DOI:
10.1039/D4AN00846D
(Minireview)
Analyst, 2024, Advance Article
New insights into lipid and fatty acid metabolism from Raman spectroscopy
Received
13th June 2024
, Accepted 3rd September 2024
First published on 5th September 2024
Abstract
One of the challenges facing biology is to understand metabolic events at a single cellular level. While approaches to examine dynamics of protein distribution or report on spatiotemporal location of signalling molecules are well-established, tools for the dissection of metabolism in single living cells are less common. Advances in Raman spectroscopy, such as stimulated Raman scattering (SRS), are beginning to offer new insights into metabolic events in a range of experimental systems, including model organisms and clinical samples, and across a range of disciplines. Despite the power of Raman imaging, it remains a relatively under-used technique to approach biological problems, in part because of the specialised nature of the analysis. To raise the profile of this method, here we consider some key studies which illustrate how Raman spectroscopy has revealed new insights into fatty acid and lipid metabolism across a range of cellular systems. The powerful and non-invasive nature of this approach offers a new suite of tools for biomolecular scientists to address how metabolic events within cells informs on or underpins biological function. We illustrate potential biological applications, discuss some recent advances, and offer a direction of travel for metabolic research in this area.
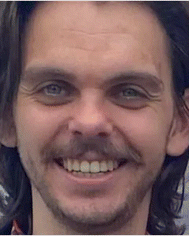 Justin C. Greig | Justin Greig is a PhD student at the Strathclyde Institute of Pharmacy and Biomedical Sciences where he studies aspects of adipocyte metabolism, in particular the mechanisms of adipocyte browning and the role of zinc alpha-2 glycoprotein in regulating adipocyte function. |
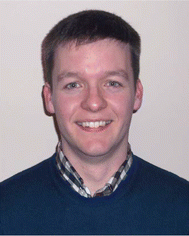 William J. Tipping | William Tipping obtained his PhD in Chemistry from the University of Edinburgh in 2017 under the guidance of Prof Alison Hulme and Prof Valerie Brunton. After a brief postdoctoral position in the same group, he joined the University of Strathclyde in 2019 to work with Prof Duncan Graham and Prof Karen Faulds. His research interests include the development of imaging probes for Raman microscopy, and the development of stimulated Raman scattering microscopy for biomedical imaging applications. |
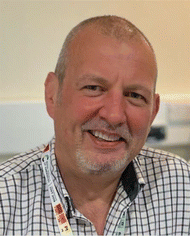 Duncan Graham | Duncan Graham is an expert in bioanalytical measurements with interests in Raman and advanced Raman spectroscopy techniques to provide new capability to life scientists. He graduated with a PhD in bioorganic chemistry from the University of Edinburgh and is an Associate Principal and Dean of the Faculty of Science at the University of Strathclyde. He was previously chair of the publishing board and an appointed trustee for the Royal Society of Chemistry. |
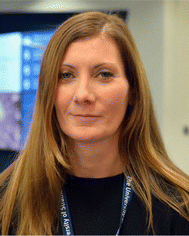 Karen Faulds | Karen Faulds is an expert in analytical spectroscopy, in particular Raman spectroscopy. She has developed several new assays for use in the life sciences based on Raman spectroscopy. She holds the post of Distinguished Professor at the University of Strathclyde and is the Head of Bionanotechnology and Analytical Chemistry. She holds a PhD from the University of Strathclyde and is an elected member of the Royal Society of Chemistry's Analytical Community Council. |
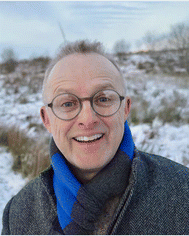 Gwyn W. Gould | Gwyn Gould is Professor of Cell Biology at the Strathclyde Institute of Pharmacy and Biomedical Sciences. His laboratory has a long-standing interest in the role of insulin on adipocyte function with an emphasis on the regulation of glucose transport, and in understanding defective insulin action in type-2 diabetes. |
1. Introduction
1.1 No two cells are the same
Driven initially by the application of single cell sequencing technologies, cellular heterogeneity is now a widely accepted feature of almost all cell types.1–5 The study of multicellular tissue dynamics has reached a new frontier as it is recognised that individual cells possess unique characteristics indicative of distinct functional roles, and that this cellular variation has profound implications for understanding cell/tissue function and for disease development.2,5–7 Using stains, antibodies, or specific labels for (a) protein(s) of interest, fluorescence microscopy has provided a step-change in knowledge and understanding of biological complexity even within a tissue composed of supposedly similar cells. By contrast, our understanding of the metabolic processes localised to specific cells within a tissue remains ill-defined. This is largely because of difficulties in identifying specific chemical moieties in living cells by means other than using specific engineered reporter molecules (e.g. such as those described for NAD+ and ATP8,9), and the limitation of population averaging effects in heterogeneous cell populations. Hence, key details of metabolic profiles within cells remain obscure. The ability to use Raman imaging, with or without non-invasive probes generating signals in the Raman spectrum, offers a way forward to resolve these issues. This has been a particularly fruitful avenue of research for those interested in fatty acid and lipid metabolism. Here we will consider how Raman has advanced this area and speculate on future developments (Fig. 1).
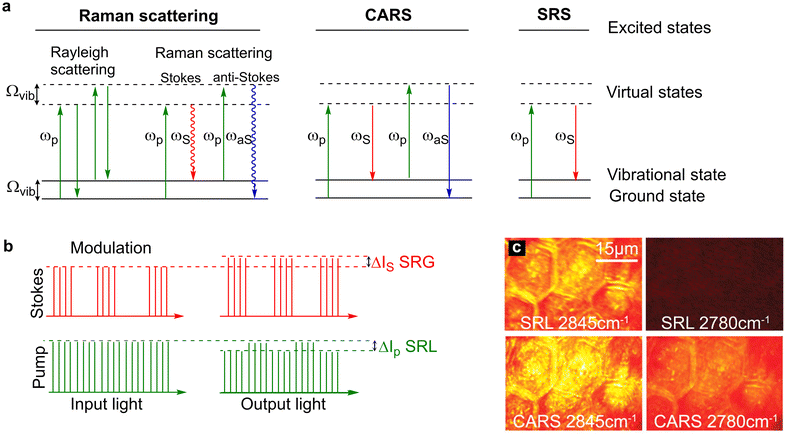 |
| Fig. 1 Energy level diagrams for Rayleigh scattering, Raman scattering, CARS and SRS. (a) Rayleigh scattering is an elastic process whereby photons are scattered with the same energy as the incident photons. Raman scattering is an inelastic process where photons are scattered with a lower energy (Stokes scattering) or higher energy (anti-Stokes scattering) relative to the incident photons. In CARS, two incident photons at the pump (ωp) and Stokes (ωS) frequencies simultaneously interact with the chemical bond which has a vibrational frequency that is equal to the difference frequency of the two beams. In doing so, the chemical bond is stimulated to a vibrational excited state. Upon interaction of the coherently excited chemical bond with a second pump photon results in the generation of an anti-Stokes photon (ωaS) which is blue shifted (higher energy than the incident photons). In SRS, two photons interact with a chemical bond such that the frequency difference between the pump and Stokes beams matches the energy of the chemical bond. In doing so, the energy of the pump photon is transferred to the chemical bond resulting in the generation of a Stokes photon with a lower energy. (b) Modulation transfer detection scheme. The Stokes beam is modulated at a high frequency which results in a SRL signal that is modulated in the pump beam and a SRG signal that is detected at the modulation frequency in the Stokes beam. The SRL signal is usually extracted from the laser noise using a lock-in amplifier. (c) A comparison of SRS and CARS imaging of mouse skin. The CH2 vibration of skin lipids is detected at 2845 cm−1, whilst the off-resonance image at 2780 cm−1 is free from signal in SRS imaging, non-resonant background is detected in the CARS image. Image adapted and reproduced from Ref. 39 with permission the American Association for the Advancement of Science (2008). | |
1.2 Lipid and fatty acid metabolism
The storage, release and breakdown of fatty acids play key roles across many facets of physiology. Adipocytes represent the best understood and most extensively studied cell type involved in such regulation, accumulating, esterifying, and storing excess fatty acids and lipids as triacylglycerides in lipid droplets.10,11 Distinctions between adipose tissue subtypes (e.g. white and brown adipocytes) are well established, but recent work has revealed considerable heterogeneity even within a single adipose depot.2,12–14 These differences appear to be developmentally programmed and are associated with differential susceptibility to diseases, including obesity and diabetes (for recent reviews, see ref. 11, 15 and 16). In addition to these depot-specific variations, adipocytes can respond to signals to prioritize lipid synthesis (lipogenic) or lipid breakdown (lipolytic) depending on the prevailing hormonal milieu.10,11
In addition to adipose tissue, other cell types can both utilise fatty acids for energy and exhibit the accumulation of excess lipid in the form of cytosolic lipid droplets.17–21 The liver plays a major role in lipid metabolism and is known to carefully integrate the distribution of fatty acids to other tissues and balance fatty acid utilisation. Non-alcoholic fatty liver disease arises because of lipid accumulation in hepatocytes exceeding fatty acid oxidation or export, resulting in the formation of lipid droplets within hepatocytes and impaired metabolic control.20,21 Like the liver, skeletal muscle uses fatty acids for energy, but in obesity, increased circulating fatty acid levels result in the accumulation of toxic lipid intermediates which drive oxidative stress. This is thought to underpin insulin resistance in muscle, an early stage in the development of type-2 diabetes.22–25 Such toxic accumulation of lipid droplets/lipid intermediates is also observed in the pancreatic beta cells of people with type-2 diabetes, resulting in aberrant insulin biosynthesis and release.17 Changes in lipid metabolism are also proposed to underlie changes in cancer cell function and may in turn modulate cell biology via changes in the plasma membrane lipidome.26,27 Hence, there is a need to quantify lipid/fatty acid metabolism in adipocytes and other cells, to understand how their metabolism changes in disease, and to develop approaches to allow the analysis of metabolic changes, ideally in real-time. Raman-based imaging has provided insight into adipose tissue structure and function, details of lipid composition, and has been shown to offer potential for either in vivo or intra-operative analysis (for recent reviews see ref. 26, 28 and 29).
Arguably, the most important aspect of Raman analysis is the ability to non-destructively image cells and study cellular events without the need for labels, genetic reporters or antibodies, hence the challenge is to integrate this with enhanced resolution and specificity of analysis. In the sections which follow, we provide a bird's-eye view of Raman imaging, then delve into specific examples where Raman-based approaches have provided new insight into fatty acid and/or lipid metabolism, illustrating these with a range of biological systems from human cells, flies, worms, fungi and bacteria chosen from the past two to three years to reflect the breadth of approaches and molecular insight which Raman can provide. We end with a speculative look to the future of the technique, highlighting some recent advances which offer great potential. We believe these approaches will be of wide general interest to the cell biology community, as the approaches discussed here are applicable across metabolism and in all kingdoms of biology.
2. What is Raman and SRS imaging?
As an aide to understanding a glossary of key terms is provided in Table 1. Readers familiar with Raman and SRS can skip ahead to section 3.
Table 1 Glossary of key terms
Rayleigh scattering |
Light scattering from a sample at the same frequency, and hence energy, as the incident radiation. |
Raman scattering |
Light scattered from a sample at a different frequency, and hence energy, as the incident radiation. |
Stokes and Anti-Stokes Raman scattering |
Inelastic scattering can result in radiation that is either scattered with a higher wavelength (lower frequency) and is referred to as Stokes Raman scattering, or radiation scattered with a lower wavelength (higher frequency) which is referred to anti-Stokes Raman scattering. |
Raman shift |
Raman spectra are reported as a shift in frequency of the scattered radiation with respect to the incident radiation. The incident radiation is typically a laser which would have a Ramn shift of 0 (i.e. elastic scattering). |
Wavenumber |
Wavenumber is a value proportional to the inverse of the wavelength in a harmonic wave. It is measured as the number of waves per unit length (centimetre), or cm−1. |
Spectral resolution |
The ability to resolve two peaks in a spectrum which is defined by the minimum wavenumber difference that can be distinguished. |
Spatial resolution |
The smallest physical distance between two measured positions within the sample. |
Chemometrics |
The application of mathematics or statistics to chemical data. |
Simulated Raman scattering (SRS) |
A nonlinear Raman scattering technique that uses a pump (ωp) and Stokes (ωs) laser which are tuned to a defined frequency representative of a specific molecular vibration (ωp − ωS). When this occurs, ωp observes a stimulated Raman loss in energy, and ωS observes a stimulated Raman gain. The pump beam is retuned to enable a different molecular vibration to be visualised. |
Coherent anti-Stokes Raman scattering (CARS) |
A nonlinear Raman scattering technique that uses two lasers, a pump (ωp) and Stokes (ωS) beam, such that the frequency difference between the two lasers is matched to a vibrational frequency in the target sample generating a strong anti-Stokes Raman signal (ωas = 2ωp-ωS). |
Hyperspectral imaging |
A three-dimensional dataset formed of x,y,λ coordinates. In hyperspectral imaging each pixel contains a spectral profile that can be used for molecular characterisation. |
Spectral phasor analysis |
A Fourier transform-based technique that represents a 3D hyperspectral image stack, as a 2D density plot in which each point – or phasor - represents a single spectrum in the original 3D dataset. |
Deconvolution (image) analysis |
Image processing technique used to improve the contrast and resolution of images captured using an optical microscope. Blurring is a common type of image distortion that can be overcome using deconvolution analysis. |
Multivariate analysis |
A branch of mathematics that deals with data that change in multiple ways with respect to a single dependent variable (a reference analyte value). |
Adam-based pointillism deconvolution (A-POD) |
This approach mimics the concept of pointillism painting (small, distinctive dots of colour are applied in patterns) and describes images with multiple discontinuous spots (virtual emitters). The virtual emitters have the same unit intensity, and the total number of emitters is fixed across the image. This characteristic allows for suppressing of imaging artefacts and describes the real emitters’ distribution. Therefore, from low-resolution images of high-density emitters, super-resolution images can be restored. |
2.1 A brief overview of Raman
Raman scattering is an optical technique that investigates the vibrational modes of molecules through inelastic scattering of the incident light source. As such, most Raman scattering experiments make use of a confocal Raman microscope coupled to a monochromatic light source, typically a laser at 405, 532, 633, 785 or 1064 nm. An objective lens is used to focus the laser onto the sample such that both elastic (referred to as Rayleigh scattering) and inelastic scattered photons (referred to as Raman scattering) are produced following the interaction with the molecules at the sample focus. Raman systems use an objective lens to focus the laser onto the sample and collect the scattered (both Rayleigh and Raman scattered) photons. By employing a spectrograph, the Stokes Raman scattered photons can be separated based on wavelength. By determining the wavelength shift in nm, which is usually expressed as a Raman shift, (ν, wavenumber cm−1), the resulting Raman spectrum can be used to identify molecular vibrations for molecular characterisation. As such, a Raman spectrum plots the wavelength shift (cm−1, x-axis) against the relative number of photon counts (usually counts per s, or equivalent, y-axis).
Raman imaging of lipids is an active area of research in part due to the numerous spectroscopic features for label-free characterisation of lipid type and composition. Perhaps the most characteristic Raman mode for lipid samples is the abundant CH2 stretches including the 2851 cm−1 (CH2 symmetric stretch) and 2881 cm−1 (CH2 asymmetric stretch), together with scissoring modes detected in the fingerprint region of the Raman spectrum (1440 cm−1).30 Fatty acid esterification can be readily detected in the Raman spectrum through C
O stretching at 1740 cm−1,31 whilst unsaturation of the alkyl chain is observed through C
C–H stretching at 3010 cm−1, C
C stretching at 1655 cm−1 and 1262 cm−1 (C
C–H deformation). An important area of development is the use of ratiometric Raman spectroscopy for molecular characterisation. As the Raman spectrum typically contains a high density of peaks for lipid species, it is possible to take the ratio of several peaks in order to characterise the lipid content in cellular lipid droplets, for example.32 Jamieson et al. demonstrated an increasing linear relationship between the intensity ratio 1262 cm−1/1440 cm−1 across a series of C18 fatty acids.33 Alternative lipid classes are readily detectable in the Raman spectrum including cholesterols, triacylglycerols and phospholipids.
2.2 CARS and SRS
In practice, Raman scattering is an intrinsically weak process, with approximately 1 in every 108 incident photons undergoing inelastic scattering.34 Therefore, the integration time per spectrum is usually slow (seconds) such that Raman imaging over an extended sample area is experimentally slow (mins to hours). In addition, it can be challenging to separate the unique molecular signature of the Raman scattering from the overwhelming Rayleigh scattered light or background fluorescence. Coherent Raman scattering (CRS) techniques aim to improve the scattering efficiency, and therefore detection sensitivity and/or imaging speed, through coherent excitation of the sample. The two most widely used CRS processes for microscopy are coherent anti-Stokes Raman scattering (CARS)35 and stimulated Raman scattering (SRS), although coherent Stokes Raman scattering (CSRS)36 has recently been reported for microscopy applications. A comparison of the three techniques discussed here is provided in Table 2.
Table 2 A comparison of spontaneous Raman scattering, CARS and SRS microscopy
|
Spontaneous Raman scattering |
CARS |
SRS |
Quantitative |
Yes |
Semi-quantitative |
Yes |
Image acquisition rate |
s per pixel |
μs per pixel |
μs per pixel |
In vivo analysis and/or live cell imaging |
Yes |
Yes |
Yes |
Spatial resolution (nm) |
∼500 |
∼300 |
∼300 |
Spectral resolution (cm−1) |
<1 |
∼10 |
∼10 |
Advantages |
Label-free, high spectral resolution, full or partial Raman spectrum at each pixel. |
Label-free, high spatial resolution, fast, hyperspectral imaging is feasible |
Label-free, high spatial resolution, fast, hyperspectral imaging is feasible, SRS spectrum matches Raman spectrum. |
Disadvantages |
Relatively slow imaging rate |
Non-resonant background, indirect quantification, complex spectral retrieval |
Some parasitic signals e.g. cross phase modulation can complicate imaging |
Coherent Raman scattering techniques require the use of two incident laser beams, termed the pump and Stokes laser. In most cases, the pump laser is a tuneable laser across the range 700–990 nm generated by an optical parametric oscillator, whilst the Stokes beam is a fixed wavelength laser, usually 1031, 1040 or 1064 nm. The two beams are spatially and temporally overlapped and focussed onto the sample. When the frequency difference between the two lasers is tuned to match a vibrational mode within the sample, coherent Raman scattering processes can occur.
CARS was first described in 196537 and generates an anti-Stokes scattering signal through four-wave mixing of the incident pump and Stokes lasers (Fig. 1a). When the difference in the frequencies of the pump and Stokes beams matches a molecular vibrational frequency (Ωvib) in the sample, a non-linear interaction between the incident photons causes vibrational resonance of the chemical bond in the sample. The excited vibrational chemical bond further interacts with an additional pump photon, resulting in the coherent generation of an anti-Stokes photon (ωas). The CARS signal is technically simple to detect using a photomultiplier tube because the signal is blue-shifted relative to the incident laser wavelengths, with the use of bandpass filters enabling the detection of the CARS signal. However, non-resonant background and resultant spectral distortions can occur during image acquisition. The nonlinear electronic response of the molecules at the laser focus can generate a non-resonant background signal in the absence of a vibrational resonance. The interference between non-resonant background and resonant vibrational signal can distort the CARS image and CARS spectrum thereby limiting the detection sensitivity and specificity.
SRS was first reported in 196238 although it was not until 2008 that the first demonstration of SRS microscopy for biological samples was realised.39 When the frequency difference between the pump and Stokes photons is matched to a vibrational frequency in the sample, stimulated Raman loss (SRL) is observed in the pump beam whilst stimulated Raman gain (SRG) is detected in the Stokes beam (Fig. 1b). The detection of SRS is achieved by detecting the SRL signal or SRG signal which is typically small (of the order 10−7 to 10−4) in the pump or Stokes beam40 respectively using a modulation transfer scheme via lock-in amplifier. Thus, when the frequency difference between the pump and Stokes beam does not match a vibrational frequency in the sample, there is no energy transfer and hence, SRL and SRG cannot occur. As such, SRS is free from non-resonant background and SRS spectra are free from spectral distortion unlike CARS, which makes SRS an attractive method for hyperspectral imaging applications for material characterisation (Fig. 1c).
Whilst SRL and SRG signals are bond-specific, these signals are weak and potentially lower than the fluctuation of laser noise, which posed a significant challenge for the implementation of SRS microscopy. As laser noise primarily occurs at low frequencies (generally <1 MHz), SRS microscopy is typically detected using high frequency modulation (>2 MHz). In most SRS systems, the Stokes beam is modulated at a frequency greater than 2 MHz and the pump beam remains unmodulated. Upon interaction with the target chemical bond in the sample, the SRL and SRG signals occur at the same frequency of modulation as the Stokes beam. Thus, it is possible to detect the SRL signal at the modulation frequency of the Stokes beam which is de-modulated via lock-in amplifier (Fig. 1b). Given that the Stokes beam is usually a fixed wavelength laser, in most cases, the SRL signal in the pump beam is detected, as the Stokes beam can be easily removed using a filter. An important advantage of SRS is that the SRL signal is directly proportional to the concentration of the target molecule in the sample enabling straightforward quantification. In addition, the SRS spectrum matches the Raman spectrum to enable direct sample characterisation. The detection sensitivity of SRS microscopy is ∼10 mM for endogenous biomolecules including C–H vibrations indicative of protein and lipid species.41 The development of bio-orthogonal Raman tagging has pushed the detection sensitivity by employing chemical groups like alkynes and nitriles which have a large Raman scattering cross section. Alternatively, the use of plasmonic materials including gold nanoarchitectures to enhance the SRS signal have been reported,42 together with the use of organic dyes to improve the detection sensitivity via electronic pre-resonance enhancement.43
2.3 Unmixing the data
Raman imaging methods typically acquire a full or partial Raman spectrum at pixel locations from a pre-defined sample area of interest. In doing so, the result is a three-dimensional dataset with coordinates of x and y for the spatial area and the spectral information contained in the Z axis. The creation of a Raman image from the intensity of a Raman band or ratio of multiple bands is easy to do. Alternatively, a wide variety of chemometric analysis techniques have been reported that have the capability to cluster Raman spectra or classify pixels within the image based on their spectral profile. Notable examples include principal component analysis (PCA), k-means cluster analysis (KMCA) and hierarchical cluster analysis amongst others. For a review of different spectral processing methods for vibrational spectroscopy, see ref. 44.
In the case of coherent Raman scattering techniques, vibrational spectroscopic information can be acquired to provide molecular compositional information on the sample. Typically, images are acquired across a portion of the spectrum by re-tuning the pump laser in between image frames. The resulting series of images can be stacked together, and each pixel location will contain a pseudo-Raman spectrum that can be used for molecular characterisation. This is referred to as hyperspectral imaging and is a popular method for SRS microscopy of biological samples. As with Raman scattering, several multivariate analysis methods have been reported to unmix hyperspectral SRS datasets mainly because the C–H stretching region is highly overlapping and many biomolecules have similar Raman spectra. Spectral unmixing using spectral phasor analysis, PCA, multivariate curve resolution, and least absolute shrinkage and selection operator (LASSO) are popular methods for unmixing hyperspectral SRS imaging datasets to enable the detection of discrete features within a sample.
Spectral phasor analysis uses the Fourier transform to project every pixel from a 3D hyperspectral SRS dataset onto the 2D phasor plane. Spectral phasor is a convenient method because it requires no prior knowledge of the sample, and clustering of the phasors based on spectral similarity can be used to identify regions in the sample which have similar, or the same, Raman spectral profile. The first example of spectral phasor analysis applied to hyperspectral SRS imaging data was reported in 2014 for label-free detection of cellular organelles,45 and since then it has been applied widely to study cellular and tissue composition,46–50 bioorthogonal Raman probes51,52 and protein aggregates.53
PCA has been applied to SRS imaging datasets for dimensionality reduction by creating lower-dimensional orthogonal projections that display maximum data variance.
K-Means cluster analysis (KMCA) is a method that groups spectra based on similarity. Initially, the operator selects the number of clusters into which the spectra will be assigned, whereupon each spectrum is assigned based on their spectral similarity to the seed locations. Each spectrum in the Raman or SRS hyperspectral stack is assigned to the cluster with the closest match to the mean spectrum of the cluster. KMCA is routinely used to identify cellular organelles using Raman spectroscopy54,55 and with SRS imaging for the localisation of cellular lipid droplets.27
Multivariate curve resolution is an alternative method which can decompose a measured spectral dataset into concentration maps and spectral profiles of the principal components. It is often coupled to alternative least squares (ALS) to unmix hyperspectral SRS imaging, including for cellular segmentation and the analysis of lipid-rich regions, see section 4.5 below which describes this approach in the model organism C. elegans.56
LASSO regression is a powerful technique in predictive analytics that introduces a penalisation factor to reduce overfitting by selectively shrinking some spectral coefficients to zero. In doing so, it can enhance the interpretation of large and complex hyperspectral datasets and has been successfully applied to studying cholesterol and unsaturated fatty acids in living cancer cells using hyperspectral SRS imaging (see section 5.2 below and57,58).
3. What does SRS/Raman tell us that other approaches do not?
As an optical technique, Raman microscopy is ideally suited for live-cell imaging. The use of near infrared laser sources can help to minimise sample damage and improve tissue penetration depth for imaging in complex sample types. A key advantage of Raman and SRS microscopy for cellular imaging applications is the fact that the detection of cellular biomolecules including proteins, lipids and DNA can be achieved based on the intrinsic Raman spectrum and without any additional labelling. As such, this is a major advantage to other optical imaging techniques based on fluorescence microscopy which require the use of reporters, stains or fluorescently labelled proteins to generate image contrast. Additionally, the linewidth of Raman peaks is much narrower than fluorescent counterparts (∼20 cm−1 vs. ∼1500 cm−1) which enables a much greater multiplexing capability for cellular imaging.59 Recently, using engineered polyynes as Raman reporters in the cell-silent region Fig. 1 of the Raman spectrum (1800–2800 cm−1), the detection of 26 discrete species was achieved in a super-multiplex imaging experiment using SRS microscopy.60 Given the broad and featureless nature of fluorescence emission, there exists a colour barrier beyond which the multiplexing of more than 5 spectral colours poses a significant challenge. A particularly attractive feature of Raman reporters is that they can be extremely small by design (down to a single chemical bond) which invokes a greatly reduced perturbation on the parent molecule. This has opened up opportunities for imaging drugs and small molecules using miniaturised Raman reporters based on alkynes and nitriles in a way that is not achievable using other optical imaging techniques.61 Furthermore, because the Raman stretching frequency is defined by the atoms which make up the molecular bond, the use of isotopic editing strategies for labelling and metabolic probing has been extremely fruitful. For example, the use of deuterium as a natural isotope of hydrogen results in a red shifting of the C–D stretching (2000–2300 cm−1) compared to C–H stretching (2800–3100 cm−1). As such, extremely minor modification of low-molecular weight metabolic precursors and drugs enables their visualisation in the cell-silent region of the Raman spectrum using deuterium labelling (e.g. see sections 4.2 and 4.4 below). Further expansion of the isotopic colour palette for Raman microscopy has demonstrated the impact of 13C labelling of alkynes and 15N probing of nitrile groups to increase the spectral colours available for imaging.62 When compared to SILAC labelling for Mass Spectrometry (MS) and proteomics experiments, Raman imaging offers the spatial resolution for probing metabolism at the subcellular scale in a way that is unachievable using these techniques. In that way, coupling Raman microscopy with metabolomics studies can offer a unique insight into probing cellular metabolism in a complementary fashion.
Historically, the detection of Raman images was too slow to be competitive with fluorescence imaging modalities, although advances in SRS microscopy have enabled rapid acquisition and high-content imaging for biomolecular characterisation which is beginning to have a translational impact in biological and medical research. With the advent of commercially available SRS imaging systems, the potential for further integration of coherent Raman imaging in biological and medical imaging is anticipated. Some new advances in methodology and analysis are outlined in section 5 below.
4. Raman-led insights into lipid and fatty acid metabolism
In this section, we present some studies of interest which reveal how Raman-based imaging can be employed to gain insight into aspects of fatty acid or lipid metabolism. We recognise this is neither an exhaustive list, nor is it entirely systematic. Our approach is to present a few very recent studies to reveal the kinds of questions that Raman can answer, and to illustrate these using different biological systems. More exhaustive reviews of both lipid metabolism,25,63 adipocyte biology10,11 and Raman64–67 can be identified.
4.1 Studying lipid biology in adipocytes using Raman imaging
Molecularly specific information on lipid metabolism can reveal novel cell phenotypes in health and disease. Lipid droplets are now recognised as highly dynamic organelles which play a major role in cellular and systemic homeostasis, and which contribute to disease aetiology, for example ectopic accumulation of lipid droplets in cells such as the liver or pancreatic beta cells are thought to be early drivers of insulin resistance.17,19,21 Lipid droplets are usually studied or identified using fluorescent lipid-soluble dyes which accumulate in droplets and can thus be quantified. While useful, these studies cannot offer insight into droplet chemical composition and thus potential heterogeneity and may provide a flawed index of droplet size because of the need for the fluorescent dye to partition unfirmly throughout the droplet. The former has been to some extent overcome using MS, but these undoubtedly powerful molecular approaches lack capacity for high throughput, cannot inform on spatial heterogeneity, are destructive and importantly are almost impossible to use for real-time dynamics (see ref. 2 for a recent review).
The application of Raman to droplet biology exemplifies the power of Raman-based imaging. The number and area of lipid droplets formed during differentiation in human adipocytes has been quantified and mapped using label-free SRS imaging.68 This is of interest because larger (hypertrophic) adipocytes are associated with metabolic disease risk69,70 and may even act as a reservoir for SARS-CoV2,71 perhaps explaining the strong link between disease outcome and obesity during the pandemic. Consistent with this notion, a recent study considered the structural and functional changes in adipose tissue in rodent models of obesity and type-1 diabetes.72 This study analysed Raman spectra using direct band analysis, ratiometric analysis and chemometric methods to identify significant spectral differences between adipocytes from control and Type-1 diabetes rodent models, and changes in unsaturation of lipid levels associated with obesity in different fat depots.72 These non-invasive methods provide a framework for future biological experiments to understand how changing lipid droplet biology relates to function and/or disease risk and begin to show how molecularly specific information can be gleaned from living cells.
This approach has been further advanced by Tratwal et al., who employed Raman micro-spectroscopy to examine the subtleties of bone marrow-derived adipocyte biology.73 Bone marrow adipocytes (BMAds) are classically observed to be comprised of two distinct subtypes (constitutive cBMAds and regulated rBMAds) which differ in the content of saturated fatty acids, the number and size of lipid droplets and biological function.74,75 These different populations are notoriously difficult to isolate and cell culture models hard to validate. Using lipid profiling at the cell population level and Raman micro-spectroscopy on single cells, Tratwal and co-workers validated the adipogenic potential of a bone marrow-derived stromal cell line, OP9 cells.73 Previous studies based on population analysis has suggested that the 16
:
1/16
:
0 fatty acid unsaturation ratio is a discriminating index for c- and rBMAds. Raman micro-spectroscopy revealed a predominance of unsaturated spectra after adipocyte induction; importantly, using the power of SRS they were able to analyse the heterogeneity of single lipid droplets within a single cell and show that the lipid droplets accumulated were not composed of uniformly saturated or unsaturated lipids, but rather were characterised by different mixtures.73 Using unguided hierarchical cluster analysis of the SRS signal from 100s of individual lipid within so-called induced OP9 cells (i.e. cells exposed to adipogenic induction media; iOP9) compared to spontaneously differentiating OP9 cells (sOP9), they found that iOP9 adipocytes not only contained larger lipid droplets but that these contained a greater frequency of unsaturated lipids than sOP9 adipocytes (Fig. 2). Based on previous studies this suggests that iOP9 adipocytes are similar in characteristics to rBMAds, and thus have opened the way to the analysis of the cell biology and role of these different populations.
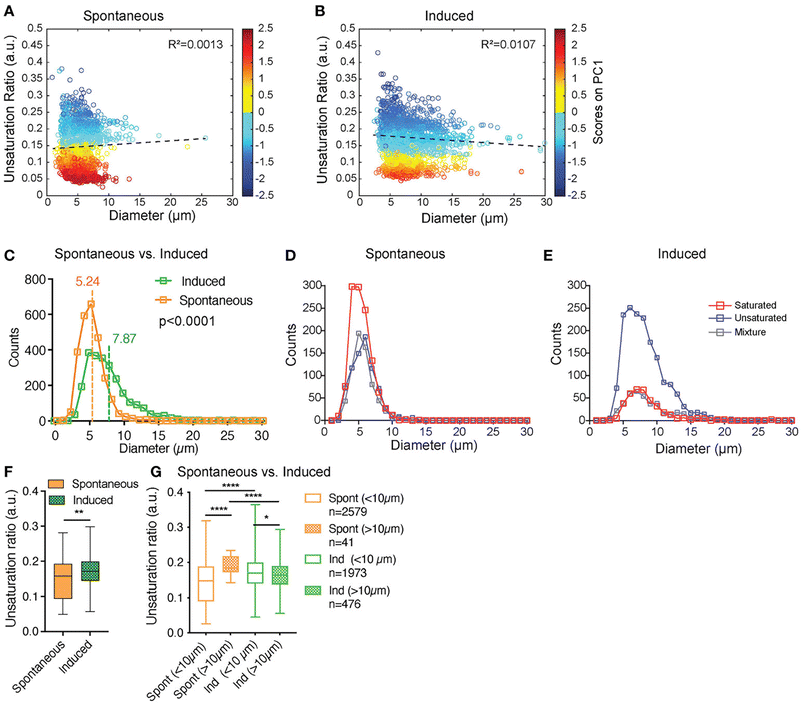 |
| Fig. 2 SRS provides insight into individual lipid droplet composition. Tratwal et al. examined the composition of individual lipid droplets in bone marrow adipocytes derived from OP9 bone marrow stromal cells, allowed to either differentiate spontaneously or in response to a defined induction cocktail (for details see ref. 73). (A and B) present the unsaturation ratio as a function of the diameter of the LDs for the two populations of cells. The circles in dark blue corresponds to LDs identified as unsaturated rich. The circles in dark red correspond to LDs identified as saturated-rich. The circles in yellow and light blue correspond to LDs identified as mixture. This Unsaturation Ratio was calculated from Raman micro-spectroscopy, defining the peak area assigned to unsaturated (C C bonds) divided by the area of saturated bonds (CH2), identified using unguided hierarchical cluster analysis. (C) separates LDs as a function of diameter showing that the LDs mean diameter of sOP9-adipocytes is significantly lower compared to iOP9-adipocytes. The power of this Raman based approach is revealed in (D and E) which shows that when separated according to LD type, a trend toward larger unsaturated lipid droplets in the induced condition is observed. (F) shows that the unsaturation ratios of sOP9 versus iOP9 at LD level. LDs from iOP9 adipocytes have a higher mean unsaturation ratio compared to LDs from sOP9 adipocytes. (G) illustrates the power of single droplet resolution: larger droplets (>10 μm) have a higher unsaturation ratio as compared to smaller lipid droplets, while smaller droplets (<10 μm) in induced OP9-adipocytes (n = 1973) have a higher unsaturation ratio than those of spontaneous OP9-adipocytes (n = 2579). Highest unsaturation ratio overall is seen in large droplets from spontaneous OP9-adipocytes (n = 41). Image from ref. 73 via https://creativecommons.org/licenses/by/4.0/. | |
These kinds of non-invasive approaches to study lipid or fatty acid metabolism are now gaining considerable traction in adipocyte biology as scientists appreciate the molecularly specific insight these approaches can deliver.26,68,72,73,76–78
4.2 Single cell SRS provides a new insight into lipid metabolism associated with phenotypic changes in a subset of tumour cells
It has long been appreciated that cancer cells exhibit distinct metabolic profiles to their non-transformed counterparts.79,80 Therapeutic resistance is a major challenge to oncology treatment. Studies have suggested that the transition from a drug-sensitive to a drug-resistant state is accompanied by changes in cellular metabolism, but this has proven difficult to study as typically, within a given population of cells, only a small fraction become drug-resistant.81,82 A good deal of recent work has focussed on understanding the change from glycolytic to fatty acid uptake and oxidation and how this relates to cancer cell function.79,83–85
A recent paper has adapted stimulated Raman scattering and spectral phasor analysis to provide single cell insight into metabolic reprogramming observed in cancer cells.7 In a striking example of the power of SRS, Tan et al. employed high throughput, large-area hyperspectral SRS imaging to resolve changes in the metabolism of single cells within a population.7 This approach involved capture of a stack of large-area hyperspectral images (200–500 cells), extraction of the SRS spectrum at each pixel and segmentation to generate maps of intracellular compartments. CellProfiler® was then used to generate maps of the cell boundaries, allowing the lipid map of individual cells to be identified. This in turn facilitated quantitation of the lipid metabolic signature on a per-cell basis. Married to the use of specific glucose or fatty acid non-invasive Raman probes, this approach allowed the authors to show that tumour cell drug-resistance (in this case to cisplatin) was accompanied by a molecular switch – a shift from glucose/glycolysis-dependent metabolism to fatty acid oxidation as a key indicator of a shift in drug-resistant cancer cells (Fig. 3).7 As well as providing new molecular understanding, this approach hints at a potential diagnostic strategy for identifying and treating cisplatin-resistant tumours. It also powerfully illustrates the insight that individual cell imaging within a population can provide and exemplifies the power of SRS since images from dozens of cells can be acquired quickly and are data rich. Notably, this group also used imaging approaches to quantify reactive oxidative species on a per cell basis.7 The potential to marry this with SRS analysis in the same experiment is worthy of further exploration. Such studies signpost the power of SRS to study specific metabolic changes in a non-invasive system, allowing the subsequent multiplexing of analysis with other signals, or indeed with other techniques such as single cell metabolomics,86 lipidomics87 or other MS-based methods.2
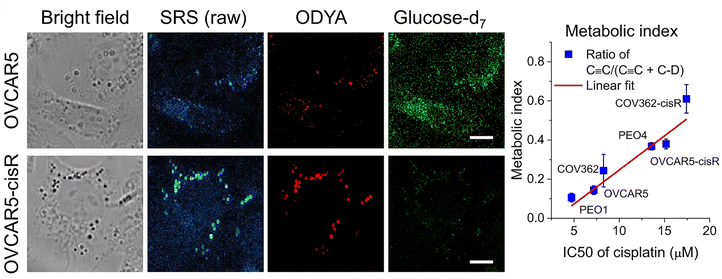 |
| Fig. 3 Metabolic phenotypic changes quantified by SRS. The ability to study distinct aspects of cellular metabolism in living cells distinguished by different Raman probes is exemplified from studies of the consequences of cisplatin resistance in cancer cells. Shown here are data from OVCAR5 cells, a model of ovarian cancer. These cells are used to model metabolic changes, such as those which accompany resistance to cisplatin therapies. Cells from control or cisplatin resistant (cisR in the figure) cells were incubated with glucose-d7 or 17-octadecynoic acid (ODYA), a fatty acid analogue with an endogenous alkyne allowing simultaneous analysis of glucose and fatty acid metabolism. Shown are representative bright field images, raw SRS images, and processed SRS images of ODYA and glucose-d7 in OVCAR5 and -cisR cells. This clearly reveals a shift from glycolytic to fatty acid metabolism in the cisplatin resistant cells. By quantifying the metabolic index of C C/(C C + C–D) to IC50s of cisplatin in various ovarian cancer cell line pairs (COV362, PEO1 and 4 and OVCAR5), the authors show a shift from glycolytic to fatty acid metabolism which is correlated with the IC50 of cisplatin in the cell type. Figure reproduced from ref. 7 with permission https://creativecommons.org/licenses/by/4.0/. | |
4.3 Spectral resolution of specific metabolites in lipid droplets in melanoma
Metastatic melanoma is a deadly form of skin cancer, many of which contain a mutation in BRAF kinase.88 A remarkable application of Raman imaging to understand cancer cell biology involved a series of melanoma cell lines, each encoding different BRAF mutations, corresponding to a different level of cancer cell differentiation and accompanied by differential susceptibility to inhibitors and immunotherapeutic interventions,6 each with a well annotated transcriptome.89 Based on spontaneous Raman spectra, it was found that the lipid/protein (CH2/CH3) ratio decreases with the progression of de-differentiation. Based on this observation, SRS imaging in real-time was employed to quantify single cells and thus capture cellular heterogeneity.6 Targeting the lipid peak (2845 cm−1, CH2 vibrations) and the protein peak (2940 cm−1, CH3 vibrations), the ratiometric images clearly showed a decrease in lipid signals from melanocytic to mesenchymal cells, indicating that the more differentiated cells are enriched in lipids. This observation strikingly correlated with transcriptomic data, and therefore gives confidence that single-cell Raman imaging correlates with transcriptional profiles. Using glucose-d7, the same group quantified de novo lipogenesis in the different cell lines and observed that de novo fatty acid synthesis is most active in differentiated cell lines; thus, single-cell Raman imaging has uncovered ‘phenotype-specific druggable susceptibilities in cancer cells’.6
Not content with this remarkable set of observations, Du et al. hypothesised that the maintenance of specific cancer cell characteristics could require specific examples of lipid biochemistry that could offer insight for new therapies. By way of a proof of concept, they showed that analysis of individual lipid droplets in one of the cell lines exhibiting a mesenchymal phenotype contained metabolic activities associated with lipid unsaturation (Fig. 4). They propose that lipid droplets in these cells act as a reservoir of unsaturated fatty acids; depletion of this pool eventually drives apoptosis.6 The data itself is exciting and provocative, but arguably just as important is their observation that ‘the mechanism and applicability underlying reported susceptibilities in our work are distinctly different from previous reports that relied on bulk analysis. This demonstration thus emphasises the unique value of subcellular pharmaco-metabolomics as a revelatory tool for uncovering new cell biology’.6 We believe that Raman-based metabolomics is set to revolutionise biology as new tools and image processing algorithms are developed.
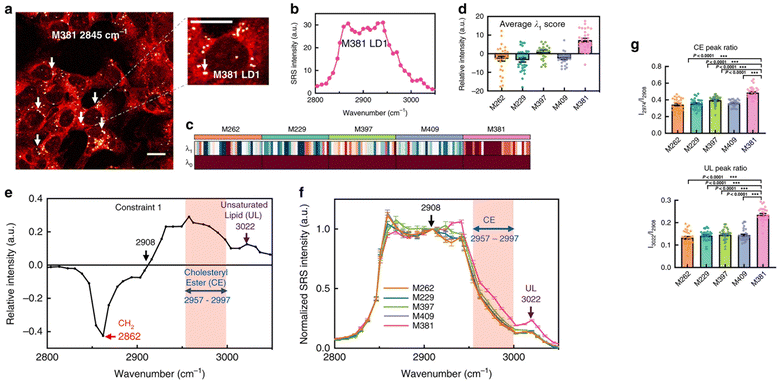 |
| Fig. 4 Changes in the biochemistry in individual lipid droplets is associated with disease. Du et al. hypothesised that the maintenance of specific cancer cell characteristics would likely be underpinned by changes in lipid biochemistry, and that hSRS could test this.6 By way of a proof of concept, they showed that analysis of individual lipid droplets in a melanoma cell line which exhibits a pronounced mesenchymal phenotype contained metabolic activities associated with lipid unsaturation, and some of their data is reproduced here. (a) shows a representative SRS image of M381 cells imaged in the CH2 (2845 cm−1) channel, the LD signal can thus be clearly identified (arrows). A zoomed-in image highlights a single LD. The authors acquired Raman spectra on LDs from a range of melanoma cells with high spectral resolution (b) and extracted key data from these spectra using surprisal analysis. Panel B shows the hSRS spectrum of the zoomed-in LD indicated in panel A at the C–H stretch region (2800–3050 cm−1). (c) shows a heatmap for scores of the top two constraints (λ0 − λ1) by surprisal analysis of hSRS spectra on LDs across five cell lines (M numbers refer to different melanoma lines – see ref. 6) Each column represents an individual LD and each row represents the constraint scores, with the average score of λ1 across five cell lines shown in (d). Molecularly specific information can be obtained from the Raman peak assignments for constraint 1 (λ1) (e). The pink shadowed range from 2957 to 2997 cm−1 is assigned to cholesteryl esters (CE), and the 3022 cm−1 peak (violet arrow) is assigned to unsaturated lipids ( C–H, UL). hSRS spectra (normalized at 2908 cm−1, the zero point revealed in e) of LDs across each cell line is shown in (f), and then used to compare across multiple cell lines (g), which shows quantification of relative CE (2974 cm−1/2908 cm−1, top panel) and UL (3022 cm−1/2908 cm−1, bottom panel) enrichment in LDs across different melanoma cell lines. Marrying this insight with transcriptomics data provides powerful new insight. Figure reproduced from ref. 6 with permission https://creativecommons.org/licenses/by/4.0/. | |
4.4 New insight into metabolic ageing in Drosophila
Drosophila are a favoured model to study the biology of ageing due to their conserved signalling pathways and short life span coupled to excellent genetic tools, high throughput and low cost90–92 thereby providing insight into how lipid metabolism directly impacts the ageing process.93,94 Metabolism in ageing fruit flies has been a point of interest due to the metabolic differences reported in ageing humans and how differences in diet impact progression in ageing. Recent studies using Raman have added credence to changes in lipid metabolism underscoring effects of ageing.
Comparison of the SRS signals of fat bodies from 7 and 35 day old flies revealed a significant increase in retinoid presence (similar lipid level increases have also been observed in C. albicans95) and a decrease in unsaturated fatty acid levels in 35 day old flies compared to their younger counterparts.96 While important, these observations are limited to a snapshot of levels at a given time. Shi and colleagues therefore developed an approach to quantify changes in lipid turnover by incorporating heavy water (D2O) into the diet, allowing de novo lipogenesis to be measured (a technique they christened DO-SRS).96,97 As deuterium (D) incorporates into the newly synthesized lipids, the SRS silent region can be utilized to quantify C–D bond formation. Alongside reduced lipid droplet size, older (day 35) flies showed reduced lipogenesis across all diets when subjected to ratiometric imaging of existing lipids (2850 cm−1) and deuterated lipids (2140 cm−1) in comparison to younger flies (Fig. 5). These data suggest that larger lipid droplets are more active and may indicate that large and small lipid droplets play different roles in metabolism. This has provided further impetus to the drive to study metabolic changes on single cells, rather than populations, and clearly illustrates that all lipid droplets are not the same.96,98
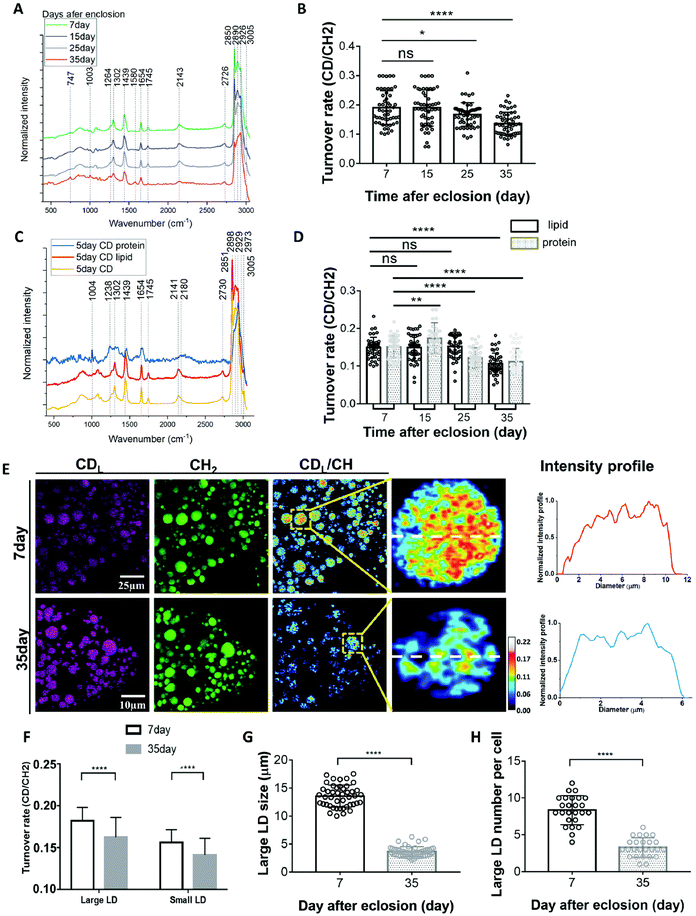 |
| Fig. 5 D2O-probed SRS to study metabolic adaption in organs from model organisms. Understanding how metabolism changes with age has far-reaching implications for human health. Marrying SRS with the power of model organisms led to the development of new approaches to define metabolic changes by incorporating heavy water (D2O) into the diet.97,98 Shown here an example of the kind of insight this approach can provide. (A), averaged Raman spectra were collected from flies of different ages labelled by 20% D2O and then imaged. Quantification of the C–D turnover by dividing the intensity of 2143 cm−1 (CD signal) to the peak at 2850 cm−1 (lipid stretch) is shown in (B). Unmixing of the C–D spectra into protein and lipid spectra is shown in (C). The peak at 2141 cm−1 corresponds to the newly synthesized D-labelled lipids, and the peak at 2180 cm−1 is newly synthesized D-labelled protein. This allows quantification of lipids and protein turnover rates by comparing 2141 cm−1 to 2850 cm−1 and 2180 cm−1 to 2929 cm−1, respectively (D).98 This methodology can then facilitate a direct comparison of metabolic activities in 7-day (young) and 35-day-old flies (representative SRS are shown in (E). Old (35-day) flies showed reduced lipid metabolism compared with young flies. SRS imaging could visualize the lipids turnover inside single LDs at the subcellular scale and subdivide this insight into small and large LDs (F–H). For further experimental details, see ref. 98. Figure reproduced from ref. 98 with permission https://creativecommons.org/licenses/by/4.0/. | |
The ability to ‘track’ deuterated molecules is a highly favourable aspect of SRS by allowing visualisation of individual lipid droplet metabolic activity and appreciation of the heterogeneity of fat depots.96,97 This has been strikingly applied to studies of how diet composition, caloric restriction, and metabolic activity impact aging96,99 where a combination of Raman and SRS have provided a wealth of insight allowing quantification of lipid droplet size and distribution. The new studies alluded to above now extended this toolkit to allow quantification of metabolic activity at the level of single cells and even single lipid droplets. These advances will likely open-up further insights when applied across other metabolic and compartmentalised processes.
Two further studies along similar lines are worthy of highlight. In the first, harnessing the power of Drosophila genetics and DO-SRS with deuterated probe molecules, in this case deuterated glucose and deuterated acetate, Li et al. observed that caloric restriction and down-regulation of the insulin signalling pathway was accompanied by a shift in brain metabolism to use acetate as a major carbon source for lipid synthesis, and provided an elegant analysis of lipid turnover at the level of single lipid droplets.100 These studies will no doubt pave the way for more detailed metabolic studies across a range of tissues and organisms by exemplifying the novelty and detail that Raman can provide. In a second study, also in flies, Spratt and colleagues used deuterated methionine to study regional and cellular specific uptake and incorporation of a specific amino acid during fly development.101 This study is worthy of comment for two reasons. First, the weaker vibrational resonance of D2O can limit the potential signal, meaning that for studies of less abundant species, it may not be an ideal choice as levels of incorporation may be too low for reliable quantification (this is often not the case for lipids, as they accumulate in lipid droplets, concentrating the lipids into a defined location allowing ready identification). Secondly, this group married SRS and fluorescence in an integrated microscope, allowing the investigation of specific cells within a complex mixture that could be identified using other labels.101 This highlights a further potential advance in this rapidly moving field.
4.5 Understanding drug resistance in Candida albicans
Antifungal resistance is a significant threat; fungal infections cause major diseases such as cryptococcosis and aspergillosis, chronic conditions such as allergic bronchopulmonary aspergillosis and a range of less-threatening conditions such as vaginitis or candidiasis.102,103 Candida albicans – a fungal pathogen – is a contributing factor in hospital acquired infections and continued use of a first-line therapy Fluconazole (the prototypical azole drug) has resulted in antifungal resistance within this strain.102 Fluconazole disrupts the ergosterol biosynthesis pathway and results in accumulation of sterol 14,24-dimethylcholesta-8,24(28)-dien-3β,6α-diol which is toxic to the fungus.104 C. albicans can form biofilms, a surface associated fungal community, which presents significant clinical issues in hospitals, e.g. in catheters etc.105
SRS has provided insights into this antifungal resistance by identifying that lipid droplets are formed via de novo lipogenesis within these biofilms during the stationary phase.104 Zhang et al. describe a novel approach to understanding the composition of these lipid droplets using pixel-wise least absolute shrinkage and selection operator (LASSO; see section 2.2) regression of the hyperspectral SRS image stack to ‘decompose’ this into specific chemical ‘maps’.95 Comparison of these chemical maps revealed differences between azole-sensitive and resistant strains of C. albicans, specifically upregulated levels of ergosteryl ester was detected in fluconazole resistant C. albicans strains. This was reflected in the sterol C
C peak (1603 cm−1) and acyl C
C peaks (1655 cm−1), absent in wild type strains. These were confirmed by referencing the spectra from pure ergosterol and glycerol trioleate and studying these chemical maps under conditions such as disruption of glycolysis. These data indicate that the accumulation of esterified ergosterol was a consequence of de novo lipogeneis,95 and suggests the hypothesis that inhibition of ergosterol esterification could be a viable therapeutic option. In an elegant set of experiments, Zhang et al. showed that treatment with oleic acid reduced the accumulation of ergosteryl ester in resistant Candida strains which in turn re-sensitised Candida to Fluconazole identifying a novel therapeutic strategy to deal with resistance.95 The molecularly specific insight that Raman can provide underscores these exciting developments.
4.6 Fatty acid production in biotechnology
While many of us are familiar with the concept of using bacteria as a source of chemicals and drugs in biotech, what is seldom well-appreciated by those not directly in the field is the difficulty faced by companies because of both cell-to-cell and genetic variation that can occur during the fermentation processes that underpin these methods. Hence, a crucial requirement for the biotech field is an ability to quantify this variability, ideally in real-time. A recent study has shown that hyperspectral SRS offers a powerful technique for monitoring the fermentation process.106 This is challenging in bacteria due to their small size which this shortens the axial signal integration length and so results in weaker SRS signals than are typically observed for larger cells. At the heart of the method developed by Tague et al., hyperspectral SRS (section 2.2) was coupled to a novel computational analysis technique that can generate predictions of chain length and saturation. After validating this using MS, they were able to optimise imaging to capture information from living cells, and by marrying SRS and time-lapse phase contrast imaging with automated image segmentation approaches, demonstrated a correlation between fatty acid production and growth, revealing metabolic and temporal heterogeneity in actively growing cultures.106 Importantly this was achieved by recoding from the same cell over a period of many hours. This work is sure to gain attention across fermentation biology-driven systems.
5. Examples of new Raman method developments in the metabolic toolkit
The approaches outlined above are characterised by the application of increasingly sophisticated Raman-based approaches in different biological systems, chosen here to illustrate how these kinds of approaches can be leveraged to gain new biological insight. Now, we turn our attention to one or two novel examples of Raman technology development which we believe new advances offer the scope for a paradigm shift, either in imaging, the biology it illuminates, or both.
5.1 Identification of specific lipid species in the absence of chemical labelling by pushing the spectral resolution limit
Hyperspectral stimulated Raman scattering (SRS) microscopy has the potential to provide comprehensive lipid characterization, including carbon chain length quantification. However, the current spectral resolution of SRS microscopy is limited to about 10 cm−1, which is not sufficient to resolve the subtle Raman shifts corresponding to chain lengths, e.g. between specific fatty acid species. This is important because population-level studies suggest that the degree of unsaturation and chain length differ in cancer cells, but how these relate to specific subsets of cells is unclear, largely because of an inability to identify chain lengths on a microscopic scale. Huang et al. have pushed the spectral resolution limit of hyperspectral SRS microscopy to 5.4 cm−1 by employing a highly efficient spectral compressor, which enabled them to differentiate eight types of saturated lipids with carbon chain lengths from C8:0 to C22:0.107 Future applications of this approach would involve application to living cells where it has the potential to reveal the role of specific lipid/fatty acid molecules across a range of experimental systems.
5.2 Single cell metabolism in cancer cells by high-content hyperspectral SRS-imaging
As noted above and elsewhere, hyperspectral SRS (hSRS) can resolve multiple spectral metabolites. This has been reported at sub-second imaging speeds and with spectral coverage of >200 cm−1 and a spectral resolution of less than 10 cm−1 (see section 5.1). Together with downstream spectral analysis methods, such as least-squares fitting and phasor segmentation, hSRS has revealed many new facets of biology, some of which were discussed above. However, many metabolites remain challenging to study, either because their signals are too weak or because their characteristic signals are either difficult to resolve or dominated by other biomolecules with strong Raman signatures. Recognising this limitation, a new approach has been developed and applied to cancer cells – high content, hyperspectral SRS (h2SRS).57
Low sensitivity issues can be overcome in SRS approaches by focussing on the C–H high wave number bands. However, this presents a problem, as all major metabolic species (proteins, lipids, nucleic acids, glucose etc.) exhibit substantially overlapping peaks within this region which cannot be resolved using existing hyperspectral data analytics approaches. This arises because of the crosstalk between the C–H vibrations in the chemical maps. Tan et al.57 reasoned that only a few metabolic species make dominant contributions at any given point, a condition that can be incorporated into the analysis of these signals as a sparsity constraint, a mathematical device in which a model is deigned to use only a small finite number of significant features at a time, disregarding less relevant ones (this approach is gaining traction across many facets of biological imaging108–110).
Using h2SRS, Tan et al. were able to identify metabolic alterations in cancer cells in response to chemotherapy in single living cells. Specifically, increased intracellular fatty acid and carbohydrate levels in brain cancer cells after cisplatin treatment and in the pancreatic cell model MIA PaCa-2 after gemcitabine treatment. This extraordinary study offers the potential to unravel many novel facets of cellular metabolism on a single cell scale using non-destructive approaches. As they note, because ‘the unmixing algorithm is a supervised approach that requires prior knowledge of the chemical composition, more detailed prior information (metabolite composition and abundance) obtained through MS can effectively guide the selection and scaling of input references beyond the current [five] components’. This powerful marriage of MS with live cell imaging using chemical probes, coupled to high-throughput and single cell resolution, hints at a future in which multiple metabolites can be studied non-invasively and with increased specificity in living cells, providing a step-forward in our understanding of single-cell metabolism.
5.3 Super-resolution meets SRS?
A further example of the power of SRS is provided by the development of a novel deconvolution algorithm: adaptive moment estimation (Adam) optimization-based pointillism deconvolution (A-PoD) which is illustrated conceptually in Fig. 6.111 Deconvolution is a computational approach, widely used in light microscopy, that can improve the contrast and resolution of digital images by removing or minimising distortion present within the images. Several deconvolution approaches are widely applied within fluorescence imaging, but these approaches cannot be applied to single-frame images in low-sensitivity detectors, such as is the case for SRS microscopy images. Building on previous attempts to overcome this issue for SRS,112 Jang et al. developed a mathematical model to allow deconvolution and applied this to mammalian cells in culture and to Drosophila brain. This approach provided molecularly specific information at a spatial resolution of less than 59 nm on a single lipid droplet, allowing comparison of nanoscopic distribution of lipids and proteins in cells and subcellular organelles which can be applied to in situ samples; this approach also represents a step-change in processing time.111 The potential application of this approach to wider aspects of biology offers a further driver for new insight.
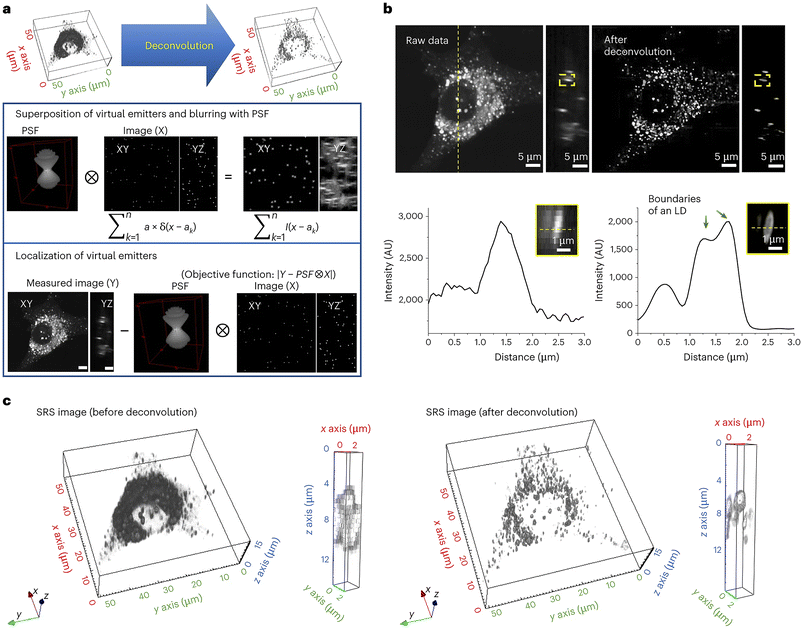 |
| Fig. 6 Deconvolving SRS images using A-POD. Deconvolution has played a key role in resolving light microscopy images. Jang et al. developed a mathematical model, A-POD (see section 5.3), to allow deconvolution and applied this to mammalian cells in culture and to Drosophila brain. This approach provided molecularly specific information at a spatial resolution of less than 59 nm on a single lipid droplet, allowing comparison of nanoscopic distribution of lipids and proteins in cells and subcellular organelles which can be applied to in situ samples; this approach also represents a step-change in processing time.111 The process is illustrated schematically, taken from Jang et al.111 which shows the conversion of SRS images into super-resolution images (a). First, a specific number of virtual emitters proportional to the overall brightness of the image are placed on an image (X), and a blurred image (S) is created through convolution of X and the PSF. When the position of each virtual emitter is adjusted such that the difference between the blurred image S and the measured image (Y) is minimized, X becomes the image with the most optimal distribution of virtual emitters. They used a modified Adam solver for the optimization in A-PoD. In (b), a three-dimensional deconvolution result of LDs (2850 cm−1) in a live cell is shown. Following deconvolution, the membrane of an individual LD was clearly visualized in the intensity profile in the lower panel. AU, arbitrary units. Three-dimensional-rendering results of the SRS image before (left) and after (right) deconvolution are shown in (c). After deconvolution, the shapes of ∼1 μm-sized LDs were clearly visible. Data reproduced from111 with permission https://creativecommons.org/licenses/by/4.0/. | |
5.4 New methods for in situ analysis of fatty acids
Using deuterium isotopes in metabolic studies has offered substantial insight through the history of metabolic studies because the deuterium is eliminated slower than hydrogen: the kinetic isotope effect.113 This has been elegantly exploited to reveal a tumour-selective cytotoxicity of γ-linoleic acid in VA-13 tumour cells;114 coupled to the use of an alkyne reporter this offers the potential for distinguishing between lipids with very similar structures (see ref. 65 for a recent review).
A further study coupled Raman microscopy with a novel spectral unmixing software (ImageCUBE) capable of separating overlapping spectra from the mixed measured spectrum within individual pixels.115 Applied initially to an analysis of five labelled fatty acids (palmitic acid-Br, oleic acid-alkyne, α-linolenic acid-d14, arachidonic acid-d8, and eicosapentaenoic acid-d5), these authors were able to show that fatty acids with higher degrees of unsaturation behaved differently and were concentrated in lipid droplets. While the biological mechanism underpinning this behaviour presently is not defined, the identification of this phenomenon suggests that further work on lipid droplet biology should focus on the substrate preferences of the enzymes involved in their biogenesis and hydrolysis. Uematsu et al. raise the interesting speculation that because polyunsaturated fatty acids are readily oxidized, their presence within lipid droplets may protect from this.115 Given the importance of fatty acid metabolism in tumour cell biology (see sections 4.2 and 5.2 above for illustrative examples), this could yet turn out to have far-reaching consequences.
5.5 New tags for multiplex imaging
The challenge facing those wishing to study complex metabolic events, such as those underpinning lipogenesis/fatty acid metabolism, involves unpicking the overlapping spectra and poor signal-to-noise ratio of some of the commonly used chemical reporters. One approach is to use bioorthogonal Raman groups, chemically functionalised to exhibit discrete functional vibration. Presently, these probes lie within the 2000–2300 cm−1 range of the Raman spectrum. A notable recent advance has come from work which uses the B–H stretch of metallacarboranes, detected at 2570 cm−1. The potential to marry these novel probes was evaluated using a metallacarborane B–H stretch in a triplex detection of a bis-alkyne and a deuterated fatty acid, all of which lie within the cell-silent region of the Raman spectrum.116 Murphy and colleagues used this approach coupled to imaging in the high wavenumber region of the cellular Raman spectrum and spectral unmixing of the hSRS dataset to enable 9-colour detection. This offers a remarkable step forward in live cellular imaging studies.
6. Conclusions and outlook
It must be emphasised that many of the advances outlined here are relevant across many fields of biology. Molecularly specific insight obtained from living cells, organoids and intact tissues offers the potential for unrivalled insight into basic biological control mechanisms. The ability to use the approaches outlined here in model organisms offers huge potential for developmental biology to be coupled firmly to metabolic changes in key defined cells, even within populations. This can offer new understanding of the triggers for developmental events such as cellularisation in flies,117 or be coupled to optogenetic controls in the study of embryogenesis.118 The marriage of powerful molecular tools to these real-time non-invasive techniques could drive considerable new insight.
The capacity to understand how metabolism is sub-compartmentalised within cells,119,120 and how this changes in diseases such as obesity, diabetes and cancer, could offer new therapeutic horizons. Driving the limit of SRS to even smaller scales is beginning to offer this kind of advance.
Using real-time read-outs, the impact of (for example) environmental factors or toxins on metabolic events can reveal new understanding of cellular behaviour, particularly if married to high specificity moleculomic analysis. The US National Research Council is driving toxicology screening away from animal studies into cell systems, using metabolomics as a key platform. The potential to couple such work to specific changes in key molecular signatures in real time in living cells offers one exciting way in which SRS-metabolic studies could reveal new understanding.
Advances in hyperspectral SRS imaging have also enabled a detailed molecular characterisation of lipid species based on the Raman spectral signature, and when coupled to chemometric analysis techniques, the potential to identify drug-induced effects and molecular features of disease has been realised. Future development of the technique particularly in improving the spectral resolution (cm−1 range) will help to advance studies in this direction.
In the context of cellular imaging, SRS has the advantage over conventional Raman imaging speed which is comparable to fluorescence imaging modalities. This feature has greatly facilitated SRS as a modality for biological microscopy. However, Raman scattering has the clear advantage of collecting a partial or full Raman spectrum at each pixel within an image. Given the favourable spectral resolution associated with Raman spectroscopy, this feat enables the detection of subtle changes that can be used to report on cellular metabolism or subtle biochemical changes that occur within a cellular population. SRS imaging has yet to reach the spectral resolution of conventional Raman microscopy, and this is anticipated to be an area under active investigation. Some early progress towards this aim has been realised through the development of broadband SRS imaging systems employing fast tuneable fibre lasers to enable rapid hyperspectral imaging.
Many studies have demonstrated the live-cell imaging capability of SRS microscopy, however, very few studies have demonstrated the long-term analysis of cellular metabolism during longitudinal live cell imaging. The development of CARS and SRS imaging towards this aim would represent a significant breakthrough for temporal monitoring of drug-cell interactions and cellular metabolism during disease onset and progression. We recently showed it was possible to visualise drug uptake over several hours in the same cellular population using bespoke perfusion chambers to do so.121 As most SRS systems are inverted microscope set ups relying on the detection of transmitted light, the design of robust chambers that can enable favourable culture conditions of temperature, pH and media exchange over hours-days would be a significant advantage.
Several research groups are beginning to demonstrate the potential of super-resolution SRS microscopy for live cell, label-free imaging at the sub-100 nm scale. Current approaches based on expansion microscopy, deconvolution image analysis, photo-switchable Raman reporters and optical engineering strategies have been developed over the course of the past 5 years. The implementation of artificial intelligence and machine learning methodology to improve the spatial resolution of SRS imaging will likely open new opportunities for studying intracellular lipid biology. Finally, the integration of SRS microscopy with other analytical techniques including photothermal imaging52 and mass spectrometry122 offer the potential for studying biological samples with unprecedented detail and with a complimentary read out.
In sum, we believe that Raman spectroscopy offers an increasingly powerful toolkit for biologists, providing molecularly specific insight at a single cell, and increasingly, sub-cellular level. Illustrated here by considering aspects of fatty acid metabolism, we contend that these approaches are beginning to rival the advances made by fluorescence microscopy and super-resolution imaging. As increasingly novel probes are developed, together with data processing algorithms, we expect the next decade to provide major advances in single cell metabolic analysis with Raman in the vanguard.‡
Abbreviations
ALS | Alternative least squares |
A-PoD | Adaptive moment estimation optimization-based pointillism deconvolution |
BMAds | Bone marrow-derived adipocytes |
CARS | Coherent anti-Stokes Raman scattering |
cisR | Cisplatin resistant (cells) |
CRS | Coherent Raman scattering |
hSRS | Hyperspectral-SRS |
h2SRS | High content, hyperspectral SRS |
KCMA | K-means cluster analysis |
LASSO | Least absolute shrinkage and selection operator |
LD | Lipid droplet |
MS | Mass spectrometry |
ODYA | 17-octadecynoic acid |
PCA | Principal component analysis |
SILAC | Stable isotope labelling by amino acids in cell culture |
SRG | Stimulated Raman gain |
SRL | Stimulated Raman loss |
SRS | Stimulated Raman scattering |
Author contributions
JCG: conceptualisation; writing – original draft; writing – review and editing; visualization. WJT: conceptualisation; writing – original draft; writing – review and editing; visualization. DG: conceptualisation; project administration; writing – review and editing. KF: conceptualisation; project administration; writing – review and editing. GWG: conceptualisation; project administration; writing – original draft. Visualization; funding acquisition. supervision.
Data availability
This is a review article and does not contain any new primary data, hence there is no relevant availability statement required.
Conflicts of interest
The authors declare no conflicts of interest.
Acknowledgements
We thank Medical Research Scotland for financial support (studentship to JCG).
References
- J. Liao, X. Y. Lu, X. Shao, L. Zhu and X. H. Fan, Uncovering an organ's molecular architecture at single-cell resolution by spatially resolved transcriptomics, Trends Biotechnol., 2021, 39, 43–58, DOI:10.1016/j.tibtech.2020.05.006.
- T. M. J. Evers, M. Hochane, S. J. Tans, R. M. A. Heeren, S. Semrau, P. Nemes and A. Mashaghi, Deciphering metabolic heterogeneity by single-cell analysis, Anal. Chem., 2019, 91, 13314–13323, DOI:10.1021/acs.analchem.9b02410.
- I. Ruz-Maldonado, J. T. Gonzalez, H. Zhang, J. Sun, A. Bort, I. Kabir, R. G. Kibbey, Y. Suarez, D. M. Greif and C. Fernandez-Hernando, Heterogeneity of hepatocyte dynamics restores liver architecture after chemical, physical or viral damage, Nat. Commun., 2024, 15, 1247, DOI:10.1038/s41467-024-45439-0.
- B. Budnik, E. Levy, G. Harmange and N. Slavov, SCoPE-MS:mass spectrometry of single mammalian cells quantifies proteome heterogeneity during cell differentiation, Genome Biol., 2018, 19, 161, DOI:10.1186/s13059-018-1547-5.
- S. J. Altschuler and L. F. Wu, Cellular heterogeneity:Do differences make a difference?, Cell, 2010, 141, 559–563, DOI:10.1016/j.cell.2010.04.033.
- J. J. Du, Y. P. Su, C. X. Qian, D. Yuan, K. Miao, D. Lee, A. H. C. Ng, R. S. Wijker, A. Ribas, R. D. Levine, J. R. Heath and L. Wei, Raman-guided subcellular pharmaco-metabolomics for metastatic melanoma cells, Nat. Commun., 2020, 11, 4830, DOI:10.1038/s41467-020-18376-x.
- Y. Y. Tan, J. J. Li, G. Y. Zhao, K. C. Huang, H. Cardenas, Y. N. Wang, D. Matei and J. X. Cheng, Metabolic reprogramming from glycolysis to fatty acid uptake and beta-oxidation in platinum-resistant cancer cells, Nat. Commun., 2022, 13, 4554, DOI:10.1038/s41467-022-32101-w.
- S. Ley-Ngardigal and G. Bertolin, Approaches to monitor ATP levels in living cells:where do we stand?, FEBS J., 2022, 289, 7940–7969, DOI:10.1111/febs.16169.
- Z. Zhang, A. Milias-Argeitis and M. Heinemann, Dynamic single-cell NAD(P)H measurement reveals oscillatory metabolism throughout the cell division cycle, Sci. Rep., 2018, 8, 2162, DOI:10.1038/s41598-018-20550-7.
- A. Santoro, T. E. McGraw and B. B. Kahn, Insulin action in adipocytes, adipose remodeling, and systemic effects, Cell Metab., 2021, 33, 748–757, DOI:10.1016/j.cmet.2021.03.019.
- B. B. Kahn, Adipose Tissue, Inter-Organ Communication, and the Path to Type 2 Diabetes:The 2016 Banting Medal for Scientific Achievement Lecture, Diabetes, 2019, 68, 3–14, DOI:10.2337/dbi18-0035.
- A. T. N. Nair, A. Wesolowska-Andersen, C. Brorsson, A. L. Rajendrakumar, S. Hapca, S. Gan, A. Y. Dawed, L. A. Donnelly, R. McCrimmon, A. S. F. Doney, C. N. A. Palmer, V. Mohan, R. M. Anjana, A. T. Hattersley, J. M. Dennis and E. R. Pearson, Heterogeneity in phenotype, disease progression and drug response in type 2 diabetes, Nat. Med., 2022, 28, 982–988, DOI:10.1038/s41591-022-01790-7.
- D. Langin, Adipocyte heterogeneity revealed by spatial transcriptomics of human adipose tissue:Painting and more, Cell Metab., 2021, 33, 1721–1722, DOI:10.1016/j.cmet.2021.08.011.
- Q. Luong, J. Huang and K. Y. Lee, Deciphering white adipose tissue heterogeneity, Biology, 2019, 8, 23, DOI:10.3390/biology8020023.
- D. Norris, P. Yang, S. Y. Shin, A. L. Kearney, H. J. Kim, T. Geddes, A. M. Senior, D. J. Fazakerley, L. K. Nguyen, D. E. James and J. G. Burchfield, Signaling heterogeneity is defined by pathway architecture and intercellular variability in protein expression, iScience, 2021, 24, 102118, DOI:10.1016/j.isci.2021.102118.
- C. R. Kahn, G. Wang and K. Y. Lee, Altered adipose tissue and adipocyte function in the pathogenesis of metabolic syndrome, J. Clin. Invest., 2019, 129, 3990–4000, DOI:10.1172/JCI129187.
- T. Horii, J. Kozawa, Y. Fujita, S. Kawata, H. Ozawa, C. Ishibashi, S. Yoneda, T. Nammo, J. I. Miyagawa, H. Eguchi and I. Shimomura, Lipid droplet accumulation in beta cells in patients with type 2 diabetes is associated with insulin resistance, hyperglycemia and beta cell dysfunction involving decreased insulin granules, Front. Endocrinol., 2022, 13, 996716, DOI:10.3389/fendo.2022.996716.
- M. Veliova, A. Petcherski, M. Liesa and O. S. Shirihai, The biology of lipid droplet-bound mitochondria, Semin. Cell Dev. Biol., 2020, 108, 55–64, DOI:10.1016/j.semcdb.2020.04.013.
- J. L. Dempsey, G. N. Ioannou and R. M. Carr, Mechanisms of lipid droplet accumulation in steatotic liver diseases, Semin. Liver Dis., 2023, 43, 367–382, DOI:10.1055/a-2186-3557.
- K. K. Bence and M. J. Birnbaum, Metabolic drivers of non-alcoholic fatty liver disease, Mol. Metab., 2021, 50, 101143, DOI:10.1016/j.molmet.2020.101143.
- D. G. Mashek, Hepatic lipid droplets: A balancing act between energy storage and metabolic dysfunction in NAFLD, Mol. Metab., 2021, 50, 101115, DOI:10.1016/j.molmet.2020.101115.
- R. Veluthakal, D. Esparza, J. M. Hoolachan, R. Balakrishnan, M. Ahn, E. Oh, C. S. Jayasena and D. C. Thurmond, Mitochondrial dysfunction, oxidative stress, and inter-organ miscommunications in T2D progression, Int. J. Mol. Biol., 2024, 25, 1504, DOI:10.3390/ijms25031504.
- D. E. James, J. Stockli and M. J. Birnbaum, The aetiology and molecular landscape of insulin resistance, Nat. Rev. Mol. Cell Biol., 2021, 22, 751–771, DOI:10.1038/s41580-021-00390-6.
- D. J. Fazakerley, J. R. Krycer, A. L. Kearney, S. L. Hocking and D. E. James, Muscle and adipose tissue insulin resistance: malady without mechanism?, J. Lipid Res., 2019, 60, 1720–1732, DOI:10.1194/jlr.R087510.
- P. E. Morales, J. L. Bucarey and A. Espinosa, Muscle lipid metabolism: Role of lipid droplets and perilipins, J. Diabetes Res., 2017, 2017, 1789395, DOI:10.1155/2017/1789395.
- S. O. Shuster, M. J. Burke and C. M. Davis, Spatiotemporal heterogeneity of de novo lipogenesis in fixed and living single cells, J. Phys. Chem. B, 2023, 127, 2918–2926, DOI:10.1021/acs.jpcb.2c08812.
- E. W. Hislop, W. J. Tipping, K. Faulds and D. Graham, Label-free imaging of lipid droplets in prostate cells using stimulated Raman scattering microscopy and multivariate analysis, Anal. Chem., 2022, 94, 8899–8908, DOI:10.1021/acs.analchem.2c002368899.
- K. Czamara, Z. Majka, E. Stanek, N. Hachlica and A. Kaczor, Raman studies of the adipose tissue:Current state-of-art and future perspectives in diagnostics, Prog. Lipid Res., 2022, 87, 101183, DOI:10.1016/j.plipres.2022.101183.
- G. Donjuán-Loredo, R. Espinosa-Tanguma and M. G. Ramírez-Elías, Raman spectroscopy in the diagnosis of metabolic syndrome, Appl. Spectrosc. Rev., 2023, 58, 159–179, DOI:10.1080/05704928.2021.1944175.
- K. Czamara, K. Majzner, M. Z. Pacia, K. Kochan, A. Kaczor and M. Baranska, Raman spectroscopy of lipids: a review, J. Raman Spectrosc., 2015, 46, 4–20, DOI:10.1002/jrs.4607.
- J. R. Beattie, S. E. J. Bell and B. W. Moss, A critical evaluation of Raman spectroscopy for the analysis of lipids:Fatty acid methyl esters, Lipids, 2004, 39, 407–419, DOI:10.1007/s11745-004-1245-z.
- L. E. Jamieson, C. Wetherill, K. Faulds and D. Graham, Ratiometric Raman imaging reveals the new anti-cancer potential of lipid targeting drugs, Chem. Sci., 2018, 9, 6935–6943, 10.1039/C8SC02312C.
- L. E. Jamieson, A. Li, K. Faulds and D. Graham, Ratiometric analysis using Raman spectroscopy as a powerful predictor of structural properties of fatty acids, R. Soc. Open Sci., 2018, 5, 181483, DOI:10.1098/rsos.181483.
- H. J. Butler, L. Ashton, B. Bird, G. Cinque, K. Curtis, J. Dorney, K. Esmonde-White, N. J. Fullwood, B. Gardner, P. L. Martin-Hirsch, M. J. Walsh, M. R. McAinsh, N. Stone and F. L. Martin, Using Raman spectroscopy to characterize biological materials, Nat. Protoc., 2016, 11, 664–687, DOI:10.1038/nprot.2016.036.
- A. Zumbusch, G. R. Holtom and X. S. Xie, Three-dimensional vibrational imaging by Coherent Anti-Stokes Raman scattering, Phys. Rev. Lett., 1999, 82, 4142–4145 CrossRef CAS.
- S. Heuke and H. Rigneault, Coherent Stokes Raman scattering microscopy (CSRS), Nat. Commun., 2023, 14, 3337, DOI:10.1038/s41467-023-38941-4.
- P. D. Maker and R. W. Terhune, Study of optical effects due to an induced polarization third order in the electric field strength, Phys. Rev., 1965, 137, 801–818 CrossRef CAS.
- E. J. Woodbury and W. K. NgRuby laser operation in the near IR, Proceedings of the Institute of Radio Engineers, 1962, 50, 2347–2348.
- C. W. Freudiger, W. Min, B. G. Saar, S. Lu, G. R. Holtom, C. He, J. C. Tsai, J. X. Kang and X. S. Xie, Label-free biomedical imaging with high sensitivity by stimulated Raman scattering microscopy, Science, 2008, 322, 1857–1861, DOI:10.1126/science.1165758.
- E. L. Dunnington, B. S. Wong and D. Fu, Innovative approaches for drug discovery:quantifying drug distribution and response with Raman imaging, Anal. Chem., 2024, 96, 7926–7944, DOI:10.1021/acs.analchem.4c01413.
- C. Zhang and J. X. Cheng, Perspective:Coherent Raman scattering microscopy, the future is bright, APL Photonics, 2018, 3, 090901, DOI:10.1063/1.5040101.
- C. Zong, R. Premasiri, H. N. Lin, Y. M. Huang, C. Zhang, C. Yang, B. Ren, L. D. Ziegler and J. X. Cheng, Plasmon-enhanced stimulated Raman scattering microscopy with single-molecule detection sensitivity, Nat. Commun., 2019, 10, 5318, DOI:10.1038/s41467-019-13230-1.
- L. Wei and W. Min, Electronic Preresonance Stimulated Raman Scattering Microscopy, J. Phys. Chem. Lett., 2018, 9, 4294–4301, DOI:10.1021/acs.jpclett.8b00204.
- H. J. Byrne, P. Knief, M. E. Keating and F. Bonnier, Spectral pre and post processing for infrared and Raman spectroscopy of biological tissues and cells, Chem. Soc. Rev., 2016, 45, 1865–1878, 10.1039/C5CS00440C.
- D. Fu and X. S. Xie, Reliable Cell Segmentation Based on Spectral Phasor Analysis of Hyperspectral Stimulated Raman Scattering Imaging Data, Anal. Chem., 2014, 86, 4115–4119, DOI:10.1021/ac500014b.
- P. Swiatlowska, W. Tipping, E. Marhuenda, P. Severi, V. Fomin, Z. Yang, Q. Xiao, D. Graham, C. Shanahan and T. Iskratsch, Hypertensive pressure mechanosensing alone triggers lipid droplet accumulation and transdifferentiation of vascular smooth muscle cells to foam cells, Adv. Sci., 2024, 11, 2308686, DOI:10.1002/advs.202308686.
- E. W. Hislop, W. J. Tipping, K. Faulds and D. Graham, Label-free cytometric evaluation of mitosis via stimulated Raman scattering microscopy and spectral phasor analysis, Anal. Chem., 2023, 95, 7244–7253, DOI:10.1021/acs.analchem.3c00212.
- W. J. Tipping, L. T. Wilson, C. An, A. A. Leventi, A. W. Wark, C. Wetherill, N. C. O. Tomkinson, K. Faulds and D. Graham, Stimulated Raman scattering microscopy with spectral phasor analysis: applications in assessing drug–cell interactions, Chem. Sci., 2022, 13, 3468–3476, 10.1039/D1SC06976D.
- M. Wei, L. Shi, Y. Shen, Z. Zhao, A. Guzman, L. J. Kaufman, L. Wei and W. Min, Volumetric chemical imaging by clearing-enhanced stimulated Raman scattering microscopy, Proc. Natl. Acad. Sci. U. S. A., 2019, 116, 6608–6617, DOI:10.1073/pnas.1813044116.
- K.-C. Huang, J. Li, C. Zhang, Y. Tan and J.-X. Cheng, Multiplex stimulated Raman scattering imaging cytometry reveals lipid-rich protrusions in cancer cells under stress conditions, iScience, 2020, 23, 100953, DOI:10.1016/j.isci.2020.100953.
- H. J. Braddick, W. J. Tipping, L. T. Wilson, H. S. Jaconelli, E. K. Grant, K. Faulds, D. Graham and N. C. O. Tomkinson, Determination of intracellular esterase activity using ratiometric Raman sensing and spectral phasor analysis, Anal. Chem., 2023, 95, 5369–5376, DOI:10.1021/acs.analchem.2c05708.
- Y. F. Zhu, X. W. Ge, H. L. Ni, J. Z. Yin, H. N. Lin, L. Wang, Y. Y. Tan, C. V. P. Dessai, Y. M. Li, X. Y. Teng and J. X. Cheng, Stimulated Raman photothermal microscopy toward ultrasensitive chemical imaging, Sci. Adv., 2023, 9, eadi2181, DOI:10.1126/sciadv.adi2181.
- B. Wong, X. Zhao, Y. Su, H. Ouyang, T. Rhodes, W. Xu, H. Xi and D. Fu, Characterizing silicone oil-induced protein aggregation with stimulated Raman scattering imaging, Mol. Pharm., 2023, 20, 4268–4276, DOI:10.1021/acs.molpharmaceut.3c00391.
- J. Dorney, F. Bonnier, A. Garcia, A. Casey, G. Chambers and H. J. Byrne, Identifying and localizing intracellular nanoparticles using Raman spectroscopy, Analyst, 2012, 137, 1111–1119, 10.1039/C2AN15977E.
- A. Borek-Dorosz, A. Pieczara, J. Orleanska, K. Brzozowski, W. Tipping, D. Graham, E. Bik, A. Kubrak, M. Baranska and K. Majzner, Raman microscopy reveals how cell inflammation activates glucose and lipid metabolism, Biochim. Biophys. Acta, Mol. Cell Res., 2024, 1871, 119575, DOI:10.1016/j.bbamcr.2023.119575.
- P. Wang, B. Liu, D. Zhang, M. Y. Belew, H. A. Tissenbaum and J.-X. Cheng, Imaging lipid metabolism in live Caenorhabditis elegans using fingerprint vibrations, Angew. Chem., Int. Ed., 2014, 53, 11787–11792, DOI:10.1002/anie.201406029.
- Y. Y. Tan, H. N. Lin and J. X. Cheng, Profiling single cancer cell metabolism via high-content SRS imaging with chemical sparsity, Sci. Adv., 2023, 9, eadg6061, DOI:10.1126/sciadv.adg6061.
- H. Lin, H. J. Lee, N. Tague, J. B. Lugagne, C. Zong, F. Deng, J. Shin, L. Tian, W. Wong, M. J. Dunlop and J. X. Cheng, Microsecond fingerprint stimulated Raman spectroscopic imaging by ultrafast tuning and spatial-spectral learning, Nat. Commun., 2021, 12, 3052, DOI:10.1038/s41467-021-23202-z.
- L. Wei, Z. Chen, L. Shi, R. Long, A. V. Anzalone, L. Zhang, F. Hu, R. Yuste, V. W. Cornish and W. Min, Super-multiplex vibrational imaging, Nature, 2017, 544, 465, DOI:10.1038/nature22051.
- F. Hu, C. Zeng, R. Long, Y. Miao, L. Wei, Q. Xu and W. Min, Supermultiplexed optical imaging and barcoding with engineered polyynes, Nat. Methods, 2018, 15, 194, DOI:10.1038/nmeth.4578.
- S. Benson, F. de Moliner, W. Tipping and M. Vendrell, Miniaturized chemical tags for optical imaging, Angew. Chem., Int. Ed., 2022, 61, e202204788, DOI:10.1002/anie.202204788.
- H. Fujioka, J. Shou, R. Kojima, Y. Urano, Y. Ozeki and M. Kamiya, Multicolor activatable raman probes for simultaneous detection of plural enzyme activities, J. Am. Chem. Soc., 2020, 142, 20701–20707, DOI:10.1021/jacs.0c09200.
- P. M. Titchenell, M. A. Lazar and M. J. Birnbaum, Unraveling the regulation of hepatic metabolism by insulin, Trends Endocrinol. Metab., 2017, 28, 497–505, DOI:10.1016/j.tem.2017.03.003.
- T. Chen, A. Yavuz and M. C. Wang, Dissecting lipid droplet biology with coherent Raman scattering microscopy, J. Cell Sci., 2022, 135, jcs252353, DOI:10.1242/jcs.252353.
- S. Egoshi, K. Dodo and M. Sodeoka, Deuterium Raman imaging for lipid analysis, Curr. Opin. Chem. Biol., 2022, 70, 102181, DOI:10.1016/j.cbpa.2022.102181.
- J. Langer, D. Jimenez de Aberasturi, J. Aizpurua, R. A. Alvarez-Puebla, B. Auguie, J. J. Baumberg, G. C. Bazan, S. E. J. Bell, A. Boisen, A. G. Brolo, J. Choo, D. Cialla-May, V. Deckert, L. Fabris, K. Faulds, F. J. Garcia de Abajo, R. Goodacre, D. Graham, A. J. Haes, C. L. Haynes, C. Huck, T. Itoh, M. Kall, J. Kneipp, N. A. Kotov, H. Kuang, E. C. Le Ru, H. K. Lee, J. F. Li, X. Y. Ling, S. A. Maier, T. Mayerhofer, M. Moskovits, K. Murakoshi, J. M. Nam, S. Nie, Y. Ozaki, I. Pastoriza-Santos, J. Perez-Juste, J. Popp, A. Pucci, S. Reich, B. Ren, G. C. Schatz, T. Shegai, S. Schlucker, L. L. Tay, K. G. Thomas, Z. Q. Tian, R. P. Van Duyne, T. Vo-Dinh, Y. Wang, K. A. Willets, C. Xu, H. Xu, Y. Xu, Y. S. Yamamoto, B. Zhao and L. M. Liz-Marzan, Present and future of surface-enhanced Raman scattering, ACS Nano, 2020, 14, 28–117, DOI:10.1021/acsnano.9b04224.
- S. W. Li, Y. P. Li, R. X. Yi, L. W. Liu and J. L. Qu, Coherent anti-Stokes Raman scattering microscopy and Its applications, Front. Phys., 2020, 8, 598420, DOI:10.3389/fphy.2020.598420.
- M. A. Ferrara, R. Ranjan and L. Sirleto, Adipocyte differentiation investigated by stimulated Raman microscopy based on femtosecond laser sources, 2020 22nd International Conference on Transparent Optical Networks (Icton 2020), 2020. DOI:10.1109/icton51198.2020.9203562.
- Z. Yang Loureiro, J. Solivan-Rivera and S. Corvera, Adipocyte heterogeneity underlying adipose tissue functions, Endocrinology, 2022, 163, bqab138, DOI:10.1210/endocr/bqab138.
- K. G. Stenkula and C. Erlanson-Albertsson, Adipose cell size: importance in health and disease, Am. J. Physiol. Regul. Integr. Comp. Physiol., 2018, 315, R284–R295, DOI:10.1152/ajpregu.00257.2017.
- J. Moser, M. Emous, P. Heeringa and I. A. Rodenhuis-Zybert, Mechanisms and pathophysiology of SARS-CoV-2 infection of the adipose tissue, Trends Endocrinol. Metab., 2023, 34, 735–748, DOI:10.1016/j.tem.2023.08.010.
- G. Donjuán-Loredo, R. Espinosa-Tanguma, F. León-Bejarano, J. A. Ramírez-Elías, R. Salgado-Delgado, F. J. González, E. Guevara and M. G. Ramírez-Elías, Raman spectroscopy for adipose tissue assessment in rat models of obesity and type 1diabetes, Appl. Spectrosc., 2021, 75, 1189–1197, DOI:10.1177/0003702821990357.
- J. Tratwal, G. Falgayrac, A. During, N. Bertheaume, C. Bataclan, D. N. Tavakol, V. Campos, L. Duponchel, G. Q. Daley, G. Penel, C. Chauveau and O. Naveiras, Raman microspectroscopy reveals unsaturation heterogeneity at the lipid droplet level and validates a model of bone marrow adipocyte subtypes, Front. Endocrinol., 2022, 13, 1001210, DOI:10.3389/fendo.2022.1001210.
- T. H. Ambrosi, A. Scialdone, A. Graja, S. Gohlke, A. M. Jank, C. Bocian, L. Woelk, H. Fan, D. W. Logan, A. Schurmann, L. R. Saraiva and T. J. Schulz, Adipocyte accumulation in the bone marrow during obesity and aging impairs stem cell-based hematopoietic and bone regeneration, Cell Stem Cell, 2017, 20, 771–784, DOI:10.1016/j.stem.2017.02.009.
- K. J. Suchacki, A. A. S. Tavares, D. Mattiucci, E. L. Scheller, G. Papanastasiou, C. Gray, M. C. Sinton, L. E. Ramage, W. A. McDougald, A. Lovdel, R. J. Sulston, B. J. Thomas, B. M. Nicholson, A. J. Drake, C. J. Alcaide-Corral, D. Said, A. Poloni, S. Cinti, G. J. Macpherson, M. R. Dweck, J. P. M. Andrews, M. C. Williams, R. J. Wallace, E. J. R. van Beek, O. A. MacDougald, N. M. Morton, R. H. Stimson and W. P. Cawthorn, Bone marrow adipose tissue is a unique adipose subtype with distinct roles in glucose homeostasis, Nat. Commun., 2020, 11, 3097, DOI:10.1038/s41467-020-16878-2.
- H. B. Castillo, S. O. Shuster, L. H. Tarekegn and C. M. Davis, Oleic acid differentially affects lipid droplet storage of synthesized lipids in hepatocytes and adipocytes, Chem. Commun., 2024, 60, 3138–3141, 10.1039/d3cc04829b.
- A. Paul, B. Chanclon, C. Brannmark, P. Wittung-Stafshede, C. S. Olofsson, I. W. Asterholm and S. H. Parekh, Comparing lipid remodeling of brown adipose tissue, white adipose tissue, and liver after one-week high fat diet intervention with quantitative Raman microscopy, J. Cell. Biochem., 2023, 124, 382–395, DOI:10.1002/jcb.30372.
- E. Stanek, M. Z. Pacia, A. Kaczor and K. Czamara, The distinct phenotype of primary adipocytes and adipocytes derived from stem cells of white adipose tissue as assessed by Raman and fluorescence imaging, Cell. Mol. Life Sci., 2022, 79, 383, DOI:10.1007/s00018-022-04391-2.
- M. De Martino, J. C. Rathmell, L. Galluzzi and C. Vanpouille-Box, Cancer cell metabolism and antitumour immunity, Nat. Rev. Immunol., 2024, 24, 654–669, DOI:10.1038/s41577-024-01026-4.
- J. R. Cantor and D. M. Sabatini, Cancer cell metabolism:one hallmark, many faces, Cancer Discovery, 2012, 2, 881–898, DOI:10.1158/2159-8290.CD-12-0345.
- G. Ouverney, D. Hottz and B. K. Robbs, Drug resistance and novel targets for cancer therapy:An overview of recent findings, Biomedicines, 2024, 24, 537, DOI:10.3390/biomedicines12040816.
- G. Housman, S. Byler, S. Heerboth, K. Lapinska, M. Longacre, N. Snyder and S. Sarkar, Drug resistance in cancer:an overview, Cancers, 2014, 6, 1769–1792, DOI:10.3390/cancers6031769.
- Y. Luo, H. Wang, B. Liu and J. Wei, Fatty acid metabolism and cancer immunotherapy, Curr. Oncol. Rep., 2022, 24, 659–670, DOI:10.1007/s11912-022-01223-1.
- H. Xu, Y. Chen, M. Gu, C. Liu, Q. Chen, M. Zhan and Z. Wang, Fatty acid metabolism reprogramming in advanced prostate cancer, Metabolites, 2021, 11, 765, DOI:10.3390/metabo11110765.
- S. C. Stadler and R. Burkhardt, Fatty acid metabolism in cancer cells - the power of plasticity, Curr. Opin. Lipidol., 2021, 32, 387–388, DOI:10.1097/MOL.0000000000000788.
- K. D. G. Saunders, H. M. Lewis, D. J. Beste, O. Cexus and M. J. Bailey, Spatial single cell metabolomics:Current challenges and future developments, Curr. Opin. Chem. Biol., 2023, 75, 102327, DOI:10.1016/j.cbpa.2023.102327.
- K. D. G. Saunders, J. von Gerichten, H. M. Lewis, P. Gupta, M. Spick, C. Costa, E. Velliou and M. J. Bailey, Single-cell lipidomics using analytical flow LC-MS characterizes the response to chemotherapy in cultured Pancreatic cancer cells, Anal. Chem., 2023, 95, 14727–14735, DOI:10.1021/acs.analchem.3c02854.
- H. Davies, G. R. Bignell, C. Cox, P. Stephens, S. Edkins, S. Clegg, J. Teague, H. Woffendin, M. J. Garnett, W. Bottomley, N. Davis, E. Dicks, R. Ewing, Y. Floyd, K. Gray, S. Hall, R. Hawes, J. Hughes, V. Kosmidou, A. Menzies, C. Mould, A. Parker, C. Stevens, S. Watt, S. Hooper, R. Wilson, H. Jayatilake, B. A. Gusterson, C. Cooper, J. Shipley, D. Hargrave, K. Pritchard-Jones, N. Maitland, G. Chenevix-Trench, G. J. Riggins, D. D. Bigner, G. Palmieri, A. Cossu, A. Flanagan, A. Nicholson, J. W. Ho, S. Y. Leung, S. T. Yuen, B. L. Weber, H. F. Seigler, T. L. Darrow, H. Paterson, R. Marais, C. J. Marshall, R. Wooster, M. R. Stratton and P. A. Futreal, Mutations of the BRAF gene in human cancer, Nature, 2002, 417, 949–954, DOI:10.1038/nature00766.
- M. F. Berger, J. Z. Levin, K. Vijayendran, A. Sivachenko, X. Adiconis, J. Maguire, L. A. Johnson, J. Robinson, R. G. Verhaak, C. Sougnez, R. C. Onofrio, L. Ziaugra, K. Cibulskis, E. Laine, J. Barretina, W. Winckler, D. E. Fisher, G. Getz, M. Meyerson, D. B. Jaffe, S. B. Gabriel, E. S. Lander, R. Dummer, A. Gnirke, C. Nusbaum and L. A. Garraway, Integrative analysis of the melanoma transcriptome, Genome Res., 2010, 20, 413–427, DOI:10.1101/gr.103697.109.
- Q. Qian and R. Niwa, Endocrine regulation of aging in the fruit fly Drosophila melanogaster, Zool. Sci., 2024, 41, 4–13, DOI:10.2108/zs230056.
- D. Clancy, S. Chtarbanova and S. Broughton, Editorial: Model organisms in aging research: Drosophila melanogaster, Front. Aging, 2022, 3, 1118299, DOI:10.3389/fragi.2022.1118299.
- R. Yamamoto, M. Palmer, H. Koski, N. Curtis-Joseph and M. Tatar, Aging modulated by the Drosophila insulin receptor through distinct structure-defined mechanisms, Genetics, 2021, 217, iyaa037, DOI:10.1093/genetics/iyaa037.
- A. A. Johnson and A. Stolzing, The role of lipid metabolism in aging, lifespan regulation, and age-related disease, Aging Cell, 2019, 18, e13048, DOI:10.1111/acel.13048.
- A. S. Mutlu, J. Duffy and M. C. Wang, Lipid metabolism and lipid signals in aging and longevity, Dev. Cell, 2021, 56, 1394–1407, DOI:10.1016/j.devcel.2021.03.034.
- M. Zhang, P. T. Dong, H. E. Eldesouky, Y. W. Zhan, H. A. Lin, Z. Wang, E. A. Salama, S. Jusuf, C. Zong, Z. C. Chen, M. N. Seleem and J. X. Cheng, Fingerprint Stimulated Raman Scattering Imaging Unveils Ergosteryl Ester as a Metabolic Signature of Azole-Resistant Candida albicans, Anal. Chem., 2023, 95, 9901–9913, DOI:10.1021/acs.analchem.3c00900.
- Y. J. Li, W. X. Zhang, A. A. Fung and L. Y. Shi, DO-SRS imaging of metabolic dynamics in aging, Analyst, 2021, 146, 7510–7519, 10.1039/d1an01638e.
- Y. Li, W. Zhang, A. A. Fung and L. Shi, DO-SRS imaging of diet regulated metabolic activities in Drosophila during aging processes, Aging Cell, 2022, 21, e13586, DOI:10.1111/acel.13586.
- Y. Li, W. Zhang, A. A. Fung and L. Shi, DO-SRS imaging of metabolic dynamics in aging Drosophila, Analyst, 2021, 146, 7510–7519, 10.1039/d1an01638e.
- Y. J. Li, W. X. Zhang, A. A. Fung and L. Y. Shi, DO-SRS imaging of diet regulated metabolic activities in Drosophila during aging processes, Aging Cell, 2022, 21, e13586, DOI:10.1111/acel.13586.
- Y. J. Li, P. L. Chang, S. Sankaran, H. Jang, Y. H. Nie, A. D. Zeng, S. Hussain, J. Y. Wu, X. Chen and L. Y. Shi, Bioorthogonal Stimulated Raman Scattering Imaging Uncovers Lipid Metabolic Dynamics in Brain During Aging, GEN Biotechnol., 2023, 2, 247–261, DOI:10.1089/genbio.2023.0017.
- S. J. Spratt, T. Mizuguchi, H. Akaboshi, H. Kosakamoto, R. Okada, F. Obata and Y. Ozeki, Imaging the uptake of deuterated methionine in with stimulated Raman scattering, Front. Chem., 2023, 11, 1141920, DOI:10.3389/fchem.2023.1141920.
- M. C. Fisher, A. Alastruey-Izquierdo, J. Berman, T. Bicanic, E. M. Bignell, P. Bowyer, M. Bromley, R. Bruggemann, G. Garber, O. A. Cornely, S. J. Gurr, T. S. Harrison, E. Kuijper, J. Rhodes, D. C. Sheppard, A. Warris, P. L. White, J. Xu, B. Zwaan and P. E. Verweij, Tackling the emerging threat of antifungal resistance to human health, Nat. Rev. Microbiol., 2022, 20, 557–571, DOI:10.1038/s41579-022-00720-1.
- L. E. Cowen, D. Sanglard, S. J. Howard, P. D. Rogers and D. S. Perlin, Mechanisms of antifungal drug resistance, Cold Spring Harbor Perspect. Med., 2014, 5, a019752, DOI:10.1101/cshperspect.a019752.
- M. Bibi, S. Murphy, R. I. Benhamou, A. Rosenberg, A. Ulman, T. Bicanic, M. Fridman and J. Berman, Combining colistin and fluconazole synergistically increases fungal membrane permeability and antifungal cidality, ACS Infect. Dis., 2021, 7, 377–389, DOI:10.1021/acsinfecdis.0c00721.
- C. J. Nobile and A. D. Johnson, Candida albicans biofilms and human disease, Annu. Rev. Microbiol., 2015, 69, 71–92, DOI:10.1146/annurev-micro-091014-104330.
- N. Tague, H. N. Lin, J. B. Lugagne, O. M. O’Connor, D. Burman, W. W. Wong, J. X. Cheng and M. J. Dunlop, Longitudinal single-cell imaging of engineered strains with stimulated Raman scattering to characterize heterogeneity in fatty acid production, Adv. Sci., 2023, 10, 2206519, DOI:10.1002/advs.202206519.
- Z. L. Huang, S. Yan, Y. R. Li, W. Ju and P. Wang, Direct
counting and imaging chain lengths of lipids by stimulated Raman scattering microscopy, Anal. Chem., 2023, 95, 5815–5819, DOI:10.1021/acs.analchem.3c00291.
- M. Nagtegaal, P. Koken, T. Amthor and M. Doneva, Fast multi-component analysis using a joint sparsity constraint for MR fingerprinting, Magn. Reson. Med., 2020, 83, 521–534, DOI:10.1002/mrm.27947.
- M. Nagtegaal, P. Koken, T. Amthor, J. de Bresser, B. Madler, F. Vos and M. Doneva, Myelin water imaging from multi-echo T(2) MR relaxometry data using a joint sparsity constraint, Neuroimage, 2020, 219, 117014, DOI:10.1016/j.neuroimage.2020.117014.
- H. Wang, S. Han and M. I. Kolobov, Quantum limits of super-resolution of optical sparse objects via sparsity constraint, Opt. Express, 2012, 20, 23235–23252, DOI:10.1364/OE.20.023235.
- H. Jang, Y. J. Li, A. A. Fung, P. Bagheri, K. Hoang, D. Skowronska-Krawczyk, X. P. Chen, J. Y. Wu, B. Bintu and L. Y. Shi, Super-resolution SRS microscopy with A-PoD, Nat. Methods, 2023, 20, 448–458, DOI:10.1038/s41592-023-01779-1.
- S. Martinez, M. Toscani and O. E. Martinez, Superresolution method for a single wide-field image deconvolution by superposition of point sources, J. Microsc., 2019, 275, 51–65, DOI:10.1111/jmi.12802.
- F. H. Westheimer, The magnitude of the primary kinetic isotope effect for compounds of hydrogen and deuterium, Chem. Rev., 1961, 61, 265–273, DOI:10.1021/cr60211a004.
- K. Dodo, A. Sato, Y. Tamura, S. Egoshi, K. Fujiwara, K. Oonuma, S. Nakao, N. Terayama and M. Sodeoka, Synthesis of deuterated γ-linolenic acid and application for biological studies:metabolic tuning and Raman imaging, Chem. Commun., 2021, 57, 2180–2183, 10.1039/d0cc07824g.
- M. Uematsu, Y. Kita, T. Shimizu and H. Shindou, Multiplex fatty acid imaging inside cells by Raman microscopy, FASEB J., 2020, 34, 10357–10372, DOI:10.1096/fj.202000514R.
- N. Murphy, W. J. Tipping, H. J. Braddick, L. T. Wilson, N. C. O. Tomkinson, K. Faulds, D. Graham and P. Farràs, Expanding the Range of Bioorthogonal Tags for Multiplex Stimulated Raman Scattering Microscopy, Angew. Chem., Int. Ed., 2023, 62, e202311530, DOI:10.1002/anie.202311530.
- B. Riggs, W. Rothwell, S. Mische, G. R. Hickson, J. Matheson, T. S. Hays, G. W. Gould and W. Sullivan, Actin cytoskeleton remodeling during early Drosophila furrow formation requires recycling endosomal components Nuclear-fallout and Rab11, J. Cell Biol., 2003, 163, 143–154, DOI:10.1083/jcb.200305115.
- C. E. Buckley, R. E. Moore, A. Reade, A. R. Goldberg, O. D. Weiner and J. D. W. Clarke, Reversible Optogenetic Control of Subcellular Protein Localization in a Live Vertebrate Embryo, Dev. Cell, 2016, 36, 117–126, DOI:10.1016/j.devcel.2015.12.011.
- I. Y. Benador, M. Veliova, M. Liesa and O. S. Shirihai, Mitochondria Bound to Lipid Droplets:Where Mitochondrial Dynamics Regulate Lipid Storage and Utilization, Cell Metab., 2019, 29, 827–835, DOI:10.1016/j.cmet.2019.02.011.
- N. K. Talari, U. Mattam, N. K. Meher, A. K. Paripati, K. Mahadev, T. Krishnamoorthy and N. B. Sepuri, Lipid-droplet associated mitochondria promote fatty-acid oxidation through a distinct bioenergetic pattern in male Wistar rats, Nat. Commun., 2023, 14, 766, DOI:10.1038/s41467-023-36432-0.
- W. J. Tipping, A. S. Merchant, R. Fearon, N. C. O. Tomkinson, K. Faulds and D. Graham, Temporal imaging of drug dynamics in live cells using stimulated Raman scattering microscopy and a perfusion cell culture system, RSC Chem. Biol., 2022, 3, 1154–1164, 10.1039/d2cb00160h.
- R. S. Chadha, J. A. Guerrero, L. Wei and L. M. Sanchez, Seeing is Believing:Developing Multimodal Metabolic Insights at the Molecular Level, ACS Cent. Sci., 2024, 10, 758–774, DOI:10.1021/acscentsci.3c01438.
Footnotes |
† These authors contributed equally. |
‡ We apologise to our colleagues whose work has not been cited for reasons for brevity. |
|
This journal is © The Royal Society of Chemistry 2024 |