DOI:
10.1039/D2MA00369D
(Paper)
Mater. Adv., 2022,
3, 6007-6018
Controlled synthesis of SPION@SiO2 nanoparticles using design of experiments†
Received
31st March 2022
, Accepted 24th May 2022
First published on 31st May 2022
Abstract
The synthesis of single-core superparamagnetic iron oxide nanoparticles (SPIONs) coated with a silica shell of controlled thickness remains a challenge, due to the dependence on a multitude of experimental variables. Herein, we utilise design of experiment (DoE) to study the formation of SPION@SiO2 nanoparticles (NPs) via reverse microemulsion. Using a 33 full factorial design, the influence of reactant concentration of tetraethyl orthosilicate (TEOS) and ammonium hydroxide (NH4OH), as well as the number of fractionated additions of TEOS on the silica shell was investigated with the aim of minimising polydispersity and increasing the population of SPION@SiO2 NPs formed. This investigation facilitated a reproducible and controlled approach for the high yield synthesis of SPION@SiO2 NPs with uniform silica shell thickness. Application of a multiple linear regression analysis established a relationship between the applied experimental variables and the resulting silica shell thickness. These experimental variables were similarly found to dictate the monodispersity of the SPION@SiO2 NPs formed. The overall population of single-core@shell particles was dependent on the interaction between the number of moles of TEOS and NH4OH, with no influence from the number of fractionated additions of TEOS. This work demonstrates the complexity of the preparative method and produces an accessible and flexible synthetic model to achieve monodisperse SPION@SiO2 NPs with controllable shell thickness.
1 Introduction
Superparamagnetic iron oxide nanoparticles (SPIONs) have been extensively researched due to their unique magnetic properties when compared to bulk iron oxide including: superparamagnetic behaviour with a large magnetic susceptibility; low Curie temperature; and high coercivity.1,2 SPIONs are inverse spinel type iron oxide nanoparticles (NPs) including Fe3O4 (magnetite) and γ-Fe2O3 (maghemite) and are typically below 20 nm in size.3,4 The distinctive properties of SPIONs have made them useful for a range of applications in catalysis, purification, and biomedicine.5–11 Most notably, these materials have shown excellent potential in biomedical diagnostic and therapeutic applications including, but not limited to, point-of-care diagnostics, magnetic resonance imaging contrast agents, hyperthermic cancer treatments, and targeted drug delivery.12–19
Synthetic pathways to SPIONs are diverse, including co-precipitation, hydrothermal or thermal decomposition, solvothermal, and sol-gel reactions, with choice of reaction depending on the desired particle size, shape, hydrophilicity/phobicity and surface functionalities.20–24 As the magnetic properties of SPIONs are dependent on their size and shape, control of such characteristics, as well as uniformity of SPIONs, is critical. This is particularly imperative for biomedical applications, where highly polydisperse SPIONs can result in variable net magnetisation, leading to unreliable and poorly reproducible diagnostic and therapeutic capabilities.25,26 Co-precipitation of Fe2+/Fe3+ salts is one of the most popular methods due to its high yield and low cost of manufacture; however, this typically produces SPIONs that are highly polydisperse, often with poor control over particle morphology, crystallinity, and aggregation.6,22,26 In contrast, thermal decomposition has greater control over size and morphology, generating monodisperse NPs with high crystallinity. In this approach, an organic-iron precursor is heated in situ with an amphiphilic surfactant or ligand such as oleic acid, a hydrophobic fatty acid, forming hydrophobic coated SPIONs which typically avoid aggregation.23,27–30
To obtain biocompatible SPIONs that are colloidally and physically stable, it is necessary to coat and functionalise the nanoparticle surface. Without this coating, the poor colloidal stability of SPIONs often leads to agglomeration under physiological conditions, leading to increased toxicity via red blood cell damage and haemolysis.14,31,32 Furthermore, uncoated SPIONs are susceptible to oxidation, which can contribute to changes in magnetic properties and chemical behaviour.2,8,33 There are various coating strategies to achieve this, including both covalent and non-covalent synthetic methods such as: surface stabilisation with citric acid; capping with oleic acid; adsorption of polymers; or coating with inorganic material, such as silica (SiO2).14,15,29,34 The latter is of particular interest as SiO2 coated SPIONs are able to undergo subsequent surface modification with a variety of silanes or ligands due to readily modifiable silica chemistry.29,35–43 This provides the opportunity for functionalisation with an array of molecules such as fluorescent dyes, bio-compatible, biological or targeting ligands.36,38,44–46 There are several coating routes to attain SPION@SiO2 core@shell NPs, with the option of either a non-porous or a mesoporous silica shell, the latter providing an opportunity to utilise the pores for cargo storage and release.35,47,48
Both sol-gel and reverse microemulsion routes are popular methods for the synthesis of non-porous SPION@SiO2 NPs. The sol-gel method typically uses hydrophilic, small molecule-stabilised, magnetic nanoparticles which are usually prepared by co-precipitation. Seeds of SiO2 form on the SPION surface in situ and grow through a Stöber mechanism under relatively mild conditions.49–54 However, SPION@SiO2 NPs prepared using this route tend to have high polydispersity and poor control over morphology, often exhibiting multiple SPION cores within a single shell. Additionally, high yields of by-product SiO2, with no SPION core, are often observed.26 Enhanced control over morphology and reduced polydispersity can be achieved using a reverse microemulsion route.29,30,55–60 In this approach, hydrophobic magnetite (usually oleic acid stabilised) is dispersed in a non-polar solvent (such as cyclohexane) along with a surfactant, e.g. IGEPAL-co-520, leading to ligand exchange. Concentrated aqueous ammonium hydroxide (NH4OH), used to hydrolyse the silica precursor and provide aqueous domains, and tetraethyl orthosilicate (TEOS) are added to the organic solution, forming a water-in-oil (w/o) emulsion. The SiO2 precursor nucleates within the aqueous domain and forms around the SPION core generating SPION@SiO2 core@shell NPs (Fig. 1). Within this approach, a number of parameters are recognised to be important in the mechanism of formation of single-core@shell particles, including concentration of reagents (silica source, basic catalyst, water and surfactant) and core particles, as well as fractionated additions of the silica source and reaction temperature.29,30,61–63 In comparison, this approach has been reported as more favourable in terms of forming single core SPION@SiO2 NPs with controlled shell thickness.
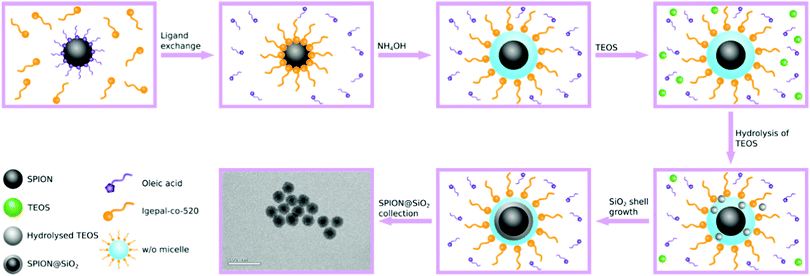 |
| Fig. 1 Mechanism of SPION@SiO2 nanoparticle synthesis via the reverse microemulsion method. Ligand exchange occurs between the oleic acid and IGEPAL-co-520, on the surface of SPIONs. Upon the addition of NH4OH, the surfactant arranges into a water/oil (w/o) micelle, in which the SPION occupies the centre. Following the addition of TEOS, NH4OH hydrolyses TEOS allowing entry of the hydrolysed TEOS molecules into the micelle. Within the micelle, the SiO2 shell grows around the SPION core, forming single-core uniform SPION@SiO2 NPs. | |
While SPION@SiO2 NPs are a prominent area of research, there are still difficulties associated with the reverse microemulsion method. Mainly, the route produces a large fraction of non-core SiO2 NPs, a non-magnetic by-product that requires additional processing to separate. Since reproducibility as well as particle size and morphology control are vital when considering such particles for biomedical applications, understanding the relationship between the reaction conditions and resulting SPION@SiO2 NPs is critical. To date, optimisation of SPION@SiO2 NPs has relied on ‘one factor at a time’ (OFAT) variation, where each experimental factor is changed individually.29,35,57,61–67 Consequently, a narrow range of the experimental domain is typically examined and variable interactions overlooked. This limits the understanding of how experimental variables affect the outcome of a synthesis, often leading to inaccurate conclusions and sub-optimal results.68
Design of experiment (DoE) is a powerful statistical tool for understanding and optimising the relationship between the experimental variables and the outcome of a process. The use of DoE for nanoparticle synthesis is gaining popularity and has provided insight into reaction mechanisms.69 For example, Lak et al. have used a DoE approach for the optimisation of SPIONs, with tailorable magnetic properties. In two separate studies, interactions were identified between experimental factors responsible for determining the dispersity and magnetisation of SPIONs formed via thermal decomposition and non-hydrolytic synthesis.23,70 Similarly, Roth et al. have employed DoE to study the formation of SPIONs using co-precipitation.22 The magnetisation of the SPIONs was deemed highly dependent on the experimental variables used, such as the molar ratio and concentration of Fe2+/Fe3+ ions. Arafa et al. used DoE to tune the properties of pregabalin-loaded niosomes for therapeutic delivery. This was made possible by examining the combined effect of different factors simultaneously.71 There have been several successful examples of the optimisation of nanoparticle synthesis and maximising their functionality using a DoE approach in the literature.23,72–80
While DoE has proved a powerful tool for nanoparticle optimisation, this approach has not been applied in the context of SPION@SiO2 NP synthesis. Herein, we implement a statistical approach to understand and predict the influence experimental factors have on the properties of SPION@SiO2 NPs formed via a reverse microemulsion method. The number of moles of the reactants NH4OH and TEOS, as well as the number of fractionated additions of TEOS were selected for investigation (vide infra 2.4 and 3.2). The size and stability of SPION@SiO2 NPs formed were characterised using transmission electron microscopy (TEM) and dynamic light scattering (DLS). Magnetic properties were evaluated using vibrating sample magnetometry (VSM). The experimental campaign was designed using a 33 full-factorial design and modelled using a response surface model (RSM) and analysed via the software JMP.81 In this way, we aim to provide a comprehensive understanding of the influence of important preparation parameters on resulting SPION@SiO2 NPs, and predictive capability to produce optimal single-core@shell particles.
2 Experimental
2.1 Chemicals
All chemicals were used as purchased with no additional purification. Cyclohexane (99.5%), and n-hexane were purchased from Fisher Scientific UK. Ammonium hydroxide (28–30%), IGEPAL-co-520, iron(III) chloride hexahydrate, oleic acid (90%), nitric acid (69%) 1-octadecane (90%), tetraethyl orthosilicate (TEOS, 99%) and sodium hydroxide pellets were purchased from Merck. Ultrapure water was obtained using a Millipore filtration system, operated at 18.2 MOhm.
2.2 Synthesis
2.2.1 SPION NPs.
Hydrophobic SPION NPs were prepared using a thermal decomposition method in accordance with the literature.29 FeCl3·6H2O (0.54 g, 2 mmol) was dissolved in water (6 mL, 333 mmol), ethanol (8 mL, 137 mmol), and hexane (14 mL, 106 mmol) at room temperature. Oleic acid (1.9 mL, 6 mmol) was added to the solution and stirred for 30 minutes. Sodium hydroxide (0.24 g, 6 mmol) was added to the stirred solution and sealed in a closed vessel and heated to 70 °C for 4 hours. The resulting solution was cooled to room temperature, forming two distinct layers. The top organic layer, containing Fe(oleate)3, was separated, collected and washed with ultrapure water three times. To remove hexane, Fe(oleate)3 was heated in a vacuum oven at 80 °C overnight. The dried Fe(oleate)3 precursor was resuspended in oleic acid (0.32 mL, 1 mmol) and 1-octadecane (12.5 mL, 38 mmol) in a sealed vessel and oxygen was degassed with N2 gas for 1 hour at room temperature. Subsequently, the solution was heated at 320 °C for 30 minutes under the inert atmosphere in a sealed vessel. The solution was cooled to room temperature, and excess ethanol was added to precipitate NPs. Hydrophobic SPIONs were collected by centrifugation and the supernatant decanted. The isolated solid was re-dispersed in hexane (approx. 1–2 mL) and subsequently precipitated in excess ethanol. This precipitation-re-dispersion process was repeated three times to purify the magnetic NPs. The SPIONs were stored in cyclohexane (5.0 mg mL−1).
2.2.2 SPION@SiO2 NPs.
Core@shell SPION@SiO2 NPs were prepared using a reverse microemulsion method adapted from the literature.29 IGEPAL-co-520 (0.5 g, 1 mmol) was dispersed in cyclohexane (11 mL, 101 mmol) and sonicated for 10 min at ambient conditions. SPIONs in cyclohexane (1 mL, 5 mg mL−1) were added to the stirred solution followed by the addition of ammonium hydroxide. The controlled addition of TEOS was performed using a fractionated drop-method; where a known volume of TEOS was added every 4 hours. A total of 3 additions were carried out per day and reacted overnight. For 6 and 8 additions, the 4th (and 7th) addition were carried out 24 (and 48) hours after the first addition, and subsequent additions were carried out following the 4 hour interval. A schematic of the synthesis can be seen in Fig. 1, detailing how the the shell is formed within the reverse micelle. Once complete, SPION@SiO2 NPs were washed in ethanol using centrifugation three times and redispersed in ethanol. During the study, the number of moles of TEOS and NH4OH, and the number of factorial drop-wise additions of TEOS were varied according to the design shown in Table S1 (ESI†).
2.3 Characterisation
Dynamic light scattering (DLS) was carried out using a Beckman Coulter DelsaMax Pro, measured at 22 °C, with samples dispersed in ultrapure water (1 mg mL−1). Transmission electron microscopy (TEM) was performed with a JEOL 2100 transmission electron microscope operated at 200 kV. Samples were prepared by depositing NPs dispersed in water (0.05 mg mL−1) onto a carbon coated 300 mesh copper grid (Agar Scientific). NP diameter and population were measured using ImageJ (version v1.53) with a sample size of approximately 300 NPs. By assuming the particles were spherical, the diameter was calculated by measuring the area of the particles, with an estimated resolution of 0.1 nm.82 This resolution was used as the bin width to produce a histogram of each population. It was then possible to determine the dispersity of the NPs using the concept of information entropy, as first introduced by Shannon, and modified to suit nanoparticle analysis as follows: |  | (1) |
where n is the number of non-empty bins and pi is the probability of a given nanoparticle size occurring within the population.83 A vibrating sample magnetometer-physical property measurement system (VSM-PPMS, Quantum Design) was employed to measure the mass susceptibility (χm) of SPION@SiO2 NPs. Samples were prepared as dry powders and recordings were performed at 25 °C, using a maximum magnetic field strength of 20
000 Oe and the minimum magnetic field strength of −20
000 Oe, with a sweep rate of 50 Oe s−1. Graphs were analysed in Origin, and smoothed with a percentile filter.
2.4 Experimental design
The experimental design was produced and studied using the statistical software JMP (version 15).81 To establish the local response surface, a 33 full-factorial design was chosen to study the synthesis of SPION@SiO2 (Fig. 2). This design allowed for the identification of main effects and interactions, in addition to non-linear effects, which were modelled as quadratic functions. Three experimental variables were selected for investigation: moles of TEOS, moles of NH4OH, and the number of fractionated additions of TEOS, as stated in Table 1. These experimental factors and their upper and lower limits were chosen based on literature evidence, being previously identified as important parameters in the hydrolysis and condensation mechanism for the silica shell formation.29,30,35,55–66 The concentration and size of the SPION NP core and the concentration of the core stabilising agent, IGEPAL-co-520 were fixed, and not included in the study, as the growth of a silica shell is primarily controlled by the aforementioned experimental factors. The centre point treatment, labelled 222, was repeated five times, totalling 31 experimental runs. These were randomised to avoid bias. It is worth noting that for ease of comparison between factors and the responses, the experimental treatments have been arranged based on the DoE pattern of the experimental factors (1,2,3). In this study, the silica shell thickness, monodispersity and population of SPION@SiO2 NP formed were used as response variables. The null hypothesis, H0, states that the experimental factors (number of mole of TEOS, number of moles of NH4OH, and the number of fractionated additions of TEOS) have no effect on the SPION@SiO2 NP formed. The alternative hypothesis, Ha, states that the experimental factors do effect the SPION@SiO2 NP formed.
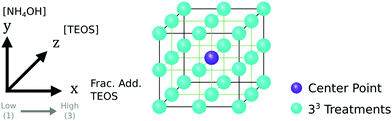 |
| Fig. 2 Schematic of a 33 full factorial experiment domain. The x-axis represents the number of fractionated additions of TEOS (Frac. Add. TEOS), the y-axis the number of moles of NH4OH, and the z-axis the the number of moles of TEOS. The blue spheres are the xyz coordinates of treatments (combinations of experimental factors and levels) used in the experimental domain. The purple sphere represents the centre point condition (xyz = 222). | |
Table 1 Experimental factors used in the 33 full-factorial design model: number of fractionated additions of TEOS, total number of moles of TEOS, and number of moles of NH4OH
Experimental factor |
Low (1) |
Centre (2) |
High (3) |
Fractionated additions |
3 |
6 |
8 |
n TEOS (mmol) |
0.16 |
1.42 |
2.69 |
n NH4OH (mmol) |
0.13 |
0.65 |
1.16 |
Following the completion and characterisation of the experimental runs, regression analysis was performed for each of the response variables: shell thickness (tshell), monodispersity (described using En), and population (% of SPION@SiO2 NPs compared to all particles formed). Using relevant statistics including the analysis of variance, lack of fit test, and goodness of fit metrics such as the r2 and root mean squared error (RMSE), the most suitable models for each response were identified. Using the model, a regression equation was generated for each response. A factor was considered active when p ≤ 0.05. Terms greater than this were deemed inactive and were iteratively removed from the model.
3 Results and discussion
3.1 Synthesis of SPION NPs
Superparamagnetic iron oxide nanoparticles (SPIONs) stabilised with oleic acid were prepared using thermal decomposition. The NPs were highly monodisperse with a size of 12 ± 2 nm (as measured from TEM analysis, Fig. S1, ESI†) and χm of 38 emu g−1 at 20
000 Oe, normalised against total mass of particle, and confirmed to be superparamagnetic (Fig. S1, ESI†). While hydrophilic SPIONs usually report a χm of approx. 70 emu g−1 at 20
000 Oe, in this case the χm is lower due to the presence of non-magnetic oleic acid contributing to the sample mass.6,84
3.2 Experimental design of synthesis of SPION@SiO2 NPs
SPIONs were coated with silica to form SPION@SiO2 NPs, using a reverse microemulsion method, as described in the Experimental Section. The formation of a silica coating on NPs using reverse microemulsion is reliant on a number of factors,29,30,57,60–65,85 with the concentrations of the silica source and the basic catalyst being reported as the most important in controlling shell thickness, presence of undesirable (non-magnetic) by-products, and uniform populations. The SiO2 shell thickness, for example, can be controlled by altering the number of moles of TEOS, with low concentrations resulting in ultra-thin 2 nm SiO2 shell thicknesses, due to the small amount of silica precursor available for coating.63 A similar effect can be achieved by changing the number of moles of aqueous NH4OH and/or the ratio of NH4OH-to-surfactant.30,61,63–65 In the former, adjusting the quantity of aqueous NH4OH affects the rate of silica hydrolysis and nucleation, where higher concentrations increase the rate of TEOS hydrolysis and increases the shell thickness; however this can also encourage the formation of non-magnetic silica by-product.29,30 The ratio of NH4OH-to-surfactant, on the other hand, determines the size and number of micelles formed, influencing the ultimate size of particles formed (since the coating step occurs within the micelle).29,30,61 Katagiri et al. have demonstrated that the silica shell could be further enlarged by subsequent additions of IGEPAL-co-520, aqueous ammonium hydroxide, and TEOS, increasing the NP diameter from 40 nm to 56 nm. Increase in shell growth only occurred with addition of the three reagents and not with subsequent additions of TEOS alone. Katagiri rationalised that the increase in shell growth was due to the expansion the microemulsion surrounding the SPION core, made possible with the addition of surfactant and aqueous NH4OH, which provided more space for the silica shell to grow.30 It should, however, be noted that the relationship between micelle/microemulsion size and the resulting particle is complex and can be impacted by a number of different parameters. It has also been found that the fractionated addition of TEOS can reduce particle polydispersity, simultaneously reducing the formation of by-product SiO2.29
Despite their identification as important experimental conditions in the formation of SPION@SiO2 NPs, these parameters have not been investigated through a DoE approach, which allows for the simultaneous variation of experimental factors.72,76–78 This not only enables the evaluation of interactions between these factors, but also reduces the impact of random variation. Furthermore, by implementing 3 levels (low, centre, high), non-linear effects can also be measured. The resulting model can be manipulated to maximise a response, such as the size of core@shell NPs. Therefore, in the study herein, the numbers of moles of TEOS and NH4OH, and fractionated additions of TEOS were investigated as primary variables of importance in the production of core@shell particles. The responses of SiO2 shell thickness, monodispersity, and population (where population is described as the percentage of single-core@shell particles with respect to the total number of particles) were analysed in order to optimise and control the properties of the NPs formed. A 33 full-factorial model was employed, and experimental conditions were generated according to Table 1.
3.3 Response surface analysis: SPION@SiO2 particle design
SPION@SiO2 NPs prepared according to the conditions outlined by the DoE were characterised using TEM, and PPMS-VSM. TEM analysis determined the NP size, monodispersity, and population of SPION@SiO2 NPs formed. Fig. 3 shows TEM images of the NPs generated at level 1 of number of moles of TEOS (0.16 mmol); TEM images of samples prepared at TEOS levels 2 and 3, and centre point conditions can be found in Fig. S2–S4 (ESI†). Box-plots showing the size distribution of the measured total diameter (dTEM) of the core@shells produced in this study can be observed in Fig. S5 (ESI†). Good reproducibility was demonstrated from repeating the centre point condition (Fig. S6, ESI†), expressed as a scatter plot of the response values compared against the mean response for the centre point. Here the response values show good precision, and are similar in value as shown in Table S1 (ESI†). Response surface analysis was used to explore relationships and interactions between experimental factors and their effect on the response variables (Table 2 and Table S1, ESI†). Across all particles produced, the dTEM of particles ranged from 15 nm to 75 nm; the thickness of the silica shell (tshell) ranged 2 nm to 32 nm; the normalised entropy (En) ranged 0.15 to 0.65 (characterising all particles to be either monodisperse or near monodisperse (vide infra)); and a single-core@shell population of 35% to 99% was observed. The SPION@SiO2 were found to be weakly superparamagnetic; the mass susceptibility plots can be found in Fig. S7 (ESI†).
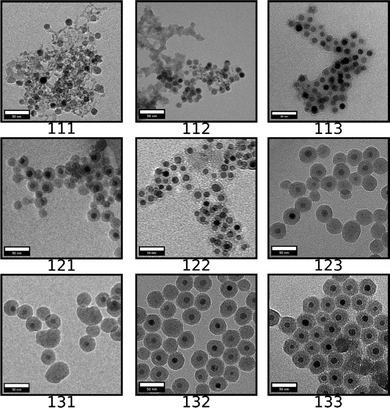 |
| Fig. 3 TEM images of SPION@SiO2 NPs produced using conditions determined from the 33 factorial model, at level 1 of the number of moles of TEOS (0.16 mmol). Scale bar is 50 nm. The rows (representing x-axis) relate to the number of fractionated additions of TEOS (Frac. Add. TEOS), which is increasing from left to right in levels of fractionated additions of TEOS, from levels 1 to 3. The columns (representing y-axis) relate to the number of moles of NH4OH, and is increasing from top to bottom in levels 1 to 3 for the number of moles of NH4OH. Each 3 digit code is the xyz coordinates for each treatment condition generated from the experimental domain, as described in Table 1, where the z-axis relates to the number of moles of TEOS. The remaining TEM images, at TEOS levels 2 and 3 can be found in Fig. S2 and S3 (ESI†). Additionally, repeats of the centre point condition (treatment 222) can be found in Fig. S4 (ESI†). | |
Table 2 Results of regression analysis of variance for all response variables. Note r2 is the ratio of sum of squares describing the proportion of variation explained by the model. The F-ratio is the ratio between explained error (r2) and unexplained error (RMSE)
Response |
Mean |
r
2
|
RMSE |
p-Value |
F-Ratio |
t
shell (nm) |
14 |
0.71 |
4.87 |
<0.0001 |
12.65 |
E
n
|
0.35 |
0.68 |
0.089 |
<0.0001 |
10.74 |
Pop. (%) |
53 |
0.32 |
18.94 |
0.014 |
4.25 |
3.3.1 Shell thickness of SPION@SiO2 nanoparticles.
The silica shell thickness, tshell, of the SPION@SiO2 NPs was determined from TEM images, Fig. 3.82 It was observed that as the level of the experimental factors increased, the shell thickness of the particles also increased. Nanoparticles produced using lower levels of the factors (i.e. treatments 111 to 122) were observed to have an ultra-thin silica shell, however the particles themselves appeared embedded within a non-uniform aggregated silica matrix, lacking discrete individual core@shell structures. It is thought that when a lower number of moles of TEOS (0.16 mmol) and NH4OH (0.13 mmol) and fewer fractionated additions of TEOS are used, the formation of by-product silica is favoured. A lower concentration of NH4OH reduces the aqueous domains present which reduces the micelle size and quantity, therefore limiting places for shell growth to occur. Fewer fractionated additions of TEOS increase the amount of TEOS within each addition available for hydrolysis. With more hydrolysed TEOS present, there is competition between the formation of silica by-product and growth of the silica shell. Due to the limited micelles available, this increased presence of hydrolysed TEOS therefore favours the formation of silica by-product. As such, these conditions encouraged the formation of non-defined core@shell structures, (as seen in the top row of Fig. 3). As the number of moles of NH4OH and fractionated additions of TEOS increased, more defined core@shells were formed, and increasing tshell was observed.
The prediction expression of tshell, as determined by regression analysis, is given by:
|  | (2) |
where
α is number of moles of TEOS,
β is the number of moles of NH
4OH and
γ is the number of fractionated additions of TEOS. The parameter estimates for the equation can be seen in
Table 3. The model was found to agree well with the data with an
r2 of 0.71 and RMSE of 4.74 (
Table 2). Analysis of variance (ANOVA) demonstrated the model was highly significant with a
p-value of < 0.0001. As such, the model explains the variance in the outcome, and
H0 is rejected. A plot of actual
vs. predicted response for shell thickness, determined from the regression analysis, is presented in
Fig. 4a and demonstrates the success of the model.
Table 3 Parameter estimates that were deemed to be active in effecting the silica shell thickness of SPION@SiO2 nanoparticles formed. The parameter estimates were calculated from the regression analysis and used in the prediction expression. In the case below, the fractionated addition of TEOS is included as a parameter, regardless of its p-value, due to its presence in the interaction term. Note that the t-ratio is the estimate divided by the standard error. If ≥1.96 (absolute value) the parameter is statistically significant. If the absolute value is <1.96 the parameter is not statistically significant. The p-value is the probability of the null hypothesis (H0) being true. The lower the p-value the less likely the H0 is true. Herein, the null hypothesis assumes the experimental factors have no impact on the outcome
Parameter |
Estimate |
Std Error |
t Ratio |
p-Value |
Intercept |
0.86 |
3.46 |
-0.25 |
0.806 |
TEOS (α) |
4.74 |
0.94 |
5.06 |
<0.0001 |
NH4OH (β) |
10.02 |
2.30 |
4.43 |
0.0002 |
Frac. Add. TEOS (γ) |
0.13 |
0.46 |
0.28 |
0.7808 |
(β −0.67)·(γ −5.65) |
3.12 |
1.09 |
2.87 |
0.0091 |
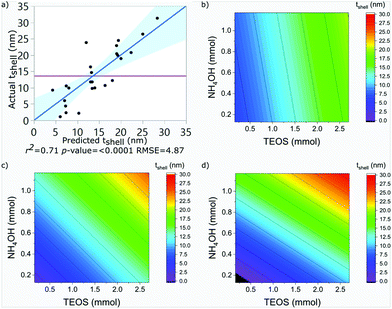 |
| Fig. 4 Regression analysis of the silica shell thickness,tshell, on SPION@SiO2 nanoparticles formed. (a) Actual vs predicted scatter plot of the silica shell thickness, where the black markers are the treatment shell thickness, the purple line is the mean shell thickness at 14 nm, the blue line is the line of fit, and the light blue bands are 0.05 significance curve; (b) 2D contour-map of regression analysis of shell thickness formed within the experimental domain, at 3 fractionated additions of TEOS; (c) the contour-map at 6 fractionated additions of TEOS; (d) the contour-map at 8 fractionated additions of TEOS. It is important to note that the black region in the bottom left-hand corner indicates that the predicted tshell is below 0 nm. This is synonymous with the lack of coating of the SPION, and hence, SPION@SiO2 NPs will not be formed at these conditions. | |
The shell thickness was observed to be linearly dependent on the number of moles of TEOS. The number of moles of NH4OH similarly impacted the size. Additionally, an interaction between the number of moles of NH4OH and the number of fractionated additions was identified. It is important to note here, that the fractionated addition of TEOS alone did not have a significant effect (p-value = 0.9263), however it is included due to its presence in the interaction term. A larger mean shell thickness was achieved for higher number of moles and more additions, while the inverse produced smaller particles. These relationships are summarised in eqn (2) and the contour maps presented in Fig. 4b–d. Overall, it was seen that increasing each of the terms increased the size of particles: increasing the number of moles of TEOS meant more material was present to form silica shells; increasing the number of moles of NH4OH increased the presence of aqueous domains, resulting in larger micelles as the number of moles increased; and increasing fractionated additions favoured the growth of the silica shell around the SPION core. These observations correlate with literature descriptions of silica shell growth,29 however this study illustrates and quantifies the relationship of the interaction between NH4OH and fractionated addition of TEOS for the first time. By combining the number of moles of each parameter and alternating the fractionated additions of TEOS, exceptional control over shell thickness can be achieved through careful manipulation of experimental conditions.
It is important to clarify that at 8 fractionated additions of TEOS between 0.1 to 0.3 mmol of NH4OH and 0.25 and 0.75 mmol of TEOS, it is predicted that no coating of the SPION core would occur. This is a limitation of the experimental design model, where the resulting models are often less reliable at the extremities of the experimental domain. In practice, these conditions are likely to produce SPION embedded in a silica matrix, similar to those formed at treatments 111, 112 and 113, for reasons discussed previously.
3.3.2 Monodispersity of SPION@SiO2 nanoparticles.
The monodispersity of the NPs, as determined from nanoparticle size distribution was assessed using nanoparticle entropy (En).83 Size distribution was analysed using a modified Shannon entropy, eqn (1). By using this approach, a system can be described as either highly monodisperse, monodisperse, near-monodisperse, or polydisperse, based on the normalised nanoparticle entropy, En. If En falls in the range 0 to 0.125, it is classified as highly monodisperse, it is monodisperse if En is between 0.125 and 0.206, it is near-monodisperse between 0.206 and 0.618, and anything above 0.618 is classified as polydisperse.83 Following this classification, all SPION@SiO2 NPs formed were classified as monodisperse or near-monodisperse (see Table S1, ESI†), excluding treatments 111 and 113, which were classified as polydisperse. Treatments 112 and 331 were excluded from analysis due to an insufficient sample size.
The prediction expression of En, as determined by regression analysis, is given by:
|  | (3) |
where
α is number of moles of TEOS,
β is the number of moles of NH
4OH and
γ is the number of fractionated additions of TEOS. The parameter estimates for the equation are shown in
Table 4. The model was found to agree well with the data, with an
r2 of 0.68 and RMSE of 0.089 (
Table 2). ANOVA demonstrated the model was highly significant with a
p-value of <0.0001, illustrating again that, overall, the experimental factors directly impact the monodispersity of the produced particles, hence,
H0 was rejected. A plot of actual
vs. predicted response of particle dispersity, determined from the regression analysis, is presented in
Fig. 5a.
Table 4 Parameter estimates that were deemed to be active in effecting the monodispersity of SPION@SiO2 nanoparticles formed, described as nanoparticle entropy (En). The parameter estimates were calculated from the regression analysis and used in the prediction expression. In the case below, the fractionated addition of TEOS is included as a parameter, regardless of its p-value, due to its presence in the interaction term
Parameter |
Estimate |
Std error |
t Ratio |
p-Value |
Intercept |
0.59 |
0.06 |
9.22 |
<0.000 |
TEOS (α) |
−0.09 |
0.02 |
−4.73 |
<0.0001 |
NH4OH (β) |
−0.19 |
0.04 |
−4.37 |
0.0003 |
Frac. Add. TEOS (γ) |
0.00 |
0.01 |
0.23 |
0.821 |
(β −6.47)·(γ −5.77) |
−0.04 |
0.02 |
−1.97 |
0.0629 |
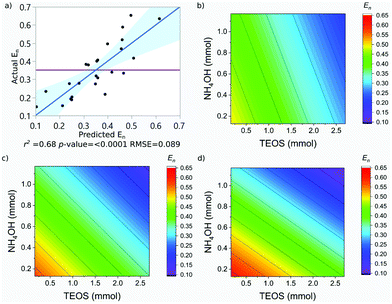 |
| Fig. 5 Regression analysis of the monodispersity of SPION@SiO2 nanoparticles formed, using nanoparticle entropy (En). Particles are classified as either highly monodisperse, monodisperse, or near-monodisperse based on the numerical normalised nanoparticle entropy En value of 0–0.125, 0.125–0.206, and 0.206–0.618 respectively. (a) Actual vs predicted scatter plot of the normalised nanoparticle entropy En, where the the black markers are the treatment En, the purple line is the mean En at 0.35, the blue line is the line of fit, and the light blue bands are a 0.05 significance curve. (b) 2D contour-map of regression analysis of particle entropy formed within the experimental domain, at 3 fractionated additions of TEOS. (c) the contour-map at 6 fractionated additions of TEOS and (d) the contour-map at 8 fractionated additions of TEOS. | |
The En was observed to be negatively dependent on the number of moles of TEOS; with increasing number of moles, there was a decrease in En. Similar behaviour was observed for NH4OH. An interaction between the number of moles of NH4OH and the number of fractionated additions of TEOS was identified. As discussed for the shell thickness, the fractionated addition of TEOS term was also included in the model, due to the presence in the interaction term, despite not exhibiting a significant effect itself (p-value = 0.821). Monodisperse populations were achieved at higher numbers of moles and more additions, while the inverse produced populations classified as near-monodisperse. These relationships are summarised in eqn (3) and the contour maps presented in Fig. 5b–d. It is of interest to note that the terms active in effecting En were also active in influencing tshell. This is due to the commonality between the mechanism, outlined in the previous section; namely that the number of moles of NH4OH influences the micelle formed and is used to hydrolyse TEOS Using fractionated additions of TEOS influences the silica shell growth and the formation of silica by-product. By impacting the micelle properties (where shell growth occurs) and rate of hydrolysis of TEOS, it is possible to create monodisperse particles; as showcased by the interaction of number of moles of NH4OH and fractionated additions of TEOS. Monodispersity of particle population is vital in the consideration of such materials for biomedical applications and hence this observation is important, as it clearly demonstrates how both the size and dispersity of particles can be carefully controlled through manipulation of experimental conditions.
3.3.3 Population of SPION@SiO2 nanoparticles formed.
Population of SPION@SiO2 nanoparticles refers to the percentage of the total measured population of particles which exist as single-core@shell particles. The regression analysis of population of SPION@SiO2 NPs was performed using the data collected from TEM analysis (Table 2 and Table S1, ESI†). The regression model correlation coefficient (r2) was 0.32, indicating that the experimental variation was large for this model. The significant experimental error is most likely due to the inherent error in the determination of population, which was conducted by TEM analysis. The associated population error for each treatment was calculated from the deviation of population of core@shells measured per TEM image. This can be be observed in Fig. 6a, which shows grouping of the data around the regression line. Nonetheless, the lack of fit test, F-test, was 0.93, demonstrating that although experimental error was large, it was statistically significant (with good fitting of the data, p-value = 0.48, Table S2, ESI†), and therefore the regression model can be used to maximise the population response. Please refer to Table S2 (ESI†) for lack of fit test information.
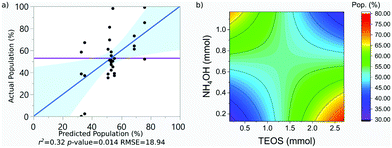 |
| Fig. 6 Regression analysis of the population of SPION@SiO2 nanoparticles formed. (a) Actual vs. predicted scatter plot of the population of SPION@SiO2 NPs, where the the black markers are the treatment populations, the purple line is the mean population at 53%, the blue line is the line of fit, and the light blue bands are 0.05 significance curve; (b) 2D contour-map of regression analysis of population of SPION@SiO2 NPs formed within the experimental domain. | |
The prediction expression for population, as determined by regression analysis, is given by:
| 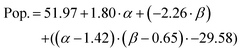 | (4) |
where
α is number of moles of TEOS, and
β is the number of moles of NH
4OH. The parameter estimates for the equation can be seen in
Table 5. Across all samples prepared, there was a mean population of 53% and the RMSE was 18.94,
Table 2. ANOVA indicated that the model was significant as the
p-value was 0.014 and the
F-value was 4.25, meaning
H0 was rejected. A plot of actual
vs. predicted population responses, determined from the regression analysis, is presented in
Fig. 6a. Here the high experimental error can be observed, from the scattering around the regression line of fit, and wide confidence bands. It is likely that other parameters could be influencing the population of SPION cores coated by silica which has not been investigated in this study, including, but not limited to, the concentration of IGEPAL-
co-520, the concentration of water, or indeed the temperature of the system.
29,30,61
Table 5 Parameter estimates deemed to be active in effecting the population of SPION@SiO2 formed. The parameter estimates were calculated from the regression analysis and used in the prediction expression. In the case below, the number of moles of TEOS and number of moles of NH4OH are still included as a parameter they are active in the (TEOS-1.43)·(NH4OH-0.65) parameter
Parameter |
Estimate |
Std error |
t Ratio |
p-Value |
Intercept |
51.97 |
8.26 |
6.29 |
<0.0001 |
TEOS (α) |
1.80 |
3.53 |
0.51 |
0.6145 |
NH4OH (β) |
−2.26 |
8.67 |
−0.26 |
0.796 |
(α −1.43)·(β −0.65) |
−29.58 |
8.39 |
−3.52 |
0.0015 |
The population of SPION@SiO2 was observed to be dependent on the interaction between the number of moles of TEOS and the number of moles of NH4OH. The term was estimated to have a negative effect on the population if the number of moles of both simultaneously increased or decreased. If the number of moles of either TEOS or NH4OH increased, while the other decreased, an increase in population of SPION@SiO2 would be observed. These trends can be found in the regression eqn (4) and contour-map in Fig. 6b, where the contour planes are curved and the map has a paraboloid-structure. It is interesting to note that the fractionated addition of TEOS was determined to have no effect on the population of core@shell particles formed, contrary to what is reported in literature.86 Instead, we found that fractionated additions of TEOS contributed only to the shell growth and monodispersity and hence the mechanism of growth, rather than population of SPION@SiO2 formed.
3.3.4 Optimisation of SPION@SiO2 NPs.
Using the regression analysis for each of the response variables, the models shown herein should allow the production of single-core@shell NPs with controlled shell thickness, good monodispersity and with high populations. In order to test the models, the prediction expressions were combined and three validation experiments were conducted, aiming to produce SPION@SiO2 NPs with total sizes of 50 nm, having a shell thickness of 18 nm, classified as monodisperse, and with a high population of core@shell particles. For these studies, the experimental conditions were taken at different coordinates of the experimental design, as seen in Table 6 and Fig. 8, demonstrating that different experimental conditions may lead to similar particles being produced. Size distribution of the measured nanoparticle diameter from TEM (dTEM), can be found in Fig. S8 (ESI†). The mass susceptibility of the nanoparticles is given in Fig. S7 (ESI†).
Table 6 Conditions of validation experiments with predicted (pred.) and observed (obs.) responses, guided by prediction expression of regression analysis. Note that the predicted error was taken from the prediction profiler, from JMP software, which uses the prediction expression to predict the outcome of a response using the conditions outlined above. The observed error for the tshell and En, was calculated using the standard deviation of the sample set. The error bar for population is based on the deviation of population of core@shells measured per TEM image
Run |
NH4OH (mmol) |
Frac. add. TEOS |
TEOS (mmol) |
t
shell (nm) Pred.± Obs.± |
E
n
Pred.± Obs.± |
Pop. (%) Pred.± Obs.± |
1 |
0.41 |
3 |
2.69 |
19 ± 4 |
0.26 ± 0.08 |
61 ± 14 |
|
|
|
|
14 ± 3 |
0.32 ± 0.02 |
54 ± 17 |
2 |
1.16 |
7 |
0.98 |
19 ± 4 |
0.27 ± 0.07 |
62 ± 14 |
|
|
|
|
28 ± 3 |
0.20 ± 0.02 |
69 ± 28 |
3 |
1.16 |
8 |
0.63 |
19 ± 5 |
0.28 ± 0.09 |
67 ± 15 |
|
|
|
|
22 ± 4 |
0.23 ± 0.01 |
77 ± 11 |
The silica shell thickness of the three validation experiments were found to be 14± 3 nm, 28± 3 nm, and 22± 3 nm, respectively, as seen in Fig. 7. Run 1 and 3 were in good agreement with the predicted thickness, as observed in Fig. 8b, whereas run 2 was larger than anticipated. There was strong agreement between the predicted and observed nanoparticle entropy, with all particles observed to be monodisperse. Furthermore there was also strong agreement between the predicted and observed population, which achieved populations of SPION@SiO2 >50% for the three samples. Run 1 used 2.69 mmol of TEOS, across 3 fractionated additions, using 0.40 mmol NH4OH. This supports the regression analysis for each of the responses: the silica shell thickness was dependent on the number of moles of TEOS and the combined interaction of number of moles of NH4OH and fractionated addition of TEOS, where the shell thickness increased as one of these terms increased. The particle dispersity was also dependent on the aforementioned factors, which decreased as the terms increased (becoming more monodisperse). Section 3.3.3 described the population of SPION@SiO2 to be dependent on the interaction of TEOS number of moles and the number of moles of NH4OH, where an increase in population when one number of moles was high, and the other number of moles was low resulted in high population yield, reflective of the conditions used in run 1. Similar observations were also observed for run 2 and 3, which used a higher number of moles of NH4OH (1.16 mmol), and lower number of moles of TEOS (0.98 mmol and 0.63 mmol, respectively) across more fractionated additions of TEOS (7 and 8 respectively). Interestingly here, particles of similar morphology, size and characteristics have been produced using different experimental conditions. These validation experiments demonstrate that DoE is valuable for the optimisation of this nanoparticle synthesis and allow flexibility for users to achieve their desired optimised NP. DoE allows accurate prediction across a variety of parameter combinations, which can be of use in scenarios where experimental set ups are limited, or can aid in considering scale-up of particle synthesis.
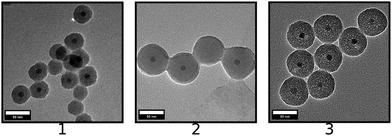 |
| Fig. 7 TEM Images of SPION@SiO2 NPs produced for validation experiments, using conditions determined via the response surface prediction expression. | |
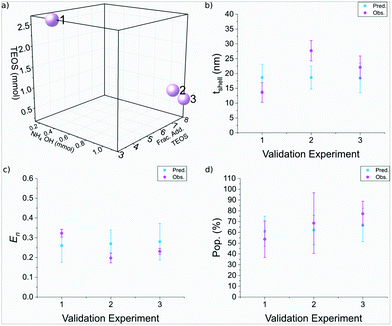 |
| Fig. 8 Comparison of observed vs. predicted responses for the validation experiments, which were designed following regression analysis. (a) Experimental design space, with the experimental coordinates of validation experiments. (b) Observed vs. predicted scatter plot for silica shell thickness. (c) Observed vs. predicted scatter plot for nanoparticle entropy. (d) Observed vs. predicted scatter plot for population of SPION@SiO2 nanoparticles formed. Note that the predicted error for each response was provided from prediction profiler, given by JMP. The observed error for the tshell and En was calculated using the standard deviation of the sample set. The error bar for population is calculated from the deviation of population of core@shells measured per TEM image. | |
4 Conclusions
Regression analysis of SPION@SiO2 indicated that the number of moles of TEOS, number of moles of NH4OH and fractionated addition of TEOS were important parameters in determining the size, monodispersity and overall quality of the population of particles formed. Throughout each of the different response models, the experimental parameters of TEOS and NH4OH number of moles were statistically relevant, regardless of the nature of their effect. On the other hand, the number of fractionated additions of TEOS was found to be significant only as part of an interaction effect in combination with either the number of moles of TEOS or number of moles of NH4OH. The cause of these dependencies lies in the mechanism of the growth of NPs.
SPION@SiO2 NPs were synthesised via a reverse microemulsion method, as seen in Fig. 1 and discussed in Section 2.2.2. For clarity and understanding the mechanism will be discussed in brief, however an in depth discussion can be found by Ding et al. and Katagiri et al.29,30 In this mechanism, cyclohexane was used as the continuous phase. Upon the addition of oleic acid-coated SPION and surfactant IGEPAL-co-520, ligand exchange occurs, resulting in IGEPAL-co-520 coated SPION. Following the addition of NH4OH, IGEPAL-co-520 stabilised water-in-oil (w/o) micelles were formed due the presence of aqueous domains provided by aqueous NH4OH. Within the w/o micelles, SPIONs occupy the centre. After the addition of TEOS, NH4OH causes the hydrolysis of TEOS, which can enter the micelle where condensation and silica growth can occur. Notably, a fractionated addition of TEOS avoids the formation of silica NP by-product by reducing the local concentration of hydrolysed TEOS and uncontrolled homogeneous nucleation.29 Within this study, there was a statistically significant interaction between the factors of numbers of moles of NH4OH, TEOS and control of its fractionated addition. Through understanding the reaction mechanism, it is clear why the regression analysis determined that these experimental factors were highly dependent on each other. TEOS is clearly needed for the growth of silica shell, with the amount added impacting the shell thickness, and monodispersity of particles formed. The number of moles of NH4OH is responsible for both the quantity and size of aqueous domains present for the hydrolysis and condensation reaction to occur, and the hydrolysis of TEOS within the reaction mechanism. It is clear that the shell thickness is directly dependent on the TEOS to provide the silica source and the presence of the aqueous domain to allow coating, with fractionated additions controlling the rate of hydrolysis to also control monodispersity through reducing the chances of formation of non-core particles. Population is similarly controlled through these mechanistic interactions. It should, however be noted that large experimental errors were observed for the population analysis, illustrating the limitation of the model for this measured outcome, and the potential influence of other parameters not included in this study.
These trends were identified and modelled through the use of DoE. From understanding these findings and their interplay in the mechanism of core@shell particle formation, they can be applied to optimise the characteristics of desired SPION@SiO2 NPs, or can be used to match specifications for a given application or experimental setup. For example, monodisperse SPION@SiO2 NPs with a yield greater than 70% which are 50 nm in size could be synthesised using low number of moles of NH4OH, and high number of moles of TEOS, over 6 fractionated additions. If altering the size of NPs are of interest, on the other hand, the protocol could be tuned through altering the amount of TEOS and NH4OH used, and using 3 fractions of TEOS. Alternatively, the (population) yield and monodispersity of NPs may be increased through using 6 fractionated additions, instead of 3 or 8. This approach has therefore yielded exploitation of the mechanism of formation of the particles to produce a desired goal. It must be emphasised, from the model there are a number of possible routes to the desired result, as observed from the validation experiments, which all produced monodisperse SPION@SiO2 NPs that had a desired shell thickness of 18 nm, at high populations.
The synthesis of NPs can be a complicated and demanding process to understand. Through using DoE, the intricate reaction process can be studied and modelled, allowing for the reaction outcome to be predicted in relation to the experimental domain used. In this study, the synthesis of SPION@SiO2 NPs through a microemulsion method has been modelled using a 33 full-factorial design. Following regression analysis, the silica shell thickness was found to be linearly dependent on the number of moles of TEOS, and the interaction between the number of moles of NH4OH and fractionated additions of TEOS, whereby increasing these terms were observed to increase the size of particles formed. Similarly the nanoparticle dispersity was dependent on the linear effect of the number of moles of TEOS, and the interaction between the number of moles of NH4OH and fractionated additions of TEOS. In this case, the increase in one of these terms resulted in the reduction in nanoparticle dispersity. The population of SPION@SiO2 NPs was effected by the interaction between TEOS and NH4OH, and not impacted by the fractionated addition of TEOS. The complexity of the model was reflective of the synthesis mechanism, where each of the reagents hold multiple roles that are dependent on each other in controlling the properties of the produced NPs. Through using a DoE approach, these underlying trends were identified and modelled and could be used for the optimisation of or tailoring of SPION@SiO2 NPs with controlled properties. It is clear that application of a DoE framework has the potential to provide insights into numerous other synthetic routes to a wide variety of materials. We hope that such approaches will be adopted more regularly in the future.
Author contributions
Clarissa L. G. Harman: Conceptualization, methodology, formal analysis, investigation, writing – original Draft. Niamh Mac Fhionnlaoich: conceptualization, formal analysis, writing – review & editing. Aaron M. King: investigation. Joseph R. H. Manning: writing – review & editing. Wu Lin: supervision. Peter Scholes: supervision. Stefan Guldin: supervision, conceptualization, writing – review & editing. Gemma-Louise Davies: supervision, conceptualization, writing – review & editing.
Conflicts of interest
There are no conflicts to declare.
Acknowledgements
The authors acknowledge financial support from the Engineering and Physical Sciences Research Council through the Centre for Doctoral Training in Advanced Therapeutics and Nanomedicines (EP/L01646X, supporting CLGH). For the purpose of open access, the authors have applied a Creative Commons Attribution (CC BY) licence to any Author Accepted Manuscript version arising. Thanks to Dr Steve York and Dr Yisong Han, at the University of Warwick's Research Technology Platform, for electron microscopy imaging.
Notes and references
- N. D. Thorat, O. M. Lemine, R. A. Bohara, K. Omri, L. El Mir and S. A. Tofail, Phys. Chem. Chem. Phys., 2016, 18, 21331–21339 RSC.
- M. Colombo, S. Carregal-Romero, M. F. Casula, G. Lucia, D. M. P. Morales, I. B. Bo, J. T. Heverhagen, D. Prosperi and W. J. Parak, Chem. Soc. Rev., 2012, 41, 4306–4334 RSC.
- D. S. Mathew and R. S. Juang, Chem. Eng. J., 2007, 129, 51–65 CrossRef CAS.
- J. Fock, L. K. Bogart, D. González-Alonso, J. I. Espeso, M. F. Hansen, M. Varón, C. Frandsen and Q. A. Pankhurst, J. Phys. D: Appl. Phys., 2017, 50, 265005 CrossRef.
- L. M. Rossi, N. J. Costa, F. P. Silva and R. Wojcieszak, Green Chem., 2014, 16, 2906–2933 RSC.
- E. Tombácz, R. Turcu, V. Socoliuc and L. Vékás, Biochem. Biophys. Res. Commun., 2015, 468, 442–453 CrossRef PubMed.
- K. Zhu, Y. Ju, J. Xu, Z. Yang, S. Gao and Y. Hou, Acc. Chem. Res., 2018, 51, 404–413 CrossRef CAS PubMed.
- L. H. Reddy, J. L. Arias, J. Nicolas and P. Couvreur, Chem. Rev., 2012, 112, 5818–5878 CrossRef CAS PubMed.
- S. Gul, S. B. Khan, I. U. Rehman, M. A. Khan and M. I. Khan, Front. Mater., 2019, 6, 1–15 CrossRef.
- C. C. Berry, J. Phys. D: Appl. Phys., 2009, 42, 224003 CrossRef.
- C. L. Harman, M. A. Patel, S. Guldin and G. L. Davies, Curr. Opin. Colloid Interface Sci., 2019, 39, 173–189 CrossRef CAS.
- H. Lee, E. Sun, D. Ham and R. Weissleder, Nat. Med., 2008, 14, 869–874 CrossRef CAS PubMed.
- F. Bertoli, G. L. Davies, M. P. Monopoli, M. Moloney, Y. K. Gun'ko, A. Salvati and K. A. Dawson, Small, 2014, 10, 3307–3315 CrossRef CAS PubMed.
- A. M. King, C. Bray, S. C. Hall, J. C. Bear, L. K. Bogart, S. Perrier and G.-L. Davies, J. Colloid Interface Sci., 2020, 579, 401–411 CrossRef CAS PubMed.
- L. Ternent, D. A. Mayoh, M. R. Lees and G. L. Davies, J. Mater. Chem. B, 2016, 4, 3065–3074 RSC.
- J. H. Lee, Y. M. Huh, Y. W. Jun, J. W. Seo, J. T. Jang, H. T. Song, S. Kim, E. J. Cho, H. G. Yoon, J. S. Suh and J. Cheon, Nat. Med., 2007, 13, 95–99 CrossRef CAS PubMed.
- R. Qiao, C. Yang and M. Gao, J. Mater. Chem., 2009, 19, 6274–6293 RSC.
- I. Sharifi, H. Shokrollahi and S. Amiri, J. Magn. Magn. Mater., 2012, 324, 903–915 CrossRef CAS.
- Y. Xianyu, Q. Wang and Y. Chen, TrAC, Trends Anal. Chem., 2018, 106, 213–224 CrossRef CAS.
- W. Wu, Z. Wu, T. Yu, C. Jiang and W. S. Kim, Sci. Technol. Adv. Mater., 2015, 16, 23501 CrossRef PubMed.
- S. Sun, H. Zeng, D. B. Robinson, S. Raoux, P. M. Rice, S. X. Wang and G. Li, J. Am. Chem. Soc., 2004, 126, 273–279 CrossRef CAS PubMed.
- H. C. Roth, S. P. Schwaminger, M. Schindler, F. E. Wagner and S. Berensmeier, J. Magn. Magn. Mater., 2015, 377, 81–89 CrossRef CAS.
- A. Lak, F. Ludwig, J. M. Scholtyssek, J. Dieckhoff, K. Fiege and M. Schilling, IEEE Trans. Magn., 2013, 49, 201–207 Search PubMed.
- W. Baaziz, B. P. Pichon, S. Fleutot, Y. Liu, C. Lefevre, J. M. Greneche, M. Toumi, T. Mhiri and S. Begin-Colin, J. Phys. Chem. C, 2014, 118, 3795–3810 CrossRef CAS.
- Z. R. Stephen, F. M. Kievit and M. Zhang, Mater. Today, 2011, 14, 330–338 CrossRef CAS PubMed.
- S. M. Dadfar, K. Roemhild, N. I. Drude, S. von Stillfried, R. Knüchel, F. Kiessling and T. Lammers, Adv. Drug Delivery Rev., 2019, 138, 302–325 CrossRef CAS PubMed.
- M. M. Goswami, Sci. Rep., 2016, 6, 1–10 CrossRef PubMed.
- J. Park, K. An, Y. Hwang, J. E. Park, H. J. Noh, J. Y. Kim, J. H. Park, N. M. Hwang and T. Hyeon, Nat. Mater., 2004, 3, 891–895 CrossRef CAS PubMed.
- H. L. Ding, Y. X. Zhang, S. Wang, J. M. Xu, S. C. Xu and G. H. Li, Chem. Mater., 2012, 24, 4572–4580 CrossRef CAS.
- K. Katagiri, M. Narahara, K. Sako and K. Inumaru, J. Sol-Gel Sci. Technol., 2017, 84, 110–117 CrossRef CAS.
- Q. Ran, Y. Xiang, Y. Liu, L. Xiang, F. Li, X. Deng, Y. Xiao, L. Chen, L. Chen and Z. Li, Sci. Rep., 2015, 5, 1–15 Search PubMed.
- T. Liu, R. Bai, H. Zhou, R. Wang, J. Liu, Y. Zhao and C. Chen, RSC Adv., 2020, 10, 7559–7569 RSC.
- A. L. Morel, S. I. Nikitenko, K. Gionnet, A. Wattiaux, J. Lai-Kee-Him, C. Labrugere, B. Chevalier, G. Deleris, C. Petibois, A. Brisson and M. Simonoff, ACS Nano, 2008, 2, 847–856 CrossRef CAS PubMed.
- T. Liu, R. Bai, H. Zhou, R. Wang, J. Liu, Y. Zhao and C. Chen, RSC Adv., 2020, 10, 7559–7569 RSC.
- B. K. Sodipo and A. A. Aziz, J. Magn. Magn. Mater., 2016, 416, 275–291 CrossRef CAS.
- K. Woo, J. Hong and J. P. Ahn, J. Magn. Magn. Mater., 2005, 293, 177–181 CrossRef CAS.
- M. Kokate, K. Garadkar and A. Gole, J. Mater. Chem. A, 2013, 1, 2022–2029 RSC.
- L. Zhang, W. F. Dong and H. B. Sun, Nanoscale, 2013, 5, 7664–7684 RSC.
- S. Wang, J. Tang, H. Zhao, J. Wan and K. Chen, J. Colloid Interface Sci., 2014, 432, 43–46 CrossRef CAS PubMed.
- M. Chellappa and U. Vijayalakshmi, Mater. Today: Proc., 2019, 9, 371–379 CAS.
- G. De Crozals, R. Bonnet, C. Farre and C. Chaix, Nano Today, 2016, 11, 435–463 CrossRef CAS.
- G. S. Demirer, A. C. Okur and S. Kizilel, J. Mater. Chem. B, 2015, 3, 7831–7849 RSC.
- S. L. Pinho, G. A. Pereira, P. Voisin, J. Kassem, V. Bouchaud, L. Etienne, J. A. Peters, L. Carlos, S. Mornet, C. F. Geraldes, J. Rocha and M. H. Delville, ACS Nano, 2010, 4, 5339–5349 CrossRef CAS PubMed.
- Y. Lu, Y. Yin, B. T. Mayers and Y. Xia, Nano Lett., 2002, 2, 183–186 CrossRef CAS.
- D. Ma, J. Guan, F. Normandin, S. Dénommée, G. Enright, T. Veres and B. Simard, Chem. Mater., 2006, 18, 1920–1927 CrossRef CAS.
- C. Atila Dinçer, N. Yıldız, N. Aydoğan and A. Çalımlı, Appl. Surf. Sci., 2014, 318, 297–304 CrossRef.
- N. Z. Knežević, E. Ruiz-Hernández, W. E. Hennink and M. Vallet-Regí, RSC Adv., 2013, 3, 9584–9593 RSC.
- M. Ménard, F. Meyer, C. Affolter-Zbaraszczuk, M. Rabineau, A. Adam, P. D. Ramirez, S. Bégin-Colin and D. Mertz, Nanotechnology, 2019, 30, 174001 CrossRef PubMed.
- S. A. McCarthy, G. L. Davies and Y. K. Gun'ko, Nat. Protoc., 2012, 7, 1677–1693 CrossRef CAS PubMed.
- Y. A. Barnakov, M. H. Yu and Z. Rosenzweig, Langmuir, 2005, 21, 7524–7527 CrossRef CAS PubMed.
- M. H. R. Farimani, N. Shahtahmasebi, M. Rezaee Roknabadi, N. Ghows and A. Kazemi, Phys. E, 2013, 53, 207–216 CrossRef CAS.
- A. P. Philipse, M. P. Van Bruggen and C. Pathmamanoharan, Langmuir, 1994, 10, 92–99 CrossRef CAS.
- D. Yang, J. Hu and S. Fu, J. Phys. Chem. C, 2009, 113, 7646–7651 CrossRef CAS.
- Y. H. Deng, C. C. Wang, J. H. Hu, W. L. Yang and S. K. Fu, Colloids Surf., A, 2005, 262, 87–93 CrossRef CAS.
- Z. Z. Xu, C. C. Wang, W. L. Yang and S. K. Fu, J. Mater. Sci., 2005, 40, 4667–4669 CrossRef CAS.
- D. K. Yi, S. T. Selvan, S. S. Lee, G. C. Papaefthymiou, D. Kundaliya and J. Y. Ying, J. Am. Chem. Soc., 2005, 127, 4990–4991 CrossRef CAS PubMed.
- F. Chen, W. Bu, Y. Chen, Y. Fan, Q. He, M. Zhu, X. Liu, L. Zhou, S. Zhang, W. Peng and J. Shi, Chem. – Asian J., 2009, 4, 1809–1816 CrossRef CAS PubMed.
- D. K. Yi, S. S. Lee, G. C. Papaefthymiou and J. Y. Ying, Chem. Mater., 2006, 18, 614–619 CrossRef CAS.
- L. Jia and Y. Kitamoto, Electrochim. Acta, 2015, 183, 148–152 CrossRef CAS.
- J. Wang, Z. H. Shah, S. Zhang and R. Lu, Nanoscale, 2014, 6, 4418–4437 RSC.
- L. Abraham, T. Thomas and M. Pichumani, J. Colloid Interface Sci., 2019, 547, 234–244 CrossRef CAS PubMed.
- F. Arriagada and K. Osseo-Asare, J. Colloid Interface Sci., 1999, 211, 210–220 CrossRef CAS PubMed.
- M. Zhang, B. L. Cushing and C. J. O'Connor, Nanotechnology, 2008, 19, 085601 CrossRef PubMed.
- M. Stjerndahl, M. Andersson, H. E. Hall, D. M. Pajerowski, M. W. Meisel and R. S. Duran, Langmuir, 2008, 24, 3532–3536 CrossRef CAS PubMed.
- F. Jiang, Y. Fu, Y. Zhu, Z. Tang and P. Sheng, J. Alloys Compd., 2012, 543, 43–48 CrossRef CAS.
- P. Tartaj and C. J. Serna, Chem. Mater., 2002, 14, 4396–4402 CrossRef CAS.
- G. L. Davies, A. Barry and Y. K. Gun'ko, Chem. Phys. Lett., 2009, 468, 239–244 CrossRef CAS.
- V. Czitrom, The American Statistician, 1999, 53, 126–131 Search PubMed.
- J. Li, Y. Qiao and Z. Wu, J. Controlled Release, 2017, 256, 9–18 CrossRef CAS PubMed.
- A. Lak, F. Ludwig, I. M. Grabs, G. Garnweitner and M. Schilling, AIP Conf. Proc., 2010, 1311, 224–230 CrossRef CAS.
- M. G. Arafa and B. M. Ayoub, Sci. Rep., 2017, 7, 1–15 CrossRef PubMed.
- N. D. Burrows, S. Harvey, F. A. Idesis and C. J. Murphy, Langmuir, 2017, 33, 1891–1907 CrossRef CAS PubMed.
- S. David, H. Marchais, K. Hervé-Aubert, D. Bedin, A. S. Garin, C. Hoinard and I. Chourpa, Int. J. Pharm., 2013, 454, 660–667 CrossRef CAS PubMed.
- L. Zhang and S. Mao, Asian J. Pharm. Sci., 2017, 12, 1–8 CrossRef PubMed.
- J. Salazar, O. Heinzerling, R. H. Müller and J. P. Möschwitzer, Int. J. Pharm., 2011, 420, 395–403 CrossRef CAS PubMed.
- S. Sood, K. Jain and K. Gowthamarajan, Colloids Surf., B, 2014, 113, 330–337 CrossRef CAS PubMed.
- R. N. Núñez, A. V. Veglia and N. L. Pacioni, Microchem. J., 2018, 141, 110–117 CrossRef.
- M. Ghanimati, M. Abdoli Senejani, T. M. Isfahani and M. A. Bodaghifard, Appl. Organomet. Chem., 2018, 32, 1–14 CrossRef.
- M. M. Pakulska, K. Vulic, R. Y. Tam and M. S. Shoichet, Adv. Mater., 2015, 27, 5002–5008 CrossRef CAS PubMed.
- E. Chiesa, R. Dorati, T. Modena, B. Conti and I. Genta, Int. J. Pharm., 2018, 536, 165–177 CrossRef CAS PubMed.
- SAS Institute Inc., JMP.
- C. A. Schneider, W. S. Rasband and K. W. Eliceiri, Nat. Methods, 2012, 9, 671–675 CrossRef CAS PubMed.
- N. Mac Fhionnlaoich and S. Guldin, Chem. Mater., 2020, 32, 3701–3706 CrossRef CAS PubMed.
- T. Q. Bui, S. N. C. Ton, A. T. Duong and H. T. Tran, J. Sci.: Adv. Mater. Dev., 2018, 3, 107–112 Search PubMed.
- P. Tartaj and C. J. Serna, Chem. Mater., 2002, 14, 4396–4402 CrossRef CAS.
- Y. Ding, P. D. Howes and A. J. DeMello, Anal. Chem., 2020, 92, 132–149 CrossRef CAS PubMed.
Footnotes |
† Electronic supplementary information (ESI) available: Tables and figures containing DoE experimental factors and response variables, lack of fit test for population of SPION@SiO2, SPION TEM and χm, TEM images at Level 2 TEOS, TEM images at Level 3 TEOS, TEM at treatment 222, box-plot of size distribution of the measured NP diameter from TEM analysis (dTEM) of all treatments, response values of centre point conditions, size distribution of validation experiments, mass susceptibility (χm) of all treatments. See DOI: https://doi.org/10.1039/d2ma00369d. Datasets are available open access, see DOI: https://doi.org/10.5522/04/20014877.v1 |
‡ APC Ltd., Building 11, Cherrywood Business Park, Loughlinstown, Dublin, D18 DH50, Ireland. |
§ Current address: Department of Chemical Engineering and Analytical Sciences, University of Manchester, The Mill, Manchester, M1 3AL. |
|
This journal is © The Royal Society of Chemistry 2022 |