Integrating advanced fitting models with experimental catalysis to maximize H2 production in dry reforming using nickel on metalized-silica-alumina catalysts†
Received
1st July 2024
, Accepted 13th September 2024
First published on 13th September 2024
Abstract
This study explores the enhancement of hydrogen production via dry reforming of methane (DRM) using nickel catalysts supported on metalized silica-alumina. By incorporating noble metals (Ir, Pd, Pt, Ru, and Rh), we significantly improve the catalysts' reducibility, basicity, and resistance to coke deposition. Our novel approach integrates a predictive model combining advanced statistical and experimental techniques to optimize catalyst performance. The active site population derived from the reduction of the NiAl2O4 phase is found to be stable and least affected under oxidizing gas stream (CO2) as well as reducible gas stream (H2) during the DRM reaction. The catalyst system is characterized by surface area and porosity, X-ray diffraction, Raman spectroscopy, thermogravimetry analysis, XPS, TEM, and various temperature-programmed reduction/desorption techniques (TPR/CO2-TPD). Notably, the 5Ni/1IrSiAl catalyst shows reduced activity due to low reducibility and basicity, whereas the 5Ni/1RhSiAl catalyst demonstrates superior performance, achieving a hydrogen yield of 62% at 700 °C and 80% at 800 °C after 300 minutes. This enhancement is attributed to the highest edge of reducibility, the maximum concentration of stable active sites “Ni” (derived from NiAl2O4 during the DRM reaction), and the optimum concentration of moderate strength basic sites. Through the application of multiple response surface methodology and central composite design, we developed a predictive model that forecasts the optimal conditions for maximizing hydrogen yield, which was experimentally validated to achieve 95.4% hydrogen yield, closely aligning with the predicted 97.6%. This study not only provides insights into the mechanistic pathways facilitated by these catalysts but also demonstrates the efficacy of computational tools in optimizing catalytic performance for industrial applications.
1. Introduction
The concentration of greenhouse gases, such as CO2 and CH4, has already passed the critical level, and now, each continent is suffering from the adverse effects of global warming. Global warming significantly affects seasonal cycles, thereby changing agricultural productivity and biodiversity.1,2 At this crucial stage, catalyst development for the dry reforming of methane reaction has drawn significant attention because of its capacity to convert greenhouse gases, CH4 and CO2, together. Again, the products of DRM are H2 and CO, which can be utilized to generate clean hydrogen energy and various synthetic applications through hydrogen. The dry reforming reaction is presented as eqn (1). It is a highly endothermic reaction and needs a high reaction temperature of 650 °C to 900 °C. Along with DRM, other competitive reactions are also running parallel in the same temperature range. The carbon formation reactions are CH4 decomposition and CO disproportionation1 (eqn (2) and (3)). The consumption of H2 by CO2 is readily evident along with DRM, known as the reverse water gas shift reaction (eqn (4)). | CH4 + CO2 ↔ 2CO + 2H2, ΔH2980 = +247 kJ mol−1 | (1) |
| CH4 ↔ C + 2H2, ΔH2980 = +75 kJ mol−1 | (2) |
| 2CO ↔ CO2 + C, ΔH2980 = −171 kJ mol−1 | (3) |
| H2 + CO2 ↔ CO + 2H2O, ΔH2980 = 41 kJ mol−1 | (4) |
Within the search for innovative solutions to utilize carbon and hydrogen sources more effectively, the dry reforming of methane presents compelling possibilities. Concurrently, the abundant availability of plastic waste, rich in carbon and hydrogen, positions it as an underexplored resource that could synergize with catalytic reforming processes to address both energy production and environmental restoration.
The DRM reaction scheme is broadly summarized in two steps: dissociation of “CH4 (into CHx; x = 1–4) and CO2 (into CO and O)” and subsequent oxidation of CHx by surface adsorbed oxygen. Among noble metals, the binding energy difference between CHx and O was found in the following order: Ru > Pd > Pt.2 The carbon deposition rate over noble metals is also in the same order. The noble metals are superior to the non-noble metal Ni in activity and durability towards the dry reforming of methane. However, high cost is the major limitation in the industrialization of noble metal-based catalysts. Mainstream catalytic development focuses on Ni-based catalyst systems, adding a small amount of noble metal as a secondary metal.3 The promotional addition of noble metals over a Ni-based catalyst does not lead to high costs and may maintain activity as high as a noble metal-based catalyst. The addition of the promoter enhanced both reducibility and catalytic activity.4 When a noble metal is added to Ni, H2 gas is effectively dissociated over the noble metal, and the dissociated hydrogen is spilt from the noble metal to NiO and turns NiO into metallic Ni. In the meantime, Ni–M (M = Pt, Pd, Ir, Ru, Rh) catalyst reducibility was enhanced. Furthermore, the addition of noble metals over Ni was also found to increase the dispersion of Ni, limit the particle size, and effectively inhibit carbon deposition.5–7 However, the CH4 dissociation barrier is also increased with alloy surfaces compared to Ni.6,8,9
Noble metals like Ru and Rh have low solubility with Ni, so with Ni, it forms a heterogenous bimetallic phase after reduction.9,10 Due to the separate identities of both metallic phases, the noble metal becomes specified for hydrogen spill, which is also helpful for retaining the metallic phase of the second metal (Ni). The Rh–Ni catalyst system over Al2O3 showed enhanced reducibility and a higher ability to dissociate H2 at a much lower temperature than the respective mono-metal catalyst.11 Ru was found to change the type of carbon deposition from a persistent graphitic one to a soft system. Graphitic-type carbon can be removed by O2, whereas soft carbon systems can be readily removed by CO2.9,12 The DRM activity over Ni-loaded or Ru–Ni-loaded catalysts is found in the following order: Al2O3 > MgAl2O4 > ZrO2 > SiO2. The Re-based catalyst system was not active below 900 °C, but the Re–Ni system showed DRM activity at relatively lower temperatures.12 The isotopically labelled 13CO2 experiment showed that carbon was deposited over Pt through the CO disproportionation reaction, whereas, over Pt–Ni, it was mainly deposited through CH4 decomposition.13 Both bidentate formate species (from CO2) and CO-species (from CH4) are formed over the Pd surface.14 The carbon bulk diffusion is higher over Ni–Pd than Ni.15 The mesoporous silica-supported nickel–palladium catalyst showed a larger fraction of exposed active sites (Ni°) than mesoporous silica-supported nickel.3 The catalytic activity of the Ni–Pd catalyst over different supports was found in the order SiO2 > Al2O3 > TiO2.16 On account of the Ni-based catalyst, nickel is poorly dispersed over silica,17,18 and Ni dispersed over alumina encounters additional acidity, leading to huge carbon deposition.19 Overall, individual silica-supported Ni or alumina-supported Ni refers to inferior catalytic activity. Upon increasing the proportion of alumina with silica, the Ni dispersion is increased.20 In the same way, the addition of silica over Ni/Al2O3 was found to enhance metal support interaction.21,22 Again, the acidity features of the support can be tuned by controlling the Si/Al ratio in the silica-alumina support. The study of Kustov et al. investigated the impact of support materials on nickel catalysts for methane dry reforming. Nickel on modified alumina-silica (SiO2–Al2O3) exhibited superior performance, emphasizing the importance of support selection for this reaction.23 The use of a silica-modified-alumina support for the mainstream catalyst (Ni + noble metal) may be more productive towards DRM. After developing a suitable mainstream catalyst, the next task is to set the best reaction conditions and gas feed composition for achieving the highest H2 yield. This task needs plenty of experiments under different conditions, which consume a lot of time and workforce. Nowadays, we have statistical modelling tools like response surface methodology (RSM) and OFAT (one factor at a time) to optimize the conditions on the reduced number of experiments.24–27 RSM has the advantage over OFAT. RSM may also consider the interaction between independent process parameters (factor) that influence the catalytic activity.28,29 In the first study, it is discussed how biogas can be turned into useful fuels like hydrogen through the process of dry reforming. The scientists created perovskite catalysts and evaluated their functionality. They discovered that replacing lanthanum with samarium or praseodymium improved stability and reduced coke buildup. Moreover, a theoretical model accurately predicted the dry reforming process, confirming its potential for future catalyst development.30 In traditional models for dry methane reforming (DMR) in solid oxide fuel cells (SOFCs), the influence of electrical current (EPOC effect) is often overlooked, leading to inaccurate performance predictions. To estimate DMR performance in SOFCs, researchers developed a model based on machine learning that considers EPOC. This innovative model is the first and provides a valuable tool for optimizing future SOFC designs.31 A new nickel-based catalyst efficiently converts methane and CO2 into syngas. Researchers utilized advanced computer modeling to optimize the process. They found that artificial neural networks outperform traditional methods in predicting the best reaction conditions for high conversion rates and desired product ratios. This approach opens the door to more efficient design of chemical processes.32 A novel machine learning model has effectively forecasted and optimized a technique for converting methane and CO2 into syngas. The model has discerned the optimal conditions for the process and has determined that the total flow rate exerts the most significant influence on conversion, while the nickel content exhibits the least impact. This methodology presents a promising avenue for crafting efficient chemical processes with limited data.33
The Ni catalyst supported on “metalized-silica-alumina” is synthesized. 5Ni/1xSiAl (x = Ir, Pd, Pt, Rh, Ru) catalysts are investigated for DRM and characterized by surface area and porosity, X-ray diffraction, Raman spectroscopy, thermogravimetry analysis, and various temperature-programmed reduction/desorption techniques. The fine-tuning of characterization results with catalytic activity is addressed. The best reaction conditions in the DRM process often involve the selection of the most effective catalyst. This selection needs techniques like response surface methodology (RSM) with centre composite design (CCD). Through CCD, various factors such as temperature, gas hourly space velocity, and CH4/CO2 ratio on the catalyst surface are systematically varied, and their effects on the system response (H2-yield) are studied. A model of the polynomial equation is developed to present and explain the relationship between these factors and the predicted H2 yield. The accuracy and the goodness of fit of this model are assessed by comparing them with experimental results using statistical tools like R2, absolute percentage error (APE), mean absolute errors (MAE), and mean absolute percentage error (MAPE). Ultimately, the goal is to determine the optimal conditions for maximizing H2 yield over the chosen catalyst system for “DRM”. The study aims to improve hydrogen production through dry methane reforming (DRM) by adding noble metals to nickel supported on (SiO2 + Al2O3) catalysts. A computer model for optimization will be used to forecast the best reaction conditions for maximizing hydrogen output. Various characterization techniques, including the N2-physisorption, XRD, Raman, TGA, TPD, CO2-TPD, XPS, and TEM, will be implemented to understand the factors affecting catalyst performance in the reaction.
Herein, a mainstream catalyst composed of Ni as the primary metal and noble metals (Ir, Pd, Pt, Rh, or Ru) as secondary metals integrated into a silica-alumina support is developed with the target of enhancing reducibility, coke resistance, and catalytic activity in DRM. Nowadays, “noble metal-modified silica-alumina” is directly supplied by the company, and catalyst preparation by impregnation of Ni over these supports will be an easy task for semiskilled workers in the industry. It indicates the practical applicability of the developed catalyst. Overall, our research advances the field of dry reforming by introducing a pioneering predictive model that synergizes advanced statistical methodologies with experimental catalysis techniques, offering a robust framework for optimizing nickel-based catalyst compositions and operational conditions, thereby setting new benchmarks in catalytic efficiency and sustainability.
2. Experimental
2.1 Materials used
Riogen Inc., 1 Deer Park Dr, Suite L3, Monmouth Junction, NJ 08852, provided the employed supports, which comprised 1% of Ir, Pd, Pt, Rh, or Ru in silica-alumina. Ni (NO3)2·6H2O, nickel nitrate hexahydrate was supplied by Alfa Aesar (98% purity). Using a Milli-Q water purification system (Millipore), ultrapure water was produced and used for all experimental needs.
2.2 Catalyst preparation
The wet impregnation technique was employed to synthesize the catalysts. Ni nitrate hexahydrate (Ni(NO3)3·6H2O, 99%) served as the active metal for the metalized-silica alumina-supported catalysts. In the target of preparing 0.1 g catalyst for DRM, the Ni precursor (equivalent to 5 wt% Ni) and support (equivalent to 0.95 wt%) are taken. For this, 0.02477 g of nickel nitrate hexahydrate and 0.095 g of metallized-silica-alumina support are taken with 20 mL of ultrapure water under stirring. The solution was magnetically stirred at 80 °C for two hours to guarantee complete mixing and uniform dispersion of the precursor on the support. The mixture was then allowed to dry at 120 °C for the entire night to remove any remaining solvents or water. After that, the dry mixture was calcined for three hours at a fixed temperature of 800 °C. The precursor-support mixture was heated to a high temperature, which caused it to solidify and encourage the formation of active catalyst sites. The catalyst material was finely ground into a powder so that it could be used in chemical reactions. 5Ni/1xSiAl (x = Ir, Pd, Pt, Ru, and Rh) was the designation given to the prepared catalysts (Table 1), where x is the type of metal present in the support, such as Pd, Pt, Ir, Ru, and Rh. The ESI† contains detailed descriptions of the catalyst's characterization and performance evaluation under the S1 and S2† headings.
Table 1 The comparative table of catalytic activity over closely related catalyst systems
Catalyst namea |
Catalyst weight (g) |
CH4 : CO2 |
GHSV (cm3 h−1 gcat−1) |
RT (°C) |
TOS (hour) |
H2-yield (%) |
Ref. |
Catalyst name is presented as a numerical value followed by an element symbol in most of the entries. The numerical value is the weight % of the element. Cat. Wt, catalyst weight; RT, reaction temperature, TOS, time on stream. In each case, the thermocouple is positioned axially at the centre of the catalyst bed.
|
Ni/Al2O3 |
0.1 |
1 |
42 000 |
700 |
7 |
55 |
34
|
5Ni/MgO |
0.1 |
1 |
42 000 |
700 |
7 |
69 |
35
|
5Ni/ZrO2 |
0.1 |
1 |
42 000 |
700 |
7 |
43 |
36
|
5Ni/MgO + TiO2 |
0.1 |
1 |
42 000 |
700 |
7 |
61 |
37
|
Ni/MgO + Al2O3 |
0.1 |
1 |
42 000 |
700 |
7 |
71 |
37
|
5Ni/MgO + ZrO2 |
0.1 |
1 |
42 000 |
700 |
7 |
46 |
37
|
5Ni5Mg/ZrO2 |
0.1 |
1 |
42 000 |
700 |
7 |
23 |
38
|
5Ni1Ce/ZrO2 |
0.1 |
1 |
42 000 |
700 |
∼7 |
47 |
36
|
5Ni/10La90Zr |
0.1 |
1 |
42 000 |
700 |
7 |
78 |
39
|
5Ni/9La91Zr |
0.1 |
1 |
42 000 |
700 |
∼7 |
80 |
36
|
5Ni2.5Ce/9La91Zr |
0.1 |
1 |
42 000 |
700 |
∼7 |
87 |
36
|
5Ni/9La91Zr |
0.1 |
1 |
42 000 |
700 |
∼7 |
58 |
40
|
5Ni1Gd/9La91Zr |
0.1 |
1 |
42 000 |
700 |
∼7 |
80 |
40
|
5Ni1Cr/9La91Zr |
0.1 |
1 |
42 000 |
700 |
∼7 |
81 |
40
|
5Ni/9W91Zr |
0.1 |
1 |
42 000 |
700 |
∼7 |
43 |
41
|
5Ni2.5Ce/9W91Zr |
0.1 |
1 |
42 000 |
700 |
7 |
78 |
41
|
5Ni15YZrO2 |
0.1 |
1 |
42 000 |
700 |
7 |
55 |
38
|
5Ni/15Y85Zr |
0.1 |
1 |
42 000 |
700 |
7 |
64 |
42
|
5Ni/13Y77Zr |
0.1 |
1 |
42 000 |
700 |
∼7 |
67 |
43
|
NiSr/Y2O3–ZrO2 |
0.1 |
1 |
42 000 |
700 |
7 |
62 |
38
|
NiGa/Y2O3–ZrO2 |
0.1 |
1 |
42 000 |
700 |
7 |
58 |
38
|
5Ni2Ce/13Y77Zr |
0.1 |
1 |
42 000 |
700 |
∼7 |
80 |
43
|
5Ni4Ba/13Y77Zr |
0.1 |
1 |
42 000 |
800 |
∼7 |
80 |
44
|
5Ni4Ho/13Y77Zr |
0.1 |
1 |
42 000 |
700 |
∼7 |
84 |
43
|
La0.6Ce0.4Ni0.9Zr0.1O3 |
0.1 |
1 |
42 000 |
800 |
7 |
83 |
45
|
CeNi0.9Zr0.01Y0.09O3 |
0.1 |
1 |
42 000 |
800 |
7 |
85 |
45
|
5Ni3Si/Al |
0.1 |
1 |
42 000 |
700 |
∼7 |
62 |
46
|
5Ni3W/Al |
0.1 |
1 |
42 000 |
700 |
∼7 |
62 |
46
|
5Ni3TiAl2O3 |
0.1 |
1 |
42 000 |
700 |
7 |
30 |
46
|
5Ni3MoAl2O3 |
0.1 |
1 |
42 000 |
700 |
7 |
39 |
46
|
5Ni/ZSM-5 |
0.15 |
1 |
42 000 |
700 |
18 |
27 |
47
|
5Ni2Ce/ZSM5 |
0.15 |
1 |
42 000 |
800 |
5 |
68 |
47
|
5Ni/1RhSiAl |
0.1 |
1 |
42 000 |
700 |
5 |
62 |
This work |
5Ni/1RhSiAl |
0.1 |
1 |
42 000 |
800 |
5 |
80 |
This work |
2.3 The design of experiment (DoE) and process optimization
The three experimental factors or reaction parameters (xi; i = 1, 2, 3), namely gas hourly space velocity (22
000, 32
000, 42
000 cm3 per g-cat per h), temperature (700 °C, 750 °C, 800 °C) and CH4/CO2 ratio (0.5, 0.75, 1), are considered for the design of experiment. Each factor has a lower limit (ximin), an upper limit (ximin), the centre point of the design (
oithe = (ximax + ximin)/2) and deviation from the mean (Δxi = (ximax − xithemin)/2). The centre point of the design is coded into a dimensionless variable (Xi) in the range of +1 and −1 through the following equation; Xi = (xi −
oi)/Δxi. These codes are the actual value of factors. The linear model of three factors approximates the system response “Ŷ through a full quadratic polynomial equation using Taylor Series expansion (eqn (5)).34 |  | (5) |
where Ŷ, X1, X2, X3, β0, βi, βii, βij; i = 1, 2, 3, ε are the predicted response system (H2 yield), the factors that affect the response variable in coded or actual values, the regression coefficients of intercept term, linear terms, quadratic terms, interaction terms and error terms, respectively. The fitness of the proposed model was assessed using analysis of variance (ANOVA) at 0.05 significance level (α). The significance of the model is validated by Fisher variation (F-value) and the probability value (P-value). The basic terms of variance and prediction statistics are mentioned in ESI S3.† Excluding the deemed insignificant factor and identifying significant factors, an equation capturing the linear impact of factors on the response variable is proposed by using the Design-Expert software package version. By using this model, the predicted values of the response variable (predicted H2 yield) are calculated. The precision of this predictive model is assessed using R2, absolute percentage error (APE), mean absolute errors (MAE) and mean absolute percentage error (MAPE). In the end, the effect of process parameters on the system response is presented by 2D (one-factor effect) and 3D (two-factor effect) simulation on the design expert program.
3. Results and discussion
3.1 Catalyst activity results
An empty stainless-steel reactor was used for the initial blank experiment, which was carried out without any catalysts. The same reaction temperatures and feed ratios were used in the experiment. The blank's CH4 and CO2 conversions at 700 °C were 0.76% and 0.74%, respectively, according to the data, and its H2/CO ratio was almost 0.18, indicating a very slow, uncatalyzed reaction in the gas phase. Table S1† displays the results of the blank test. The catalytic activities of 5Ni/1xSiAl (x = Ir, Pd, Pt, Ru, and Rh) catalysts in terms of H2 yield and CO yield are shown in Fig. 1. The 5Ni/1IrSiAl catalyst's initial H2 yield and CO yield are 41% and 45%, respectively, which are lower than those of the other catalytic systems. At the end of 300 minutes, the H2 yield and CO yield are slowed down to 33% and 37%, respectively. Interestingly, the initial activity of the three catalysts, namely 5Ni/1RuSiAl, 5Ni/1PtSiAl, and 5Ni/1PdSiAl catalysts, is the same (48% H2 yield and 53% CO yield). However, the activity reduction over the 5Ni/1RuSiAl catalyst is minimal after 300 minutes, and the activity drop over the 5Ni/1PdSiAl catalyst is greatest. Following a 300-minute duration, the catalytic activity of each catalyst is observed in the subsequent order: 5Ni/1RuSiAl (YH2 = 41%, YCO = 47%) > 5Ni/1PtSiAl (YH2 = 36%, YCO = 41%) > 5Ni/1PdSiAl (YH2 = 28%, YCO = 29%). The catalytic activity of the Rh-metalized 5Ni/1RhSiAl catalyst reaches its peak performance. The 5Ni/1RhSiAl catalyst has achieved 62% H2 yield and 66% CO yield at the end of 300 minutes. According to the stoichiometry of the DRM reaction, H2 yield and CO yield remain equal. However, it is always noticeable that the CO yield is always slightly more than the H2 yield over the current catalyst system (Fig. 1C). It indicates the presence of an H2-consuming reaction like a reverse water gas shift reaction over the catalyst surface. The effect of temperature on the DRM reaction over the best catalyst (5Ni/1RhSiAl) is presented in Fig. 1D. Over the 5Ni/1RhSiAl catalyst, the H2 yield is just 2.5% and 6.5% at 450 °C and 500 °C, respectively. With increasing the temperature from 550 °C to 800 °C, the H2 yield is increased from 16.3% to 80%. The increase of catalytic activity with increasing temperature towards DRM indicates the endothermic nature of the reaction. Table 1 displays the catalytic activity of a closely related catalyst system operated at 42
000 cm3 per g-cat per h gas hourly space velocity and CH4/CO2 gas feed ratio 1 and 700–800 °C reaction temperature over 0.1–0.15 g catalyst.34–47 Under the given conditions, the 5Ni/1RhSiAl catalyst is found to be quite competent with the lanthana-zirconia supported Ni catalyst system and the yttria-zirconia supported Ni catalyst system. The supremacy of the current catalyst can be further proven by optimizing reaction conditions and it is studied in Section 3.7.
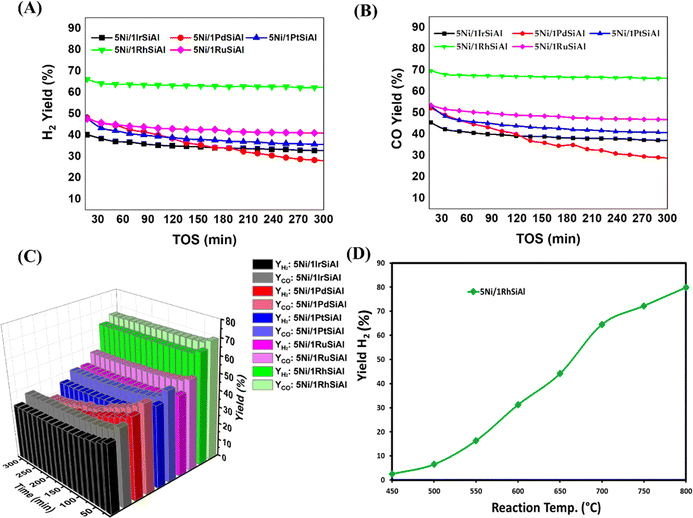 |
| Fig. 1 Catalytic activity results in 5Ni/1xSiAl (x = Ir, Pd, Pt, Ru, and Rh) catalysis: (A) H2 yield vs. TOS, (B) CO yield vs. TOS, (C) “H2 yield-CO yield” vs. TOS (min), (D) H2 yield vs. reaction temperature. Reaction condition of (A–C): reduction temperature: 800 °C, reaction temperature 700 °C, gas hourly space velocity 42 000 cm3 per g-cat per h and CH4: CO2: N2 gas feed ratio 3 : 3:1. Reaction condition of (D): all conditions are the same as A–C except temperature. | |
3.2 Characterization results
The surface area and porosity results of 5Ni/1xSiAl (x = Ir, Pd, Pt, Ru, and Rh) catalysts are shown in Fig. 2 and Table 2. All catalysts show type IV adsorption isotherms with an H1 hysteresis loop, indicating the presence of cylindrical mesopores of invariable pore size 6.2 nm. For the H1 hysteresis loop, the desorption branch is recommended for pore analysis.48 The dV/dlog
W vs. W plot (V is volume and W is pore width) also shows monomodal pore size distribution and pores of size in the range 6.6–6.3 nm are exclusively present over the catalyst (inset figures in Fig. 2). Upon metallization of the support using 1 wt% noble metals, the surface area of the 5Ni/1xSiAl (x = Ir, Pd, Pt, Ru, and Rh) catalyst is influenced markedly. 5Ni/1PtSiAl has the highest surface area (344 m2 g−1), whereas the 5Ni/1RuSiAl catalyst has the least surface area (313 m2 g−1) and pore volume (0.51 cm3 g−1). The surface areas of 5Ni/1PdSiAl and 5Ni/1RhSiAl catalysts are comparable (334–338 m2 g−1). The surface parameter of the 5Ni/1IrSiAl catalyst can be specified by the presence of the largest pore volume (0.54 cm3 g−1).
 |
| Fig. 2 The adsorption isotherm and porosity of (A) 5Ni/1IrSiAl, (B) 5Ni/1PdSiAl, (C) 5Ni/1PtSiAl, (D) 5Ni/1RhSiAl and (E) 5Ni/1RuSiAl. | |
Table 2 The surface area, pore volume, and pore diameter of 5Ni/1xSiAl (x = Ir, Pd, Pt, Ru, and Rh) catalysts
Sample |
BET-surface area (m2 g−1) |
Pore volume (cm3 g−1) |
Average-pore diameter (nm) |
5Ni/1IrSiAl |
341 |
0.54 |
6.3 |
5Ni/1PdSiAl |
334 |
0.53 |
6.3 |
5Ni/1PtSiAl |
344 |
0.52 |
6.2 |
5Ni/1RhSiAl |
338 |
0.52 |
6.2 |
5Ni/1RuSiAl |
313 |
0.51 |
6.3 |
The X-ray diffraction patterns of 5Ni/1xSiAl (x = Ir, Pd, Pt, Rh, Ru) catalysts are shown in Fig. 3. 5Ni/1xSiAl (x = Ir, Pd, Pt, Rh, Ru) catalysts have a crystalline hexagonal silicon oxide phase (at Bragg's angle 2θ = 28.16°; JCPDS reference number 01-081-0067), cubic NiO phase (at Bragg's angle 37.2°, 43.2°, 62.8°; JCPDS reference number 01-078-0423) and cubic nickel aluminum oxide phase (at Bragg's angle 2θ = 37°, 45°, 65.5°; JCPDS reference number 00-001-1299). The Ir-metalized 5Ni/1IrSiAl catalyst has an iridium oxide phase (at Bragg's angle 27.7°, 34.2°, 53.6°).49 In the same way, ruthenium, platinum, and palladium metalized catalysts have a tetragonal-ruthenium oxide phase (28°, 35°, 54°; JCPDS reference number 00-021-1172), cubic platinum oxide (at Bragg's angle 2θ = 41°; JCPDS reference number 01-074-1879), and tetragonal palladium oxide phase (at Bragg's angle 2θ = 34°; JCPDS reference number 00-041-1107) respectively. Noticeably, the cubic alumina phase is found at a higher Bragg angle (at 2θ = 45.8°, 66.8°; JCPDS reference number 00-001-1303) than the cubic NiAl2O4 phase over the 5Ni/1PtSiAl catalyst.26,27 Over the 5Ni/1RhSiAl catalyst, mostly the cubic NiAl2O4 phase is detected. It indicates that at the calcination temperature, Ni2+ may get sufficient energy to overcome the alumina's surface barrier; after that, Ni may interact with Al2O3 and form a spinel NiAl2O4 phase. It seems that in the presence of Rh, the reaction between NiO and Al2O3 is speeding up, and NiAl2O4 is formed exclusively over the 5Ni/1RhSiAl catalyst rather than the 5Ni/1PtSiAl catalyst.
 |
| Fig. 3 X-ray diffraction pattern of (A) 5Ni/1PtSiAl and 5Ni/1RuSiAl, (B) 5Ni/1IrSiAl, 5Ni/1PdSiAl and 5Ni/1RhSiAl and (C) 5Ni/1xSiAl (x = Ir, Pd, Pt, Rh, Ru). | |
The Raman spectra of fresh 5Ni/1xSiAl (x = Ir, Pd, Pt, Ru, and Rh) catalysts are shown in Fig. 4A. The Raman spectra for Ni–O vibrations are coupled with the vibration of the alumina support. The Raman peaks of about 508 cm−1 and 860 cm−1 are attributed to the T2g vibration mode of NiO over Al2O3 and the A1g vibration mode of NiO in NiAl2O4, respectively.50,51 Apart from these, other Raman bands at 410 cm−1, 508 cm−1, 679 cm−1, 857 cm−1, 924 cm−1, 1049 cm−1, and 1161 cm−1 also appear over the catalyst surface. It is interesting to note that the band position does not vary on the noble metal type. It indicates that the above Raman bands are related to alumina and silica. However, the characteristic peak for α-Al2O3 is absent at 378 cm−1 and 433 cm−1. The other two pure forms of alumina (γ-Al2O3 or η-Al2O3) were reported not to give rise to the Raman band. It can be anticipated that embedding the SiO4 unit in an aluminate matrix in the current catalyst system may give rise to various bands. In metalized-silica-alumina, the bands at 1160 cm−1, 1050 cm−1, 921 cm−1, and 410 cm−1 are associated with silicon–oxygen vibration, whereas the Raman band at 679 cm−1 is attributed to Al–O vibration.52,53 The Raman band of spent catalysts is shown in Fig. 4B. There is no carbon band for the Rh metalized 5Ni/1RhSiAl catalyst. The rest of the catalysts showed a Raman band at 1340 cm−1 for the D band (sp2 hybridized carbons in aromatic rings), ∼1580 cm−1 for the G band (sp2 hybridized carbons in aromatic rings and olefins), and a second-order band at 2671 (cm−1).54 The ID/IG ratio is highest (1.51) over the Pd metalized 5Ni/1PdSiAl catalyst. It indicates that carbon deposit over the 5Ni/1PdSiAl catalyst has a minimum degree of graphitization. Pt and Ir metalized 5Ni/1PtSiAl and 5Ni/1IrSiAl catalysts have equal intensity of ID and IG peaks of carbon. The thermogravimetry profile of the 5Ni/1xSiAl (x = Ir, Pd, Pt, Ru, and Rh) catalysts is shown in Fig. 4C. The TGA result shows that the spent 5Ni/1RhSiAl catalyst has minimum weight loss (5.31%), whereas 5Ni/1PdSiAl undergoes huge weight loss. When the Raman and TGA findings are compared, it can be concluded that spent 5Ni/1PdSiAl loses the most weight (41.38%) as a result of massive carbon deposition, with diamond-type carbon accounting for 1.5 times the amount of carbon in the carbon deposit compared to graphitic type carbon. The remaining spent 5Ni/1xSiAl catalysts (where x = Ir, Pt, and Ru) exhibit an 8–11% weight decrease and have an equal amount of graphitic and diamond-type carbon.
 |
| Fig. 4 (A) Raman spectra of fresh. (B) Raman spectra of spent. (C) Thermogravimetry analysis of spent 5Ni/1xSiAl (x = Ir, Pd, Pt, Ru, and Rh) catalysts operated at 700 °C reaction temperature and obtained after 300 min TOS. | |
Two carbon formation mechanisms have been discovered: dissolution–precipitation and surface growth.55–57 The carbon–Ni interface was reported as a nucleation center for carbon growth in the surface growth mechanism,57 whereas, in the dissolution–precipitation mechanism, carbon is dissolved at Ni metal and then precipitates/nucleates at the metal–support interface.56 Leung et al. showed that the carbon nano-structures are nurtured over catalyst without concurrent decreases in CH4 reforming under the dissolution–precipitation mechanism.46 Baker et al. used controlled atmosphere electron microscopy to directly observe how various metal and alloy particles catalyze carbon growth. They developed a mechanism to account for the growth characteristics of carbon.55 The researchers established methods for controlling the growth of these structures. They found that the addition of a second metal to the catalyst and the strength of the metal–support interaction can play an important role in modifying the carbon growth characteristics. In the current catalyst system also, it can be anticipated that the addition of a second metal like Ir, Pd, Pt, Rh, and Ru along with the first metal “Ni” may induce the carbon growth process as the 5Ni/1RhSiAl catalyst has no carbon band in Raman spectra, whereas the carbon deposit over the 5Ni/1PdSiAl catalyst has less degree of graphitization than 5Ni/1PtSiAl and 5Ni/1IrSiAl. It indicates that the addition of a second metal may affect metal–support interaction vis-à-vis modifying the carbon growth process.
To shed light on the carbon growth process, the TEM images of fresh and spent 5Ni/1RhSiAl catalysts are shown in Fig. 5. The spent 5Ni/1RhSiAl catalyst shows a multiwalled carbon nanotube which is free from partitions at intervals. It indicates that the wettability of quasi-liquid metal and carbon is good.58,59 The addition of a new carbon layer below the metal pushes the metal forward and leaves a hollow behind during the periodic elongation–contraction process of carbon nanotube growth. The diameter of the carbon nanotube and the diameter of the hollow region of the carbon nanotube are found to be 18.34 nm and 7.6 nm. The average particle size over the spent 5Ni/1RhSiAl catalyst is grown to 8 nm (concerning 4.18 nm in the fresh catalyst).
 |
| Fig. 5 Transmission electron microscopy of the fresh 5Ni/1RhSiAl catalyst (A) at 100 nm scale (B) 50 nm scale. Transmission electron microscopy of the spent 5Ni/1RhSial catalyst (D) at 100 nm scale (E) 50 nm scale (F) 50 nm scale (G) 20 nm scale (H) 5 nm scale. Particle size distribution of (C) fresh 5Ni/1RhSiAl catalyst (I) spent 5Ni/1RhSiAl catalyst. | |
Over the alumina-supported Ni catalyst, the reduction profiles of surface interacted NiO species and NiAl2O4 species are generally reported at intermediate (∼600 °C) and high temperature (∼800 °C) in the literature.21,60 The reduction peak at about 800 °C is evident over the 5Ni/1xSiAl (x = Ir, Pd, Pt, Ru, and Rh) catalyst system, but the reduction peak at about ∼600 °C is shifted to a lower temperature in the case of 5Ni/1PtSiAl and 5Ni/1RhSiAl catalysts (Fig. 6A). It indicates the higher edge of reducibility over 5Ni/1PtSiAl and Ni/1RhSiAl catalysts. In the 5Ni/1RhSiAl catalyst, no peaks are observed in the low-temperature range. It was reported that a catalyst sample that was calcined at a high temperature (700 °C) showed a diffuse reduction peak for RhOx at 400 °C. In contrast, if the same catalyst is calcined at a low temperature (300 °C), it has a reduction peak for RhOx at about 125 °C.61 Overall, it can be said that 5Ni/1RhSiAl has a higher edge of reducibility than the remaining catalysts, and the reduction peak centered about 470 °C is due to the reduction of RhOx as well as “surface interacted NiO”. Both reducible species RhOx and NiO are reduced in the same temperature range. It is reported that decorating noble metal (Rh) about Ni metal was found to maintain the metallic state of Ni by dissociating H2 over noble metal (Rh) and transferring spillover “H” to Ni.35,36 Apart from the Ni-related reduction profile, the noble metal oxide and metal hydride reduction peaks are also evident over the 5Ni/1xSiAl (x = Ir, Pd, Pt, Rh, and Ru) catalyst system. Ru-metalized 5Ni/1RuSiAl, Ir-metalized 5Ni/1IrSiAl, Pd metalized 5Ni/1PdSiAl and Pt metalized 5Ni/1PtSiAl catalysts have additional reduction peaks at 150 °C for RuO2,62,63 at 250 °C for IrO2,64 at 450 °C for PdO65 and at 450 °C for PtOx. The Pd-metalized 5Ni/1PdSiAl catalyst is also characterized by a negative reduction peak at 75 °C for PdHx.66 Some hydrogen was dissociatively adsorbed on the palladium and formed palladium hydrides, which are unstable.67 Palladium hydride is decomposed at 75 °C, and H2 is released, which is observed as a sharp negative reduction peak in the TPR profile. The diffuse negative peaks in other catalysts are due to hydrogen spillover into mesopores of Ni-containing silica-alumina.21,68
 |
| Fig. 6 (A) H2-TPR, (B) CO2-TPD of spent of 5Ni/1xSiAl (x = Ir, Pd, Pt, Rh, Ru) catalysts, (C) cyclic H2TPR-CO2TPD-H2TPR profile of the 5Ni/1IrSiAl catalyst, (D) cyclic H2TPR-CO2TPD-H2TPR profile of the 5Ni/1PdSiAl catalyst, (E) cyclic H2TPR-CO2TPD-H2TPR profile of the 5Ni/1PtSiAl catalyst, (F) cyclic H2TPR-CO2TPD-H2TPR profile of the 5Ni/1RhSiAl catalyst, (G) cyclic H2TPR-CO2TPD-H2TPR profile of the 5Ni/1RuSiAl catalyst, and (H) final H2-TPR (in cyclic H2TPR-CO2TPD-H2TPR profile) of the 5Ni/1xSiAl (x = Ir, Pd, Pt, Rh, and Ru) catalyst. | |
After reductive pre-treatment, the noble metal oxide/hydride, interacted NiO-species, and NiAl2O4 species are reduced into metallic form. The CH4 decomposition is carried out over the assembly of noble metal and Ni. Now, as per the basicity of the reduced catalyst, CO2 gas from the reaction feed interacts with the catalyst's surface. So, the CO2-TPD profile of the reduced catalyst needs to be explained. The CO2-TPD profile of the reduced catalyst is centered at about 150 °C and 375 °C Fig. 6B. Under reductive treatment, the surface hydroxyl and reducible metal oxide are reduced into water and metal, respectively. Therefore, it is not possible to link the low-temperature CO2 desorption peak, which is around 150 °C, to weak basic sites carried by surface hydroxyl. It is possible to correlate the desorption peak at around 150 °C and 375 °C with the basic sites of moderate strength and strong basic sites, respectively.60 The 5Ni/1IrSiAl catalyst has the lowest basic site intensity, while the 5Ni/1RhSiAl catalyst has the highest intensity of moderate-strength basic sites. The fundamental characteristics of the remaining catalysts, 5Ni/1PdSiAl, 5Ni/1PtSiAl, and 5Ni/1RuSiAl are comparable. The H2-TPR profile of the fresh catalyst and CO2-TPD profile of reduced catalysts give the idea of the extent of decomposition of CH4 as well as oxidation of the decomposed-CH4 species (CH4−x; x = 1–4) by CO2. The fine-tuning between reducibility and basicity of the catalyst controls the activity towards dry reforming of methane reaction. However, it should be mentioned that the metallic state of metal (active sites of reaction) may also be oxidized in the presence of oxidizing gas CO2.69 If it happens, catalyst activity will not persist longer. To understand the distribution of active sites under oxidizing gas CO2, we have carried out cyclic H2TPR-CO2TPD-H2TPR of all catalysts and found that the active sites are rearranged under CO2 gas feed (Fig. 6C–G). Upon sequential reduction (by first H2-TPR), oxidation (by CO2-TPD), and reduction (by last H2-TPR), it is found that the reduction peak of about 800 °C exists. The reduction peak in this temperature range is attributed to the reduction of NiAl2O4 species. In the longer run, the active sites derived from the reduction of NiAl2O4 remain present over the catalyst surface. The highest concentration of active sites is found over Rh and Pt-metalized catalysts, while the order of active site concentration over the other catalysts is observed in the following order: 5Ni/1RuSiAl > 5Ni/1PdSiAl > 5Ni/1IrSiAl (Fig. 6H).
After the 5Ni/1RhSiAl catalyst, the Ru metalized 5Ni/1Ru/SiAl catalyst has attained the highest DRM activity. To understand the distribution of charge and the type of surface oxide over 5Ni/1RhSiAl and 5Ni/1RuSiAl catalysts, XPS analysis is carried out in Fig. 7. The binding energy of Al(2p) at 74.30 eV confirms the Al+3 oxidation state.70 In the literature, the binding energy 103–104 eV is reported for SiO2.71,72 Si(2p) XPS spectra are noticed at about 102.6 eV. The decrease in binding energy indicates the rise of covalent character.21 In the literature, the binding energy at 102.6 eV is reported for aluminum(III) oxide silicate and kaolinite.73–75 The Si(2p) XPS spectra of the current catalyst system justify the bonding between silica and alumina through the Si–O–Al bond. In the same way, the peak doublet of Ni(2p) XPS spectra at 856.5 and 874.6 eV indicates the presence of Ni+2.76 O(1s) XPS spectra are discussed as overlapping the contribution of different metal oxides and surface-adsorbed species. O(1s) spectra at 532.3 eV are attributed to surface hydroxy species.77,78 Interestingly, the XPS spectra of both 5Ni/1RhSiAl and 5Ni/1RuSiAl catalysts are very similar. It shows that the charge distribution across the surface of the 5Ni/1RhSiAl and 5Ni/1RuSiAl catalysts does not significantly change with the addition of supports containing Rh and Ru.
 |
| Fig. 7 X-ray photoelectron spectra of 5Ni/1RhSiAl and 5Ni/1RuSiAl catalysts: (A) Al(2p) spectra, (B) Si(2p) spectra, (C) Ni(2p) spectra and (D) O(1s) spectra. | |
3.3 Discussion
The metalized-silica-alumina support has Si–O–Al linkages, which are capable of stabilizing the active metal over the catalyst surface for the high-temperature DRM reaction. 5Ni/1xSiAl (x = Ir, Pd, Pt, Rh, and Ru) catalysts have mesoporous cylindrical pores where the pore size remains invariant upon loading different types of supports. However, the surface area is influenced effectively by metal type, i.e., 5Ni/1PtSiAl and 5Ni/1RuSiAl catalysts have the highest and least surface area, respectively. However, the influence of the surface area on catalytic activity was not evident. The cubic NiAl2O4 phase is easily evident over the 5Ni/1xSiAl (x = Ir, Pd, Pt, Rh, and Ru) catalyst system. Cyclic H2TPR-CO2TPD-H2TPR results show that under the reducing and oxidizing gas stream (H2 and CO2) during DRM, active sites derived from the cubic NiAl2O4 phase remain present over the catalyst, which drives the reaction constantly. The cyclic H2TPR-CO2TPD-H2TPR experiment and XRD results show that the intensity of such active sites (derived from NiAl2O4) is minimal over the 5Ni/1IrSiAl catalyst. CO2 TPD of the reduced catalyst also indicates the presence of minimum basic sites over the 5Ni/1IrSiAl catalyst. The 5Ni/1IrSiAl catalyst's lower reducibility and basicity profile (compared to other catalysts) result in lower activity (YH2 = 33%, YCO = 37%) towards DRM. 5Ni/1RuSiAl, 5Ni/1PdSiAl, and 5Ni/1PtSiAl catalysts show similar catalytic activity (YH2 = 48%, YCO = 53%) towards DRM at the beginning. But at a higher time on stream, the catalyst's activity drops to a different extent. The possible reason for the depletion of catalytic activity over these three catalysts may be explained. The basic profiles of 5Ni/1RuSiAl, 5Ni/1PdSiAl, and 5Ni/1PtSiAl are similar. This means that all three surfaces have a similar extent of interaction with CO2. In terms of reducibility, the 5Ni/1PtSiAl catalyst has a higher edge of reducibility (than 5Ni/1RuSiAl and 5Ni/1PdSiAl) at the beginning of the reaction. Again, during the DRM reaction under reducing and oxidizing gas stream, the concentration of active sites derived from NiAl2O4 is highest over 5Ni/1PtSiAl. The strong interaction of “Pt with CO” and “Pd with CO” was reported,79,80 which may induce a carbon monoxide disproportionation reaction (2CO → CO2 + C). It results in huge coke deposition. Raman spectra and thermogravimetry analysis of spent catalysts show significantly high carbon deposition over 5N/1PdSiAl and 5Ni/1PtSiAl catalysts. Thermogravimetry analysis of the spent 5N/1PdSiAl catalyst shows a maximum ∼41% weight loss due to massive carbon deposition. It results in quick catalyst deactivation, and the H2 yield (%) decreased from 48% to 26% within the 300 minute time on stream. Of these three catalysts, the 5Ni/1RuSiAl catalyst had the least amount of carbon deposited on it. The H2-yield of the 5Ni/1RuSiAl catalyst is 41%. The 5Ni/1RhSiAl catalyst has the highest edge of reducibility at the beginning of the DRM reaction, the highest concentration of active sites derived from NiAl2O4 (during the DRM reaction), the highest concentration of moderate strength basic sites, and minimum carbon deposit. These favorable surface properties result in optimum catalytic activity (62% H2 yield) over the 5Ni/1RhSiAl catalyst at a 700 °C reaction temperature. It is needed to optimize reaction conditions to achieve the highest H2-yield over the 5Ni/1RhSiAl catalyst for DRM.
3.4 The proposed reaction mechanism over the Ni catalyst supported by metalized-silica alumina
Now, the proposed reaction mechanism over the current catalyst system needs to be elaborated. The initial requirement of DRM is stable active sites and basic sites for interaction/activation of CH4 and CO2, respectively. Over the current 5Ni/1MSiAl (M = Ir, Pt, Pd, Rh, Ru), there are two active centres, Ni and noble metal. Ni exsolved from the reduction of NiAl2O4 about 800 °C serves as stable active sites.21 Hydrogen dissociated at one metal centre is transferred to another metal centre, which helps to retain the metallic state of each other.1 In this way, stable active sites are retained during the DRM reaction over the catalyst. 5Ni/1IrSiAl contains few stable active sites (derived from NiAl2O4) and few basic sites resulting in inferior activity. Over the 5Ni/1RhSiAl catalyst, the maximum concentration of Ni (derived from NiAl2O4) is present. Overall, 5Ni/1RhSiAl is enriched with moderate strength basic sites and coke resistance properties, which ensures the best catalytic activity. The reaction over 5Ni/1RhSiAl is initiated as activation of the C–H bond (in methane) and C–O bond (in CO2) at active metal sites and basic sites of the catalyst respectively. CH4 is dissociated as CHx (x = 1–3) and (x/2) H2, whereas CO2 is dissociated into CO and O (eqn (6) and (7)). The dissociated atomic oxygen oxidizes CHx into the CHxO intermediate, which is further decomposed into (x/2) H2 and CO1,81 (eqn (8)). In total, equimolar CH4 and CO2 are converted into syngas with a stoichiometric H2/CO ratio of 1 (eqn (9)). At the same time, some of the carbon dissolves in metal and forms quasi-liquid metal (NixCy) with good wettability over 5Ni/1RhSiAl. The addition of a new carbon layer pushes metals forward and leaves a hollow behind, which results in the formation of carbon nanotubes. |  | (6) |
| CHx (x = 1–3) + O → CHxO → CO + (x/2) H2 | (8) |
| Total, CH4 + CO2 → 2CO + 2H2 | (9) |
3.5 Prediction and optimization processes
5Ni/1RhSiAl is found to be the best DRM catalyst over CH4
:
CO2
:
N2 gas feed (in 3
:
3
:
1 ratio) at 700 °C and 42
000 cm3 per g-cat per h gas hourly space velocity. The 5Ni/1RhSiAl catalyst is further considered for optimization of reaction conditions by RSM through center composite design (CCD). The center point of design for gas hourly space velocity, temperature, and CH4/CO2 ratio is coded into dimensionless variables X1, X2, and X3, respectively, in the range of +1 and −1, as shown in Table 3. The actual values of factors for the polynomial equation (shown in eqn (5)), which approximates the system response as predicted H2 yield (Y). The central composite design (CCD) method was employed to identify the optimal transformation, ensuring data normalization or variance equalization.4 The fitness of regression models was assessed using analysis of variance and prediction statistics in Table 3. This assessment is conducted at elevated R2 value (0.975), very small P-values (<0.0001), and high F-values within the 95% confidence limit. The fitted model demonstrates an accuracy of approximately 97% in accounting for the variability in H2 yield. After identifying significant factor effects and excluding those deemed insignificant, the suggested models were determined using Design-Expert software package version 13, as shown below. |  | (10) |
Table 3 Actual values, coded values, multi-regression statistics, and analysis of variance for the process parameters/factorsa
Process parameters |
Symbol (for the centre point of design) |
Actual value |
Coded values |
Sum of squares |
df |
Mean square |
F-value |
P-value |
T for temperature (°C) and SV for gas hourly space velocity (cm3 per g-cat per h).
|
Model |
— |
— |
— |
2979.78 |
3 |
993.26 |
182.32 |
<0.0001 |
A: T |
X
1
|
700 |
−1 |
1718.59 |
1 |
1718.59 |
315.45 |
<0.0001 |
750 |
0 |
800 |
+1 |
B: SV (cm3 per g-cat per h) |
X
2
|
22 000 |
−1 |
580.77 |
1 |
580.77 |
106.6 |
<0.0001 |
32 000 |
0 |
42 000 |
+1 |
C: CH4/CO2 |
X
3
|
0.5 |
−1 |
680.43 |
1 |
680.43 |
124.89 |
<0.0001 |
0.75 |
0 |
1 |
+1 |
The equation, expressed in terms of actual factors (T: temperature, SV: gas hourly space velocity and the ratio CH4/CO2), is utilized for predicting the response at specified levels of each factor. It is crucial to specify the levels in the original units corresponding to each factor. By the proposed model (2), Table 4 displays the experimental and predicted values of the response variable. It illustrates a close alignment between the predicted responses from the fitted model and the actual values. This is evident from the low absolute error rates and a mean absolute error percentage (MAPE) of 2.28 for the fitted model. Additionally, the normal probability plot of the estimated errors in Fig. 8A demonstrates that the straight line, symbolizing a normal distribution, closely coincides with the residuals. This alignment functions as an indicator of the accuracy of the estimated model. Assessing a model's performance model can also be achieved through a plot of predicted versus actual values. In Fig. 8B, the plot of predicted versus actual values for the response variable shows a significant alignment with the line X = Y, and the slope, approaching one, indicates a substantial agreement between the model predictions and experimental data.
Table 4 Experimental and predicted values of the response variable for different values of the factor's variables
Run |
Factors |
H2 yield (%) |
T
|
SV |
CH4/CO2 |
Exp. |
Pred. |
Error (%) |
1 |
800 |
42 000 |
1 |
68.98 |
69.91 |
1.35 |
2 |
700 |
22 000 |
1 |
58.5 |
58.93 |
0.74 |
3 |
750 |
42 000 |
0.75 |
65.67 |
65.05 |
0.94 |
4 |
700 |
32 000 |
0.75 |
56.14 |
59.56 |
6.09 |
5 |
700 |
42 000 |
1 |
45.63 |
43.69 |
4.25 |
6 |
800 |
42 000 |
0.5 |
88.14 |
86.41 |
1.96 |
7 |
800 |
32 000 |
0.75 |
87.783 |
85.78 |
2.28 |
8 |
750 |
32 000 |
0.5 |
82.15 |
80.92 |
1.50 |
9 |
700 |
42 000 |
0.5 |
57.55 |
60.19 |
4.59 |
10 |
800 |
22 000 |
1 |
85.4 |
85.15 |
0.29 |
11 |
750 |
32 000 |
0.75 |
72.94 |
72.67 |
0.37 |
12 |
750 |
32 000 |
0.75 |
73.31 |
72.67 |
0.87 |
13 |
750 |
32 000 |
0.75 |
72.64 |
72.67 |
0.04 |
14 |
700 |
22 000 |
0.5 |
78.33 |
75.43 |
3.70 |
15 |
800 |
22 000 |
0.5 |
96.94 |
99.65 |
4.86 |
16 |
750 |
32 000 |
1 |
62.11 |
64.42 |
3.72 |
17 |
750 |
22 000 |
0.75 |
83.01 |
80.29 |
3.28 |
18 |
750 |
32 000 |
0.75 |
72.81 |
72.67 |
0.19 |
Mean |
2.28 |
|
|
|
|
|
 |
| Fig. 8 (A) Normal probability plots of errors in the prediction process for the model of % H2 yield. (B) The actual and predicted values for H2 yield. | |
3.6 Simulation on the design expert program
3.6.1 One factor effect (2D) plot.
The impact of individual process parameters on the reaction responses is depicted in Fig. 9, which illustrates that elevating the temperature, reducing the GHSV value, and decreasing the CH4
:
CO2 ratio result in an increased H2 yield.
 |
| Fig. 9 The relationship between the reaction parameters and H2 yield %. | |
3.6.2 Two factor effect (3D plot).
With the aid of eqn (10), the design expert program constructs the response surface plots for the predicted formation of the various components comprising the reaction system versus two process variables while keeping the third at a constant level or value, as shown below. Fig. 10 and 11 show the three-dimensional response surface plot, which represents the significant effects of the factors (temperature, GHSV, and CH4
:
CO2) on the variation of the response variable (H2 yield). Fig. 10 shows the surface plots, which represent the functional relationship between a designated response variable (H2 yield) and the two-factor variables (temperature and gas hourly space velocity) with a CH4
:
CO2 feed ratio fixed at 0.675. It represents how these factors significantly affect the variation of H2 yield. It is shown that when the temperature increases and the GHSV decreases, the H2 yield increases. Fig. 11 shows the surface plots that represent the functional relationship between a designated response variable (H2 yield) and the two-factor variables (temperature and CH4
:
CO2) with GHSV fixed at 23
800 cm3 per g-cat per h. The response surface shows that with increasing the temperature and decreasing the CH4
:
CO2, the H2 yield increases. It is observed to increase from 45.63% at 700 °C to 96.94% at 800 °C. The temperature significantly influences H2 yield in the dry reforming of methane due to the endothermic nature of the reaction. Sensitivity to reaction temperature suggests the importance of optimizing conditions for methane dry reforming, thereby enhancing process efficiency.
 |
| Fig. 10 The relationship between the temperature T, GHSV, and H2 yield % with a CH4: CO2 feed ratio fixed at 0.675. | |
 |
| Fig. 11 The relationship between temperature T, CH4: CO2, and H2 yield % with GHSV fixed at 23 800 cm3 per g-cat per h. | |
4. Conclusion
Adequate Si–O–Al linkage is present in metalized silica-alumina with 1 wt% noble metal, which also contains 5 wt% Ni of active metal for DRM. The initial concentration of active sites is responsible for the initial activity of the catalyst, but under oxidizing and reducing gas feed (CO2 and H2) during DRM, the active sites are reorganized, and active sites derived from NiAl2O4 are determined for activity. The 1 wt% Ir metalized 5Ni/1IrSiAl catalyst has a minimum concentration of such active sites and a minimum population of basicity, which implies low (YH2 = 33%). 5Ni/1RuSiAl, 5Ni/1PdSiAl and 5Ni/1PtSiAl catalysts have a similar extent of interaction with CO2 and similar initial catalytic activity. High interaction of Pd with CO over 5Ni/1PdSiAl may start the CO disproportionation reaction and massive coke formation (∼41% weight loss) and inferior H2 yield (28%). The 1 wt% Pt metalized 5Ni/1PtSiAl catalyst has adequate interaction between Pt and CO. However, this catalyst initially has a higher value of reducibility and the highest concentration of active sites driven from NiAl2O4 during the reaction. Over the 5Ni/1PtSiAl catalyst, carbon resistance and H2 yield (36%) are improved during 300 minutes of TOS. Further, the 5Ni/1RuSiAl catalyst further has less carbon deposition and a higher H2 yield (41%). The highest concentration of moderate strength basic sites, highest edge of reducibility, and the highest concentration of Ni derived from the NiAl2O4 phase (during the DRM reaction) over the 5Ni/1RhSiAl catalyst result in minimum coke deposition as well as maximum catalytic activity (62% hydrogen yield at 700 °C reaction temperature and 80% hydrogen yield at 800 °C reaction temperature). Utilizing the multiple response surface methodology (RSM) and central composite design (CCD) statistical technique, a linear model was inferred to predict the response variable. Statistical tools were employed to examine the accuracy of the model. The findings revealed that the reaction temperature exerted the most pronounced influence on enhancing the H2 yield. Notably, the H2 yield increased from 45.63% at 700 °C to 96.94% at 800 °C. Optimization of reaction conditions is performed over the 5Ni/1RhSiAl catalyst by RSM through central composite design (CCD). The model predicted an optimal H2 yield of 97.6% under specific conditions over 5Ni/1RhSiAl: 798 °C temperature, 0.5 CH4/CO2 ratio, and 26
521 cm3 per g-cat per h gas hourly space velocity. However, experimental validation under these conditions yielded an H2 yield of 95.4%. The experimental finding is close to the prediction results, indicating the theoretical models' validity. These advancements could improve the efficiency of hydrogen production and promote the creation of eco-friendly technologies, which are vital for the future health of our environment and energy resources. The prediction and validation results for several studies are stated in Table S2.†
Data availability
The datasets generated and/or analyzed during the current study are available from the corresponding author on reasonable request. Specific data include:
1. Raw data: the raw experimental data from the sustainable energy and fuels study, including initial measurements and observations, are stored in a secure institutional repository and can be accessed upon request to the corresponding author.
2. Processed data: processed data that support the findings of this study are available in the ESI† of this article. This includes detailed calculations, derived data points, and processed datasets.
3. Supporting Information: Additional supporting information and detailed methodologies are included within the ESI† files attached to this article.
Requests for data and materials should be addressed to Ahmed S. Al-Fatesh at E-mail: aalfaesh@ksu.edu.sa.
Conflicts of interest
The authors declare no conflict of interest.
Acknowledgements
The authors would like to extend their sincere appreciation to the Researchers Supporting Project number (RSP2024R511), King Saud University, Riyadh, Saudi Arabia.
References
- J. Sasson Bitters, T. He, E. Nestler, S. D. Senanayake, J. G. Chen and C. Zhang, J. Energy Chem., 2022, 68, 124–142 CrossRef CAS.
- W. Y. Wang and G. C. Wang, Catal. Sci. Technol., 2021, 11, 1395–1406 RSC.
- C. Pan, Z. Guo, H. Dai, R. Ren and W. Chu, Int. J. Hydrogen Energy, 2020, 45, 16133–16143 CrossRef CAS.
- M. Hasani Estalkhi, M. Yousefpour, H. Koohestan and Z. Taherian, Int. J. Hydrogen Energy, 2024, 68, 1344–1351 CrossRef CAS.
- S. Katheria, G. Deo and D. Kunzru, Appl. Catal., A, 2019, 570, 308–318 CrossRef CAS.
- Z. Liu, F. Gao, Y. A. Zhu, Z. Liu, K. Zhu and X. Zhou, Chem. Commun., 2020, 56, 13536–13539 RSC.
- D. Pashchenko and I. Makarov, Energy, 2021, 222, 119993 CrossRef CAS.
- S. Roy, S. Hariharan and A. K. Tiwari, J. Phys. Chem. C, 2018, 122, 10857–10870 CrossRef CAS.
- H. Zhou, T. Zhang, Z. Sui, Y. A. Zhu, C. Han, K. Zhu and X. Zhou, Appl. Catal., B, 2018, 233, 143–159 CrossRef CAS.
- T. Mozammel, D. Dumbre, R. Hubesch, G. D. Yadav, P. R. Selvakannan and S. K. Bhargava, Energy Fuels, 2020, 34, 16433–16444 CrossRef CAS.
- P. N. Romano, J. F. S. de Carvalho Filho, J. M. A. R. de Almeida and E. F. Sousa-Aguiar, Catal. Today, 2022, 394–396, 348–356 CrossRef CAS.
- D. Zubenko, S. Singh and B. A. Rosen, Appl. Catal., B, 2017, 209, 711–719 CrossRef CAS.
- M. A. Vasiliades, C. M. Damaskinos, K. K. Kyprianou, M. Kollia and A. M. Efstathiou, Catal. Today, 2020, 355, 788–803 CrossRef CAS.
- J. D. Jiménez, L. E. Betancourt, M. Danielis, H. Zhang, F. Zhang, I. Orozco, W. Xu, J. Llorca, P. Liu, A. Trovarelli, J. A. Rodríguez, S. Colussi and S. D. Senanayake, ACS Catal., 2022, 12, 12809–12822 CrossRef PubMed.
- P. Zambaldi, L. Haug, S. Penner and B. Klötzer, Catalysts, 2022, 12(3), 311 CrossRef CAS.
- B. Steinhauer, M. R. Kasireddy, J. Radnik and A. Martin, Appl. Catal., A, 2009, 366, 333–341 CrossRef CAS.
- P. Riani, E. Spennati, M. V. Garcia, V. S. Escribano, G. Busca and G. Garbarino, Int. J. Hydrogen Energy, 2023, 48, 24976–24995 CrossRef CAS.
- A. K. Dharmadhikari, F. A. Rajgara and D. Mathur, Opt. Lett., 2006, 31, 2184–2186 CrossRef CAS PubMed.
- M. Usman, W. M. A. Wan Daud and H. F. Abbas, Renew. Sustainable Energy Rev., 2015, 45, 710–744 CrossRef CAS.
- M. Houalla, F. Delannay and B. Delmon, J. Chem. Soc. Faraday Trans. 1 Phys. Chem. Condens. Phases, 1980, 76, 1766–1772 CAS.
- A. S. Al-Fatesh, R. Kumar, S. O. Kasim, A. A. Ibrahim, A. H. Fakeeha, A. E. Abasaeed, R. Alrasheed, A. Bagabas, M. L. Chaudhary, F. Frusteri and B. Chowdhury, Catal. Today, 2020, 348, 236–242 CrossRef CAS.
- N. Patel, A. H. Fakeeha, S. B. Alreshaidan, M. F. Alotibi, A. I. Osman, A. H. Al-Muhtaseb, M. A. Mahyoub, R. Kumar, A. E. Abasaeed and A. S. Al-Fatesh, Catal. Lett., 2024, 154, 2475–2487 CrossRef CAS.
- A. L. Kustov, T. R. Aymaletdinov, A. A. Shesterkina, K. B. Kalmykov, P. V Pribytkov, I. V Mishin, S. F. Dunaev and L. M. Kustov, Mendeleev Commun., 2024, 34, 221–223 CrossRef CAS.
- A. S. Al-Fatesh, M. M. Alrashed, R. A. El-Salamony, M. H. Roushdy, S. M. Alwan, A. I. Osman, M. Bayazed, A. H. Fakeeha, A. A. Ibrahim and R. Kumar, J. CO2 Util., 2023, 75, 102578 CrossRef CAS.
- M. A. Hossain, B. V. Ayodele, C. K. Cheng and M. R. Khan, J. Energy Inst., 2019, 92, 177–194 CrossRef CAS.
- C. C. Chong, Y. W. Cheng, H. D. Setiabudi, N. Ainirazali, D. V. N. Vo and B. Abdullah, Int. J. Hydrogen Energy, 2020, 45, 8507–8525 CrossRef CAS.
- I. Izhab, M. Asmadi and N. A. Saidina Amin, Int. J. Hydrogen Energy, 2021, 46, 24754–24767 CrossRef CAS.
- A. Motomura, Y. Nakaya, C. Sampson, T. Higo, M. Torimoto, H. Tsuneki, S. Furukawa and Y. Sekine, RSC Adv., 2022, 12, 28359–28363 RSC.
- H. U. Hambali, A. A. Jalil, A. A. Abdulrasheed, T. J. Siang, A. H. K. Owgi and F. F. A. Aziz, Chem. Eng. Sci., 2021, 231, 116320 CrossRef CAS.
- A. G. Georgiadis, N. D. Charisiou, V. Sebastian, G. I. Siakavelas, S. J. Hinder, A. I. Tsiotsias, M. A. Goula, W. Wang, S. Mascotto and B. Ehrhardt, An experimental and theoretical approach for the biogas dry reforming reaction using perovskite-derived La0. 8X0. 2NiO3-d catalysts (X= Sm, Pr, Ce), Renewable Energy, 2024, 227, 120511 CrossRef CAS.
- S. Zeng, Y. Zhang, J. Li, Z. Liu, S. Shen, Z. Ou, P. Song, R. Yuan, D. Dong, H. Xie, M. Ni, Z. Shao and B. Chen, Int. J. Hydrogen Energy, 2024, 50, 1220–1231 CrossRef.
- T. S. Gendy, R. A. El-Salamony, M. M. Alrashed, A. Bentalib, A. I. Osman, R. Kumar, A. H. Fakeeha and A. S. Al-Fatesh, Mol. Catal., 2024, 562, 114216 CrossRef CAS.
- Y. Cai, D. Mei, Y. Chen, A. Bogaerts and X. Tu, J. Energy Chem., 2024, 96, 153–163 CrossRef CAS.
- A. S. Al-Fatesh, N. A. Bamatraf, S. B. Alreshaidan, J. K. Abu-Dahrieh, N. patel, A. A. Ibrahim, A. H. Fakeeha, A. bin Jumah and R. Kumar, Arab. J. Sci. Eng., 2024, 49, 8031–8047 CrossRef CAS.
- N. Patel, A. S. Al-Fatesh, N. A. Bamatraf, A. I. Osman, S. B. Alreshaidan, A. H. Fakeeha, I. Wazeer and R. Kumar, Catal. Lett., 2024, 154, 3441–3456 CrossRef CAS.
- J. Khatri, A. S. Al-Fatesh, A. H. Fakeeha, A. A. Ibrahim, A. E. Abasaeed, S. O. Kasim, A. I. Osman, R. Patel and R. Kumar, Mol. Catal., 2021, 504, 111498 CrossRef CAS.
- F. G. M. de Medeiros, F. W. B. Lopes and B. R. de Vasconcelos, Catalysts, 2022, 12, 363 CrossRef CAS.
- A. N. Kurdi, A. A. Ibrahim, A. S. Al-Fatesh, A. A. Alquraini, A. E. Abasaeed and A. H. Fakeeha, RSC Adv., 2022, 12, 10846–10854 RSC.
- F. Huang, R. Wang, C. Yang, H. Driss, W. Chu and H. Zhang, J. Energy Chem., 2016, 25, 709–719 CrossRef.
- A. S. Al-Fatesh, J. Khatri, R. Kumar, V. Kumar Srivastava, A. I. Osman, T. S. AlGarni, A. A. Ibrahim, A. E. Abasaeed, A. H. Fakeeha and D. W. Rooney, Energy Sci. Eng., 2022, 10, 866–880 CrossRef CAS.
- R. Patel, A. S. Al-Fatesh, A. H. Fakeeha, Y. Arafat, S. O. Kasim, A. A. Ibrahim, S. A. Al-Zahrani, A. E. Abasaeed, V. K. Srivastava and R. Kumar, Int. J. Hydrogen Energy, 2021, 46, 25015–25028 CrossRef CAS.
- R. Patel, A. H. Fakeeha, S. O. Kasim, M. L. Sofiu, A. A. Ibrahim, A. E. Abasaeed, R. Kumar and A. S. Al-Fatesh, Mol. Catal., 2021, 510, 111676 CrossRef CAS.
- A. H. Fakeeha, R. Patel, N. El Hassan, S. A. Al-Zahrani, A. S. Al-Awadi, L. Frusteri, H. Bayahia, A. I. Alharth, A. S. Al-Fatesh and R. Kumar, Int. J. Hydrogen Energy, 2022, 47, 38242–38257 CrossRef CAS.
- A. S. Al-Fatesh, R. Patel, V. K. Srivastava, A. A. Ibrahim, M. A. Naeem, A. H. Fakeeha, A. E. Abasaeed, A. A. Alquraini and R. Kumar, ACS Omega, 2022, 7, 16468–16483 CrossRef CAS PubMed.
- A. E. Abasaeed, M. L. Sofiu, K. Acharya, A. I. Osman, A. H. Fakeeha, R. L. AL-Otaibi, A. A. Ibrahim, A. S. Al-Awadi, H. Bayahia, S. A. Al-Zahrani, R. Kumar and A. S. Al-Fatesh, Energy Sci. Eng., 2023, 11, 1436–1450 CrossRef CAS.
- A. S. Al-Fatesh, M. L. Chaudhary, A. H. Fakeeha, A. A. Ibrahim, F. Al-Mubaddel, S. O. Kasim, Y. A. Albaqmaa, A. A. Bagabas, R. Patel and R. Kumar, Processes, 2021, 9, 1–15 CrossRef.
- K. J. Chaudhary, A. S. Al-Fatesh, A. A. Ibrahim, A. I. Osman, A. H. Fakeeha, M. Alhoshan, N. Alarifi, A. H. Al-Muhtaseb and R. Kumar, Energy Convers. Manag. X, 2024, 23, 100631 CAS.
- S. Bhattar, A. Abedin, D. Shekhawat, J. Daniel and J. J. Spivey, Appl. Catal., A, 2020, 602, 117721 CrossRef CAS.
- A. Osaka, T. Takatsuna and Y. Miura, J. Non. Cryst. Solids, 1994, 178, 313–319 CrossRef CAS.
- A. V. Ghule, K. Ghule, S. H. Tzing, T. H. Punde, H. Chang and Y. C. Ling, J. Solid State Chem., 2009, 182, 3406–3411 CrossRef CAS.
- Y. T. Chan, C. H. Wu, P. Shen and S. Y. Chen, Appl. Phys. A Mater. Sci. Process., 2014, 116, 1065–1073 CrossRef CAS.
- P. McMillan and B. Piriou, J. Non. Cryst. Solids, 1982, 53, 279–298 CrossRef CAS.
- A. V. Ghule, K. Ghule, T. Punde, J. Y. Liu, S. H. Tzing, J. Y. Chang, H. Chang and Y. C. Ling, Mater. Chem. Phys., 2010, 119, 86–92 CrossRef CAS.
- N. García-Gómez, J. Valecillos, A. Remiro, B. Valle, J. Bilbao and A. G. Gayubo, Appl. Catal. B Environ., 2021, 297, 120445 CrossRef.
- R. T. K. Baker, Carbon N. Y., 1989, 27, 315–323 CrossRef CAS.
- S. L. Leung, J. Wei, W. L. Holstein, M. Avalos-Borja and E. Iglesia, J. Phys. Chem. C, 2020, 124, 20143–20160 CrossRef CAS.
- S. Helveg, C. López-Cartes, J. Sehested, P. L. Hansen, B. S. Clausen, J. R. Rostrup-Nielsen, F. Abild-Pedersen and J. K. Nørskov, Nature, 2004, 427, 426–429 CrossRef CAS PubMed.
- R. J. B. Motta, C. B. Dornelas and R. M. de Almeida, J. Braz. Chem. Soc., 2023, 34, 734–744 CAS.
- X. Hu, Y. Hu, Q. Xu, X. Wang, G. Li, H. Cheng, X. Zou and X. Lu, Int. J. Hydrogen Energy, 2020, 45, 4244–4253 CrossRef CAS.
- A. E. Abasaeed, M. S. Lanre, S. O. Kasim, A. A. Ibrahim, A. I. Osman, A. H. Fakeeha, A. Alkhalifa, R. Arasheed, F. Albaqi, N. S. Kumar, W. U. Khan, R. Kumar, F. Frusteri, A. S. Al-Fatesh and A. A. Bagabas, Int. J. Hydrogen Energy, 2023, 48, 26492–26505 CrossRef CAS.
- R. Burch, P. K. Loader and N. A. Cruise, Appl. Catal., A, 1996, 147, 375–394 CrossRef CAS.
- R. Fiorenza, L. Spitaleri, A. Gulino and S. Scirè, Catalysts, 2018, 8, 1–18 CrossRef.
- J. N. Park, J. Kuk Shon, M. Jin, S. Sung Kong, K. Moon, G. Ok Park, J. H. Boo and J. Man Kim, React. Kinet. Mech. Catal., 2011, 103, 87–99 CrossRef CAS.
- X. Hong, B. Li, Y. Wang, J. Lu, G. Hu and M. Luo, Appl. Surf. Sci., 2013, 270, 388–394 CrossRef CAS.
- S. A. Yashnik, Y. A. Chesalov, A. V. Ishchenko, V. V. Kaichev and Z. R. Ismagilov, Appl. Catal., B, 2017, 204, 89–106 CrossRef CAS.
- F. Huang, J. Chen, W. Hu, G. Li, Y. Wu, S. Yuan, L. Zhong and Y. Chen, Appl. Catal., B, 2017, 219, 73–81 CrossRef CAS.
- L. F. Chen, J. A. Wang, M. A. Valenzuela, X. Bokhimi, D. R. Acosta and O. Novaro, J. Alloys Compd., 2006, 417, 220–223 CrossRef CAS.
- S. Saeed, T. M. Aboul-Fotouh and I. Ashour, Pet. Coal, 2016, 58, 611–621 Search PubMed.
- V. De Coster, N. V. Srinath, P. Yazdani, H. Poelman and V. V Galvita, J. Phys. Chem. Lett., 2022, 13, 7947–7952 CrossRef CAS PubMed.
- B. R. Strohmeier, Surf. Sci. Spectra, 1994, 3, 135–140 CrossRef CAS.
- N. Patel, A. H. Fakeeha, S. B. Alreshaidan, M. F. Alotibi, A. I. Osman, A. H. Al-Muhtaseb, M. A. Mahyoub, R. Kumar, A. E. Abasaeed and A. S. Al-Fatesh, Catal. Lett., 2024, 154, 2475–2487 CrossRef CAS.
- M. L. Miller and R. W. Linton, Anal. Chem., 1985, 57, 2314–2319 CrossRef CAS.
- P. R. Anderson and W. E. Swartz, Inorg. Chem., 1974, 13, 2293–2294 CrossRef CAS.
- C. D. Wagner, D. E. Passoja, H. F. Hillery, T. G. Kinisky, H. A. Six, W. T. Jansen and J. A. Taylor, J. Vac. Sci. Technol., 1982, 21, 933–944 CrossRef CAS.
- T. L. Barr, Appl. Surf. Sci., 1983, 15, 1–35 CrossRef CAS.
- M. C. Biesinger, B. P. Payne, L. W. M. Lau, A. Gerson and R. S. C. Smart, Surf. Interface Anal., 2009, 41, 324–332 CrossRef CAS.
- I. Lucentini, A. Casanovas and J. Llorca, Int. J. Hydrogen Energy, 2019, 44, 12693–12707 CrossRef CAS.
- T. Yang, Y. Huo, Y. Liu, Z. Rui and H. Ji, Appl. Catal., B, 2017, 200, 543–551 CrossRef CAS.
- T. Arikan, A. M. Kannan and F. Kadirgan, Int. J. Hydrogen Energy, 2013, 38, 2900–2907 CrossRef CAS.
- K. Machida and M. Enyo, J. Electrochem. Soc., 1987, 134, 1472–1474 CrossRef CAS.
- E. C. Moura and R. M. Claro, Int. J. Public Health, 2012, 57, 127–133 CrossRef PubMed.
- A. I. Osman, A. Ayati, P. Krivoshapkin, B. Tanhaei, M. Farghali, P.-S. Yap and A. Abdelhaleem, Coordination-driven innovations in low-energy catalytic processes: Advancing sustainability in chemical production, Coord. Chem. Rev., 2024, 514, 215900 CrossRef CAS.
Footnote |
† Electronic supplementary information (ESI) available: S1. Catalyst Characterization, S2. Catalyst Performance Evaluation, S3. The basic terms of variance and prediction statistic, S4. Methodology, Fig. S1. Experimental setup for conducting methane reforming, Table S1. The results of background conversions, and Table S2. The prediction and validation results. See DOI: https://doi.org/10.1039/d4se00867g |
|
This journal is © The Royal Society of Chemistry 2024 |