DOI:
10.1039/C9CC04750F
(Highlight)
Chem. Commun., 2019,
55, 9895-9903
Droplet microfluidics: from proof-of-concept to real-world utility?
Received
20th June 2019
, Accepted 17th July 2019
First published on 17th July 2019
Abstract
Droplet microfluidics constitutes a diverse and practical tool set that enables chemical and biological experiments to be performed at high speed and with enhanced efficiency when compared to conventional instrumentation. Indeed, in recent years, droplet-based microfluidic tools have been used to excellent effect in a range of applications, including materials synthesis, single cell analysis, RNA sequencing, small molecule screening, in vitro diagnostics and tissue engineering. Our 2011 Chemical Communications Highlight Article [Chem. Commun., 2011, 47, 1936–1942] reviewed some of the most important technological developments and applications of droplet microfluidics, and identified key challenges that needed to be addressed in the short term. In the current contribution, we consider the intervening eight years, and assess the contributions that droplet-based microfluidics has made to experimental science in its broadest sense.
Droplets: smaller, faster, better
The field of droplet microfluidics has evolved at a startling rate since its emergence in the scientific literature in 1997.1 The initial period of development saw an explosion of innovation in device design and function,2–5 and then settled into a period of sustained growth with diverse applications emerging across the chemical and biological sciences.6–8 It is fair to say that during the past eight years we have witnessed a coming-of-age, where a plethora of droplet-based structures, concepts, components and processes are now being adopted and leveraged by end-users to engender completely new science and innovation. Indeed, our 2011 article in this journal addressed developments in the first decade, focusing on the ‘emergence’ phase of the technology, with a significant emphasis on establishing functional components (such as droplet generators, mergers, splitters and traps), routes to controllable compartmentalisation, and application to simple biological and chemical problems.9 In the current article, we aim to survey the intervening eight years, with a focus on innovations in droplet manipulation and detection methods, and more importantly, on where droplet-based microfluidics has made the biggest impact in terms of enabling novel science. We hope to convey through this how droplet microfluidics has now become an established and go-to experimental system for many scientists with ‘real world’ problems to tackle.
To summarise briefly the advantages of droplet-based microfluidics, we note that these systems maintain the inherent advantages of all microfluidic systems, including low sample volumes, the facile integration of different functionalities and an exquisite control of heat and mass transport, whilst overcoming problems related to Taylor dispersion, surface–molecule interactions and slow mixing that plague continuous-flow (or single-phase) microfluidic systems. In addition, droplet-based microfluidic systems offer unique features, such as the ability to define and form ultra-small assay volumes, negligible cross-contamination and sample adsorption, and enhanced mixing due to chaotic advection.10 Of additional note are the exceptionally high droplet generation frequencies (up to hundreds of kHz), the ability to control the droplet payload in a rapid and efficient manner and access to sub-millisecond mixing times, lending the technique to ultra-high-throughput experimentation.
Although traditional droplet generation methods (based on crossflow structures, flow-focusing geometries and co-flow structures) are still heavily used and extremely effective in creating large numbers of monodisperse droplets, we have recently seen the emergence and adoption of other innovative techniques.6 For example, parallel droplet generators based on step emulsification have been used for the robust, scalable and high-throughput production of droplets. Such droplet-generators integrate a shallow microchannel containing two co-flowing immiscible fluids and an abrupt (or step-like) opening to a deeper and wider reservoir, and hold great promise for a range of production-scale and industrial applications.11 In addition, additive manufacturing (or 3D printing) technologies are beginning to show utility as rapid fabrication and prototyping tools for microfluidic devices, allowing the realisation of complex 3D geometries that cannot be obtained through the use of standard lithographic approaches.12 In this respect, “equipment-free” droplet generation and handling has emerged as a powerful new method, expanding applications of droplet microfluidic systems in resource-limited settings, and providing for field-deployable instruments.13 With a view to eradicating the complex peripheral architecture that is typically required to run a droplet microfluidic experiment, techniques such as vacuum-induced pumping,14 lid pumps (where positive and negative pressures are generated by pulling or pushing pumping lids)15 and confinement gradients (where channel height variations subject immiscible interfaces to gradients of confinement to generate droplets)16 are all useful in providing simple, reliable and portable platforms for droplet-based experimentation.
Droplet manipulations
The ability to precisely manipulate droplets after their generation is key in maximising their utility as reaction, assay or storage vessels. Despite the establishment and widespread adoption of a range of droplet manipulation tools discussed in our previous paper,9 there have been a number of interesting recent developments, as shown in Fig. 1a. For example, the controlled merging of droplets containing different reaction precursors is an elegant way to initiate and process reactions. Recently, hydrodynamic self-rectification, where pressure is geometrically controlled in a droplet trap and bypass network, has been used to trigger drop coalescence and confinement-guided breakup, and mimic functions traditionally provided by well-plates and pipettes.17 Additionally, laser-based heating has been used to robustly induce the fusion of droplets stabilised with thermally-responsive poly(N-isopropylacrylamide) (PNIPAM) microgels.18 Conversely, the controlled break-up of droplets is a key operation in many situations, and one that has recently been accomplished through the use of pneumatic valves,19 electrostatic potentials,20 and surface acoustic waves.21 Furthermore, rapid on-chip droplet dilution has been demonstrated using immobilised high-concentration mother droplets that fuse with incoming solvent droplets and output daughter droplets whose concentrations diminish in a logarithmic fashion with droplet number.22
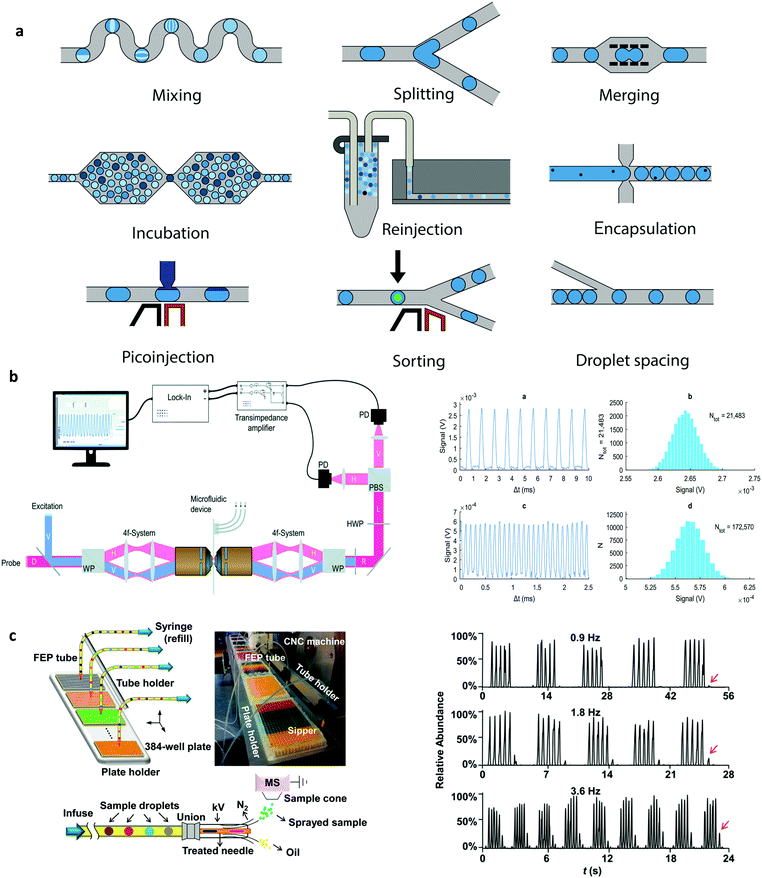 |
| Fig. 1 Droplet manipulations: (a) Unit operations that can be combined and arranged for the versatile control of droplets. Detection systems for droplet microfluidics: (b) Differential detection photothermal interferometry enables label free droplet detection at high throughput (>10 kHz). Reproduced in part from ref. 35, with permission from the Royal Society of Chemistry. (c) Electrospray ionization mass spectrometry for the high throughput study of enzyme inhibition. The droplet interface incorporates an elegant oil removal system prior to droplet analysis. Reproduced in part from ref. 43, with permission from the American Chemical Society. | |
The addition of material or reagents into droplets can also be achieved using a pico-injector.23 A pico-injector injects reagents directly into preformed droplets and operates in a similar fashion to electrically-mediated droplet fusion. When a droplet passes the pico-injector, electrodes opposite a channel containing reagent are energised, destabilising water–oil interfaces and allowing reagent to enter the droplet. As the droplet moves downstream, it remains connected to the orifice by a narrow bridge of fluid, which eventually breaks to form a new droplet. Since the entire process takes only a few milliseconds, pico-injectors have been used to excellent effect in the multiplexed analysis of enzyme kinetics,24 digital detection of RNA,25 and protein crystallisation screening.26 Finally, the ability to sort droplets based on their content is a necessary component of many biochemical assays, since sorting allows the selective capture and isolation of relevant droplets from extremely large populations. Critically, recent developments in droplet-sorting technologies have leveraged both passive and active strategies, including the use of viscoelastic fluid properties,27 electric fields,20 electric capacitance28 and magnetic rails,29 to allow for the rapid and robust selection of droplets with user-defined properties.
Small volume detection
A vital requirement of any chemical or biological experiment is the ability to identify and quantify relevant species within the assay volume. Detection within the pL volumes that characterise microfluidically produced droplets is especially challenging due to the dearth of sample present, and the fact that droplets within segmented flows are produced at high generation frequencies and are almost always moving at high speed through the system. Accordingly, the development and integration of sensitive detection methods has played and will continue to play a crucial role in ensuring that information retrieval rates match information generation rates. Further, some interesting and powerful detection modalities have recently emerged to complement traditional time-integrated fluorescence-based methods.
Optical detection
As noted, fluorescence-based methods are almost exclusively used to probe droplets in segmented flows. The exquisite sensitivity and selectivity of time-integrated emission spectroscopies are ideally suited to non-invasively probing pL-volume droplets produced at kHz frequencies and moving with high linear velocities.30 That said, much recent work has focused on integrating a range of novel spectroscopic methods able to extract larger amounts of chemical and biological information. For example, fluorescence lifetime imaging has been used to excellent effect to probe mixing phenomena within pL-volume droplets with a temporal resolution of 1 μs,31 and more recently fluorescence lifetime-activated droplet sorting has been introduced as a novel technology for droplet manipulations within segmented flows.32 More importantly, much recent effort has been focused on the use of label-free techniques for droplet detection. For example, both surface-enhanced Raman spectroscopy (SERS) and surface-enhanced Resonance Raman spectroscopy (SERRS) have been shown to allow for the rapid and sensitive analysis of droplet contents, yielding key structural information on contained analytes and with sub-millisecond time resolution.33 Additionally, developments in focal plane array detectors have facilitated the use of FT-IR spectroscopic imaging as an effective droplet interrogation tool able to study dynamic systems with a temporal resolution of 100 ms.34 Finally, exciting contemporary developments in photothermal spectroscopies have allowed the label-free detection of analytes in a volume-independent manner, with nM detection limits within fL-volume droplets at rates in excess of 10
000 droplets per second, as shown in Fig. 1b.35
Electrochemical analysis
Electrochemical detection is an attractive alternative to optical techniques when probing sub-nL-volume droplets.36 Electrochemical sensors are small, low-cost, sensitive and exhibit short response times, and despite the discontinuous nature of segmented flows (that causes the generation of transient electrochemical signals), a number of electrochemical approaches have been used to good effect in the analysis of electroactive species contained within nL-volume droplets. For example, capacitive sensors have been used to probe droplet size and speed, whilst also serving as key components in droplet sorting applications.37 In addition, potentiometry has been used to measure rapid kinetics in nL-volume droplets, with ion-selective electrodes being successfully used to probe RNA–Mg2+ binding kinetics with dead times as low as 60 ms.38 Alternatively, chronoamperometry has been shown to be highly adept at studying electrocatalytic reactions and intravenous drugs within nL-volume droplets,39,40 with concentration detection limits in the low μM to high nM regime.
Hyphenated techniques
The use of hyphenated detection platforms, where droplets within a segmented flow are transported off chip for analysis by an external instrument, can significantly enhance the accessible information content from a chemical or biological experiment (i.e., droplet). In this respect, much activity has recently focused on coupling droplet-based microfluidic platforms with mass spectrometers.41 Mass spectrometry (MS), in a range of embodiments, is an immensely powerful analytical tool, able to elucidate the chemical identity or structure of both small and large molecules. To this end, a number of recent studies have demonstrated the robust and efficient interfacing of droplet-based microfluidic platforms with both matrix-assisted laser desorption ionization-MS (MALDI-MS)42 and electrospray ionization-MS (ESI-MS)43 for the high-throughput analysis of proteins and enzymes down to femtomole levels (Fig. 1c). The utility of such hyphenated detection tools in contemporary biological experimentation is without doubt, and facilitated by a range of passive microfluidic strategies for oil removal prior to sample delivery to the mass spectrometer. Finally, it should not be forgotten that despite relatively poor detection sensitivities, nuclear magnetic resonance (NMR) spectroscopy is one of the most effective techniques for extracting information on molecular structure and dynamics. Unsurprisingly, much recent attention has focused on improving mass (and to a lesser extent concentration) sensitivities, with microcoils proving to be highly adept in measuring mass-limited samples.44 In this respect, a number of recent studies have demonstrated high-resolution NMR spectroscopy in pL–μL volumes45,46 and with pmole detection limits.47 Such advances suggest that contemporary NMR techniques may soon capable of extracting information from single (sub-nL) droplets.
Finding the killer app
The utility of droplet-based microfluidics has already been demonstrated in a tremendous array of applications. Whilst comprehensive reviews detailing many of these are available elsewhere,48 herein we highlight what we consider to be areas in which droplet-based microfluidic platforms have made the biggest impact in the last eight years.
Single-cell experimentation
The most significant applications of droplet-based microfluidics have almost certainly been in area of single-cell experimentation, where the challenge lies not just in analysing single cells, but in conducting high-throughput analysis of many thousands of individual cells. This is a task to which droplet microfluidics is perfectly suited.49 The simple fact that microfluidically-produced droplets are similar in size to human cells, means that they can be used to house, probe, manipulate and sort cells in a direct, rapid and large-scale fashion.50 Indeed, much early effort was focused on the realisation of functional components able to perform operations such as cell culturing, freezing, thawing, lysis, electroporation, incubation and gene delivery in aqueous and hydrogel droplets.48 However, the real power of droplet-based systems for single-cell experimentation lies in their ability to mediate extremely detailed and rapid experimentation in a highly parallel fashion. This promise has been realised through a range of elegant studies in recent years, resulting in formidable droplet-based platforms for a range of single-cell applications. Highlight achievements include the development of the inDrop51 and Drop-seq52 platforms, which allow low-cost droplet-based single cell RNA sequencing (Fig. 2). Other studies have demonstrated genomic,53 protein expression54 and metabolic55 analyses on individual cells encapsulated within droplets.56 Further, as single-cell heterogeneity has drawn much attention in molecular studies of differentiation,57 signalling58 and disease,59 there has been accompanying interest in droplet-barcoding for sequencing and profiling cells in multicellular organisms.60 Droplet-mediated single cell experimentation has also achieved impact in directed evolution of enzymes, where for example, droplet-based approaches have facilitated large-scale screening of the enzyme horseradish peroxidase, resulting in the identification of new mutants with catalytic rates more than ten times faster than their parent.61
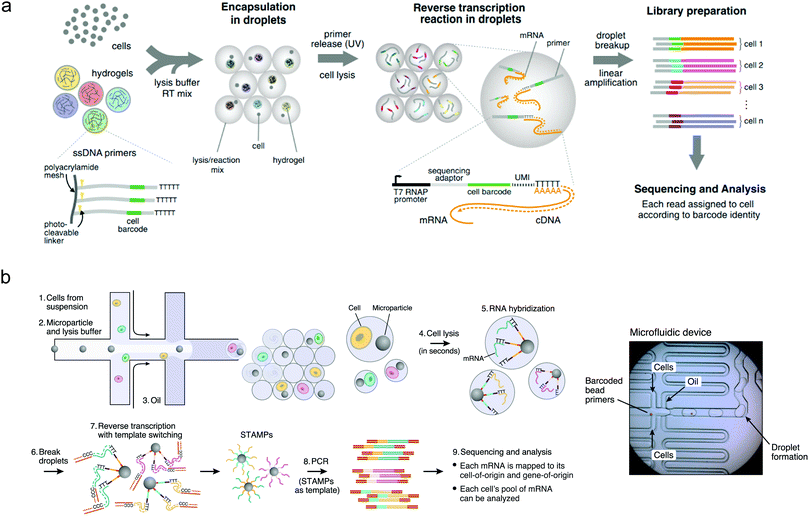 |
| Fig. 2 Droplet sequencing for high-throughput single-cell analysis: (a) Method based on the use of hydrogel barcodes containing DNA primers (inDrop) and (b) method based on microparticles (Drop-seq). Barcode or microparticles are delivered into droplets containing cells, lysis buffer and RT-PCR buffer, using a flow-focusing platform. Linear PCR is used for sequence analysis. Images adapted with permission from ref. 51 and 52, from Elsevier. | |
Nucleic acid detection and analysis
Droplet-based microfluidic systems provide an excellent technological platform for amplifying nucleic acids. In this regard, perhaps the most compelling application is digital droplet PCR (ddPCR), which enables extremely sensitive detection of specific nucleic acid sequences (down to the single copy level), and is based on the statistical analysis of a large number of compartmentalised PCR reactions.62,63 Put simply, ddPCR involves the segregation of a sample into millions of smaller volumes, which statistically will either be empty or will contain a single copy of target nucleic acid. Thermal cycling of such droplets will generate signal only in droplets that initially contained a copy of the sequence. Accordingly, both sequence identification and quantitation occur via a simple process of counting. Unsurprisingly, commercial products based on this approach are now available and widely used in molecular biology laboratories.64,65 That said, ddPCR often involves a number of complex off-chip operations, such as droplet incubation and droplet re-injection.62 In an attempt to simplify the amplification process, alternative isothermal amplification strategies have recently been explored. Such strategies include loop-mediated isothermal amplification (LAMP),66,67 rolling circle amplification (RCA),68 recombinase polymerase amplification (RPA)69 and exponential amplification reaction (EXPAR).70 Regardless of the specifics of each method, all operate at moderate temperatures (between room temperature and 60 °C) and do not require thermal cycling. Amongst these, LAMP has drawn the most attention due to its high sensitivity, high DNA product formation and reduced reaction times. In addition, using droplet LAMP (dLAMP) has a demonstrated benefit of enhanced inhibitor resistance compared to standard LAMP, and feasibility for point-of-care analysis.71,72 Another highlight from this growing body of works includes the performance of (the enzyme-free) hybridization chain reaction (HCR) in droplets for sensing miRNA at room temperature at rates of 500 detection events per minute.73
Droplet barcoding
The recognition and monitoring of specific droplets amongst much larger droplet populations is a particular challenge when performing large-scale experimentation. For relatively small populations, this can be achieved by simply ordering droplets along an extended channel or by catching droplets in trapping structures along the flow path.74,75 However, for populations comprising many thousands of droplets, such strategies are unfeasible and alternative methods must be employed. The addition of small molecule- or quantum dot-based fluorophore mixtures to droplets can enable barcoding of a few thousand droplets at any one time, but is limited by spectral crosstalk in the condensed phase.76 In contrast, DNA barcoding, which involves the delivery of unique single DNA strands to each droplet, yields an encoding capacity of 4n (where n is the number of nucleotides in the DNA sequence), and is therefore practically unlimited in its ability to barcode exceptionally large droplet populations.77 Accordingly, droplet barcode sequencing has become the state-of-the-art in barcoding technologies, for example in single-cell sequencing, as illustrated in Fig. 2a and b.78,79
Functional material synthesis
The benefits of droplet-based microfluidic reactors over both continuous-flow and flask-based methods for material synthesis are well-recognised,80 with features such as rapid mixing, controlled particle nucleation and growth and uniform heat transfer allowing the preparation of high-quality materials with bespoke properties. These features, allied with the ability to vary the chemical payload of individual droplets, enable a range of complex synthetic processes to be performed in high-throughput.81 The utility of droplets for producing monodisperse nanocrystalline materials has recently been showcased through the generation of a wide range of materials, including metal nanoparticles (such as Au,82 Ag83 and Pd84) ceramic nanomaterials,85 nanocomposites,86 quantum dots,87 organic nanomaterials,88 metal–organic frameworks89 and perovskite nanoparticles.90 In all cases, care has been paid to developing systems that can cope with extreme conditions, such as high temperatures and aggressive reagents. Despite the plethora of synthetic routes for their production, experimental validation of models for nanoparticle nucleation and growth has rarely been investigated due to the difficulties associated with extricating kinetic data on short timescales. In this regard, recent and exciting developments have leveraged droplet-based systems to uncover reaction dynamics and mechanisms associated with the synthesis of II–VI, III–V and IV–VI semiconductor nanocrystals and metal halide perovskites (Fig. 3a).91–93
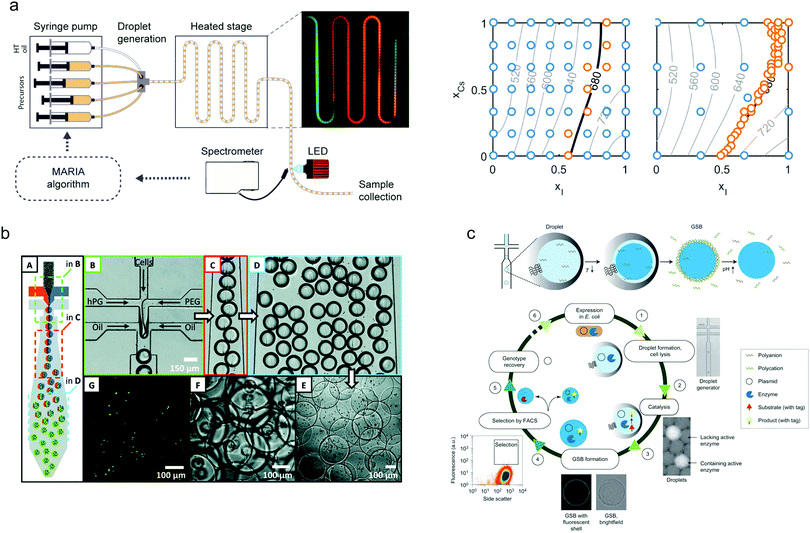 |
| Fig. 3 Nanoparticle synthesis using droplet microfluidics: (a) A Kriging algorithm-driven droplet reactor for targeted synthesis of lead halide perovskite nanocrystals; left hand side: schematic of the droplet-based capillary reactor, right hand side: a full parametric scan (left plot) compared with a targeted parametric scan (right plot). The algorithm-driven experiment, after an initial course scan of parameters (blue points), is able to effectively explore the parameter space to generate a range of nanocrystals emitting at 680 nm. Image reproduced from ref. 93, with permission from the American Chemical Society. Microgel synthesis using droplet microfluidics: (b) Cell-laden microgel synthesis using thiol–ene click reactions generates monodisperse particles under mild conditions. Image adapted from ref. 94, with permission from the American Chemical Society. (c) Synthesis of biomimetic gel beads, surrounded with a polyelectrolyte shell that enclose an enzyme, encoding DNA and a fluorescent product, for the directed evolution of phosphotriesterase. Image reproduced from ref. 95, with permission from Nature Springer. | |
Finally, droplet-based platforms have also been used to good effect in the synthesis of a range of functional biomaterials. Of particular note is the use of droplet-templated hydrogels for encapsulating cells (Fig. 3b),94 enzymes (Fig. 3c),95 DNA96 and even microorganisms.97 Significantly, encapsulation of cells and organisms within such droplets has been shown to improve long-term viability and enables efficient 3D cell culture98 and cell-based drug delivery.99 Moreover, the synthesis of proteinosomes (hollow self-assembled protein conjugates) has been demonstrated via a droplet approach, where proteinosome size distributions were significantly reduced when compared to the conventional approaches.100
Today and tomorrow
It is fair to say that almost two decades after its introduction, droplet-based microfluidics has now matured into a robust and powerful technology set, enabling the extremely rapid generation of high-quality chemical and biological information. In the concluding remarks of our 2011 article, we asked whether droplet-based platforms could “efficiently integrate large numbers of functional components within a single device to perform highly complex experiments” and could the “information generated be utilised by machining learning techniques to create “intelligent” systems able to direct and automate the experimentation process”. In the last eight years, both of these questions have been answered to a large degree. First, and as discussed herein, droplet-based microfluidic platforms now typically incorporate a large number of functional components that allow complex experiments on a range of chemical and biological systems. The statistical power of generating and analysing large numbers of compartmentalised assay volumes is a particular strength of droplet microfluidics, and one that can still be enhanced through the development of new methods that allow faster generation of uniformly-sized droplet populations. In this respect, step emulsification has shown great promise, and we anticipate that it will find significant future utility in diagnostic applications (e.g., ddPCR or isothermal amplification) where simple methods of droplet generation are key, and in a range of large-scale screening/selection applications, such as in directed evolution.61 Second, the last eight years has seen the emergence of artificial intelligence (AI) as a revolutionary tool in the chemical and biological sciences.101,102 As noted, such developments provide an exciting opportunity for droplet-based technologies, with the rapid data generation capabilities being well-matched to the advanced analytical capabilities of AI algorithms. Unsurprisingly, deep learning and reinforcement learning algorithms have already been used in conjunction with droplet-based microfluidic systems to good effect,103,104 with more conventional machining learning approaches being used, for example, to generate novel nanomaterials with user-defined properties.93 More generally, as large-scale droplet-based platforms (incorporating real-time analysis) develop, data volume and generation rates will greatly exceed the interpretive capabilities of conventional analytics, and thus the integration of deep learning algorithms that can rapidly analyse characterisation data and feed instructions back into the microfluidic system to control the nature or composition of new droplets will be crucial.
On a more technical level, maximising the amount of information that can be extracted from each droplet continues to be a challenge. Although, and as discussed, a range of novel and powerful detection methods are now being used, the routine application of high-content methods (such as those based on vibrational spectroscopies and electrochemical techniques) will likely be critical in engendering important new applications in the chemical and biological sciences, where conventional fluorescence-based detection schemes are inappropriate. Finally, and with a view to the application of droplet-based microfluidics in “out-of-lab” settings, the use of additive manufacturing techniques will become increasingly important in creating advanced microfluidic components.105 Indeed, the use of pumpless fluid manipulation schemes106 will likely allow the implementation of droplet-based analysis systems in resource-limited, remote and at-home settings in the short- to medium-term.
Final thoughts
Droplet-based microfluidics is now, without doubt, an important, robust and embedded technology platform within the chemical and biological sciences. Since our 2011 article, the field has greatly matured with respect to both technical features and the areas of application, with real-world success being evidenced through a range of mainstream commercial products that are being aggressively applied to key biological and healthcare related problems (e.g., 10× Genomics,107 Drop-seq108 and nucleic acid quantification via Droplet Digital™ PCR systems from Bio-Rad64). Although there is still so much potential to be unlocked, it is fair to say that droplets have now become (as we had hoped) an indispensable tool in chemical and biological research.
Conflicts of interest
There are no conflicts to declare.
Acknowledgements
A. S. N would like to thank the State Secretariat for Education, Research and Innovation (SERI) in Switzerland for a Swiss Government Excellence Scholarship (ESKAS No. 2016.0728). P. D. H. would like to acknowledge support from European Union's Horizon 2020 research and innovation program through the Individual Marie Sklodowska-Curie Fellowship “Ampidots” under grant agreement no. 701994.
References
- T. Kawakatsu, Y. Kikuchi and M. Nakajima, J. Am. Oil Chem. Soc., 1997, 74, 317–321 CrossRef CAS.
- H. Song, J. D. Tice and R. F. Ismagilov, Angew. Chem., Int. Ed., 2003, 42, 768–772 CrossRef CAS PubMed.
- T. Thorsen, R. W. Roberts, F. H. Arnold and S. R. Quake, Phys. Rev. Lett., 2001, 86, 4163–4166 CrossRef CAS PubMed.
- P. Poulin, H. Stark, T. Lubensky and D. Weitz, Science, 1997, 275, 1770–1773 CrossRef CAS PubMed.
- S. L. Anna, N. Bontoux and H. A. Stone, Appl. Phys. Lett., 2003, 82, 364–366 CrossRef CAS.
- O. J. Dressler, X. Casadevall i Solvas and A. J. deMello, Annu. Rev. Anal. Chem., 2017, 10, 1–24 CrossRef PubMed.
- Y. Ding, J. Choo and A. J. deMello, Microfluid. Nanofluid., 2017, 21, 58 CrossRef.
- D. T. Chiu, A. J. deMello, D. Di Carlo, P. S. Doyle, C. Hansen, R. M. Maceiczyk and R. C. Wootton, Chem, 2017, 2, 201–223 CAS.
- X. C. I. Solvas and A. J. deMello, Chem. Commun., 2011, 47, 1936–1942 RSC.
- Y. Zhu and Q. Fang, Anal. Chim. Acta, 2013, 787, 24–35 CrossRef CAS PubMed.
- M. L. Eggersdorfer, H. Seybold, A. Ofner, D. A. Weitz and A. R. Studart, Proc. Natl. Acad. Sci. U. S. A., 2018, 115, 9479–9484 CrossRef CAS PubMed.
- J. M. Zhang, A. A. Aguirre-Pablo, E. Q. Li, U. Buttner and S. T. Thoroddsen, RSC Adv., 2016, 6, 81120–81129 RSC.
- H. Tanaka, S. Yamamoto, A. Nakamura, Y. Nakashoji, N. Okura, N. Nakamoto, K. Tsukagoshi and M. Hashimoto, Anal. Chem., 2015, 87, 4134–4143 CrossRef CAS PubMed.
- J. R. Haliburton, S. C. Kim, I. C. Clark, R. A. Sperling, D. A. Weitz and A. R. Abate, Biomicrofluidics, 2017, 11, 034111 CrossRef CAS PubMed.
- S. Begolo, D. V. Zhukov, D. A. Selck, L. Li and R. F. Ismagilov, Lab Chip, 2014, 14, 4616–4628 RSC.
- R. Dangla, S. C. Kayi and C. N. Baroud, Proc. Natl. Acad. Sci. U. S. A., 2013, 110, 853–858 CrossRef CAS.
- S. S. Bithi, W. S. Wang, M. Sun, J. Blawzdziewicz and S. A. Vanapalli, Biomicrofluidics, 2014, 8, 034118 CrossRef PubMed.
- J. Sun, W. Wang, F. He, Z. H. Chen, R. Xie, X. J. Ju, Z. Liu and L. Y. Chu, RSC Adv., 2016, 6, 64182–64192 RSC.
- J. H. Choi, S. K. Lee, J. M. Lim, S. M. Yang and G. R. Yi, Lab Chip, 2010, 10, 456–461 RSC.
- R. de Ruiter, A. M. Pit, V. M. de Oliveira, M. H. G. Duits, D. van den Ende and F. Mugele, Lab Chip, 2014, 14, 883–891 RSC.
- J. H. Jung, G. Destgeer, B. Ha, J. Park and H. J. Sung, Lab Chip, 2016, 16, 3235–3243 RSC.
- X. Niu, F. Gielen, J. B. Edel and A. J. Demello, Nat. Chem., 2011, 3, 437–442 CrossRef CAS PubMed.
- B. O'Donovan, D. J. Eastburn and A. R. Abate, Lab Chip, 2012, 12, 4029–4032 RSC.
- S. L. Sjostrom, H. N. Joensson and H. A. Svahn, Lab Chip, 2013, 13, 1754–1761 RSC.
- D. J. Eastburn, A. Sciambi and A. R. Abate, PLoS One, 2013, 8, e62961 CrossRef CAS PubMed.
- S. B. Li, M. L. Zeng, T. Gaule, M. J. McPherson and F. C. Meldrum, Small, 2017, 13, 2372–2394 Search PubMed.
- A. C. Hatch, A. Patel, N. R. Beer and A. P. Lee, Lab Chip, 2013, 13, 1308–1315 RSC.
- A. M. Pit, R. de Ruiter, A. Kumar, D. Wijnperle, M. H. G. Duits and F. Mugele, Biomicrofluidics, 2015, 9, 044116 CrossRef PubMed.
- B. Teste, N. Jamond, D. Ferraro, J. L. Viovy and L. Malaquin, Microfluid. Nanofluid., 2015, 19, 141–153 CrossRef CAS.
- X. Casadevall i Solvas, X. Niu, K. Leeper, S. Cho, S.-I. Chang, J. B. Edel and A. J. deMello, J. Visualized Exp., 2011, e3437 Search PubMed.
- X. Casadevall i Solvas, M. Srisa-Art, A. J. deMello and J. B. Edel, Anal. Chem., 2010, 82, 3950–3956 CrossRef CAS PubMed.
- S. Hasan, D. Geissler, K. Wink, A. Hagen, J. J. Heiland and D. Belder, Lab Chip, 2019, 19, 403–409 RSC.
- E. Prado, A. Colin, L. Servant and S. Lecomte, J. Phys. Chem. C, 2014, 118, 13965–13971 CrossRef CAS.
- K. L. A. Chan, X. Niu, A. J. deMello and S. G. Kazarian, Anal. Chem., 2011, 83, 3606–3609 CrossRef CAS PubMed.
- R. M. Maceiczyk, D. Hess, F. W. Chiu and S. Stavrakis, Lab Chip, 2017, 17, 3654–3663 RSC.
- B.-K. Kim, A. Boika, J. Kim, J. E. Dick and A. J. Bard, J. Am. Chem. Soc., 2014, 136, 4849–4852 CrossRef CAS PubMed.
- C. Elbuken, T. Glawdel, D. Chan and C. L. Ren, Sens. Actuators, A, 2011, 171, 55–62 CrossRef CAS.
- Z. Y. Han, W. T. Li, Y. Y. Huang and B. Zheng, Anal. Chem., 2009, 81, 5840–5845 CrossRef CAS.
- A. Suea-Ngam, P. Rattanarat, O. Chailapakul and M. Srisa-Art, Anal. Chim. Acta, 2015, 883, 45–54 CrossRef CAS PubMed.
- A. Suea-Ngam, P. Rattanarat, K. Wongravee, O. Chailapakul and M. Srisa-Art, Talanta, 2016, 158, 134–141 CrossRef CAS PubMed.
- X. Feng, B. F. Liu, J. Li and X. Liu, Mass Spectrom. Rev., 2015, 34, 535–557 CrossRef CAS PubMed.
- F. Pereira, X. Z. Niu and A. J. deMello, PLoS One, 2013, 8, e63087 CrossRef CAS PubMed.
- S. Sun and R. T. Kennedy, Anal. Chem., 2014, 86, 9309–9314 CrossRef CAS PubMed.
- A. J. Oosthoek-deVries, J. Bart, R. M. Tiggelaar, J. W. Janssen, P. J. M. van Bentum, H. J. Gardeniers and A. P. Kentgens, Anal. Chem., 2017, 89, 2296–2303 CrossRef CAS PubMed.
- E. Montinaro, M. Grisi, M. Letizia, L. Pethö, M. Gijs, R. Guidetti, J. Michler, J. Brugger and G. Boero, PLoS One, 2018, 13, e0192780 CrossRef CAS PubMed.
- W. Hale, G. Rossetto, R. Greenhalgh, G. Finch and M. Utz, Lab Chip, 2018, 18, 3018–3024 RSC.
- M. Mompeán, R. M. Sánchez-Donoso, A. De La Hoz, V. Saggiomo, A. H. Velders and M. V. Gomez, Nat. Commun., 2018, 9, 108 CrossRef PubMed.
- L. Shang, Y. Cheng and Y. Zhao, Chem. Rev., 2017, 117, 7964–8040 CrossRef CAS PubMed.
- A. Huebner, M. Srisa-Art, D. Holt, C. Abell, F. Hollfelder, A. deMello and J. Edel, Chem. Commun., 2007, 1218–1220 RSC.
- H. N. Joensson and H. Andersson Svahn, Angew. Chem., Int. Ed., 2012, 51, 12176–12192 CrossRef CAS PubMed.
- A. M. Klein, L. Mazutis, I. Akartuna, N. Tallapragada, A. Veres, V. Li, L. Peshkin, D. A. Weitz and M. W. Kirschner, Cell, 2015, 161, 1187–1201 CrossRef CAS PubMed.
- E. Z. Macosko, A. Basu, R. Satija, J. Nemesh, K. Shekhar, M. Goldman, I. Tirosh, A. R. Bialas, N. Kamitaki and E. M. Martersteck, Cell, 2015, 161, 1202–1214 CrossRef CAS PubMed.
- A. Rotem, O. Ram, N. Shoresh, R. A. Sperling, A. Goren, D. A. Weitz and B. E. Bernstein, Nat. Biotechnol., 2015, 33, 1165–1172 CrossRef CAS PubMed.
- P. A. Romero, T. M. Tran and A. R. Abate, Proc. Natl. Acad. Sci. U. S. A., 2015, 112, 7159–7164 CrossRef CAS PubMed.
- F. Del Ben, M. Turetta, G. Celetti, A. Piruska, M. Bulfoni, D. Cesselli, W. T. Huck and G. Scoles, Angew. Chem., Int. Ed., 2016, 128, 8723–8726 CrossRef.
- L. Mazutis, J. Gilbert, W. L. Ung, D. A. Weitz, A. D. Griffiths and J. A. Heyman, Nat. Protoc., 2013, 8, 870–891 CrossRef CAS PubMed.
- G. X. Zheng, J. M. Terry, P. Belgrader, P. Ryvkin, Z. W. Bent, R. Wilson, S. B. Ziraldo, T. D. Wheeler, G. P. McDermott and J. Zhu, Nat. Commun., 2017, 8, 14049 CrossRef CAS PubMed.
- F. Wimmers, N. Subedi, N. van Buuringen, D. Heister, J. Vivié, I. Beeren-Reinieren, R. Woestenenk, H. Dolstra, A. Piruska and J. F. Jacobs, Nat. Commun., 2018, 9, 3317 CrossRef PubMed.
- E. Papalexi and R. Satija, Nat. Rev. Immunol., 2018, 18, 35–45 CrossRef CAS PubMed.
- C. T. Fincher, O. Wurtzel, T. de Hoog, K. M. Kravarik and P. W. Reddien, Science, 2018, 360, eaaq1736 CrossRef PubMed.
- J. J. Agresti, E. Antipov, A. R. Abate, K. Ahn, A. C. Rowat, J.-C. Baret, M. Marquez, A. M. Klibanov, A. D. Griffiths and D. A. Weitz, Proc. Natl. Acad. Sci. U. S. A., 2010, 107, 4004–4009 CrossRef CAS PubMed.
- Y. Schaerli, R. C. Wootton, T. Robinson, V. Stein, C. Dunsby, M. A. Neil, P. M. French, A. J. deMello, C. Abell and F. Hollfelder, Anal. Chem., 2009, 81, 302–306 CrossRef CAS PubMed.
- M. M. Kiss, L. Ortoleva-Donnelly, N. R. Beer, J. Warner, C. G. Bailey, B. W. Colston, J. M. Rothberg, D. R. Link and J. H. Leamon, Anal. Chem., 2008, 80, 8975–8981 CrossRef CAS PubMed.
- Bio-Rad, http://www.bio-rad.com/en-ch/applications-technologies/droplet-digital-pcr-ddpcr-technology, (accessed June 2019).
- M. Baker, Nature, 2012, 9, 541–544 CAS.
- J. Rodriguez-Manzano, M. A. Karymov, S. Begolo, D. A. Selck, D. V. Zhukov, E. Jue and R. F. Ismagilov, ACS Nano, 2016, 10, 3102–3113 CrossRef CAS PubMed.
- Y.-D. Ma, K. Luo, W.-H. Chang and G.-B. Lee, Lab Chip, 2018, 18, 296–303 RSC.
- M. S. Hede, S. Fjelstrup, F. Lötsch, R. M. Zoleko, A. Klicpera, M. Groger, J. Mischlinger, L. Endame, L. Veletzky and R. Neher, Sci. Rep., 2018, 8, 4122 CrossRef PubMed.
- E.-C. Yeh, C.-C. Fu, L. Hu, R. Thakur, J. Feng and L. P. Lee, Sci. Adv., 2017, 3, e1501645 CrossRef PubMed.
- K. Zhang, D.-K. Kang, M. M. Ali, L. Liu, L. Labanieh, M. Lu, H. Riazifar, T. N. Nguyen, J. A. Zell and M. A. Digman, Lab Chip, 2015, 15, 4217–4226 RSC.
- G. Nixon, J. A. Garson, P. Grant, E. Nastouli, C. A. Foy and J. F. Huggett, Anal. Chem., 2014, 86, 4387–4394 CrossRef CAS PubMed.
- X. Lin, X. Huang, K. Urmann, X. Xie and M. R. Hoffmann, ACS Sens., 2019, 4, 242–249 CrossRef CAS PubMed.
- S. Guo, W. N. Lin, Y. Hu, G. Sun, D.-T. Phan and C.-H. Chen, Lab Chip, 2018, 18, 1914–1920 RSC.
- R. R. Pompano, W. Liu, W. Du and R. F. Ismagilov, Annu. Rev. Anal. Chem., 2011, 4, 59–81 CrossRef CAS PubMed.
- W. Shi, J. Qin, N. Ye and B. Lin, Lab Chip, 2008, 8, 1432–1435 RSC.
- X.-H. Ji, W. Cheng, F. Guo, W. Liu, S.-S. Guo, Z.-K. He and X.-Z. Zhao, Lab Chip, 2011, 11, 2561–2568 RSC.
- N. Shembekar, C. Chaipan, R. Utharala and C. A. Merten, Lab Chip, 2016, 16, 1314–1331 RSC.
- P. D. Hebert, A. Cywinska and S. L. Ball, Proc. R. Soc. London, Ser. B, 2003, 270, 313–321 CrossRef CAS PubMed.
- E. Coissac, P. M. Hollingsworth, S. Lavergne and P. Taberlet, Mol. Ecol., 2016, 25, 1423–1428 CrossRef CAS PubMed.
- S. Marre and K. F. Jensen, Chem. Soc. Rev., 2010, 39, 1183–1202 RSC.
- Y. Zhao, Y. Cheng, L. Shang, J. Wang, Z. Xie and Z. Gu, Small, 2015, 11, 151–174 CrossRef CAS PubMed.
- S. Abalde-Cela, P. Taladriz-Blanco, M. G. de Oliveira and C. Abell, Sci. Rep., 2018, 8, 2440 CrossRef PubMed.
- L. Xu, J. Peng, M. Yan, D. Zhang and A. Q. Shen, Chem. Eng. Process., 2016, 102, 186–193 CrossRef CAS.
- G. Niu, A. Ruditskiy, M. Vara and Y. Xia, Chem. Soc. Rev., 2015, 44, 5806–5820 RSC.
- J. B. Wacker, I. Lignos, V. K. Parashar and M. A. Gijs, Lab Chip, 2012, 12, 3111–3116 RSC.
- T. Gu, C. Zheng, F. He, Y. Zhang, S. A. Khan and T. A. Hatton, Lab Chip, 2018, 18, 1330–1340 RSC.
- A. Nightingale, S. Krishnadasan, D. Berhanu, X. Niu, C. Drury, R. McIntyre and E. Valsami-Jones, Lab Chip, 2011, 11, 1221–1227 RSC.
- V. Singh, S. Jang, N. K. Vishwakarma and D.-P. Kim, NPG Asia Mater., 2018, 10, e456 CrossRef.
- M. Faustini, J. Kim, G. Y. Jeong, J. Y. Kim, H. R. Moon, W. S. Ahn and D. P. Kim, J. Am. Chem. Soc., 2013, 135, 14619–14626 CrossRef CAS PubMed.
- I. Lignos, V. Morad, Y. Shynkarenko, C. Bernasconi, R. M. Maceiczyk, L. Protesescu, F. Bertolotti, S. Kumar, S. T. Ochsenbein, N. Masciocchi, A. Guagliardi, C.-J. Shih, M. I. Bodnarchuk, A. J. deMello and M. V. Kovalenko, ACS Nano, 2018, 12, 5504–5517 CrossRef CAS PubMed.
- M. Abolhasani, C. W. Coley, L. Xie, O. Chen, M. G. Bawendi and K. F. Jensen, Chem. Mater., 2015, 27, 6131–6138 CrossRef CAS.
- I. Lignos, S. Stavrakis, G. Nedelcu, L. Protesescu, A. J. deMello and M. V. Kovalenko, Nano Lett., 2016, 16, 1869–1877 CrossRef CAS PubMed.
- L. Bezinge, R. M. Maceiczyk, I. Lignos, M. V. Kovalenko and A. J. deMello, ACS Appl. Mater. Interfaces, 2018, 10, 18869–18878 CrossRef CAS PubMed.
- T. Rossow, J. A. Heyman, A. J. Ehrlicher, A. Langhoff, D. A. Weitz, R. Haag and S. Seiffert, J. Am. Chem. Soc., 2012, 134, 4983–4989 CrossRef CAS PubMed.
- M. Fischlechner, Y. Schaerli, M. F. Mohamed, S. Patil, C. Abell and F. Hollfelder, Nat. Chem., 2014, 6, 791–796 CrossRef CAS PubMed.
- E. Y. Basova and F. Foret, Analyst, 2015, 140, 22–38 RSC.
- G. Aubry, M. Zhan and H. Lu, Lab Chip, 2015, 15, 1424–1431 RSC.
- S. Utech, R. Prodanovic, A. S. Mao, R. Ostafe, D. J. Mooney and D. A. Weitz, Adv. Healthcare Mater., 2015, 4, 1628–1633 CrossRef CAS PubMed.
- J. Wan, Polymers, 2012, 4, 1084–1108 CrossRef CAS.
- M. Ugrinic, A. Zambrano, S. Berger, S. Mann and T.-Y. D. Tang, Chem. Commun., 2018, 54, 287–290 RSC.
- K.-H. Yu, A. L. Beam and I. S. Kohane, Nat. Biomed. Eng., 2018, 2, 719–731 CrossRef PubMed.
- S. Webb, Nature, 2018, 554, 555–557 CrossRef CAS PubMed.
- J. Riordon, D. Sovilj, S. Sanner, D. Sinton and E. W. Young, Trends Biotechnol., 2018, 37, 310–324 CrossRef PubMed.
- O. J. Dressler, P. D. Howes, J. Choo and A. J. deMello, ACS Omega, 2018, 3, 10084–10091 CrossRef CAS.
- S. Waheed, J. M. Cabot, N. P. Macdonald, T. Lewis, R. M. Guijt, B. Paull and M. C. Breadmore, Lab Chip, 2016, 16, 1993–2013 RSC.
- B. Zhao, X. Cui, W. Ren, F. Xu, M. Liu and Z.-G. Ye, Sci. Rep., 2017, 7, 11319 CrossRef PubMed.
- 10X Genomics, http://www.10xgenomics.com, (accessed June 2019).
- McCarroll, http://mccarrolllab.org/dropseq/, (accessed June 2019).
|
This journal is © The Royal Society of Chemistry 2019 |
Click here to see how this site uses Cookies. View our privacy policy here.