DOI:
10.1039/D2LC01096H
(Critical Review)
Lab Chip, 2023,
23, 1726-1751
Recent advances in microfluidics for single-cell functional proteomics
Received
28th November 2022
, Accepted 16th February 2023
First published on 22nd February 2023
Abstract
Single-cell proteomics (SCP) reveals phenotypic heterogeneity by profiling individual cells, their biological states and functional outcomes upon signaling activation that can hardly be probed via other omics characterizations. This has become appealing to researchers as it enables an overall more holistic view of biological details underlying cellular processes, disease onset and progression, as well as facilitates unique biomarker identification from individual cells. Microfluidic-based strategies have become methods of choice for single-cell analysis because they allow facile assay integrations, such as cell sorting, manipulation, and content analysis. Notably, they have been serving as an enabling technology to improve the sensitivity, robustness, and reproducibility of recently developed SCP methods. Critical roles of microfluidics technologies are expected to further expand rapidly in advancing the next phase of SCP analysis to reveal more biological and clinical insights. In this review, we will capture the excitement of the recent achievements of microfluidics methods for both targeted and global SCP, including efforts to enhance the proteomic coverage, minimize sample loss, and increase multiplexity and throughput. Furthermore, we will discuss the advantages, challenges, applications, and future prospects of SCP.
1. Introduction
Complex biological systems are regulated by dynamic changes in individual cells, including cell types and their biological states, as well as their interactions with the cellular microenvironments. The conventional ensemble measurements of the population in many cases cannot represent the exact state of an individual cell as it averages the differences among different cells. The effect of such a heterogeneity on both normal and pathological samples urges single-cell analyses at the genomic, transcriptomic, and proteomic levels. The advent of omics-based molecular profiling down to the single-cell level has been one of the most important breakthroughs in recent biological research.1 Technological improvements in genomics and transcriptomics have progressively advanced over the last two decades, enabling characterization of the whole genome and transcriptome from a single cell due to the feasibility of the genetic amplification.2–4 While recent reports indicate that the proteomic profiles poorly correlates with the mRNA expressions,5,6 analyzing proteome from a single cell has been challenging due to the lack of an amplification strategy and the wide dynamic range of proteome.7 To elucidate the missing inference among translational processes, comprehensive characterization of proteins is necessitated to complement genetic measurements at the single-cell level.
Single-cell proteomics (SCP) is a fast-growing research field, and its implementation to study biological systems has revealed a plethora of key insights regarding phenotypes among isogenic cells and cellular processes within the same cell.7–10 SCP profiling has been majorly developed as targeted and non-targeted (global) methods. Targeted methods are employed to detect the secreted and intracellular proteins based on labeled affinity probes such as antibodies. On the other hand, mass spectrometry (MS) is the main instrumentation for global proteomics analysis, which has been broadly used to profile near-complete proteomes from bulk samples.11 Yet, the feasibility and sensitivity of MS are significantly compromised at the single-cell level when using the traditional proteomics preparation workflows.12
To address these limitations, enormous effort has thus been devoted to the miniaturization of sample preparations, optimization of analytical workflows, and integration of instruments, aiming to achieve a more streamlined SCP protocol with minimal sample-loss. Microfluidics, in particular, has been well-suited and employed for such endeavors. It is a multidisciplinary platform for accurate handling, observing, and processing of nano- to femto-liter fluidic samples into desired microengineered compartments.13,14 In addition, microfluidics-based methods possess intrinsic properties of cost-effective analysis, low consumptions of samples and reagents, increased reaction kinetics, and reduced contact area, hence resulting in more sensitive detections.14 Over the last decade, microfluidics systems have prosperously emerged as multi-tasking platforms on which single-cell studies can be facilitated by versatile integrations, multiplexing capacity, and maximized throughput (Fig. 1).15 The entire proteomic workflow using microfluidics systems can be carried out within several nanoliters of volume (e.g., one-pot protocol), reducing sample loss significantly. Despite this, however, SCP investigations using MS and microfluidics remain challenging because of the overall system integration, desired proteome coverage, and required sensitivity, e.g., to detect proteins ranging from high abundance to low copy numbers.16 As heterogeneous individual cells express unique proteome elicited from both intrinsic and extrinsic factors, their roles towards advances in clinical medicine and broader exploration of cell biology must be addressed by SCP techniques.17 Few reviews have discussed SCP developments with respect to microfluidics-based strategies,16,18 yet most of their focus were on either multi-omics approaches (with little emphasis on MS-based SCP)16,19–21 or fundamentals of microfluidics technologies.22,23 In this review, recent developments in microfluidics-based SCP, including their distinct features, advantages, limitations, applications, and future prospects, will be systematically discussed. We will introduce the capabilities of microfluidics and targeted analysis of single-cell proteins, and then describe in detail the state-of-the-art microfluidics methods for MS-based global SCP analysis, with particular attention on the miniaturization of sample preparation, enhanced proteome depth, and the biological information disclosed by these approaches. Lastly, we will discuss the challenges and opportunities for advancing the field of SCP.
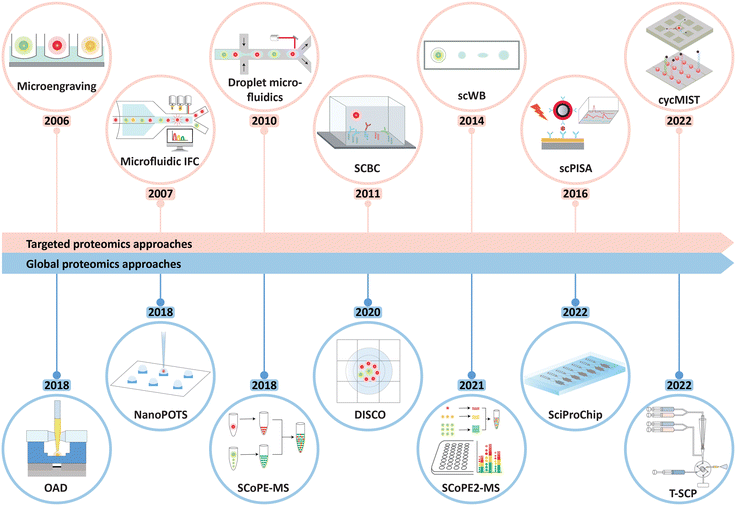 |
| Fig. 1 Timeline showing the development of major miniaturized technologies for single-cell functional proteomics in recent years, classified by the targeted and non-targeted (global) approaches. The abbreviations and acronyms are explained as follows: microfluidics IFC: microfluidics imaging flow cytometry, SCBC: single-cell barcode chip, scWB: single-cell western blotting, scPISA: single-cell plasmonic immunosandwich assay, cycMIST: single-cell cyclic multiplex in situ tagging, OAD: oil–air droplet, NanoPOTS: nanodroplet processing in one pot for trace samples, SCoPE-MS: single-cell proteomics by mass spectrometry, DISCO: digital microfluidic isolation of single cells for omics, SCoPE2-MS: single-cell proteomics by mass spectrometry 2, SciProChip: single-cell integrated proteomics chip, and T-SCP: true single-cell-derived proteomics method. | |
2. Developments of microfluidics for biomolecular analyses down to the single-cell level
Historically, the inception of microfluidics devices correlates with the start of microelectronics and semiconductor device fabrications.24 Since the demonstration of the first lab-on-a-chip device as a miniaturized chromatography system,25 various microfluidics systems, such as a total chemical analysis system (TAS),26 droplet microfluidics,27 digital microfluidics,28 and many others, have been developed to address respective research needs.29,30 Among notable developments, G. Whitesides et al. introduced the soft lithography micropatterning which allowed the use of an elastomeric material, poly(dimethylsiloxane) (PDMS), to fabricate pattern transfer elements using replica molding.31 Subsequently, S. Quake et al. applied the soft lithography to make multilayer microfluidics that enables custom fluid control via embedded valves and peristaltic pumps.32 Since then, microfluidic systems have shown unparalleled capabilities in the areas of bioengineering, biomedical science, and cell biology.33–35 To further achieve biomolecular sensitivity down to the single-cell level, reduction in reagent consumption and effective collection of cell contents play pivotal roles. In the following sections, a few selected efforts for cell processing, including cell isolation, manipulation, lysis, as well as microfluidics interfacing towards downstream instrumentations, are discussed.
2.1 Microfluidic devices for cell handling
Single-cell methods require isolation of individual cells prior to subsequent sample processing. As such, cell-handling techniques have been incorporated into various microfluidics platforms for biological studies.36 Typical single-cell methods, including droplets, micropillars, microwells, and integrated valve traps, are briefly discussed below. In droplet microfluidics, single cells are encapsulated, along with other reagents, by segmentation of an aqueous flow forming droplets within an immiscible carrier fluid (Fig. 2a(i)).37,38 A droplet size is controlled via tuning differential flow rates of the immiscible fluid, thus enabling the production of thousands of droplets within seconds.39,40 A micropillar array exploits morphological traps to confine movement of cells and thus capture them upon a cell suspension solution flowing through. The cell capturing usually takes tens of seconds, and micropillars can be chemically functionalized to improve efficiency and selectivity (Fig. 2a(ii)).41–44 Microwells for cell trapping leverage size-dependent trapping by molding wells into dimensions comparable to the size of targeted cells. The ease of open-wells fabrication offers adjustable scalability and functionality, which is ideal for a high-throughput workflow (Fig. 2a(iii)).45–47 Another approach utilizes pressure-controlled valves, coupled with circuits of microchannels, to trap cells.48 This method allows integration of a series of operations on the same chip, enabling automation and parallelization (Fig. 2a(iv)). In addition to the methods mentioned above, extra functions like acoustics (Fig. 2b(i)),49 optical tweezers (Fig. 2b(ii)),50 magnetics (Fig. 2b(iii)),51 and dielectrophoresis52,53 (Fig. 2b(iv)) have been applied to facilitate cell processing and sorting on demand. These techniques of cell sorting utilize external fields of force to deflect the flowing path of the targeted cells as they travel through the microchannels. Due to chip design flexibility, microfluidics-based single-cell methods offer ease of integration to downstream cellular manipulation and analyses, which is a feature most off-the-shelf cell sorting instruments cannot provide.
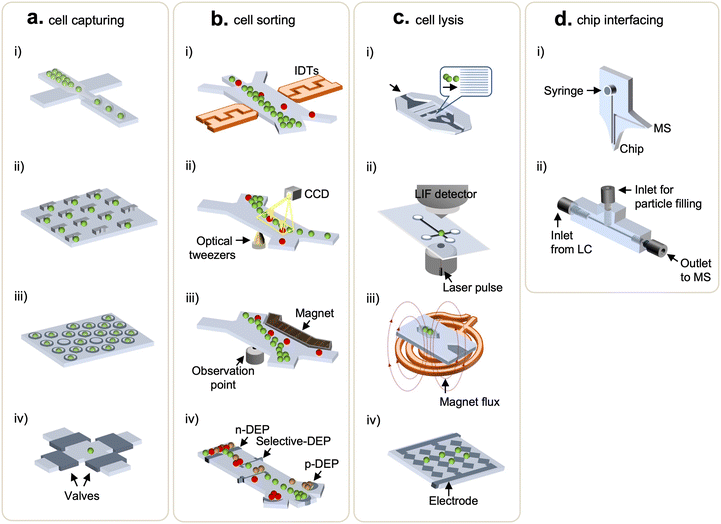 |
| Fig. 2 Microfluidic devices used for cell handling and chip interfacing. (a) Cell capturing methods: (i) droplets, (ii) U-shaped micro traps, (iii) microwells, and (iv) valve-based traps. (b) Cell sorting methods: (i) acoustic waves, (ii) optics, (iii) magnetics, and (iv) dielectrophoresis. (c) Cell lysis methods: (i) mechanical lysis, (ii) laser lysis, (iii) thermal lysis using wireless induction heating system, and (iv) electrical lysis. (d) Chip-interfacing methods: (i) integrated dual-probe interfacing microchip and (ii) 3D-printed multilayer interfacing microfluidic chip to LC-MS. | |
2.2 Microdevices for cell lysis
To maximize analytical sensitivity, cell lysis methods that enable effective extraction of intracellular content are of high importance. As such, diverse microfluidics were demonstrated for on-chip cell lysis.54 Chen et al. made a tri-layered structure (PMMA-silicon sheet-PDMS) to pretreat rat whole blood and extract PCR-amplifiable DNA from white blood cells in 50 min via detergent-based lysis.55 Irimia et al. coordinated thermopneumatic actuators with valves to mix single-cell suspension and minute lysing detergent (25 pL) in microchambers where cell lysis was enabled by drawing out the air along the virtual wall of liquid–air interfaces.56 Another chemical lysis working on pL-scale microwells was proposed by Sasuga et al.57 This method followed a typical microwell-based cell manipulation, in which the lysis reagent was injected through a flow cell (between a PDMS slab and glass coverslip bonded by double-sided tapes) to allow single-cell measurements of intracellular proteins. Meanwhile, to avoid sample clean-up steps, detergent-free lysis methods, such as via mechanical (Fig. 2c(i)), laser (Fig. 2c(ii)), magnetic (Fig. 2c(iii)), and electrical (Fig. 2c(iv)) perturbation, were developed using microfluidic devices.58,59 In terms of mechanical lysis, sharp nanostructured barbs rupture cells via shearing stress (Fig. 2c(i)), and Lee et al. added a dielectrophoretic force generated by multi-electrode pairs to position and lyse cells.60 Lai et al. shone a laser microbeam on cells to disrupt the membranes through the creation, expansion, and collapse of cavitation bubbles (Fig. 2c(ii)),61 enabling the lysis of specific target cells without affecting nearby ones.62,63 To realize thermal lysis, a temperature sensor and a micro-platinum heater were deposited on a silicon substrate.64 Also, wireless induction heaters were equipped to heat up extensive surfaces on which large volumes of samples can be processed at a time (Fig. 2c(iii).65) Note that the aforementioned microfluidics-facilitated cell lysis methods are not necessarily deployed for proteomic studies yet, nonetheless, they might ameliorate SCP analyses. Overall, microfluidics present controlled lysis capability using different modalities that are adjustable to the single-cell assays. More importantly, this feature can be readily integrated with existing functional modules within microfluidics to build streamlined workflows, avoiding sample losses (due to sample transfer) and hence improving sensitivity.
2.3 Microfluidics integration to downstream detection techniques
Successful efforts into coupling microfluidics with analytical techniques, such as matrix-assisted laser desorption/ionization-time of flight MS (MALDI-TOF MS) and liquid chromatography MS (LC-MS), improve the sensitivity of proteomics analysis for mass-limited samples.66–68 In this section, we focus on microfluidics integrations to LC-MS. Pan et al. developed a single-probe which was a miniaturized interfacing device directly coupled to a mass spectrometer.69 This method obviated the standard MS sample preparation and was compatible with the MS inlet, allowing real-time single-cell MS analysis. Furthermore, to address challenges in poorly-ionizable samples, the dual-probe microchip (Fig. 2d(i)) equipped with a 3-in-1 setting (i.e., sampling, solvent injection, and ESI) was introduced by Huang et al.70 An ultrahigh pressure sample separation is generally required in most MS-based proteomic analysis to improve a resolution of MS readouts and to reduce analysis time;71,72 however, this feature often brings on failure of microfluidic chips and leakage at connectors. To address it, Eeltink's group recently developed a microfluidic modulator chip to interface with high-pressure LC operation (up to 65 MPa).73 Likewise, Chen et al. also proposed a high pressure-resistant and 3D-printed multilayered microfluidic chip that could operate at a back pressure of 13.4 MPa in the LC-MS system.74 The interfacing chip had two inlets, where one linked to LC and the other for particle filling, and one outlet connecting to a mass spectrometer (Fig. 2d(ii)). Collectively, the endeavors to integrate functional modules within microfluidics and directly couple them with LC-MS systems have an attractive prospect for streamlined workflows, in order to significantly minimize sample losses and increase analytical sensitivity. The methods developed for single-cell proteomics are at the early stage with regard to the required sensitivity and deeper proteomic coverage. The ongoing progress of targeted and global proteomic technologies will be discussed in the next sections.
3. Microfluidics systems for targeted-based single cell proteomics analysis
In targeted proteomics techniques, proteins are analyzed by using the affinity probes with sensing labels (e.g., conjugated fluorophores, isotope tags, or quantum dots), which emit or transduce signals.16,75–78 To achieve the high sensitivity and facilitate targeted proteomics identification at the single-cell level, microfluidics have been increasingly used, primarily because they offer advantages including higher effective concentration during labeling, controllable cell manipulation, substantially reduced reagent consumption, and design flexibility to increase multiplexity and throughput.10,79,80 In the following, we review targeted-based SCP methods that are enabled by microfluidics systems, with particular focus on their developments in the last 5 years, as their earlier developments had been discussed in the previous reviews.81
3.1 Cytometry-based single-cell methods
Flow cytometry (FCM) is a standard profiling technology that offers enumeration and analysis of thousands of cells per second, using quantification of fluorescent emissions and scattered light of single cells.82 The use of FCM and fluorescent antibodies to profile proteins from cells has been the mainstream and widely used in the biological science.83 Nevertheless, FCM has limited intracellular spatial information, and it typically requires sample volumes of tens-to-hundreds of μL. When scaling a flow cytometer down to a microfluidic size, the bandwidth of a multi-parametric measurement is compromised, e.g., characterization of cell morphology at a high-throughput setting (>2000 cells per s) is challenging.84,85 To address it, imaging flow cytometry (IFC) hybridizes the advantage of high-throughput FCM and high-resolution imaging microscopy, enabling cellular information-rich detections (Fig. 3a).86–89 Further, IFC integrated with microfluidics allows a simple optical implementation and an exquisite control on cell manipulations.90–92 Note that, in most IFC microsystems, the trade-off between a fluidic throughput and an optical detection fuels a challenge in obtaining decent spatial resolution at high throughput. To address this, Holzner et al. introduced the multiparametric IFC using multi-color fluorescence and bright-field analysis that enabled high-resolution and high-throughput extraction of cellular morphological features such as accurate cell sizing and detection of cytoplasmic proteins in human cells.93 The approach combined stroboscopic illumination (i.e., a technique which generates blur-free images for objects moving at high linear velocities) with elasto-inertial 3D cell focusing to effectively control the flow velocity and cell positioning, respectively, thus achieving acquisition rates of 104 cells per s.
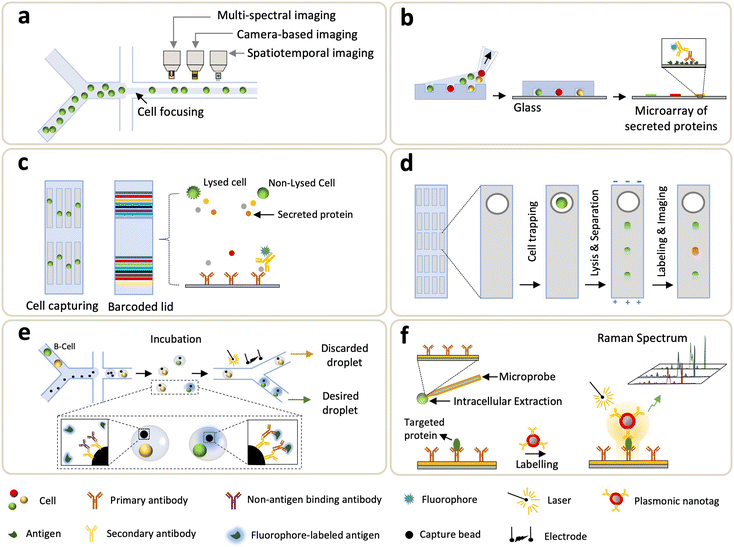 |
| Fig. 3 Single-cell technologies for targeted proteomics. (a) Imaging flow cytometry (IFC). (b) Microengraving. (c) Single-cell barcode chips (SCBCs). (d) Single-cell western blotting (scWB). (e) Droplet-based microfluidics. (f) Single-cell plasmonic immunosandwich assay (scPISA). | |
One of the main limitations of FCM roots in spectral overlaps of signals retrieved from fluorescent labels.94 To address this issue, Bandura et al. introduced the mass cytometry (cytometry by time of flight (CyTOF)) that synergized FCM and inductively coupled MS (ICP-MS), for simultaneous immuno-detections of ∼20 surface antigens in single cells.95 In principle, up to 100 parameters can be achieved from single-cell analysis, as ∼100 isotopes are available to label antibodies.96,97 Using this technique, cells were immunostained by antibodies labeled with custom multi-atomic tags, followed by submission to ICP-TOF-MS analyses. Based on surface markers, single-cell CyTOF is employed to identify heterogeneity in a human hematopoietic continuum.75,98 Porpiglia et al. used single-cell CyTOF for the concurrent analysis of cell surface markers and myogenic transformation factors, whereby they were able to resolve heterogeneity of myogenic compartments and characterize myogenic progressions ranging from stem to progenitor in skeletal muscle cells.99 To address limited phenotyping and resolutions in DNA barcodes, Wroblewska et al. developed a barcoding system that operated at the protein domain where a high-dimensional single-cell CRISPR screening was facilitated by CyTOF.100 With this method, more than 100 protein barcodes (Pro-codes) were generated through synthesizing modules and encoding triplet combinations of linear epitopes. The expressing vectors of Pro-codes transfected cells were subjected to subsequent CyTOF analyses, enabling the detection of 364 Pro-code populations by using just 14 antibodies. CyTOF is also used for the identification of clinically important immune reconstitutions.101 Recently, Stern et al. studied the evolving changes in immune reconstitution at different phases of human cytomegalovirus reactivation, which is a major complication after allogeneic hematopoietic stem cell transplantation.102 In this study, CyTOF maximized the data capacity extracted from limited sample sizes (in terms of a cell number and blood volume). In general, CyTOF is more advantageous to multiplexed analyses than FCM is. Since there is no overlap between detection channels, an absolute quantification is possible due to independent elemental responses to the sample matrix.
Meanwhile, FCM and CyTOF incur the loss of crucial spatial information when processing single-cell suspension for fluorescence. To address this, single-cell analysis of tissue samples, including (but not limited to) frozen tissue sections and formalin-fixed paraffin-embedded (FFPE) tissue, has been devised by expanding mass cytometry into imaging mass cytometry (IMC).103,104 IMC applies laser to ablate tissues or tissue sections which are stained with isotope-labeled antibodies, scanned and vaporized by pulsed laser, and transferred to ICP-MS detection prior to mass cytometry analysis. Jackson et al. recently utilized an IMC panel of 35 biomarkers to quantify clinical samples of breast cancer tissues.105 A tumor single-cell phenotype was identified by the expression of marker genes and the spatial features of individual cells from ∼8.55 × 105 cells in 381 images. Single-cell IMC provides spatially-resolved analysis which potentially sheds light on investigating phenotypic heterogeneity and its role in tumor architecture. Currently, the multiplexing capability of an IMC panel reaches to 40 biomarkers.106 To dig out the information undetectable in 2D IMC, Kuett et al. expanded the spatial resolution to 3D to allow the study of tumor invasion in TME.107 Gerdtsson et al. also demonstrated the integration of IMC with high definition single cell analysis (HD-SCA) assays that used immunofluorescence to characterize rare tumor cells from millions of leukocytes deposited on a glass slide.108 By using IMC, an in-depth phenotyping of a liquid biopsy was carried out over subcellular localization of markers within CTCs identified by HD-SCA.
3.2 Microengraving method
The microengraving method exploits sub-nanoliter wells to trap cells, and cell-secreted proteins are captured by a pretreated glass slide onto which affinity probes (either antibody or antigen) are coated. Love et al. pioneered microengraving biochips, where microwells were prepared by deposition of a cell suspension, allowing cells to settle down prior to removing an excess of untrapped cells (Fig. 3b).109 A glass slide engraved with antibodies or antigens was then taped on the confined single-cells, and the secreted proteins were interrogated by fluorescence immunoassay. Schubert et al. developed a single molecule array (SiMoA) platform that was capable of counting a low number of proteins from individual cells.110 SiMoA showcased that prostate-specific antigen expression varied among prostate cancer cells and shifted with genetic drift. Jia et al. explored the microengraving method to study longitudinal recall responses of nonhuman primates to protein-conjugate vaccine.111 Since the debut, continuous efforts to improve the sensitivity of microengraving methods have pushed the detection toward low abundant proteins from single cells. Li et al. introduced a signal-amplifying method in protein detection using a microwell platform where axial resolution of optics (i.e., field of depth) was increased, and the captured fluorescent tags at the side-wall and edge also contributed to signal.112 Compared to traditional microengraving methods that fluorescence solely emits from the flat surface, notably, the edge-enhancement microwell immunoassay increased the sensitivity by 6-fold. Another way to enhance fluorescence is to replace organic dyes with semiconductor quantum dots, which can be used as fluorescent reporters to detect secreted proteins from single cells.113,114 Altogether, microwell-based assays exhibit improved sensitivity than conventional enzyme-linked immunosorbent spot (ELISpot) assays do. In addition, this method offers advantages such as cell isolation adaptable to downstream analyses and enables kinetic studies in single-cell secretomes via multiple antibody-engraved glass slides.115,116
3.3 Barcode-based approaches
One of the first barcode-based methods for profiling single-cell proteins was the single-cell barcode chip (SCBC) developed by Heath's group.117 In this system, single cells were processed through microfluidics and isolated in microchambers where cell secretion or lysis is triggered, and the targeted analytes are captured and fluorescently detected by the “barcode array” where various DNA-encoded antibodies are coated on the predefined regions of a glass substrate (Fig. 3c). Using SCBCs, functional heterogeneity from more than ten secreted proteins, collected from macrophages and T cells, were characterized upon microenvironmental activation.117 Since its introduction, efforts are continuously made on SCBCs to improve the assay performance and extend their utility for biomedical applications.118–122 For instance, the utility of an SCBC uncovered mechanistic insights of drug tolerance via obtaining time-resolved characterizations of trajectories between the drug-naive and drug-tolerant states.118,120 Kravchenko-Balasha et al. exploited SCBCs to analyze the secreted proteins of isolated glioblastoma brain cancer cells.119 Later, the multi-omics workflow was integrated with SCBC: Xu et al. measured transcripts and lysed proteins,123 and Su et al. investigated cellular heterogeneity by both cytoplasmic proteins and metabolites associated with the selected cancer markers that functionally changed during an early drug response.122 More recently, Wang et al. introduced a single-cell multi-index secreted biomarker profiling approach using graphene oxide quantum dots (GOQD); the results showed that GOQD-assembled substrate led to a 2× higher density of antibody immobilization (compared to poly-L-lysine substrate) and lower background noise.124 Over the years, the SCBC has been armed with evolving microsystems, leading to an increased multiplexity (>42 proteins), improved throughput (>1000 cells), and higher fluorescent resolution.
Zhao et al. also introduced another barcode-enabled SCP strategy based on multiplexed in situ tagging (MIST), in which tens-to-hundreds of molecular targets in single cells can be analyzed.125,126 To achieve this, a monolayer of ssDNA-encoded microbeads is patterned on a glass slide, hybridized with complementary DNA–antibody conjugates and then enclosed by PDMS microwells containing single-cells. Through fluorescence sandwich ELISA, each kind of bead is then analyzed for detection of a particular protein across thousands of arrays. Subsequent development of this approach led to the single-cell cyclic multiplex in situ tagging (CycMIST) platform, in which UV-cleavable DNA-barcoded antibodies were used to enable multi-round labeling and multi-cycle decoding processes for achieving high throughput and sensitivity.127 The combined effect of high-density DNA arrays and small-sized microwells allowed the detection of ∼180 protein copies from a single cell.
3.4 Microfluidic capillary electrophoresis and single-cell western blotting
Capillary electrophoresis (CE) is an analytical method highlighted by its high separation efficiency and sensitive ion detection using a high electric field.128 Traditional CE suffers from long separation time, large sample consumption, and slow dissipation of joule heating. Microfluidic CE (MCE), on the other hand, is capable of addressing these limitations. MCE uses microchannels for electrophoretic separation of biomolecules in nanoliter-scale where the analysis time takes tens of seconds,129,130 and it can be deployed for separation and detection of intracellular content extracted from single cells.131 Huang et al. developed an MCE to detect low-copy-number (LCN) proteins from single cells by using single-molecule fluorescence measurements.132 Note that this method has relatively low throughput and limited multiplexing capacity. To improve throughput, Mainz et al. utilized a light-programmable and cell-permeable reporter to simultaneously analyze kinase activity from single cells.133 Li et al. also proposed a multicolor fluorescence detection-based microfluidic device for single-cell manipulation, which offered effective electrophoretic separation to realize concurrent detections of multiple small molecules.134 Overall, MCE leverages short distances and relatively short timescales to allow rapid completion of all steps taken in the traditional CE.
Another electrophoretic method used in microfluidics is the single-cell western blotting (scWB) developed by A. Herr group, where identification and quantification of specific proteins depend on their migration through a thin layer of gel matrix conjugated with antibody probing (Fig. 3d).135 The scWB combined western blots with an open-microwell array, thus increasing the assay throughput and enabling the reliable handling of ∼2000 neural stem cells. Note that earlier developments of scWB primarily focused on handling a larger amount, e.g., >1000, of input cells, and thus fell short of processing mass-limited input cells.135–137 To address it, Sinkala et al. integrated a rare-cell workflow with scWB to profile protein expression (plexity = 8) from single circulating tumor cell (CTCs).138 Specifically, cells collected from patient's blood were selected by the size and deformability, and the large nucleated cells were stained and identified, followed by a transfer to microwells. These cells were then lysed, and the associated proteins were electrophoretically injected into the gel, where polyacrylamide gel electrophoresis (PAGE), blotting, and immunoprobing steps were sequentially conducted. Recently, differential fractionation was integrated into electrophoretic separation: particularly, J. Vlassakis et al. introduced single-cell protein interaction fractionation through electrophoresis and immunoassay readout (SIFTER) for quantification of multimeric protein-complex in single cells.139 In this work, bidirectional electrophoresis was performed, and monomers and depolymerized protein complexes were fractionated and immobilized in gel regions around the microwells, followed by quantification using the in-gel immunoprobing. In addition to the advantages of high throughput and an affordable multiplexity, scWB increases the specificity of antibodies due to electrophoretic separation.
3.5 Droplet-based microfluidics
Droplet microfluidics enables quantification of secretomes released from single cells through a droplet encapsulation with fluorescent probes in nanoliter-volume droplets, overcoming the technical limitations in microfluidic FCM which is unable to detect secreted proteins.140–148 Droplet microfluidics was also employed in analyzing intracellular proteins in which one to three cells were lysed electrically prior to the formation of droplets.149 Ding et al. recently used co-flow droplet microfluidics to encapsulate primary cells (taken from tissues) together with capture microbeads and fluorophore-labeled antigens (Fig. 3e). During droplet incubation, a desired cell (e.g., B-cell) secreted primary antibody to mediate the binding between fluorophore-labeled antigen and a microbead. Subsequently the desired droplets, which were “labeled” by the fluorescent antigen, were sorted by fluorescent signals (Fig. 3e).150 Droplet microfluidic method yields high-throughput analysis; however, alignment of detection beads (on a focal plane) makes quantifications of the secreted antibodies challenging. To address this, immobilization of droplets was demonstrated by transforming the encapsulation of thermosetting oil into solid after droplet generation.151 Eyer et al., on the other hand, introduced a droplet-based microfluidic technology (DropMAP), eliciting single-cell analyses from droplets immobilized on the 2D array. DropMAP enables real-time measurements to quantify antibody-secreting rate, immunoaffinity, and associated kinetics.152,153 Regarding the operating principle of DropMAP, two aqueous phases are encapsulated in which one contained cells or calibration antibodies and the other contains reagents (fluorescent labels and 300-nm paramagnetic nanoparticles). The binding capacity of antibodies were increased due to the use of nanoparticles. When applying a magnetic field, the nanoparticles (designed to capture the secreted immunoglobulin) crosslinked, forming a micrometer-sized aggregate. To facilitate encapsulations of a single cell inside droplets and increase multiplexity, Khajvand et al. integrated droplet microfluidics with antibody barcodes.154 Arrays of ∼180 pL-sized chambers were used to boost single-cell capture efficiency (over 80% with a viability of >90%), and this method substantially improves on-chip trapping, isolation, and long-term incubation of single cells in stationary droplets. Droplet-based microfluidics, in general, boasts fast encapsulation, an increase of target concentration, a reduction in cross-contamination between samples, and capability to bridge genotype and phenotype.
3.6 Other developing methods
Towards unraveling the heterogeneity and functional states of cells, LCN proteins (existing at <1000 molecules per cell) play an essential role as they are involved in multiple cellular functions such as metabolism, signaling, and regulation of gene expression.155,156 Thus, methods that enable the in-depth analysis of LCN proteins is essential. However, probing these low-abundant proteins at a single-cell level poses a daunting challenge due to instrumental limitations (e.g., resolution, signal-to-noise ratio, and limit of detection (LoD)). To address these unmet needs, Liu et al. reported a single-cell plasmonic immunosandwich assay (scPISA) approach that integrated an in vivo immunoaffinitive extraction with the plasmon-enhanced Raman scattering (PERS) detection (Fig. 3f).157,158 ScPISA quantitatively assess the expression of LCN proteins in single (living) cells, providing high sensitivity (LoD = 7 molecules) and specificity (low cross-reactivity among probes) at a single-molecule level.158
Taken together, microfluidics-coupled targeted SCP methods discussed so far clearly showcased their potential in driving key biological understanding for both basic and translational research. Although targeted-based SCP methods have intrinsic limitations due to the necessity for affinity labeling, the synergistic coordination of microfluidics and ultrasensitive biosensing approaches may complement the field of LCN proteome in which MS-based global SCP falls short. As no one-size-fits-all instrument exists, the comprehensive mapping alternately retrieved from both targeted and global SCP shall be combined together to illustrate the unknown variation between genome and the associated proteome. In the next section, MS-based global SCP profiling technologies will be discussed.159
4. Mass spectrometry-based global single-cell proteomics profiling technologies
Substantial efforts have been devoted to increase the sensitivity of non-targeted proteomic workflows to reach the single-cell resolution with good coverage, robustness, and reliability. Compared to affinity-based methods, non-targeted proteomics profiling allows a global mapping of the cellular proteome and thus is ideal for discovery-oriented basic research and clinical investigations. Recent advances in various sample preparation methods, automated instrumentations, and LC-MS developments have enabled the quantification of >1000 proteins from a single cell using label-free approaches. In the following, we will discuss these exciting developments, which include a brief discussion about evolution of LC-MS systems for single-cell analysis, microfluidics-enabled upstream sample processing, and subsequent data acquisition and analysis workflows (Fig. 4).
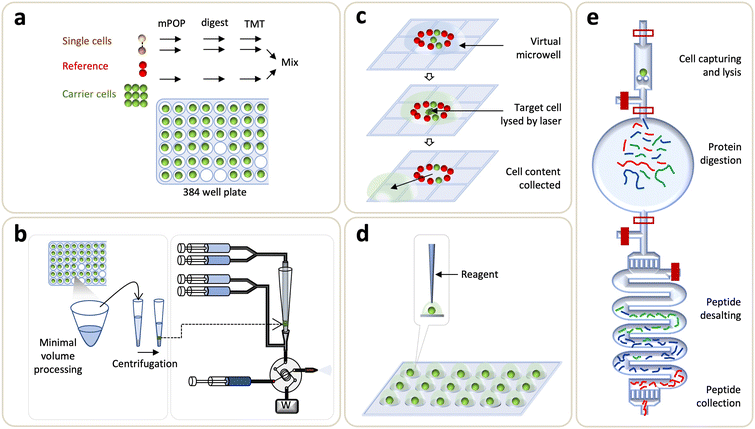 |
| Fig. 4 Single-cell technologies for non-targeted proteomics. (a) Single-cell proteomics by mass spectrometry (SCoPE-MS). (b) True single-cell-derived proteomics (T-SCP) method. (c) Digital microfluidic based chip. (d) Nanodroplet processing in one-pot (nanoPOTS) chip. (e) Single-cell integrated proteomic microfluidic chip (SciProChip). | |
4.1 Evolution of MS for microproteomics and single-cell proteomics
MS-based proteomics is undoubtedly the most comprehensive approach for large-scale analysis of the composition, structure, and dynamic alterations of a proteome, which can provide functional link and molecular mechanism of proteins to biology or diseases. It has been widely applied for the characterization of proteins, post-translational modifications and proteform dynamics for various samples such as cell lines, animal models, and human clinical specimens. Its implementations for protein biomarker discovery have inspired tremendous advancements in enhancing proteomics profiling sensitivity to overcome analytical challenges of clinical specimens, which are usually present in minute amounts such as a subset of primary immune and rare cells.160,161 While MS-based proteomics have identified >103–104 proteins varying from a microscale (microgram, >1000 cells) to bulk sample, MS-centric SCP was primarily hindered by the lack of miniaturized sample processing methods, efficient separation strategies, and sensitive MS instrumentation.8,10,162–164 With recent advances in miniaturized sample preparation together with improvements in LC separation and MS instrumentation, the pursuits of sensitive measurement down to a single cell have been rapidly expanding. These advances are discussed in the following sections with a detailed focus on microfluidics-based SCP analysis.
Miniaturized sample preparation.
In the conventional proteomics workflow, which usually requires a large amount of samples (>105 cells; 20 μg to 1 mg protein), to the small-scale microproteomics (<1000 cells; <1 μg protein),160,165,166 most endeavors focused on improving sample pretreatment in the microvials and custom tips to enhance the profiling sensitivity. For instance, Kulak et al. reported the in-StageTip (iST) method, where sample manipulation was undertaken in tips containing very small disks made of reversed phase beads.167 Manza et al. introduced the filter aided sample preparation (FASP), in which sodium dodecyl sulfate (SDS) and other contaminants that interfere the LC-MS/MS analysis were removed by molecular weight cutoff filtration device.168 Likewise, Hughes et al. developed the single-pot solid-phase-enhanced sample preparation (SP3) that used surface functionalized paramagnetic beads for peptide desalting.169 These techniques minimized sample transfer processes, enabling enhanced analysis down to a few hundreds of cells with proteomics coverage in a range of 3500–4500.170 Meanwhile, Chen et al. reported an integrated proteome analysis device (iPAD) for probing 100 cells (iPAD-100), whereby live cells were injected into the device for subsequent proteomic processing and analysis.171 The device consisted of an inlet needle dipped into a cell suspension, a 10-port valve controlling the process of sample treatment, a micro syringe for delivering samples, a capillary loop (40 cm long, 100 μm inner diameter (i.d.)) for protein digestion, and a C8 column for desalting. The iPAD-100 achieved identification of 635 proteins from 100 cells. Later on, the device was upgraded as an integrated system (iPAD-1) for SCP profiling.172 With a key component of the 4.5-fold smaller capillary (22 μm in i.d.) for input of single-cells, cell lysis and protein digestion were accomplished within 2 nL, and the results showed significantly reduced sample loss. Overall, the iPAD-1 achieved identification of an average of 180 proteins from a single HeLa cell.
Advancement in LC instrumentation.
Significant advancements in enhancing chromatographic separation and resolution greatly contributed to the ultrasensitive proteome analysis. Miniaturization of LC i.d. and a lower flow rate have also been known to enhance the separation and hence substantially increase the ion delivery to a mass spectrometer and enhance the detection sensitivity.163 Various narrow-bore columns have been reported, including reduction in an i.d. ranging from 75 μm to 30 μm at a flow rate of 50 nL min−1 to enhance signal intensity by 3-fold,173 ultranarrow-bore-packed column with a 20 μm i.d. at a flow rate of 20 nL min−1 to achieve ∼800 protein coverage from single cell,163 and 10- to 100-fold improvement in sensitivity using picoLC system (2 μm i.d. and 800 pl min−1) compared with a 30 μm-i.d. column.174 Several groups reported microfluidic pillar array columns (μPAC) for low input proteomics, which are composed of narrower pillars with 2.5 μm of interpillar distance packed with highly-ordered stationary C18 beads operating at 250 nL min−1 of a flow rate, demonstrating consistent quantification of >1000 proteins from a single cell.8,72,175
Advancement in mass spectrometry instrumentation.
The rapid technological advancements in sensitivity, speed, and resolution of recent generations of MS instrumentation have enhanced the sensitivity ranging from microproteomics to SCP. To date, the majority of SCP studies have employed various generations of orbitrap-based mass spectrometers due to the high resolution, improved ion transmission, duty cycle, and detector sensitivity.166 The recently released timsTOF instrument equipped with a trapped ion mobility device on time-of-flight (TOF) MS adds another dimension of gas-phase ion fractionation, which enhances sensitivity in proteome coverage by enabling efficient data acquisition of peptide ions from single cells.176,177 Ion utilization was further improved by the parallel accumulation–serial fragmentation of precursors either in the data-dependent (ddaPASEF) or data-independent (diaPASEF) mode. The addition of field asymmetric ion mobility spectrometry (FAIMS), which filters out the singly charged ions prior to MS analysis, also increased over twofold protein identifications.8,164 Additionally, instrument parameters play critical roles to detect extremely low-abundant proteins from low-input samples. Parameters such as increasing maximum ion input and long injection time have been optimized to enhance ion signals. Multiple studies have demonstrated that optimized acquisition methods (such as DIA) led to increased ion utilization which further resulted in enhanced coverage for SCP.178,179 Together, these improvements drastically enhanced sensitivity resulting in increased protein identification and quantification from single-cell samples.
SCoPE-MS.
Slavov's group pioneered a multiplexed-based single-cell proteomics by mass spectrometry (SCoPE-MS) that achieved identification of >1000 proteins.180 This method has integrated several key components. To minimize the cleanup-related losses, chemical lysis was replaced with mechanical lysis using focused acoustic sonication. Secondly, isobaric tandem mass tags (TMTs) were applied to add a carrier channel with much higher protein abundance, such as 200 carrier cells in one TMT channel to boost the low abundant peptide signals at the MS1 levels, and then were mixed for subsequent LC-MS/MS analysis. Since carrier cells were more likely source-experiencing sample loss and can increase the detectability of peptides, SCoPE-MS significantly enhanced the proteome coverage to >1000 proteins from single cells.178,180,181 To further improve the protein extraction step, the updated version (SCoPE2) adopted the minimal proteomic sample preparation (mPOP) method, and the sonication step for cell lysis was replaced by a freeze-heat cycle in pure water.7 The mPOP employed 384-well plates, PCR thermocyclers, and liquid dispensers to automate the sample preparation and increase throughput. Also, SCoPE2 employed a shorter LC gradient (60 min) and reduced lysis volume from 10 to 1 μL (i.e., a 10-fold reduction). As such, the method was able to quantify ∼1000 proteins from a single mammalian cell, and over 3000 proteins were detected from 1490 single monocytes and macrophages cells by using 11-plex and 16-plex TMTs (Fig. 4a). Overall, these methods brought a new perspective to enhance the sensitivity of SCP profiling. However, like many label-based methods, SCoPE-MS and SCoPE2 reported the challenges accompanied with TMT labeling, including the inherent interference presented from co-isolated precursors of the abundant carrier cells and other non-targeted peptides,182 as well as the compressed ion ratios that reduce quantification accuracy.183
SCeptre.
Following the developments of SCoPE, Schoof et al. reported a similar approach named as single cell proteomics readout of expression (SCeptre).8 This workflow collectively offered (1) higher throughput, (2) improved quantitative accuracy, (3) integrated cell-sorting data, and (4) computational workflow.8 In this method, the FACS-sorted cells were dispensed into individual wells of a 384-well plate. The individual cells were lysed via snap-freezing and heating, followed by overnight trypsin proteolysis. The sensitivity is boosted by the 16-plex TMT labeling and analysis by a FAIMS-coupled orbitrap MS. In this study, the 14 TMT-labeled single cells were pooled with 200-cell-equivalent booster aliquot, thus 24 samples could be retrieved per 384-well plate, representing high-throughput analysis of 336 single-cells per plate. Because large-scale multiplexed single-cell analysis poses a challenge for data analysis, the computational workflow based on python was also developed to integrate cell sorting and downstream data analysis. Altogether, SCeptre workflow allowed analysis of 112 cells per day and quantifies nearly 1000 proteins per cell. This approach demonstrated fast and high throughput sample preparation as well as the ability to explore cellular heterogeneity in primary leukaemia.8
T-SCP.
Recently, Mann and coworkers also introduced a sensitive LC-MS based SCP workflow named true single-cell-derived proteomics (T-SCP, Fig. 4b).177 This workflow consisted of major features including (1) miniaturized sample preparation, (2) very low flow-rate LC (100 nL min−1), and (3) an updated timsTOF MS, resulting in >10-fold enhanced sensitivity. In principle, the sample preparation starts with sorting individual cells into a 384-well plate which contains 1 μL of lysis buffer, and lysis is facilitated by heating at 72 °C. Next, extracted proteins were digested and the resultant peptides were desalted with disposable trap columns. The clean peptides were loaded to LC coupled to timsTOF MS. The results showed that ∼850–1900 proteins (with match-between-runs; MBR) were identified from 1–6 Hela cells using the ddaPASEF mode,177 while the diaPASEF mode was able to identify 2083 proteins from a single-cell.
Overall, the SCoPE-MS, SCP-SCeptre, and T-SCP demonstrated the leaping advancements in improved sensitivity, quantification accuracy, and throughput for single-cell analysis in both conventional tubes and multi-well plates. The potential sample loss from large surface contact may leave room for further improvement from newer generations of instrumentation. As a consequence, microfluidics has emerged as a newly prevailing candidate to address these limitations.
4.2 Digital microfluidics chips: DISCO and DMF-SP3
Digital microfluidics (DMF) devices are another version of the stationery droplet microfluidics approach, in which a discrete microdroplet (100 nL–10 μL) is manipulated on an open array of adjacent electrodes driven by electrical potentials. Pioneering works done by the Wheeler's group has established DMF as a versatile platform for various cell-based assays,184 sample preparation for MS,185–187 as well as proteomic sample preparation including lysis, reduction, alkylation, and digestion.188 Later on, on-chip sample clean-up step was demonstrated by solid-phase extraction on porous polymer monoliths.189 To address the inherent limitations in removing contaminant detergents, Leipert et al. reported a DMF platform, based on the single-pot and solid-phase-enhanced sample preparation (DMF-SP3), for direct peptide cleanup on a magnetic bead.190 The DMF-SP3 method enabled the identification of 1200 and 2500 proteins from 100 and 500 Jurkat T cells, respectively.190 Subsequently, the group enhanced the sensitivity of DMF-SP3 using a FAIMS and ion fractionation to identify ∼5000 proteins from single nematodes.191 Combining with isobaric labeling, a recent extension of DMF-SP3 has reported an average of 1815 protein groups from 75 Jurkat T cells.192
Recently, Wheeler's group developed a new DMF method, named digital microfluidic isolation of single cells for omics (DISCO), along with image-based data analysis (Fig. 4c).80 In DISCO, the cells of interest, which were selected by manual operation using the custom-controlled software or the automated operation driven by artificial intelligence (AI), were isolated on a device from the diverse and heterogeneous population ranging from ∼100 to 300 cells. The selected cells were then lysed by focused high-energy laser pulse to skip the peptide desalting step. This approach identified an average of 88, 427, and 699 proteins from 0 (i.e., a control), 1, and 5 U87 cells, respectively.
4.3 Droplet microchips: OAD and nanoPOTS
In this category of microfluidics approaches, stationary nanoliter reactors were used to perform the one-pot protocol for proteomic sample preparation in order to avoid sample transfer, reduce contact surface area, and hence minimize sample loss. Li et al. developed a disposable nanoliter scale oil–air droplet (OAD) chip to carry out multi-step sample treatment and injection for SCP analysis. The chip had four layers: the oil layer where evaporation was substantially minimized, the isolation layer where oil was separated from a droplet, the droplet layer where sample preparation took place, and the chip positioning layers where all layers were coaxially aligned and assembled. Droplet manipulation was controlled by the tapered tip-fused silica capillary probe connecting to a syringe pump. Both the syringe and capillary probe were filled with water and segmented from the aspirated solution by fluorinated oil. To ease the coaxial alignment between a capillary probe and an OAD, a self-aligning monolithic (SAM) device was used. In the first step, a cell suspension was deposited on the droplet layer and the proteomic workflow was performed at a processing volume of ∼550 nL. A minimum sample loss was achieved through a direct coupling of the miniaturized droplet microreactor and SAM via the LC column. To benchmark the method, 100, 50, 10, and 1 HeLa cells were profiled for protein identification with average numbers of 1063, 418, 108, and 36, respectively; while 355 proteins were identified from a single mouse oocyte.193
On the other hand, Kelly's group introduced an open-well based platform, named nanodroplet processing in one pot for trace samples (nanoPOTS), for SCP profiling (Fig. 4d).162 An array of 3 × 7 spots was formed on a glass slide using photolithography and wet etching techniques, with elevated hydrophilic nanodroplet reaction vessels (i.e., nanowells) on a hydrophobic surface. In this platform, serially diluted cell solutions were deposited onto nanowells and the cell numbers were confirmed by microscopy. A custom-made liquid handling robotic system was used to dispense cells and reagents to a single droplet for proteomic sample preparation. A humidified chamber was constructed to reduce sample evaporation during the operation. Fused silica capillary was used to collect the digested peptides released from the nanowell, and the desalting was performed by further connection to SPE column with PEEK union, followed by LC-MS/MS analysis. The platform achieved proteome coverage of >3000 proteins collected from 10–100 HeLa cells using the MBR algorithm. The platform was further enhanced by improving both the sample preparation and LC-MS sections. The earliest utilization of nanoPOTS for SCP identified an average of 670 protein groups from single HeLa cells.194 To improve the throughput and robustness, a nanoPOTS autosampler was developed to fully automate the sample injection transferring from nanoPOTS to LC-MS system.195 The combination of nanoPOTS with ultralow-flow nanoLC and FAIMS further showed that the proteome coverage of a single HeLa cell could be enhanced to identify >1000 proteins.164 Recently, the new version of a nested nanoPOTS (N2) chip adopted further miniaturization of total sample processing volume varying from 200 nL to 30 nL, as well as incorporated isobaric labeling workflows to enhance throughput ranging from 27 to 240 samples per assay and to improve the sample recovery.9 The N2 platform enabled an average identification of ∼1500 proteins from mammalian cells across a series of single cells.
4.4 Integrated microfluidics device: SciProChip-DIA
Multilayer microfluidic devices have been employed in various research domains including life sciences and systems biology due to their precise fluid manipulation and ability to perform complex protocols.13,32,33 To explore its utility in SCP, Gebreyesus et al. recently developed an integrated proteomics chip (iProChip, for processing 1–100 cells) and its extended version (SciProChip, for processing at the single-cell level), coupled with data-independent acquisition (DIA) MS, as all-in-one stations featuring the streamlined and multiplexed workflow used in nanoproteomics and SCP.10 The chips have two layers: the flow-layer and the control-layer. A flow-layer holds the entire proteomic workflow and coheres with a control-layer, allowing automated and accurate fluid manipulation via microvalves and the custom-designed program (Fig. 4e). Basically, the microfluidics used in iProChip and SciProChip is composed of three main functional blocks: (1) cell-capture and lysis chamber, (2) protein digestion vessel, and (3) C18 beads-packed peptide desalting module. The cell chambers were composed of wedge-shaped pairs of pillars with 5 μm spacing, whose numbers were determined according to the desired sample sizes of 10–100 cells in iProChip and a single cell in SciProChip. The flow of a cell solution of appropriate density enabled the size-based capturing of mammalian cells within a few seconds, accompanied by real time microscopy monitoring. The protein digestion vessel was designed to have a total processing volume of ∼300 nL (iProChip) and ∼78 nL (SciProChip), assisting the digestion of proteins into peptides at the nanoliter scale. The desalting module was fabricated by packing a serpentine channel with C18 beads to facilitate an efficient on-chip peptide clean-up. As such, the streamlined workflow enables an all-in-one operation, obviating the need to externally perform cell sorting and peptide desalting.
Data-dependent acquisition (DDA) is one of the most common data acquisition approaches, where most intense peptide ions are selected serially for fragmentation. Despite its wide acceptance, the semi-stochastic nature of DDA leads to inherent limitations of missing values compromising the proteome coverage depth. Recently, DIA MS has emerged as a powerful alternative technique allowing to record peptide ions in parallel fashion in a series of small isolation windows over multiple scans, thereby achieving enhanced and reproducible proteome identification. Compared to previous SCP reports, to our knowledge, DIA MS was firstly introduced in this study. The application of DIA MS using custom-made spectra libraries constructed by samples of comparable cell numbers significantly enhanced 2.3-fold more protein identifications for mass limited samples when compared to DDA mode. At single cell level, coupling SciProChip with DIA MS achieved an average identification of ∼1500 protein groups, highlighting one of the most sensitive proteomic coverages from a single mammalian cell. Such proteomics coverage allowed the detection of important yet low abundant lung cancer druggable target proteins (e.g., EGFR, TP53, CDK1) at different sample amounts. Besides, the platform achieved the identification coverage of kinase proteins and key immune-related surface receptors. This method offers advantages of completely streamlined and simultaneous single-cell analysis in semi-automated fashion, within a single chip. The method efficiently avoided sample losses related to sample transfers and ease of operation. However, the platform still depends upon manual transfer of resulting peptides to the LC-MS/MS, incurring sample loss. The future effort is required to interface the chip directly to the LC-MS/MS. In addition, the proposed design considerations of the microfluidic system must address the concern of relatively low throughputs to strengthen the merits of the method.
4.5 Other recent developments: proteoCHIP, WinO, and PiSPA
Very recently, a new automated and miniaturized device, called proteoCHIP, in combination with cellenOne single-cell isolation instrument was introduced for multiplexed SCP.196 The proteoCHIP is made up of PTFE-based slides and consists of two parts. The nanowell module contains 12 sets, and each set has 16 nanowells each designed to process 192 single-cells simultaneously. Another part is the funnel module designed for sample pooling, and it is directly interfaced with an HPLC autosampler. The sample preparation starts with dispensing a 40 nL cocktail buffer (containing lysis buffer and enzymes) into each nanowell using the cellenOne platform. Then, image-based sorted cells are deposited into nanowell, and the chip is incubated at 50° for two hours to enable cell lysis and protein digestion. The reaction is performed at elevated humidity by using a layer of hexadecane to prevent sample evaporation. After TMT labeling, the samples are pooled via centrifugation and directly injected to LC-MS/MS. The approach identified >2500 proteins across 170 multiplexed single cells from HeLa and HEK-293 cells. Utilizing a TMT-based multiplexed workflow, the proteoCHIP platform allows profiling up to 572 single cells per experiment. The developed platform is accessible via commercial products.196
Recently, Masuda and colleagues introduced a water droplet-in-oil digestion (WinO) approach.197 This method uses immiscibility of solvents (water and oil) to reduce sample loss during sample preparation and to increase protein recovery. Briefly, an extraction buffer containing 1 μL of water droplet is prepared with phase transfer surfactants and hydrophobic carboxyl-coated nanomagnetic beads, and then cells are loaded in ethyl acetate to mix with the extraction buffer. After adding 1 μL of reduction and alkylation buffer, proteins are digested overnight. The resulting peptides are TMT-labeled and pooled together, and surfactants were removed by using phase transfer approach. Finally, the peptides were purified by using the stop-and-go-extraction tips prior to injection into the LC-MS/MS. This approach identified nearly 500 proteins from single cells.
The abovementioned SCP methods are capable of identifying 500 to 1500 proteins from individual single cells. A very recent SCP workflow called pick-up single cell proteomic analysis (PiSPA) achieved deep identification of 3000 proteins in 3 different kinds of single tumor cells. This method employed a microfluidic liquid handling robot for sorting and dispensing single cells into a LC-MS/MS system compatible vial. Additionally, this platform was operated to automate the pickup of sample preparation regents, in order to subsequently achieve cell lysis, protein reduction, alkylation, and digestion, as well as enzyme deactivation. The sample vials were then placed in the autosampler of the LC system and peptides were analyzed by timsTOF MS. The PiSPA platform demonstrated the highest sensitivity with significant improvement in proteome depth over current SCP methods.198
5. Applications of SCP in biological and clinical research
5.1 Applications of targeted single-cell protein assays
In this section, we present the biological and clinical applications of various microfluidics-based targeted SCP assays (Fig. 5 and Table 1). Due to unique capabilities of microfluidics, their implementation for targeted SCP has facilitated various cell biology studies and clinical research.199 For example, CyTOF is among one of the most widely used approaches for targeted SCP analysis,200 providing applications to explore cell phenotype in distinct brain cells (based on signaling markers and cytokines),201 immune cell differentiation,102,200,202 cell heterogeneity based on surface markers,75,99 and cancer-related signaling.203 CyTOF in combination with cell imaging has also been applied to explore the subcellular distribution of proteins in individual cells within a tissue or during cellular processes, advancing our understanding of immune cell function for immunotherapeutic applications.204,205 Recently, Neuperger and coworkers used single-cell CyTOF to investigate heterogeneity in non-small cell lung cancer cell (NSCLC) lines and intratumor heterogeneity in lung adenocarcinoma based on expression pattern of 13 proteins.206 Likewise, SCBC approach is used to measure multiplexed protein abundances (determined by copy numbers produced by the cell (or cells)) from a wide variety of secreted, cytoplasmic or membrane proteins. By carefully selecting and monitoring expression of specific protein markers, SCBCs can be a tool to investigate a multitude of biological systems. For example, heterogeneity (cell–cell variation) can be resolved by monitoring the differential expression of critical phenotypic markers, transcription factors, and signaling effectors which are involved in different signaling pathways. SCBC approach has also been employed to explore cellular interactions and communications by measuring secretory proteins released by cells incubated in the close proximity. This approach is used to understand how cells exert their activities on each other at varying separations and how signaling proteins influence neighboring cells.119,121,207 SCBC analysis for selected protein markers has indeed emerged as a promising tool to study functional heterogeneity,117 signaling pathways,208 cell–cell interaction,207 cellular communication,119 immune cell responses,209 and CTCs profiling.210 Su et al. applied SCBC chip to monitor 18 proteins including phenotypic markers, transcription factors, signaling effectors in single melanoma cell to explore drug-induced signaling dynamics.121 This study unveiled phenotypic transition of melanoma cells in response to the treatment of BRAF inhibitors, which activated alternate pathways including MEK/ERK and NF-κB that further accelerated tumor progression and drug-resistant phenotype. Furthermore, Ullal and colleagues used SCBC to explore the inter- and intra-tumor heterogeneity in lung adenocarcinoma patients with 90 target markers which were carefully selected to cover the hallmark pathways (e.g., DNA damage, cell death) and diagnostic markers employed in clinic.211
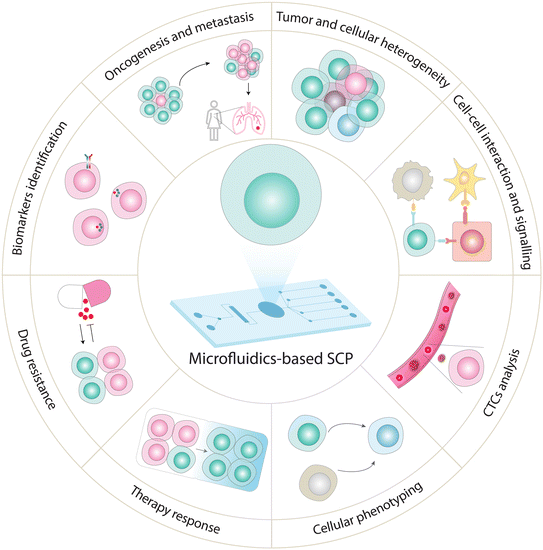 |
| Fig. 5 Emerging applications of SCP analysis using targeted and global approaches. SCP analysis has the potential to measure heterogeneity in different cell lines and dissect tissue heterogeneity,7,180,231,235,237 explore cancer development and progression,177 identify biomarkers,10,238 unveil cellular responses to drugs,227 investigate mechanistic insights into drug resistance,108,205,214 profile CTCs (non-invasive diagnosis and real-time cancer monitoring),231 discover functional proteins to track cellular phenotypes (cell types and cell-state),7,167 and analyze cellular signaling events.9,10 | |
Table 1 Overview of microfluidic-based targeted and global SCP methods
Technique |
Detection method |
Proteins detected per cell |
Throughput |
Advantages |
Disadvantages |
Applications |
Ref. |
Cytometry-based single-cell methods |
Fluorescence detection |
20–30 |
104 |
Suitability for (1) analysis of cell surface and intracellular proteins (2) sorting cells |
Limited multiplexing capacity; spatial & spectral overlapping; inability to detect secreted proteins |
Diagnostic assessment; characterization & quantification of phenotypes |
88
|
93
|
Rare-earth-metal-labelled antibody detection |
40–100 |
100 |
No overlap among detection channels; suitability for absolute proteomic quantification; chemically stable multi-atomic tags |
Inability to analyze live cells; large sample loss |
Cell biology studies; immunotherapeutics; surface phenotyping |
95
|
99
|
101
|
201
|
202
|
Microengraving |
Fluorescence detection |
10–20 |
100–104 |
High throughput and sensitivity; recovered cells for downstream analysis; kinetic studies allowed |
Low proteome coverage |
Immunology; cell–cell interactions |
109
|
110
|
111
|
Barcode-based approaches |
DNA encoded antibody arrays |
40–180 |
100–104 |
High-throughput & sensitive analysis; high multiplexity; suitability for both secreted & intracellular protein analysis |
Necessity of big libraries used for barcoded antibodies |
Drug-induced signaling dynamics study; inter & intra tumor heterogeneity; functional heterogeneity; cellular communication |
119
|
121
|
208
|
211
|
scWB |
UV-initiated blotting & antibody probing |
10 |
2–2000 |
Rapid analysis; low cost; integration capability; high specificity |
Low proteome coverage |
Cellular heterogeneity; cell differentiation; monitoring of drug response; prognosis prediction |
135
|
136
|
137
|
138
|
scPISA |
PERS |
6 |
1 |
Simple & rapid method; minimal invasion |
Low throughput; low proteome coverage |
Protein–protein interactions; cell signaling |
157
|
Droplet microfluidics |
Fluorescence detection |
10 |
104 |
Sensitive & rapid detection; increased target concentration |
Low proteome coverage |
Surface receptors detection, isolation & identification of CTCs from whole-blood samples |
149
|
150
|
152
|
213
|
SCoPE & SCeptre |
DDA MS |
∼1000 |
1500–3000 |
High throughput; low cost; scalability |
TMT labelling; no individual cell measured; limited quantification accuracy |
Cellular heterogeneity; biomarker discovery; cell phenotyping |
7
|
8
|
180
|
227
|
T-SCP |
DIA MS |
∼2000 |
384 |
Sample preparation integrated with chromatography; ion mobility separation of peptides |
Limited scalability |
Cancer development & progression; cell signaling; heterogeneity; spatial proteomics |
177
|
Digital microfluidics |
DDA MS |
∼427 |
1 |
Multi-omics analysis; image-based data analysis from single cells; selective cell lysis |
Low throughput |
Cellular processes analysis |
80
|
Oil–air droplet |
DDA MS |
∼36 |
1 |
Minimized sample loss, high-efficiency injection of samples |
Low throughput; stochastic cell sorting |
Cell heterogeneity; cell signaling |
193
|
nanoPOTS & N2 chip |
DDA MS |
∼1000–1500 |
27–243 |
Minimal sample loss; flexibility of adds-on (e.g., LCD/FACS) |
Specialized tools; intensive training for operation |
Cell signaling; heterogeneity; spatial proteomics; biomarker discovery |
162
|
164
|
237
|
SciProChip |
DIA MS |
∼1500 |
20 |
Streamlined & multiplexed workflow; semi-automated operation; low sample contamination |
Low throughput; necessity of a control system; intensive training for operation |
Cell signaling; biomarkers detection |
10
|
Using scWB system, Sinkala et al. investigated the heterogeneity of CTCs (based on 12 protein expressions) derived from ER+ breast cancer patients.138 They observed three CTCs' phenotypes, a biophysical phenotype, and two CTCs' subpopulations based on the GAPDH expression level. This shows that protein profiling of CTCs is important to understand phenotypic characteristics of tumor origins and potential metastatic lesions. This scWB approach also demonstrated its ability to interrogate the cellular heterogeneity and monitor single-cell differentiation of neural stem cells.135,136 The applications of scWB have been reviewed in detail by a recently published work.212 On the other hand, Joensson and colleagues used droplet microfluidics to detect surface receptors such as CD19, CCR5, and EpCAM markers from single-cell, diagnosing lymphoma and prostate cancers.213,214 Similarly, Jurkat and Raji cells were differentiated at an individual cell level by the expression pattern of CD3 and C19 markers.215 Furthermore, droplet microfluidics were also applied to isolate and identify CTCs from whole-blood based on detection of biomarker proteins such as EpCAM and Her-2 from breast cancer patients.216 The application of droplet microfluidics on the measurement of receptor tyrosine kinase activity in lung cancer cells also revealed cellular heterogeneity against the targeted therapy gefitinib, a tyrosine kinase inhibitor.217 The results showed varying levels of drug resistance among individual cells upon treatment with gefitinib and potential to screen drug effects in single cells.
Reza et al. used surface-enhanced Raman spectroscopy (SERS)-based microfluidic assay to monitor 3 surface markers (MCSP, MCAM, and LNGFR) in melanoma patient-derived CTCs.218 They found differences in the expression pattern of receptor proteins before the initiation and during the cancer therapy. Patients with metastatic melanoma revealed a fraction of CTCs that continuously express elevated levels of receptors during and after therapy, indicating the presence of resistant CTCs that evolve over time and cause disease progression. Meanwhile, using CycMIST platform, highly multiplexed profiling has been achieved for 182 proteins, including transcription factors, surface markers, and signaling proteins from single mouse brain cells derived from the Alzheimer's disease (AD) model or wild-type mice.127 The results revealed differentially expressed AD-pathology related proteins, such as apoptotic and certain glial activation markers, between the AD and wild-type mice, confirming the results from previous studies in brains of AD patients.127
5.2 Applications of MS-based global single-cell proteomics
Recent mass-spectrometry-based SCP studies have shown increasing proteomic coverage in the range of 1000–2000 proteins.7,9,10,177 Such global proteomic profiling started to interrogate cellular heterogeneity, i.e., cell-to-cell differences in the protein composition abundances, and to monitor alterations in signaling pathways. This information could be used to examine how different cells respond to therapy or to identify small groups of drug-resistance cells within a tumor. The current SCP methods have the potential to enable further understanding of biological systems or therapeutic development. Some selected applications are summarized below (Table 1).
Cell signaling.
Single-cell proteomic profiling enables comprehensive mapping of signaling pathways, which can provide insights into the overall architecture of “signaling signatures” under a specific cellular or pathological condition and aid in understanding the molecular mechanism of tumorigenesis, and identifies changes in signaling events in response to drug. Recently, Gebreyesus et al. demonstrated the mapping of NSCLC and B-cell receptor (BCR) signaling pathways in single PC9 and MEC-1 cells by the iProChip-DIA approach.10 The study identified several important drug targets and crucial immune cell-related surface receptors. This sensitive profiling of these important pathways at the single-cell level and identification of key drivers (CD19, CD21, CD22, and CD81) of cancer immune response may allow exploration of signaling features in cancer cells and immune responses.10 The initial version of the nanoPOTS platform demonstrated the clinical application of the device for characterization of single human pancreatic islet sections (10 μm in thickness, equivalent to 100 cells) from diabetic patients. They observed the differential expression pattern of several proteins associated with type I diabetes (T1D) pathology.162 Importantly, the drastic down-regulated abundances of pancreatic βcell-specific proteins, including insulin and PCSK1, revealed the destruction of β-cells in T1D pancreatic islets, while increased expression of β-2-microglobulin and HLA class-I antigen defined features of type I diabetes. The versatility of this platform was also demonstrated to assess changes in differential proteomic expression during hair cell development.219 Recently, the updated version of nanoPOTs, N2, was used to uncover cellular heterogeneity and enabled the classification of 3 types of cells (epithelial cells, monocyte/macrophage-like cells, and colon cancer cells). Additionally, nanoPOTS platform combined with subcellular localization information was used to directly identify cell-type-specific surface markers to explore tissue substructures and cellular microenvironments.9
Protein–protein interactions.
Protein–protein interactions (PPIs) and protein complex formations are important in cellular processes, and they are often elucidated by measuring the abundances of relevant proteins (covariation) within the signaling pathways.208,220 This type of measurement allows the study of perturbed PPIs using drug-treatment or mutation studies.221,222 While recent proteomics studies have attempted to explore regulatory PPIs and mapping of dynamic networks of protein complexes at the microproteomics scale,7,10 characterization of such interactions at the single-cell level is still at its infancy. For example, Furlan and colleagues used a microfluidic affinity purification coupled MS (AP-MS) to capture protein–protein interactions (>200 types) from 1.2 × 104 input cells, representing a 50–100-fold less input material compared to conventional approaches.221 Their results detected all the core SMC1A interactors, which collectively constitute a cohesion ring around DNA to modulate DNA damage repair, chromosome segregation, and transcriptional regulation. Several other studies, meanwhile, used proximity labeling proteomic approaches to explore dynamic and transient PPIs while maintaining spatial information.223,224 For example, cell-type specific (neuron and astrocytes) signaling networks and changes in signaling network in response to external stimuli have been deciphered by the PPI analysis.225 Altogether, these studies demonstrate the utility of microfluidic devices in combination with MS to study the cellular responses against perturbed PPIs at the microproteomics scale, and pave the way towards its implementation at the single-cell level.226
Cellular heterogeneity.
Recent SCP analyses demonstrated the ability to reveal cell variability (heterogeneity) in heterogeneous populations of biological systems, which were previously masked by bulk sample analysis. By individual cell profiling, we can investigate molecular features of cellular phenotypes in the tumor microenvironment (TME), which may accelerate our understanding of tumor growth and progression. Slavov and colleagues developed the SCoPE-MS approach to investigate cellular heterogeneity in embryonic stem cells.180 This approach resolved time-dependent differentiating stem cells into single embryoid body and embryonic stem cells based on their proteomic changes during differentiation. Additionally, the method uncovered a coordinated relationship between mRNA and protein abundances in single cells. These results show the strength of SCP to classify cell types and gene regulation patterns at the single-cell level.180 In an improved version, SCoPE2 identified differential proteomics patterns during the differentiation of monocytes into macrophage-like cells.7 Interestingly, the results showed that monocytes exhibited increased expression of proteins associated with cell proliferation, while macrophage-like cells showed increased levels of cell adhesion proteins. Additionally, they demonstrated that the protein abundance of p53 correlates more closely with their targeted protein expression in comparison to their transcript levels, suggesting post-transcriptional regulation.7 Furthermore, SCeptre has been applied to characterize stem cell hierarchies,8 showing distinct differentiation pathways of leukemic stem cells. Very recently, single-cell chemical proteomics (SCCP) derived from SCoPE-MS was developed to explore time-dependent proteomic changes in human lung adenocarcinoma cells upon treatment of anti-cancer drugs including camptothecin, tomudex and methotrexate.227 The results showed the emergence of cellular subpopulations of surviving (uncommitted to death) and dying cells (committed to death), which were found to enriched either with proteins involved in metabolic pathways or proteasome-related pathways, respectively. This study demonstrated the utility of SCP to uncover heterogeneous responses, including drug resistance, and diverse cellular phenotypes upon anti-cancer drug treatments.
Spatial tissue proteomics.
SCP technologies are revolutionizing spatial biology since they provide insight into the spatial organization of cells and sub-cellular compartments within complex biological systems (e.g., tissues and TME). Most MS-based SCP investigations involved cell lines or cells isolated from tissue slices. However, the information regarding spatial context of proteome expression was often lost during sample preparation. As a step to circumvent such limitations, recent progress in SCP technologies, microscopy, and data analysis have started to enable the 3D mapping of proteome expression to explore single-cell heterogeneity, protein translocation events, and the network of cell–cell interactions and their spatial context.228 To advance clinical research, SCP approaches have demonstrated the potential to map spatial distribution of proteome with subcellular resolution across tissues.229
Many biological systems are highly heterogeneous, consisting of different cell types, intermixed subpopulations, and tissue substructures (e.g., regions with similar spatial expression patterns).230 Spatially resolved proteomics provides a new way to decode the spatial protein profile, which may provide insight into the complex pathogenesis of diseases. Recent SCP approaches have been successfully applied to dissect tissue regions at the single-cell resolution to resolve their heterogeneity. For example, Xu et al. integrated laser-capture microdissection (LCM) with a spintip-based sample preparation device (SISPROT) to dissect clinical tissue samples, enabling spatial resolution and cell-type resolved mapping of the colon tumor and surrounding tissues.231 In an improved version, immunohistochemistry SISPROT (IHC-SISPROT) was used to spatially resolve the TME of hepatocellular carcinoma. Interestingly, the results found that the CD81 receptor on cancer-associated fibroblasts (CAFs) directly communicates with the GPC3 receptor on cancer cells. This is an example of signal-crosstalk between CAFs and cancer cells to remodel the extracellular matrix.232,233 Meanwhile, nanoPOTS integrated with LCM was also used to identify differences in cell-type specific or tissue subsection-type proteomes in human cancer tissues,234 rat brain, and mouse uterus.235 Recently, LCM-nanoPOTS was modified with a hanging drop format to find tissue-region specific or cell-type specific biomarkers of mouse uterine tissues.236 Using this approach, the results showed proteins enriched in the luminal epithelium were related to ion channels and transporters, while extracellular matrix and collagen-related proteins were enriched in the stromal region. The applications of these approaches demonstrated the potential of proteome mapping with high spatial resolution across tissue regions to advance biomedical research. Furthermore, this approach can be applied to explore the pathology of TMEs and disease diagnosis.
Mund et al. recently introduced AI-guided image analysis coupled to ultrasensitive proteomics workflow, collectively called deep visual proteomics (DVP), to analyze single-cell isolated from LCM and sub-cellular proteome.237 DVP was applied to salivary gland carcinoma tissue and melanoma tissue to study tissue heterogeneity and molecular alterations during cancer development and progression, aiming to discover therapeutic vulnerabilities. Cellular images and bioinformatics tools were employed to determine protein signature from identified ∼1000 s of proteins associated with cellular heterogeneity at the single-cell level. This approach revealed cellular phenotypic differences between cancer and adjacent normal-appearing cells in salivary gland carcinoma tissue while preserving complete spatial information. Furthermore, the spatially resolved proteomes of melanoma tissue revealed upregulation of mRNA splicing and reduced interferon signaling during healthy melanocytes transformation into invasive melanoma. These spatially resolved proteome uncovered new insight of tumor progression mechanisms and potential therapeutic targets for cancer treatment.237
Biomarker discovery and therapeutics monitoring.
Single-cell protein and proteome analyses demonstrate their promising potential to uncover cellular status, therapeutic targets, and cell-specific protein markers.8,9,238 For example, the study using the iProChip–DIA demonstrated that important drug targets, biomarkers and surface proteins associated with the NSCLC pathway could be identified from a single lung cancer cell.10 Further, the results also detected different immune markers expressing across ∼5 orders of magnitude from individual leukemia cells. This shows the potential of SCP to measure biomarkers over wide dynamic ranges and to detect druggable targets from individual cells, supporting the notation that SCP methods can serve as an ideal platform to accelerate therapeutic applications such as in the chimeric antigen receptor (CAR) T cell therapy pipeline. Although few MS-based studies have been applied to CAR-T cell-based therapies, their application to single-cell, to the best of our knowledge, is currently lacking.209,239–241 Nevertheless, selective monitoring of proteins from single cells has proven to accelerate CAR T therapy for leukemia and myeloma cancers.242,243 Therefore, proteomic measurements have the potential to predict the therapeutic potency of CAR T cells and to identify biomarkers in tumors at the single-cell level.
SCP profiling of CTCs can offer crucial insight into the mechanisms of tumor metastasis for better understanding of disease progression and treatment response. The initial attempts have demonstrated the feasibility to differentiate CTCs from healthy blood cells based on the proteomic profiling.244,245 Although various targeted-based proteomics approaches have been applied to the single-cell level, global proteome characterization of individual CTCs is yet to be analyzed.138,210 With the potential add-on functionality of facile single-cell handling on-chip, recent microfluidics-based SCP could be a viable option to characterize CTCs, which can provide non-invasive monitoring of disease progression and aid in tailoring therapies for patients.
6. Future perspectives and conclusion
The SCP research is prospering at an unprecedented pace, and continuous efforts toward ultrasensitive profiling have benefited from multiple factors, such as those exciting technological and analytical developments discussed thus far (Table 1). From a user's perspective, choosing the most appropriate SCP method is likely to be subject-specific; there is unlikely a single SCP strategy that fits all purposes. Affinity-based SCP methods are ideal for probing a specific set of proteins whose functions are key determinants underlying a known biological phenomenon or in driving disease progression. Meanwhile, MS-based workflows offer unparalleled features that allow discovery-oriented investigations to study the composition, abundance, and functional states of proteins in relation to key biological processes, and will be favorable choices for global proteome profiling.11 Note that, despite the remarkable advancements highlighted so far, both targeted and global SCP analyses still have room for further improvements. In the following, an overview of the technological landscape as well as future perspectives are presented.
Until now, targeted SCP methods have reported analyses with detection of up to 182 proteins from the same batch of single cells using the CycMIST method.127 Undoubtedly, for affinity-based SCP, a major bottleneck is the availability and specificity of reliable antibodies, as well as the capacity and compatibility of corresponding fluorescent probes and detection channels. To move forward, developing more orthogonal affinity probes that allow flexible tagging and multiplexed chemical functionalization will enable the investigations of targeted SCP with higher throughput. Another strategy for targeted SCP replaces antibodies with aptamers which yield a higher multiplexity (>1300 proteins), while other metrics (e.g., dynamic range and specificity) would be affected.246
Considering the LCN proteins, which are usually masked in MS-derived measurements, targeted SCP combined with a novel transducer may provide a niche of ultrasensitivity. Laser-induced fluorescence (LIF) and SERS that are integrated with microfluidics-based instrumentation have spearheaded the advances in detecting LCN proteins.132,157,158,247,248 To increase the multiplexity and throughputs, other sensing modalities are also worth considering. For instance, magnetoresistive biosensing is one of the strong candidates since its ultrasensitivity comes from the fact that most biological samples exhibit negligible magnetic background.249–256 This matrix insensitivity substantially eases the complexity in sample pretreatment. Moreover, the investigation of magnetorelaxometry indicates that the temporal signature may increase multiplexity by varying magnetic nanoparticles' materials and sizes.255–257 As such, the integration of microfluidics with magnetoresistive nanosensors is expected to benefit targeted SCP analysis for the LCN regime.
MS is the instrumental backbone of global proteomics profiling. Yet global SCP faces challenges associated with the inefficient delivery of peptide ions to a mass spectrometer, and limited throughput for analyzing more single-cells in a single run. To facilitate global SCP using MS, experimental and computational efforts have been implemented to increase analytical throughputs and sensitivity. As shown in this review, recent experimental improvements in SCP technologies consistently emphasize reducing sample loss by minimizing sample volume and scaling the analysis from multiple samples. While these approaches offered miniaturization of overall workflow, they currently lack the throughput to process hundreds of single cells. To increase throughput, multiplexing via TMT labeling requires additional sample handling steps to offer considerable improvement in MS throughput. A microfluidic platform for seamless integration of miniaturized single-cell sample preparation and labeling is urgently needed to enable SCP analysis with higher-throughput. Another aspect to push sensitivity forward is the improvements in (1) transfer of peptide samples to LC and (2) chromatographic separations for single-cell level samples that further enable efficient ion delivery to MS instruments. In this niche, microfluidic devices may be directly coupled to LC-MS/MS to further enhance single-cell identification depth. In a longer run, the direct coupling of microfluidics-based separations, fractionations, and ionization to MS may further circumvent the need of time-consuming LC separations. On the other hand, microfluidics devices can be functionalized or packed with proper enrichment beads/materials to enrich post-translationally modified proteins (such as those being phosphorylated or glycosylated) from low-sample sizes (or even single-cell), or to purify (by sorting and isolation) rare cells such as CTCs for subsequent SCP analysis to identify new drug targets and biomarkers. Furthermore, automated column composition (C18-particles or μPAC), column length, gradient time, flow rate, and other orthogonal to LC approaches are key areas to be considered for highly sensitive “lossless” SCPs. Together with the above capabilities, designated data acquisition strategies and appropriate data analysis pipelines (e.g., sample-sized library) prospectively maximize proteomic depth, reproducibility, sensitivity, and throughputs for large-scale clinical SCPs.258
Apart from improvements in microfluidics-based workflow, the standardized computational pipeline to analyze downstream SCP data is of high interest.258,259 The current spectral data processing approaches exhibit limited identification rate, relatively time-consuming construction of libraries and throughput which compromises the extraction of biologically critical information from SCP data. To this end, deep learning-based models might be employed with computational pipelines to boost identification and generate predicted libraries to complement experimental libraries with the least possible resources.
At current proteomic depth, SCP technologies have demonstrated several applications at single-cell resolution. These applications have been broadening from cell lines to spatially resolved tumor tissues at an individual cell level. Most of these studies focus on either improvement in sample preparation or optimization of data acquisition strategies, and the state-of-the-art enables the identification of 1000–1500 proteins per cell.7,8,10,180 To cope with microproteomics, recently, plexDIA combines non-isobaric mass tags with DIA to analyze nanogram-level samples, thus increasing throughput and quantifying ∼1000 proteins per cell with 98% of data completeness.260 Looking forward, SCP MS is expected to achieve the goals of measuring more than few thousand proteins and quantifying functionally relevant proteins across individual cells, which will further catalyze SCP's utilities toward broader biological systems and guiding the future of medicine. The progress, in turn, will accelerate the development of clinical assays for diagnosing diseases and probing drug targets for cancer treatment. Recent SCP developments also have the potential to transform developmental biology. With identification of >1000 proteins from typical mammalian cells, microfluidics-based approaches are expected to detect a few thousands of proteins from large-sized cells such as oocytes or early stage embryos.261 With above-mentioned improvements, SCP shall provide valuable insights into stem cell differentiation, early stage embryonic development and organ development.
With the advances in microfluidics-enabled cell assays, micromanipulation of single cells validates controllable sampling of nucleic acids and proteins, paving the way for the studies in dynamic cell biology where cell-to-cell communication, microenvironmental regulation and spatiotemporal cell dynamics are disclosed.262–265 Since sole reliance on uni-omic information limitedly answers and connects the underlying biological uncertainty between genotypes and phenotypes; several works, such as CITE-seq76 and nanoSPLITS,266 integrate proteomics workflow with well-established single-cell RNA sequencing technologies to enable bi-modal analysis. Recently, various microfluidics platforms (whether droplet or microwell) have been proposed with multimodal analysis,267,268 and the resulting big-data framework necessitates the implementation of deep learning on bioinformatics that computationally urges the advent of the multi-omics era.269–271 On the horizon, it is also anticipated that multilayer microfluidics can be designed to accommodate multiple functional modules for conducting either the multi-omics or dynamic cell biology experiments.
In this review, we have discussed the pivotal developments of miniaturized technology in SCP analysis as well as the emerging field of single-cell microfluidics, since they provide platforms and insights to elucidate long-standing enigma in developmental biology, cell heterogeneity, tumor progression, metastasis, etc. Given multiplexing capacity, flexibility in throughputs, precise manipulation of single-cells, adaptability to automation, and compatibility with downstream bioanalytical tools; further developments in microfluidic-based approaches will continuously cast light on single-cell functional proteomics and lay the foundation of the multi-omics era.
Author contributions
Sofani Tafesse Gebreyesus: conceptualization, investigation, visualization, writing – original draft, writing – review & editing. Gul Muneer: investigation, visualization, writing – original draft, writing – review & editing. Chih-Cheng Huang: visualization, investigation, writing – original draft, writing – review & editing. Asad Ali Siyal: conceptualization, writing – original draft, writing – review & editing. Mihir Anand: visualization. Yu-Ju Chen: funding acquisition, supervision, writing – review & editing. Hsiung-Lin Tu: conceptualization, funding acquisition, investigation, supervision, writing – original draft, writing – review & editing.
Conflicts of interest
The authors declare that they have no known competing financial interests or personal relationships that could have appeared to influence the work reported in this paper.
Acknowledgements
This work was supported by the Ministry of Science and Technology (MOST 110-2113-M-001-019-MY2) and Academia Sinica (AS-GC-111-M03) in Taiwan.
References
-
Institute of Medicine, Board on Health Care Services, Board on Health Sciences Policy, Committee on the Review of Omics-Based Tests for Predicting Patient Outcomes in Clinical Trials, C. M. Micheel, S. J. Nass and G. S. Omenn, Evolution of Translational Omics: Lessons Learned and the Path Forward, 2012 Search PubMed
.
-
D. J. Perry, in Hemostasis and Thrombosis Protocols, ed. D. J. Perry and K. J. Pasi, Humana Press, Totowa, NJ, 1999, pp. 31–38 Search PubMed
.
- C. Gawad, W. Koh and S. R. Quake, Nat. Rev. Genet., 2016, 17, 175–188 CrossRef CAS PubMed
.
- F. Tang, C. Barbacioru, Y. Wang, E. Nordman, C. Lee, N. Xu, X. Wang, J. Bodeau, B. B. Tuch, A. Siddiqui, K. Lao and M. A. Surani, Nat. Methods, 2009, 6, 377–382 CrossRef CAS PubMed
.
- A. Koussounadis, S. P. Langdon, I. H. Um, D. J. Harrison and V. A. Smith, Sci. Rep., 2015, 5, 10775 CrossRef PubMed
.
- S. H. Payne, Trends Biochem. Sci., 2015, 40, 1–3 CrossRef CAS PubMed
.
- H. Specht, E. Emmott, A. A. Petelski, R. G. Huffman, D. H. Perlman, M. Serra, P. Kharchenko, A. Koller and N. Slavov, Genome Biol., 2021, 22, 50 CrossRef CAS PubMed
.
- E. M. Schoof, B. Furtwängler, N. Üresin, N. Rapin, S. Savickas, C. Gentil, E. Lechman, U. auf dem Keller, J. E. Dick and B. T. Porse, Nat. Commun., 2021, 12, 3341 CrossRef CAS PubMed
.
- J. Woo, S. M. Williams, L. M. Markillie, S. Feng, C.-F. Tsai, V. Aguilera-Vazquez, R. L. Sontag, R. J. Moore, D. Hu, H. S. Mehta, J. Cantlon-Bruce, T. Liu, J. N. Adkins, R. D. Smith, G. C. Clair, L. Pasa-Tolic and Y. Zhu, Nat. Commun., 2021, 12, 6246 CrossRef CAS PubMed
.
- S. T. Gebreyesus, A. A. Siyal, R. B. Kitata, E. S.-W. Chen, B. Enkhbayar, T. Angata, K.-I. Lin, Y.-J. Chen and H.-L. Tu, Nat. Commun., 2022, 13, 37 CrossRef CAS PubMed
.
- A. Doerr, Nat. Methods, 2019, 16, 20 CrossRef CAS PubMed
.
- B. Sun and S. Kumar, J. Proteome Res., 2022, 21, 1808–1815 CrossRef CAS PubMed
.
- G. M. Whitesides, Nature, 2006, 442, 368–373 CrossRef CAS PubMed
.
- S. F. Berlanda, M. Breitfeld, C. L. Dietsche and P. S. Dittrich, Anal. Chem., 2021, 93, 311–331 CrossRef CAS PubMed
.
- D. Gao, H. Liu, Y. Jiang and J.-M. Lin, Lab Chip, 2013, 13, 3309–3322 RSC
.
- L. F. Vistain and S. Tay, Trends Biochem. Sci., 2021, 46, 661–672 CrossRef CAS PubMed
.
- D. Mahdessian, A. J. Cesnik, C. Gnann, F. Danielsson, L. Stenström, M. Arif, C. Zhang, T. Le, F. Johansson, R. Schutten, A. Bäckström, U. Axelsson, P. Thul, N. H. Cho, O. Carja, M. Uhlén, A. Mardinoglu, C. Stadler, C. Lindskog, B. Ayoglu, M. D. Leonetti, F. Pontén, D. P. Sullivan and E. Lundberg, Nature, 2021, 590, 649–654 CrossRef CAS PubMed
.
- M. Labib and S. O. Kelley, Nat. Rev. Chem., 2020, 4, 143–158 CrossRef
.
- X. Xu, J. Wang, L. Wu, J. Guo, Y. Song, T. Tian, W. Wang, Z. Zhu and C. Yang, Small, 2020, 16, 1903905 CrossRef CAS PubMed
.
- H. Xie and X. Ding, Adv. Sci., 2022, 9, 2105932 CrossRef CAS PubMed
.
- J. Källberg, W. Xiao, D. V. Assche, J.-C. Baret and V. Taly, Lab Chip, 2022, 22, 2403–2422 RSC
.
- D. Liu, M. Sun, J. Zhang, R. Hu, W. Fu, T. Xuanyuan and W. Liu, Analyst, 2022, 147, 2294–2316 RSC
.
- D. Anggraini, N. Ota, Y. Shen, T. Tang, Y. Tanaka, Y. Hosokawa, M. Li and Y. Yalikun, Lab Chip, 2022, 22, 1438–1468 RSC
.
-
J. Andrus, US Pat., US3122817A, 1964 Search PubMed
.
- S. C. Terry, J. H. Jerman and J. B. Angell, IEEE Trans. Electron Devices, 1979, 26, 1880–1886 Search PubMed
.
- A. Manz, N. Graber and H. M. Widmer, Sens. Actuators, B, 1990, 1, 244–248 CrossRef CAS
.
- T. Thorsen, R. W. Roberts, F. H. Arnold and S. R. Quake, Phys. Rev. Lett., 2001, 86, 4163–4166 CrossRef CAS PubMed
.
- R. B. Fair, Microfluid. Nanofluid., 2007, 3, 245–281 CrossRef CAS
.
- D. S. Tawfik and A. D. Griffiths, Nat. Biotechnol., 1998, 16, 652–656 CrossRef CAS PubMed
.
- P. B. Umbanhowar, V. Prasad and D. A. Weitz, Langmuir, 2000, 16, 347–351 CrossRef CAS
.
- D. C. Duffy, J. C. McDonald, O. J. A. Schueller and G. M. Whitesides, Anal. Chem., 1998, 70, 4974–4984 CrossRef CAS PubMed
.
- M. A. Unger, H.-P. Chou, T. Thorsen, A. Scherer and S. R. Quake, Science, 2000, 288, 113–116 CrossRef CAS PubMed
.
- E. K. Sackmann, A. L. Fulton and D. J. Beebe, Nature, 2014, 507, 181–189 CrossRef CAS PubMed
.
- T. A. Duncombe, A. M. Tentori and A. E. Herr, Nat. Rev. Mol. Cell Biol., 2015, 16, 554–567 CrossRef CAS PubMed
.
- Y. Yang, Y. Chen, H. Tang, N. Zong and X. Jiang, Small Methods, 2020, 4, 1900451 CrossRef CAS
.
- A. Folch and M. Toner, Biotechnol. Prog., 1998, 14, 388–392 CrossRef CAS PubMed
.
- S. Köster, F. E. Angilè, H. Duan, J. J. Agresti, A. Wintner, C. Schmitz, A. C. Rowat, C. A. Merten, D. Pisignano, A. D. Griffiths and D. A. Weitz, Lab Chip, 2008, 8, 1110–1115 RSC
.
- P. Zhu and L. Wang, Lab Chip, 2016, 17, 34–75 RSC
.
- J. F. Edd, D. D. Carlo, K. J. Humphry, S. Köster, D. Irimia, D. A. Weitz and M. Toner, Lab Chip, 2008, 8, 1262–1264 RSC
.
- G. Kamalakshakurup and A. P. Lee, Lab Chip, 2017, 17, 4324–4333 RSC
.
- A. M. Skelley, O. Kirak, H. Suh, R. Jaenisch and J. Voldman, Nat. Methods, 2009, 6, 147–152 CrossRef CAS PubMed
.
- D. Di Carlo, N. Aghdam and L. P. Lee, Anal. Chem., 2006, 78, 4925–4930 CrossRef CAS PubMed
.
- F. Shen, X. Li and P. C. H. Li, Biomicrofluidics, 2014, 8, 014109 CrossRef PubMed
.
- J. Zhou, C. Tu, Y. Liang, B. Huang, Y. Fang, X. Liang and X. Ye, Analyst, 2020, 145, 1706–1715 RSC
.
- A. Revzin, K. Sekine, A. Sin, R. G. Tompkins and M. Toner, Lab Chip, 2005, 5, 30–37 RSC
.
- J. Yuan and P. A. Sims, Sci. Rep., 2016, 6, 33883 CrossRef CAS PubMed
.
- J. R. Rettig and A. Folch, Anal. Chem., 2005, 77, 5628–5634 CrossRef CAS PubMed
.
- J. W. Hong, V. Studer, G. Hang, W. F. Anderson and S. R. Quake, Nat. Biotechnol., 2004, 22, 435–439 CrossRef CAS PubMed
.
- P. Li, Z. Mao, Z. Peng, L. Zhou, Y. Chen, P.-H. Huang, C. I. Truica, J. J. Drabick, W. S. El-Deiry, M. Dao, S. Suresh and T. J. Huang, Proc. Natl. Acad. Sci. U. S. A., 2015, 112, 4970–4975 CrossRef CAS PubMed
.
- X. Wang, S. Chen, M. Kong, Z. Wang, K. D. Costa, R. A. Li and D. Sun, Lab Chip, 2011, 11, 3656–3662 RSC
.
- A. Myklatun, M. Cappetta, M. Winklhofer, V. Ntziachristos and G. G. Westmeyer, Sci. Rep., 2017, 7, 6942 CrossRef PubMed
.
- D. R. Gossett, W. M. Weaver, A. J. Mach, S. C. Hur, H. T. K. Tse, W. Lee, H. Amini and D. Di Carlo, Anal. Bioanal. Chem., 2010, 397, 3249–3267 CrossRef CAS PubMed
.
- Y. Li, C. Dalton, H. J. Crabtree, G. Nilsson and K. V. I. S. Kaler, Lab Chip, 2007, 7, 239–248 RSC
.
- L. Nan, Z. Jiang and X. Wei, Lab Chip, 2014, 14, 1060–1073 RSC
.
- X. Chen, D. Cui, C. Liu, H. Li and J. Chen, Anal. Chim. Acta, 2007, 584, 237–243 CrossRef CAS PubMed
.
- D. Irimia, R. G. Tompkins and M. Toner, Anal. Chem., 2004, 76, 6137–6143 CrossRef CAS PubMed
.
- Y. Sasuga, T. Iwasawa, K. Terada, Y. Oe, H. Sorimachi, O. Ohara and Y. Harada, Anal. Chem., 2008, 80, 9141–9149 CrossRef CAS PubMed
.
- D. D. Carlo, K.-H. Jeong and L. P. Lee, Lab Chip, 2003, 3, 287–291 RSC
.
- S.-S. Yun, S. Y. Yoon, M.-K. Song, S.-H. Im, S. Kim, J.-H. Lee and S. Yang, Lab Chip, 2010, 10, 1442–1446 RSC
.
- S.-W. Lee and Y.-C. Tai, Sens. Actuators, A, 1999, 73, 74–79 CrossRef CAS
.
- H.-H. Lai, P. A. Quinto-Su, C. E. Sims, M. Bachman, G. P. Li, V. Venugopalan and N. L. Allbritton, J. R. Soc., Interface, 2008, 5, S113–S121 CrossRef CAS PubMed
.
- H. Li, C. E. Sims, H. Y. Wu and N. L. Allbritton, Anal. Chem., 2001, 73, 4625–4631 CrossRef CAS PubMed
.
- P. A. Quinto-Su, H.-H. Lai, H. H. Yoon, C. E. Sims, N. L. Allbritton and V. Venugopalan, Lab Chip, 2008, 8, 408–414 RSC
.
- C. Ke, A.-M. Kelleher, H. Berney, M. Sheehan and A. Mathewson, Sens. Actuators, B, 2007, 120, 538–544 CrossRef CAS
.
- S. Baek, J. Min and J.-H. Park, Lab Chip, 2010, 10, 909–917 RSC
.
- X. Feng, B.-F. Liu, J. Li and X. Liu, Mass Spectrom. Rev., 2015, 34, 535–557 CrossRef CAS PubMed
.
- O. Guillaume-Gentil, T. Rey, P. Kiefer, A. J. Ibáñez, R. Steinhoff, R. Brönnimann, L. Dorwling-Carter, T. Zambelli, R. Zenobi and J. A. Vorholt, Anal. Chem., 2017, 89, 5017–5023 CrossRef CAS PubMed
.
- S. Mao, W. Li, Q. Zhang, W. Zhang, Q. Huang and J.-M. Lin, TrAC, Trends Anal. Chem., 2018, 107, 43–59 CrossRef CAS
.
- N. Pan, W. Rao, N. R. Kothapalli, R. Liu, A. W. G. Burgett and Z. Yang, Anal. Chem., 2014, 86, 9376–9380 CrossRef CAS PubMed
.
- C.-M. Huang, Y. Zhu, D.-Q. Jin, R. T. Kelly and Q. Fang, Anal. Chem., 2017, 89, 9009–9016 CrossRef CAS PubMed
.
- J. R. Yates, C. I. Ruse and A. Nakorchevsky, Annu. Rev. Biomed. Eng., 2009, 11, 49–79 CrossRef CAS PubMed
.
- W. De Malsche, H. Eghbali, D. Clicq, J. Vangelooven, H. Gardeniers and G. Desmet, Anal. Chem., 2007, 79, 5915–5926 CrossRef CAS PubMed
.
- J. De Vos, M. Dams, K. Broeckhoven, G. Desmet, B. Horstkotte and S. Eeltink, Anal. Chem., 2020, 92, 2388–2392 CrossRef CAS PubMed
.
- P.-C. Chen, W.-Z. Zhang, W.-R. Chen, Y.-C. Jair, Y.-H. Wu, Y.-H. Liu, P.-Z. Chen, L.-Y. Chen and P.-S. Chen, Sens. Actuators, B, 2022, 350, 130888 CrossRef CAS
.
- S. C. Bendall, E. F. Simonds, P. Qiu, E. D. Amir, P. O. Krutzik, R. Finck, R. V. Bruggner, R. Melamed, A. Trejo, O. I. Ornatsky, R. S. Balderas, S. K. Plevritis, K. Sachs, D. Pe'er, S. D. Tanner and G. P. Nolan, Science, 2011, 332, 687–696 CrossRef CAS PubMed
.
- M. Stoeckius, C. Hafemeister, W. Stephenson, B. Houck-Loomis, P. K. Chattopadhyay, H. Swerdlow, R. Satija and P. Smibert, Nat. Methods, 2017, 14, 865–868 CrossRef CAS PubMed
.
- G. M. Hamza, V. B. Bergo, S. Mamaev, D. M. Wojchowski, P. Toran, C. R. Worsfold, M. P. Castaldi and J. C. Silva, Int. J. Mol. Sci., 2020, 21, 2016 CrossRef CAS PubMed
.
- A. M. Sadaghiani, S. H. Verhelst and M. Bogyo, Curr. Opin. Chem. Biol., 2007, 11, 20–28 CrossRef CAS PubMed
.
- Y. Liu, X. Chen, Y. Zhang and J. Liu, Analyst, 2019, 144, 846–858 RSC
.
- J. Lamanna, E. Y. Scott, H. S. Edwards, M. D. Chamberlain, M. D. M. Dryden, J. Peng, B. Mair, A. Lee, C. Chan, A. A. Sklavounos, A. Heffernan, F. Abbas, C. Lam, M. E. Olson, J. Moffat and A. R. Wheeler, Nat. Commun., 2020, 11, 5632 CrossRef CAS PubMed
.
- Y. Lu, L. Yang, W. Wei and Q. Shi, Lab Chip, 2017, 17, 1250–1263 RSC
.
- V. V. Krishhan, I. H. Khan and P. A. Luciw, Crit. Rev. Biotechnol., 2009, 29, 29–43 CrossRef CAS PubMed
.
- J. P. Robinson and M. Roederer, Science, 2015, 350, 739–740 CrossRef CAS PubMed
.
- X. Mao, S.-C. S. Lin, C. Dong and T. J. Huang, Lab Chip, 2009, 9, 1583–1589 RSC
.
- J. P. Golden, J. S. Kim, J. S. Erickson, L. R. Hilliard, P. B. Howell, G. P. Anderson, M. Nasir and F. S. Ligler, Lab Chip, 2009, 9, 1942–1950 RSC
.
- N. S. Barteneva, E. Fasler-Kan and I. A. Vorobjev, J. Histochem. Cytochem., 2012, 60, 723–733 CrossRef CAS PubMed
.
- D. A. Basiji, W. E. Ortyn, L. Liang, V. Venkatachalam and P. Morrissey, Clin. Lab. Med., 2007, 27, 653–670 CrossRef PubMed
.
- L. F. Grimwade, K. A. Fuller and W. N. Erber, Methods, 2017, 112, 39–45 CrossRef CAS PubMed
.
- S. Stavrakis, G. Holzner, J. Choo and A. deMello, Curr. Opin. Biotechnol., 2019, 55, 36–43 CrossRef CAS PubMed
.
- A. S. Rane, J. Rutkauskaite, A. deMello and S. Stavrakis, Chem, 2017, 3, 588–602 CAS
.
- T. Miura, H. Mikami, A. Isozaki, T. Ito, Y. Ozeki and K. Goda, Biomed. Opt. Express, 2018, 9, 3424–3433 CrossRef CAS PubMed
.
- Y. Han and Y.-H. Lo, Sci. Rep., 2015, 5, 13267 CrossRef CAS PubMed
.
- G. Holzner, B. Mateescu, D. van Leeuwen, G. Cereghetti, R. Dechant, S. Stavrakis and A. deMello, Cell Rep., 2021, 34, 108824 CrossRef CAS PubMed
.
- M. Roederer, Cytometry, 2001, 45, 194–205 CrossRef CAS PubMed
.
- D. R. Bandura, V. I. Baranov, O. I. Ornatsky, A. Antonov, R. Kinach, X. Lou, S. Pavlov, S. Vorobiev, J. E. Dick and S. D. Tanner, Anal. Chem., 2009, 81, 6813–6822 CrossRef CAS PubMed
.
- O. Ornatsky, D. Bandura, V. Baranov, M. Nitz, M. A. Winnik and S. Tanner, J. Immunol. Methods, 2010, 361, 1–20 CrossRef CAS PubMed
.
- M. Angelo, S. C. Bendall, R. Finck, M. B. Hale, C. Hitzman, A. D. Borowsky, R. M. Levenson, J. B. Lowe, S. D. Liu, S. Zhao, Y. Natkunam and G. P. Nolan, Nat. Med., 2014, 20, 436–442 CrossRef CAS PubMed
.
- S. C. Bendall, K. L. Davis, E. D. Amir, M. D. Tadmor, E. F. Simonds, T. J. Chen, D. K. Shenfeld, G. P. Nolan and D. Pe'er, Cell, 2014, 157, 714–725 CrossRef CAS PubMed
.
- E. Porpiglia, N. Samusik, A. T. V. Ho, B. D. Cosgrove, T. Mai, K. L. Davis, A. Jager, G. P. Nolan, S. C. Bendall, W. J. Fantl and H. M. Blau, Nat. Cell Biol., 2017, 19, 558–567 CrossRef CAS PubMed
.
- A. Wroblewska, M. Dhainaut, B. Ben-Zvi, S. A. Rose, E. S. Park, E.-A. D. Amir, A. Bektesevic, A. Baccarini, M. Merad, A. H. Rahman and B. D. Brown, Cell, 2018, 175, 1141–1155 CrossRef CAS PubMed
, e16.
- L. Stern, H. McGuire, S. Avdic, S. Rizzetto, B. Fazekas de St Groth, F. Luciani, B. Slobedman and E. Blyth, Front. Immunol., 2018, 9, 01672 CrossRef PubMed
.
- L. Stern, H. M. McGuire, S. Avdic, B. Fazekas de St Groth, D. Gottlieb, A. Abendroth, E. Blyth and B. Slobedman, Nat. Commun., 2022, 13, 2603 CrossRef CAS PubMed
.
- Q. Chang, O. Ornatsky and D. Hedley, Curr. Protoc. Cytom., 2017, 82, 12.47.1–12.47.8 CAS
.
- R. Catena, L. M. Montuenga and B. Bodenmiller, J. Pathol., 2018, 244, 479–484 CrossRef CAS PubMed
.
- H. W. Jackson, J. R. Fischer, V. R. T. Zanotelli, H. R. Ali, R. Mechera, S. D. Soysal, H. Moch, S. Muenst, Z. Varga, W. P. Weber and B. Bodenmiller, Nature, 2020, 578, 615–620 CrossRef CAS PubMed
.
- C. Giesen, H. A. O. Wang, D. Schapiro, N. Zivanovic, A. Jacobs, B. Hattendorf, P. J. Schüffler, D. Grolimund, J. M. Buhmann, S. Brandt, Z. Varga, P. J. Wild, D. Günther and B. Bodenmiller, Nat. Methods, 2014, 11, 417–422 CrossRef CAS PubMed
.
- L. Kuett, R. Catena, A. Özcan, A. Plüss, P. Schraml, H. Moch, N. de Souza and B. Bodenmiller, Nat. Cancer, 2022, 3, 122–133 CrossRef CAS PubMed
.
- E. Gerdtsson, M. Pore, J.-A. Thiele, A. S. Gerdtsson, P. D. Malihi, R. Nevarez, A. Kolatkar, C. R. Velasco, S. Wix, M. Singh, A. Carlsson, A. J. Zurita, C. Logothetis, A. A. Merchant, J. Hicks and P. Kuhn, Convergent Sci. Phys. Oncol., 2018, 4, 015002 CrossRef PubMed
.
- J. C. Love, J. L. Ronan, G. M. Grotenbreg, A. G. van der Veen and H. L. Ploegh, Nat. Biotechnol., 2006, 24, 703–707 CrossRef CAS PubMed
.
- S. M. Schubert, S. R. Walter, M. Manesse and D. R. Walt, Anal. Chem., 2016, 88, 2952–2957 CrossRef CAS PubMed
.
- B. Jia, L. K. McNeil, C. D. Dupont, K. Tsioris, R. M. Barry, I. L. Scully, A. O. Ogunniyi, C. Gonzalez, M. W. Pride, T. M. Gierahn, P. A. Liberator, K. U. Jansen and J. C. Love, PLoS One, 2017, 12, e0183738 CrossRef PubMed
.
- Q. Li, S. A. Bencherif and M. Su, Anal. Chem., 2021, 93, 10292–10300 CrossRef CAS PubMed
.
- V. Herrera, S.-C. J. Hsu, M. K. Rahim, C. Chen, L. Nguyen, W. F. Liu and J. B. Haun, Analyst, 2019, 144, 980–989 RSC
.
- V. Herrera, S.-C. J. Hsu, V. Y. Naveen, W. F. Liu and J. B. Haun, Anal. Chem., 2022, 94, 658–668 CrossRef CAS PubMed
.
- J. H. Choi, A. O. Ogunniyi, M. Du, M. Du, M. Kretschmann, J. Eberhardt and J. C. Love, Biotechnol. Prog., 2010, 26, 888–895 CrossRef CAS PubMed
.
- N. Varadarajan, B. Julg, Y. J. Yamanaka, H. Chen, A. O. Ogunniyi, E. McAndrew, L. C. Porter, A. Piechocka-Trocha, B. J. Hill, D. C. Douek, F. Pereyra, B. D. Walker and J. C. Love, J. Clin. Invest., 2011, 121, 4322–4331 CrossRef CAS PubMed
.
- C. Ma, R. Fan, H. Ahmad, Q. Shi, B. Comin-Anduix, T. Chodon, R. C. Koya, C.-C. Liu, G. A. Kwong, C. G. Radu, A. Ribas and J. R. Heath, Nat. Med., 2011, 17, 738–743 CrossRef CAS PubMed
.
- M. Fallahi-Sichani, N. J. Moerke, M. Niepel, T. Zhang, N. S. Gray and P. K. Sorger, Mol. Syst. Biol., 2015, 11, 797 CrossRef PubMed
.
- N. Kravchenko-Balasha, Y. S. Shin, A. Sutherland, R. D. Levine and J. R. Heath, Proc. Natl. Acad. Sci. U. S. A., 2016, 113, 5520–5525 CrossRef CAS PubMed
.
- S. M. Shaffer, M. C. Dunagin, S. R. Torborg, E. A. Torre, B. Emert, C. Krepler, M. Beqiri, K. Sproesser, P. A. Brafford, M. Xiao, E. Eggan, I. N. Anastopoulos, C. A. Vargas-Garcia, A. Singh, K. L. Nathanson, M. Herlyn and A. Raj, Nature, 2017, 546, 431–435 CrossRef CAS PubMed
.
- Y. Su, W. Wei, L. Robert, M. Xue, J. Tsoi, A. Garcia-Diaz, B. Homet Moreno, J. Kim, R. H. Ng, J. W. Lee, R. C. Koya, B. Comin-Anduix, T. G. Graeber, A. Ribas and J. R. Heath, Proc. Natl. Acad. Sci. U. S. A., 2017, 114, 13679–13684 CrossRef CAS PubMed
.
- Y. Su, M. E. Ko, H. Cheng, R. Zhu, M. Xue, J. Wang, J. W. Lee, L. Frankiw, A. Xu, S. Wong, L. Robert, K. Takata, D. Yuan, Y. Lu, S. Huang, A. Ribas, R. Levine, G. P. Nolan, W. Wei, S. K. Plevritis, G. Li, D. Baltimore and J. R. Heath, Nat. Commun., 2020, 11, 2345 CrossRef CAS PubMed
.
- A. M. Xu, Q. Liu, K. L. Takata, S. Jeoung, Y. Su, I. Antoshechkin, S. Chen, M. Thomson and J. R. Heath, Lab Chip, 2018, 18, 3251–3262 RSC
.
- C. Wang, C. Wang, Y. Wu, J. Gao, Y. Han, Y. Chu, L. Qiang, J. Qiu, Y. Gao, Y. Wang, F. Song, Y. Wang, X. Shao, Y. Zhang and L. Han, Adv. Healthcare Mater., 2022, 11, 2102800 CrossRef CAS PubMed
.
- P. Zhao, S. Bhowmick, J. Yu and J. Wang, Adv. Sci., 2018, 5, 1800672 CrossRef PubMed
.
- M. A. A. Abdullah and J. Wang, ACS Sens., 2019, 4, 2296–2302 CrossRef CAS PubMed
.
- L. Yang, A. Ball, J. Liu, T. Jain, Y.-M. Li, F. Akhter, D. Zhu and J. Wang, Nat. Commun., 2022, 13, 3548 CrossRef CAS PubMed
.
- C. A. Monnig and R. T. Kennedy, Anal. Chem., 1994, 66, 280–314 CrossRef PubMed
.
- E. Buyuktuncel, Curr. Pharm. Anal., 2019, 15, 109–120 CrossRef CAS
.
- T. Kawai, Anal. Sci., 2021, 37, 27–36 CrossRef CAS PubMed
.
- M. A. A. Ragab and E. I. El-Kimary, Crit. Rev. Anal. Chem., 2021, 51, 709–741 CAS
.
- B. Huang, H. Wu, D. Bhaya, A. Grossman, S. Granier, B. K. Kobilka and R. N. Zare, Science, 2007, 315, 81–84 CrossRef CAS PubMed
.
- E. R. Mainz, Q. Wang, D. S. Lawrence and N. L. Allbritton, Angew. Chem., Int. Ed., 2016, 55, 13095–13098 CrossRef CAS PubMed
.
- Q. Li, P. Chen, Y. Fan, X. Wang, K. Xu, L. Li and B. Tang, Anal. Chem., 2016, 88, 8610–8616 CrossRef CAS PubMed
.
- A. J. Hughes, D. P. Spelke, Z. Xu, C.-C. Kang, D. V. Schaffer and A. E. Herr, Nat. Methods, 2014, 11, 749–755 CrossRef CAS PubMed
.
- C.-C. Kang, J.-M. G. Lin, Z. Xu, S. Kumar and A. E. Herr, Anal. Chem., 2014, 86, 10429–10436 CrossRef CAS PubMed
.
- C.-C. Kang, K. A. Yamauchi, J. Vlassakis, E. Sinkala, T. A. Duncombe and A. E. Herr, Nat. Protoc., 2016, 11, 1508–1530 CrossRef PubMed
.
- E. Sinkala, E. Sollier-Christen, C. Renier, E. Rosàs-Canyelles, J. Che, K. Heirich, T. A. Duncombe, J. Vlassakis, K. A. Yamauchi, H. Huang, S. S. Jeffrey and A. E. Herr, Nat. Commun., 2017, 8, 14622 CrossRef CAS PubMed
.
- J. Vlassakis, L. L. Hansen, R. Higuchi-Sanabria, Y. Zhou, C. K. Tsui, A. Dillin, H. Huang and A. E. Herr, Nat. Commun., 2021, 12, 4969 CrossRef CAS PubMed
.
- J. J. Agresti, E. Antipov, A. R. Abate, K. Ahn, A. C. Rowat, J.-C. Baret, M. Marquez, A. M. Klibanov, A. D. Griffiths and D. A. Weitz, Proc. Natl. Acad. Sci. U. S. A., 2010, 107, 4004–4009 CrossRef CAS PubMed
.
- M. T. Guo, A. Rotem, J. A. Heyman and D. A. Weitz, Lab Chip, 2012, 12, 2146–2155 RSC
.
- L. Mazutis, J. Gilbert, W. L. Ung, D. A. Weitz, A. D. Griffiths and J. A. Heyman, Nat. Protoc., 2013, 8, 870–891 CrossRef CAS PubMed
.
- B. E. Debs, R. Utharala, I. V. Balyasnikova, A. D. Griffiths and C. A. Merten, Proc. Natl. Acad. Sci. U. S. A., 2012, 109, 11570–11575 CrossRef PubMed
.
- V. Chokkalingam, J. Tel, F. Wimmers, X. Liu, S. Semenov, J. Thiele, C. G. Figdor and W. T. S. Huck, Lab Chip, 2013, 13, 4740–4744 RSC
.
- L. Qiu, F. Wimmers, J. Weiden, H. A. Heus, J. Tel and C. G. Figdor, Chem. Commun., 2017, 53, 8066–8069 RSC
.
- F. Wimmers, N. Subedi, N. van Buuringen, D. Heister, J. Vivié, I. Beeren-Reinieren, R. Woestenenk, H. Dolstra, A. Piruska, J. F. M. Jacobs, A. van Oudenaarden, C. G. Figdor, W. T. S. Huck, I. J. M. de Vries and J. Tel, Nat. Commun., 2018, 9, 3317 CrossRef PubMed
.
- Y. Yuan, J. Brouchon, J. M. Calvo-Calle, J. Xia, L. Sun, X. Zhang, K. L. Clayton, F. Ye, D. A. Weitz and J. A. Heyman, Lab Chip, 2020, 20, 1513–1520 RSC
.
- M. N. Hsu, S.-C. Wei, S. Guo, D.-T. Phan, Y. Zhang and C.-H. Chen, Small, 2018, 14, 1802918 CrossRef PubMed
.
- C. Martino, M. Zagnoni, M. E. Sandison, M. Chanasakulniyom, A. R. Pitt and J. M. Cooper, Anal. Chem., 2011, 83, 5361–5368 CrossRef CAS PubMed
.
- R. Ding, K.-C. Hung, A. Mitra, L. W. Ung, D. Lightwood, R. Tu, D. Starkie, L. Cai, L. Mazutis, S. Chong, D. A. Weitz and J. A. Heyman, RSC Adv., 2020, 10, 27006–27013 RSC
.
- W. Wu, S. Zhang, T. Zhang and Y. Mu, ACS Appl. Mater. Interfaces, 2021, 13, 6081–6090 CrossRef CAS PubMed
.
- K. Eyer, R. C. L. Doineau, C. E. Castrillon, L. Briseño-Roa, V. Menrath, G. Mottet, P. England, A. Godina, E. Brient-Litzler, C. Nizak, A. Jensen, A. D. Griffiths, J. Bibette, P. Bruhns and J. Baudry, Nat. Biotechnol., 2017, 35, 977–982 CrossRef CAS PubMed
.
- M. Heo, G. Chenon, C. Castrillon, J. Bibette, P. Bruhns, A. D. Griffiths, J. Baudry and K. Eyer, Commun. Biol., 2020, 3, 614 CrossRef CAS PubMed
.
- T. Khajvand, P. Huang, L. Li, M. Zhang, F. Zhu, X. Xu, M. Huang, C. Yang, Y. Lu and Z. Zhu, Lab Chip, 2021, 21, 4823–4830 RSC
.
- C. M. Revankar, D. F. Cimino, L. A. Sklar, J. B. Arterburn and E. R. Prossnitz, Science, 2005, 307, 1625–1630 CrossRef CAS PubMed
.
- S. Ghaemmaghami, W.-K. Huh, K. Bower, R. W. Howson, A. Belle, N. Dephoure, E. K. O'Shea and J. S. Weissman, Nature, 2003, 425, 737–741 CrossRef CAS PubMed
.
- J. Liu, D. Yin, S. Wang, H.-Y. Chen and Z. Liu, Angew. Chem., Int. Ed., 2016, 55, 13215–13218 CrossRef CAS PubMed
.
- J. Liu, H. He, D. Xie, Y. Wen and Z. Liu, Nat. Protoc., 2021, 16, 3522–3546 CrossRef CAS PubMed
.
- N. L. Anderson, Clin. Chem., 2010, 56, 177–185 CrossRef CAS PubMed
.
- P. Feist and A. B. Hummon, Int. J. Mol. Sci., 2015, 16, 3537–3563 CrossRef CAS PubMed
.
- H. B. Gutstein, J. S. Morris, S. P. Annangudi and J. V. Sweedler, Mass Spectrom. Rev., 2008, 27, 316–330 CrossRef CAS PubMed
.
- Y. Zhu, P. D. Piehowski, R. Zhao, J. Chen, Y. Shen, R. J. Moore, A. K. Shukla, V. A. Petyuk, M. Campbell-Thompson, C. E. Mathews, R. D. Smith, W.-J. Qian and R. T. Kelly, Nat. Commun., 2018, 9, 882 CrossRef PubMed
.
- Y. Cong, Y. Liang, K. Motamedchaboki, R. Huguet, T. Truong, R. Zhao, Y. Shen, D. Lopez-Ferrer, Y. Zhu and R. T. Kelly, Anal. Chem., 2020, 92, 2665–2671 CrossRef CAS PubMed
.
- Y. Cong, K. Motamedchaboki, S. A. Misal, Y. Liang, A. J. Guise, T. Truong, R. Huguet, E. D. Plowey, Y. Zhu, D. Lopez-Ferrer and R. T. Kelly, Chem. Sci., 2021, 12, 1001–1006 RSC
.
- K. Kasuga, Y. Katoh, K. Nagase and K. Igarashi, Proteomics, 2017, 17, 1600420 CrossRef PubMed
.
- R. T. Kelly, Mol. Cell. Proteomics, 2020, 19, 1739–1748 CrossRef CAS PubMed
.
- N. A. Kulak, G. Pichler, I. Paron, N. Nagaraj and M. Mann, Nat. Methods, 2014, 11, 319–324 CrossRef CAS PubMed
.
- L. L. Manza, S. L. Stamer, A.-J. L. Ham, S. G. Codreanu and D. C. Liebler, Proteomics, 2005, 5, 1742–1745 CrossRef CAS PubMed
.
- C. S. Hughes, S. Foehr, D. A. Garfield, E. E. Furlong, L. M. Steinmetz and J. Krijgsveld, Mol. Syst. Biol., 2014, 10, 757 CrossRef PubMed
.
- M. Sielaff, J. Kuharev, T. Bohn, J. Hahlbrock, T. Bopp, S. Tenzer and U. Distler, J. Proteome Res., 2017, 16, 4060–4072 CrossRef CAS PubMed
.
- Q. Chen, G. Yan, M. Gao and X. Zhang, Anal. Chem., 2015, 87, 6674–6680 CrossRef CAS PubMed
.
- X. Shao, X. Wang, S. Guan, H. Lin, G. Yan, M. Gao, C. Deng and X. Zhang, Anal. Chem., 2018, 90, 14003–14010 CrossRef CAS PubMed
.
- Y. Zhu, R. Zhao, P. D. Piehowski, R. J. Moore, S. Lim, V. J. Orphan, L. Paša-Tolić, W.-J. Qian, R. D. Smith and R. T. Kelly, Int. J. Mass Spectrom., 2018, 427, 4–10 CrossRef CAS PubMed
.
- P. Xiang, Y. Zhu, Y. Yang, Z. Zhao, S. M. Williams, R. J. Moore, R. T. Kelly, R. D. Smith and S. Liu, Anal. Chem., 2020, 92, 4711–4715 CrossRef CAS PubMed
.
- J. Stadlmann, O. Hudecz, G. Krššáková, C. Ctortecka, G. Van Raemdonck, J. Op De Beeck, G. Desmet, J. M. Penninger, P. Jacobs and K. Mechtler, Anal. Chem., 2019, 91, 14203–14207 CrossRef CAS PubMed
.
- F. Meier, M. A. Park and M. Mann, Mol. Cell. Proteomics, 2021, 20, 100138 CrossRef CAS PubMed
.
- A.-D. Brunner, M. Thielert, C. Vasilopoulou, C. Ammar, F. Coscia, A. Mund, O. B. Hoerning, N. Bache, A. Apalategui, M. Lubeck, S. Richter, D. S. Fischer, O. Raether, M. A. Park, F. Meier, F. J. Theis and M. Mann, Mol. Syst. Biol., 2022, 18, e10798 CrossRef CAS PubMed
.
- C.-F. Tsai, R. Zhao, S. M. Williams, R. J. Moore, K. Schultz, W. B. Chrisler, L. Pasa-Tolic, K. D. Rodland, R. D. Smith, T. Shi, Y. Zhu and T. Liu, Mol. Cell. Proteomics, 2020, 19, 828–838 CrossRef CAS PubMed
.
- Y. Wang, T.-S. M. Lih, L. Chen, Y. Xu, M. D. Kuczler, L. Cao, K. J. Pienta, S. R. Amend and H. Zhang, Clin. Proteomics, 2022, 19, 24 CrossRef CAS PubMed
.
- B. Budnik, E. Levy, G. Harmange and N. Slavov, Genome Biol., 2018, 19, 161 CrossRef PubMed
.
- M. Dou, G. Clair, C.-F. Tsai, K. Xu, W. B. Chrisler, R. L. Sontag, R. Zhao, R. J. Moore, T. Liu, L. Pasa-Tolic, R. D. Smith, T. Shi, J. N. Adkins, W.-J. Qian, R. T. Kelly, C. Ansong and Y. Zhu, Anal. Chem., 2019, 91, 13119–13127 CrossRef CAS PubMed
.
- A. L. Christoforou and K. S. Lilley, Anal. Bioanal. Chem., 2012, 404, 1029–1037 CrossRef CAS PubMed
.
- J. J. O'Brien, J. D. O'Connell, J. A. Paulo, S. Thakurta, C. M. Rose, M. P. Weekes, E. L. Huttlin and S. P. Gygi, J. Proteome Res., 2018, 17, 590–599 CrossRef PubMed
.
- I. Barbulovic-Nad, H. Yang, P. S. Park and A. R. Wheeler, Lab Chip, 2008, 8, 519–526 RSC
.
- A. R. Wheeler, H. Moon, C.-J. Kim, J. A. Loo and R. L. Garrell, Anal. Chem., 2004, 76, 4833–4838 CrossRef CAS PubMed
.
- A. R. Wheeler, H. Moon, C. A. Bird, R. R. Ogorzalek Loo, C.-J. Kim, J. A. Loo and R. L. Garrell, Anal. Chem., 2005, 77, 534–540 CrossRef CAS PubMed
.
- H. Moon, A. R. Wheeler, R. L. Garrell, J. A. Loo and C.-J. Kim, Lab Chip, 2006, 6, 1213–1219 RSC
.
- V. N. Luk and A. R. Wheeler, Anal. Chem., 2009, 81, 4524–4530 CrossRef CAS PubMed
.
- H. Yang, J. M. Mudrik, M. J. Jebrail and A. R. Wheeler, Anal. Chem., 2011, 83, 3824–3830 CrossRef CAS PubMed
.
- J. Leipert and A. Tholey, Lab Chip, 2019, 19, 3490–3498 RSC
.
- M. K. Steinbach, J. Leipert, C. Blurton, M. Leippe and A. Tholey, J. Proteome Res., 2022, 21, 1986–1996 CrossRef CAS PubMed
.
- J. Leipert, M. K. Steinbach and A. Tholey, Anal. Chem., 2021, 93, 6278–6286 CrossRef CAS PubMed
.
- Z.-Y. Li, M. Huang, X.-K. Wang, Y. Zhu, J.-S. Li, C. C. L. Wong and Q. Fang, Anal. Chem., 2018, 90, 5430–5438 CrossRef CAS PubMed
.
- Y. Zhu, G. Clair, W. B. Chrisler, Y. Shen, R. Zhao, A. K. Shukla, R. J. Moore, R. S. Misra, G. S. Pryhuber, R. D. Smith, C. Ansong and R. T. Kelly, Angew. Chem., 2018, 130, 12550–12554 CrossRef
.
- S. M. Williams, A. V. Liyu, C.-F. Tsai, R. J. Moore, D. J. Orton, W. B. Chrisler, M. J. Gaffrey, T. Liu, R. D. Smith, R. T. Kelly, L. Pasa-Tolic and Y. Zhu, Anal. Chem., 2020, 92, 10588–10596 CrossRef CAS PubMed
.
-
C. Ctortecka, D. Hartlmayr, A. Seth, S. Mendjan, G. Tourniaire and K. Mechtler, bioRxiv, 2022, preprint, bioRxiv:2021.04.14.439828, DOI:10.1101/2021.04.14.439828.
- T. Masuda, Y. Inamori, A. Furukawa, M. Yamahiro, K. Momosaki, C.-H. Chang, D. Kobayashi, H. Ohguchi, Y. Kawano, S. Ito, N. Araki, S.-E. Ong and S. Ohtsuki, Anal. Chem., 2022, 94, 10329–10336 CrossRef CAS PubMed
.
-
Y. Wang, Z.-Y. Guan, S.-W. Shi, Y.-R. Jiang, Q. Wu, J. Wu, J.-B. Chen, W.-X. Ying, Q.-Q. Xu, Q.-X. Fan, H.-F. Wang, L. Zhou, J.-Z. Pan and Q. Fang, bioRxiv, 2022, preprint, bioRxiv:2022.06.28.498038, DOI:10.1101/2022.06.28.498038.
- J. R. Heath, A. Ribas and P. S. Mischel, Nat. Rev. Drug Discovery, 2016, 15, 204–216 CrossRef CAS PubMed
.
- T. Zhang, A. R. Warden, Y. Li and X. Ding, Clin. Transl. Med., 2020, 10, e206 CAS
.
- B. Ajami, N. Samusik, P. Wieghofer, P. P. Ho, A. Crotti, Z. Bjornson, M. Prinz, W. J. Fantl, G. P. Nolan and L. Steinman, Nat. Neurosci., 2018, 21, 541–551 CrossRef CAS PubMed
.
- M. H. Y. Chng, M. Q. Lim, A. Rouers, E. Becht, B. Lee, P. A. MacAry, D. C. Lye, Y. S. Leo, J. Chen, K. Fink, L. Rivino and E. W. Newell, Immunity, 2019, 51, 1119–1135 CrossRef CAS PubMed
, e5.
- X.-K. Lun, D. Szklarczyk, A. Gábor, N. Dobberstein, V. R. T. Zanotelli, J. Saez-Rodriguez, C. von Mering and B. Bodenmiller, Mol. Cell, 2019, 74, 1086–1102 CrossRef CAS PubMed
, e5.
- S. J. Im, M. Hashimoto, M. Y. Gerner, J. Lee, H. T. Kissick, M. C. Burger, Q. Shan, J. S. Hale, J. Lee, T. H. Nasti, A. H. Sharpe, G. J. Freeman, R. N. Germain, H. I. Nakaya, H.-H. Xue and R. Ahmed, Nature, 2016, 537, 417–421 CrossRef CAS PubMed
.
- K. J. Hiam-Galvez, B. M. Allen and M. H. Spitzer, Nat. Rev. Cancer, 2021, 21, 345–359 CrossRef CAS PubMed
.
- P. Neuperger, J. Á. Balog, L. Tiszlavicz, J. Furák, N. Gémes, E. Kotogány, K. Szalontai, L. G. Puskás and G. J. Szebeni, Cancers, 2022, 14, 144 CrossRef CAS PubMed
.
- J. Wang, D. Tham, W. Wei, Y. S. Shin, C. Ma, H. Ahmad, Q. Shi, J. Yu, R. D. Levine and J. R. Heath, Nano Lett., 2012, 12, 6101–6106 CrossRef CAS PubMed
.
- Q. Shi, L. Qin, W. Wei, F. Geng, R. Fan, Y. Shik Shin, D. Guo, L. Hood, P. S. Mischel and J. R. Heath, Proc. Natl. Acad. Sci. U. S. A., 2012, 109, 419–424 CrossRef CAS PubMed
.
- Q. Xue, E. Bettini, P. Paczkowski, C. Ng, A. Kaiser, T. McConnell, O. Kodrasi, M. F. Quigley, J. Heath, R. Fan, S. Mackay, M. E. Dudley, S. H. Kassim and J. Zhou, J. Immunother. Cancer., 2017, 5, 85 CrossRef PubMed
.
- L. Yang, Z. Wang, Y. Deng, Y. Li, W. Wei and Q. Shi, Anal. Chem., 2016, 88, 11077–11083 CrossRef CAS PubMed
.
- A. V. Ullal, V. Peterson, S. S. Agasti, S. Tuang, D. Juric, C. M. Castro and R. Weissleder, Sci. Transl. Med., 2014, 6, 219ra9 Search PubMed
.
- G. H. Meftahi, Z. Bahari, A. Zarei Mahmoudabadi, M. Iman and Z. Jangravi, Biochem. Mol. Biol. Educ., 2021, 49, 509–517 CrossRef CAS PubMed
.
- H. N. Joensson, M. L. Samuels, E. R. Brouzes, M. Medkova, M. Uhlén, D. R. Link and H. Andersson-Svahn, Angew. Chem., Int. Ed., 2009, 48, 2518–2521 CrossRef CAS PubMed
.
- T. Konry, I. Smolina, J. M. Yarmush, D. Irimia and M. L. Yarmush, Small, 2011, 7, 395–400 CrossRef CAS PubMed
.
- P. Shahi, S. C. Kim, J. R. Haliburton, Z. J. Gartner and A. R. Abate, Sci. Rep., 2017, 7, 44447 CrossRef CAS PubMed
.
- P. G. Schiro, M. Zhao, J. S. Kuo, K. M. Koehler, D. E. Sabath and D. T. Chiu, Angew. Chem., 2012, 124, 4696–4700 CrossRef
.
- R. Ramji, M. Wang, A. A. S. Bhagat, D. Tan Shao Weng, N. V. Thakor, C. Teck Lim and C.-H. Chen, Biomicrofluidics, 2014, 8, 034104 CrossRef PubMed
.
- K. K. Reza, S. Dey, A. Wuethrich, J. Wang, A. Behren, F. Antaw, Y. Wang, A. A. I. Sina and M. Trau, ACS Nano, 2021, 15, 11231–11243 CrossRef CAS PubMed
.
- Y. Zhu, M. Scheibinger, D. C. Ellwanger, J. F. Krey, D. Choi, R. T. Kelly, S. Heller and P. G. Barr-Gillespie, eLife, 2019, 8, e50777 CrossRef CAS PubMed
.
- N. Slavov, PLoS Biol., 2022, 20, e3001512 CrossRef CAS PubMed
.
- C. Furlan, R. A. M. Dirks, P. C. Thomas, R. C. Jones, J. Wang, M. Lynch, H. Marks and M. Vermeulen, Nat. Commun., 2019, 10, 1525 CrossRef PubMed
.
- A. L. Richards, M. Eckhardt and N. J. Krogan, Mol. Syst. Biol., 2021, 17, e8792 CrossRef CAS PubMed
.
- T. C. Branon, J. A. Bosch, A. D. Sanchez, N. D. Udeshi, T. Svinkina, S. A. Carr, J. L. Feldman, N. Perrimon and A. Y. Ting, Nat. Biotechnol., 2018, 36, 880–887 CrossRef CAS PubMed
.
- F. Liu, D. Morderer, M. C. Wren, S. A. Vettleson-Trutza, Y. Wang, B. E. Rabichow, M. R. Salemi, B. S. Phinney, B. Oskarsson, D. W. Dickson and W. Rossoll, Acta Neuropathol. Commun., 2022, 10, 22 CrossRef CAS PubMed
.
- X. Sun, H. Sun, X. Han, P.-C. Chen, Y. Jiao, Z. Wu, X. Zhang, Z. Wang, M. Niu, K. Yu, D. Liu, K. K. Dey, A. Mancieri, Y. Fu, J.-H. Cho, Y. Li, S. Poudel, T. C. Branon, A. Y. Ting and J. Peng, Anal. Chem., 2022, 94, 5325–5334 CrossRef CAS PubMed
.
- I. M. Lazar, J. Deng, M. A. Stremler and S. Ahuja, Microsyst. Nanoeng., 2019, 5, 1–16 CrossRef PubMed
.
- Á. Végvári, J. E. Rodriguez and R. A. Zubarev, Anal. Chem., 2022, 94, 9261–9269 CrossRef PubMed
.
- V. Petrosius and E. M. Schoof, Transl. Oncol., 2023, 27, 101556 CrossRef CAS PubMed
.
- A. Mund, A.-D. Brunner and M. Mann, Mol. Cell, 2022, 82, 2335–2349 CrossRef CAS PubMed
.
- R. Satija, J. A. Farrell, D. Gennert, A. F. Schier and A. Regev, Nat. Biotechnol., 2015, 33, 495–502 CrossRef CAS PubMed
.
- R. Xu, J. Tang, Q. Deng, W. He, X. Sun, L. Xia, Z. Cheng, L. He, S. You, J. Hu, Y. Fu, J. Zhu, Y. Chen, W. Gao, A. He, Z. Guo, L. Lin, H. Li, C. Hu and R. Tian, Anal. Chem., 2018, 90, 5879–5886 CrossRef CAS PubMed
.
- H. Peng, E. Zhu and Y. Zhang, Biomark. Res., 2022, 10, 59 CrossRef PubMed
.
- B. Liu, A. W. Bell, S. Paranjpe, W. C. Bowen, J. S. Khillan, J.-H. Luo, W. M. Mars and G. K. Michalopoulos, Hepatology, 2010, 52, 1060–1067 CrossRef CAS PubMed
.
- Y. Zhu, M. Dou, P. D. Piehowski, Y. Liang, F. Wang, R. K. Chu, W. B. Chrisler, J. N. Smith, K. C. Schwarz, Y. Shen, A. K. Shukla, R. J. Moore, R. D. Smith, W.-J. Qian and R. T. Kelly, Mol. Cell. Proteomics, 2018, 17, 1864–1874 CrossRef CAS PubMed
.
- P. D. Piehowski, Y. Zhu, L. M. Bramer, K. G. Stratton, R. Zhao, D. J. Orton, R. J. Moore, J. Yuan, H. D. Mitchell, Y. Gao, B.-J. M. Webb-Robertson, S. K. Dey, R. T. Kelly and K. E. Burnum-Johnson, Nat. Commun., 2020, 11, 8 CrossRef CAS PubMed
.
- Y. Kwon, P. D. Piehowski, R. Zhao, R. L. Sontag, R. J. Moore, K. E. Burnum-Johnson, R. D. Smith, W.-J. Qian, R. T. Kelly and Y. Zhu, Lab Chip, 2022, 22, 2869–2877 RSC
.
- A. Mund, F. Coscia, A. Kriston, R. Hollandi, F. Kovács, A.-D. Brunner, E. Migh, L. Schweizer, A. Santos, M. Bzorek, S. Naimy, L. M. Rahbek-Gjerdrum, B. Dyring-Andersen, J. Bulkescher, C. Lukas, M. A. Eckert, E. Lengyel, C. Gnann, E. Lundberg, P. Horvath and M. Mann, Nat. Biotechnol., 2022, 40, 1231–1240 CrossRef CAS PubMed
.
- T. H. Visal, P. den Hollander, M. Cristofanilli and S. A. Mani, Br. J. Cancer, 2022, 127, 173–184 CrossRef CAS PubMed
.
- A. I. Salter, R. G. Ivey, J. J. Kennedy, V. Voillet, A. Rajan, E. J. Alderman, U. J. Voytovich, C. Lin, D. Sommermeyer, L. Liu, J. R. Whiteaker, R. Gottardo, A. G. Paulovich and S. R. Riddell, Sci. Signaling, 2018, 11, eaat6753 CrossRef PubMed
.
- M. C. Ramello, I. Benzaïd, B. M. Kuenzi, M. Lienlaf-Moreno, W. M. Kandell, D. N. Santiago, M. Pabón-Saldaña, L. Darville, B. Fang, U. Rix, S. Yoder, A. Berglund, J. M. Koomen, E. B. Haura and D. Abate-Daga, Sci. Signaling, 2019, 12, eaap9777 CrossRef CAS PubMed
.
- A. A. Griffith, K. P. Callahan, N. G. King, Q. Xiao, X. Su and A. R. Salomon, J. Proteome Res., 2022, 21, 395–409 CrossRef CAS PubMed
.
- J. Rossi, P. Paczkowski, Y.-W. Shen, K. Morse, B. Flynn, A. Kaiser, C. Ng, K. Gallatin, T. Cain, R. Fan, S. Mackay, J. R. Heath, S. A. Rosenberg, J. N. Kochenderfer, J. Zhou and A. Bot, Blood, 2018, 132, 804–814 CrossRef CAS PubMed
.
- J. Y. Spiegel, S. Patel, L. Muffly, N. M. Hossain, J. Oak, J. H. Baird, M. J. Frank, P. Shiraz, B. Sahaf, J. Craig, M. Iglesias, S. Younes, Y. Natkunam, M. G. Ozawa, E. Yang, J. Tamaresis, H. Chinnasamy, Z. Ehlinger, W. Reynolds, R. Lynn, M. C. Rotiroti, N. Gkitsas, S. Arai, L. Johnston, R. Lowsky, R. G. Majzner, E. Meyer, R. S. Negrin, A. R. Rezvani, S. Sidana, J. Shizuru, W.-K. Weng, C. Mullins, A. Jacob, I. Kirsch, M. Bazzano, J. Zhou, S. Mackay, S. J. Bornheimer, L. Schultz, S. Ramakrishna, K. L. Davis, K. A. Kong, N. N. Shah, H. Qin, T. Fry, S. Feldman, C. L. Mackall and D. B. Miklos, Nat. Med., 2021, 27, 1419–1431 CrossRef CAS PubMed
.
- S. Li, B. D. Plouffe, A. M. Belov, S. Ray, X. Wang, S. K. Murthy, B. L. Karger and A. R. Ivanov, Mol. Cell. Proteomics, 2015, 14, 1672–1683 CrossRef CAS PubMed
.
- Y. Zhu, J. Podolak, R. Zhao, A. K. Shukla, R. J. Moore, G. V. Thomas and R. T. Kelly, Anal. Chem., 2018, 90, 11756–11759 CrossRef CAS PubMed
.
- J. G. Smith and R. E. Gerszten, Circulation, 2017, 135, 1651–1664 CrossRef CAS PubMed
.
- L. Lesser-Rojas, P. Ebbinghaus, G. Vasan, M.-L. Chu, A. Erbe and C.-F. Chou, Nano Lett., 2014, 14, 2242–2250 CrossRef CAS PubMed
.
- M. Shi, X. Geng, C. Wang and Y. Guan, Anal. Chem., 2019, 91, 11493–11496 CrossRef CAS PubMed
.
- R. S. Gaster, D. A. Hall, C. H. Nielsen, S. J. Osterfeld, H. Yu, K. E. Mach, R. J. Wilson, B. Murmann, J. C. Liao, S. S. Gambhir and S. X. Wang, Nat. Med., 2009, 15, 1327–1332 CrossRef CAS PubMed
.
- R. S. Gaster, L. Xu, S.-J. Han, R. J. Wilson, D. A. Hall, S. J. Osterfeld, H. Yu and S. X. Wang, Nat. Nanotechnol., 2011, 6, 314–320 CrossRef CAS PubMed
.
- J.-R. Lee, D. M. Magee, R. S. Gaster, J. LaBaer and S. X. Wang, Expert Rev. Proteomics, 2013, 10, 65–75 CrossRef CAS PubMed
.
-
X. Zhou, C.-C. Huang and D. A. Hall, in Biosensing and Nanomedicine X, International Society for Optics and Photonics, 2017, vol. 10352, p. 103520F Search PubMed
.
- T. Klein, W. Wang, L. Yu, K. Wu, K. L. M. Boylan, R. I. Vogel, A. P. N. Skubitz and J.-P. Wang, Biosens. Bioelectron., 2019, 126, 301–307 CrossRef CAS PubMed
.
- C.-C. Huang, P. Ray, M. Chan, X. Zhou and D. A. Hall, Biosens. Bioelectron., 2020, 169, 112362 CrossRef CAS PubMed
.
- C.-C. Huang, X. Zhou and D. A. Hall, Sci. Rep., 2017, 7, 45493 CrossRef CAS PubMed
.
- X. Zhou, E. Mai, M. Sveiven, C. Pochet, H. Jiang, C.-C. Huang and D. A. Hall, IEEE J. Solid-State Circuits, 2021, 56, 2171–2181 Search PubMed
.
- P. Liu, K. Skucha, M. Megens and B. Boser, IEEE Trans. Magn., 2011, 47, 3449–3451 Search PubMed
.
- A. A. Siyal, E. S.-W. Chen, H.-J. Chan, R. B. Kitata, J.-C. Yang, H.-L. Tu and Y.-J. Chen, Anal. Chem., 2021, 93, 17003–17011 CrossRef CAS PubMed
.
- C. Vanderaa and L. Gatto, Curr. Protoc., 2023, 3, e658 Search PubMed
.
- J. Derks, A. Leduc, G. Wallmann, R. G. Huffman, M. Willetts, S. Khan, H. Specht, M. Ralser, V. Demichev and N. Slavov, Nat. Biotechnol., 2022, 1–10 Search PubMed
.
- L. Sun, K. M. Dubiak, E. H. Peuchen, Z. Zhang, G. Zhu, P. W. Huber and N. J. Dovichi, Anal. Chem., 2016, 88, 6653–6657 CrossRef CAS PubMed
.
- T. Frank and S. Tay, Lab Chip, 2015, 15, 2192–2200 RSC
.
- M. Junkin, A. J. Kaestli, Z. Cheng, C. Jordi, C. Albayrak, A. Hoffmann and S. Tay, Cell Rep., 2016, 15, 411–422 CrossRef CAS PubMed
.
- C. Zhang, H.-L. Tu, G. Jia, T. Mukhtar, V. Taylor, A. Rzhetsky and S. Tay, Sci. Adv., 2019, 5, eaav7959 CrossRef CAS PubMed
.
- J. Lin, C. Jordi, M. Son, H. Van Phan, N. Drayman, M. F. Abasiyanik, L. Vistain, H.-L. Tu and S. Tay, Nat. Commun., 2019, 10, 3544 CrossRef PubMed
.
-
J. M. Fulcher, L. M. Markillie, H. D. Mitchell, S. M. Williams, K. M. Engbrecht, R. J. Moore, J. Cantlon-Bruce, J. W. Bagnoli, A. Seth, L. Paša-Tolić and Y. Zhu, bioRxiv, 2022, preprint, bioRxiv:2022.05.17.492137, DOI:10.1101/2022.05.17.492137.
- Y. Li, H. Li, Y. Xie, S. Chen, R. Qin, H. Dong, Y. Yu, J. Wang, X. Qian and W. Qin, Anal. Chem., 2021, 93, 14059–14067 CrossRef CAS PubMed
.
- Z. Xu, E. Heidrich-O'Hare, W. Chen and R. H. Duerr, Genome Biol., 2022, 23, 135 CrossRef CAS PubMed
.
- A. Gayoso, Z. Steier, R. Lopez, J. Regier, K. L. Nazor, A. Streets and N. Yosef, Nat. Methods, 2021, 18, 272–282 CrossRef CAS PubMed
.
- J. Lakkis, A. Schroeder, K. Su, M. Y. Y. Lee, A. C. Bashore, M. P. Reilly and M. Li, Nat. Mach. Intell., 2022, 1–13 Search PubMed
.
- J. R. Sempionatto, J. A. Lasalde-Ramírez, K. Mahato, J. Wang and W. Gao, Nat. Rev. Chem., 2022, 1–17 Search PubMed
.
Footnote |
† These authors contributed equally to this work. |
|
This journal is © The Royal Society of Chemistry 2023 |
Click here to see how this site uses Cookies. View our privacy policy here.