-Containing MOF, J. Am. Chem. Soc., 2021, 143, 196–205 CrossRef CAS PubMed.
- A. J. Wyrwoll, P. Lautenschläger, A. Bach, B. Hellack, A. Dybowska and T. A. J. Kuhlbusch, et al. Size matters – The phototoxicity of TiO2 nanomaterials, Environ. Pollut., 2016, 208, 859–867 CrossRef CAS PubMed.
- A. Marucco, E. Carella and I. Fenoglio, A comparative study on the efficacy of different probes to predict the photo-activity of nano-titanium dioxide toward biomolecules, RSC Adv., 2015, 5, 89559–89568 RSC.
- H. Zhang, Z. Ji, T. Xia, H. Meng, C. Low-Kam and R. Liu, et al. Use of Metal Oxide Nanoparticle Band Gap To Develop a Predictive Paradigm for Oxidative Stress and Acute Pulmonary Inflammation, ACS Nano, 2012, 6, 4349–4368 CrossRef CAS PubMed.
- M. Arakha, J. Roy, P. S. Nayak, B. Mallick and S. Jha, Zinc oxide nanoparticle energy band gap reduction triggers the oxidative stress resulting into autophagy-mediated apoptotic cell death, Free Radical Biol. Med., 2017, 110, 42–53 CrossRef CAS PubMed.
- A. Paruthi, J. M. Brown, E. Panda, A. R. S. Gautam, S. Singh and S. K. Misra, Transformation in band energetics of CuO nanoparticles as a function of solubility and its impact on cellular response, NanoImpact, 2021, 22, 100324 CrossRef CAS PubMed.
- Y. A. Çetin, B. Martorell, F. Serratosa, N. Aguilera-Porta and M. Calatayud, Analyzing the TiO 2 surface reactivity based on oxygen vacancies computed by DFT and DFTB methods, J. Phys.: Condens. Matter, 2022, 34, 314004 CrossRef PubMed.
- H.-H. Chou, S. Y.-H. Liou and M. Calatayud, Modelling rutile TiO2nanorod growth preferences: A density functional theory study, Catal. Today, 2020, 356, 49–55 CrossRef CAS.
- G. Mancardi, A. Mikolajczyk, V. K. Annapoorani, A. Bahl, K. Blekos and J. Burk, et al. A computational view on nanomaterial intrinsic and extrinsic features for nanosafety and sustainability, Mater. Today, 2023, 67, 344–370 CrossRef.
- V. I. Dumit, A. Ammar, M. I. Bakker, M. A. Bañares, C. Bossa and A. Costa, et al. From principles to reality. FAIR implementation in the nanosafety community, Nano Today, 2023, 51, 101923 CrossRef.
- E. Moschini, M. Gualtieri, M. Colombo, U. Fascio, M. Camatini and P. Mantecca, The modality of cell-particle interactions drives the toxicity of nanosized CuO and TiO2 in human alveolar epithelial cells, Toxicol. Lett., 2013, 222, 102–116 CrossRef CAS PubMed.
- F. C. Simeone and A. L. Costa, Assessment of cytotoxicity of metal oxide nanoparticles on the basis of fundamental physical-chemical parameters: A robust approach to grouping, Environ. Sci.: Nano, 2019, 6, 3102–3112 RSC.
- J. J. Reinosa, M. M. Rojo, A. del Campo, M. Martín-González and J. F. Fernández, Highly Efficient Antimicrobial Ceramics Based on Electrically Charged Interfaces, ACS Appl. Mater. Interfaces, 2019, 11, 39254–39262 CrossRef CAS PubMed.
- L. Zuo and D. Wijegunawardana, Redox Role of ROS and Inflammation in Pulmonary Diseases, in Advances in Experimental Medicine and Biology, 2021, pp. 187–204 Search PubMed.
- M. Cuillel, M. Chevallet, P. Charbonnier, C. Fauquant, I. Pignot-Paintrand and J. Arnaud, et al. Interference of CuO nanoparticles with metal homeostasis in hepatocytes under sub-toxic conditions, Nanoscale, 2014, 6, 1707–1715 RSC.
- P. Mantecca, E. Moschini, G. Chirico and G. Capitani, Surface bio-interactions stand at the base of the short-term nano CuO-induced cell oxidative stress: insights for a safe(r)-by-design approach, 2022, ResearchSquare, 2022, preprint, DOI:10.21203/rs.3.rs-1306935/v2.
- A. Semisch, J. Ohle, B. Witt and A. Hartwig, Cytotoxicity and genotoxicity of nano- and microparticulate copper oxide: Role of solubility and intracellular bioavailability, Part. Fibre Toxicol., 2014, 11 DOI:10.1186/1743-8977-11-10.
- B. M. Strauch, R. K. Niemand, N. L. Winkelbeiner and A. Hartwig, Comparison between micro- and nanosized copper oxide and water soluble copper chloride: Interrelationship between intracellular copper concentrations, oxidative stress and DNA damage response in human lung cells, Part. Fibre Toxicol., 2017, 14 DOI:10.1186/s12989-017-0209-1.
- S. Diabaté, L. Armand, S. Murugadoss, M. Dilger, S. Fritsch-Decker and C. Schlager, et al. Air–liquid interface exposure of lung epithelial cells to low doses of nanoparticles to assess pulmonary adverse effects, Nanomaterials, 2021, 11, 1–23 Search PubMed.
- Z. Wu, M. Li, D. R. Mullins and S. H. Overbury, Probing the surface sites of CeO2 nanocrystals with well-defined surface planes via methanol adsorption and desorption, ACS Catal., 2012, 2, 2224–2234 CrossRef CAS.
- Z. Wu, M. Li, J. Howe, H. M. Meyer and S. H. Overbury, Probing defect sites on CeO2 nanocrystals with well-defined surface planes by raman spectroscopy and O2 adsorption, Langmuir, 2010, 26, 16595–16606 CrossRef CAS PubMed.
- A. Trovarelli, Catalysis by Ceria and Related Materials, 2002, vol. 2, DOI:10.1142/P249.
- L. Tran, M. A. Bañares and R. Rallo, Modelling the Toxicity of Nanoparticles, Springer International Publishing: Cham, 2017, DOI:10.1007/978-3-319-47754-1.
- M. Ziemba, C. Schilling, M. V. Ganduglia-Pirovano and C. Hess, Toward an Atomic-Level Understanding of Ceria-Based Catalysts: When Experiment and Theory Go Hand in Hand, Acc. Chem. Res., 2021, 54, 2884–2893 CrossRef CAS PubMed.
- C. Schilling, A. Hofmann, C. Hess and M. V. Ganduglia-Pirovano, Raman Spectra of Polycrystalline CeO2: A Density Functional Theory Study, J. Phys. Chem. C, 2017, 121, 20834–20849 CrossRef CAS.
- M. Ziemba, M. V. Ganduglia-Pirovano and C. Hess, Elucidating the Oxygen Storage-Release Dynamics in Ceria Nanorods by Combined Multi-Wavelength Raman Spectroscopy and DFT, J. Phys. Chem. Lett., 2020, 11, 8554–8559 CrossRef CAS PubMed.
- S. Mittal and A. K. Pandey, Cerium Oxide Nanoparticles Induced Toxicity in Human Lung Cells: Role of ROS Mediated DNA Damage and Apoptosis, BioMed Res. Int., 2014, 2014, 1–14 CrossRef PubMed.
- J. Keller, W. Wohlleben, L. Ma-Hock, V. Strauss, S. Gröters and K. Küttler, et al. Time course of lung retention and toxicity of inhaled particles: short-term exposure to nano-Ceria, Arch. Toxicol., 2014, 88, 2033–2059 CrossRef CAS PubMed.
- P. Jiang, L. Zhang, X. Liu, C. Ye, P. Zhu and T. Tan, et al. Tuning oxidant and antioxidant activities of ceria by anchoring copper single-site for antibacterial application, Nat. Commun., 2024, 15, 1–15 Search PubMed.
- F. Fragou, A. Zindrou, Y. Deligiannakis and M. Louloudi, Engineering of Oxygen-Deficient Nano-CeO2−x with Tunable Biocidal and Antioxidant Activity, ACS Appl. Nano Mater., 2024, 7, 10552–10564 CrossRef CAS.
- Q. Wang, K. L. Yeung and M. A. Bañares, Operando Raman-online FTIR investigation of ceria, vanadia/ceria and gold/ceria catalysts for toluene elimination, J. Catal., 2018, 364, 80–88 CrossRef CAS.
- Q. Wang, Y. Li, A. Serrano-Lotina, W. Han, R. Portela and R. Wang, et al. Operando Investigation of Toluene Oxidation over 1D Pt@CeO2Derived from Pt Cluster-Containing MOF, J. Am. Chem. Soc., 2021, 143, 196–205 CrossRef CAS PubMed.
- E. G. Heckert, S. Seal and W. T. Self, Fenton-like reaction catalyzed by the rare earth inner transition metal cerium, Environ. Sci. Technol., 2008, 42, 5014–5019 CrossRef CAS PubMed.
- Y. Xue, Q. Luan, D. Yang, X. Yao and K. Zhou, Direct evidence for hydroxyl radical scavenging activity of cerium oxide nanoparticles, J. Phys. Chem. C, 2011, 115, 4433–4438 CrossRef CAS.
- S. S. Hardas, R. Sultana, G. Warrier, M. Dan, R. L. Florence and Wu, Rat brain pro-oxidant effects of peripherally administered 5 nm ceria 30 days after exposure, Neurotoxicology, 2012, 33(5), 1147–1155 CrossRef CAS PubMed.
- P. Zhang, Y. Ma, Z. Zhang, X. He, J. Zhang and Z. Guo, et al. Biotransformation of ceria nanoparticles in cucumber plants, ACS Nano, 2012, 6, 9943–9950 CrossRef CAS PubMed.
- X. Shi, Q. Liu, Z. Gong, Z. Jin, H. Guo and X. Wen, et al. Vacancy-rich Fe-Ce mixed oxides as nanoenzymes for efficient antioxidant activity in vitro, Appl. Surf. Sci., 2024, 664, 160256 CrossRef CAS.
- M. D. Driessen, S. Mues, A. Vennemann, B. Hellack, A. Bannuscher and V. Vimalakanthan, et al. Proteomic analysis of protein carbonylation: A useful tool to unravel nanoparticle toxicity mechanisms, Part. Fibre Toxicol., 2015, 12, 1–18 CrossRef PubMed.
- J. Cohen, Z. Ji, T. Xia and P. Demokritou, An integrated approach for the in vitro dosimetry of engineered nanomaterials: Relevant in vitro dose (RID) functions Background (1 of 2), Part. Fibre Toxicol., 2014, 11, 1–12 CrossRef PubMed.
- M. Hull, A. J. Kennedy, C. Detzel, P. Vikesland and M. A. Chappell, Moving beyond mass: The unmet need to consider dose metrics in environmental nanotoxicology studies, Environ. Sci. Technol., 2012, 46, 10881–10882 CrossRef CAS PubMed.
- I. Fenoglio, M. Tomatis, D. Lison, J. Muller, A. Fonseca and J. B. Nagy, et al. Reactivity of carbon nanotubes: Free radical generation or scavenging activity?, Free Radical Biol. Med., 2006, 40, 1227–1233 CrossRef CAS PubMed.
|
This journal is © The Royal Society of Chemistry 2024 |