Targeting current and future threats: recent methodological trends in environmental antimicrobial resistance research and their relationships to risk assessment
Received
31st January 2022
, Accepted 7th June 2022
First published on 26th July 2022
Abstract
Antimicrobial resistance (AMR) is a growing public health threat. Improved surveillance of AMR's genetic indicators in environmental reservoirs should lead to a more comprehensive understanding of the problem at a global scale, as with SARS-CoV-2 monitoring in sewage. However, the “best” monitoring approach is unclear. Some scientific works have emphasized monitoring for the abundance of already-known antimicrobial resistance genes (ARGs); others have emphasized monitoring for the potential of new ARGs to arise. The goal of this study was to examine which methods were employed by highly-cited papers studying AMR in environmental engineering and agricultural systems, thus providing insight into current and future methodological trends for monitoring ARGs. We searched recent (2018–2020) literature documenting AMR in five environmental matrices: wastewater, surface water, drinking water, stormwater, and livestock manure. We selected the most highly-cited papers across these matrices (89 papers from 17
809 initial results) and categorized them as using targeted methods (e.g., qPCR), non-targeted methods (e.g., shotgun metagenomics), or both. More than 80% of papers employed targeted methods. Only 33% employed non-targeted methods, and the use of targeted versus non-targeted methods varied by environmental matrix. We posit that improving AMR surveillance in environmental reservoirs requires assessing risk, and that different monitoring approaches imply different objectives for risk assessment. Targeted methods are appropriate for quantifying known threats, particularly in environmental matrices where direct human exposure is likely (e.g., drinking water). However, long-term studies employing non-targeted methods are needed to provide an understanding of how frequently new threats (i.e., novel ARGs) arise.
Water impact
The majority of recent impactful research on environmental reservoirs of antimicrobial resistance has used targeted methods (e.g. qPCR) to quantify known antibiotic resistance genes (ARGs). To advance the field of antimicrobial resistance risk assessment, non-targeted methods (e.g. metagenomics) in longer time-scale studies are needed to determine the frequency by which new ARGs arise.
|
1. Introduction
Antimicrobial resistance (AMR) is a global health threat which may result in a financial burden of approximately 100 trillion USD and over 10 million deaths by 2050.1 AMR manifests as antimicrobial resistant bacteria (ARB) in clinical infections and is mediated at the genetic level by antimicrobial resistance genes (ARGs). ARGs are naturally occurring,2,3 with human activity contributing to their increased abundance and diversity worldwide,4,5 such that ARGs (and the ARB that carry them) are regarded as environmental pollutants.6 Thus, both are ubiquitous in the environment and can be transferred among humans, animals, and environmental media (e.g., water, air, and soil). Consequently, antimicrobial resistance requires a global “One Health” response – a response that considers the intersection of human, animal, and environmental health systematically.7,8,155
This response must be guided by public health data. That is, the occurrence of antimicrobial resistant infections in humans ideally should be linked to each of the One Health sectors and, moreover, to the various human activities within those sectors that drive increasing resistance (e.g., antibiotic use in human medicine and livestock agriculture). Understanding these links in detail would inform strategies for mitigating the impact of human activities on antimicrobial resistance. However, this information is largely lacking because the individual relationships of interest represent small effects in a complex real-world system. Studying them empirically would require enormous numbers of human subjects enrolled in observational studies with little to no control over the relevant environmental factors. Thus, the epidemiological studies required to document links between environmental antibiotic resistance and human health are prohibitively large and expensive.
As a result, scientific and regulatory leaders have proposed risk assessment as an alternative.7,9–11 Risk assessment represents a predictive modeling approach, and as such, its strengths relative to the empirical measurements of epidemiology are feasibility and flexibility. Risk assessment can be carried out with limited data to assess emerging hazards, so long as its interpretation is tempered by appropriate acknowledgement of those limitations. Risk assessment can be conducted prospectively in order to project the effects of multiple risk mitigation strategies before they are implemented. Risk assessment also allows for synthesis of otherwise disparate information for the purpose of balancing competing risks, and by extension, the competing interests of different One Health sectors.
2. Paradigms for risk assessment of antimicrobial resistance
In our view, two distinct paradigms have emerged with respect to framing risk assessment for antimicrobial resistance. Adopting the language of Zhang et al., the first of these is concerned with estimating risk for current threats, i.e., hazards that are already known to exist.12 The second is concerned with estimating risk for future threats, i.e., novel and unknown hazards that have yet to emerge.12 These represent profoundly different objectives for risk assessment and can be explained by analogy with the current COVID-19 pandemic. In that case, current threats would relate to the risk of infection from a known variant of SARS-CoV-2, whereas future threats would relate to the risk of a new variant emerging. Both types of risk are important considerations for formulating public health policy, but each requires its own approach to risk assessment.
These two paradigms effectively simplify the wide variety of specific approaches and priorities that have been proposed by others for AMR risk assessment. Moreover, we see close analogs of these two ideas expressed repeatedly in prior works. They are expressed clearly in Finley et al.'s distinction between ancient and “new” antibiotic resistance genes,13 in Bengtsson-Palme and Larsson's reference to known resistance genes and novel resistance determinants,14 and in Larsson and Flach's delineation of transmission events versus evolutionary events.15 More generally, we interpret these previous authors' terminology as converging on the same principles underlying Zhang et al.'s concepts of current and future AMR threats, such that their framework seems broadly applicable.
We also argue that the dichotomy of current threats versus future threats relates to the analytical methods used in quantifying hazards during exposure assessment. With respect to ARGs, exposure to current threats can be estimated readily provided enough empirical data are available to characterize their circulation in a population. More specifically, current threats have known nucleic acid sequences. They can therefore be targeted via methods based on polymerase chain reaction (PCR) to estimate their absolute abundance in a given environment, and abundance can be used to index risk. On the other hand, exposure to future threats depends on a very different set of criteria, like the nature of relevant environmental matrices, microbial diversity and density, and the selective pressure(s) involved. Furthermore, the nucleic acid sequences of future threats cannot be targeted because they are as-yet unknown. We therefore propose that the diversity of ARGs in a given environment can be used to index risk for future threats. That is, we assume the more ARGs an individual is exposed to, the higher the risk is that they are exposed to a novel ARG that has yet to emerge on a wider scale.
Finally, we note that diversity can be estimated only using open-ended methods, in which the ARGs present in a given environmental compartment are not necessarily known a priori (e.g., shotgun metagenomics). Thus, we argue that the distinction between current and future threats for ARGs also corresponds to an analogous distinction between targeted and non-targeted molecular methods.
The goal of this perspective was to determine how recent and influential studies have characterized ARGs in environmental media. We sought out highly cited papers from 2018–2020 across five different environmental matrices and examined how the use of these methods aligned with the current threat versus future threat paradigm for risk assessment. We identified several PCR-based approaches (e.g., qPCR, ddPCR, and HT-qPCR) as examples of targeted analytical methods for detecting current threats, and we identified metagenomics as a non-targeted method for potentially identifying future threats. Specifically, we aimed to 1) identify which methods were used in each study, 2) identify discrepancies in methods used across environmental matrices, 3) assess how these methods fill knowledge gaps in risk assessment for antimicrobial resistance, and 4) propose research priorities to address risk assessment for antimicrobial resistance more effectively in the future. We focused on drinking water, wastewater, groundwater, stormwater, and livestock manure because these environmental compartments each represent semi-independent points that could be managed to alter the impacts of antimicrobial resistance on the global One Health scale.
3. Literature review approach
3.1. Literature identification
Our goal in identifying relevant literature was to obtain a current “snapshot” of high-impact ARG studies in the environmental field. Thus, we made no attempt to conduct a comprehensive or detailed literature review. Instead, we designed our initial search to be broad, quickly implemented, and easily reproduced. On April 24, 2021 we searched for the terms “(antibiotic OR antimicrobial) resist* gene” on Scopus (Fig. 1). This initial search returned 83
400 documents. These were restricted further by limiting to the 3 most recent complete years at the time of the search (i.e., 2018–2020) and by limiting to “articles” only (i.e., reviews, letters, conference papers, and other Scopus document types were excluded). These additional restrictions reduced the search to 17
809 documents (Fig. 1).
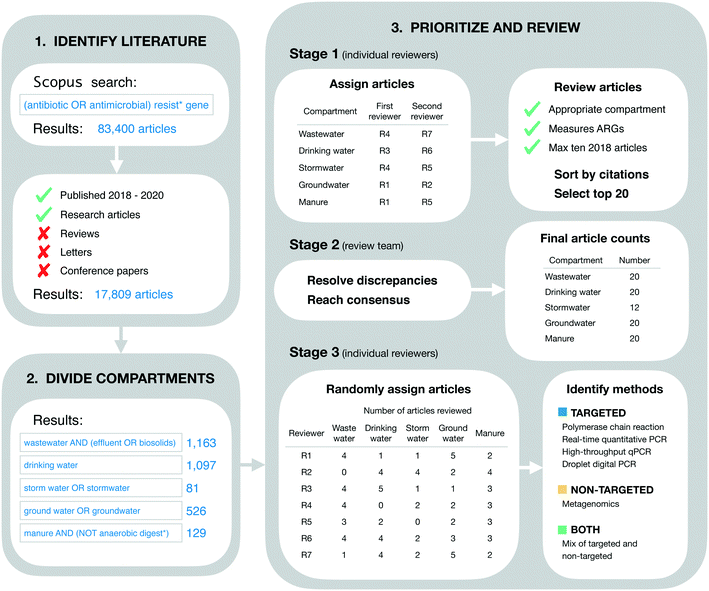 |
| Fig. 1 Methodological approach for conducting literature review. | |
3.2. Restriction and sub-division by environmental compartment
The literature search was restricted further to include only those documents addressing five environmental compartments of interest, which we refer to colloquially as wastewater, drinking water, stormwater, groundwater, and livestock manure. More formally, these compartments were defined using the Scopus search terms “wastewater AND (effluent OR biosolids)” (1163 documents), “drinking water” (1097 documents), “storm water OR stormwater” (81 documents), “ground water OR groundwater” (526 documents), and “manure AND (NOT anaerobic digest*)” (129 documents), respectively (Fig. 1). These search terms were applied within the group of 17
809 documents referred to in the previous section.
Our five environmental compartments were selected because they represent potential points of control for managing AMR in the environment. Additionally, consideration of distinct environmental compartments allowed for comparison of research practices in sub-topics of the environmental ARG research field. It is noted these compartments are high level and that subsets within these compartments could be parsed out further. The drinking water studies, for example, included samples from source waters, treatment processes within a drinking water treatment plant, and finished tap water, all of which can have different antimicrobial resistance threats, exposure risks, and interactions with the microbial community. Hospital and industrial effluents feed into municipal wastewater treatment plants and are incorporated under the wastewater umbrella because municipal wastewater treatment plants collect the diverse inputs into municipal sewers.
3.3. Document prioritization and review process
Documents were prioritized within each environmental compartment based on total citations. More specifically, documents were sorted on total citations (as reported in the Scopus database), then reviewed for inclusion in order from most to least citations. Our rationale for this approach was two-fold. First, we sought to include a maximum of 20 documents from each environmental compartment in order to expedite our review process. Second, we assumed that total citations were an appropriate measure of impact across scholarly works. That is, we assumed that the more a given paper has been cited by other works, the more relevant and influential it is for the environmental ARG research field as a whole. We understand this is not strictly the case as articles can be cited for many different reasons, but this approach is reproducible.
The review process was structured in three stages. In the first stage, the full list of documents for each environmental compartment was reviewed independently by two of the authors for inclusion. The review in this stage was based on article titles and abstracts alone, and inclusion criteria were general. Specifically, it was verified that the article did in fact address the compartment of interest (based on whether or not the authors reported collecting samples from that compartment), and it was verified that ARGs were measured as one of the variables in the study (using any analytical approach and/or method). Additionally, reviewers were instructed to include a maximum of 10 articles from 2018, since the extra time in publication for these articles could result in more citations, and we sought to avoid biasing our results towards those older documents. Each of the two independent reviewers for each environmental compartment produced their own list of “top 20” articles for that compartment, which were forwarded on to the lead author (TRB) for the second stage of review.
In the second stage, each pair of lists for each environmental compartment were compared to identify discrepancies. Discrepancies were resolved by the lead author in consultation with co-authors and based on further review of the articles in question as necessary (e.g., by reviewing materials and methods sections to clarify the nature of sampling and/or analytical approach used). This stage of review produced five consensus lists, one for each environmental compartment. The lists for wastewater, livestock manure, drinking water, and groundwater each contained the target number of 20 documents, while the stormwater list contained only 12 (see Results for additional detail). These five lists were combined into a single list of 89 documents for the final stage of review (three documents were shared between compartments as discussed in section 4.1).
In the final stage, all 89 documents were reviewed in further detail to extract information of interest for the current study. In particular, the methods section of each document was reviewed in detail to identify the analytical method(s) used: polymerase chain reaction (PCR), real-time quantitative PCR (qPCR), high-throughput qPCR (HT-qPCR), droplet digital PCR (ddPCR), and/or metagenomic analysis. Documents reporting the use of PCR, qPCR, HT-qPCR, and/or ddPCR (but not metagenomics) were classified as using “targeted” methods. Documents reporting the use of metagenomics (but not any of the PCR-based approaches) were classified as using a “non-targeted” method. Documents reporting a mix of targeted and non-targeted methods were classified as “both.” Furthermore, post hoc assessment of our search results revealed a small number of studies using approaches that we had not initially anticipated and, rather than exclude these studies, we made the following assignments: isolation on selective media followed by PCR screen (n = 3; targeted), functional gene arrays (n = 2; targeted), and isolation on selective media followed by genomic sequencing and functional annotation (n = 2; non-targeted). Review assignments for this stage were randomized with respect to those in the first stage, such that reviewers were likely to screen papers other than those they had already reviewed in the first stage. For a full process overview, see Fig. 1.
4. Results and discussion
4.1. Characteristics of reviewed documents
Overall, we reviewed a total of 89 documents, 11 less than the maximum possible with our inclusion criteria. The discrepancy was due to a shortfall in the stormwater compartment. More specifically, the groundwater,16–35 livestock manure,36–55 drinking water,56–75 and wastewater25,57,76–93 compartments each included the target number of 20 documents, while the stormwater (n = 12)26,94–104 compartment did not have enough documents meeting our criteria (Fig. 1). This finding suggests that stormwater might be under-studied relative to the other four compartments. Additionally, three documents were shared between compartments, one between the groundwater and wastewater compartments,25 one between the groundwater and stormwater compartments,26 and one between the drinking water and wastewater compartments.57
These 89 documents were highly cited. The average citation count per document was 14.0 citations per year (from 2018–2020). Citation counts also varied by environmental compartment, from a minimum of 3.9 average citations per document per year (stormwater) to a maximum of 31.8 average citations per document per year (wastewater). These high citation counts are consistent with our approach to literature identification and review. We intentionally prioritized highly-cited documents in order to expedite our review process while maintaining focus on the most influential works in the field. Furthermore, variation in citation counts across the environmental matrix likely reflects varying publication practices and varying sizes of the research communities in different sub-fields of environmental AMR research. This finding emphasizes the importance of our decision to sub-divide the literature by environmental compartment in order to control for these differences.
4.2. Methods used in reviewed documents
The primary focus of our literature review was on categorizing documents with respect to the methods they employed for analyzing ARGs. Studies reporting use of PCR, qPCR, HT-qPCR, and/or ddPCR were classified as using “targeted” approaches, while studies reporting use of metagenomics were classified as using a “non-targeted” approach (“both” was an additional category and ad hoc designations were made for less common analytical methods, see section 3.3). Before this work commenced, we hypothesized that metagenomics would be the predominant method employed across recent impactful papers. Metagenomics is a newer approach and provides more information on the types of genes present, and we assumed that the most recent method would appear in the most recent documents. However, a majority of documents in our final results employed a targeted method. Overall, 85% of documents were classified as using a targeted approach or both targeted and non-targeted approaches together, while only 33% employed metagenomics or metagenomics with a targeted approach (Fig. 2).
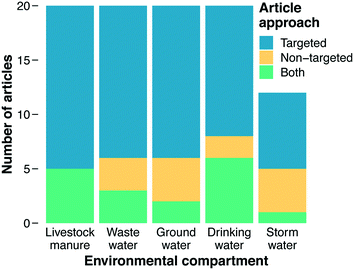 |
| Fig. 2 AMR methods employed by environmental compartment. | |
Several factors may account for this finding, the first of which relates to utility and feasibility. For example, the PCR-based analytical methods underlying the targeted category are almost certainly more affordable and analytically accessible than metagenomics. Thus, it could be that researchers implicitly (or even explicitly) regard quantification of current threats as more feasible than quantification of future threats. Alternatively, it could be that researchers regard quantification of current threats as more useful than quantification of future threats because the current threats are more immediate. For example, at least some ARGs are known because they have already emerged and caused harm in a clinical setting.105,106 Thus, these current threats present a higher immediate risk than future threats that have yet to emerge.
4.3. Variation among approaches across environmental matrices
Varying trends in the methods used to characterize AMR in environmental matrices were found (Fig. 2). In each environmental matrix at least half or more of the studies used targeted methods. The distribution of methods for stormwater was notable compared to other compartments, with the combination of non-targeted and both comprising 42% of the methods, the largest percentage of any of the matrices. As an under-studied field of research, a wider breadth of studies with varying methods could indicate that the field of stormwater research has yet to define a consistent technique for AMR characterization, whereas other fields have (e.g., manure and wastewater). Stormwater AMR is also a newer area of research in comparison to manure and wastewater which raises the question: are appropriate methods selected to address a question, or instead, are standard methods used in hopes of finding an answer to a yet-to-be defined question? Livestock manure and wastewater had the highest counts for targeted approaches. This result indicates that the priority of these fields is quantifying what we know and highlighting that quantification is important for surveillance and understanding how treatment systems work. A crucial component of surveillance, however, is identification of new genes for which non-targeted approaches are required.
Drinking water could also be viewed as a growing field in antibiotic resistance research. The metagenomic fraction (i.e., non-targeted plus both) was larger in drinking water (40%) than in wastewater, manure, or groundwater. Metagenomics could enable researchers to determine if disinfection impacts the diversity of AMR in drinking water as well as if selective pressures are being exerted. As discussed in section 4.8, time-series studies that collect samples from the same location over time could shed light on the role of selective pressures. These questions are also relevant to wastewater and manure where dense biomass and the selective pressures from different pollutants could be generating hotspots for horizontal gene transfer and resistome diversification. Thus, future directions for wastewater and manure should increase focus on metagenomics to answer these crucial questions. Moreover, only one of the 20 wastewater papers sampled biosolids. Wastewater effluent is a hotter topic than wastewater biosolids, but biosolids are also high biomass samples that should be given more attention in the future.
4.4. Implications of using targeted versus non-targeted methods for risk assessment
The framework of current and future threats relates directly to several important principles underlying the practice of risk assessment. These include the very definition of risk itself, as well as the general procedure involved in producing risk estimates. By definition, risk is formally identified as the product of two distinct quantities – 1) the probability of exposure to a hazard and 2) the damage caused once exposed.107 Procedurally, risk assessment consists of four steps: 1) hazard identification, 2) hazard characterization (a.k.a., dose–response assessment), 3) exposure assessment, and 4) risk characterization.108,109 Thus, recognizing the terms “threat” and “hazard” as synonyms, the current threat versus future threat paradigm relates directly to the nature of hazards for which probabilities and damage are quantified as well as to the foundational steps of any subsequent risk assessments carried out.
In this work, we have argued that current threats and future threats correspond to targeted and non-targeted analytical methods, respectively. The relative use of targeted versus non-targeted methods varies across the environmental compartments reviewed here, with targeted methods predominating overall. Both methods have value, but they have different implications for risk assessment. Resolving the question of which method(s) should be used moving forward requires one to consider the nature of the environmental compartment under study. More specifically, we find it important to ask two related questions with respect to risk assessment for AMR in any specific environmental compartment:
1. Is this compartment a source where new ARGs and/or ARB commonly/frequently emerge?
2. Is this compartment an immediate point of human exposure for ARGs and/or ARB?
In other words, we propose that different environmental compartments correspond to different types of hazards. For compartments where the answer to question 1 is “yes”, these would be categorized as future threats. For compartments where the answer to question 2 is “yes”, these would be categorized as current threats. More generally, with questions 1 and 2 answered for a given environmental compartment, appropriate methods can be selected to inform risk assessment. Admittedly, question 1 is very difficult to answer. Further work is needed to understand the reservoir of resistance genes and their stability over time and among locations within each environmental compartment. This gap in understanding also attests to the need for studying and identifying future threats across compartments. As this knowledge grows and we answer question 1 for each compartment we can better understand how to mitigate risks and stop the spread of novel AMR.
We believe livestock manure and wastewater are more likely sources of new ARGs and/or ARB due to their high genetic diversity, high biomass concentrations, and presence of chemical stressors. Thus, they would represent future threats under the paradigm proposed here, and non-targeted approaches would be the appropriate tool to employ. Research supports that animals can be reservoirs of new, unknown ARGs.110 In contrast, drinking water and groundwater are immediate points of human exposure for ARGs and ARB because large populations consume them on a daily basis.111,112 Thus, they would represent current threats, and targeted methods might be prioritized in these compartments to assess risk. Finally, stormwater crosses both categories. It represents an immediate point of human exposure to the extent it impacts recreational water, and it also can be mixed with sanitary sewage, metals, and other pollutants, thereby producing potential for emergence of new ARGs and/or ARB.
4.5. Strengths and limitation of targeted vs. non-targeted approaches
There are a few critical differences in the conclusions that can be drawn from taking a targeted quantitative PCR approach versus a non-targeted metagenomics approach. Targeted approaches can measure absolute abundances of ARGs and are particularly suited for time-series analyses that can inform about developing risks as ARGs and ARB increase. The limitation to targeted approaches is that they typically identify a narrow range of targets and are designed to match already known resistance genes. In contrast, non-targeted metagenomics approaches require no prior information and can instead detect any gene present as long as the depth of sequencing is adequate113,114 and the functional annotations are accurate.115,116 That is, qPCR is limited to examination of “current threats”, whereas metagenomics provides the opportunity to identify future threats. Primary limitations to the non-targeted approach include the cost of sequencing as well as available expertise for appropriately analyzing samples. Additionally, non-targeted approaches do not provide absolute quantification as readily as qPCR can provide. Ideally in all compartments, metagenomic sequencing would provide information about the diversity and relative abundances of the ARGs and ARBs and that information could then be used to follow up with targeted approaches to quantify changing absolute abundances.
Metagenomics also allows for a more nuanced hazard identification, i.e., metagenomics can identify resistance genes that currently do exist but have not yet been identified by researchers. Longitudinal metagenomics can help understand the relative abundance of resistance alleles that arise through de novo mutation that are not typically detected in qPCR. Examples of this include loss-of-function mutations in negative regulators of efflux pumps that can cause cross-resistance to many antibiotics and the S81L mutation in DNA gyrase conferring resistance to fluoroquinolones.117–120 Surveillance with metagenomics methods can detect emerging resistance genes, including selective sweeps of alleles in a population.121–123 Other examples of current, yet unidentified risks that can be identified when shotgun sequences are analyzed include ARGs that were repurposed from other functions, newly mobilizable ARGs that were introduced onto unexpected plasmids or phage genomes, epistatically controlled ARGs where the genetic background unlocked new features, and pleiotropic ARGs.124–128 Metagenomics provides genomic context to the ARGs. Genome assemblies and annotations can predict if the ARGs are located on a chromosome, plasmid, resistance island, prophage, or other mobile genetic elements (MGEs).129–131 Accurate taxonomic assignment of the ARG-harboring microbe can also help inform risks. A chromosomally-encoded ARG from a non-pathogenic environmental isolate should be considered lower risk than an ARG on a MGE in an opportunistic pathogen. All of these nuances can and should influence risk assessments relating to ARGs and possible human exposure from environmental sources.
4.6. The role of genetic elements in characterizing AMR threats
MGEs are DNA regions that specialize in moving within and between genomes. These elements include plasmids, prophage, transposons, integrons, insertion sequences, and integrative conjugative elements. In most environments, MGEs play a crucial role in moving DNA (genes) among cells, and this has resulted in both significant within-species diversity (known as the species pangenome) and gene transfer between microbial species (i.e., horizontal gene transfer). Ultimately, MGEs provide access to genetic determinants that would otherwise be unlikely to arise in a particular microbial lineage and thus accelerate the timeframe in which new combinations of antibiotic resistance can emerge. In other words, MGEs are key components in understanding future threats related to AMR and have been key components in understanding current threats.132
It is known that the widespread use of antibiotics by humans has resulted in the selection of a diverse set of antibiotic resistance determinants that are now routinely carried by a variety of mobile elements.132,133 For example, the increase in disease severity from the pathogens Pseudomonas aeruginosa and Acinetobacter baumannii was the result of resistance acquisition by mobile elements.132,134,135 Gene transfer among microbes occurs more frequently when microbial densities are highest, which often occurs in engineered systems (e.g., wastewater treatment). It is also thought that antibiotic pollution creates hotspots for the assembly of ever more complex mobile elements with greater capacities for mobilization,132 so engineered environments are primary locations for these developments.
It is clear that MGEs play a large role in the fate of ARGs both within and among environments and thus would be key in assessing ARG risks. However, it is not trivial to quantify MGE activity in situ, to categorize their relationships with ARGs, or ultimately to place them into a health risk framework. These challenges have so far limited the number of studies examining MGEs in an environmental risk framework.136 There is some evidence that the risk associated with different MGEs varies. Prophages seemingly are less likely to carry ARGs than other elements,137 and plasmids can be grouped based on their promiscuity,138 but more work is needed to make these measures useful.
As with ARGs, methodological considerations challenge assessing MGEs in an environmental context. MGEs are diverse, so it is difficult to pick meaningful targets to quantify when using PCR-based methods. By nature of being mobile, identical MGEs can be found in many different microbes, so with short-read DNA sequencing technologies, it is difficult to identify both the element and its host source. This identification is becoming easier with long-read sequencing technologies. Our results indicate that it is common for studies (63% of included papers) to quantify one or a few MGEs, the most common of which is the integron-integrase gene intI1. Interestingly, the proportion of papers that quantified MGEs varied quite a bit between matrices. For both drinking water and wastewater categories, 75% of papers investigated MGEs while only 33% of stormwater papers included them. Groundwater (50%) and manure (70%) were in the middle of the environmental compartments. MGEs relate to risk from AMR by serving as a mechanism to spread ARGs to previously non-resistant organisms, and so as MGEs increase in numbers and diversity so too does AMR.139 In this context, characterizing the quantity and/or diversity of MGEs is likely to inform on future AMR hazards, but health risk assessment is challenging because MGEs themselves are not directly harmful to human health.
4.7. Quantitative microbial risk assessment (QMRA) of AMR in the environment
In the terminology used here, published risk assessments for antimicrobial resistance to-date have focused largely on current threats. This stems from the use of quantitative microbial risk assessment (QMRA) in their approach, which requires that a specific hazard be identified. Examples include risk assessments for methicillin-resistant Staphylococcus aureus,140 fluoroquinolone-resistant Campylobacter jejuni,141,142 ceftiofur-resistant Salmonella,143 ampicillin-resistant Enterococcus faecium infections,144 quinupristin–dalfopristin resistance in Enterococcus faecium infections,145,146 macrolide resistance in both Enterococcus faecium and Campylobacter spp. infections,147,148 and fluoroquinolone and multidrug resistance in Salmonella infections.142 Notably, none of these prior QMRAs for AMR focus on the environmental compartments noted as points of exposure in section 4.4 (i.e., drinking water, groundwater), and only one focuses on the role of the environment in general. The vast majority focus on foodborne exposures. Thus, QMRA for AMR in the environment is still in its infancy, even in terms of current threats, and improved hazard identification (e.g., identifying a consensus group of high-priority AMR hazards relative to environmental transmission) could add substantially to the field's development.
Moreover, because the standard QMRA framework requires identification of specific hazards, QMRA itself is not well-suited to risk assessment for future threats. For example, the dose–response characteristics of future threats cannot be studied because the organisms and/or genes involved are, by definition, unknown. They cannot be isolated in a lab and administered in human feeding trials, as in the classic dose–response experiments used for QMRA of susceptible gastrointestinal pathogens.108 Even for current threats, dose–response models are extremely limited. We know of only two models intended specifically for ARB (for gentamicin-resistant Escherichia coli and for methicillin-resistant Staphylococcus aureus).140,149 In other cases, dose–response models would have to be extrapolated from corresponding susceptible bacteria.
More generally, and returning to the SARS-CoV-2 analogy from section 2, we find it useful to consider the impact that each new variant has had on that pandemic's trajectory. Public health measures intended to mitigate transmission of COVID have been devised and implemented in the near-complete absence of the sort of dose–response information typically used to conduct QMRA. Furthermore, the most relevant risks with respect to SARS-CoV-2 seem to stem from what we would call future threats. Will a new variant be more or less infectious than the one currently circulating? Will vaccines be more or less effective against a new variant? This observation raises an important question with respect to AMR risk assessment. Given the potential importance of future threats to the long-term impacts of AMR, is it crucial to understand the dose–response characteristics of existing “variants” of ARB and/or ARGs? Is this information the limiting factor for risk assessments of environmental AMR?
Regardless of the answer, the genetic recombination of existing ARB and ARGs is likely to be useful. To that point, we argue that understanding the genetic diversity of ARGs in various environments provides an index for potential emergence of new genotypes – the precursor event for future epidemics (i.e., future threats). The literature search we have presented here suggests that, despite the relatively long-standing establishment of techniques for metagenomics analyses in the environmental field, metagenomic approaches in recent environmental AMR studies appear to be under-represented relative to qPCR and other “targeted” methods. This is particularly true for environments where future threats might be of greatest concern, like livestock manure and municipal wastewater where high biomass concentrations and genetic diversity provide ample opportunity for the emergence of new ARGs that could threaten the usefulness of antibiotics in the future.
More generally, we propose that risk assessment for future threats could be based on a combination of data from targeted and non-targeted methods. Specifically, AMR threats can be characterized in terms of both their sources and exposure routes (e.g., see questions 1 and 2 in section 4.4). Moreover, numerous combinations of sources (e.g., livestock manure, municipal wastewater) and exposure routes (e.g., drinking water, recreational water, person-to-person contact) exist. Systematic One Health risk assessment for AMR could therefore be based on relative ranking of risk for the various source-exposure combinations of interest, with risk in sources indexed by the diversity of ARGs present and risk for exposure routes indexed by the abundance of ARGs present. The risk index for sources could be quantified using non-targeted approaches, assuming ARG diversity is proportional to the rate at which novel ARGs emerge. In contrast, the risk index for exposure routes could be quantified using targeted approaches, assuming that the abundance of ARGs transmitted per person per unit time is proportional to the rate at which a potential future threat would spread once it emerges. This approach is tractable, integrates both types of data, and lends itself to policy and management interventions at both the source and the point of exposure.
4.8. Implications on future research and the importance of time-series research
The value of using targeted versus non-targeted methods also relates to timescales and goals of a particular study. If the research goal is to identify the frequency that new ARGs arise in a compartment, then real-time monitoring using non-targeted methods will need to be employed. If the research goal is to assess treatment efficiency of ARGs, at a wastewater treatment plant (WWTP) for example, then employing targeted analysis to quantify influent and effluent ARGs over time would be required. Data processing time is also an important component. If a WWTP wanted to adjust process control parameters based on observed ARG removal, targeted methods such as qPCR can generate data in a manner of hours and be used to impact process operations if desired. Operational parameters could be tweaked and then abundance measured to determine if those changes improved ARG removal.
On the other hand, over a longer timescale of months, it would be important to know if a WWTP serves as a hotspot for the proliferation of antibiotic resistant organisms, which could contribute to the emergence of new ARGs and/or horizontal gene transfer, thereby increasing the diversity of ARB. HGT is dependent on many factors, including cell density, host and recipient phylogeny (i.e. cell structures/defenses), MGE diversity and concentration, and the stresses caused by the physical environment. WWTPs and other water infrastructure alter these factors in ways that suggest HGT and selection for new combinations of mobile elements could be elevated when compared to most natural environments, and a few studies have indicated that this is the case.150–153 In theory, a more diverse set of MGEs carried in a microbially diverse system would lead to ever-increasing access by individual organisms to a diverse gene pool and greater potential for the development of new resistant microbes of consequence to human health. The mitigating factor to this scenario of ever-increasing ARGs is the fitness cost that is often associated with acquiring and maintaining ARGs and MGEs in the absence of inhibitory concentrations of antibiotics. Thus, it is important to establish a baseline of current ARG/MGE diversity conditions for each treatment plant in order to evaluate its role in the development of new resistant microorganisms. At this time, metagenomics performed through time is the method that could deliver the necessary information. There is currently a dearth of research employing metagenomics over long time-scales within a given compartment. This dearth represents a crucial gap to fill for the advancement of risk assessment on antimicrobial resistance. Furthermore, based on emerging sequencing technologies such as Oxford Nanopore,154 in the near future long read metagenomic sequencing will become more common. These sequences will eventually be able to be read in near real-time, meaning that within hours of sampling the MGEs, ARGs, and their host organisms could be known. This approach could likely change how risk assessment is done and help fill in gaps described above about identifying current and future threats.
Future work could also look more deeply at specific niches in any of the five compartments surveyed in this paper. For instance, identification of sewers as a hotspot of threats might be managed differently than wastewater treatment effluent hotspots. Sewers could be hotspots because of environmental conditions within a sewer or because of potential hospital or industrial effluents that feed into them. Wastewater treatment effluent as a hotspot could depend on the treatment processes used at a given plant.
In this perspective, we set out to understand which methodologies have been used in recent highly-cited papers addressing AMR in environmental matrices. Based on our results, most studies implicitly focus on current AMR threats. Moving forward, however, it will be critical to refine approaches geared towards identifying the risks associated with as-yet unknown future threats.
Author credit statement
TB: conceptualization, data curation, formal analysis, investigation, methodology, project administration, supervision, writing – original draft, writing – review and editing. RN: conceptualization, data curation, formal analysis, validation, investigation, writing – original draft, writing – review and editing. LK: investigation, writing – review and editing. EL: data curation, formal analysis, investigation, visualization, writing – review and editing. KO: investigation, writing – review and editing. SMT: investigation, writing – review and editing. CM: writing – review and editing. PM: conceptualization, investigation, methodology, project administration, supervision, validation, writing – original draft, writing – review and editing.
Conflicts of interest
There are no conflicts of interest to declare.
Acknowledgements
This material is based upon work supported by the National Science Foundation (NSF) under Grant Numbers (2027288), (2027233), and (2051336) and by the Department of the Army U.S. Army Corps of Engineers (USACE) Engineer Research and Development Center (ERDC) (W9132T2220001). Any opinions, findings, and conclusions or recommendations expressed in this material are those of the author(s) and do not necessarily reflect the views of the NSF or USACE ERDC. This study was funded by the USDA-Agricultural Research Service (Project No. 5090-12630-006-00D).
References
- Y. Hu, L. Jiang, X. Sun, J. Wu, L. Ma, Y. Zhou, K. Lin, Y. Luo and C. Cui, Risk assessment of antibiotic resistance genes in the drinking water system, Sci. Total Environ., 2021, 800, 149650 CrossRef CAS PubMed.
- J. Clardy, M. A. Fischbach and C. R. Currie, The natural history of antibiotics, Curr. Biol., 2009, 19, R437–R441 CrossRef CAS PubMed.
- R. Chait, K. Vetsigian and R. Kishony, What counters antibiotic resistance in nature?, Nat. Chem. Biol., 2011, 8, 2–5 CrossRef PubMed.
- X. M. Han, H. W. Hu, X. Z. Shi, J. T. Wang, L. L. Han, D. Chen and J. Z. He, Impacts of reclaimed water irrigation on soil antibiotic resistome in urban parks of victoria, australia, Environ. Pollut., 2016, 211, 48–57 CrossRef CAS PubMed.
- Y. Yang, G. Liu, W. Song, C. Ye, H. Lin, Z. Li and W. Liu, Plastics in the marine environment are reservoirs for antibiotic and metal resistance genes, Environ. Int., 2019, 123, 79–86 CrossRef CAS PubMed.
- A. Pruden, R. Pei, H. Storteboom and K. H. Carlson, Antibiotic resistance genes as emerging contaminants: studies in northern colorado, Environ. Sci. Technol., 2006, 40, 7445–7450 CrossRef CAS PubMed.
- T. U. Berendonk, C. M. Manaia, C. Merlin, D. Fatta-Kassinos, E. Cytryn, F. Walsh, H. Bürgmann, H. Sørum, M. Norström, M.-N. Pons, N. Kreuzinger, P. Huovinen, S. Stefani, T. Schwartz, V. Kisand, F. Baquero and J. Luis Martinez, Tackling antibiotic resistance: the environmental framework, Nat. Rev. Microbiol., 2015, 13, 310–317 CrossRef CAS PubMed.
- A. Pruden, P. J. Vikesland, B. C. Davis and A. M. de Roda Husman, Seizing the moment: now is the time for integrated global surveillance of antimicrobial resistance in wastewater environments, Curr. Opin. Microbiol., 2021, 64, 91–99 CrossRef CAS PubMed.
- N. J. Ashbolt, A. Amézquita, T. Backhaus, P. Borriello, K. K. Brandt, P. Collignon, A. Coors, R. Finley, W. H. Gaze, T. Heberer, J. R. Lawrence, D. G. J. Larsson, S. A. McEwen, J. J. Ryan, J. Schönfeld, P. Silley, J. R. Snape, C. Van den Eede and E. Topp, Human health risk assessment (hhra) for environmental development and transfer of antibiotic resistance, Environ. Health Perspect., 2013, 121, 993–1001 CrossRef PubMed.
- J. L. Martínez, T. M. Coque and F. Baquero, What is a resistance gene? ranking risk in resistomes, Nat. Rev. Microbiol., 2014, 13, 116–123 CrossRef PubMed.
- C. M. Manaia, Assessing the risk of antibiotic resistance transmission from the environment to humans: non-direct proportionality between abundance and risk, Trends Microbiol., 2017, 25, 173–181 CrossRef CAS PubMed.
- A. N. Zhang, J. M. Gaston, C. L. Dai, S. Zhao, M. Poyet, M. Groussin, X. Yin, L. G. Li, M. C. M. van Loosdrecht, E. Topp, M. R. Gillings, W. P. Hanage, J. M. Tiedje, K. Moniz, E. J. Alm and T. Zhang, An omics-based framework for assessing the health risk of antimicrobial resistance genes, Nat. Commun., 2021, 12, 1–11 CrossRef PubMed.
- R. L. Finley, P. Collignon, D. G. J. Larsson, S. A. Mcewen, X.-Z. Li, W. H. Gaze, R. Reid-Smith, M. Timinouni, D. W. Graham and E. Topp, The scourge of antibiotic resistance: the important role of the environment, Clin. Infect. Dis., 2013, 57, 704–710 CrossRef PubMed.
- J. Bengtsson-Palme and D. G. J. Larsson, Antibiotic resistance genes in the environment: prioritizing risks, Nat. Rev. Microbiol., 2015, 13, 396 CrossRef CAS PubMed.
- D. G. J. Larsson and C.-F. Flach, Antibiotic resistance in the environment, Nat. Rev. Microbiol., 2022, 20, 257–269 CrossRef CAS PubMed.
- Z. Han, Y. Zhang, W. An, J. Lu, J. Hu and M. Yang, Antibiotic resistomes in drinking water sources across a large geographical scale: multiple drivers and co-occurrence with opportunistic bacterial pathogens, Water Res., 2020, 183, 116088 CrossRef CAS PubMed.
- F. Z. Gao, H. Y. Zou, D. L. Wu, S. Chen, L. Y. He, M. Zhang, H. Bai and G. G. Ying, Swine farming elevated the proliferation of acinetobacter with the prevalence of antibiotic resistance genes in the groundwater, Environ. Int., 2020, 136, 105484 CrossRef PubMed.
- X. Li, C. Liu, Y. Chen, H. Huang and T. Ren, Antibiotic residues in liquid manure from swine feedlot and their effects on nearby groundwater in regions of north china, Environ. Sci. Pollut. Res., 2018, 25, 11565–11575 CrossRef CAS PubMed.
- L. Tong, L. Qin, C. Guan, M. E. Wilson, X. Li, D. Cheng, J. Ma, H. Liu and F. Gong, Antibiotic resistance gene profiling in response to antibiotic usage and environmental factors in the surface water and groundwater of honghu lake, china, Environ. Sci. Pollut. Res., 2020, 27, 31995–32005 CrossRef CAS PubMed.
- M. Harb, P. Wang, A. Zarei-Baygi, M. H. Plumlee and A. L. Smith, Background antibiotic resistance and microbial communities dominate effects of advanced purified water recharge to an urban aquifer, Environ. Sci. Technol. Lett., 2019, 6, 578–584 CrossRef CAS.
- D. Wu, M. Zhang, L. He, H. Zou, Y. Liu, B. Li, Y.-Y. Yang, C. Liu, L.-Y. He and G.-G. Ying, Contamination profile of antibiotic resistance genes in ground water in comparison with surface water, Sci. Total Environ., 2020, 715, 136975 CrossRef CAS PubMed.
- R. Mi, R. Patidar, A. Farenhorst, Z. Cai, S. Sepehri, E. Khafipour and A. Kumar, Detection of fecal bacteria and antibiotic resistance genes in drinking water collected from three first nations communities in manitoba, canada, FEMS Microbiol. Lett., 2019, 366, fnz067 CrossRef CAS PubMed.
- L. Huang, Y. Xu, J. Xu, J. Ling, L. Zheng, X. Zhou and G. Xie, Dissemination of antibiotic resistance genes (args) by rainfall on a cyclic economic breeding livestock farm, Int. Biodeterior. Biodegrad., 2019, 138, 114–121 CrossRef CAS.
- E. Szekeres, C. Chiriac, A. Baricz, T. Szőke-Nagy, I. Lung, M.-L. Soran, K. Rudi, N. Dragos and C. Coman, Investigating antibiotics, antibiotic resistance genes, and microbial contaminants in groundwater in relation to the proximity of urban areas, Environ. Pollut., 2018, 236, 734–744 CrossRef CAS PubMed.
- X. Liu, G. Zhang, Y. Liu, S. Lu, P. Qin, X. Guo, B. Bi, L. Wang, B. Xi, F. Wu, W. Wang and T. Zhang, Occurrence and fate of antibiotics and antibiotic resistance genes in typical urban water of beijing, china, Environ. Pollut., 2019, 246, 163–173 CrossRef CAS PubMed.
- C. Stange and A. Tiehm, Occurrence of antibiotic resistance genes and microbial source tracking markers in the water of a karst spring in germany, Sci. Total Environ., 2020, 742, 140529 CrossRef CAS PubMed.
- R. Elkayam, A. Aharoni, D. Vaizel-Ohayon and O. Sued, Viral and microbial pathogens, indicator microorganisms, microbial source tracking indicators, and antibiotic resistance genes in a confined managed effluent, J. Environ. Eng., 2018, 144, 05017011 CrossRef.
- O. O. Adelowo, S. Caucci, O. A. Banjo, O. C. Nnanna, E. O. Awotipe, F. B. Peters, O. E. Fagade and T. U. Berendonk, Extended spectrum beta-lactamase (esbl)-producing bacteria isolated from hospital wastewaters, rivers and aquaculture sources in nigeria, Environ. Sci. Pollut. Res., 2018, 25, 2744–2755 CrossRef CAS PubMed.
- Z. Shi, H. Yin, J. D. Van Nostrand, J. W. Voordeckers, Q. Tu, Y. Deng, M. Yuan, A. Zhou, P. Zhang, N. Xiao, D. Ning, Z. He, L. Wu and J. Zhou, Functional gene array-based ultrasensitive and quantitative detection of microbial populations in complex communities, mSystems, 2019, 4, 296–315 Search PubMed.
- H. Zou, B. Berglund, H. Xu, X. Chi, Q. Zhao, Z. Zhou, H. Xia, X. Li and B. Zheng, Genetic characterization and virulence of a carbapenem-resistant raoultella ornithinolytica isolated from well water carrying a novel megaplasmid containing blandm-1, Environ. Pollut., 2020, 260, 114041 CrossRef CAS PubMed.
- L. E. Hubbard, C. E. Givens, D. W. Griffin, L. R. Iwanowicz, M. T. Meyer and D. W. Kolpin, Poultry litter as potential source of pathogens and other contaminants in groundwater and surface water proximal to large-scale confined poultry feeding operations, Sci. Total Environ., 2020, 735, 139459 CrossRef CAS PubMed.
- D. P. Matlou, M. E. A. T. Bissong, C. D. K. Tchatchouang, M. R. Adem, F. E. T. Foka, A. Kumar and C. N. Ateba, Virulence profiles of vancomycin-resistant enterococci isolated from surface and ground water utilized by humans in the north west province, south africa: a public health perspective, Environ. Sci. Pollut. Res., 2019, 26, 15105–15114 CrossRef CAS PubMed.
- M. T. Gekenidis, W. Qi, J. Hummerjohann, R. Zbinden, F. Walsh and D. Drissner, Antibiotic-resistant indicator bacteria in irrigation water: high prevalence of extended-spectrum beta-lactamase (esbl)-producing escherichia coli, PLoS One, 2018, 13, e0207857 CrossRef PubMed.
- Y. Zhang, Y. Zhang, Z. Kuang, J. Xu, C. Li, Y. Li, Y. Jiang and J. Xie, Comparison of microbiomes and resistomes in two karst groundwater sites in chongqing, china, Groundwater, 2019, 57, 807–818 CrossRef CAS PubMed.
- A. Kothari, Y.-W. Wu, J.-M. Chandonia, M. Charrier, L. Rajeev, A. M. Rocha, D. C. Joyner, T. C. Hazen, S. W. Singer, A. Mukhopadhyay, E. Stephen and C. Winans, Large circular plasmids from groundwater plasmidomes span multiple incompatibility groups and are enriched in multimetal resistance genes, MBio, 2019, 10, e02899-18 CrossRef PubMed.
- L. Zhang, L. Li, G. Sha, C. Liu, Z. Wang and L. Wang, Aerobic composting as an effective cow manure management strategy for reducing the dissemination of antibiotic resistance genes: an integrated meta-omics, J. Hazard. Mater., 2020, 386, 121895 CrossRef CAS PubMed.
- Q. Yuan, Y. Zhai, B. Mao and N. Hu, Antibiotic resistance genes and inti1 prevalence in a swine wastewater treatment plant and correlation with metal resistance, bacterial community and wastewater parameters, Ecotoxicol. Environ. Saf., 2018, 161, 251–259 CrossRef CAS PubMed.
- C. Pu, Y. Yu, J. Diao, X. Gong, J. Li and Y. Sun, Exploring the persistence and spreading of antibiotic resistance from manure to biocompost, soils and vegetables, Sci. Total Environ., 2019, 688, 262–269 CrossRef CAS PubMed.
- R. Barrios, H. Khuntia, S. Bartelt-Hunt, J. E. Gilley, A. M. Schmidt, D. D. Snow and X. Li, Fate and transport of antibiotics and antibiotic resistance genes in runoff and soil as affected by the timing of swine manure slurry application, Sci. Total Environ., 2020, 712, 136505 CrossRef CAS PubMed.
- L. Lan, X. Kong, H. Sun, C. Li and D. Li, High removal efficiency of antibiotic
resistance genes in swine wastewater via nanofiltration and reverse osmosis processes, J. Environ. Manage., 2019, 231, 439–445 CrossRef CAS PubMed.
- C. Pu, H. Liu, G. Ding, Y. Sun, X. Yu, J. Chen, J. Ren and X. Gong, Impact of direct application of biogas slurry and residue in fields: in situ analysis of antibiotic resistance genes from pig manure to fields, J. Hazard. Mater., 2018, 344, 441–449 CrossRef CAS PubMed.
- H. Guo, J. Gu, X. Wang, X. Tuo, J. Yu and R. Zhang, Key role of cyromazine in the distribution of antibiotic resistance genes and bacterial community variation in aerobic composting, Bioresour. Technol., 2019, 274, 418–424 CrossRef CAS PubMed.
- Z. Ma, H. Wu, K. Zhang, X. Xu, C. Wang, W. Zhu and W. Wu, Long-term low dissolved oxygen accelerates the removal of antibiotics and antibiotic resistance genes in swine wastewater treatment, Chem. Eng. J., 2018, 334, 630–637 CrossRef CAS.
- J. Wallace, E. Garner, A. Pruden and D. Aga, Occurrence and transformation of veterinary antibiotics and antibiotic resistance genes in dairy manure treated by advanced anaerobic digestion and conventional treatment methods, Environ. Pollut., 2018, 236, 764–772 CrossRef CAS PubMed.
- Y. Liu, E. Cui, A. Neal, X. Zhang, Z. Li, Y. Xiao, Z. Du, F. Gao, X. Fan and C. Hu, Reducing water use by alternate-furrow irrigation with livestock wastewater reduces antibiotic resistance gene abundance in the rhizosphere but not in the non-rhizosphere, Sci. Total Environ., 2019, 648, 12–24 CrossRef CAS PubMed.
- F. Gao, L. He, L. He, H. Zou, M. Zhang, D. Wu, Y.-S. Liu, Y.-J. Shi, H. Bai and G.-G. Ying, Untreated swine wastes changed antibiotic resistance and microbial community in the soils and impacted abundances of antibiotic resistance genes in the vegetables, Sci. Total Environ., 2020, 741, 140482 CrossRef CAS PubMed.
- J. Zhang, T. Lu, Y. Chai, Q. Sui, P. Shen and Y. Wei, Which animal type contributes the most to the emission of antibiotic resistance genes in large-scale swine farms in china?, Sci. Total Environ., 2019, 658, 152–159 CrossRef CAS PubMed.
- X. Huang, J. Zheng, S. Tian, C. Liu, L. Liu, L. Wei, H. Fan, T. Zhang, L. Wang, G. Zhu and K. Xu, Higher temperatures do not always achieve better antibiotic resistance gene removal in anaerobic digestion of swine manure, Appl. Environ. Microbiol., 2019, 85, e02878-18 CrossRef PubMed.
- H. Li, W. Cheng, B. Li, Y. Xu and X. Zheng, The fate of antibiotic resistance genes during co-composting of swine manure with cauliflower and corn straw, Bioresour. Technol., 2020, 300, 122669 CrossRef CAS PubMed.
- J. Zhang, T. Lu, P. Shen, Q. Sui, H. Zhong, J. Liu, J. Tong and Y. Wei, The role of substrate types and substrate microbial community on the fate of antibiotic resistance genes during anaerobic digestion, Chemosphere, 2019, 229, 461–470 CrossRef CAS PubMed.
- M. Couch, G. E. Agga, J. Kasumba, R. R. Parekh, J. H. Loughrin and E. D. Conte, Abundances of tetracycline resistance genes and tetracycline antibiotics during anaerobic digestion of swine waste, J. Environ. Qual., 2019, 48, 171–178 CrossRef CAS PubMed.
- E. Lopatto, J. Choi, A. Colina, L. Ma, A. Howe and S. Hinsa-Leasure, Characterizing the soil microbiome and quantifying antibiotic resistance gene dynamics in agricultural soil following swine cafo manure application, PLoS One, 2019, 14, e0220770 CrossRef CAS PubMed.
- J. Zhang, H. Lin, J. Ma, W. Sun, Y. Yang and X. Zhang, Compost-bulking agents reduce the reservoir of antibiotics and antibiotic resistance genes in manures by modifying bacterial microbiota, Sci. Total Environ., 2019, 649, 396–404 CrossRef CAS PubMed.
- Z. Chen, Y. Wang and Q. Wen, Effects of chlortetracycline on the fate of multi-antibiotic resistance genes and the microbial community during swine manure composting, Environ. Pollut., 2018, 237, 977–987 CrossRef CAS PubMed.
- X. Wu, Z. Tian, Z. Lv, Z. Chen, Y. Liu, X. Yong, J. Zhou, X. Xie, H. Jia and P. Wei, Effects of copper salts on performance, antibiotic resistance genes, and microbial community during thermophilic anaerobic digestion of swine manure, Bioresour. Technol., 2020, 300, 122728 CrossRef CAS PubMed.
- H. C. Su, Y. S. Liu, C. G. Pan, J. Chen, L. Y. He and G. G. Ying, Persistence of antibiotic resistance genes and bacterial community changes in drinking water treatment system: from drinking water source to tap water, Sci. Total Environ., 2018, 616–617, 453–461 CrossRef CAS PubMed.
- E. Garner, C. Chen, K. Xia, J. Bowers, D. M. Engelthaler, J. McLain, M. A. Edwards and A. Pruden, Metagenomic characterization of antibiotic resistance genes in full-scale reclaimed water distribution systems and corresponding potable systems, Environ. Sci. Technol., 2018, 52, 6113–6125 CrossRef CAS PubMed.
- C. Stange, J. Sidhu, S. Toze and A. Tiehm, Comparative removal of antibiotic resistance genes during chlorination, ozonation, and uv treatment, Int. J. Hyg. Environ. Health, 2019, 222, 541–548 CrossRef CAS PubMed.
- H. Wang, Y. Shen, C. Hu, X. Xing and D. Zhao, Sulfadiazine/ciprofloxacin promote opportunistic pathogens occurrence in bulk water of drinking water distribution systems, Environ. Pollut., 2018, 234, 71–78 CrossRef CAS PubMed.
- H. Zhang, F. Chang, P. Shi, L. Ye, Q. Zhou, Y. Pan and A. Li, Antibiotic resistome alteration by different disinfection strategies in a full-scale drinking water treatment plant deciphered by metagenomic assembly, Environ. Sci. Technol., 2019, 53, 2141–2150 CrossRef CAS PubMed.
- E. Garner, M. Inyang, E. Garvey, J. Parks, C. Glover, A. Grimaldi, E. Dickenson, J. Sutherland, A. Salveson, M. A. Edwards and A. Pruden, Impact of blending for direct potable reuse on premise plumbing microbial ecology and regrowth of opportunistic pathogens and antibiotic resistant bacteria, Water Res., 2019, 151, 75–86 CrossRef CAS PubMed.
- K. Zhang, R. Xin, Z. Zhao, Y. Ma, Y. Zhang and Z. Niu, Antibiotic resistance genes in drinking water of china: occurrence, distribution and influencing factors, Ecotoxicol. Environ. Saf., 2020, 188, 109837 CrossRef CAS PubMed.
- J. Lu, Z. Tian, J. Yu, M. Yang and Y. Zhang, Distribution and abundance of antibiotic resistance genes in sand settling reservoirs and drinking water treatment plants across the yellow river, china, Water, 2018, 10, 246 CrossRef.
- K. Wan, M. Zhang, C. Ye, W. Lin, L. Guo, S. Chen and X. Yu, Organic carbon: an overlooked factor that determines the antibiotic resistome in drinking water sand filter biofilm, Environ. Int., 2019, 125, 117–124 CrossRef CAS PubMed.
- K. D. Sandberg, S. Ishii and T. M. Lapara, A microfluidic quantitative polymerase chain reaction method for the simultaneous analysis of dozens of antibiotic resistance and heavy metal resistance genes, Environ. Sci. Technol. Lett., 2018, 5, 20–25 CrossRef CAS.
- I. Douterelo, C. Calero-Preciado, V. Soria-Carrasco and J. B. Boxall, Whole metagenome sequencing of chlorinated drinking water distribution systems, Environ. Sci.: Water Res. Technol., 2018, 4, 2080–2091 RSC.
- Y. Hu, T. Zhang, L. Jiang, Y. Luo, S. Yao, D. Zhang, K. Lin and C. Cui, Occurrence and reduction of antibiotic resistance genes in conventional and advanced drinking water treatment processes, Sci. Total Environ., 2019, 669, 777–784 CrossRef CAS PubMed.
- T. Zhang, Y. Hu, L. Jiang, S. Yao, K. Lin, Y. Zhou and C. Cui, Removal of antibiotic resistance genes and control of horizontal transfer risk by uv, chlorination and uv/chlorination treatments of drinking water, Chem. Eng. J., 2019, 358, 589–597 CrossRef CAS.
- Y. Hu, L. Jiang, T. Zhang, L. Jin, Q. Han, D. Zhang, K. Lin and C. Cui, Occurrence and removal of sulfonamide antibiotics and antibiotic resistance genes in conventional and advanced drinking water treatment processes, J. Hazard. Mater., 2018, 360, 364–372 CrossRef CAS PubMed.
- Z. C. Zhou, W. Q. Feng, Y. Han, J. Zheng, T. Chen, Y. Y. Wei, M. Gillings, Y. G. Zhu and H. Chen, Prevalence and transmission of antibiotic resistance and microbiota between humans and water environments, Environ. Int., 2018, 121, 1155–1161 CrossRef CAS PubMed.
- J. Zheng, T. Chen and H. Chen, Antibiotic resistome promotion in drinking water during biological activated carbon treatment: is it influenced by quorum sensing?, Sci. Total Environ., 2018, 612, 1–8 CrossRef CAS PubMed.
- L. Ma, B. Li and T. Zhang, New insights into antibiotic resistome in drinking water and management perspectives: a metagenomic based study of small-sized microbes, Water Res., 2019, 152, 191–201 CrossRef CAS PubMed.
- H. Hao, D. Y. Shi, D. Yang, Z. W. Yang, Z. G. Qiu, W. L. Liu, Z. Q. Shen, J. Yin, H. R. Wang, J. W. Li, H. Wang and M. Jin, Profiling of intracellular and extracellular antibiotic resistance genes in tap water, J. Hazard. Mater., 2019, 365, 340–345 CrossRef CAS PubMed.
- H. Huang, S. Zeng, X. Dong, D. Li, Y. Zhang, M. He and P. Du, Diverse and abundant antibiotics and antibiotic resistance genes in an urban water system, J. Environ. Manage., 2019, 231, 494–503 CrossRef CAS PubMed.
- M. Zhang, L. Chen, C. Ye and X. Yu, Co-selection of antibiotic resistance via copper shock loading on bacteria from a drinking water bio-filter, Environ. Pollut., 2018, 233, 132–141 CrossRef CAS PubMed.
- Y. Zhang, A. Li, T. Dai, F. Li, H. Xie, L. Chen and D. Wen, Cell-free dna: a neglected source for antibiotic resistance genes spreading from wwtps, Environ. Sci. Technol., 2018, 52, 248–257 CrossRef CAS PubMed.
- S. Liu, H. Qu, D. Yang, H. Hu, W. Liu, Z. Qiu, A. Hou, J. Guo, J.-W. Li, Z.-Q. Shen and M. Jin, Chlorine disinfection increases both intracellular and extracellular antibiotic resistance genes in a full-scale wastewater treatment plant, Water Res., 2018, 136, 131–136 CrossRef CAS PubMed.
- I. Iakovides, I. Michael-Kordatou, N. Moreira, A. R. Ribeiro, T. Fernandes, M. F. R. Pereira, O. C. Nunes, C. M. Manaia, A. M. T. Silva and D. Fatta-Kassinosa, Continuous ozonation of urban wastewater: removal of antibiotics, antibiotic-resistant escherichia coli and antibiotic resistance genes and phytotoxicity, Water Res., 2019, 159, 333–347 CrossRef CAS PubMed.
- H. Liao, X. Lu, C. Rensing, V. Petri Friman, S. Geisen, Z. Chen, Z. Yu, Z. Wei, S. Zhou and Y. Zhu, Hyperthermophilic composting accelerates the removal of antibiotic resistance genes and mobile genetic elements in sewage sludge, Environ. Sci. Technol., 2018, 52, 266–276 CrossRef CAS PubMed.
- Q. Wang, P. Wang and Q. Yang, Occurrence and diversity of antibiotic resistance in untreated hospital wastewater, Sci. Total Environ., 2018, 621, 990–999 CrossRef CAS PubMed.
- S. Ren, C. Boo, N. Guo, S. Wang, M. Elimelech and Y. Wang, Photocatalytic reactive ultrafiltration membrane for removal of antibiotic resistant bacteria and antibiotic resistance genes from wastewater effluent, Environ. Sci. Technol., 2018, 52, 8666–8673 CrossRef CAS PubMed.
- N. Sabri, H. Schmitt, B. Van der Zaan, H. W. Gerritsen, T. Zuidema, H. H. M. Rijnaarts and A. A. M. Langenhoff, Prevalence of antibiotics and antibiotic resistance genes in a wastewater effluent-receiving river in the netherlands, J. Environ. Chem. Eng., 2020, 8, 102245 CrossRef CAS.
- T. Le, C. Ng, N. Tran, H. Chen and K. Gin, Removal of antibiotic residues, antibiotic resistant bacteria and antibiotic resistance genes in municipal wastewater by membrane bioreactor systems, Water Res., 2018, 145, 498–508 CrossRef CAS PubMed.
- P. Karaolia, I. Michael-Kordatou, E. Hapeshi, C. Drosou, Y. Bertakis, D. Christofilos, G. S. Armatas, L. Sygellou, T. Schwartz, N. P. Xekoukoulotakis and D. Fatta-Kassinosa, Removal of antibiotics, antibiotic-resistant bacteria and their associated genes by graphene-based tio2 composite photocatalysts under solar radiation in urban wastewaters, Appl. Catal., B, 2018, 224, 810–824 CrossRef CAS.
- X. An, J. Su, B. Li, W. Ouyang, Y. Zhao, Q. Chen, L. Cui, H. Chen, M. R. Gillings, T. Zhang and Y.-G. Zhua, Tracking antibiotic resistome during wastewater treatment using high throughput quantitative pcr, Environ. Int., 2018, 117, 146–153 CrossRef CAS PubMed.
- N. Moreira, C. Narciso-da-Rocha, M. Polo-López, L. M. Pastrana-Martínez, J. L. Faria, C. M. Manaia, P. Fernández-Ibáñez, O. C. Nunes and A. M. T. Silva, Solar treatment (h2o2, tio2-p25 and go-tio2 photocatalysis, photo-fenton) of organic micropollutants, human pathogen indicators, antibiotic resistant bacteria and related genes in urban wastewater, Water Res., 2018, 135, 195–206 CrossRef CAS PubMed.
- F. Ju, K. Beck, X. Yin, A. Maccagnan, C. McArdell, H. P. Singer, D. R. Johnson, T. Zhang and H. Bürgmann, Wastewater treatment plant resistomes are shaped by bacterial composition, genetic exchange, and upregulated expression in the effluent microbiomes, ISME J., 2019, 13, 346–360 CrossRef PubMed.
- R. Zhao, J. Feng, J. Liu, W. Fu, X. Li and B. Li, Deciphering of microbial community and antibiotic resistance genes in activated sludge reactors under high selective pressure of different antibiotics, Water Res., 2019, 151, 388–402 CrossRef CAS PubMed.
- A. Karkman, K. Pärnänen and D. Larsson, Fecal pollution can explain antibiotic resistance gene abundances in anthropogenically impacted environments, Nat. Commun., 2019, 10, 80 CrossRef CAS PubMed.
- B. T. T. Chu, M. L. Petrovich, A. Chaudhary, D. Wright, B. Murphy, G. Wells and R. Poretsky, Metagenomics reveals the impact of wastewater treatment plants on the dispersal of microorganisms and genes in aquatic sediments, Appl. Environ. Microbiol., 2018, 84, e02168-17 CrossRef PubMed.
- D. Cacace, D. Fatta-Kassinos, C. Manaia, E. Cytryn, N. Kreuzinger, L. Rizzo, P. Karaolia, T. Schwartz, J. Alexander, C. Merlin, H. Garelick, H. Schmitt, D. de Vries, C. U. Schwermer, S. Meric, C. B. Ozkal, M.-N. Pons, D. Kneis and T. U. Berendonk, Antibiotic resistance genes in treated wastewater and in the receiving water bodies: a pan-european survey of urban settings, Water Res., 2019, 162, 320–330 CrossRef CAS PubMed.
- K. Pärnänen, C. Narciso-da-Rocha, D. Kneis, T. U. Berendonk, D. Cacace, T. T. Do, C. Elpers, D. Fata-Kassinos, I. Henriques, T. Jaeger, A. Karkman, J. L. Martinez, S. G. Michael, I. Michael-Kordatou, K. O'Sullivan, S. Rodriguez-Mozaz, T. Schwartz, H. Sheng, H. Sørum, R. D. Stedtfeld, J. M. Tiedje, S. V. Della Giustina, F. Walsh, I. Vaz-Moreira, M. Virta and C. M. Manaia, Antibiotic resistance in european wastewater treatment plants mirrors the pattern of clinical antibiotic resistance prevalence, Sci. Adv., 2019, 5, eaau9124 CrossRef PubMed.
- P. Lorenzo, A. Adriana, S. Jessica, B. Carles, F. Marinella, L. Marta, B. J. Luis and S. Pierre, Antibiotic resistance in urban and hospital wastewaters and their impact on a receiving freshwater ecosystem, Chemosphere, 2018, 206, 70–82 CrossRef CAS PubMed.
- S. Lee, M. Suits, D. Wituszynski, R. Winston, J. Martin and J. Lee, Residential urban stormwater runoff: a comprehensive profile of microbiome and antibiotic resistance, Sci. Total Environ., 2020, 723, 138033 CrossRef CAS PubMed.
- M. M. McConnell, L. T. Hansen, K. D. Neudorf, J. L. Hayward, R. C. Jamieson, C. K. Yost and A. Tong, Sources of antibiotic resistance genes in a rural river system, J. Environ. Qual., 2018, 47, 997–1005 CrossRef CAS PubMed.
- R. Carney, M. Labbate, N. Siboni, K. Tagg, S. Mitrovic and J. R. Seymour, Urban beaches are environmental hotspots for antibiotic resistance following rainfall, Water Res., 2019, 167, 115081 CrossRef CAS PubMed.
- D. A. P. R. Dewi, B. Götz and T. Thomasa, Diversity and genetic basis for carbapenem resistance in a coastal marine environment, Appl. Environ. Microbiol., 2020, 86, e02939-19 CrossRef PubMed.
- W. Ahmed, Q. Zhang, A. Lobos, J. Senkbeil, M. J. Sadowsky, V. J. Harwood, N. Saeidi, O. Marinoni and S. Ishii, Precipitation influences pathogenic bacteria and antibiotic resistance gene abundance in storm drain outfalls in coastal sub-tropical waters, Environ. Int., 2018, 116, 308–318 CrossRef CAS PubMed.
- J. Sidhu, V. Gupta, C. Stange, J. Ho, N. Harris, K. Barry, D. Gonzalez, J. D. Van Nostran, J. Zhou, D. Page, A. Tiehm and S. Toze, Prevalence of antibiotic resistance and virulence genes in the biofilms from an aquifer recharged with stormwater, Water Res., 2020, 185, 116269 CrossRef CAS PubMed.
- M. Białasek and A. Miłobędzka, Revealing antimicrobial resistance in stormwater with minion, Chemosphere, 2020, 258, 127392 CrossRef PubMed.
- A. Chaudhary, I. Kauser, A. Ray and R. Poretsky, Taxon-driven functional shifts associated with storm flow in an urban stream microbial community, mSphere, 2018, 3, e00194-18 CrossRef PubMed.
- D. Baral, B. I. Dvorak, D. Admiraal, S. Jia, C. Zhang and X. Li, Tracking the sources of antibiotic resistance genes in an urban stream during wet weather using shotgun metagenomic analyses, Environ. Sci. Technol., 2018, 52, 9033–9044 CrossRef CAS PubMed.
- K. Sen, T. Berglund, M. A. Soares, B. Taheri, Y. Ma, L. Khalil, M. Fridge, J. Lu and R. J. Turner, Antibiotic resistance of e. coli isolated from a constructed wetland dominated by a crow roost, with emphasis on esbl and ampc containing e. coli, Front. Microbiol., 2019, 10, 1034 CrossRef PubMed.
- M. Sun, M. Ye, Z. Zhang, S. Zhang, Y. Zhao, S. Deng, L. Kong, R. Ying, B. Xia, W. Jiao, J. Cheng, Y. Feng, M. Liu and F. Hu, Biochar combined with polyvalent phage therapy to mitigate antibiotic resistance pathogenic bacteria vertical transfer risk in an undisturbed soil column, J. Hazard. Mater., 2019, 365, 1–8 CrossRef CAS PubMed.
- A. Y. Peleg, H. Seifert and D. L. Paterson, Acinetobacter baumannii: emergence of a successful pathogen, Clin. Microbiol. Rev., 2008, 21, 538–582 CrossRef CAS PubMed.
- P. Savard and T. M. Perl, A call for action: managing the emergence of multidrug-resistant enterobacteriaceae in the acute care settings, Curr. Opin. Infect. Dis., 2012, 25, 371–377 CrossRef PubMed.
-
T. Aven, Y. Ben-Haim, H. B. Andersen, T. Cox, E. L. Droguett, M. Greenberg, S. Guikema, W. Kröger, O. Renn, K. M. Thompson and E. Zio, Society for risk analysis glossary, 2018 Search PubMed.
-
C. N. Haas, J. B. Rose and C. P. Gerba, Quantitative microbial risk assessment, Quantitative microbial risk assessment, John Wiley & Sons, Inc., Hoboken, New Jersey, 2nd edn, 2014 Search PubMed.
-
NRC, Risk assessment in the federal government: managing the process, Risk assessment in the federal government: managing the process, National Academies Press, Washington, D.C., 1983 Search PubMed.
- J. Larsen, C. L. Raisen, X. Ba, N. J. Sadgrove, G. F. Padilla-González, M. S. J. Simmonds, I. Loncaric, H. Kerschner, P. Apfalter, R. Hartl, A. Deplano, S. Vandendriessche, B. Č. Bolfíková, P. Hulva, M. C. Arendrup, R. K. Hare, C. Barnadas, M. Stegger, R. N. Sieber, R. L. Skov, A. Petersen, Ø. Angen, S. L. Rasmussen, C. Espinosa-Gongora, F. M. Aarestrup, L. J. Lindholm, S. M. Nykäsenoja, F. Laurent, K. Becker, B. Walther, C. Kehrenberg, C. Cuny, F. Layer, G. Werner, W. Witte, I. Stamm, P. Moroni, H. J. Jørgensen, H. de Lencastre, E. Cercenado, F. García-Garrote, S. Börjesson, S. Haeggman, V. Perreten, C. J. Teale, A. S. Waller, B. Pichon, M. D. Curran, M. J. Ellington, J. J. Welch, S. J. Peacock, D. J. Seilly, F. J. E. Morgan, J. Parkhill, N. F. Hadjirin, J. A. Lindsay, M. T. G. Holden, G. F. Edwards, G. Foster, G. K. Paterson, X. Didelot, M. A. Holmes, E. M. Harrison and A. R. Larsen, Emergence of methicillin resistance predates the clinical use of antibiotics, Nature, 2022, 602, 135–141 CrossRef CAS PubMed.
- L. Andrade, M. Kelly, P. Hynds, J. Weatherill, A. Majury and J. O'Dwyer, Groundwater resources as a global reservoir for antimicrobial-resistant bacteria, Water Res., 2020, 170, 115360 CrossRef CAS PubMed.
- E. Sanganyado and W. Gwenzi, Antibiotic resistance in drinking water systems: occurrence, removal, and human health risks, Sci. Total Environ., 2019, 669, 785–797 CrossRef CAS PubMed.
- C. Quince, A. W. Walker, J. T. Simpson, N. J. Loman and N. Segata, Shotgun metagenomics, from sampling to analysis, Nat. Biotechnol., 2017, 35, 833–844 CrossRef CAS PubMed.
- H. S. Gweon, L. P. Shaw, J. Swann, N. De Maio, M. AbuOun, R. Niehus, A. T. M. Hubbard, M. J. Bowes, M. J. Bailey, T. E. A. Peto, S. J. Hoosdally, A. S. Walker, R. P. Sebra, D. W. Crook, M. F. Anjum, D. S. Read and N. Stoesser, The impact of sequencing depth on the inferred taxonomic composition and amr gene content of metagenomic samples, Environ. Microbiome, 2019, 14, 1–15 CrossRef CAS PubMed.
- J. J. Davis, A. R. Wattam, R. K. Aziz, T. Brettin, R. Butler, R. M. Butler, P. Chlenski, N. Conrad, A. Dickerman, E. M. Dietrich, J. L. Gabbard, S. Gerdes, A. Guard, R. W. Kenyon, D. MacHi, C. Mao, D. Murphy-Olson, M. Nguyen, E. K. Nordberg, G. J. Olsen, R. D. Olson, J. C. Overbeek, R. Overbeek, B. Parrello, G. D. Pusch, M. Shukla, C. Thomas, M. Vanoeffelen, V. Vonstein, A. S. Warren, F. Xia, D. Xie, H. Yoo and R. Stevens, The patric bioinformatics resource center: expanding data and analysis capabilities, Nucleic Acids Res., 2020, 48, D606 CAS.
- B. P. Alcock, A. R. Raphenya, T. T. Y. Lau, K. K. Tsang, M. Bouchard, A. Edalatmand, W. Huynh, A. L. V. Nguyen, A. A. Cheng, S. Liu, S. Y. Min, A. Miroshnichenko, H. K. Tran, R. E. Werfalli, J. A. Nasir, M. Oloni, D. J. Speicher, A. Florescu, B. Singh, M. Faltyn, A. Hernandez-Koutoucheva, A. N. Sharma, E. Bordeleau, A. C. Pawlowski, H. L. Zubyk, D. Dooley, E. Griffiths, F. Maguire, G. L. Winsor, R. G. Beiko, F. S. L. Brinkman, W. W. L. Hsiao, G. V. Domselaar and A. G. McArthur, CARD 2020: antibiotic resistome surveillance with the comprehensive antibiotic resistance database, Nucleic Acids Res., 2020, 48, D517–D525 CrossRef CAS PubMed.
- E. Geisinger, G. Vargas-Cuebas, N. J. Mortman, S. Syal, Y. Dai, E. L. Wainwright, D. Lazinski, S. Wood, Z. Zhu, J. Anthony, T. van Opijnen and R. R. Isberg, The landscape of phenotypic and transcriptional responses to ciprofloxacin in acinetobacter baumannii: acquired resistance alleles modulate drug-induced sos response and prophage replication, MBio, 2019, 10, e01127-19 CrossRef PubMed.
- A. Santos-Lopez, C. W. Marshall, M. R. Scribner, D. J. Snyder and V. S. Cooper, Evolutionary pathways to antibiotic resistance are dependent upon environmental structure and bacterial lifestyle, eLife, 2019, 8, e47612 CrossRef CAS PubMed.
- I. Garratt, P. Aranega-Bou, J. M. Sutton, G. Moore and M. E. Wand, Long-term exposure to octenidine in a simulated sink trap environment results in selection of pseudomonas aeruginosa, citrobacter, and enterobacter isolates with mutations in efflux pump regulators, Appl. Environ. Microbiol., 2021, 87, 1–14 CrossRef PubMed.
- F. Sanz-García, S. Hernando-Amado and J. L. Martínez, Evolution under low antibiotic concentrations: a risk for the selection of pseudomonas aeruginosa multidrug-resistant mutants in nature, Environ. Microbiol., 2022, 24(3), 1279–1293 CrossRef PubMed.
- D. F. K. dos Santos, P. Istvan, B. F. Quirino and R. H. Kruger, Functional metagenomics as a tool for identification of new antibiotic resistance genes from natural environments, Microb. Ecol., 2017, 73, 479–491 CrossRef CAS PubMed.
- L. Hadjadj, S. A. Baron, S. M. Diene and J. M. Rolain, How to discover new antibiotic resistance genes?, Expert Rev. Mol. Diagn., 2019, 19, 349–362 CrossRef CAS PubMed.
- M. L. Bendall, S. L. R. Stevens, L. K. Chan, S. Malfatti, P. Schwientek, J. Tremblay, W. Schackwitz, J. Martin, A. Pati, B. Bushnell, J. Froula, D. Kang, S. G. Tringe, S. Bertilsson, M. A. Moran, A. Shade, R. J. Newton, K. D. McMahon and R. R. Malmstrom, Genome-wide selective sweeps and gene-specific sweeps in natural bacterial populations, ISME J., 2016, 10, 1589–1601 CrossRef PubMed.
- K. J. Card, J. A. Jordan and R. E. Lenski, Idiosyncratic variation in the fitness costs of tetracycline-resistance mutations in escherichia coli, Evolution, 2021, 75, 1230–1238 CrossRef CAS PubMed.
- A. R. Hall, Genotype-by-environment interactions due to antibiotic resistance and adaptation in escherichia coli, J. Evol. Biol., 2013, 26, 1655–1664 CrossRef CAS PubMed.
- K. Yahara, K. C. Ma, T. D. Mortimer, K. Shimuta, S. I. Nakayama, A. Hirabayashi, M. Suzuki, M. Jinnai, H. Ohya, T. Kuroki, Y. Watanabe, M. Yasuda, T. Deguchi, V. Eldholm, O. B. Harrison, M. C. J. Maiden, Y. H. Grad and M. Ohnishi, Emergence and evolution of antimicrobial resistance genes and mutations in neisseria gonorrhoeae, Genome Med., 2021, 13, 1–12 CrossRef PubMed.
- S. A. Suarez and A. C. Martiny, Gene amplification uncovers large previously unrecognized cryptic antibiotic resistance potential in e. coli, Microbiol. Spectrum, 2021, 9(3), e00289-21 CrossRef PubMed.
- H. Carolus, S. Pierson, J. F. Muñoz, A. Subotić, R. B. Cruz, C. A. Cuomo and P. Van Dijck, Genome-wide analysis of experimentally evolved candida auris reveals multiple novel mechanisms of multidrug resistance, MBio, 2021, 12, e03333-20 CrossRef PubMed.
- B. Li, Y. Yang, L. Ma, F. Ju, F. Guo, J. M. Tiedje and T. Zhang, Metagenomic and network analysis reveal wide distribution and co-occurrence of environmental antibiotic resistance genes, ISME J., 2015, 9, 2490–2502 CrossRef CAS PubMed.
- J. Guo, J. Li, H. Chen, P. L. Bond and Z. Yuan, Metagenomic analysis reveals wastewater treatment plants as hotspots of antibiotic resistance genes and mobile genetic elements, Water Res., 2017, 123, 468–478 CrossRef CAS PubMed.
- M. G. Durrant, M. M. Li, B. A. Siranosian, S. B. Montgomery and A. S. Bhatt, A bioinformatic analysis of integrative mobile genetic elements highlights their role in bacterial adaptation, Cell Host Microbe, 2020, 27, 140–153 CrossRef CAS PubMed.
- M. R. Gillings, Evolutionary consequences of antibiotic use for the resistome, mobilome, and microbial pangenome, Front. Microbiol., 2013, 4, 4 Search PubMed.
- C. A. Michael, D. Dominey-Howes and M. Labbate, The antimicrobial resistance crisis: causes, consequences, and management, Front. Public Health, 2014, 2, 145 Search PubMed.
- T. Baba, F. Takeuchi, M. Kuroda, H. Yuzawa, K. I. Aoki, A. Oguchi, Y. Nagai, N. Iwama, K. Asano, T. Naimi, H. Kuroda, L. Cui, K. Yamamoto and K. Hiramatsu, Genome and virulence determinants of high virulence community-acquired mrsa, Lancet, 2002, 359, 1819–1827 CrossRef CAS.
- F. Rossi, L. Diaz, A. Wollam, D. Panesso, Y. Zhou, S. Rincon, A. Narechania, G. Xing, T. S. R. Di Gioia, A. Doi, T. T. Tran, J. Reyes, J. M. Munita, L. P. Carvajal, A. Hernandez-Roldan, D. Brandão, I. M. van der Heijden, B. E. Murray, P. J. Planet, G. M. Weinstock and C. A. Arias, Transferable vancomycin resistance in a community-associated mrsa lineage, N. Engl. J. Med., 2014, 370, 1524–1531 CrossRef CAS PubMed.
- A. Vassallo, S. Kett, D. Purchase and M. Marvasi, Antibiotic-resistant genes and bacteria as evolving contaminants of emerging concerns (e-cec): is it time to include evolution in risk assessment?, Antibiotics, 2021, 10, 1066 CrossRef CAS PubMed.
- F. Enault, A. Briet, L. Bouteille, S. Roux, M. B. Sullivan and M. A. Petit, Phages rarely encode antibiotic resistance genes: a cautionary tale for virome analyses, ISME J., 2017, 11, 237–247 CrossRef CAS PubMed.
- S. Redondo-Salvo, R. Fernández-López, R. Ruiz, L. Vielva, M. de Toro, E. P. C. Rocha, M. P. Garcillán-Barcia and F. de la Cruz, Pathways for horizontal gene transfer in bacteria revealed by a global map of their plasmids, Nat. Commun., 2020, 11, 1–13 CrossRef PubMed.
- G. Garriss, M. K. Waldor and V. Burrus, Mobile antibiotic resistance encoding elements promote their own diversity, PLoS Genet., 2009, 5, e1000775 CrossRef PubMed.
- M. E. Schoen, T. K. Peckham, J. H. Shirai, J. C. Kissel, D. Thapaliya, T. C. Smith and J. S. Meschke, Risk of nasal colonization of methicillin-resistant staphylococcus aureus during preparation of contaminated retail pork meat, Microb. Risk Anal., 2020, 16, 100136 CrossRef.
- S. Anderson, R. Woo and L. Crawford, Risk assessment of the impact on human health of resistant campylobacter jejuni from fluoroquinolone use in beef cattle, Food Control, 2001, 12, 13–25 CrossRef CAS.
- H. S. Hurd, M. B. Vaughn, D. Holtkamp, J. Dickson and L. Warnick, Quantitative risk from fluoroquinolone-resistant salmonella and campylobacter due to treatment of dairy heifers with enrofloxacin for bovine respiratory disease, Foodborne Pathog. Dis., 2010, 7, 1305–1322 CrossRef CAS PubMed.
- S. J. G. Otto, C. A. Carson, R. L. Finley, M. K. Thomas, R. J. Reid-Smith and S. A. Mcewen, Estimating the number of human cases of ceftiofur-resistant salmonella enterica serovar heidelberg in québec and ontario, canada, Clin. Infect. Dis., 2014, 59, 1281–1290 CrossRef CAS PubMed.
- L. A. Cox, D. A. Popken and J. J. Mathers, Human health risk assessment of penicillin/aminopenicillin resistance in enterococci due to penicillin use in food animals, Risk Anal., 2009, 29, 796–805 CrossRef PubMed.
- L. A. Cox and D. A. Popken, Quantifying human health risks from virginiamycin used in chickens, Risk Anal., 2004, 24, 271–288 CrossRef PubMed.
- L. A. Cox, D. A. Popken, J. Sun, X. P. Liao and L. X. Fang, Quantifying human health risks from virginiamycin use in food animals in china, Risk Anal., 2020, 40, 1244–1257 CrossRef PubMed.
- H. Hurd, S. Doores, D. Hayes, A. Mathew, J. Maurer, P. Silley, R. S. Singer and R. N. Jones, Public health consequences of macrolide use in food animals: a deterministic risk assessment, J. Food Prot., 2004, 67, 980–992 CrossRef CAS PubMed.
- H. S. Hurd, C. Enøe, L. Sørensen, H. Wachman, S. M. Corns, K. M. Bryden and M. Grenier, A stochastic assessment of the public health risks of the use of macrolide antibiotics in food animals, Risk Anal., 2008, 28, 695–710 CrossRef PubMed.
- S. Chandrasekaran and S. C. Jiang, A dose response model for quantifying the infection risk of antibiotic-resistant bacteria, Sci. Rep., 2019, 9, 1–11 CrossRef CAS PubMed.
- M. R. Gillings, M. P. Holley and H. W. Stokes, Evidence for dynamic exchange of qac gene cassettes between class 1 integrons and other integrons in freshwater biofilms, FEMS Microbiol. Lett., 2009, 296, 282–288 CrossRef CAS PubMed.
- A. Moura, I. Henriques, K. Smalla and A. Correia, Wastewater bacterial communities bring together broad-host range plasmids, integrons and a wide diversity of uncharacterized gene cassettes, Res. Microbiol., 2010, 161, 58–66 CrossRef CAS PubMed.
- L. Rizzo, C. Manaia, C. Merlin, T. Schwartz, C. Dagot, M. C. Ploy, I. Michael and D. Fatta-Kassinos, Urban wastewater treatment plants as hotspots for antibiotic resistant bacteria and genes spread into the environment: a review, Sci. Total Environ., 2013, 447, 345–360 CrossRef CAS PubMed.
- J. Gatica, V. Tripathi, S. Green, C. M. Manaia, T. Berendonk, D. Cacace, C. Merlin, N. Kreuzinger, T. Schwartz, D. Fatta-Kassinos, L. Rizzo, C. U. Schwermer, H. Garelick, E. Jurkevitch and E. Cytryn, High throughput analysis of integron gene cassettes in wastewater environments, Environ. Sci. Technol., 2016, 50, 11825–11836 CrossRef CAS PubMed.
- K. Kathirvel, O. Rudhra, S. G. K. Rajapandian, N. Venkatesh Prajna, P. Lalitha and B. Devarajan, Characterization of antibiotic resistance and virulence genes of ocular methicillin-resistant staphylococcus aureus strains through complete genome analysis, Exp. Eye Res., 2021, 212, 108764 CrossRef CAS PubMed.
- L. Jin, A. Pruden, A. B. Boehm, P. J. J. Alvarez, L. Raskin, T. Kohn and X. Li, Integrating environmental dimensions of “One Health” to combat antimicrobial resistance: Essential research needs, Environ. Sci. Technol., 2022 DOI:10.1021/acs.est.2c01651.
|
This journal is © The Royal Society of Chemistry 2022 |