DOI:
10.1039/D4AY00651H
(Critical Review)
Anal. Methods, 2024,
16, 4664-4682
Mass spectrometry-intensive top-down proteomics: an update on technology advancements and biomedical applications
Received
9th April 2024
, Accepted 25th June 2024
First published on 27th June 2024
Abstract
Proteoforms are all forms of protein molecules from the same gene because of variations at the DNA, RNA, and protein levels, e.g., alternative splicing and post-translational modifications (PTMs). Delineation of proteins in a proteoform-specific manner is crucial for understanding their biological functions. Mass spectrometry (MS)-intensive top-down proteomics (TDP) is promising for comprehensively characterizing intact proteoforms in complex biological systems. It has achieved substantial progress in technological development, including sample preparation, proteoform separations, MS instrumentation, and bioinformatics tools. In a single TDP study, thousands of proteoforms can be identified and quantified from a cell lysate. It has also been applied to various biomedical research to better our understanding of protein function in regulating cellular processes and to discover novel proteoform biomarkers of diseases for early diagnosis and therapeutic development. This review covers the most recent technological development and biomedical applications of MS-intensive TDP.
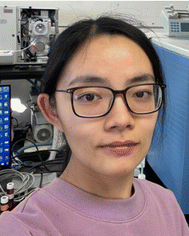 Tian Xu | Dr Tian Xu obtained her BS degree at Minzu University of China and completed MS degree at Chinese Academy of Sciences. In 2018, she joined Dr Liangliang Sun's lab for developing automated capillary isoelectric focusing-mass spectrometry techniques for top-down proteomics. She earned her PhD in Chemistry in 2023 and is currently doing top-down proteomics research as a postdoctoral fellow at Dr Neil L. Kelleher's research group at Northwestern University. |
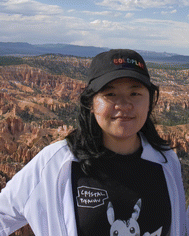 Qianjie Wang | Dr Qianjie Wang obtained her Bachelor's degree in Chemistry from Nanjing University. She joined the PhD programs at Michigan State University Chemistry and Biochemistry in 2018 co-mentored by Dr Liangliang Sun in Chemistry and Dr Peter Lundquist in BMB. Her PhD research focused on combining top-down and bottom-up proteomics to study the plastoglobules (lipoprotein particles in chloroplast) proteome and remobilization under different stress. She earned her PhDs in Chemistry and BMB in 2024. She will start her career at AstraZeneca in July 2024. |
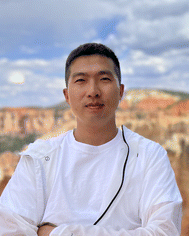 Qianyi Wang | Qianyi Wang obtained his BS and Master degrees from Beijing Normal University and joined the MSU Chemistry PhD program in Fall 2019. His research has been focused on the development of novel capillary electrophoresis-mass spectrometry (CE-MS) techniques for top-down proteomics (TDP) to improve the characterization of proteoforms carrying post-translational modifications (PTMs) and to discover novel proteoform biomarkers of cancer. |
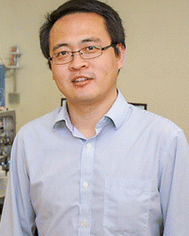 Liangliang Sun | Dr Sun is an Associate Professor of Chemistry at Michigan State University. He received his PhD degree in Analytical Chemistry in 2011 from Dalian Institute of Chemical Physics, Chinese Academy of Sciences, advised by Profs. Yukui Zhang and Lihua Zhang. Before joining MSU, he worked with Prof. Norman Dovichi at University of Notre Dame as a postdoctoral fellow and later a Research Assistant Professor (2011–2016). His research lab focuses on developing novel capillary electrophoresis-mass spectrometry methodologies for multi-level proteomics to characterize proteins at the peptide, proteoform, and protein complex levels with high throughput, high sensitivity, and single-cell resolution. |
1. Introduction
Proteins are direct functional participants in cells that link genotype and phenotype. Diverse forms of proteins (also termed “proteoforms”) can be derived from a single protein-coding gene due to gene mutations, RNA alternative splicing, and protein post-translational modifications (PTMs), resulting in various functional outcomes.1–7 Proteomics studies that interrogate sequence and PTM features of proteoforms are crucial for understanding their roles in complex biological and disease events. Compared to conventional bottom-up proteomics (BUP), which acquires regional information about proteoforms by mapping peptides, TDP deciphers the combinatorial PTMs and amino acid variations in individual proteoforms, enabling a comprehensive insight into the proteoform landscape of biological subjects. A universal MS-intensive TDP workflow includes protein extraction from biological samples, separation of proteoforms by liquid chromatography (LC) or capillary electrophoresis (CE), proteoform mass measurement and fragmentation by state-of-the-art mass spectrometers, and interpretation of proteomics data by advanced bioinformatics tools, Fig. 1.8
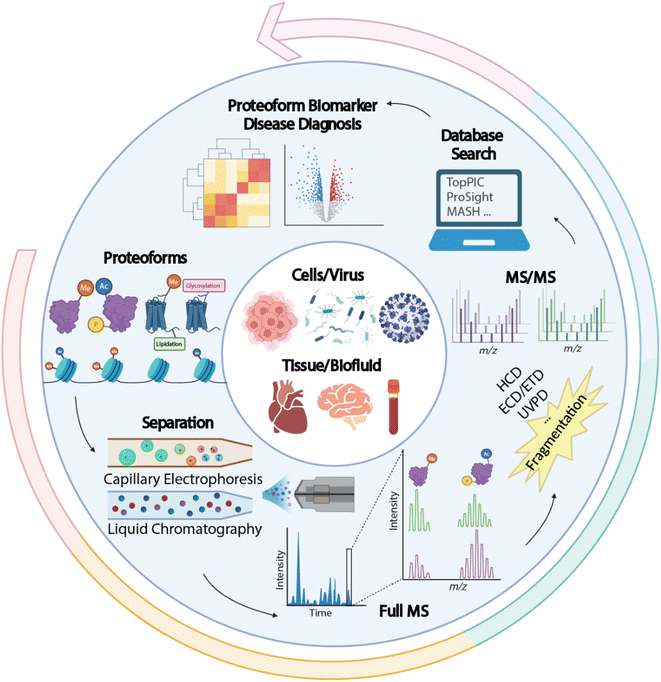 |
| Fig. 1 Schematic of a typical top-down proteomics (TDP) workflow, including proteoform extraction from various biological samples, separation by liquid chromatography (LC) or capillary electrophoresis (CE), mass spectrometry (MS) and tandem MS (MS/MS) via various gas-phase fragmentation techniques, and database search using bioinformatics tools for proteoform identification and quantification to discover new proteoform biomarkers of diseases. The figure is created using the BioRender and is used here with permission. | |
The complexity of proteoform identification arises from the need to identify and localize PTMs, determine amino acid sequences, and account for unknown or ambiguous genes.9 Based on the five-level classification system for proteoform identifications, around 40–60% of level 1 proteoforms can be identified without ambiguity.10–12 However, MS-intensive TDP is still considered challenging in multiple aspects. First, current large-scale TDP studies mainly focused on proteoforms smaller than 30 kDa. Complete characterization of large proteoforms is difficult due to coelution in separation and low sensitivity in MS detection. Second, MS-intensive TDP of membrane proteins is challenging due to their low solubility in MS-compatible buffers. Third, delineating heavily modified proteoforms (e.g., histones) requires much more effort to improve separations, fragmentations, and bioinformatics tools for accurate PTM determination and localization. Fourth, achieving the identification (ID) of proteoforms of thousands of protein-coding genes from human cells in a single study is challenging for MS-intensive TDP due to the extremely high sample complexity. It has been estimated that more than one million proteoforms are present in the human body.13 Early large-scale MS-based TDP studies have generally achieved hundreds to thousands of proteoform IDs from several hundred genes in human cells. Lastly, more work is needed to improve the bioinformatics tools for proteoform ID and quantification to advance TDP in the abovementioned areas.14–17
During the last several years, MS-intensive TDP has made substantial progress in addressing these challenges. This process has been driven by drastic technological improvements in sample preparation, separation, MS instrumentation, fragmentation strategies, and bioinformatics tools. Several reviews have summarized the related topics in earlier years.8,18–20 In this review, we updated the most recent technological advancements in MS-intensive TDP, with a particular focus on large proteoforms, membrane proteins, heavily modified proteoforms, global proteoform profiling, high-throughput quantification, and bioinformatics tools. In addition, the review introduced the latest biomedical applications of MS-intensive TDP.
2. Technological development
2.1 Characterization of large proteoforms
Large proteoform ID from complex cell lysates has been dramatically restricted in global MS-intensive TDP studies. To date, most large-scale TDP efforts focused on proteoforms smaller than 30 kDa due to multiple technical challenges. For complex cell lysates, proteoform coelution in separation causes ionization suppression of large and low-abundance proteoforms.21,22 Also, the signal-to-noise (S/N) of proteoforms from MS detection decreases exponentially with increasing proteoform mass, due to a much broader charge state and isotopic distributions.23,24 In terms of MS instrumentation, inadequate transmission and mass resolution impede large proteoform IDs. Finally, limited backbone cleavage coverage of large proteoforms using conventional collision-based fragmentation methods constantly causes problems in precise sequence ID and PTM localization.25,26
Sample fractionation has been extensively applied in recent years to reduce sample complexity, which significantly benefits the downstream characterization of large proteoforms with top-down MS. Size exclusion chromatography (SEC) has been considered as a practical approach to fractionate proteoforms by size, enabling better characterization of large proteoforms. Coupling serial size exclusion chromatography (sSEC) fractionation and reversed-phase liquid chromatography (RPLC)-MS allowed a 15-fold increase in the number of detected proteoforms above 60 kDa.25 Further application of sSEC-RPLC-MS and MS/MS with Proteoform Suite software17 achieved the ID of large proteoforms up to 140 kDa from human heart samples.26 The 140 kDa protein (endogenous human cardiac myosin binding protein C) was identified by top-down MS for the first time. Most recently, the ID of intact large proteoforms (myosin heavy chain, ∼223 kDa) from single cells (single muscle fibers, SMFs) was reported via advanced RPLC-MS-based TDP.27 The Zhang group developed an SEC-RPLC-MS platform utilizing a novel monolithic RP capillary column for TDP of an E. coli lysate, identifying 347 proteoforms larger than 30 kDa in a single RPLC run.28 While advanced SEC and RPLC techniques have been widely utilized to identify large proteoforms, the global characterization of large proteoforms from cell lysates remains challenging. One of the primary reasons is the limited separation capacity of SEC and RPLC for large proteoforms.
Besides LC, electrophoretic methods are promising for large proteoform separations. Capillary zone electrophoresis (CZE) separates analytes under an electric field according to their charge-to-size ratios. As CZE performs separation in an open tubular fused silica capillary containing no stationary phase, it can achieve higher separation efficiency and less sample loss for large proteoforms than many conventional LC techniques.29–33 Additionally, CZE-MS/MS has been well recognized as a valuable technique for TDP as the nanoflow CE-MS interface (i.e., 50–300 nL min−1) provides high sensitivity for detection.32–38 The Kelleher group utilized a GELFrEE (gel-eluted liquid fraction entrapment electrophoresis)-CZE-MS/MS platform to improve the detection of large proteoforms.39 Thirty proteins in the mass range of 30–80 kDa from the Pseudomonas aeruginosa whole-cell lysate were identified. The Sun group coupled FAIMS (high field asymmetric waveform ion mobility spectrometry) to CZE-MS/MS to achieve online two-dimensional separations (IMS and CZE) for improving the ID of large proteoforms, Fig. 2.40 By altering the compensation voltages from −50 to 30 V, FAIMS enabled the efficient fractionation of proteoform ions in the gas phase, with a median proteoform mass range from less than 10 kDa to about 30 kDa. CZE-FAIMS-MS/MS boosted the number of proteoform IDs six-fold in the 20–45 kDa range. Apart from CZE, capillary isoelectric focusing (cIEF) has been well known for ultra-high resolution protein separation based on their isoelectric points (pIs).41,42 cIEF-MS has been widely used for top-down MS characterization of proteoforms.32,43 Several research groups have demonstrated that cIEF-MS is highly efficient for the characterization of charge variants of monoclonal antibodies (mAbs, ∼150 kDa).43–47 Recently, the Sun group developed a native cIEF-MS technique for the delineation of large protein complexes and the accurate determination of pIs of various forms of large protein complexes.48 More studies are still needed to improve the performance of CE-MS/MS for the global characterization of large proteoforms from cell lysates. The Takemori group developed passive eluting proteins from polyacrylamide gels as intact species for MS (“PEPPI-MS”) for MS-intensive TDP.49 The PEPPI is a gel-based electrophoresis method for high-resolution separation of proteoforms based on their masses and recovery of proteoforms from polyacrylamide gels. A 68% median recovery rate for <100 kDa proteins and a 57% median recovery rate for proteins larger than 100 kDa were reported previously. Coupling PEPPI to RPLC-MS and MS/MS identified nearly 60 proteoforms higher than 30 kDa from an E. coli cell lysate. Although PEPPI efficiently separates large proteoforms, the PEPPI workflow includes protein cleanup steps before MS analysis. More careful evaluations are needed for comprehensive proteoform ID and accurate quantification using the PEPPI-based approach.50
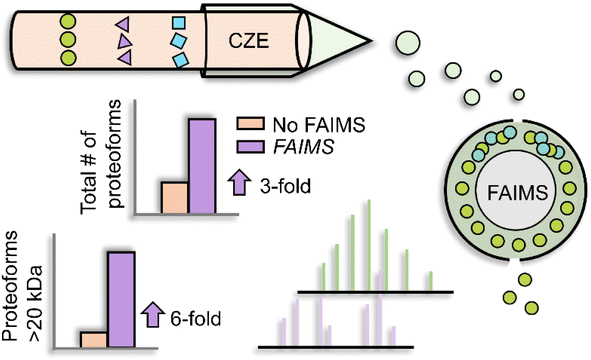 |
| Fig. 2 Schematic of capillary zone electrophoresis (CZE)-field asymmetric waveform ion mobility spectrometry (FAIMS)-mass spectrometry (MS) and MS/MS for top-down proteomics (TDP) of a complex sample. Using nine different compensation voltages, FAIMS achieved online gas phase fractionation, resulting in a nearly 3-fold increase in the number of proteoform IDs. This method particularly benefited the identification of relatively large proteoforms compared to CZE-MS/MS alone. Reproduced from ref. 40 with permission from the American Chemical Society, copyright 2023. | |
Significant efforts have also been made to improve the MS instrumentation to enable accurate mass determination of large proteoforms and to boost the sensitivity of TDP for large proteoforms. One big issue of large proteoform ID is the unresolved isotopic peaks in each charge state due to the limited mass resolution of mass spectrometers, making accurate charge determination challenging.17,23 The development of charge detection MS (CDMS) provided a useful solution for large proteoforms, enabling mass analysis of megadalton particles with the direct readout of an ion's charge.51 At the single-ion regime, the measured image current is proportional to the charge of the ion, enabling CDMS.52 Orbitrap-based individual ion MS (I2MS) determines the charge of individual ions by acquiring hundreds of mass spectra with a single ion per scan.53 It then creates high-resolution mass spectra of proteoforms and protein complexes (megadalton) for accurate mass determination. The I2MS technique substantially boosts TDP's sensitivity compared to conventional data acquisition with an ensemble of ions. I2MS detected over 500 proteoforms from a GELFrEE fraction of a human cell lysate via direct sample infusion, and the conventional data acquisition failed to detect any clear proteoform signal. Further coupling I2MS with ambient nanospray desorption electrospray ionization (nanoDESI) enabled proteoform-level imaging of tissue samples with the detection and ID of hundreds of proteoforms up to 70 kDa.54 The Heck group also developed a novel single-molecule MS technique using an Orbitrap mass analyzer through frequency chasing of individual megadalton ions.55,56 The technique has reached incredibly high mass resolution (>100
000) at m/z 35
000 and enabled accurate mass determination of megadalton complexes. Coupling these single-ion MS techniques with in-front high-capacity liquid-phase separations will drastically advance the TDP of large proteoforms and protein complexes.
Another big challenge of large proteoform ID with the TDP approach is the limited backbone cleavage coverage from conventional gas-phase fragmentation techniques (i.e., collision-induced dissociation, CID, and higher energy collision dissociation, HCD), impeding the accurate localization of PTMs and sequence variations. Electron or photon-based fragmentation techniques (i.e., electron transfer dissociation, ETD, electron capture dissociation, ECD, and ultraviolet photodissociation, UVPD) have demonstrated their substantially better performance than HCD for large proteoform fragmentation.57–59 However, even with advanced fragmentation methods, resolving the overlapping peaks and complex spectra of large proteoforms remains challenging. The spectral complexity introduced by multiple charge states can be reduced by ion–ion reactions, such as proton transfer charge reaction (PTCR), where a multiply charged cation reacts with a singly charged reagent anion to generate cations with reduced charge.60 For intact proteoforms, using PTCR with isolated precursors (<1.5 m/z) has doubled the identification of proteoforms over 30 kDa.61 Broadband isolation of the entire charge state distribution of intact proteins enabled the detection of proteins ranging from 14 to 148 kDa.62 At the tandem mass spectra scale, PTCR limited signal overlap produced by ETD and improved the sequence coverage of proteins spanning from 29 to 56 kDa.63 The PTCR MS3via HCD further increased the sequence coverage of 56 kDa glutamate dehydrogenase to 44%. Post-activation ion mobility spectrometry also simplified the mass spectra and enabled unambiguous assignment of typically overlapping product ions.64
2.2 MS-intensive TDP of membrane proteins
Despite membrane protein-coding genes being projected to constitute around 30% of the human proteome, membrane proteins (MPs), which play critical roles in cellular function and serve as therapeutic targets, tend to be underrepresented in most global proteomic studies. The low solubility of MPs in MS-compatible buffers is the main issue of MS-based TDP of MPs. A typical top-down MS analysis of membrane proteoforms requires detergent micelles, organic solvent, ionic liquid, or membrane mimetics (e.g., nanodiscs, lipid vesicles, bicelles) to keep the solubility prior to MS.65–68 Great efforts have been made in extraction methods, separation techniques, and MS instrumentation during the last several years to advance TDP of MPs.
Advanced MP purification/extraction methods with multidimensional liquid-phase separations have been used for MP characterization by TDP. The Ge group developed a novel photo-cleavable surfactant (Azo) to enable TDP of integral membrane proteins (IMPs) by providing the solubility of IMPs and compatibility with MS using UV irradiation-based degradation.69 More work remains to be done to make Azo more broadly used in TDP studies, e.g., more systematic and critical evaluations of Azo for IMPs in terms of degradation, MS interference, and its compatibility with online LC-MS/MS or CE-MS/MS. They also used cloud point extraction to enrich the MPs and identified 188 and 124 endogenous IMPs from human embryonic kidney cells and cardiac tissues, respectively, via coupling SEC with RPLC-MS/MS.70 The Zhang group developed a novel C8-functional amine-bridged hybrid monolith capillary column for RPLC-MS/MS analysis of MPs.71 Integrating ultracentrifugation-based MP purification, GELFrEE fractionation, and RPLC-MS/MS identified 3100 membrane proteoforms up to 50 kDa from a mouse hippocampus sample, representing the largest TDP dataset of membrane proteoforms. Beyond RPLC-MS/MS, CZE-MS/MS has also been deployed for the TDP of MPs. The Sun group recently developed an efficient CZE-MS/MS method for TDP of IMPs from a mouse brain sample.72 The method employs a sample buffer containing 30% (v/v) formic acid and 60% (v/v) methanol for solubilizing IMPs and utilizes a separation buffer of 30% (v/v) acetic acid and 30% (v/v) methanol for maintaining the solubility of IMPs during the CZE separation. Coupling SEC fractionation with CZE-MS/MS identified 276 IMP proteoforms with 1–4 transmembrane domains (TMDs), demonstrating the high potential of SEC-CZE-MS/MS for TDP of IMPs. However, further improvements are required for the global characterization of large IMPs using CZE-MS/MS.
Advancements in MS instrumentation have allowed more accurate characterization of MPs and MP complexes. The Robinson group delineated rhodopsin receptor signaling cascade in a native membrane using an advanced Orbitrap mass spectrometer.73 Various gas-phase fragmentation techniques (i.e., ETD, CID, HCD, UVPD, and infrared multiphoton dissociation, IRMPD) have been validated for IMP fragmentation. ETD favors the cleavage in the soluble region of IMPs, while CID and HCD have a dramatic bias towards TMDs, due to the lack of basic residues.74 Compared with the 28% sequence coverage of the c-subunit of ATP synthase (8 kDa) by CAD, activated ion ECD (ai-ECD) achieved 72% coverage by irradiating the precursor ion using infrared prior to ECD.75 Regarding the fragmentation of MP complexes from native MS, the sequence coverage is typically relatively low (10–20%).76 UVPD fragments protein ions via the absorption of high-energy photons. The ions are directly dissociated without energy redistribution, preserving bound ligands and labile PTMs.77 UVPD enhanced the sequence coverage of aquaporin Z (AqpZ, 99 kDa) and mechanosensitive channel of large conductance (MscL, 79 kDa) to 45% and 53%, respectively, compared with 30% and 34% obtained from HCD.78 However, the enhancement of UVPD is also highly dependent on the membrane complex structure and sequence. Infrared multiphoton dissociation (IRMPD) accumulates many low-energy photons and gradually increases the vibrational energy to break the amide (C–N) bonds. It has also been used to liberate IMPs from micelles.79 Using a modified Orbitrap Eclipse Tribrid MS with a continuous wave far IR CO2 laser, the Robinson group achieved selective detergent removal and a successive cleavage of adjacent residues in TMDs with a 26% sequence coverage of AmtB.80 Moreover, the Ruotolo group improved IMP sequence coverage to 40–60% by applying IR photoactivation before HCD.81 While significant progress has been made in MS instrumentation for more comprehensive characterization of MPs, combining advanced MP extraction and separation techniques with novel MS/MS methodologies for global characterization of MPs in whole cell lysates has not been done and needs further effort.
2.3 MS-intensive TDP for global profiling of proteoforms in complex samples
Global TDP profiling of complex samples is impeded by the extremely high sample complexity. Multi-dimensional separations of proteoforms before MS and MS/MS have been utilized to boost the proteome coverage of MS-based TDP.
The Kelleher group applied cell selection by immunomagnetic enrichment and fluorescence-activated cell sorting (FACS) to selectively enrich specific cell types from human blood samples, followed by RPLC-MS/MS-based proteoform profiling.82 Nearly 30
000 nonredundant proteoforms and 1690 proteins were identified from 21 different human hematopoietic cell types and plasma. Thousands of proteoforms were identified from each cell type. The Kelleher group also combined CZE-MS/MS and RPLC-MS/MS to characterize proteoforms across five human tissues with an ID of ∼11
500 proteoforms.12 They showed that proteoforms from CZE and RPLC approaches are complementary, and CZE-MS/MS offers a much better sensitivity than RPLC-MS/MS for proteoform IDs. The Sun group coupled LC fractionation to CZE-MS/MS for TDP of colorectal cancer cells (CRC cells, SW480 and SW620) and identified 23
622 proteoforms of 2332 proteins.5 The technique improved the number of proteoform IDs by nearly fivefold compared to previous TDP datasets of human cancer cells. In addition, the Sun group coupled SEC to automated cIEF-MS/MS for TDP of zebrafish brains with the ID and quantification of thousands of proteoforms.83 The Wu group developed an online two-dimensional ultrahigh-pressure nano-LC system for high-pH and low-pH RPLC separations of proteoforms prior to MS and MS/MS.84 The online platform identified over 1000 intact proteoforms from only 5 μg of an E. coli cell lysate. Those studies document that high-capacity liquid-phase separations (LC and CE) are vital for global proteoform profiling from cells and tissues. Dramatic improvement in proteoform separation is still needed to characterize proteoforms in cells or tissues comprehensively.
With the improvement of the sample preparation method and LC-MS/MS or CE-MS/MS, achieving TDP measurement of proteoforms in mass-limited biological samples (i.e., nanoproteomics in single cell scale) is possible. Nanoproteomics refers to the proteomics analysis of small cell populations, spanning from individual cells to clusters of cells that contain less than 1 μg protein amount in total.85,86 Coupling nanoPOTS-based sample preparation87 and advanced capillary RPLC-MS/MS has enabled TDP of a small number of mammalian cells with the ID of hundreds of proteoforms from tens to hundreds of HeLa cells.88 The Ge group developed a highly sensitive TDP workflow for SMFs, Fig. 3.27 They employed a one-pot sample processing approach to reduce protein loss and a capillary RPLC-MS/MS system with micro-flow-nanospray ionization (MnESI) to boost the sensitivity of MS measurement. They observed significant phosphorylation heterogeneity of proteoforms of myosin light chain 2 (MLC-2) in different SMFs. The Ivanov group reported TDP of single mammalian cells using CZE-MS/MS with the assistance of on-capillary cell lysis.89 Dozens of proteoforms were identified by MS/MS from single HeLa cells. The Sun group integrated laser capture microdissection, one-step sample processing, and CZE-MS/MS for TDP of isolated zebrafish brain regions.11 Over 400 proteoforms were identified from one LCM brain section with a 500 μm2 area and 20 μm thickness. Recently, the Wu group developed a novel CZE-MS platform (Spray capillary) for handling pL–nL level biological samples for CZE-MS analysis.90 Although the technique has not been thoroughly investigated for TDP of small numbers of mammalian cells, it showed exciting results for single-cell analysis of metabolites. These technical advancements open the door to MS-based TDP of mass-limited biological samples. However, substantial work is still needed to improve the whole TDP workflow for reliable and quantitative measurement of proteoforms in trace samples.
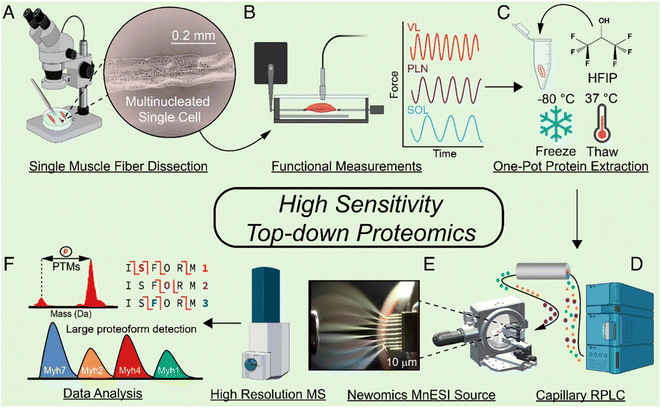 |
| Fig. 3 Schematic of the highly sensitive top-down proteomics (TDP) workflow for the characterization of proteoforms in single muscle fibers, including the mechanical dissociation of SMFs (A), velocity measurement of each muscle in a force transducer (B), protein extraction (C), capillary RPLC separation of SMF extracts (D), MnESI ionization of SMF proteins coupled with high-resolution MS analysis (E), and data analysis of the TDP data by MS/MS as well as the large proteoform detection (F). Reproduced from ref. 27 with permission from the National Academy of Sciences (NAS), copyright 2023. | |
2.4 MS-intensive TDP of highly modified proteoforms
Proteoforms can be heavily modified by various PTMs such as phosphorylation, methylation, acetylation, and glycosylation. The PTMs regulate protein functions. Delineation of proteoforms carrying various PTMs (e.g., histone) is vital for understanding their biological functions and is a challenging task for MS-based TDP.
Histones, acting as the fundamental framework within the nucleosome where genomic DNA is intricately packed, are essential for both the regulation of epigenetic gene expression and the maintenance of chromatin's structural stability91–95 The protruded N-terminal tails of the four core histones (H2A, H2B, H3, and H4) from the nucleosome structure contain a wide variety of PTMs, such as methylation, acetylation, phosphorylation, and citrullination.93,96 With the diverse PTMs and high heterogeneity of histones, fully deciphering how histone proteoforms contribute to epigenetic gene regulation remains difficult.97,98 High-resolution separation and extensive gas-phase fragmentation of histone proteoforms are crucial. The Sun group developed SEC-CZE-MS/MS (HCD) and CZE-FAIMS-MS/MS (HCD) for high-capacity separation and highly sensitive TDP measurement of histone proteoforms, leading to the ID of nearly 400 and 600 histone proteoforms from a commercial calf thymus sample.99,100 PTM localization for TDP analysis continues to pose a hindrance because the production of substantial fragment ions from highly efficient gas-phase fragmentation is vital for confidently mapping the sequence and confirming the PTM information.101 To accurately localize the PTMs on histone proteoforms, the Brodbelt group integrated UVPD with gas-phase PTCR to achieve up to 91% sequence coverage of calf thymus histone H4 with N-terminal acetylation, K12 acetylation, and R3 dimethylation.102 To separate highly isomeric histone proteoforms, the Jensen group applied trapped ion mobility spectrometry (TIMS)-time-of-flight (TOF) to resolve the isomers of monoacetylated H4 histone, providing a useful approach for the separation of highly similar histone proteoforms by their conformational differences.103 Another challenge for TDP analysis of histone proteins is associated with accurately differentiating the near-isobaric modifications, such as distinguishing acetylation and trimethylation as well as formylation and dimethylation (ΔM = 0.0364 Da). The high mass resolution MS/MS and the low mass tolerance are extremely essential to assigning correct fragment ions of histone proteoforms.104 We expect that researchers will integrate the advanced separation techniques, gas-phase fragmentation methods and high-resolution mass spectrometers together soon to characterize histone proteoforms better.
Protein glycosylation modulates a wide range of cell functions and is a highly diverse type of PTM.105,106N- and O-linked glycosylation are the two main types of glycosylation. Due to the enormous structural microheterogeneity, efficient separation and ultra-high-resolution MS measurement are necessary for the top-down MS characterization of glycoproteoforms. The spike (S) protein, essential for severe acute respiratory syndrome coronavirus 2 (SARS-CoV-2) virus entry, is highly glycosylated and contains 66 potential N-linked glycosylation sites within each trimer.107–109 Interestingly, three possible O-linked glycosylation sites have been predicted, and two of them were identified at the receptor-binding domain (RBD) of the S protein subunit S1.110,111 The abundant glycosylation on S protein enables the SARS-CoV-2 to escape innate and adaptive immune responses by protecting the epitopes from antibody neutralization.109,112 However, due to the enormous microheterogeneity of N-glycans and O-glycans, each glycosylation site could result in numerous possibilities of variable glycans with different arrangements and branched structures. Therefore, interpreting highly glycosylated S protein in a proteoform-specific manner is essential to provide new insights into virus binding, fusion, cell entry, and the development of vaccines. The Ge group explored the structural heterogeneity of different conformers of S protein RBD by native top-down TIMS-MS/MS and applied ultrahigh-resolution Fourier transform ion cyclotron resonance (FTICR)-MS/MS to deeply analyze the glycosylation sites and the corresponding glycans by denatured top-down MS.113 Overall, they disclosed eight S protein RBD O-glycosylated proteoforms, a core 2-fucosylated glycan structure, and the relative quantification information, proving that the combination of IMS and ultrahigh-resolution MS is capable of characterizing highly glycosylated S protein RBD. Another approach to investigate the glycosylation heterogeneity of S protein is using CDMS. The Jarrold group utilized top-down CDMS measurements to study the heterogeneity of glycosylation at the 66 N-glycan sites of S protein trimer, offering much more accurate insights into the glycosylation heterogeneity of S protein compared to bottom-up glycoproteomics data.114 Those works studied purified glycoproteins using the top-down MS approach. However, much effort must be made to achieve global profiling of glycoproteoforms in cells or tissues.
2.5 MS-intensive TDP for high-throughput proteoform quantification
Most quantitative TDP studies employ a label-free approach for relative proteoform quantification by comparing the proteoform intensity across different biological conditions.5,82,115,116 The label-free approach is simple and applicable to various biological samples. However, when analyzing many biological samples, it suffers from low throughput due to the extended time required for multiple sample preparations, effective online separation, high-resolution mass spectrometry, and time-consuming data processing. Different stable isotopic labeling methods have been investigated to boost the throughput of MS-based quantitative TDP.117–125 Tandem mass tags (TMT) and dimethyl isotope labeling have recently been systematically studied for quantitative TDP of complex cell lysates.121–125 The Wang group developed a dimethyl isotope labeling approach for LC-MS/MS-based quantitative TDP and quantified over 2000 proteoforms from yeast cell lysates under anaerobic and aerobic conditions.121 The isotopic labeling efficiency can be as high as 99%. The Wu group developed TMT-based approach for quantitative TDP of complex samples122–125 and quantified hundreds of proteoforms from E. coli cell lysates.122 The TMT labeling efficiency for E. coli proteoforms was better than 80%. Most recently, the Wu group developed an optimized workflow for TMT-based quantitative TDP and applied the approach to HeLa cell lysates, enabling the quantification of hundreds of proteoforms per LC-MS/MS run with the TMT labeling efficiency better than 90%.125 The results indicate that the dimethyl and TMT labeling approaches are promising for high-throughput quantitative TDP of complex samples. However, some issues still exist for those strategies. First, labeling efficiency depends on many factors, for example, the structure and size of the proteoforms. It is possible to have under-labeling and over-labeling situations, which will undoubtedly impact the proteoform ID and quantification. Second, it is challenging to label large proteoforms using, for example, TMT, due to the high organic solvent concentration in the labeling system, which leads to the precipitation of large proteoforms.122 Third, we need to balance the quality of proteoform ID and quantification for the TMT-labelled proteoforms because fragmentation of TMT labeling and proteoform backbone requires drastically different energies.125 Finally, advanced bioinformatics tools are needed to process the data for relative proteoform quantification based on the stable isotopic labeling approaches. The most widely used bioinformatics tools for MS-based TDP usually mainly support label-free-based proteoform quantification.
2.6 Bioinformatics tools for MS-intensive TDP
Advanced bioinformatics tools are extremely important for the accurate identification and quantification of proteoforms in MS-based TDP. Two main approaches for TDP analysis is either by searching against an annotated database such as ProSight, which uses Poisson matching for the reported modifications in the UniProtKB XML,15 or against a protein sequence database like TopPIC suite, which employs spectral alignment based on mass ladders and nonspecific mass shifts.14 Notable tools include but not limited to pTop – a spectral alignment software incorporating machine learning for detecting precursors more precisely,126 Informed Proteomics – a spectral alignment suite using a graph-based approach combining with LC-MS feature,127 MetaMorpheus – integrating database search of BUP and TDP,128 and MASH – an interface of multiple algorithms and visualization.16 In the last several years, some important advancements in bioinformatics tools have improved proteoform characterization in MS-based TDP studies.
The current bioinformatics tools for MS-based TDP have unsatisfied performance for ID of large proteoforms (i.e., >30 kDa) because the software typically relies on isotopically resolved peaks in each charge state to determine the accurate monoisotopic mass of proteoforms or their fragment ions for subsequent database searches, which is called deconvolution. Typical high-resolution mass spectrometers cannot offer high enough mass resolution to achieve isotopically resolved peaks for large proteoforms. Alternatively, for unresolved isotopic peaks, the average mass can be determined based on the charge state distribution, which is utilized in UniDec and TDPortal.129 Minor changes in deconvolution parameters may resolve distinct results, and the erroneous deconvolution of proteoforms leads to incorrect false discovery rate estimation at the proteoform-level.130
Recently, Jeong et al. presented an ultrafast deconvolution tool for TDP, FLASHDeconv, capable of analyzing both isotopically resolved and unresolved peaks for broad charge and mass range in MS spectra, which is especially useful for large proteoform ID.131 The same research group further developed an intelligent online data acquisition algorithm FLASHIda, enabling ultrafast proteoform deconvolution in real time during data acquisition.132 The commonly used data-dependent acquisition (DDA) method selects the top N most intense peaks for fragmentation. However, this method tends to choose multiple charge state peaks from a high abundance proteoform, leading to the difficulty in the ID of low abundance proteoforms. FLASHIda enables less redundant selection of precursor ions via real-time FLASHDeconv, boosting the proteoform coverage in MS-based TDP studies. There is another real-time deconvolution and precursor selection software for MS-based TDP, MetaDrive, which aims to combine the multiple charge states of the same proteoform to improve the quality of the MS/MS spectra and the backbone cleavage coverage of proteoforms.133 This approach could be potentially valuable for bettering the sequence coverage of large proteoforms.
Another reason of complex spectra is the internal fragment ions, which contain neither N- nor C-terminus of proteoforms and are not recognized by most software, especially when a combination of fragmentation is employed.134,135 More recently, the Loo group highlighted the value of internal fragment ions for boosting proteoform sequence coverage from various fragmentation techniques and developed a bioinformatics tool (i.e., ClipsMS) to facilitate the use of internal fragment ions in top-down MS studies.136–140 The information on internal fragment ions improves the sequence coverage of large proteoforms substantially. The Loo group combined ECD (e-MSion), HCD, and internal fragment ion assignments for top-down MS analysis of the whole NIST mAb, producing a 75% sequence coverage, the highest sequence coverage of an intact mAb by TDP so far.139 Besides the ClipsMS software by the Loo group, the Smith group implemented internal fragment ion functionality in their MetaMorpheus software for MS-based TDP of cell lysates with internal fragment ion annotation.141 The approach improved the number of proteoform IDs and the distinguishment of similar proteoforms significantly compared to the conventional terminal fragment ion approach. However, the internal fragment ion assignment requires high mass accuracy and special attention in controlling false positive hits. Additionally, to make the internal fragment ions more useful for general MS-intensive TDP studies, integration of automated annotation of internal fragment ions in the most widely used bioinformatics tools (i.e., ProSight15 and TopPIC14) will be critically important.
Although various bioinformatics tools have been developed to improve the quality of proteoform characterization, alternative methods are still needed to verify the identified proteoforms from the database search, especially those proteoforms carrying PTMs (e.g., phosphorylation and acetylation). The Sun group reported high linear correlations (R2 = 0.98) between predicted and experimental electrophoretic mobility of proteoforms identified by CZE-MS/MS-based TDP.142 The Liu group also demonstrated the solid linear correlations between the predicted and experimental migration/retention time of proteoforms identified in CZE-MS/MS and RPLC-MS/MS studies.143 More importantly, the proteoforms carrying phosphorylation and/or acetylation PTM can be confirmed by comparing their predicted and experimental electrophoretic mobility due to the charge reduction from those PTMs.142 The results indicate that integrating the predicted and experimental electrophoretic mobility or retention time information into the database search bioinformatics tools could potentially improve the confidence and accuracy of proteoform IDs. The Liu group also developed a new bioinformatics tool, PTM-TBA (PTM characterization by top-down and bottom-up MS and annotations), based on the TopPIC software to better the localization of PTMs on identified proteoforms via combining the top-down and bottom-up MS data and PTM annotations in the UniProt database.144
Besides proteoform ID, some new bioinformatics tools have been developed for label-free proteoform quantification. The Tholey group developed MSTopDiff to improve the annotation of artificial and biological modifications of the detected proteoforms.145 The Petyuk group developed a companion R package (TopPICR) for TopPIC to enable label-free quantification.146 The TopPICR tool performs many functions related to label-free quantification, including ID filter, retention time alignment across runs, mass recalibration, and proteoform feature intensity calculation with the match-between-runs approach.
One significant problem in bioinformatics for MS-based TDP is that proteoform IDs from the same dataset using different bioinformatics tools are substantially different in deconvolution results and proteoform IDs.147 This phenomenon might be due to the differences in, for example, mass deconvolution, the database for search, and the scoring algorithm for matching experimental and theoretical data for the TDP bioinformatics tools. The Ge group built MASH Explorer as a universal and user-friendly software for MS-based TDP.148 The software combines several widely used spectral deconvolution tools and database search algorithms into one platform, providing flexibility and consistency for proteoform IDs using TDP. It can also process TDP data from different vendor formats, which is particularly useful for the broad TDP community.
3. Biomedical applications of MS-intensive TDP
In recent years, MS-based TDP has been applied to various biomedical applications due to substantial technological improvement. The studies focus on mapping the proteoform landscape of disease-relevant samples or disease-specific genes for a clear understanding of the molecular mechanism of disease development, the discovery of potential biomarkers for disease diagnosis, and the innovation of precise therapeutics.
3.1 Cancer biology
Proteomics profiling of protein changes in the cancer process benefits cancer biomarker discovery and understanding of cancer-specific mechanisms and phenotypes. BUP was the dominant proteomic approach in cancer research in the past decades but is challenging in capturing the combinatorial PTMs or sequence variations in proteins.149–151 Rapid dissemination of TDP is filling this gap. The Kelleher group integrated TDP and BUP to study patient-derived breast tumor xenografts and demonstrated the significance of TDP in acquiring aberrations of cancers at the proteoform level.152 The Sun group performed a comprehensive TDP study of a pair of metastatic and nonmetastatic CRC cell lines (SW480 and SW620 cells).5 It revealed the drastic transformation of CRC cells in proteoform profiles after metastasis, Fig. 4. The study disclosed differentially expressed phosphorylated proteoforms of important cancer-related genes in well-known CRC pathways between the SW480 and SW620 cell lines, and those proteoforms could be proteoform biomarkers of CRC metastasis. Targeted immunoprecipitation (IP)-TDP characterization and quantification of KRAS4b proteoforms in CRC cell lines and tumors by the Kelleher group revealed crosstalk between genetically encoded mutations and proteoform PTMs.153 Their follow-up TDP study of KRAS isoforms (KRAS4a and KRAS4b) across various cell and tissue samples showed distinct modifications between the two isoforms.7 Functional characterization of a KRAS4b proteoform with truncation at C185 residue revealed their loss of the ability to interact with the plasma membrane, resulting in decreased activation of downstream MAPK signaling. Most recently, D'Ippolito et al. developed an LC-MS/MS-based TDP approach to assess KRAS4B compound engagement in vitro, which is promising to be applied for wide screening of targeted KARS inhibitors for cancer treatment.154 Holt et al. presented a quantitative TDP method with high throughput for studying triple-negative breast cancer cells. It was typically used to evaluate an HDAC inhibitor's impact on histone H4 proteoforms to probe chromatin dynamics and interactions.155 McGee et al. innovated proteoform imaging by developing an individual-ion MS-based multiplexed workflow for top-down tandem MS and label-free quantification. Out of around 1000 identified proteoforms from the same patient, 303 differential proteoforms were discovered between stroma and tumor.156 We expect more and more MS-based TDP studies to be performed in either discovery or targeted mode to bridge cancer genotypes and phenotypes and assist cancer therapeutics development.
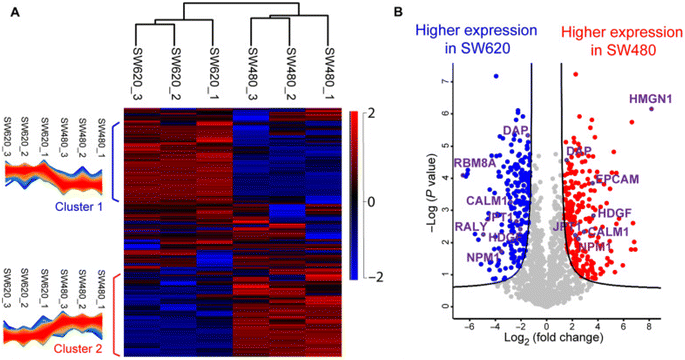 |
| Fig. 4 Summary of the label-free proteoform quantification data of metastatic (SW620) and non-metastatic (SW480) colorectal cancer (CRC) cells from size exclusion chromatography (SEC)-capillary zone electrophoresis (CZE)-tandem mass spectrometry (MS/MS). (A) Heatmap and clusters of the quantified proteoforms from the two cell lines. (B) Volcano plot analysis indicating significantly differentially expressed proteoforms. Reproduced from ref. 5 with permission from the American Association for the Advancement of Science (AAAS), copyright 2022. | |
3.2 Neurodegenerative diseases
Several key proteins, such as amyloid beta, alpha-synuclein, tau, etc., are closely involved in the pathogenesis of Alzheimer's disease (AD), Parkinson's disease (PD), and amyotrophic lateral sclerosis (ALS). The Petyuk group developed a RPLC-FAIMS-MS/MS technique for TDP of brain tissue from an AD patient and achieved the identification of a variety of proteoforms from synuclein, tau, and amyloid beta.157 In particular, diverse fragments of splice isoforms of tau found in this study provide insight into their proteolytic degradation pathways and their functions. The Knierman group characterized 11 alpha-synuclein proteoforms with truncations or PTMs (e.g., phosphorylation) across Parkinson's disease tissues and normal brain tissues.158 The proteoform carrying serine-129 phosphorylation, a widely accepted pathology-associated modification, presented significant differences between disease tissues and controls. The Agar group coupled hydrogen–deuterium exchange with MS to investigate the structural perturbation of SOD1 induced by mutations and PTMs.159 They observed that diverse mutations and PTMs of SOD1 could cause its structural perturbation, which is potentially associated with the progression of sporadic ALS. The DeMarco group recently introduced their work using targeted qualitative and quantitative MS to discover frontotemporal lobar degeneration (FTLD)-specific TDP-43 proteoforms.160 They found truncated TDP-43 proteoforms, especially C-terminal fragments, were highly enriched in FTLD-TDP cases, which can serve as a biomarker with high diagnostic accuracy. Those studies have demonstrated the potential of MS-based TDP for providing novel and useful proteoform-specific information about neurodegenerative diseases. However, MS-based TDP of vital neurodegenerative disease-related proteins, e.g., tau, faces some technical challenges. Intact tau is heavily modified and large (>30 kDa).161 Loo lab implemented direct spray-based native TDMS with ECD fragmentation and ion mobility MS to explore PTMs and structural information of recombinant tau and characterize CLR01 inhibitor binding site on tau.162 Extensive TDP measurement of complex tau proteoforms in endogenous samples still requires high-capacity LC/CE separation, highly sensitive MS detection, and extensive gas-phase fragmentation technologies.
3.3 Cardiac injury
Cardiac injury causes millions of deaths worldwide each year.163 The underlying molecular mechanism of cardiac injury and dysfunction remains unclear. Cardiac troponin I (cTnI) is a key cardiac regulatory protein and a biomarker of cardiac injury. It can form complexes with other cardiac troponin (cTn) proteins to modulate calcium-mediated interaction between actin and myosin to control cardiac contraction. The Ge group performed immunoaffinity enrichment and quantitative TDP of cTnI in normal and diseased heart tissues and reported a strong correlation between phosphorylation on Ser22/23 and heart disease phenotype.164 They also developed a novel nanoproteomics approach based on surface functionalized nanoparticles for sensitive enrichment and TDP characterization of cTnI proteoforms from serum, which is highly applicable for disease diagnosis and evaluation of treatment progress.165 Moreover, their TDP studies were also extended to define sarcomeric proteoform landscape underlying hypertrophic cardiomyopathy (HCM) and ischemic cardiomyopathy (ICM) pathophysiology.166,167 In HCM cases, the concerted reducing phosphorylation in crucial myofilament and Z-disk proteins manifested their PTM crosstalk in the sarcomere and dysregulation of protein kinase A pathways.166 Altered sarcomeric proteoforms were also observed in HCM patients receiving septal myectomy, which are aligned with the same clinical phenotypes despite their differences in HCM-induced mutations. Quantitative TDP comparison of nonfailing donors and end-stage ICM patients showed differential expressions of sarcomeric proteins (e.g., cTnI, ENH2, β-Tpm, α-SKA, etc.) and modifications (e.g., phosphorylation increase in cTnT, calsarcin-1).167 Additionally, Bodin et al. combined TDP with BUP and MALDI-TOF MS to profile acute pathophysiological myocardial changes after cardiac electrical shocks using a sheep model.168 Ramirez-Sagredo et al. applied quantitative TDP to study alternation of intact mitochondrial proteoforms in murine hearts associated with cardiac aging.169 The data in those studies demonstrate the clinical value of proteoform-specific measurement using MS-based TDP.
3.4 Diabetes and cardiovascular diseases (CVD)
Apolipoproteins are important components of lipoprotein particles for transporting blood lipids and are closely associated with diabetes and cardiovascular disease. Apolipoproteins A-I (APOA1, 243 amino acids) and A-II (APOA2, 77 amino acids) are the two most abundant proteins in high-density lipoproteins (HDLs) particles that participate in lipid metabolism.170 The Wang group developed a novel microfluidic-chip-based nanoLC-MS platform for direct monitoring of crucial diabetes biomarkers, including glycated and oxidated APOA1.171 Another TDP study of apolipoproteins reported typical higher abundant APOA1 and APOA2 oxidation in patients with type II diabetes (T2D) and CVD.170 A recent targeted TDP study combining selected-ion monitoring and ETD MS characterized eighteen AOPA1 proteoforms in human serum. The quantitative comparison of two groups of participants with the highest and lowest HDL cholesterol efflux (HDL-E) highlighted elevated canonical, carboxymethylated, and fatty acylated APOA1 proteoforms in high HDL-E patients.172 The Kelleher group introduced a novel procedure coupling native GELFrEE with LC-MS to study APOA1 and APOA2 proteoform heterogeneity across different HDL particle sizes.173 The work indicated higher acylated (fatty acid modification) APOA1 levels in larger HDL particles but similar APOA2 proteoform abundance across size fractions. Besides APOA1 and APOA2, Apolipoprotein C-III (APOC3, 79 amino acids) is also associated with coronary artery disease. APOC3 presents in both HDL and low-density lipoproteins (LDL) and functions as a regulator to control plasma triglyceride levels.170,174 Quantitative TDP of apolipoproteins in human HDL by Mazur et al. found specific APOC3 glycoform linked to coronary artery disease.175 TDP of APOC3 detected various glycoforms that share a core glycan chain but with variations in sialic acid residues.170 The Nedelkov group reported significantly greater ratios of non-sialylated APOC3 proteoforms and sialylated proteoforms in overweight and obese people relative to healthy people.176 The Gordts group also demonstrated that a higher abundance of disialylated over monosialylated APOC3 correlates with lower triglyceride levels in plasma, highlighting the potential value of APOC3 glycoforms as biomarkers for evaluating diabetes and CVD risk.177 Those MS-based TDP studies shed new light on the functions of specific proteoforms of APOA1, APOA2, and APOC3 in modulating diabetes and CVD.
3.5 Infectious disease
MS-based TDP studies were previously implemented to explore potential mechanisms associated with pathogenic bacteria and virus-induced infectious disease and have been summarized in recent reviews.178,179 In recent years, SARS-CoV-2 has caused millions of deaths each year, calling for urgent action to study infection mechanisms, pathogenesis, and therapies. The glycosylated spike (S) protein on SARS-CoV-2 is essential in mediating viral infectivity. S protein RBD becomes the focus of many studies since it is the center region interacting with the angiotensin-converting enzyme 2 (ACE2) receptor on host cells and a promising target for therapeutics. The Ge group applied MS-based TDP to elucidate O-glycoform heterogeneity of SARS-CoV-2 and its Omicron variant on both structure and molecular signature aspects.113,180 In their studies, native TIMS-MS analysis enabled deciphering the structural heterogeneity of RBD proteoforms in SARS-CoV-2 and its Omicron variant samples; denaturing top-down MS on FTICR produced in-depth characterization of glycoforms and accurate localization of glycosylation sites.113,180 Several Omicron-specific O-glycoforms were identified for the first time, providing precious insight into the capability of escaping immunological protection.180 Additionally, different combinations of approaches have been explored to characterize highly complex glycoforms on RBD better. The Domínguez-Vega group carried out the structural and functional characterization of O- and N-glycosylation on RBD expressed in two different mammalian cells using CE-MS and matrix-assisted laser desorption ionization in source-decay (MALDI-ISD) MS.181 The Zhou group evaluated three different separation techniques (RPLC, CE, and hydrophilic interaction chromatography (HILIC)) for resolving glycoforms of intact recombinant RBDs and achieved 200 peaks using HILIC.182 Moreover, MS-based TDP is critical for evaluating immune responses after COVID-19 infection. Recent TDP mapping of immunoglobulin (Ig) repertoire by I2MS after COVID exposure or receiving vaccine documented that the I2MS workflow is robust for assessing immune responses to vaccination and pathogens.183
4. Conclusions and future directions
Biologists have widely accepted proteomics and applied it to basic and translational research.184 However, as the routine approach in proteomics, BUP has difficulties delineating proteoforms because an intact proteoform picture is lost during the enzymatic process. In the past decade, MS-intensive TDP has made tremendous progress in technological development for understanding protein functions in diseases and development via measuring intact proteoforms. Discovery and targeted TDP have been successfully applied to study the function of proteoforms in various diseases (e.g., cancer, neurodegenerative diseases, cardiac injury, diabetes and cardiovascular diseases, and infectious diseases). TDP has discovered novel proteoform biomarkers of diseases.185 We expect that with further technological development of TDP, biologists will widely accept it to offer complementary information to BUP about the roles played by proteins in modulating biological processes.
Much more effort is needed to advance the technique of MS-intensive TDP to facilitate its broad adoption in biological research. Technical development is needed to boost proteome coverage, throughput, and quality of proteoform characterization. Integration of novel sample preparation methods, efficient liquid-phase separations, advanced MS (e.g., charge detection MS, individual ion MS, and native MS), and better bioinformatics tools could be extremely valuable for solving the technical challenges of TDP. We must point out that high-quality and global top-down MS measurement of intact proteoforms, especially large and heavily modified ones, needs mass spectrometers with high speed, high mass resolution, and extensive proteoform gas-phase fragmentation. Those instrumentation are extremely expensive and have high maintenance costs, which is one critical factor limiting the broad use of MS-based TDP.
There are several new frontiers in the MS-intensive TDP field. First, increasing TDP applications are rolling from the conventional denaturing TDP into the native mode (native proteomics) to characterize protein complexes in cells.48,186–191 Second, single-cell TDP will be another hot research area to decipher cell-to-cell heterogeneity at the proteoform level.27,88,89 Third, the human proteoform project185 will be one focus of the TDP community. Fourth, deciphering proteoform function in biological processes and disease progression is essential for the TDP field. As mentioned in this review, many TDP studies have been done to better our understanding of protein function in various diseases. We expect more and more such studies to be carried out with better proteome coverage and proteoform characterization due to the continuous advancements of MS-based TDP techniques. Finally, combining MS-intensive TDP and BUP is crucial to advance TDP for high throughput and extensive characterization of proteoforms in complex samples. BUP data can provide rich information on types and sites of PTMs, which is extremely useful to interpret PTM combinations on proteoforms from TDP measurement. It is also essential to have a standardized procedure from sample preparation to database search, as the emerging field of TDP continues to develop.68,147 Unbiased quality control that singles out high-quality mass spectra is a gateway for building up TDP spectral libraries and, ultimately, the human proteoform atlas.192
Data availability
All the necessary materials related to this work are included in the main manuscript.
Conflicts of interest
The authors declare no competing financial interest.
Acknowledgements
We thank the support from the National Cancer Institute (NCI) through the grant R01CA247863, and from the National Institute of General Medical Sciences (NIGMS) through grants R01GM125991 and R01GM118470. We also thank the support from the National Science Foundation (CAREER Award, Grant DBI1846913).
References
- L. M. Smith and N. L. Kelleher, Consortium for Top Down Proteomics. Proteoform: a single term describing protein complexity, Nat. Methods, 2013, 10(3), 186–187 CrossRef CAS.
- L. M. Smith and N. L. Kelleher, Proteoforms as the next proteomics currency, Science, 2018, 359(6380), 1106–1107 CrossRef CAS PubMed.
- H. A. Costa, M. G. Leitner and M. L. Sos,
et al., Discovery and functional characterization of a neomorphic PTEN mutation, Proc. Natl. Acad. Sci. U. S. A., 2015, 112(45), 13976–13981 CrossRef CAS PubMed.
- X. Yang, J. Coulombe-Huntington and S. Kang,
et al., Widespread Expansion of Protein Interaction Capabilities by Alternative Splicing, Cell, 2016, 164(4), 805–817 CrossRef CAS.
- E. N. McCool, T. Xu and W. Chen,
et al., Deep top-down proteomics revealed significant proteoform-level differences between metastatic and nonmetastatic colorectal cancer cells, Sci. Adv., 2022, 8(51), eabq6348 CrossRef CAS , * The first example of quantitative TDP of colorectal cancer (CRC) cells to understand CRC metastasis better..
- T. Tucholski, W. Cai and Z. R. Gregorich,
et al., Distinct hypertrophic cardiomyopathy genotypes result in convergent sarcomeric proteoform profiles revealed by top-down proteomics, Proc. Natl. Acad. Sci. U. S. A., 2020, 117(40), 24691–24700 CrossRef CAS.
- L. M. Adams, C. J. DeHart and B. S. Drown,
et al., Mapping the KRAS proteoform landscape in colorectal cancer identifies truncated KRAS4B that decreases MAPK signaling, J. Biol. Chem., 2023, 299(1), 102768 CrossRef CAS.
- L. V. Schaffer, R. J. Millikin and R. M. Miller,
et al., Identification and Quantification of Proteoforms by Mass Spectrometry, Proteomics, 2019, 19(10), e1800361 CrossRef , ** A nice review about TDP technologies..
- L. M. Smith, P. M. Thomas and M. R. Shortreed,
et al., A five-level classification system for proteoform identifications, Nat. Methods, 2019, 16, 939–940 CrossRef CAS PubMed.
- Q. Wang, L. Sun and P. K. Lundquist, Large-scale top-down proteomics of the Arabidopsis thaliana and chloroplast proteomes, Proteomics, 2023, e2100377 CrossRef.
- R. A. Lubeckyj and L. Sun, Laser capture microdissection-capillary zone electrophoresis- tandem mass spectrometry (LCM-CZE-MS/MS) for spatially resolved top-down proteomics: a pilot study of zebrafish brain, Mol. Omics, 2022, 18, 112–122 RSC.
- B. S. Drown, K. Jooß and R. D. Melani,
et al., Mapping the Proteoform Landscape of Five Human Tissues, J. Proteome Res., 2022, 21(5), 1299–1310 CrossRef CAS PubMed.
- R. Aebersold, J. N. Agar and I. J. Amster,
et al., How many human proteoforms are there?, Nat. Chem. Biol., 2018, 14(3), 206–214 CrossRef CAS PubMed.
- Q. Kou, L. Xun and X. Liu, TopPIC: a software tool for top-down mass spectrometry-based proteoform identification and characterization, Bioinformatics, 2016, 32(22), 3495–3497 CrossRef CAS.
- R. D. LeDuc, G. K. Taylor and Y. B. Kim,
et al., ProSight PTM: an integrated environment for protein identification and characterization by top-down mass spectrometry, Nucleic Acids Res., 2004, 32(Web Server issue), W340–W345 CrossRef CAS.
- W. Cai, H. Guner and Z. R. Gregorich,
et al., MASH Suite Pro: A Comprehensive Software Tool for Top-Down Proteomics, Mol. Cell. Proteomics, 2016, 15(2), 703–714 CrossRef CAS PubMed.
- A. J. Cesnik, M. R. Shortreed and L. V. Schaffer,
et al., Proteoform Suite: Software for Constructing, Quantifying, and Visualizing Proteoform Families, J. Proteome Res., 2018, 17(1), 568–578 CrossRef CAS PubMed.
- K. A. Brown, J. A. Melby and D. S. Roberts,
et al., Top-down proteomics: challenges, innovations, and applications in basic and clinical research, Expert Rev. Proteomics, 2020, 17(10), 719–733 CrossRef CAS PubMed.
- J. S. Brodbelt, Deciphering combinatorial post-translational modifications by top-down mass spectrometry, Curr. Opin. Chem. Biol., 2022, 70, 102180 CrossRef CAS PubMed.
- T. K. Toby, L. Fornelli and N. L. Kelleher, Progress in Top-Down Proteomics and the Analysis of Proteoforms, Annu. Rev. Anal. Chem., 2016, 9(1), 499–519 CrossRef CAS PubMed.
- T. N. Tiambeng, Z. Wu and J. A. Melby,
et al., Size Exclusion Chromatography Strategies and MASH Explorer for Large Proteoform Characterization, Methods Mol. Biol., 2022, 2500, 15–30 CrossRef CAS PubMed.
- L. Fornelli and T. K. Timothy, Characterization of large intact protein ions by mass spectrometry: What directions should we follow?, Biochim. Biophys. Acta, Proteins Proteomics, 1870,(4), 140758 CrossRef PubMed.
- P. D. Compton, L. Zamdborg and P. M. Thomas,
et al., On the scalability and requirements of whole protein mass spectrometry, Anal. Chem., 2011, 83(17), 6868–6874 CrossRef CAS PubMed.
- J. O. Kafader, R. D. Melani and L. F. Schachner,
et al., Native vs Denatured: An in-Depth Investigation of Charge State and Isotope Distributions, J. Am. Soc. Mass Spectrom., 2020, 31, 574–581 CrossRef CAS PubMed.
- W. Cai, T. Tucholski and B. Chen,
et al., Top-Down Proteomics of Large Proteins up to 223 kDa Enabled by Serial Size Exclusion Chromatography Strategy, Anal. Chem., 2017, 89(10), 5467–5475 CrossRef CAS PubMed.
- L. V. Schaffer, T. Tucholski and M. R. Shortreed,
et al., Intact-Mass Analysis Facilitating the Identification of Large Human Heart Proteoforms, Anal. Chem., 2019, 91(17), 10937–10942 CrossRef CAS.
- J. A. Melby, K. A. Brown and Z. R. Gregorich,
et al., High sensitivity top-down proteomics captures single muscle cell heterogeneity in large proteoforms, Proc. Natl. Acad. Sci. U. S. A., 2023, 120(19), e2222081120 CrossRef CAS , * An excellent example of TDP measurment of mass-limited biological samples via advanced technologies..
- C. Wang, Y. Liang and B. Zhao,
et al., Ethane-Bridged Hybrid Monolithic Column with Large Mesopores for Boosting Top-Down Proteomic Analysis, Anal. Chem., 2022, 94(16), 6172–6179 CrossRef CAS PubMed.
- Y. Li, S. Miao and J. Tan,
et al., Capillary electrophoresis: A three-year literature review, Anal. Chem., 2024, 96, 7799–7816 CrossRef CAS PubMed.
- K. Bagwe, N. Gould and K. R. Johnson,
et al., Single-cell omic molecular profiling using capillary electrophoresis-mass spectrometry, Trends Anal. Chem., 2023, 165, 117117 CrossRef CAS.
- A.-K. Schwenzer, L. Kruse and K. Jooß,
et al., Capillary electrophoresis-mass spectrometry for protein analyses under native conditions: Current progress and perspectives, Proteomics, 2024, 24(2–4), e2300135 CrossRef.
- D. Chen, E. N. McCool and Z. Yang,
et al., Recent advances (2019-2021) of capillary electrophoresis-mass spectrometry for multilevel proteomics, Mass Spectrom. Rev., 2023, 42(2), 617–642 CrossRef CAS PubMed , ** A comprehensive review of CE-MS for BUP, TDP and native proteomics..
- F. P. Gomes and J. R. Yates 3rd, Recent trends of capillary electrophoresis-mass spectrometry in proteomics research, Mass Spectrom. Rev., 2019, 38(6), 445–460 CrossRef CAS PubMed.
- R. A. Lubeckyj, A. R. Basharat and X. Shen,
et al., Large-Scale Qualitative and Quantitative Top-Down Proteomics Using Capillary Zone Electrophoresis-Electrospray Ionization-Tandem Mass Spectrometry with Nanograms of Proteome Samples, J. Am. Soc. Mass Spectrom., 2019, 30(8), 1435–1445 CrossRef CAS PubMed.
- T. T. T. N. Nguyen, N. J. Petersen and K. D. Rand, A simple sheathless CE-MS interface with a sub-micrometer electrical contact fracture for sensitive analysis of peptide and protein samples, Anal. Chim. Acta, 2016, 936, 157–167 CrossRef CAS PubMed.
- E. J. Maxwell, X. Zhong and H. Zhang,
et al., Decoupling CE and ESI for a more robust interface with MS, Electrophoresis, 2010, 31, 1130–1137 CrossRef CAS.
- J. Schlecht, A. Stolz and A. Hofmann,
et al., nanoCEasy: An Easy, Flexible, and Robust Nanoflow Sheath Liquid Capillary Electrophoresis-Mass Spectrometry Interface Based on 3D Printed Parts, Anal. Chem., 2021, 93, 14593–14598 CrossRef CAS PubMed.
- X. Han, Y. Wang and A. Aslanian,
et al., In-line separation by capillary electrophoresis prior to analysis by top-down mass spectrometry enables sensitive characterization of protein complexes, J. Proteome Res., 2014, 13(12), 6078–6086 CrossRef CAS PubMed.
- Y. Li, P. D. Compton and J. C. Tran,
et al., Optimizing capillary electrophoresis for top-down proteomics of 30-80 kDa proteins, Proteomics, 2014, 14(10), 1158–1164 CrossRef PubMed.
- T. Xu, Q. Wang and Q. Wang,
et al., Coupling High-Field Asymmetric Waveform Ion Mobility Spectrometry with Capillary Zone Electrophoresis-Tandem Mass Spectrometry for Top-Down Proteomics, Anal. Chem., 2023, 95(25), 9497–9504 CrossRef CAS PubMed.
- Q. Tang, A. K. Harrata and C. S. Lee, Capillary isoelectric focusing-electrospray mass spectrometry for protein analysis, Anal. Chem., 1995, 67, 3515–3519 CrossRef CAS.
- P. T. Ljiljana, K. J. Pamela and A. A. Gordon,
et al., High Throughput Proteome-Wide Precision Measurements of Protein Expression Using Mass Spectrometry, J. Am. Chem. Soc., 1999, 121, 7949–7950 CrossRef.
- T. Xu and L. Sun, A Mini Review on Capillary Isoelectric Focusing-Mass Spectrometry for Top-Down Proteomics, Front. Chem., 2021, 9, 651757 CrossRef CAS.
- T. Xu, L. Han and A. M. George Thompson,
et al., An improved capillary isoelectric focusing-mass spectrometry method for high-resolution characterization of monoclonal antibody charge variants, Anal. Methods, 2022, 14(4), 383–393 RSC.
- J. Dai, J. Lamp and Q. Xia,
et al., Capillary Isoelectric Focusing-Mass Spectrometry Method for the Separation and Online Characterization of Intact Monoclonal Antibody Charge Variants, Anal. Chem., 2018, 90(3), 2246–2254 CrossRef CAS PubMed.
- L. Wang, T. Bo and Z. Zhang,
et al., High Resolution Capillary Isoelectric Focusing Mass Spectrometry Analysis of Peptides, Proteins, And Monoclonal Antibodies with a Flow-through Microvial Interface, Anal. Chem., 2018, 90(15), 9495–9503 CrossRef CAS PubMed.
- S. Mack, D. Arnold and G. Bogdan,
et al., A novel microchip-based imaged CIEF-MS system for comprehensive characterization and identification of biopharmaceutical charge variants, Electrophoresis, 2019, 40(23–24), 3084–3091 CrossRef CAS.
- T. Xu, L. Han and L. Sun, Automated Capillary Isoelectric Focusing-Mass Spectrometry with Ultrahigh Resolution for Characterizing Microheterogeneity and Isoelectric Points of Intact Protein Complexes, Anal. Chem., 2022, 94(27), 9674–9682 CrossRef CAS PubMed.
- A. Takemori, D. S. Butcher and V. M. Harman,
et al., PEPPI-MS: Polyacrylamide-Gel-Based Prefractionation for Analysis of Intact Proteoforms and Protein Complexes by Mass Spectrometry, J. Proteome Res., 2020, 19(9), 3779–3791 CrossRef CAS PubMed.
- A. Takemori, P. T. Kaulich and L. Cassidy,
et al., Size-Based Proteome Fractionation through Polyacrylamide Gel Electrophoresis Combined with LC–FAIMS–MS for In-Depth Top-Down Proteomics, Anal. Chem., 2022, 94(37), 12815–12821 CrossRef CAS PubMed.
- D. Z. Keifer, T. Motwani and C. M. Teschke,
et al., Measurement of the accurate mass of a 50 MDa infectious virus, Rapid Commun. Mass Spectrom., 2016, 30(17), 1957–1962 CrossRef CAS PubMed.
- T. P. Wörner, J. Snijder and A. Bennett,
et al., Resolving heterogeneous macromolecular assemblies by Orbitrap-based single-particle charge detection mass spectrometry, Nat. Methods, 2020, 17, 395–398 CrossRef PubMed.
- J. O. Kafader, R. D. Melani and K. R. Durbin,
et al., Multiplexed mass spectrometry of individual ions improves measurement of proteoforms and their complexes, Nat. Methods, 2020, 17(4), 391–394 CrossRef CAS PubMed , * A strong demonstration of the principle and application of I2MS for highly sensitive TDP..
- P. Su, J. P. McGee and K. R. Durbin,
et al., Highly multiplexed, label-free proteoform imaging of tissues by individual ion mass spectrometry, Sci. Adv., 2022, 8(32), eabp9929 CrossRef CAS PubMed.
- T. P. Wörner, K. Aizikov and J. Snijder,
et al., Frequency chasing of individual megadalton ions in an Orbitrap analyser improves precision of analysis in single-molecule mass spectrometry, Nat. Chem., 2022, 14(5), 515–522 CrossRef PubMed.
- M. A. Zenaidee and J. A. Loo, Seeing flying molecular elephants more clearly, Nat. Chem., 2022, 14(5), 482–483 CrossRef CAS PubMed.
- N. M. Riley, M. S. Westphall and J. J. Coon, Sequencing Larger Intact Proteins (30-70 kDa) with Activated Ion Electron Transfer Dissociation, J. Am. Soc. Mass Spectrom., 2018, 29(1), 140–149 CrossRef CAS.
- J. F. Greisch, M. A. den Boer and S. H. Lai,
et al., Extending Native Top-Down Electron Capture Dissociation to MDa Immunoglobulin Complexes Provides Useful Sequence Tags Covering Their Critical Variable Complementarity-Determining Regions, Anal. Chem., 2021, 93(48), 16068–16075 CrossRef CAS PubMed.
- J. B. Shaw, W. Li and D. D. Holden,
et al., Complete protein characterization using top-down mass spectrometry and ultraviolet photodissociation, J. Am. Chem. Soc., 2013, 135(34), 12646–12651 CrossRef CAS PubMed.
- S. J. Pitteri and S. A. McLuckey, Recent developments in the ion/ion chemistry of high-mass multiply charged ions, Mass Spectrom. Rev., 2005, 24, 931–958 CrossRef CAS PubMed.
- R. Huguet, C. Mullen and K. Srzentić,
et al., Proton transfer charge reduction enables high-throughput top-down analysis of large proteoforms, Anal. Chem., 2019, 91, 15732–15739 CrossRef CAS PubMed.
- A. O. Bailey, R. Huguet and C. Mullen,
et al., Ion–ion charge reduction addresses multiple challenges common to denaturing intact mass analysis, Anal. Chem., 2022, 94, 3930–3938 CrossRef CAS PubMed.
- J. T. Kline, C. Mullen and K. R. Durbin,
et al., Sequential ion–ion reactions for enhanced gas-phase sequencing of large intact proteins in a tribrid orbitrap mass spectrometer, J. Am. Soc. Mass Spectrom., 2021, 32, 2334–2345 CrossRef CAS PubMed.
- N. B. Borotto and K. A. Graham, Fragmentation and mobility separation of peptide and protein ions in a trapped-ion mobility device, Anal. Chem., 2021, 93, 9959–9964 CrossRef CAS PubMed.
- C. V. Robinson, Mass spectrometry: From plasma proteins to mitochondrial membranes, Proc. Natl. Acad. Sci. U. S. A., 2019, 116(8), 2814–2820 CrossRef CAS PubMed.
- H. Chu, Q. Zhao and J. Liu,
et al., Ionic Liquid-Based Extraction System for In-Depth Analysis of Membrane Protein Complexes, Anal. Chem., 2022, 94(2), 758–767 CrossRef CAS PubMed.
- J. E. Keener, G. Zhang and M. T. Marty, Native Mass Spectrometry of Membrane Proteins, Anal. Chem., 2021, 93, 583–597 CrossRef CAS PubMed.
- D. P. Donnelly, C. M. Rawlins and C. J. DeHart,
et al., Best practices and benchmarks for intact protein analysis for top-down mass spectrometry, Nat. Methods, 2019, 16(7), 587–594 CrossRef CAS PubMed.
- K. A. Brown, B. Chen and T. M. Guardado-Alvarez,
et al., A photocleavable surfactant for top-down proteomics, Nat. Methods, 2019, 16(5), 417–420 CrossRef CAS PubMed.
- K. A. Brown, T. Tucholski and A. J. Alpert,
et al., Top-Down Proteomics of Endogenous Membrane Proteins Enabled by Cloud Point Enrichment and Multidimensional Liquid Chromatography-Mass Spectrometry, Anal. Chem., 2020, 92(24), 15726–15735 CrossRef CAS PubMed.
- Y. Sun, Y. Liang and C. Wang,
et al., Enhancing Top-Down Characterization of Membrane Proteoforms with C8-Functional Amine-Bridged Hybrid Monoliths, Anal. Chem., 2023, 95(17), 6846–6853 CrossRef CAS PubMed.
- Q. Wang, T. Xu and F. Fang,
et al., Capillary Zone Electrophoresis-Tandem Mass Spectrometry for Top-Down Proteomics of Mouse Brain Integral Membrane Proteins, Anal. Chem., 2023, 95(34), 12590–12594 CrossRef CAS PubMed.
- S. Chen, T. Getter and D. Salom,
et al., Capturing a rhodopsin receptor signalling cascade across a native membrane, Nature, 2022, 604(7905), 384–390 CrossRef CAS PubMed.
- O. S. Skinner, A. D. Catherman and B. P. Early,
et al., Fragmentation of integral membrane proteins in the gas phase, Anal. Chem., 2014, 86(9), 4627–4634 CrossRef CAS PubMed.
- V. Zabrouskov and J. P. Whitelegge, Increased Coverage in the Transmembrane Domain with Activated-Ion Electron Capture Dissociation for Top-Down Fourier-Transform Mass Spectrometry of Integral Membrane Proteins, J. Proteome Res., 2007, 6, 2205–2210 CrossRef CAS PubMed.
- C. Lantz, B. Wei and B. Zhao,
et al., Native Top-Down Mass Spectrometry with Collisionally Activated Dissociation Yields Higher-Order Structure Information for Protein Complexes, J. Am. Chem. Soc., 2022, 144(48), 21826–21830 CrossRef CAS PubMed.
- S. D. Dunham, B. Wei and C. Lantz,
et al., Impact of Internal Fragments on Top-Down Analysis of Intact Proteins by 193 nm UVPD, J. Proteome Res., 2023, 22(1), 170–181 CrossRef CAS PubMed.
- S. N. Sipe, J. W. Patrick and A. Laganowsky,
et al., Enhanced Characterization of Membrane Protein Complexes by Ultraviolet Photodissociation Mass Spectrometry, Anal. Chem., 2020, 92(1), 899–907 CrossRef CAS PubMed.
- I. D. Campuzano, H. Li and D. Bagal,
et al., Native MS Analysis of Bacteriorhodopsin and an Empty Nanodisc by Orthogonal Acceleration Time-of-Flight, Orbitrap and Ion Cyclotron Resonance, Anal. Chem., 2016, 88(24), 12427–12436 CrossRef CAS PubMed.
- C. A. Lutomski, T. J. El-Baba and J. D. Hinkle,
et al., Infrared Multiphoton Dissociation Enables Top-Down Characterization of Membrane Protein Complexes and G Protein-Coupled Receptors, Angew. Chem., Int. Ed. Engl., 2023, 62(36), e202305694 CrossRef CAS PubMed.
- B. R. Juliano, J. W. Keating and B. T. Ruotolo, Infrared Photoactivation Enables Improved Native Top-Down Mass Spectrometry of Transmembrane Proteins, Anal. Chem., 2023, 95, 13361–13367 CrossRef CAS PubMed.
- R. D. Melani, V. R. Gerbasi and L. C. Anderson,
et al., The Blood Proteoform Atlas: A reference map of proteoforms in human hematopoietic cells, Science, 2022, 375(6579), 411–418 CrossRef CAS PubMed , * The first comprehensive TDP study of blood proteoforms with the identification of 30
000 proteoforms..
- T. Xu, X. Shen and Z. Yang,
et al., Automated Capillary Isoelectric Focusing-Tandem Mass Spectrometry for Qualitative and Quantitative Top-Down Proteomics, Anal. Chem., 2020, 92(24), 15890–15898 CrossRef CAS PubMed.
- Z. Wang, D. Yu and K. A. Cupp-Sutton,
et al., Development of an Online 2D Ultrahigh-Pressure Nano-LC System for High-pH and Low-pH Reversed Phase Separation in Top-Down Proteomics, Anal. Chem., 2020, 92(19), 12774–12777 CrossRef CAS PubMed.
- Y. Shen, N. Tolić and C. Masselon,
et al., Nanoscale proteomics, Anal. Bioanal. Chem., 2004, 378(4), 1037–1045 CrossRef CAS PubMed.
- L. Yi, P. D. Piehowski and T. Shi,
et al., Advances in microscale separations towards nanoproteomics applications, J. Chromatogr. A, 2017, 1523, 40–48 CrossRef CAS PubMed.
- Y. Zhu, P. D. Piehowski and R. Zhao,
et al., Nanodroplet processing platform for deep and quantitative proteome profiling of 10-100 mammalian cells, Nat. Commun., 2018, 9(1), 882 CrossRef PubMed.
- M. Zhou, N. Uwugiaren and S. M. Williams,
et al., Sensitive Top-Down Proteomics Analysis of a Low Number of Mammalian Cells Using a Nanodroplet Sample Processing Platform, Anal. Chem., 2020, 92(10), 7087–7095 CrossRef CAS PubMed.
- K. R. Johnson, Y. Gao and M. Greguš,
et al., On-capillary Cell Lysis Enables Top-down Proteomic Analysis of Single Mammalian Cells by CE-MS/MS, Anal. Chem., 2022, 94(41), 14358–14367 CrossRef CAS PubMed.
- L. Huang, M. Fang and K. A. Cupp-Sutton,
et al., Spray-Capillary-Based Capillary Electrophoresis Mass Spectrometry for Metabolite Analysis in Single Cells, Anal. Chem., 2021, 93(10), 4479–4487 CrossRef CAS PubMed.
- A. I. Badeaux and Y. Shi, Emerging roles for chromatin as a signal integration and storage platform, Nat. Rev. Mol. Cell Biol., 2013, 14(4), 211–224 CrossRef CAS PubMed.
- H. Kimura, Histone modifications for human epigenome analysis, J. Hum. Genet., 2013, 58(7), 439–445 CrossRef CAS PubMed.
- T. Jenuwein and C. D. Allis, Translating the histone code, Science, 2001, 293(5532), 1074–1080 CrossRef CAS PubMed.
- C. L. Woodcock, A. I. Skoultchi and Y. Fan, Role of linker histone in chromatin structure and function: H1 stoichiometry and nucleosome repeat length, Chromosome Res., 2006, 14(1), 17–25 CrossRef CAS PubMed.
- H. Kimura, Histone dynamics in living cells revealed by photobleaching, DNA Repair, 2005, 4(8), 939–950 CrossRef CAS PubMed.
- C. D. Allis and T. Jenuwein, The molecular hallmarks of epigenetic control, Nat. Rev. Genet., 2016, 17(8), 487–500 CrossRef CAS PubMed.
- Y. Zhao and B. A. Garcia, Comprehensive Catalog of Currently Documented Histone Modifications, Cold Spring Harbor Perspect. Biol., 2015, 7(9), a025064 CrossRef PubMed.
- D. T. Brown, Histone variants: are they functionally heterogeneous?, Genome Biol., 2001, 2(7), REVIEWS0006 CrossRef CAS PubMed.
- D. Chen, Z. Yang and X. Shen,
et al., Capillary Zone Electrophoresis-Tandem Mass Spectrometry As an Alternative to Liquid Chromatography-Tandem Mass Spectrometry for Top-down Proteomics of Histones, Anal. Chem., 2021, 93(10), 4417–4424 CrossRef CAS PubMed.
- Q. Wang, F. Fang and Q. Wang,
et al., Capillary zone electrophoresis-high field asymmetric ion mobility spectrometry-tandem mass spectrometry for top-down characterization of histone proteoforms, Proteomics, 2024, 24(3–4), e2200389 CrossRef PubMed.
- J. S. Brodbelt, Deciphering combinatorial post-translational modifications by top-down mass spectrometry, Curr. Opin. Chem. Biol., 2022, 70, 102180 CrossRef CAS PubMed.
- J. N. Walker, R. Lam and J. S. Brodbelt, Enhanced Characterization of Histones Using 193 nm Ultraviolet Photodissociation and Proton Transfer Charge Reduction, Anal. Chem., 2023, 95(14), 5985–5993 CrossRef CAS PubMed.
- F. Berthias, H. A. Thurman and G. Wijegunawardena,
et al., Top-Down Ion Mobility Separations of Isomeric Proteoforms, Anal. Chem., 2023, 95(2), 784–791 CAS.
- A. Moradian, A. Kalli and M. J. Sweredoski,
et al., The top-down, middle-down, and bottom-up mass spectrometry approaches for characterization of histone variants and their post-translational modifications, Proteomics, 2014, 14(4–5), 489–497 CrossRef CAS PubMed.
- A. Varki, Biological roles of glycans, Glycobiology, 2017, 27(1), 3–49 CrossRef CAS PubMed.
- C. Reily, T. J. Stewart, M. B. Renfrow and J. Novak, Glycosylation in health and disease, Nat. Rev. Nephrol., 2019, 15(6), 346–366 CrossRef PubMed.
- R. Yan, Y. Zhang and Y. Li,
et al., Structural basis for the recognition of SARS-CoV-2 by full-length human ACE2, Science, 2020, 367(6485), 1444–1448 CrossRef CAS PubMed.
- M. Hoffmann, H. Kleine-Weber and S. Schroeder,
et al., SARS-CoV-2 Cell Entry Depends on ACE2 and TMPRSS2 and Is Blocked by a Clinically Proven Protease Inhibitor, Cell, 2020, 181(2), 271–280.e8 CrossRef CAS PubMed.
- Y. Watanabe, J. D. Allen and D. Wrapp,
et al., Site-specific glycan analysis of the SARS-CoV-2 spike, Science, 2020, 369(6501), 330–333 CrossRef CAS PubMed.
- K. G. Andersen, A. Rambaut and W. I. Lipkin,
et al., The proximal origin of SARS-CoV-2, Nat. Med., 2020, 26(4), 450–452 CrossRef CAS PubMed.
- A. Shajahan, N. T. Supekar and A. S. Gleinich,
et al., Deducing the N- and O-glycosylation profile of the spike protein of novel coronavirus SARS-CoV-2, Glycobiology, 2020, 30(12), 981–988 CrossRef CAS PubMed.
- Y. Watanabe, T. A. Bowden and I. A. Wilson,
et al., Exploitation of glycosylation in enveloped virus pathobiology, Biochim. Biophys. Acta, Gen. Subj., 2019, 1863(10), 1480–1497 CrossRef CAS PubMed.
- D. S. Roberts, M. Mann and J. A. Melby,
et al., Structural O-Glycoform Heterogeneity of the SARS-CoV-2 Spike Protein Receptor-Binding Domain Revealed by Top-Down Mass Spectrometry, J. Am. Chem. Soc., 2021, 143(31), 12014–12024 CrossRef CAS PubMed.
- L. M. Miller, L. F. Barnes and S. A. Raab,
et al., Heterogeneity of Glycan Processing on Trimeric SARS-CoV-2 Spike Protein Revealed by Charge Detection Mass Spectrometry, J. Am. Chem. Soc., 2021, 143(10), 3959–3966 CrossRef CAS PubMed.
- S. Wu, J. N. Brown and N. Tolić,
et al., Quantitative analysis of human salivary gland-derived intact proteome using top-down mass spectrometry, Proteomics, 2014, 14, 1211–1222 CrossRef CAS PubMed.
- I. Ntai, K. Kim and R. T. Fellers,
et al., Applying Label-Free Quantitation to Top Down Proteomics, Anal. Chem., 2014, 86, 4961–4968 CrossRef CAS PubMed.
- J. Sinclair and J. F. Timms, Quantitative profiling of serum samples using TMT protein labelling, fractionation and LC–MS/MS, Methods, 2011, 54, 361–369 CrossRef CAS PubMed.
- H. Fang, K. Xiao and Y. Li,
et al., Intact Protein Quantitation Using Pseudoisobaric Dimethyl Labeling, Anal. Chem., 2016, 88, 7198–7205 CrossRef CAS PubMed.
- C.-W. Hung and A. Tholey, Tandem Mass Tag Protein Labeling for Top-Down Identification and Quantification, Anal. Chem., 2012, 84, 161–170 CrossRef CAS PubMed.
- S. Wiese, K. A. Reidegeld and H. E. Meyer,
et al., Protein labeling by iTRAQ: A new tool for quantitative mass spectrometry in proteome research, Proteomics, 2007, 7, 340–350 CrossRef CAS PubMed.
- Z. Liu, R. Wang and J. Liu,
et al., Global Quantification of Intact Proteins via Chemical Isotope Labeling and Mass Spectrometry, J. Proteome Res., 2019, 18, 2185–2194 CrossRef CAS PubMed.
- D. Yu, Z. Wang and K. A. Cupp-Sutton,
et al., Quantitative Top-Down Proteomics in Complex Samples Using Protein-Level Tandem Mass Tag Labeling, J. Am. Soc. Mass Spectrom., 2021, 32, 1336–1344 CrossRef CAS PubMed.
- K. A. Cupp-Sutton and S. Wu, High-throughput quantitative top-down proteomics, Mol. Omics, 2020, 16, 91–99 RSC.
- Y. Guo, D. Yu and K. A. Cupp-Sutton,
et al., Optimization of protein-level tandem mass tag (TMT) labeling conditions in complex samples with top-down proteomics, Anal. Chim. Acta, 2022, 1221, 340037 CrossRef CAS PubMed.
- Y. Guo, T. Chowdhury and M. Seshadri,
et al., Optimization of Higher-Energy Collisional Dissociation Fragmentation Energy for Intact Protein-Level Tandem Mass Tag Labeling, J. Proteome Res., 2023, 22, 1406–1418 CrossRef CAS PubMed.
- R.-X. Sun, L. Luo and L. Wu,
et al., pTop 1.0: A High-Accuracy and High-Efficiency Search Engine for Intact Protein Identification, Anal. Chem., 2016, 88, 3082–3090 CrossRef CAS PubMed.
- J. Park, P. D. Piehowski and C. Wilkins,
et al., Informed-Proteomics: Open Source Software Package for Top-down Proteomics, Nat. Methods, 2017, 14, 909–914 CrossRef CAS PubMed.
- S. K. Solntsev, M. R. Shortreed, B. L. Frey and L. M. Smith, Enhanced Global Post-translational Modification Discovery with MetaMorpheus, J. Proteome Res., 2018, 17(5), 1844–1851 CrossRef CAS PubMed.
- M. T. Marty, A. J. Baldwin and E. G. Marklund,
et al., Bayesian deconvolution of mass and ion mobility spectra: From binary interactions to polydisperse ensembles, Anal. Chem., 2015, 87, 4370–4376 CrossRef CAS PubMed.
- K. Jeong, P. T. Kaulich and W. Jung,
et al., Precursor deconvolution error estimation: The missing puzzle piece in false discovery rate in top-down proteomics, Proteomics, 2024, 3–4, 2300068 CrossRef PubMed.
- K. Jeong, J. Kim and M. Gaikwad,
et al., FLASHDeconv: Ultrafast, High-Quality Feature Deconvolution for Top-Down Proteomics, Cell Syst., 2020, 10, 213–218.e6 CrossRef CAS PubMed.
- K. Jeong, M. Babović and V. Gorshkov,
et al., FLASHIda enables intelligent data acquisition for top–down proteomics to boost proteoform identification counts, Nat. Commun., 2022, 13, 4407 CrossRef CAS PubMed.
- L. Lu, M. Scalf and M. R. Shortreed,
et al., Mesh fragmentation improves dissociation efficiency in top-down proteomics, J. Am. Soc. Mass Spectrom., 2021, 32, 1319–1325 CrossRef CAS PubMed.
- S. R. Harvey, M. Porrini and A. Konijnenberg,
et al., Dissecting the dynamic conformations of the metamorphic protein lymphotactin, J. Phys. Chem. B, 2014, 118(43), 12348–12359 CrossRef CAS PubMed.
- K. R. Durbin, O. S. Skinner and R. T. Fellers,
et al., Analyzing internal fragmentation of electrosprayed ubiquitin ions during beam-type collisional dissociation, J. Am. Soc. Mass Spectrom., 2015, 26(5), 782–787 CrossRef CAS PubMed.
- M. A. Zenaidee, C. Lantz and T. Perkins,
et al., Internal Fragments Generated by Electron Ionization Dissociation Enhance Protein Top-Down Mass Spectrometry, J. Am. Soc. Mass Spectrom., 2020, 31(9), 1896–1902 CrossRef CAS PubMed.
- M. A. Zenaidee, B. Wei and C. Lantz,
et al., Internal Fragments Generated from Different Top-Down Mass Spectrometry Fragmentation Methods Extend Protein Sequence Coverage, J. Am. Soc. Mass Spectrom., 2021, 32(7), 1752–1758 CrossRef CAS PubMed.
- B. Wei, M. A. Zenaidee and C. Lantz,
et al., Towards understanding the formation of internal fragments generated by collisionally activated dissociation for top-down mass spectrometry, Anal. Chim. Acta, 2022, 1194, 339400 CrossRef CAS PubMed.
- B. Wei, C. Lantz and W. Liu,
et al., Added Value of Internal Fragments for Top-Down Mass Spectrometry of Intact Monoclonal Antibodies and Antibody-Drug Conjugates, Anal. Chem., 2023, 95(24), 9347–9356 CrossRef CAS PubMed.
- C. Lantz, M. A. Zenaidee and B. Wei,
et al., ClipsMS: An Algorithm for
Analyzing Internal Fragments Resulting from Top-Down Mass Spectrometry, J. Proteome Res., 2021, 20(4), 1928–1935 CrossRef CAS PubMed.
- Z. Rolfs and L. M. Smith, Internal Fragment Ions Disambiguate and Increase Identifications in Top-Down Proteomics, J. Proteome Res., 2021, 20(12), 5412–5418 CrossRef CAS PubMed.
- D. Chen, R. A. Lubeckyj and Z. Yang,
et al., Predicting Electrophoretic Mobility of Proteoforms for Large-Scale Top-Down Proteomics, Anal. Chem., 2020, 92, 3503–3507 CrossRef CAS PubMed.
- W. Chen, E. N. McCool and L. Sun,
et al., Evaluation of Machine Learning Models for Proteoform Retention and Migration Time Prediction in Top-Down Mass Spectrometry, J. Proteome Res., 2022, 21, 1736 CrossRef CAS PubMed.
- W. Chen, Z. Ding and Y. Zang,
et al., Characterization of Proteoform Post-Translational Modifications by Top-Down and Bottom-Up Mass Spectrometry in Conjunction with Annotations, J. Proteome Res., 2023, 22, 3178–3189 CrossRef CAS PubMed.
- P. T. Kaulich, K. Winkels and T. B. Kaulich,
et al., MSTopDiff: A Tool for the Visualization of Mass Shifts in Deconvoluted Top-Down Proteomics Data for the Database-Independent Detection of Protein Modifications, J. Proteome Res., 2022, 21, 20–29 CrossRef CAS PubMed.
- E. A. Martin, J. M. Fulcher and M. Zhou,
et al., TopPICR: A Companion R Package for Top-Down Proteomics Data Analysis, J. Proteome Res., 2023, 22, 399–409 CrossRef CAS PubMed.
- D. L. Tabb, K. Jeong and K. Druart,
et al., Comparing Top-Down Proteoform Identification: Deconvolution, PrSM Overlap, and PTM Detection, J. Proteome Res., 2023, 22, 2199–2217 CrossRef CAS PubMed.
- Z. Wu, D. S. Roberts and J. A. Melby,
et al., MASH Explorer: A Universal Software Environment for Top-Down Proteomics, J. Proteome Res., 2020, 19, 3867–3876 CrossRef CAS PubMed.
- M. A. Eckert, F. Coscia and A. Chryplewicz,
et al., Proteomics reveals NNMT as a master metabolic regulator of cancer-associated fibroblasts, Nature, 2019, 569(7758), 723–728 CrossRef CAS PubMed.
- A. A. Cohen, N. Geva-Zatorsky and E. Eden,
et al., Dynamic proteomics of individual cancer cells in response to a drug, Science, 2008, 322(5907), 1511–1516 CrossRef CAS PubMed.
- P. R. Srinivas, M. Verma and Y. Zhao,
et al., Proteomics for cancer biomarker discovery, Clin. Chem., 2002, 48(8), 1160–1169 CAS.
- I. Ntai, R. D. LeDuc and R. T. Fellers,
et al., Integrated Bottom-Up and Top-Down Proteomics of Patient-Derived Breast Tumor Xenografts, Mol. Cell. Proteomics, 2016, 15(1), 45–56 CrossRef CAS PubMed.
- I. Ntai, L. Fornelli and C. J. DeHart,
et al., Precise characterization of KRAS4b proteoforms in human colorectal cells and tumors reveals mutation/modification cross-talk, Proc. Natl. Acad. Sci. U. S. A., 2018, 115(16), 4140–4145 CrossRef CAS PubMed.
- R. A. D'Ippolito, D. Rabara, M. A. Blanco and E. Alberico,
et al., A Top-Down Proteomic Assay to Evaluate KRAS4B-Compound Engagement, Anal. Chem., 2024, 96(13), 5223–5231 CrossRef PubMed.
- M. V. Holt, T. Wang and N. L. Young, High-throughput quantitative top-down proteomics: histone H4, J. Am. Soc. Mass Spectrom., 2019, 30(12), 2548–2560 CrossRef CAS PubMed.
- J. P. McGee, P. Su, K. R. Durbin, M. A. Hollas, N. W. Bateman, G. L. Maxwell, T. P. Conrads, R. T. Fellers, R. D. Melani, J. M. Camarillo and J. O. Kafader, Automated imaging and identification of proteoforms directly from ovarian cancer tissue, Nat. Commun., 2023, 14(1), 6478 CrossRef CAS PubMed.
- J. M. Fulcher, A. Makaju and R. J. Moore,
et al., Enhancing Top-Down Proteomics of Brain Tissue with FAIMS, J. Proteome Res., 2021, 20(5), 2780–2795 CrossRef CAS PubMed.
- J. F. Kellie, R. E. Higgs and J. W. Ryder,
et al., Quantitative measurement of intact alpha-synuclein proteoforms from post-mortem control and Parkinson's disease brain tissue by intact protein mass spectrometry, Sci. Rep., 2014, 4, 5797 CrossRef CAS PubMed.
- N. D. Schmitt and J. N. Agar, Parsing disease-relevant protein modifications from epiphenomena: perspective on the structural basis of SOD1-mediated ALS, J. Mass Spectrom., 2017, 52(7), 480–491 CrossRef CAS PubMed.
- L. M. Forgrave, K. M. Moon, J. E. Hamden, Y. Li, P. Lu, L. J. Foster, I. R. Mackenzie and M. L. DeMarco, Truncated TDP-43 proteoforms diagnostic of frontotemporal dementia with TDP-43 pathology, Alzheimer’s Dementia, 2024, 20(1), 103–111 CrossRef CAS PubMed.
- H. Wesseling, W. Mair and M. Kumar,
et al., Tau PTM Profiles Identify Patient Heterogeneity and Stages of Alzheimer's Disease, Cell, 2020, 183(6), 1699–1713.e13 CrossRef CAS PubMed.
- M. Nshanian, C. Lantz, P. Wongkongkathep and T. Schrader,
et al., Native top-down mass spectrometry and ion mobility spectrometry of the interaction of tau protein with a molecular tweezer assembly modulator, J. Am. Soc. Mass Spectrom., 2018, 30(1), 16–23 CrossRef PubMed.
- H. V. Tran, M. Charles and R. C. Garrett,
et al., Ten-year trends in traumatic cardiac injury and outcomes: a trauma registry analysis, Ann. Thorac. Surg., 2020, 110(3), 844–848 CrossRef PubMed.
- J. Zhang, M. J. Guy and H. S. Norman,
et al., Top-down quantitative proteomics identified phosphorylation of cardiac troponin I as a candidate biomarker for chronic heart failure, J. Proteome Res., 2011, 10(9), 4054–4065 CrossRef CAS PubMed.
- T. N. Tiambeng, D. S. Roberts and K. A. Brown,
et al., Nanoproteomics enables proteoform-resolved analysis of low-abundance proteins in human serum, Nat. Commun., 2020, 11(1), 3903 CrossRef CAS PubMed.
- T. Tucholski, W. Cai and Z. R. Gregorich,
et al., Distinct hypertrophic cardiomyopathy genotypes result in convergent sarcomeric proteoform profiles revealed by top-down proteomics, Proc. Natl. Acad. Sci. U. S. A., 2020, 117(40), 24691–24700 CrossRef CAS PubMed.
- E. A. Chapman, T. J. Aballo and J. A. Melby,
et al., Defining the Sarcomeric Proteoform Landscape in Ischemic Cardiomyopathy by Top-Down Proteomics, J. Proteome Res., 2023, 22(3), 931–941 CrossRef CAS PubMed.
- A. Bodin, V. Labas, A. Bisson, A. P. Teixeira-Gomes, H. Blasco, D. Tomas, L. Combes-Soia, P. Marcelo, E. Miquelestorena-Standley, C. Baron and D. Angoulvant, Acute pathophysiological myocardial changes following intra-cardiac electrical shocks using a proteomic approach in a sheep model, Sci. Rep., 2020, 10(1), 20252 CrossRef CAS PubMed.
-
A. Ramirez-Sagredo, A. Sunny, K. Cupp-Sutton, et al., Characterizing Age-related Changes in Intact Mitochondrial Proteoforms in Murine Hearts using Quantitative Top-Down Proteomics, Res. Sq. [Preprint], 2024, DOI:10.21203/rs.3.rs-3868218/v1.
- D. Nedelkov, Mass spectrometric studies of apolipoprotein proteoforms and their role in lipid metabolism and type 2 diabetes, Proteomes, 2017, 5(4), 27 CrossRef CAS PubMed.
- P. Mao and D. Wang, Top-down proteomics of a drop of blood for diabetes monitoring, J. Proteome Res., 2014, 13(3), 1560–1569 CrossRef CAS PubMed.
- H. D. S. Seckler, L. Fornelli and R. K. Mutharasan,
et al., A targeted, differential top-down proteomic methodology for comparison of ApoA-I proteoforms in individuals with high and low HDL efflux capacity, J. Proteome Res., 2018, 17(6), 2156–2164 CrossRef CAS PubMed.
- C. Lloyd-Jones, H. dos Santos Seckler and N. DiStefano,
et al., Preparative Electrophoresis for HDL Particle Size Separation and Intact-Mass Apolipoprotein Proteoform Analysis, J. Proteome Res., 2023, 22(5), 1455–1465 CrossRef CAS PubMed.
- J. Borén, C. J. Packard and M. R. Taskinen, The roles of ApoC-III on the metabolism of triglyceride-rich lipoproteins in humans, Front. Endocrinol., 2020, 11, 474 CrossRef PubMed.
- M. T. Mazur, H. L. Cardasis, D. S. Spellman, A. Liaw, N. A. Yates and R. C. Hendrickson, Quantitative analysis of intact apolipoproteins in human HDL by top-down differential mass spectrometry, Proc. Natl. Acad. Sci. U. S. A., 2010, 107(17), 7728–7733 CrossRef CAS PubMed.
- H. N. Yassine, O. Trenchevska and A. Ramrakhiani,
et al., The association of human apolipoprotein C-III sialylation proteoforms with plasma triglycerides, PLoS One, 2015, 10(12), e0144138 CrossRef PubMed.
- N. C. Kegulian, B. Ramms and S. Horton,
et al., ApoC-III glycoforms are differentially cleared by hepatic TRL (triglyceride-rich lipoprotein) receptors, Arterioscler., Thromb., Vasc. Biol., 2019, 39(10), 2145–2156 CrossRef CAS PubMed.
- Z. R. Gregorich and Y. Ge, Top-down proteomics in health and disease: Challenges and opportunities, Proteomics, 2014, 14(10), 1195–1210 CrossRef CAS PubMed.
- Y. Simanjuntak, K. Schamoni-Kast and A. Grün,
et al., Top-Down and Bottom-Up Proteomics Methods to Study RNA Virus Biology, Viruses, 2021, 13(4), 668 CrossRef CAS PubMed.
- D. S. Roberts, M. Mann and B. H. Li,
et al., Distinct core glycan and O-glycoform utilization of SARS-CoV-2 Omicron variant Spike protein RBD revealed by top-down mass spectrometry, Chem. Sci., 2022, 13(36), 10944–10949 RSC.
- C. Gstottner, T. Zhang and A. Resemann,
et al., Structural and functional characterization of SARS-CoV-2 RBD domains produced in mammalian cells, Anal. Chem., 2021, 93(17), 6839–6847 CrossRef PubMed.
- J. W. Wilson, A. Bilbao and J. Wang,
et al., Online hydrophilic interaction chromatography (HILIC) enhanced top-down mass spectrometry characterization of the SARS-CoV-2 spike receptor-binding domain, Anal. Chem., 2022, 94(15), 5909–5917 CrossRef CAS PubMed.
- R. D. Melani, B. J. Des Soye and J. O. Kafader,
et al., Next-generation serology by mass spectrometry: readout of the SARS-CoV-2 antibody repertoire, J. Proteome Res., 2021, 21(1), 274–288 CrossRef PubMed.
- F. Meissner, J. Geddes-McAlister and M. Mann,
et al., The emerging role of mass spectrometry-based proteomics in drug discovery, Nat. Rev. Drug Discovery, 2022, 21(9), 637–654 CrossRef CAS PubMed.
- L. M. Smith, J. N. Agar and J. Chamot-Rooke,
et al., The Human Proteoform Project: Defining the human proteome, Sci. Adv., 2021, 7(46), eabk0734 CrossRef CAS PubMed.
- X. Shen, Q. Kou and R. Guo,
et al., Native Proteomics in Discovery Mode Using Size-Exclusion Chromatography-Capillary Zone Electrophoresis-Tandem Mass Spectrometry, Anal. Chem., 2018, 90(17), 10095–10099 CrossRef CAS PubMed.
- O. S. Skinner, N. A. Haverland and L. Fornelli,
et al., Top-down characterization of endogenous protein complexes with native proteomics, Nat. Chem. Biol., 2018, 14(1), 36–41 CrossRef CAS PubMed.
- A. M. Belov, R. Viner and M. R. Santos,
et al., Analysis of Proteins, Protein Complexes, and Organellar Proteomes Using Sheathless Capillary Zone Electrophoresis - Native Mass Spectrometry, J. Am. Soc. Mass Spectrom., 2017, 28(12), 2614–2634 CrossRef CAS PubMed.
- Y. Ge, E. Chapman and D. Roberts,
et al., Structure and dynamics of endogenous protein complexes in human heart tissue captured by native nanoproteomics, Res. Sq., 2023, rs.3.rs-3108087 Search PubMed.
- J. Yates III, F. Gomes and K. Durbin,
et al., Native top-down proteomics reveals EGFR-ERα signaling crosstalk in breast cancer cells dissociates NUTF2 dimers to modulate ERα signaling and cell growth, Res. Sq., 2023, rs.3.rs-3097806 Search PubMed.
- M. R. Mehaffey, Q. Xia and J. S. Brodbelt, Uniting Native Capillary Electrophoresis and Multistage Ultraviolet Photodissociation Mass Spectrometry for Online Separation and Characterization of Escherichia coli Ribosomal Proteins and Protein Complexes, Anal. Chem., 2020, 92(22), 15202–15211 CrossRef CAS PubMed.
- D. B. Lima, A. R. F. Silva and M. Dupré,
et al., Top-Down Garbage Collector: a tool for selecting high-quality top-down proteomics mass spectra, Bioinformatics, 2019, 35, 3489–3490 CrossRef CAS PubMed.
Footnote |
† Those two authors contributed equally to this work. |
|
This journal is © The Royal Society of Chemistry 2024 |